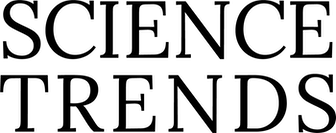
Home » experimental control important
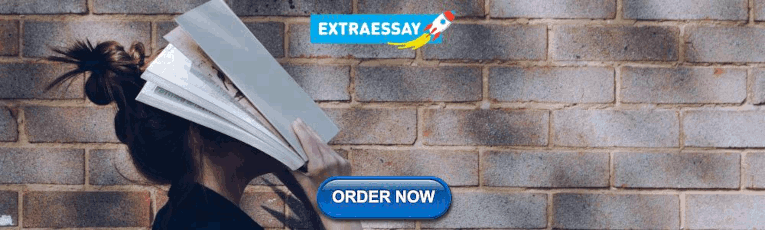
What An Experimental Control Is And Why It’s So Important
Daniel Nelson
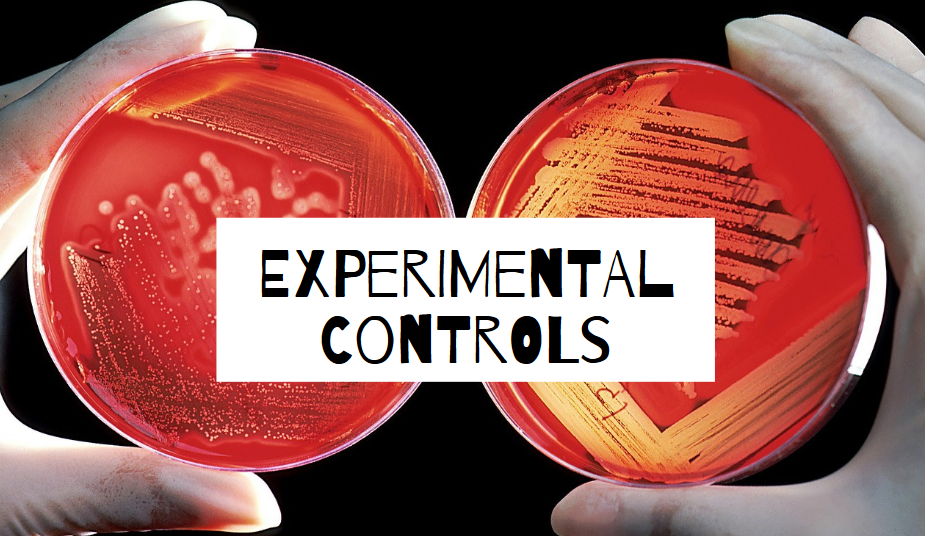
An experimental control is used in scientific experiments to minimize the effect of variables which are not the interest of the study. The control can be an object, population, or any other variable which a scientist would like to “control.”
You may have heard of experimental control, but what is it? Why is an experimental control important? The function of an experimental control is to hold constant the variables that an experimenter isn’t interested in measuring.
This helps scientists ensure that there have been no deviations in the environment of the experiment that could end up influencing the outcome of the experiment, besides the variable they are investigating. Let’s take a closer look at what this means.
You may have ended up here to understand why a control is important in an experiment. A control is important for an experiment because it allows the experiment to minimize the changes in all other variables except the one being tested.
To start with, it is important to define some terminology.
Terminology Of A Scientific Experiment
Negative | The negative control variable is a variable or group where no response is expected |
Positive | A positive control is a group or variable that receives a treatment with a known positive result |
Randomization | A randomized controlled seeks to reduce bias when testing a new treatment |
Blind experiments | In blind experiments, the variable or group does not know the full amount of information about the trial to not skew results |
Double-blind experiments | A double-blind group is where all parties do not know which individual is receiving the experimental treatment |
Randomization is important as it allows for more non-biased results in experiments. Random numbers generators are often used both in scientific studies as well as on 지노 사이트 to make outcomes fairer.
Scientists use the scientific method to ask questions and come to conclusions about the nature of the world. After making an observation about some sort of phenomena they would like to investigate, a scientist asks what the cause of that phenomena could be. The scientist creates a hypothesis, a proposed explanation that answers the question they asked. A hypothesis doesn’t need to be correct, it just has to be testable.
The hypothesis is a prediction about what will happen during the experiment, and if the hypothesis is correct then the results of the experiment should align with the scientist’s prediction. If the results of the experiment do not align with the hypothesis, then a good scientist will take this data into consideration and form a new hypothesis that can better explain the phenomenon in question.
Independent and Dependent Variables
In order to form an effective hypothesis and do meaningful research, the researcher must define the experiment’s independent and dependent variables . The independent variable is the variable which the experimenter either manipulates or controls in an experiment to test the effects of this manipulation on the dependent variable. A dependent variable is a variable being measured to see if the manipulation has any effect.
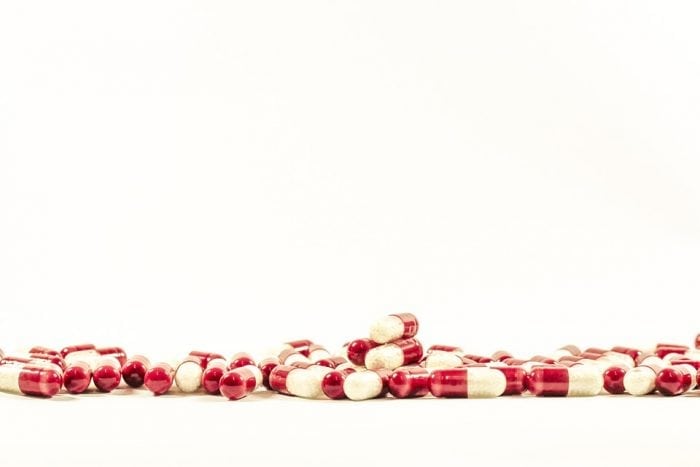
Photo: frolicsomepl via Pixabay, CC0
For instance, if a researcher wanted to see how temperature impacts the behavior of a certain gas, the temperature they adjust would be the independent variable and the behavior of the gas the dependent variable.
Control Groups and Experimental Groups
There will frequently be two groups under observation in an experiment, the experimental group, and the control group . The control group is used to establish a baseline that the behavior of the experimental group can be compared to. If two groups of people were receiving an experimental treatment for a medical condition, one would be given the actual treatment (the experimental group) and one would typically be given a placebo or sugar pill (the control group).
Without an experimental control group, it is difficult to determine the effects of the independent variable on the dependent variable in an experiment. This is because there can always be outside factors that are influencing the behavior of the experimental group. The function of a control group is to act as a point of comparison, by attempting to ensure that the variable under examination (the impact of the medicine) is the thing responsible for creating the results of an experiment. The control group is holding other possible variables constant, such as the act of seeing a doctor and taking a pill, so only the medicine itself is being tested.
Why Are Experimental Controls So Important?
Experimental controls allow scientists to eliminate varying amounts of uncertainty in their experiments. Whenever a researcher does an experiment and wants to ensure that only the variable they are interested in changing is changing, they need to utilize experimental controls.
Experimental controls have been dubbed “controls” precisely because they allow researchers to control the variables they think might have an impact on the results of the study. If a researcher believes that some outside variables could influence the results of their research, they’ll use a control group to try and hold that thing constant and measure any possible influence it has on the results. It is important to note that there may be many different controls for an experiment, and the more complex a phenomenon under investigation is, the more controls it is likely to have.
Not only do controls establish a baseline that the results of an experiment can be compared to, they also allow researchers to correct for possible errors. If something goes wrong in the experiment, a scientist can check on the controls of the experiment to see if the error had to do with the controls. If so, they can correct this next time the experiment is done.
A Practical Example
Let’s take a look at a concrete example of experimental control. If an experimenter wanted to determine how different soil types impacted the germination period of seeds , they could set up four different pots. Each pot would be filled with a different soil type, planted with seeds, then watered and exposed to sunlight. Measurements would be taken regarding how long it took for the seeds to sprout in the different soil types.
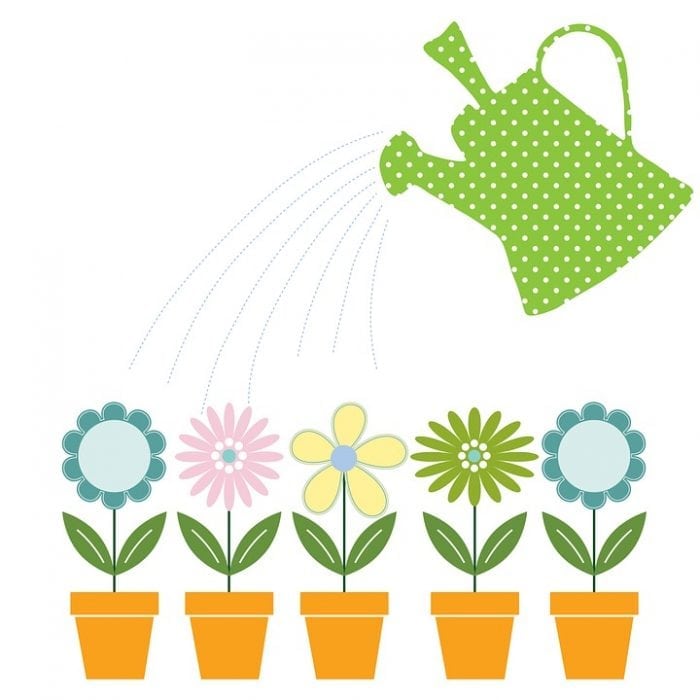
Photo: Kaz via Pixabay, CC0
A control for this experiment might be to fill more pots with just the different types of soil and no seeds or to set aside some seeds in a pot with no soil. The goal is to try and determine that it isn’t something else other than the soil, like the nature of the seeds themselves, the amount of sun they were exposed to, or how much water they are given, that affected how quickly the seeds sprouted. The more variables a researcher controlled for, the surer they could be that it was the type of soil having an impact on the germination period.
Not All Experiments Are Controlled
“It doesn’t matter how beautiful your theory is, it doesn’t matter how smart you are. If it doesn’t agree with experiment, it’s wrong.” — Richard P. Feynman
While experimental controls are important , it is also important to remember that not all experiments are controlled. In the real world, there are going to be limitations on what variables a researcher can control for, and scientists often try to record as much data as they can during an experiment so they can compare factors and variables with one another to see if any variables they didn’t control for might have influenced the outcome. It’s still possible to draw useful data from experiments that don’t have controls, but it is much more difficult to draw meaningful conclusions based on uncontrolled data.
Though it is often impossible in the real world to control for every possible variable, experimental controls are an invaluable part of the scientific process and the more controls an experiment has the better off it is.
← Previous post
Next post →
Related Posts
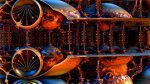

An official website of the United States government
The .gov means it’s official. Federal government websites often end in .gov or .mil. Before sharing sensitive information, make sure you’re on a federal government site.
The site is secure. The https:// ensures that you are connecting to the official website and that any information you provide is encrypted and transmitted securely.
- Publications
- Account settings
Preview improvements coming to the PMC website in October 2024. Learn More or Try it out now .
- Advanced Search
- Journal List
- v.20(10); 2019 Oct 4

Why control an experiment?
John s torday.
1 Department of Pediatrics, Harbor‐UCLA Medical Center, Torrance, CA, USA
František Baluška
2 IZMB, University of Bonn, Bonn, Germany
Empirical research is based on observation and experimentation. Yet, experimental controls are essential for overcoming our sensory limits and generating reliable, unbiased and objective results.

We made a deliberate decision to become scientists and not philosophers, because science offers the opportunity to test ideas using the scientific method. And once we began our formal training as scientists, the greatest challenge beyond formulating a testable or refutable hypothesis was designing appropriate controls for an experiment. In theory, this seems trivial, but in practice, it is often difficult. But where and when did this concept of controlling an experiment start? It is largely attributed to Roger Bacon, who emphasized the use of artificial experiments to provide additional evidence for observations in his Novum Organum Scientiarum in 1620. Other philosophers took up the concept of empirical research: in 1877, Charles Peirce redefined the scientific method in The Fixation of Belief as the most efficient and reliable way to prove a hypothesis. In the 1930s, Karl Popper emphasized the necessity of refuting hypotheses in The Logic of Scientific Discoveries . While these influential works do not explicitly discuss controls as an integral part of experiments, their importance for generating solid and reliable results is nonetheless implicit.
… once we began our formal training as scientists, the greatest challenge beyond formulating a testable or refutable hypothesis was designing appropriate controls for an experiment.
But the scientific method based on experimentation and observation has come under criticism of late in light of the ever more complex problems faced in physics and biology. Chris Anderson, the editor of Wired Magazine, proposed that we should turn to statistical analysis, machine learning, and pattern recognition instead of creating and testing hypotheses, based on the Informatics credo that if you cannot answer the question, you need more data. However, this attitude subsumes that we already have enough data and that we just cannot make sense of it. This assumption is in direct conflict with David Bohm's thesis that there are two “Orders”, the Explicate and Implicate 1 . The Explicate Order is the way in which our subjective sensory systems perceive the world 2 . In contrast, Bohm's Implicate Order would represent the objective reality beyond our perception. This view—that we have only a subjective understanding of reality—dates back to Galileo Galilei who, in 1623, criticized the Aristotelian concept of absolute and objective qualities of our sensory perceptions 3 and to Plato's cave allegory that reality is only what our senses allow us to see.
The only way for systematically overcoming the limits of our sensory apparatus and to get a glimpse of the Implicate Order is through the scientific method, through hypothesis‐testing, controlled experimentation. Beyond the methodology, controlling an experiment is critically important to ensure that the observed results are not just random events; they help scientists to distinguish between the “signal” and the background “noise” that are inherent in natural and living systems. For example, the detection method for the recent discovery of gravitational waves used four‐dimensional reference points to factor out the background noise of the Cosmos. Controls also help to account for errors and variability in the experimental setup and measuring tools: The negative control of an enzyme assay, for instance, tests for any unrelated background signals from the assay or measurement. In short, controls are essential for the unbiased, objective observation and measurement of the dependent variable in response to the experimental setup.
The only way for systematically overcoming the limits of our sensory apparatus […] is through the Scientific Method, through hypothesis‐testing, controlled experimentation.
Nominally, both positive and negative controls are material and procedural; that is, they control for variability of the experimental materials and the procedure itself. But beyond the practical issues to avoid procedural and material artifacts, there is an underlying philosophical question. The need for experimental controls is a subliminal recognition of the relative and subjective nature of the Explicate Order. It requires controls as “reference points” in order to transcend it, and to approximate the Implicate Order.
This is similar to Peter Rowlands’ 4 dictum that everything in the Universe adds up to zero, the universal attractor in mathematics. Prior to the introduction of zero, mathematics lacked an absolute reference point similar to a negative or positive control in an experiment. The same is true of biology, where the cell is the reference point owing to its negative entropy: It appears as an attractor for the energy of its environment. Hence, there is a need for careful controls in biology: The homeostatic balance that is inherent to life varies during the course of an experiment and therefore must be precisely controlled to distinguish noise from signal and approximate the Implicate Order of life.
P < 0.05 tacitly acknowledges the explicate order
Another example of the “subjectivity” of our perception is the level of accuracy we accept for differences between groups. For example, when we use statistical methods to determine if an observed difference between control and experimental groups is a random occurrence or a specific effect, we conventionally consider a p value of less than or equal to 5% as statistically significant; that is, there is a less than 0.05 probability that the effect is random. The efficacy of this arbitrary convention has been debated for decades; suffice to say that despite questioning the validity of that convention, a P value of < 0.05 reflects our acceptance of the subjectivity of our perception of reality.
… controls are essential for the unbiased, objective observation and measurement of the dependent variable in response to the experimental setup.
Thus, if we do away with hypothesis‐testing science in favor of informatics based on data and statistics—referring to Anderson's suggestion—it reflects our acceptance of the noise in the system. However, mere data analysis without any underlying hypothesis is tantamount to “garbage in‐garbage out”, in contrast to well‐controlled imaginative experiments to separate the wheat from the chaff. Albert Einstein was quoted as saying that imagination was more important than knowledge.
The ultimate purpose of the scientific method is to understand ourselves and our place in Nature. Conventionally, we subscribe to the Anthropic Principle, that we are “in” this Universe, whereas the Endosymbiosis Theory, advocated by Lynn Margulis, stipulates that we are “of” this Universe as a result of the assimilation of the physical environment. According to this theory, the organism endogenizes external factors to make them physiologically “useful”, such as iron as the core of the hemoglobin molecule, or ancient bacteria as mitochondria.
… there is a fundamental difference between knowing via believing and knowing based on empirical research.
By applying the developmental mechanism of cell–cell communication to phylogeny, we have revealed the interrelationships between cells and explained evolution from its origin as the unicellular state to multicellularity via cell–cell communication. The ultimate outcome of this research is that consciousness is the product of cellular processes and cell–cell communication in order to react to the environment and better anticipate future events 5 , 6 . Consciousness is an essential prerequisite for transcending the Explicate Order toward the Implicate Order via cellular sensory and cognitive systems that feed an ever‐expanding organismal knowledge about both the environment and itself.
It is here where the empirical approach to understanding nature comes in with its emphasis that knowledge comes only from sensual experience rather than innate ideas or traditions. In the context of the cell or higher systems, knowledge about the environment can only be gained by sensing and analyzing the environment. Empiricism is similar to an equation in which the variables and terms form a product, or a chemical reaction, or a biological process where the substrates, aka sensory data, form products, that is, knowledge. However, it requires another step—imagination, according to Albert Einstein—to transcend the Explicate Order in order to gain insight into the Implicate Order. Take for instance, Dmitri Ivanovich Mendeleev's Periodic Table of Elements: his brilliant insight was not just to use Atomic Number to organize it, but also to consider the chemical reactivities of the Elements by sorting them into columns. By introducing chemical reactivity to the Periodic Table, Mendeleev provided something like the “fourth wall” in Drama, which gives the audience an omniscient, god‐like perspective on what is happening on stage.
The capacity to transcend the subjective Explicate Order to approximate the objective Implicate Order is not unlike Eastern philosophies like Buddhism or Taoism, which were practiced long before the scientific method. An Indian philosopher once pointed out that the Hindus have known for 30,000 years that the Earth revolves around the sun, while the Europeans only realized this a few hundred years ago based on the work of Copernicus, Brahe, and Galileo. However, there is a fundamental difference between knowing via believing and knowing based on empirical research. A similar example is Aristotle's refusal to test whether a large stone would fall faster than a small one, as he knew the answer already 7 . Galileo eventually performed the experiment from the Leaning Tower in Pisa to demonstrate that the fall time of two objects is independent of their mass—which disproved Aristotle's theory of gravity that stipulated that objects fall at a speed proportional to their mass. Again, it demonstrates the power of empiricism and experimentation as formulated by Francis Bacon, John Locke, and others, over intuition and rationalizing.
Even if our scientific instruments provide us with objective data, we still need to apply our consciousness to evaluate and interpret such data.
Following the evolution from the unicellular state to multicellular organisms—and reverse‐engineering it to a minimal‐cell state—reveals that biologic diversity is an artifact of the Explicate Order. Indeed, the unicell seems to be the primary level of selection in the Implicate Order, as it remains proximate to the First Principles of Physiology, namely negative entropy (negentropy), chemiosmosis, and homeostasis. The first two principles are necessary for growth and proliferation, whereas the last reflects Newton's Third Law of Motion that every action has an equal and opposite reaction so as to maintain homeostasis.
All organisms interact with their surroundings and assimilate their experience as epigenetic marks. Such marks extend to the DNA of germ cells and thus change the phenotypic expression of the offspring. The offspring, in turn, interacts with the environment in response to such epigenetic modifications, giving rise to the concept of the phenotype as an agent that actively and purposefully interacts with its environment in order to adapt and survive. This concept of phenotype based on agency linked to the Explicate Order fundamentally differs from its conventional description as a mere set of biologic characteristics. Organisms’ capacities to anticipate future stress situations from past memories are obvious in simple animals such as nematodes, as well as in plants and bacteria 8 , suggesting that the subjective Explicate Order controls both organismal behavior and trans‐generational evolution.
That perspective offers insight to the nature of consciousness: not as a “mind” that is separate from a “body”, but as an endogenization of physical matter, which complies with the Laws of Nature. In other words, consciousness is the physiologic manifestation of endogenized physical surroundings, compartmentalized, and made essential for all organisms by forming the basis for their physiology. Endocytosis and endocytic/synaptic vesicles contribute to endogenization of cellular surroundings, allowing eukaryotic organisms to gain knowledge about the environment. This is true not only for neurons in brains, but also for all eukaryotic cells 5 .
Such a view of consciousness offers insight to our awareness of our physical surroundings as the basis for self‐referential self‐organization. But this is predicated on our capacity to “experiment” with our environment. The burgeoning idea that we are entering the Anthropocene, a man‐made world founded on subjective senses instead of Natural Laws, is a dangerous step away from our innate evolutionary arc. Relying on just our senses and emotions, without experimentation and controls to understand the Implicate Order behind reality, is not just an abandonment of the principles of the Enlightenment, but also endangers the planet and its diversity of life.
Further reading
Anderson C (2008) The End of Theory: the data deluge makes the scientific method obsolete. Wired (December 23, 2008)
Bacon F (1620, 2011) Novum Organum Scientiarum. Nabu Press
Baluška F, Gagliano M, Witzany G (2018) Memory and Learning in Plants. Springer Nature
Charlesworth AG, Seroussi U, Claycomb JM (2019) Next‐Gen learning: the C. elegans approach. Cell 177: 1674–1676
Eliezer Y, Deshe N, Hoch L, Iwanir S, Pritz CO, Zaslaver A (2019) A memory circuit for coping with impending adversity. Curr Biol 29: 1573–1583
Gagliano M, Renton M, Depczynski M, Mancuso S (2014) Experience teaches plants to learn faster and forget slower in environments where it matters. Oecologia 175: 63–72
Gagliano M, Vyazovskiy VV, Borbély AA, Grimonprez M, Depczynski M (2016) Learning by association in plants. Sci Rep 6: 38427
Katz M, Shaham S (2019) Learning and memory: mind over matter in C. elegans . Curr Biol 29: R365‐R367
Kováč L (2007) Information and knowledge in biology – time for reappraisal. Plant Signal Behav 2: 65–73
Kováč L (2008) Bioenergetics – a key to brain and mind. Commun Integr Biol 1: 114–122
Koshland DE Jr (1980) Bacterial chemotaxis in relation to neurobiology. Annu Rev Neurosci 3: 43–75
Lyon P (2015) The cognitive cell: bacterial behavior reconsidered. Front Microbiol 6: 264
Margulis L (2001) The conscious cell. Ann NY Acad Sci 929: 55–70
Maximillian N (2018) The Metaphysics of Science and Aim‐Oriented Empiricism. Springer: New York
Mazzocchi F (2015) Could Big Data be the end of theory in science? EMBO Rep 16: 1250–1255
Moore RS, Kaletsky R, Murphy CT (2019) Piwi/PRG‐1 argonaute and TGF‐β mediate transgenerational learned pathogenic avoidance. Cell 177: 1827–1841
Peirce CS (1877) The Fixation of Belief. Popular Science Monthly 12: 1–15
Pigliucci M (2009) The end of theory in science? EMBO Rep 10: 534
Popper K (1959) The Logic of Scientific Discovery. Routledge: London
Posner R, Toker IA, Antonova O, Star E, Anava S, Azmon E, Hendricks M, Bracha S, Gingold H, Rechavi O (2019) Neuronal small RNAs control behavior transgenerationally. Cell 177: 1814–1826
Russell B (1912) The Problems of Philosophy. Henry Holt and Company: New York
Scerri E (2006) The Periodic Table: It's Story and Significance. Oxford University Press, Oxford
Shapiro JA (2007) Bacteria are small but not stupid: cognition, natural genetic engineering and socio‐bacteriology. Stud Hist Philos Biol Biomed Sci 38: 807–818
Torday JS, Miller WB Jr (2016) Biologic relativity: who is the observer and what is observed? Prog Biophys Mol Biol 121: 29–34
Torday JS, Rehan VK (2017) Evolution, the Logic of Biology. Wiley: Hoboken
Torday JS, Miller WB Jr (2016) Phenotype as agent for epigenetic inheritance. Biology (Basel) 5: 30
Wasserstein RL, Lazar NA (2016) The ASA's statement on p‐values: context, process and purpose. Am Statist 70: 129–133
Yamada T, Yang Y, Valnegri P, Juric I, Abnousi A, Markwalter KH, Guthrie AN, Godec A, Oldenborg A, Hu M, Holy TE, Bonni A (2019) Sensory experience remodels genome architecture in neural circuit to drive motor learning. Nature 569: 708–713
Ladislav Kováč discussed the advantages and drawbacks of the inductive method for science and the logic of scientific discoveries 9 . Obviously, technological advances have enabled scientists to expand the borders of knowledge, and informatics allows us to objectively analyze ever larger data‐sets. It was the telescope that enabled Tycho Brahe, Johannes Kepler, and Galileo Galilei to make accurate observations and infer the motion of the planets. The microscope provided Robert Koch and Louis Pasteur insights into the microbial world and determines the nature of infectious diseases. Particle colliders now give us a glimpse into the birth of the Universe, while DNA sequencing and bioinformatics have enormously advanced biology's goal to understand the molecular basis of life.
However, Kováč also reminds us that Bayesian inferences and reasoning have serious drawbacks, as documented in the instructive example of Bertrand Russell's “inductivist turkey”, which collected large amounts of reproducible data each morning about feeding time. Based on these observations, the turkey correctly predicted the feeding time for the next morning—until Christmas Eve when the turkey's throat was cut 9 . In order to avoid the fate of the “inductivist turkey”, mankind should also rely on Popperian deductive science, namely formulating theories, concepts, and hypotheses, which are either confirmed or refuted via stringent experimentation and proper controls. Even if our scientific instruments provide us with objective data, we still need to apply our consciousness to evaluate and interpret such data. Moreover, before we start using our scientific instruments, we need to pose scientific questions. Therefore, as suggested by Albert Szent‐Györgyi, we need both Dionysian and Apollonian types of scientists 10 . Unfortunately, as was the case in Szent‐Györgyi's times, the Dionysians are still struggling to get proper support.
There have been pleas for reconciling philosophy and science, which parted ways owing to the rise of empiricism. This essay recognizes the centrality experiments and their controls for the advancement of scientific thought, and the attendant advance in philosophy needed to cope with many extant and emerging issues in science and society. We need a common “will” to do so. The rationale is provided herein, if only.
Acknowledgements
John Torday has been a recipient of NIH Grant HL055268. František Baluška is thankful to numerous colleagues for very stimulating discussions on topics analyzed in this article.
EMBO Reports (2019) 20 : e49110 [ PMC free article ] [ PubMed ] [ Google Scholar ]
Contributor Information
John S Torday, Email: ude.alcu@yadrotj .
František Baluška, Email: ed.nnob-inu@aksulab .
The Scholarly Kitchen
What’s Hot and Cooking In Scholarly Publishing
Understanding Experimental Controls
- Experimentation
Much of the training that scientists receive in graduate school is experiential, you learn how to do an experiment by working in a laboratory and performing experiments. In my opinion, not enough time and effort is devoted to understanding the philosophy and methods of experimental design.
An experiment without the proper controls is meaningless. Controls allow the experimenter to minimize the effects of factors other than the one being tested. It’s how we know an experiment is testing the thing it claims to be testing.
This goes beyond science — controls are necessary for any sort of experimental testing, no matter the subject area. This is often why so many bibliometric studies of the research literature are so problematic. Inadequate controls are often performed which fail to eliminate the effects of confounding factors, leaving the causality of any effect seen to be undetermined.
Novartis’ David Glass has put together the videos below, showing some of the basics of experimental validation and controls (Full disclosure: I was an editor on the first edition of David’s book on experimental design). These short videos offer quick lessons in positive and negative controls, as well as how to validate your experimental system.
These are great starting points, and I highly recommend Glass’ book, now in its second edition , if you want to dig deeper and understand the nuances of the different types of negative and positive controls, not to mention method and reagent controls, subject controls, assumption controls and experimentalist controls.
David Crotty
David Crotty is a Senior Consultant at Clarke & Esposito, a boutique management consulting firm focused on strategic issues related to professional and academic publishing and information services. Previously, David was the Editorial Director, Journals Policy for Oxford University Press. He oversaw journal policy across OUP’s journals program, drove technological innovation, and served as an information officer. David acquired and managed a suite of research society-owned journals with OUP, and before that was the Executive Editor for Cold Spring Harbor Laboratory Press, where he created and edited new science books and journals, along with serving as a journal Editor-in-Chief. He has served on the Board of Directors for the STM Association, the Society for Scholarly Publishing and CHOR, Inc., as well as The AAP-PSP Executive Council. David received his PhD in Genetics from Columbia University and did developmental neuroscience research at Caltech before moving from the bench to publishing.
7 Thoughts on "Understanding Experimental Controls"
We could add one more necessary control in this experiment–controlling for variability in individual response.
In the three videos, the experimenter may only detect differences between groups (or average differences). He is unable to detect changes in individuals. Some participants may be more sensitive to caffeine than others, some may show negative changes, and some may show no changes at all. If we take the blood pressure of participants before they drink coffee, we have a baseline measurement for all individuals. We also have a check on whether the experimenter was able to randomly assign participants to each treatment group.
In effect, each individual is their own control, with a before and after measurement. The experimenter is looking at the change in response of the individual rather than the average effect of the group. It is a much more sensitive way to structure and analyze experiments like this.
- By Phil Davis
- Nov 2, 2018, 8:57 AM
Agreed, these videos only skim the surface (his book goes into much greater detail about a much wider range of controls).
- By David Crotty
- Nov 2, 2018, 9:05 AM
Most experimenters who use random assignment to control and treatment groups have found that post-test only design works as well as pre-/post-test design.
- Nov 2, 2018, 10:01 AM
I don’t see how. By controlling for a potentially large source of variability—the individual participant—statistical tests become much more sensitive to changes than averaging all of that variability by group in a simple post-test design. Second, it is a check to see whether the randomization of participants into groups was successful. In many RTCs in the clinical sciences, there is recruitment bias, allowing for the sicker patients to be placed in the treatment group, for example.
- Nov 2, 2018, 12:55 PM
No mention of Institutional Review Board?! The IRB will raise Dr. Johnson’s own blood pressure.
And then there’s the issue of Dr. Johnson’s White Coat — that might trigger considerable individual variation. (My own blood pressure readings change markedly in the course of a visit to the doctor. )
- Nov 2, 2018, 4:59 PM
I believe that IRB approval is discussed in the video on system validation.
- Nov 2, 2018, 5:02 PM
Late to the debate, but I think those are wonderful. Maybe next Control Kitty will ask just how he assembled all those volunteers for his test to be representative and blinding to minimize bias. Were they self-selected? A bunch of caffeine habituated javaheads who responded to an ad in the coffee shop? I could see another video on randomization and sampling frames. I’m sure David Glass’s book goes into all that, but well, I have a shelf full of related books and I’m unlikely to benefit from and want to buy another. Unless maybe he hooks with another clever video or two. Go Kitty! Except, ~900 views! That’s sad. I might have sneak in citations to them. (I tend to get chastised by reviewers/editors for citing non-scholarly sources.) Something like this might slip under the editor’s radar: Glass, D. 2018. Experimental Design for Biologists: 1. System Validation. Video (4:06 minutes). YouTube. https://www.youtube.com/watch?v=qK9fXYDs–8 [Accessed November 11, 2018].
- By Chris Mebane
- Nov 12, 2018, 12:17 AM
Comments are closed.
Related Articles:
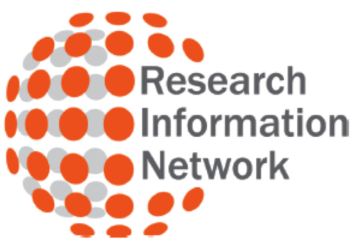
Next Article:
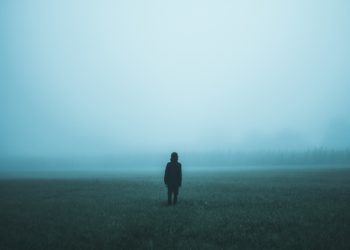
Have a language expert improve your writing
Run a free plagiarism check in 10 minutes, automatically generate references for free.
- Knowledge Base
- Methodology
- Controlled Experiments | Methods & Examples of Control
Controlled Experiments | Methods & Examples of Control
Published on 19 April 2022 by Pritha Bhandari . Revised on 10 October 2022.
In experiments , researchers manipulate independent variables to test their effects on dependent variables. In a controlled experiment , all variables other than the independent variable are controlled or held constant so they don’t influence the dependent variable.
Controlling variables can involve:
- Holding variables at a constant or restricted level (e.g., keeping room temperature fixed)
- Measuring variables to statistically control for them in your analyses
- Balancing variables across your experiment through randomisation (e.g., using a random order of tasks)
Table of contents
Why does control matter in experiments, methods of control, problems with controlled experiments, frequently asked questions about controlled experiments.
Control in experiments is critical for internal validity , which allows you to establish a cause-and-effect relationship between variables.
- Your independent variable is the colour used in advertising.
- Your dependent variable is the price that participants are willing to pay for a standard fast food meal.
Extraneous variables are factors that you’re not interested in studying, but that can still influence the dependent variable. For strong internal validity, you need to remove their effects from your experiment.
- Design and description of the meal
- Study environment (e.g., temperature or lighting)
- Participant’s frequency of buying fast food
- Participant’s familiarity with the specific fast food brand
- Participant’s socioeconomic status
Prevent plagiarism, run a free check.
You can control some variables by standardising your data collection procedures. All participants should be tested in the same environment with identical materials. Only the independent variable (e.g., advert colour) should be systematically changed between groups.
Other extraneous variables can be controlled through your sampling procedures . Ideally, you’ll select a sample that’s representative of your target population by using relevant inclusion and exclusion criteria (e.g., including participants from a specific income bracket, and not including participants with colour blindness).
By measuring extraneous participant variables (e.g., age or gender) that may affect your experimental results, you can also include them in later analyses.
After gathering your participants, you’ll need to place them into groups to test different independent variable treatments. The types of groups and method of assigning participants to groups will help you implement control in your experiment.
Control groups
Controlled experiments require control groups . Control groups allow you to test a comparable treatment, no treatment, or a fake treatment, and compare the outcome with your experimental treatment.
You can assess whether it’s your treatment specifically that caused the outcomes, or whether time or any other treatment might have resulted in the same effects.
- A control group that’s presented with red advertisements for a fast food meal
- An experimental group that’s presented with green advertisements for the same fast food meal
Random assignment
To avoid systematic differences between the participants in your control and treatment groups, you should use random assignment .
This helps ensure that any extraneous participant variables are evenly distributed, allowing for a valid comparison between groups .
Random assignment is a hallmark of a ‘true experiment’ – it differentiates true experiments from quasi-experiments .
Masking (blinding)
Masking in experiments means hiding condition assignment from participants or researchers – or, in a double-blind study , from both. It’s often used in clinical studies that test new treatments or drugs.
Sometimes, researchers may unintentionally encourage participants to behave in ways that support their hypotheses. In other cases, cues in the study environment may signal the goal of the experiment to participants and influence their responses.
Using masking means that participants don’t know whether they’re in the control group or the experimental group. This helps you control biases from participants or researchers that could influence your study results.
Although controlled experiments are the strongest way to test causal relationships, they also involve some challenges.
Difficult to control all variables
Especially in research with human participants, it’s impossible to hold all extraneous variables constant, because every individual has different experiences that may influence their perception, attitudes, or behaviors.
But measuring or restricting extraneous variables allows you to limit their influence or statistically control for them in your study.
Risk of low external validity
Controlled experiments have disadvantages when it comes to external validity – the extent to which your results can be generalised to broad populations and settings.
The more controlled your experiment is, the less it resembles real world contexts. That makes it harder to apply your findings outside of a controlled setting.
There’s always a tradeoff between internal and external validity . It’s important to consider your research aims when deciding whether to prioritise control or generalisability in your experiment.
Experimental designs are a set of procedures that you plan in order to examine the relationship between variables that interest you.
To design a successful experiment, first identify:
- A testable hypothesis
- One or more independent variables that you will manipulate
- One or more dependent variables that you will measure
When designing the experiment, first decide:
- How your variable(s) will be manipulated
- How you will control for any potential confounding or lurking variables
- How many subjects you will include
- How you will assign treatments to your subjects
Cite this Scribbr article
If you want to cite this source, you can copy and paste the citation or click the ‘Cite this Scribbr article’ button to automatically add the citation to our free Reference Generator.
Bhandari, P. (2022, October 10). Controlled Experiments | Methods & Examples of Control. Scribbr. Retrieved 3 September 2024, from https://www.scribbr.co.uk/research-methods/controlled-experiments/
Is this article helpful?
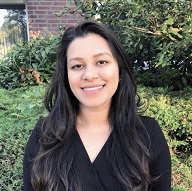
Pritha Bhandari
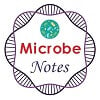
Microbe Notes
Controlled Experiments: Definition, Steps, Results, Uses
Controlled experiments ensure valid and reliable results by minimizing biases and controlling variables effectively.
Rigorous planning, ethical considerations, and precise data analysis are vital for successful experiment execution and meaningful conclusions.
Real-world applications demonstrate the practical impact of controlled experiments, guiding informed decision-making in diverse domains.
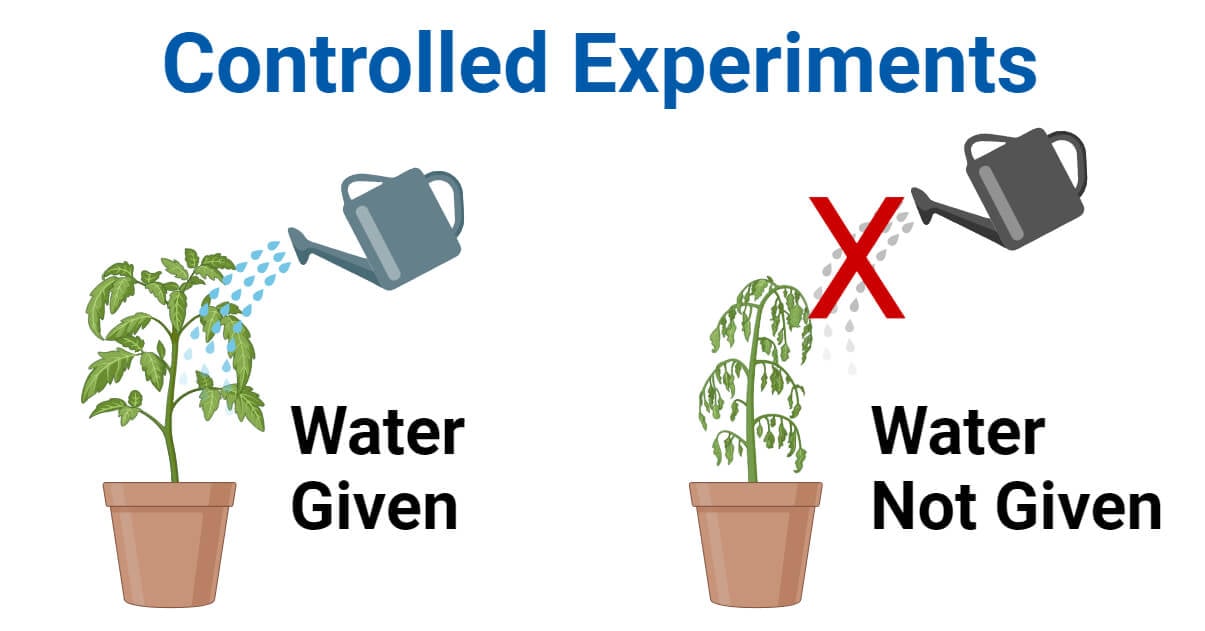
Controlled experiments are the systematic research method where variables are intentionally manipulated and controlled to observe the effects of a particular phenomenon. It aims to isolate and measure the impact of specific variables, ensuring a more accurate causality assessment.
Table of Contents
Interesting Science Videos
Importance of controlled experiments in various fields
Controlled experiments are significant across diverse fields, including science, psychology, economics, healthcare, and technology.
They provide a systematic approach to test hypotheses, establish cause-and-effect relationships, and validate the effectiveness of interventions or solutions.
Why Controlled Experiments Matter?
Validity and reliability of results.
Controlled experiments uphold the gold standard for scientific validity and reliability. By meticulously controlling variables and conditions, researchers can attribute observed outcomes accurately to the independent variable being tested. This precision ensures that the findings can be replicated and are trustworthy.
Minimizing Biases and Confounding Variables
One of the core benefits of controlled experiments lies in their ability to minimize biases and confounding variables. Extraneous factors that could distort results are mitigated through careful control and randomization. This enables researchers to isolate the effects of the independent variable, leading to a more accurate understanding of causality.
Achieving Causal Inference
Controlled experiments provide a strong foundation for establishing causal relationships between variables. Researchers can confidently infer causation by manipulating specific variables and observing resulting changes. The capability informs decision-making, policy formulation, and advancements across various fields.
Planning a Controlled Experiment
Formulating research questions and hypotheses.
Formulating clear research questions and hypotheses is paramount at the outset of a controlled experiment. These inquiries guide the direction of the study, defining the variables of interest and setting the stage for structured experimentation.
Well-defined questions and hypotheses contribute to focused research and facilitate meaningful data collection.
Identifying Variables and Control Groups
Identifying and defining independent, dependent, and control variables is fundamental to experimental planning.
Precise identification ensures that the experiment is designed to isolate the effect of the independent variable while controlling for other influential factors. Establishing control groups allows for meaningful comparisons and robust analysis of the experimental outcomes.
Designing Experimental Procedures and Protocols
Careful design of experimental procedures and protocols is essential for a successful controlled experiment. The step involves outlining the methodology, data collection techniques, and the sequence of activities in the experiment.
A well-designed experiment is structured to maintain consistency, control, and accuracy throughout the study, thereby enhancing the validity and credibility of the results.
Conducting a Controlled Experiment
Randomization and participant selection.
Randomization is a critical step in ensuring the fairness and validity of a controlled experiment. It involves assigning participants to different experimental conditions in a random and unbiased manner.
The selection of participants should accurately represent the target population, enhancing the results’ generalizability.
Data Collection Methods and Instruments
Selecting appropriate data collection methods and instruments is pivotal in gathering accurate and relevant data. Researchers often employ surveys, observations, interviews, or specialized tools to record and measure the variables of interest.
The chosen methods should align with the experiment’s objectives and provide reliable data for analysis.
Monitoring and Maintaining Experimental Conditions
Maintaining consistent and controlled experimental conditions throughout the study is essential. Regular monitoring helps ensure that variables remain constant and uncontaminated, reducing the risk of confounding factors.
Rigorous monitoring protocols and timely adjustments are crucial for the accuracy and reliability of the experiment.
Analysing Results and Drawing Conclusions
Data analysis techniques.
Data analysis involves employing appropriate statistical and analytical techniques to process the collected data. This step helps derive meaningful insights, identify patterns, and draw valid conclusions.
Common techniques include regression analysis, t-tests , ANOVA , and more, tailored to the research design and data type .
Interpretation of Results
Interpreting the results entails understanding the statistical outcomes and their implications for the research objectives.
Researchers analyze patterns, trends, and relationships revealed by the data analysis to infer the experiment’s impact on the variables under study. Clear and accurate interpretation is crucial for deriving actionable insights.
Implications and Potential Applications
Identifying the broader implications and potential applications of the experiment’s results is fundamental. Researchers consider how the findings can inform decision-making, policy development, or further research.
Understanding the practical implications helps bridge the gap between theoretical insights and real-world application.
Common Challenges and Solutions
Addressing ethical considerations.
Ethical challenges in controlled experiments include ensuring informed consent, protecting participants’ privacy, and minimizing harm.
Solutions involve thorough ethics reviews, transparent communication with participants, and implementing safeguards to uphold ethical standards throughout the experiment.
Dealing with Sample Size and Statistical Power
The sample size is crucial for achieving statistically significant results. Adequate sample sizes enhance the experiment’s power to detect meaningful effects accurately.
Statistical power analysis guides researchers in determining the optimal sample size for the experiment, minimizing the risk of type I and II errors .
Mitigating Unforeseen Variables
Unforeseen variables can introduce bias and affect the experiment’s validity. Researchers employ meticulous planning and robust control measures to minimize the impact of unforeseen variables.
Pre-testing and pilot studies help identify potential confounders, allowing researchers to adapt the experiment accordingly.
A controlled experiment involves meticulous planning, precise execution, and insightful analysis. Adhering to ethical standards, optimizing sample size, and adapting to unforeseen variables are key challenges that require thoughtful solutions.
Real-world applications showcase the transformative potential of controlled experiments across varied domains, emphasizing their indispensable role in evidence-based decision-making and progress.
- https://www.khanacademy.org/science/biology/intro-to-biology/science-of-biology/a/experiments-and-observations
- https://www.scribbr.com/methodology/controlled-experiment/
- https://link.springer.com/10.1007/978-1-4899-7687-1_891
- http://ai.stanford.edu/~ronnyk/GuideControlledExperiments.pdf
- https://www.ncbi.nlm.nih.gov/pmc/articles/PMC6776925/
- https://www.ncbi.nlm.nih.gov/pmc/articles/PMC4017459/
- https://www.merriam-webster.com/dictionary/controlled%20experiment
About Author
Krisha Karki
Leave a Comment Cancel reply
Save my name, email, and website in this browser for the next time I comment.
Have a language expert improve your writing
Run a free plagiarism check in 10 minutes, generate accurate citations for free.
- Knowledge Base
Methodology
- Guide to Experimental Design | Overview, Steps, & Examples
Guide to Experimental Design | Overview, 5 steps & Examples
Published on December 3, 2019 by Rebecca Bevans . Revised on June 21, 2023.
Experiments are used to study causal relationships . You manipulate one or more independent variables and measure their effect on one or more dependent variables.
Experimental design create a set of procedures to systematically test a hypothesis . A good experimental design requires a strong understanding of the system you are studying.
There are five key steps in designing an experiment:
- Consider your variables and how they are related
- Write a specific, testable hypothesis
- Design experimental treatments to manipulate your independent variable
- Assign subjects to groups, either between-subjects or within-subjects
- Plan how you will measure your dependent variable
For valid conclusions, you also need to select a representative sample and control any extraneous variables that might influence your results. If random assignment of participants to control and treatment groups is impossible, unethical, or highly difficult, consider an observational study instead. This minimizes several types of research bias, particularly sampling bias , survivorship bias , and attrition bias as time passes.
Table of contents
Step 1: define your variables, step 2: write your hypothesis, step 3: design your experimental treatments, step 4: assign your subjects to treatment groups, step 5: measure your dependent variable, other interesting articles, frequently asked questions about experiments.
You should begin with a specific research question . We will work with two research question examples, one from health sciences and one from ecology:
To translate your research question into an experimental hypothesis, you need to define the main variables and make predictions about how they are related.
Start by simply listing the independent and dependent variables .
Research question | Independent variable | Dependent variable |
---|---|---|
Phone use and sleep | Minutes of phone use before sleep | Hours of sleep per night |
Temperature and soil respiration | Air temperature just above the soil surface | CO2 respired from soil |
Then you need to think about possible extraneous and confounding variables and consider how you might control them in your experiment.
Extraneous variable | How to control | |
---|---|---|
Phone use and sleep | in sleep patterns among individuals. | measure the average difference between sleep with phone use and sleep without phone use rather than the average amount of sleep per treatment group. |
Temperature and soil respiration | also affects respiration, and moisture can decrease with increasing temperature. | monitor soil moisture and add water to make sure that soil moisture is consistent across all treatment plots. |
Finally, you can put these variables together into a diagram. Use arrows to show the possible relationships between variables and include signs to show the expected direction of the relationships.
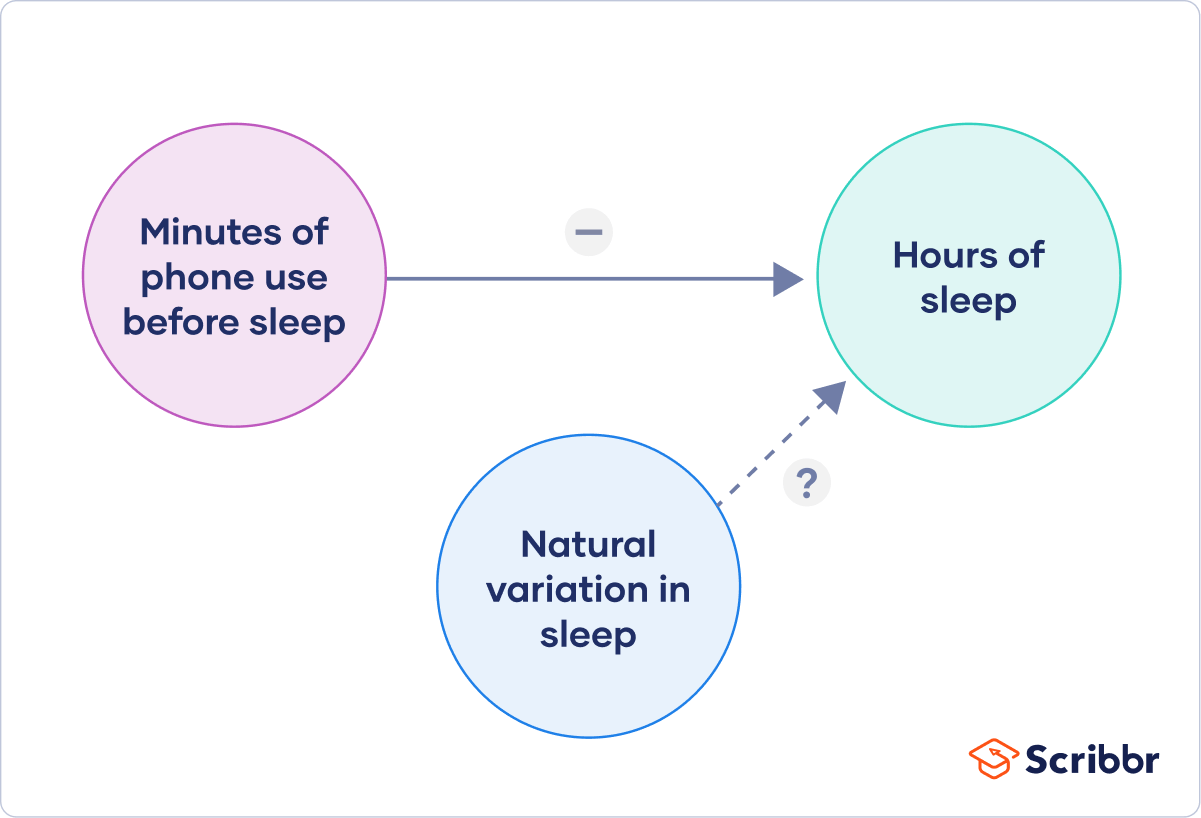
Here we predict that increasing temperature will increase soil respiration and decrease soil moisture, while decreasing soil moisture will lead to decreased soil respiration.
Prevent plagiarism. Run a free check.
Now that you have a strong conceptual understanding of the system you are studying, you should be able to write a specific, testable hypothesis that addresses your research question.
Null hypothesis (H ) | Alternate hypothesis (H ) | |
---|---|---|
Phone use and sleep | Phone use before sleep does not correlate with the amount of sleep a person gets. | Increasing phone use before sleep leads to a decrease in sleep. |
Temperature and soil respiration | Air temperature does not correlate with soil respiration. | Increased air temperature leads to increased soil respiration. |
The next steps will describe how to design a controlled experiment . In a controlled experiment, you must be able to:
- Systematically and precisely manipulate the independent variable(s).
- Precisely measure the dependent variable(s).
- Control any potential confounding variables.
If your study system doesn’t match these criteria, there are other types of research you can use to answer your research question.
How you manipulate the independent variable can affect the experiment’s external validity – that is, the extent to which the results can be generalized and applied to the broader world.
First, you may need to decide how widely to vary your independent variable.
- just slightly above the natural range for your study region.
- over a wider range of temperatures to mimic future warming.
- over an extreme range that is beyond any possible natural variation.
Second, you may need to choose how finely to vary your independent variable. Sometimes this choice is made for you by your experimental system, but often you will need to decide, and this will affect how much you can infer from your results.
- a categorical variable : either as binary (yes/no) or as levels of a factor (no phone use, low phone use, high phone use).
- a continuous variable (minutes of phone use measured every night).
How you apply your experimental treatments to your test subjects is crucial for obtaining valid and reliable results.
First, you need to consider the study size : how many individuals will be included in the experiment? In general, the more subjects you include, the greater your experiment’s statistical power , which determines how much confidence you can have in your results.
Then you need to randomly assign your subjects to treatment groups . Each group receives a different level of the treatment (e.g. no phone use, low phone use, high phone use).
You should also include a control group , which receives no treatment. The control group tells us what would have happened to your test subjects without any experimental intervention.
When assigning your subjects to groups, there are two main choices you need to make:
- A completely randomized design vs a randomized block design .
- A between-subjects design vs a within-subjects design .
Randomization
An experiment can be completely randomized or randomized within blocks (aka strata):
- In a completely randomized design , every subject is assigned to a treatment group at random.
- In a randomized block design (aka stratified random design), subjects are first grouped according to a characteristic they share, and then randomly assigned to treatments within those groups.
Completely randomized design | Randomized block design | |
---|---|---|
Phone use and sleep | Subjects are all randomly assigned a level of phone use using a random number generator. | Subjects are first grouped by age, and then phone use treatments are randomly assigned within these groups. |
Temperature and soil respiration | Warming treatments are assigned to soil plots at random by using a number generator to generate map coordinates within the study area. | Soils are first grouped by average rainfall, and then treatment plots are randomly assigned within these groups. |
Sometimes randomization isn’t practical or ethical , so researchers create partially-random or even non-random designs. An experimental design where treatments aren’t randomly assigned is called a quasi-experimental design .
Between-subjects vs. within-subjects
In a between-subjects design (also known as an independent measures design or classic ANOVA design), individuals receive only one of the possible levels of an experimental treatment.
In medical or social research, you might also use matched pairs within your between-subjects design to make sure that each treatment group contains the same variety of test subjects in the same proportions.
In a within-subjects design (also known as a repeated measures design), every individual receives each of the experimental treatments consecutively, and their responses to each treatment are measured.
Within-subjects or repeated measures can also refer to an experimental design where an effect emerges over time, and individual responses are measured over time in order to measure this effect as it emerges.
Counterbalancing (randomizing or reversing the order of treatments among subjects) is often used in within-subjects designs to ensure that the order of treatment application doesn’t influence the results of the experiment.
Between-subjects (independent measures) design | Within-subjects (repeated measures) design | |
---|---|---|
Phone use and sleep | Subjects are randomly assigned a level of phone use (none, low, or high) and follow that level of phone use throughout the experiment. | Subjects are assigned consecutively to zero, low, and high levels of phone use throughout the experiment, and the order in which they follow these treatments is randomized. |
Temperature and soil respiration | Warming treatments are assigned to soil plots at random and the soils are kept at this temperature throughout the experiment. | Every plot receives each warming treatment (1, 3, 5, 8, and 10C above ambient temperatures) consecutively over the course of the experiment, and the order in which they receive these treatments is randomized. |
Finally, you need to decide how you’ll collect data on your dependent variable outcomes. You should aim for reliable and valid measurements that minimize research bias or error.
Some variables, like temperature, can be objectively measured with scientific instruments. Others may need to be operationalized to turn them into measurable observations.
- Ask participants to record what time they go to sleep and get up each day.
- Ask participants to wear a sleep tracker.
How precisely you measure your dependent variable also affects the kinds of statistical analysis you can use on your data.
Experiments are always context-dependent, and a good experimental design will take into account all of the unique considerations of your study system to produce information that is both valid and relevant to your research question.
If you want to know more about statistics , methodology , or research bias , make sure to check out some of our other articles with explanations and examples.
- Student’s t -distribution
- Normal distribution
- Null and Alternative Hypotheses
- Chi square tests
- Confidence interval
- Cluster sampling
- Stratified sampling
- Data cleansing
- Reproducibility vs Replicability
- Peer review
- Likert scale
Research bias
- Implicit bias
- Framing effect
- Cognitive bias
- Placebo effect
- Hawthorne effect
- Hindsight bias
- Affect heuristic
Experimental design means planning a set of procedures to investigate a relationship between variables . To design a controlled experiment, you need:
- A testable hypothesis
- At least one independent variable that can be precisely manipulated
- At least one dependent variable that can be precisely measured
When designing the experiment, you decide:
- How you will manipulate the variable(s)
- How you will control for any potential confounding variables
- How many subjects or samples will be included in the study
- How subjects will be assigned to treatment levels
Experimental design is essential to the internal and external validity of your experiment.
The key difference between observational studies and experimental designs is that a well-done observational study does not influence the responses of participants, while experiments do have some sort of treatment condition applied to at least some participants by random assignment .
A confounding variable , also called a confounder or confounding factor, is a third variable in a study examining a potential cause-and-effect relationship.
A confounding variable is related to both the supposed cause and the supposed effect of the study. It can be difficult to separate the true effect of the independent variable from the effect of the confounding variable.
In your research design , it’s important to identify potential confounding variables and plan how you will reduce their impact.
In a between-subjects design , every participant experiences only one condition, and researchers assess group differences between participants in various conditions.
In a within-subjects design , each participant experiences all conditions, and researchers test the same participants repeatedly for differences between conditions.
The word “between” means that you’re comparing different conditions between groups, while the word “within” means you’re comparing different conditions within the same group.
An experimental group, also known as a treatment group, receives the treatment whose effect researchers wish to study, whereas a control group does not. They should be identical in all other ways.
Cite this Scribbr article
If you want to cite this source, you can copy and paste the citation or click the “Cite this Scribbr article” button to automatically add the citation to our free Citation Generator.
Bevans, R. (2023, June 21). Guide to Experimental Design | Overview, 5 steps & Examples. Scribbr. Retrieved September 3, 2024, from https://www.scribbr.com/methodology/experimental-design/
Is this article helpful?
Rebecca Bevans
Other students also liked, random assignment in experiments | introduction & examples, quasi-experimental design | definition, types & examples, how to write a lab report, "i thought ai proofreading was useless but..".
I've been using Scribbr for years now and I know it's a service that won't disappoint. It does a good job spotting mistakes”
» » » , updated on are processes and procedures designed to minimize the influence of on an experiment other than the . The goal is to produce results that are valid, reliable, and reproducible. The following are basic types of experiment control. Methods that are designed to reduce error in conducting the experiment. For example, a process of taking measurements twice to reduce the potential for measurement error. The practice of keeping a variable constant to minimize its influence on results. Ideally, all that can influence results that aren't the are controlled in this manner. In some cases, variables can't be held constant but steps are taken to reduce their variation. For example, in an outdoor experiment with plants it may be impossible to keep wind at a constant but a fence structure may be used to prevent extreme variations in wind from one spot to the next on the field. The process of conducting the experiment in the exact same way on a except that the independent variables are a placebo that is not expected to produce a result. For example, an experiment on plants where one group of plants are given a fertilizer delivered in a solution and a control group that are given the same amount of the solution that contains no fertilizer. A is not exposed to the experimental treatment but is exposed to another treatment that is known to work. In other words, the independent variables in a positive control group are changed to a known method for achieving the desired result. For example, an experiment on a new fertilizer may have a positive control that uses a fertilizer that is known to increase plant growth. Selecting members of treatment and control groups at random. For example, a field may be divided into 36 small plots and these plots assigned at random to a treatment group and two different . The practice of not informing participants whether they are in the treatment or (placebo) group. This is done to encourage the placebo effect whereby people may show some improvement simply because they believe they are being treated. The practice of not informing some of the experimenters which of the participants in an experiment are in a control group. This is done to eliminate unintentional bias. For practical reasons, some of the experimenters may need to know the members of the control group such as the individual who performs the randomization. The ultimate test of the validity and reliability of an experiment is to have results reproduced by independent third parties with similar but potentially different experiments. In some fields, such as Psychology, reproducibility rates are remarkably low.
ExperimentsExperiment VariablesIndependent variables. Dependent Variable
Scientific ControlExtraneous variables, control group, negative control vs positive control, negative control, innovation process, business model innovation, knowledge economy, adoption rate, early adopters, research & development, entrepreneurship, new articles. What Is a Controlled Experiment?Definition and Example
A controlled experiment is one in which everything is held constant except for one variable . Usually, a set of data is taken to be a control group , which is commonly the normal or usual state, and one or more other groups are examined where all conditions are identical to the control group and to each other except for one variable. Sometimes it's necessary to change more than one variable, but all of the other experimental conditions will be controlled so that only the variables being examined change. And what is measured is the variables' amount or the way in which they change. Controlled Experiment
Example of a Controlled ExperimentLet's say you want to know if the type of soil affects how long it takes a seed to germinate, and you decide to set up a controlled experiment to answer the question. You might take five identical pots, fill each with a different type of soil, plant identical bean seeds in each pot, place the pots in a sunny window, water them equally, and measure how long it takes for the seeds in each pot to sprout. This is a controlled experiment because your goal is to keep every variable constant except the type of soil you use. You control these features. Why Controlled Experiments Are ImportantThe big advantage of a controlled experiment is that you can eliminate much of the uncertainty about your results. If you couldn't control each variable, you might end up with a confusing outcome. For example, if you planted different types of seeds in each of the pots, trying to determine if soil type affected germination, you might find some types of seeds germinate faster than others. You wouldn't be able to say, with any degree of certainty, that the rate of germination was due to the type of soil. It might as well have been due to the type of seeds. Or, if you had placed some pots in a sunny window and some in the shade or watered some pots more than others, you could get mixed results. The value of a controlled experiment is that it yields a high degree of confidence in the outcome. You know which variable caused or did not cause a change. Are All Experiments Controlled?No, they are not. It's still possible to obtain useful data from uncontrolled experiments, but it's harder to draw conclusions based on the data. An example of an area where controlled experiments are difficult is human testing. Say you want to know if a new diet pill helps with weight loss. You can collect a sample of people, give each of them the pill, and measure their weight. You can try to control as many variables as possible, such as how much exercise they get or how many calories they eat. However, you will have several uncontrolled variables, which may include age, gender, genetic predisposition toward a high or low metabolism, how overweight they were before starting the test, whether they inadvertently eat something that interacts with the drug, etc. Scientists try to record as much data as possible when conducting uncontrolled experiments, so they can see additional factors that may be affecting their results. Although it is harder to draw conclusions from uncontrolled experiments, new patterns often emerge that would not have been observable in a controlled experiment. For example, you may notice the diet drug seems to work for female subjects, but not for male subjects, and this may lead to further experimentation and a possible breakthrough. If you had only been able to perform a controlled experiment, perhaps on male clones alone, you would have missed this connection.
![]() Controlled ExperimentReviewed by: BD Editors Controlled Experiment DefinitionA controlled experiment is a scientific test that is directly manipulated by a scientist, in order to test a single variable at a time. The variable being tested is the independent variable , and is adjusted to see the effects on the system being studied. The controlled variables are held constant to minimize or stabilize their effects on the subject. In biology, a controlled experiment often includes restricting the environment of the organism being studied. This is necessary to minimize the random effects of the environment and the many variables that exist in the wild. In a controlled experiment, the study population is often divided into two groups. One group receives a change in a certain variable, while the other group receives a standard environment and conditions. This group is referred to as the control group , and allows for comparison with the other group, known as the experimental group . Many types of controls exist in various experiments, which are designed to ensure that the experiment worked, and to have a basis for comparison. In science, results are only accepted if it can be shown that they are statistically significant . Statisticians can use the difference between the control group and experimental group and the expected difference to determine if the experiment supports the hypothesis , or if the data was simply created by chance. Examples of Controlled ExperimentMusic preference in dogs. Do dogs have a taste in music? You might have considered this, and science has too. Believe it or not, researchers have actually tested dog’s reactions to various music genres. To set up a controlled experiment like this, scientists had to consider the many variables that affect each dog during testing. The environment the dog is in when listening to music, the volume of the music, the presence of humans, and even the temperature were all variables that the researches had to consider. In this case, the genre of the music was the independent variable. In other words, to see if dog’s change their behavior in response to different kinds of music, a controlled experiment had to limit the interaction of the other variables on the dogs. Usually, an experiment like this is carried out in the same location, with the same lighting, furniture, and conditions every time. This ensures that the dogs are not changing their behavior in response to the room. To make sure the dogs don’t react to humans or simply the noise of the music, no one else can be in the room and the music must be played at the same volume for each genre. Scientist will develop protocols for their experiment, which will ensure that many other variables are controlled. This experiment could also split the dogs into two groups, only testing music on one group. The control group would be used to set a baseline behavior, and see how dogs behaved without music. The other group could then be observed and the differences in the group’s behavior could be analyzed. By rating behaviors on a quantitative scale, statistics can be used to analyze the difference in behavior, and see if it was large enough to be considered significant. This basic experiment was carried out on a large number of dogs, analyzing their behavior with a variety of different music genres. It was found that dogs do show more relaxed and calm behaviors when a specific type of music plays. Come to find out, dogs enjoy reggae the most. Scurvy in SailorsIn the early 1700s, the world was a rapidly expanding place. Ships were being built and sent all over the world, carrying thousands and thousands of sailors. These sailors were mostly fed the cheapest diets possible, not only because it decreased the costs of goods, but also because fresh food is very hard to keep at sea. Today, we understand that lack of essential vitamins and nutrients can lead to severe deficiencies that manifest as disease. One of these diseases is scurvy. Scurvy is caused by a simple vitamin C deficiency, but the effects can be brutal. Although early symptoms just include general feeling of weakness, the continued lack of vitamin C will lead to a breakdown of the blood cells and vessels that carry the blood. This results in blood leaking from the vessels. Eventually, people bleed to death internally and die. Before controlled experiments were commonplace, a simple physician decided to tackle the problem of scurvy. James Lind, of the Royal Navy, came up with a simple controlled experiment to find the best cure for scurvy. He separated sailors with scurvy into various groups. He subjected them to the same controlled condition and gave them the same diet, except one item. Each group was subjected to a different treatment or remedy, taken with their food. Some of these remedies included barley water, cider and a regiment of oranges and lemons. This created the first clinical trial , or test of the effectiveness of certain treatments in a controlled experiment. Lind found that the oranges and lemons helped the sailors recover fast, and within a few years the Royal Navy had developed protocols for growing small leafy greens that contained high amounts of vitamin C to feed their sailors. Related Biology Terms
Cite This ArticleSubscribe to our newsletter, privacy policy, terms of service, scholarship, latest posts, white blood cell, t cell immunity, satellite cells, embryonic stem cells, popular topics, amino acids, water cycle, endocrine system, adenosine triphosphate (atp), pituitary gland, horticulture, animal cell. ![]() 10 Experimental Control Examples![]() Chris Drew (PhD) Dr. Chris Drew is the founder of the Helpful Professor. He holds a PhD in education and has published over 20 articles in scholarly journals. He is the former editor of the Journal of Learning Development in Higher Education. [Image Descriptor: Photo of Chris] Learn about our Editorial Process ![]() Experimental control refers to the practice of isolating the effects of a single variable in an experiment to ensure that this variable is the only factor affecting the results. Generally, it involves identifying all possible confounding variables and controlling for them so you can truly see whether the independent variable is causing the expected effect on the dependent variable. This methodology allows the researcher to draw definitive conclusions about cause-and-effect relationships. This approach is considered the gold standard for determining cause-and-effect in scientific research. Experimental Control ExamplesThe following are some methods for controlling variables in experimental design : 1. Random AssignmentIn experimental research, random assignment is an often-employed method for controlling variables. Its purpose is to minimize bias and ensure that participant characteristics are evenly distributed across all groups. The method involves assigning participants to groups randomly rather than based on specific characteristics. This ensures that the resulting observations are due to the intervention or test variable, not to differences between groups. Random assignment therefore helps in controlling for confounding variables and establishing causal relationships. Random Assignment Example : Suppose you’re investigating a new math teaching method’s effectiveness. You’d start off by collecting 100 volunteer students. Every student gets a number, 1 to 100, and a computer algorithm randomly assigns each student to either Group A or B. Group A is taught math using the new method, while Group B continues with the traditional teaching style. After a period of testing, you’d gauge the difference between Group A and B’s math scores to assess the new method’s effectiveness. 2. BlindingBlinding is another commonly used technique in experimental research for the control of variables. It serves to eliminate bias, specifically observer and subject bias. In a blinded study, one or more parties involved do not have information about the interventions received by each participant. There are various degrees of blinding, from single-blind, where the participant doesn’t know their group assignment, to double-blind, where both the participant and the investigator are oblivious. Blinding Example: Let’s envision a scenario where a researcher is studying the impact of a new exercise routine on weight loss. In this case, the participants wouldn’t know if they were part of the control group doing routine exercises or the experimental group trying the new routine—this is a single-blind experiment. If we advance by one step to a double-blind experiment, not only would the participants be in the dark, but the investigators assessing the results wouldn’t know which group each participant belongs to either. Thus, blinding keeps conscious and subconscious biases at bay and ensures the reliability of the results. 3. Negative Control GroupsUtilization of negative control groups is a fundamental method for controlling variables in experimental research. Control groups enable researchers to isolate the effects of the variable they are studying. A negative control group consists of participants who do not receive the treatment or intervention being studied. They provide a baseline against which to measure the effects of the treatment on the experimental group. Implementing a control group helps maintain the integrity and validity of the results by eliminating the chance that outside factors are causing the observed effects. Negative Control Group Example: Let’s investigate a new dietary supplement’s effectiveness on improving memory. You would divide your participants into an experimental group and a control group. The experimental group would take the supplement regularly, but the control group would be given a placebo – a capsule identical in appearance but without the active ingredient. After the trial period, you’d compare data on memory capabilities between the two groups. If the experimental group showed substantial improvements compared to the control group, then it’s possible to conclude that the supplement positively affects memory. 4. Positive Control GroupsPositive control groups serve as another crucial method of controlling variables in experimental research. These groups help validate the outcome of an experiment by ensuring that it produces a known result. A positive control group is given a treatment that is already known to produce the expected effect. This helps researchers ensure the experiment is working as designed and demonstrates the capability of the experimental setup to generate positive results. In essence, positive control groups contribute to the reliability of experimental results by confirming that the research protocol is effective. Positive Control Group Example: For instance, in a study designed to test a new antibiotic’s effectiveness, you might create a positive control group that receives a current, widely-accepted antibiotic known to effectively treat the condition. The experimental group gets the new antibiotic. If both groups show improved health, you have evidence that your experimental setup (i.e., ability to gauge improvement) works and that the new antibiotic could be effective. If the positive control group doesn’t show improvement, it may indicate issues with the process or the antibiotic used for the positive control. ![]() 5. MatchingMatching is a statistically sound method often used to control variables in experimental research. This approach aims to create comparable groups in terms of specific characteristics that may influence the study’s outcome. In a matched study, each participant in the experimental group has a direct counterpart in the control group with similar demographics or other traits. These characteristics could include age, gender, ethnicity, body mass index, disease severity, and more. Matching helps ensure that any differences between the experimental and control groups are due to the independent variable under study, not other confounding variables. Matching Example: Let’s assume you’re researching the effects of a new rehabilitation program for stroke patients. Matching might be used to ensure each participant in the group receiving the new rehabilitation is paired with a participant in the regular care group of the same age, gender, and severity of stroke. By doing so, you ensure that the differences observed are likely due to the rehabilitation program and not other unrelated factors. 6. CounterbalancingCounterbalancing is often applied as a method for controlling variables in experimental research, significantly in studies involving repeated measures or sequences. It is used to manage the potential impact of order effects such as learning, habituation, or fatigue. In counterbalancing, researchers alternate the order in which participants experience conditions or treatments. The aim is to balance the potential impact of the condition order across participants, neutralizing confounding influences tied to the sequence. By using this method, researchers can ensure that the observed results are not biased due to the order of presentation. Counterbalancing Example: Suppose you’re investigating the effect of different types of background noise (music, silence, or white noise) on concentration levels. All participants are tested under these conditions, but the order in which they experience them differs. Some participants might first work with music playing, then in silence, and finally with white noise. Others could start with silence, then white noise, and finally music. By varying and balancing these conditions, you ensure that you aren’t just measuring whether the ability to concentrate changes simply because of the order in which the variables are experienced. 7. Parallel Groups DesignParallel groups design is all about conducting studies in the exact same time period to control for changes that might take place across different time periods. This approach is widely used in clinical trials and offers an effective means of reducing potential biases. In this setup, participants are assigned to different groups, and each group receives a different treatment or intervention simultaneously. All groups are measured during the same time period, reducing the effects of time-dependent covariates. This method helps ensure that observed variations are due to the different treatments rather than other external factors. Parallel Groups Design Example: Consider a trial in which you’re testing the effectiveness of two different vaccines against a disease. You have two groups of participants that are fairly similar in terms of age, health, etc. Group A receives Vaccine 1, and Group B receives Vaccine 2. This testing happens parallelly. After a defined period, you measure the health status of participants in both groups to determine which vaccine was more effective. Since both groups were tested during the same time period, any differences can largely be attributed to the difference in vaccines, not an evolution in time or other outside factors. 8. Factorial DesignIn a factorial design, all combinations of the various levels of the independent variables investigated are tested. This allows us to understand not just the main effects of our variables, but also any potential interaction effects between them. Imagine you want find out how the amount of sunlight and water given to a plant affects how fast it grows. here, you have two variables (sunlight and water) that will interact in a variety of ways, so you’d want to study each interaction:
This is a factorial design – it allows you to see how different combinations of sunlight and water affect how fast sunflowers grow. Implementing this method helps in increasing the efficiency and scope of experiments without requiring significantly more subjects or resources. Factorial Design Example: Suppose you’re studying the effects of diet and exercise on weight loss. You have two levels of diet (low calorie, normal calorie), and two levels of exercise (regular exercise, no exercise). So, in a 2×2 factorial design, you have four conditions: low calorie diet with regular exercise, low calorie diet with no exercise, normal calorie diet with regular exercise, and normal calorie diet with no exercise. Participants would be randomly assigned to one of these four conditions, allowing you to examine the separate effects of diet and exercise and their combined effect on weight loss. ![]() 9. Crossover DesignIn crossover design, each participant acts as their control by receiving both the treatment and the placebo at different times. The crossover design has the advantage of eliminating the potential variance between individuals because the same subjects are subjected to both the treatment and the control condition. This method requires fewer subjects, increases statistical power, and is beneficial when the effects of the treatment are temporary and when no carry-over effects take place. However, it’s crucial to include a ‘wash-out’ period between treatments to reduce potential carry-over effects. Crossover Design Example: In a study testing the effect of a new painkiller on headache relief, participants would be randomly allocated to receive either the painkiller or a placebo first. After a predefined time period, there would be a wash-out phase during which no medications are administered. Following the wash-out phase, those initially given the painkiller would get a placebo, and those first given the placebo would receive the painkiller. In the end, researchers compare the data from when each group received the painkiller and placebos, not confounded by individual variance as each participant received both treatment and control conditions. 10. Stratified Random SamplingStratified random sampling entails dividing the population into subgroups or strata based on specific characteristics, and then randomly selecting participants from each subgroup. This method ensures that the sample accurately represents the overall population. It is beneficial when researchers believe certain characteristics (like age, sex, or ethnicity) in the population group could influence the outcome of the study. Stratification results in increased statistical precision and ensures that all segments of a population are represented, helping to achieve variable control . Stratified Random Sampling Example : For instance, if you’re carrying out a health survey, you could divide the population into age categories (under 20, 20-29, 30-39, and so on). From each age group you can then randomly select participants. This ensures that your sample encompasses all age groups and when you share your research, you can share credible conclusions about specific age categories as well as the wider population. Experimental research methodologies necessitate rigorous controls to ensure valid and reliable results. Techniques like random assignment, blinding, and usage of control groups manage confounding variables, enhancing the integrity of the findings. Researchers have developed a range of methods for increasing control over the experiment and better measuring cause and effect . But it’s important to be judicious in knowing which experimental controls to use in which circumstance, based largely on the study design and research question. ![]()
Leave a Comment Cancel ReplyYour email address will not be published. Required fields are marked *
![]() What happens in experimental research is that the researcher alters the independent variables so as to determine their impacts on the dependent variables. Therefore, when the experiment is controlled, you can expect that the researcher will control all other variables except for the independent variables . This is done so that the other variables do not have an influence on the dependent variables. In this article, we are going to consider controlled experiment, how important it is in a study, and how it can be designed. But before we dig deep, let us look at the definition of a controlled experiment. What is a Controlled Experiment?In a scientific experiment, a controlled experiment is a test that is directly altered by the researcher so that only one variable is studied at a time. The single variable being studied will then be the independent variable. This independent variable is manipulated by the researcher so that its effect on the hypothesis or data being studied is known. While the researcher studies the single independent variable, the controlled variables are made constant to reduce or balance out their impact on the research. To achieve a controlled experiment, the research population is mostly distributed into two groups. Then the treatment is administered to one of the two groups, while the other group gets the control conditions. This other group is referred to as the control group. The control group gets the standard conditions and is placed in the standard environment and it also allows for comparison with the other group, which is referred to as the experimental group or the treatment group. Obtaining the difference between these two groups’ behavior is important because in any scientific experiment, being able to show the statistical significance of the results is the only criterion for the results to be accepted. So to determine whether the experiment supports the hypothesis, or if the data is a result of chance, the researcher will check for the difference between the control group and experimental group. Then the results from the differences will be compared with the expected difference. For example, a researcher may want to answer this question, do dogs also have a music taste? In case you’re wondering too, yes, there are existing studies by researchers on how dogs react to different music genres. Back to the example, the researcher may develop a controlled experiment with high consideration on the variables that affect each dog. Some of these variables that may have effects on the dog are; the dog’s environment when listening to music, the temperature of the environment, the music volume, and human presence. The independent variable to focus on in this research is the genre of the music. To determine if there is an effect on the dog while listening to different kinds of music, the dog’s environment must be controlled. A controlled experiment would limit interaction between the dog and other variables. In this experiment, the researcher can also divide the dogs into two groups, one group will perform the music test while the other, the control group will be used as the baseline or standard behavior. The control group behavior can be observed along with the treatment group and the differences in the two group’s behavior can be analyzed. What is an Experimental Control?Experimental control is the technique used by the researcher in scientific research to minimize the effects of extraneous variables. Experimental control also strengthens the ability of the independent variable to change the dependent variable. For example, the cause and effect possibilities will be examined in a well-designed and properly controlled experiment if the independent variable (Treatment Y) causes a behavioral change in the dependent variable (Subject X). In another example, a researcher feeds 20 lab rats with an artificial sweetener and from the researcher’s observation, six of the rats died of dehydration. Now, the actual cause of death may be artificial sweeteners or an unrelated factor. Such as the water supplied to the rats being contaminated or the rats could not drink enough, or suffering a disease. Read: Nominal, Ordinal, Interval & Ratio Variable + [Examples] For a researcher, eliminating these potential causes one after the other will consume time, and be tedious. Hence, the researcher can make use of experimental control. This method will allow the researcher to divide the rats into two groups: one group will receive the artificial sweetener while the other one doesn’t. The two groups will be placed in similar conditions and observed in similar ways. The differences that now occur in morbidity between the two groups can be traced to the sweetener with certainty. From the example above, the experimental control is administered as a form of a control group. The data from the control group is then said to be the standard against which every other experimental outcome is measured. Purpose & Importance of Control in Experimentation1. One significant purpose of experimental controls is that it allows researchers to eliminate various confounding variables or uncertainty in their research. A researcher will need to use an experimental control to ensure that only the variables that are intended to change, are changed in research. 2. Controlled experiments also allow researchers to control the specific variables they think might have an effect on the outcomes of the study. The researcher will use a control group if he/she believes some extra variables can form an effect on the results of the study. This is to ensure that the extra variable is held constant and possible influences are measured. 3. Controlled experiments establish a standard that the outcome of a study should be compared to, and allow researchers to correct for potential errors. Read more: What are Cross-Sectional Studies: Examples, Definition, Types Methods of Experimental ControlHere are some methods used to achieve control in experimental research
Control groups are required for controlled experiments. Control groups will allow the researcher to run a test on fake treatment, and comparable treatment. It will also compare the result of the comparison with the researcher’s experimental treatment. The results will allow the researcher to understand if the treatment administered caused the outcome or if other factors such as time, or others are involved and whether they would have yielded the same effects. For an example of a control group experiment, a researcher conducting an experiment on the effects of colors in advertising, asked all the participants to come individually to a lab. In this lab, environmental conditions are kept the same all through the research. For the researcher to determine the effect of colors in advertising, each of the participants is placed in either of the two groups: the control group or the experimental group. In the control group, the advertisement color is yellow to represent the clothing industry while blue is given as the advertisement color to the experimental group to represent the clothing industry also. The only difference in these two groups will be the color of the advertisement, other variables will be similar.
Masking occurs in an experiment when the researcher hides condition assignments from the participants. If it’s double-blind research, both the researcher and the participants will be in the dark. Masking or blinding is mostly used in clinical studies to test new treatments. Masking as a control measure takes place because sometimes, researchers may unintentionally influence the participants to act in ways that support their hypotheses. In another scenario, the goal of the study might be revealed to the participants through the study environment and this may influence their responses. Masking, however, blinds the participants from having a deeper knowledge of the research whether they’re in the control group or the experimental group. This helps to control and reduce biases from either the researcher or the participants that could influence the results of the study.
Random assignment or distribution is used to avoid systematic differences between participants in the experimental group and the control group. This helps to evenly distribute extraneous participant variables, thereby making the comparison between groups valid. Another usefulness of random assignment is that it shows the difference between true experiments from quasi-experiments. Learn About: Double-Blind Studies in Research: Types, Pros & Cons How to Design a Controlled ExperimentFor a researcher to design a controlled experiment, the researcher will need:
Then, when the researcher is designing the experiment, he or she must decide on:
How you design your experimental control is highly significant to your experiment’s external and internal validity. Controlled Experiment Examples1. A good example of a controlled group would be an experiment to test the effects of a drug. The sample population would be divided into two, the group receiving the drug would be the experimental group while the group receiving the placebo would be the control group (Note that all the variables such as age, and sex, will be the same). The only significant difference between the two groups will be the taking of medication. You can determine if the drug is effective or not if the control group and experimental group show similar results. 2. Let’s take a look at this example too. If a researcher wants to determine the impact of different soil types on the germination period of seeds, the researcher can proceed to set up four different pots. Each of the pots would be filled with a different type of soil and then seeds can be planted on the soil. After which each soil pot will be watered and exposed to sunlight. The researcher will start to measure how long it took for the seeds to sprout in each of the different soil types. Control measures for this experiment might be to place some seeds in a pot without filling the pot with soil. The reason behind this control measure is to determine that no other factor is responsible for germination except the soil. Here, the researcher can also control the amount of sun the seeds are exposed to, or how much water they are given. The aim is to eliminate all other variables that can affect how quickly the seeds sprouted. Experimental controls are important, but it is also important to note that not all experiments should be controlled and It is still possible to get useful data from experiments that are not controlled. Explore: 21 Chrome Extensions for Academic Researchers in 2021 Problems with Controlled ExperimentsIt is true that the best way to test for cause and effect relationships is by conducting controlled experiments. However, controlled experiments also have some challenges. Some of which are:
Control Group vs an Experimental GroupThere is a thin line between the control group and the experimental group. That line is the treatment condition. As we have earlier established, the experimental group is the one that gets the treatment while the control group is the placebo group. All controlled experiments require control groups because control groups will allow you to compare treatments, and to test if there is no treatment while you compare the result with your experimental treatment. Therefore, both the experimental group and the control group are required to conduct a controlled experiment FAQs about Controlled Experiments
The control group is different from the control condition. However, the control condition is administered to the control group.
The negative control is the group where no change or response is expected while the positive control is the group that receives the treatment with a certainty of a positive result. While the controlled experiment is beneficial to eliminate extraneous variables in research and focus on the independent variable only to cause an effect on the dependent variable. Researchers should be careful so they don’t lose real-life relatability to too controlled experiments and also, not all experiments should be controlled. ![]() Connect to Formplus, Get Started Now - It's Free!
![]() You may also like: How to do a Meta Analysis: Methodology, Pros & ConsIn this article, we’ll go through the concept of meta-analysis, what it can be used for, and how you can use it to improve how you... ![]() Extraneous Variables Explained: Types & ExamplesIn this article, we are going to discuss extraneous variables and how they impact research. Descriptive Research Designs: Types, Examples & MethodsUltimate guide to Descriptive research. Definitions, designs, types, examples and methodology. Lurking Variables Explained: Types & ExamplesIn this article, we’ll discuss what a lurking variable means, the several types available, its effects along with some real-life examples Formplus - For Seamless Data CollectionCollect data the right way with a versatile data collection tool. try formplus and transform your work productivity today..
Randomized Controlled TrialWhat is a randomized controlled trial. A Randomized Controlled Trial (RCT) is a scientific study that evaluates the effectiveness of an intervention by randomly assigning participants from an eligible population into either a treatment group that receives the intervention or a control group that does not. The Basic IdeaImagine that you wanted to know how effective an antidepressant is. While you could give the medication to an entire group of people who experience depression, it would be difficult to accurately measure its effectiveness because you wouldn’t be able to compare the results with a group of people who hadn’t taken the medication. As such, you may design a clinical experiment where one group of people receive the antidepressant, and the other group—the control group—would not. In a randomized controlled trial, participants are randomly selected to be in the treatment (or experimental) group or the control group to avoid selection bias. By comparing the outcomes of the treatment group to the control group, you would be able to assess the effectiveness of the antidepressant more accurately. RandomizationThe hope in a randomized controlled trial is that the only significant difference between the people in the control and treatment groups is whether they receive the treatment or intervention being studied. Due to the random allocation of people into the groups, participant characteristics such as age, race, and gender are usually balanced, which allows researchers to attribute any difference in outcome to the intervention. There are a few different ways to randomize participants: 1
Randomization is done with the aim of balancing known and unknown confounders across groups, but it's not guaranteed that all characteristics will be perfectly balanced, especially in smaller trials. Randomized controlled trials are the most rigorous way of determining whether a cause-effect relation exists between treatment and outcome and for assessing the cost effectiveness of a treatment. – Bonnie Sibbald and Martin Roland, researchers for the National Primary Care Research and Development Centre at the University of Manchester, in their 1998 paper Understanding controlled trials: Why are randomised controlled trials important? 2 Control group : A group of participants that do not receive the intervention that is being studied, whose outcomes are compared to a treatment group Treatment group : A group of participants that receives the intervention that is being studied. Selection bias : A flaw in a research study where there is bias in the sample selection process, such as allocating people into a control or treatment group. For example, if researchers ask people to volunteer for each group, it could lead people with specific characteristics to volunteer and skew the outcomes. 3 Single-blind experimen t: An experimental design in which the participants do not know whether they are receiving the treatment or a placebo, but the researchers do. Double-blind experiment : An experimental design where both the participants and the researchers are unaware as to what condition each of the participants are in. Placebo : A faux substance or intervention used in a blind experiment so that participants are unaware if they are receiving the intervention. Using a placebo in an experiment allows researchers to identify whether outcomes are psychological effects of thinking someone received treatment or actually due to receiving the intervention. Power (Statistical Power) : ensuring that you have enough people in both the control and treatment groups to see a statistical association between treatment and outcome. If the effect of a treatment is minimal, you will require a larger sample size to infer correlation. Confounding Variable : an extraneous variable that is not appropriately controlled in a study. The presence of a confounding variable can create a false impression of a cause-and-effect relationship between treatment and outcome. For example, if a researcher is trying to see if exercise leads to weight loss, a confounding variable would be diet. Response bias : our tendency to provide inaccurate, or even false, answers to self-report questions, such as those asked on surveys or in structured interviews. It’s difficult to pinpoint exactly when randomized controlled trials began, but James Lind, a Scottish physician, is often credited as the first person to conduct a controlled trial in 1754. During the eighteenth century, the British were involved in the War of Austrian Succession against France and Spain, with many men at sea in battle. At this time, more sailors were dying of scurvy than actually dying in combat. People didn’t know what was causing the illness, but Lind was determined to find out. During a voyage, Lind divided 12 sick sailors into six different pairs, and provided them each with different interventions. Notably, one pair received oranges and lemons—and they were the only sailors whose health improved. From this experiment, Lind concluded that citrus fruit helped to cure scurvy. 4 While Lind’s sample size looks nothing like today’s clinical trials, and we can’t be sure if the pairs were randomly assigned, it was one of the first times that various conditions (including a control condition) were used in testing the effectiveness of an intervention. Throughout the 19th century, the use of control groups became more popular, but researchers were not yet aware of the importance of randomizing the groups. Randomized controlled trials, as we know them today, began to take shape in the mid-20th century. The British Medical Research Council’s experiment using streptomycin as a treatment for pulmonary tuberculosis (1948) is often cited as the first real randomized controlled trial. In this study, patients with pulmonary tuberculosis were randomly assigned to either a control group, that would only be treated through bed rest (at the time, the current standard treatment for pulmonary tuberculosis), or a treatment group, where they would receive streptomycin and bed rest. The researchers had no prior knowledge as to who would receive which treatment until they were given an envelope right before seeing a patient, and the patients were unaware that they were in a trial, minimizing the influence of bias (and maximizing the unethicalness ). The researchers were more accurately able to draw a relationship between receiving streptomycin and improved health outcomes. With each group being made up of around 55 patients, only four patients in the treatment group died within six months of receiving treatment compared to 15 that were assigned only to bed rest. 5 After the 1948 experiment, randomized controlled trials gained popularity and quickly became the gold-standard in most fields, especially in medical research. James Lind 6A Scottish physician who is credited with conducting the first randomized controlled trial in 1754, where he studied the effects of six different interventions to cure scurvy in sailors. He found that eating citrus fruit helped to diminish symptoms of scurvy. Throughout his career, Lind continued to advocate for the health of seamen, outlining best hygiene practices and environmental factors that would lead to better health when at sea. Archie Cochrane 7Often referred to as the father of evidence-based medicine, Cochrane was a Scottish medical researcher who believed there was a lack of scientific evidence backing medical interventions in the 20th century. He conducted one of the first clinical trials in 1941, exploring how yeast could reduce starvation in prisoners. 8 Throughout his career, Cochrane was a strong advocate for the need for proper assessment of reliable evidence in medical care. Austin Bradford Hill 9A British scientist who began his career in economics but later transitioned into medical research, Hill pioneered the randomization component of randomized controlled trials. He proposed the randomization of subjects into treatment and control groups in the British Medical Research Council’s 1948 study on the efficacy of streptomycin in treating pulmonary tuberculosis. This trial set the standard for future control trials. Later, Hill worked with Richard Doll to demonstrate the causal relationship between smoking and lung cancer. ConsequencesRandomized controlled trials are considered the gold standard in clinical research and are required by regulatory bodies, such as the The Food and Drug Administration, to approve new treatments for the market. They are effective as they help to isolate the effects of an intervention and more accurately identify a cause-and-effect relationship. While other study designs can find causal associations between an intervention and outcome, they cannot rule out that there may be other factors influencing these outcomes. Randomized controlled trials provide greater command over variables, ensuring that the study is measuring what it is meant to be measuring. Moreover, randomized controlled trials help to minimize participant and researcher bias. Through the random allocation of participants into the treatment and control groups, the study avoids selection bias, minimizing the influence of demographic information on outcomes. Randomized controlled trials that are double-blind further minimize bias, as neither participant or researcher knows who is receiving the intervention. This allows researchers to know if outcomes are due to an intervention or due to the placebo effect , where participants report outcomes because they psychologically feel better, believing they have received treatment. While we usually discuss randomized controlled trials in relation to clinical research, they are also valued in other fields. A notable example is the Perry Preschool Project, where American psychologist David Weikart examined how the intervention of high-quality early childhood education would affect the future potential of low-income, barriered children. Weikart randomly divided 123 children into either the intervention group or the control group and monitored outcomes from 1962 to 1967, finding that early, high-quality intervention led to positive outcomes in education, economic performance, crime prevention, health, family, and children. 10 Today, randomized controlled trials have transformed medical research, behavioral interventions, and policy-making, helping researchers make evidence-based conclusions about the efficacy of an intervention. ControversiesWhile randomized controlled trials are known as the gold-standard in medical and health research, there are still a few drawbacks. If researchers use simple or block randomization, groups may not have balanced characteristics, skewing the results. Additionally, randomized controlled trials require large sample sizes, long durations, and substantial financial resources to conduct effectively. Some interventions may require years to discover significant outcomes or results—and it’s never guaranteed. Additionally, since randomized controlled trials are longitudinal, sometimes taking years, researchers are likely to lose participants along the way. 11 The use of a control group that does not receive treatment also comes with some potential issues. If the experiment is not blind, and some participants know they are not receiving the intervention, it is more difficult to get them to continue to share their outcomes. They may lose interest in participating in the study because they do not feel like they are benefitting from it. Those that do continue to participate could fall victim to response bias —where people provide inaccurate or false answers to self-report questions—especially if they are part of the treatment group and want to share positive results. One way to combat this is through double-blind experiments, with neither researcher or patient knowing whether an individual is receiving the treatment or placebo. This also negates the impact of the demand characteristic bias, where participants change their behavior to fit the outcome they think the experiment is looking for. It is also argued that withholding an intervention from a group that would benefit from it—like medication that can help to cure a disease—is unethical. Ideally, all people suffering from the disease would be able to access medication, but to ensure that the medication is effective, randomized controlled trials can be necessary. Being able to more accurately tie an intervention to a positive outcome, as other factors are mitigated through randomization, researchers are more confident in the effectiveness of an intervention, which could help hundreds more down the line. Randomized Controlled Trials and Charitable Giving 12Charities and non-profit organizations have to compete against one another for donor resources. It is therefore important that these organizations leverage behavioral insights to understand how best to attract and retain donors. In 2013, the Zurich Community Trust conducted a study to see how the framing of a donation ask influenced the likelihood that someone would donate. The Zurich Community Trust randomly divided 702 of their existing donors into one of three treatment groups. The first group—the control group—received a message that asked them to make a one-off increase in their donations with the usual options of £1, £2, £3, £5, or £10. Group two received a message that invited them to increase their donations annually with the same amount options as group one. Group three received a message that invited them to increase their donations annually by £2, £4, £6, £8, or £10. The Zurich Community Trust found that participants in the control group, who were asked for one-off donations, increased their donations by more than those who were offered the chance to increase donations annually. From this study, the trust could infer that asking donors year over year to increase their donations would result in greater donations rather than asking them from the start to commit to an annual increase. Using Randomized Controlled Trials to Test the Effectiveness of Community Therapy 13Randomized controlled trials are often used in behavioral science to study the effectiveness of an intervention. Depression and anxiety place a significant burden on health services, however, it can be challenging to convince individuals to seek out professional help. This may be due to the stigma around receiving mental health support, which may be why a community setting could motivate more people to get treatment. In 2018, researchers in Scotland conducted a study to determine if community-based cognitive behavioral therapy (CBT) had a positive effect on depression and anxiety. Participants were allocated to two groups: one that received treatment immediately, and another that would receive treatment a few months later. The researchers monitored the feelings of anxiety and depression in both groups—comparing the results of the group that had already started attending community therapy groups to those that hadn’t. They found that CBT classes within a community setting were effective for reducing depression and anxiety. To make the study stronger and determine that it was the community aspect of therapy that led to positive outcomes, the researchers could have compared a treatment group to another group of participants receiving individual therapy. However, allowing both groups to receive the intervention at different times allowed for more people to receive the help they needed. Related TDL ContentHow behavioral science informs policy making. In this podcast episode, we sat down with Dr. Sarah Ball, a former policymaker, to learn more about how behavioral science and randomized controlled trials influence policymaking. Dr. Ball questions the heavy push and promotion of randomized controlled trials in assessing interventions, which can influence the projects that behavioral scientists work on. How personalized text messages increased fine repayments by 30%People often fail to pay their overdue court fines, requiring the need for bailiff interventions that can be timely and costly. In this article, we explore a randomized controlled trial conducted by the Courts Service in the UK, to see if sending personalized text messages to people who owed court fines would push them to pay their fines without needing to send a bailiff.
Case studiesFrom insight to impact: our success stories, is there a problem we can help with, about the author. ![]() Emilie Rose JonesEmilie currently works in Marketing & Communications for a non-profit organization based in Toronto, Ontario. She completed her Masters of English Literature at UBC in 2021, where she focused on Indigenous and Canadian Literature. Emilie has a passion for writing and behavioural psychology and is always looking for opportunities to make knowledge more accessible. We are the leading applied research & innovation consultancyOur insights are leveraged by the most ambitious organizations. ![]() I was blown away with their application and translation of behavioral science into practice. They took a very complex ecosystem and created a series of interventions using an innovative mix of the latest research and creative client co-creation. I was so impressed at the final product they created, which was hugely comprehensive despite the large scope of the client being of the world's most far-reaching and best known consumer brands. I'm excited to see what we can create together in the future. Heather McKee BEHAVIORAL SCIENTIST GLOBAL COFFEEHOUSE CHAIN PROJECT OUR CLIENT SUCCESSAnnual revenue increase. By launching a behavioral science practice at the core of the organization, we helped one of the largest insurers in North America realize $30M increase in annual revenue . Increase in Monthly UsersBy redesigning North America's first national digital platform for mental health, we achieved a 52% lift in monthly users and an 83% improvement on clinical assessment. Reduction In Design TimeBy designing a new process and getting buy-in from the C-Suite team, we helped one of the largest smartphone manufacturers in the world reduce software design time by 75% . Reduction in Client Drop-OffBy implementing targeted nudges based on proactive interventions, we reduced drop-off rates for 450,000 clients belonging to USA's oldest debt consolidation organizations by 46% ![]() Group Conformity![]() Synthetic Population![]() Eager to learn about how behavioral science can help your organization?Get new behavioral science insights in your inbox every month.. Advertisement Lab-grown stem cells could be a 'breakthrough' for cancer treatmentStem cells made in the lab may one day aid cancer treatment by reducing our reliance on donors By James Woodford 2 September 2024 ![]() Stem cells are produced by bone marrow and can turn into different types of blood cells KATERYNA KON / SPL/ Alamy Human blood stem cells have been made in a laboratory for the first time, which could significantly improve how we treat certain types of cancer . The lab-grown cells have so far only been tested in mice, but when infused into the animals, the cells became functional bone marrow at similar levels to those seen after umbilical cord blood cell transplants. A new understanding of how your blood type influences your health Treating cancers such as leukaemia and lymphoma via radiation and chemotherapy can destroy the blood-forming cells in bone marrow. A stem cell transplant means that new, healthy bone marrow and blood cells can grow. Umbilical cords are a particularly rich source of stem cells, but donations are limited and the transplant can be rejected by the body. The new method would allow researchers to produce stem cells from the actual patient, eliminating the supply issue and reducing the risk that their body would reject them. First, human blood or skin cells were turned into so-called pluripotent stem cells through a process called reprogramming. “This involves temporarily turning on four genes, with the result that the patient cells revert to an early stage of development when they can become any cell in the body,” says Andrew Elefanty at the Murdoch Children’s Research Institute in Melbourne. Sign up to our Health Check newsletterGet the most essential health and fitness news in your inbox every Saturday. The second stage involved turning the pluripotent cells into blood stem cells. “We first make thousands of small floating balls of cells, a few hundred cells in each ball, and direct them to change from being stem cells to sequentially become blood vessels and then blood cells,” says Elefanty. This process, called differentiation, takes about two weeks and makes millions of blood cells, he says. These cells were then infused into mice that lacked an immune system and became functional bone marrow in up to 50 per cent of cases. This means it made the same cells that carry oxygen and fight infections as healthy human bone marrow does, says Elefanty. “It is this unique ability to make all the blood cell types for a prolonged period of time that defines the cells as blood stem cells,” he says. ![]() We are finally improving prostate cancer diagnoses – here's how Cases of prostate cancer are surging alarmingly around the world. Thankfully, we are developing more accurate tests that can catch the condition early Abbas Shafiee at the University of Queensland in Brisbane says the work is a “magnificent breakthrough” towards new therapies for blood cancers. “It has not been done before and it has a lot of potential for the future.” But even once animal testing is complete, a lot of research in humans needs to be done before the approach can be used in clinics, he says. Simon Conn at Flinders University in Adelaide, Australia, says a key advantage of the team’s approach is that it could be scaled up to produce “an essentially never-ending supply” of blood stem cells. But he adds that the rate of success and the diversity of the blood cells varied. “This suggests the treatment, even at the preclinical stage in mice, is not consistent, which will need to be addressed prior to any clinical trials in human patients,” he says. Journal reference: Nature Biotechnology DOI: 10.1038/s41587-024-02360-7
Sign up to our weekly newsletterReceive a weekly dose of discovery in your inbox! We'll also keep you up to date with New Scientist events and special offers. More from New ScientistExplore the latest news, articles and features ![]() Stem cells grown in labs for experimental therapies pose a cancer riskSubscriber-only ![]() Wiping stem cells 'clean' could make them easier to produce![]() Genetically modified stem cells extend lifespan of mice by 20 per cent![]() Stem cells treat diabetes without triggering immune response in micePopular articles. Trending New Scientist articles Experimental Method In PsychologySaul McLeod, PhD Editor-in-Chief for Simply Psychology BSc (Hons) Psychology, MRes, PhD, University of Manchester Saul McLeod, PhD., is a qualified psychology teacher with over 18 years of experience in further and higher education. He has been published in peer-reviewed journals, including the Journal of Clinical Psychology. Learn about our Editorial Process Olivia Guy-Evans, MSc Associate Editor for Simply Psychology BSc (Hons) Psychology, MSc Psychology of Education Olivia Guy-Evans is a writer and associate editor for Simply Psychology. She has previously worked in healthcare and educational sectors. On This Page: The experimental method involves the manipulation of variables to establish cause-and-effect relationships. The key features are controlled methods and the random allocation of participants into controlled and experimental groups . What is an Experiment?An experiment is an investigation in which a hypothesis is scientifically tested. An independent variable (the cause) is manipulated in an experiment, and the dependent variable (the effect) is measured; any extraneous variables are controlled. An advantage is that experiments should be objective. The researcher’s views and opinions should not affect a study’s results. This is good as it makes the data more valid and less biased. There are three types of experiments you need to know: 1. Lab ExperimentA laboratory experiment in psychology is a research method in which the experimenter manipulates one or more independent variables and measures the effects on the dependent variable under controlled conditions. A laboratory experiment is conducted under highly controlled conditions (not necessarily a laboratory) where accurate measurements are possible. The researcher uses a standardized procedure to determine where the experiment will take place, at what time, with which participants, and in what circumstances. Participants are randomly allocated to each independent variable group. Examples are Milgram’s experiment on obedience and Loftus and Palmer’s car crash study .
2. Field ExperimentA field experiment is a research method in psychology that takes place in a natural, real-world setting. It is similar to a laboratory experiment in that the experimenter manipulates one or more independent variables and measures the effects on the dependent variable. However, in a field experiment, the participants are unaware they are being studied, and the experimenter has less control over the extraneous variables . Field experiments are often used to study social phenomena, such as altruism, obedience, and persuasion. They are also used to test the effectiveness of interventions in real-world settings, such as educational programs and public health campaigns. An example is Holfing’s hospital study on obedience .
3. Natural ExperimentA natural experiment in psychology is a research method in which the experimenter observes the effects of a naturally occurring event or situation on the dependent variable without manipulating any variables. Natural experiments are conducted in the day (i.e., real life) environment of the participants, but here, the experimenter has no control over the independent variable as it occurs naturally in real life. Natural experiments are often used to study psychological phenomena that would be difficult or unethical to study in a laboratory setting, such as the effects of natural disasters, policy changes, or social movements. For example, Hodges and Tizard’s attachment research (1989) compared the long-term development of children who have been adopted, fostered, or returned to their mothers with a control group of children who had spent all their lives in their biological families. Here is a fictional example of a natural experiment in psychology: Researchers might compare academic achievement rates among students born before and after a major policy change that increased funding for education. In this case, the independent variable is the timing of the policy change, and the dependent variable is academic achievement. The researchers would not be able to manipulate the independent variable, but they could observe its effects on the dependent variable.
Key TerminologyEcological validity. The degree to which an investigation represents real-life experiences. Experimenter effectsThese are the ways that the experimenter can accidentally influence the participant through their appearance or behavior. Demand characteristicsThe clues in an experiment lead the participants to think they know what the researcher is looking for (e.g., the experimenter’s body language). Independent variable (IV)The variable the experimenter manipulates (i.e., changes) is assumed to have a direct effect on the dependent variable. Dependent variable (DV)Variable the experimenter measures. This is the outcome (i.e., the result) of a study. Extraneous variables (EV)All variables which are not independent variables but could affect the results (DV) of the experiment. EVs should be controlled where possible. Confounding variablesVariable(s) that have affected the results (DV), apart from the IV. A confounding variable could be an extraneous variable that has not been controlled. Random AllocationRandomly allocating participants to independent variable conditions means that all participants should have an equal chance of participating in each condition. The principle of random allocation is to avoid bias in how the experiment is carried out and limit the effects of participant variables. Order effectsChanges in participants’ performance due to their repeating the same or similar test more than once. Examples of order effects include: (i) practice effect: an improvement in performance on a task due to repetition, for example, because of familiarity with the task; (ii) fatigue effect: a decrease in performance of a task due to repetition, for example, because of boredom or tiredness. ![]()
![]()
Your support helps make our show possible and unlocks access to our sponsor-free feed. Want to see a cool trick? Make a tiny battery with these 3 household items![]() Emily Kwong ![]() Rebecca Ramirez Madeline K. Sofia ![]() Electrical circuit can be created with lemons to power a small light source. A chemical reaction between the copper and zinc plates and the citric acid produces a small current, thus powering a light bulb. Andriy Onufriyenko/Getty Images hide caption Electrical circuit can be created with lemons to power a small light source. A chemical reaction between the copper and zinc plates and the citric acid produces a small current, thus powering a light bulb. We're going "Back to School" today, revisiting a classic at-home experiment that turns lemons into batteries — powerful enough to turn on a clock or a small lightbulb. But how does the science driving the "lemon battery" show up in those household batteries we use daily? We get into just that today with environmental engineer Jenelle Fortunato about the fundamentals of electric currents and the inner workings of batteries. You can build your very own lemon battery using Science U's design here , written by Fortunato and Christopher Gorski of Penn State College of Engineering. A reminder: Do NOT play with household batteries. Be safe out there, scientists! Want us to cover more science basics? Email us your ideas at [email protected] — we might feature them on a future episode! Listen to every episode of Short Wave sponsor-free and support our work at NPR by signing up for Short Wave+ at plus.npr.org/shortwave . Listen to Short Wave on Spotify , Apple Podcasts and Google Podcasts . This episode was originally produced by Rebecca Ramirez and edited by Viet Le. The encore version was produced and edited by Rebecca Ramirez.
Information
InitiativesYou are accessing a machine-readable page. In order to be human-readable, please install an RSS reader. All articles published by MDPI are made immediately available worldwide under an open access license. No special permission is required to reuse all or part of the article published by MDPI, including figures and tables. For articles published under an open access Creative Common CC BY license, any part of the article may be reused without permission provided that the original article is clearly cited. For more information, please refer to https://www.mdpi.com/openaccess . Feature papers represent the most advanced research with significant potential for high impact in the field. A Feature Paper should be a substantial original Article that involves several techniques or approaches, provides an outlook for future research directions and describes possible research applications. Feature papers are submitted upon individual invitation or recommendation by the scientific editors and must receive positive feedback from the reviewers. Editor’s Choice articles are based on recommendations by the scientific editors of MDPI journals from around the world. Editors select a small number of articles recently published in the journal that they believe will be particularly interesting to readers, or important in the respective research area. The aim is to provide a snapshot of some of the most exciting work published in the various research areas of the journal. Original Submission Date Received: .
![]() Article Menu![]()
Find support for a specific problem in the support section of our website. Please let us know what you think of our products and services. Visit our dedicated information section to learn more about MDPI. JSmol ViewerEnhanced second-order rc equivalent circuit model with hybrid offline–online parameter identification for accurate soc estimation in electric vehicles under varying temperature conditions. ![]() 1. Introduction
2. Establish an Improved Lithium-Ion Battery Model2.1. obtaining the external characteristic curve of the battery, 2.2. optimized equivalent circuit model, 3. acquisition of battery characteristic parameters, 3.1. offline parameter acquisition, 3.2. online parameter acquisition, 3.3. offline combined with online parameter acquisition, 4. simulation and comparison, 5. experiment validation, 6. conclusions, author contributions, data availability statement, conflicts of interest.
Click here to enlarge figure
Share and CiteZhou, H.; He, Q.; Li, Y.; Wang, Y.; Wang, D.; Xie, Y. Enhanced Second-Order RC Equivalent Circuit Model with Hybrid Offline–Online Parameter Identification for Accurate SoC Estimation in Electric Vehicles under Varying Temperature Conditions. Energies 2024 , 17 , 4397. https://doi.org/10.3390/en17174397 Zhou H, He Q, Li Y, Wang Y, Wang D, Xie Y. Enhanced Second-Order RC Equivalent Circuit Model with Hybrid Offline–Online Parameter Identification for Accurate SoC Estimation in Electric Vehicles under Varying Temperature Conditions. Energies . 2024; 17(17):4397. https://doi.org/10.3390/en17174397 Zhou, Hao, Qiaoling He, Yichuan Li, Yangjun Wang, Dongsheng Wang, and Yongliang Xie. 2024. "Enhanced Second-Order RC Equivalent Circuit Model with Hybrid Offline–Online Parameter Identification for Accurate SoC Estimation in Electric Vehicles under Varying Temperature Conditions" Energies 17, no. 17: 4397. https://doi.org/10.3390/en17174397 Article MetricsArticle access statistics, further information, mdpi initiatives, follow mdpi. ![]() Subscribe to receive issue release notifications and newsletters from MDPI journals Immuno-pathogenesis study of local infectious bronchitis virus G1-1 lineage variant showed altered tissue tropism in experimental broiler chickens
Cite this article
Infectious bronchitis (IB) is an acute contagious disease of poultry caused by infectious bronchitis virus (IBV). This study investigated the immunopathogenesis and tissue tropism of an Indian IBV field isolate (IBV/Chicken/India/IVRI/Rajasthan/01/2023) in experimental broiler chickens. This isolate belongs to the G1-1 lineage and is closely associated with the Mass genotype. 10 6.23 EID 50 /0.2 mL of the virus was administered intranasally and intraocularly to the IBV-challenge group, whereas uninoculated allantoic fluid was administered to the control group. Clinical signs, gross and histopathological lesions, immunohistochemistry (IHC), viral load, humoral responses, and the relative expression of immune response genes were evaluated at seven observation points. The infected group showed a significant reduction in weight gain from 3 dpi onwards, with clinical signs of varying severity from 3 to − 11 dpi. Gross lesions and microscopic changes were observed in the nasal turbinates, trachea, lungs, and kidneys, mainly representing epithelial degeneration and necrosis with mononuclear infiltrates. The caecal tonsils also showed microscopic lesions at 7–9 dpi. Absolute viral load estimation in the organs corroborated the lesion severity scores and IHC results. The expression of innate immune responses broadly demonstrated higher expression in the trachea and lungs of the IBV-infected group during the early phase of infection, whereas similar responses were observed in the kidneys and caecal tonsils during the later phases of infection. This study suggests that the given IBV isolate may cause significant production losses in broilers and exhibit tissue tropism for both respiratory and non-respiratory tissues, triggering varying innate and adaptive immune responses. This is a preview of subscription content, log in via an institution to check access. Access this articleSubscribe and save.
Price excludes VAT (USA) Tax calculation will be finalised during checkout. Instant access to the full article PDF. Rent this article via DeepDyve Institutional subscriptions ![]() Data availabilityNo datasets were generated or analysed during the current study. Abdel-Moneim AS, Zlotowski P, Veits J, Keil GM, Teifke JP (2009) Immunohistochemistry for detection of avian infectious bronchitis virus strain M41 in the proventriculus and nervous system of experimentally infected chicken embryos. Virol J 6:1–7. https://doi.org/10.1186/1743-422X-6-15 Article CAS Google Scholar Adzhar A, Shaw K, Britton P, Cavanagh D (1996) Universal oligonucleotides for the detection of infectious bronchitis virus by the polymerase chain reaction. Avian Pathol 25:817–836. https://doi.org/10.1080/03079459608419184 Article PubMed CAS Google Scholar Alvarado IR, Villegas P, El-Attrache J, Brown TP (2003) Evaluation of the protection conferred by commercial vaccines against the California 99 isolate of infectious bronchitis virus. Avian Dis 47:1298–1304. https://doi.org/10.1637/6040 Amarasinghe A, De Silva Senapathi U, Abdul-Cader MS, Popowich S, Marshall F, Cork SC, van der Meer F, Gomis S, Abdul-Careem MF (2018) Comparative features of infections of two Massachusetts (Mass) infectious bronchitis virus (IBV) variants isolated from western Canadian layer flocks. BMC Vet Res 14:1–12. https://doi.org/10.1186/s12917-018-1720-9 Ariaans MP, van de Haar PM, Hensen EJ, Vervelde L (2009) Infectious bronchitis virus induces acute interferon-gamma production through polyclonal stimulation of chicken leukocytes. Virol 385:68–73. https://doi.org/10.1016/j.virol.2008.11.021 Awad F, Hutton S, Forrester A, Baylis M, Ganapathy K (2016) Heterologous live infectious bronchitis virus vaccination in day-old commercial broiler chicks: clinical signs, ciliary health, immune responses and protection against variant infectious bronchitis viruses. Avian Pathol 45:169–177. https://doi.org/10.1080/03079457.2015.1137866 Bande F, Arshad SS, Omar AR, Hair-Bejo M, Mahmuda A, Nair V (2017) Global distributions and strain diversity of avian infectious bronchitis virus: a review. Anim Health Res Rev 18:70–83. https://doi.org/10.1017/S1466252317000044 Article PubMed Google Scholar Benyeda Z, Szeredi L, Mató T, Süveges T, Balka G, Abonyi-Tóth Z, Rusvai M, Palya V (2010) Comparative histopathology and immunohistochemistry of QX-like, Massachusetts and 793/B serotypes of infectious bronchitis virus infection in chickens. J Comp Pathol 143:276–283. https://doi.org/10.1016/j.jcpa.2010.04.007 Article PubMed PubMed Central Google Scholar Boroomand Z, Asasi K, Mohammadi A (2012) Pathogenesis and tissue distribution of avian infectious bronchitis virus isolate IRFIBV32 (793/B serotype) in experimentally infected broiler chickens. Sci World J 2012:402537. https://doi.org/10.1100/2012/402537 Callison SA, Hilt DA, Boynton TO, Sample BF, Robison R, Swayne DE, Jackwood MW (2006) Development and evaluation of a real-time Taqman RT-PCR assay for the detection of infectious bronchitis virus from infected chickens. J Virol Methods 138:60–65. https://doi.org/10.1016/j.jviromet.2006.07.018 Article PubMed PubMed Central CAS Google Scholar Cavanagh D (2003) Severe acute respiratory syndrome vaccine development: experiences of vaccination against avian infectious bronchitis coronavirus. Avian Pathol 32:567–582. https://doi.org/10.1080/03079450310001621198 Chen BY, Hosi S, Nunoya T, Itakura C (1996) Histopathology and immunohistochemistry of renal lesions due to infectious bronchitis virus in chicks. Avian Pathol 25:269–283. https://doi.org/10.1080/03079459608419141 Chhabra R, Ball C, Chantrey J, Ganapathy K (2015) Immune responses to virulent and vaccine strains of infectious bronchitis viruses in chickens. Viral Immunol 28:478–488. https://doi.org/10.1089/vim.2015.0027 Chhabra R, Ball C, Chantrey J, Ganapathy K (2018) Differential innate immune responses induced by classical and variant infectious bronchitis viruses in specific pathogen free chicks. Dev Comp Immunol 87:16–23. https://doi.org/10.1016/j.dci.2018.04.026 Cong F, Liu X, Han Z, Shao Y, Kong X, Liu S (2013) Transcriptome analysis of chicken kidney tissues following coronavirus avian infectious bronchitis virus infection. BMC Genomics 14:743. https://doi.org/10.1186/1471-2164-14-743 Cook JK, Orbell SJ, Woods MA, Huggins MB (1999) Breadth of protection of the respiratory tract provided by different live-attenuated infectious bronchitis vaccines against challenge with infectious bronchitis viruses of heterologous serotypes. Avian Pathol 28:477–485. https://doi.org/10.1080/03079459994506 Emikpe BO, Ohore OG, Olujonwo M, Akpavie SO (2010) Prevalence of antibodies to infectious bronchitis virus (IBV) in chickens in southwestern Nigeria. Afr J Microbiol Res 4:092–095 Google Scholar Gelb J Jr, Nix WA, Gellman SD (1998) Infectious bronchitis virus antibodies in tears and their relationship to immunity. Avian Dis 42:364–374. https://doi.org/10.2307/1592487 Ghadakchi H, Dadras H, Pourbakhsh SA, Hosseini SMH (2005) Standardization of an enzyme-linked immunosorbent assay for detection of infectious bronchitis virus antibody. Arch Razi Inst 59:75–83 CAS Google Scholar Grgić H, Hunter DB, Hunton P, Nagy E (2008) Pathogenicity of infectious bronchitis virus isolates from Ontario chickens. Can J Vet Res 72:403–410 PubMed Google Scholar Hassan MSH, Buharideen SM, Ali A, Najimudeen SM, Goldsmith D, Coffin CS, Cork SC, van der Meer F, Abdul-Careem MF (2022) Efficacy of commercial infectious bronchitis vaccines against Canadian delmarva (DMV/1639) infectious bronchitis virus infection in layers. Vaccines 10:1194. https://doi.org/10.3390/vaccines10081194 He Y, Xie Z, Dai J, Cao Y, Hou J, Zheng Y, Wei T, Mo M, Wei P (2016) Responses of the toll-like receptor and melanoma differentiation-associated protein 5 signaling pathways to avian infectious bronchitis virus infection in chicks. Virol Sin 31:57–68. https://doi.org/10.1007/s12250-015-3696-y Jackwood MW, de Wit S (2013) Infectious bronchitis. In: Swayne DE, Glisson JR, McDougald LR, Nolan LK, Suarez DL, Nair V (eds) Diseases of poultry, 13th edn. Wiley, Chichester, UK, pp 139–159 Chapter Google Scholar Jang H, Koo BS, Jeon EO, Lee HR, Lee SM, Mo IP (2013) Altered pro-inflammatory cytokine mRNA levels in chickens infected with infectious bronchitis virus. Poult Sci 92:2290–2298. https://doi.org/10.3382/ps.2013-03116 Jiang L, Zhao W, Han Z, Chen Y, Zhao Y, Sun J, Li H, Shao Y, Liu L, Liu S (2017) Genome characterization, antigenicity and pathogenicity of a novel infectious bronchitis virus type isolated from south China. Infect Genet Evol 54:437–446. https://doi.org/10.1016/j.meegid.2017.08.006 Jones RM, Ellis RJ, Cox WJ, Errington J, Fuller C, Irvine RM, Wakeley PR (2011) Development and validation of RT-PCR tests for the detection and S1 genotyping of infectious bronchitis virus and other closely related gammacoronaviruses within clinical samples. Transbound Emerg Dis 58:411–420. https://doi.org/10.1111/j.1865-1682.2011.01222.x Kameka AM, Haddadi S, Kim DS, Cork SC, Abdul-Careem MF (2014) Induction of innate immune response following infectious bronchitis corona virus infection in the respiratory tract of chickens. Virology 450:114–121. https://doi.org/10.1016/j.virol.2013.12.001 Kannaki TR, Venkatesh Yadav J, Priyanka E, Lakshman M (2021) Pathology, tissue tropism and antibody response of nephropathogenic infectious bronchitis virus (IBV) Indian isolate in experimentally infected chicken. VirusDis 32:568–575. https://doi.org/10.1007/s13337-021-00693-4 Kint J, Fernandez-Gutierrez M, Maier HJ, Britton P, Langereis MA, Koumans J, Wiegertjes GF, Forlenza M (2015) Activation of the chicken type I interferon response by infectious bronchitis coronavirus. J Virol 89:1156–1167. https://doi.org/10.1128/JVI.02671-14 Kotani T, Wada S, Tsukamoto Y, Kuwamura M, Yamate J, Sakuma S (2000) Kinetics of lymphocytic subsets in chicken tracheal lesions infected with infectious bronchitis virus. J Vet Med Sci 62:397–401. https://doi.org/10.1292/jvms.62.397 Lee CC, Wu CC, Lin TL (2012) Characterization of chicken melanoma differentiation-associated gene 5 (MDA5) from alternative translation initiation. Comp Immunol Microbiol Infect Dis 35:335–343. https://doi.org/10.1016/j.cimid.2012.02.004 Lin SY, Chen HW (2017) Infectious bronchitis virus variants: molecular analysis and pathogenicity investigation. Int J Mol Sci 18:2030. https://doi.org/10.3390/ijms18102030 Livak KJ, Schmittgen TD (2001) Analysis of relative gene expression data using real-time quantitative PCR and the 2(-Delta Delta C(T)) method. Methods 25:402–408. https://doi.org/10.1006/meth.2001.1262 Lucio B, Fabricant J (1990) Tissue tropism of three cloacal isolates and Massachusetts strain of infectious bronchitis virus. Avian Dis 34:865–870. https://doi.org/10.2307/1591375 Luna LG (1968) Manual of histologic staining methods of the Armed Forces Institute of Pathology. McGraw-Hill Ma T, Xu L, Ren M, Shen J, Han Z, Sun J, Zhao Y, Liu S (2019) Novel genotype of infectious bronchitis virus isolated in China. Vet Microbiol 230:178–186. https://doi.org/10.1016/j.vetmic.2019.01.020 Manswr B, Ball C, Forrester A, Chantrey J, Ganapathy K (2020) Immunopathogenesis of infectious bronchitis virus Q1 in specific pathogen free chicks. Microb Pathog 149:104535. https://doi.org/10.1016/j.micpath.2020.104535 Mohammadi A, Asasi K, Boroomand Z, Namazi F, Hosseinian SA (2015) Viral quantity and pathological changes in broilers experimentally infected by IRFIBV32 isolate of infectious bronchitis virus. VirusDis 26:319–323. https://doi.org/10.1007/s13337-015-0286-4 Article Google Scholar Najafi H, Ghalyanchi Langeroudi A, Hashemzadeh M, Karimi V, Madadgar O, Khaltabadi Farahani R, Ghafouri SA, Maghsoudloo H, Seifouri P, Madhi A (2017) Pathogenicity study of Iranian genotype of avian infectious bronchitis virus (IR-1). Vet Res Forum 8:35–41 PubMed PubMed Central Google Scholar Najimudeen SM, Barboza-Solis C, Ali A, Buharideen SM, Isham M, Hassan I, Ojkic MSH, Van Marle D, Cork G, van der Meer SC, Boulianne F, Abdul-Careem M MF (2022) Pathogenesis and host responses in lungs and kidneys following Canadian 4/91 infectious bronchitis virus (IBV) infection in chickens. Virology 566:75–88. https://doi.org/10.1016/j.virol.2021.11.013 Nakamura K, Cook JK, Otsuki K, Huggins MB, Frazier JA (1991) Comparative study of respiratory lesions in two chicken lines of different susceptibility infected with infectious bronchitis virus: histology, ultrastructure and immunohistochemistry. Avian Pathol 20:241–257. https://doi.org/10.1080/03079459108418761 Okino CH, Santos IL, Fernando FS, Alessi AC, Wang X, Montassier HJ (2014) Inflammatory and cell-mediated immune responses in the respiratory tract of chickens to infection with avian infectious bronchitis virus. Viral Immunol 27:383–391. https://doi.org/10.1089/vim.2014.0054 Okino CH, Mores MA, Trevisol IM, Coldebella A, Montassier HJ, Brentano L (2017) Early immune responses and development of pathogenesis of avian infectious bronchitis viruses with different virulence profiles. PLoS ONE 12:e0172275. https://doi.org/10.1371/journal.pone.0172275 Owen RL, Cowen BS, Hattel AL, Naqi SA, Wilson RA (1991) Detection of viral antigen following exposure of one-day-old chickens to the Holland 52 strain of infectious bronchitis virus. Avian Pathol 20:663–673. https://doi.org/10.1080/03079459108418805 Pensaert M, Lambrechts C (1994) Vaccination of chickens against a Belgian nephropathogenic strain of infectious bronchitis virus B1648 using attenuated homologous and heterologous strains. Avian Pathol 23:631–641. https://doi.org/10.1080/03079459408419033 Raja A, Dhinakar Raj G, Kumanan K (2020) Emergence of variant avian infectious bronchitis virus in India. Iran J Vet Res 21:33–39 PubMed PubMed Central CAS Google Scholar Ramakrishnan S, Annamalai A, Sachan S, Kumar A, Sharma BK, Govindaraj E, Chellappa MM, Dey S, Krishnaswamy N (2015) Synergy of lipopolysaccharide and resiquimod on type I interferon, pro-inflammatory cytokine, Th1 and Th2 response in chicken peripheral blood mononuclear cells. Mol Immunol 64:177–182. https://doi.org/10.1016/j.molimm.2014.11.013 Rasoli M, Yeap SK, Tan SW, Roohani K, Kristeen-Teo YW, Alitheen NB, Rahaman YA, Aini I, Bejo MH, Kaiser P, Omar AR (2015) Differential modulation of immune response and cytokine profiles in the bursae and spleen of chickens infected with very virulent infectious bursal disease virus. BMC Vet Res 11:1–14. https://doi.org/10.1186/s12917-015-0377-x Reed LJ, Muench H (1938) A simple method of estimating fifty per cent endpoints. Am J Epidemiol 27:493–497. https://doi.org/10.1093/oxfordjournals.aje.a118408 Schoggins JW, Wilson SJ, Panis M, Murphy MY, Jones CT, Bieniasz P, Rice CM (2011) A diverse range of gene products are effectors of the type I interferon antiviral response. Nature 472:481–485. https://doi.org/10.1038/nature09907 St Paul M, Paolucci S, Barjesteh N, Wood RD, Sharif S (2013) Chicken erythrocytes respond to toll-like receptor ligands by up-regulating cytokine transcripts. Res Vet Sci 95:87–91. https://doi.org/10.1016/j.rvsc.2013.01.024 Takaoka A, Yanai H (2006) Interferon signalling network in innate defence. Cell Microbiol 8:907–922. https://doi.org/10.1111/j.1462-5822.2006.00716.x Valastro V, Holmes EC, Britton P, Fusaro A, Jackwood MW, Cattoli G, Monne I (2016) S1 gene-based phylogeny of infectious bronchitis virus: an attempt to harmonize virus classification. Infect Genet Evol 39:349–364. https://doi.org/10.1016/j.meegid.2016.02.015 Wu G, Liu L, Qi Y, Sun Y, Yang N, Xu G, Zhou H, Li X (2015) Splenic gene expression profiling in White Leghorn layer inoculated with the Salmonella enterica serovar Enteritidis. Anim Genet 46:617–626. https://doi.org/10.1111/age.12341 Yang X, Li J, Liu H, Zhang P, Chen D, Men S, Li X, Wang H (2018) Induction of innate immune response following introduction of infectious bronchitis virus (IBV) in the trachea and renal tissues of chickens. Microb Pathog 116:54–61. https://doi.org/10.1016/j.micpath.2018.01.008 Zhang X, Deng T, Lu J, Zhao P, Chen L, Qian M, Guo Y, Qiao H, Xu Y, Wang Y, Li X, Zhang G, Wang Z, Bian C (2020) Molecular characterization of variant infectious bronchitis virus in China, 2019: implications for control programmes. Transbound Emerg Dis 67:1349–1355. https://doi.org/10.1111/tbed.13477 Zhou J, Chu H, Li C, Wong BH, Cheng ZS, Poon VK, Sun T, Lau CC, Wong KK, Chan JY, Chan JF, To KK, Chan KH, Zheng BJ, Yuen KY (2014) Active replication of Middle East respiratory syndrome coronavirus and aberrant induction of inflammatory cytokines and chemokines in human macrophages: implications for pathogenesis. J Infect Dis 209:1331–1342. https://doi.org/10.1093/infdis/jit504 Zhou H, Zhang M, Tian X, Shao H, Qian K, Ye J, Qin A (2017) Identification of a novel recombinant virulent avian infectious bronchitis virus. Vet Microbiol 199:120–127. https://doi.org/10.1016/j.vetmic.2016.12.038 Download references AcknowledgementsThis work was supported by the research fund of Indian Council of Agricultural Research– Consortium Research Platform on Vaccines and Diagnostics (CRP on V&D), India under grant F. No, 14 − 2/PME/ICAR-CRP/14–15/500. We gratefully acknowledge the Director, Indian Council of Agricultural Research-Indian Veterinary Research Institute (ICAR- IVRI), Izatnagar, India. Author informationAuthors and affiliations. Division of Pathology, Indian Council of Agricultural Research (ICAR)-Indian Veterinary Research Institute, Izatnagar, Bareilly, Uttar Pradesh, 243 122, India Megha Sharma, Faslu A. T. Rahman, Kuldeep Dhama, G. Saikumar & Asok M. Kumar CADRAD, Indian Council of Agricultural Research (ICAR)-Indian Veterinary Research Institute, Izatnagar, Bareilly, Uttar Pradesh, 243 122, India Gaurav Sharma Division of Veterinary Biotechnology, Indian Council of Agricultural Research (ICAR)-Indian Veterinary Research Institute, Izatnagar, Bareilly, Uttar Pradesh, 243 122, India Sohini Dey & Madhan Mohan Chellappa Division of Pharmacology and Toxicology, Indian Council of Agricultural Research (ICAR)-Indian Veterinary Research Institute, Izatnagar, Bareilly, Uttar Pradesh, 243 122, India Anshuk Sharma Division of Pathology, ICAR-Indian Veterinary Research Institute, Izatnagar, Bareilly, Uttar Pradesh, 243 122, India Asok M. Kumar You can also search for this author in PubMed Google Scholar ContributionsM.S. - Investigation; Methodology; Writing - original draft; Data curation; Formal analysis; F. R. - Investigation; Methodology; Data curation; Formal analysis; Writing - review & editing; G.S. - Methodology; Data curation; Formal analysis; Resources; Software; Validation; S.D. - Methodology; Data curation; Formal analysis; Resources; Software; Validation; M.M. - Methodology; Data curation; Formal analysis; Resources; Software; Validation; A.S. - Methodology; Data curation; Formal analysis; Software; Validation; K.D. - Methodology; Data curation; Formal analysis; Resources; Software; Validation; S.K. - Methodology; Data curation; Formal analysis; Resources; Software; Validation; Funding acquisition; Supervision; A.K.- Conceptualization; Data curation; Formal analysis; Funding acquisition; Investigation; Methodology; Project administration; Resources; Supervision; Validation; Visualization; Writing - review & editing. Corresponding authorCorrespondence to Asok M. Kumar . Ethics declarationsCompeting interests. The authors declare no competing interests. Disclosure statementThe authors declare that they have no competing interests. Additional informationPublisher’s note. Springer Nature remains neutral with regard to jurisdictional claims in published maps and institutional affiliations. Supplementary InformationSupplementary material 1, rights and permissions. Springer Nature or its licensor (e.g. a society or other partner) holds exclusive rights to this article under a publishing agreement with the author(s) or other rightsholder(s); author self-archiving of the accepted manuscript version of this article is solely governed by the terms of such publishing agreement and applicable law. Reprints and permissions About this articleSharma, M., Rahman, F.A., Sharma, G. et al. Immuno-pathogenesis study of local infectious bronchitis virus G1-1 lineage variant showed altered tissue tropism in experimental broiler chickens. Vet Res Commun (2024). https://doi.org/10.1007/s11259-024-10525-7 Download citation Received : 05 July 2024 Accepted : 30 August 2024 Published : 02 September 2024 DOI : https://doi.org/10.1007/s11259-024-10525-7 Share this articleAnyone you share the following link with will be able to read this content: Sorry, a shareable link is not currently available for this article. Provided by the Springer Nature SharedIt content-sharing initiative
![]() |
IMAGES
VIDEO
COMMENTS
An experimental control is used in scientific experiments to minimize the effect of variables which are not the interest of the study. The control can be an object, population, or any other variable which a scientist would like to "control." ... A control is important for an experiment because it allows the experiment to minimize the changes in ...
Random assignment is a hallmark of a "true experiment"—it differentiates true experiments from quasi-experiments. Example: Random assignment To divide your sample into groups, you assign a unique number to each participant. You use a computer program to randomly place each number into either a control group or an experimental group.
In an experiment, the control is a standard or baseline group not exposed to the experimental treatment or manipulation.It serves as a comparison group to the experimental group, which does receive the treatment or manipulation. The control group helps to account for other variables that might influence the outcome, allowing researchers to attribute differences in results more confidently to ...
A true experiment (a.k.a. a controlled experiment) always includes at least one control group that doesn't receive the experimental treatment.. However, some experiments use a within-subjects design to test treatments without a control group. In these designs, you usually compare one group's outcomes before and after a treatment (instead of comparing outcomes between different groups).
Controls also help to account for errors and variability in the experimental setup and measuring tools: The negative control of an enzyme assay, for instance, tests for any unrelated background signals from the assay or measurement. In short, controls are essential for the unbiased, objective observation and measurement of the dependent ...
A controlled experiment is a research study in which participants are randomly assigned to experimental and control groups. A controlled experiment allows researchers to determine cause and effect between variables. One drawback of controlled experiments is that they lack external validity (which means their results may not generalize to real ...
A scientific control is an experiment or observation designed to minimize the effects of variables other than the independent variable (i.e. confounding variables ). [ 1] This increases the reliability of the results, often through a comparison between control measurements and the other measurements. Scientific controls are a part of the ...
An experiment without the proper controls is meaningless. Controls allow the experimenter to minimize the effects of factors other than the one being tested. It's how we know an experiment is testing the thing it claims to be testing. This goes beyond science — controls are necessary for any sort of experimental testing, no matter the ...
The types of groups and method of assigning participants to groups will help you implement control in your experiment. Control groups. Controlled experiments require control groups. Control groups allow you to test a comparable treatment, no treatment, or a fake treatment, and compare the outcome with your experimental treatment.
Experimental design refers to how participants are allocated to different groups in an experiment. Types of design include repeated measures, independent groups, and matched pairs designs. ... The sample would be split into two groups: experimental (A) and control (B). For example, group 1 does 'A' then 'B,' and group 2 does 'B ...
If you're seeing this message, it means we're having trouble loading external resources on our website. If you're behind a web filter, please make sure that the domains *.kastatic.org and *.kasandbox.org are unblocked.
Identifying Variables and Control Groups. Identifying and defining independent, dependent, and control variables is fundamental to experimental planning. Precise identification ensures that the experiment is designed to isolate the effect of the independent variable while controlling for other influential factors.
Table of contents. Step 1: Define your variables. Step 2: Write your hypothesis. Step 3: Design your experimental treatments. Step 4: Assign your subjects to treatment groups. Step 5: Measure your dependent variable. Other interesting articles. Frequently asked questions about experiments.
The control group and experimental group are compared against each other in an experiment. The only difference between the two groups is that the independent variable is changed in the experimental group. The independent variable is "controlled", or held constant, in the control group. A single experiment may include multiple experimental ...
Negative Control. The process of conducting the experiment in the exact same way on a control group except that the independent variables are a placebo that is not expected to produce a result. For example, an experiment on plants where one group of plants are given a fertilizer delivered in a solution and a control group that are given the ...
Controlled Experiment. A controlled experiment is simply an experiment in which all factors are held constant except for one: the independent variable. A common type of controlled experiment compares a control group against an experimental group. All variables are identical between the two groups except for the factor being tested.
A positive control group is an experimental control that will produce a known response or the desired effect. A positive control is used to ensure a test's success and confirm an experiment's validity. For example, when testing for a new medication, an already commercially available medication could serve as the positive control.
In a controlled experiment, the study population is often divided into two groups. One group receives a change in a certain variable, while the other group receives a standard environment and conditions. This group is referred to as the control group, and allows for comparison with the other group, known as the experimental group. Many types of ...
The following are some methods for controlling variables in experimental design: 1. Random Assignment. In experimental research, random assignment is an often-employed method for controlling variables. Its purpose is to minimize bias and ensure that participant characteristics are evenly distributed across all groups.
Developing a control for an experiment depends on the independent variables being tested. When testing new medication, the control group doesn't receive it. If testing the effect of sunlight on the growth of a flower, the control group of flowers might be grown inside and away from the sun. Here are the steps to take when performing an ...
A controlled experiment is defined as an experiment in which all the variable factors in an experimental group and a comparison control group are kept the same except for one variable factor in ...
Research. Controlled Experiments: Methods, Examples & Limitations. What happens in experimental research is that the researcher alters the independent variables so as to determine their impacts on the dependent variables. Therefore, when the experiment is controlled, you can expect that the researcher will control all other variables except for ...
As such, you may design a clinical experiment where one group of people receive the antidepressant, and the other group—the control group—would not. In a randomized controlled trial, participants are randomly selected to be in the treatment (or experimental) group or the control group to avoid selection bias.
Human blood stem cells have been made in a laboratory for the first time, which could significantly improve how we treat certain types of cancer.. The lab-grown cells have so far only been tested ...
This article presents an experimental study that evaluated droop control strategies in DC microgrids with parallel-connected converters. In a decentralized control scheme, it is critical to ensure voltage regulation and load sharing in each converter to maintain a stable operation. Two scenarios are considered: the first involves two converters operating in parallel as voltage mode control, a ...
There are three types of experiments you need to know: 1. Lab Experiment. A laboratory experiment in psychology is a research method in which the experimenter manipulates one or more independent variables and measures the effects on the dependent variable under controlled conditions. A laboratory experiment is conducted under highly controlled ...
The researchers investigated the control of hole delamination and hole tapering through the optimization of parameters using response surface methodology (RSM) and multi-objective genetic algorithm (MOGA) techniques. ... the study conducted an experiment to examine the CO 2 laser cutting process of a 4-mm-thick sheet of glass fiber-reinforced ...
Just in time for the return of the school year, we're going "Back To School" by revisiting a classic at-home experiment that turns lemons into batteries — powerful enough to turn on a clock or a ...
Accurate estimation of State-of-Charge (SoC) is essential for ensuring the safe and efficient operation of electric vehicles (EVs). Currently, second-order RC equivalent circuit models do not account for the influence of battery charging and discharging states on battery parameters. Additionally, offline parameter identification becomes inaccurate as the battery ages. Online identification ...
At 1 dpi, no difference was observed in the antibody titres between the control and infected groups. However, the antibody titres were markedly higher in the infected group at 3 (p < 0.01) and at 5, 7, 9, 11, and 14 (p < 0.001) dpi, as compared to the control. Furthermore, antibody titres differed significantly in the infected group at all dpi.