Random Assignment in Psychology: Definition & Examples
Julia Simkus
Editor at Simply Psychology
BA (Hons) Psychology, Princeton University
Julia Simkus is a graduate of Princeton University with a Bachelor of Arts in Psychology. She is currently studying for a Master's Degree in Counseling for Mental Health and Wellness in September 2023. Julia's research has been published in peer reviewed journals.
Learn about our Editorial Process
Saul Mcleod, PhD
Editor-in-Chief for Simply Psychology
BSc (Hons) Psychology, MRes, PhD, University of Manchester
Saul Mcleod, PhD., is a qualified psychology teacher with over 18 years of experience in further and higher education. He has been published in peer-reviewed journals, including the Journal of Clinical Psychology.
Olivia Guy-Evans, MSc
Associate Editor for Simply Psychology
BSc (Hons) Psychology, MSc Psychology of Education
Olivia Guy-Evans is a writer and associate editor for Simply Psychology. She has previously worked in healthcare and educational sectors.
In psychology, random assignment refers to the practice of allocating participants to different experimental groups in a study in a completely unbiased way, ensuring each participant has an equal chance of being assigned to any group.
In experimental research, random assignment, or random placement, organizes participants from your sample into different groups using randomization.
Random assignment uses chance procedures to ensure that each participant has an equal opportunity of being assigned to either a control or experimental group.
The control group does not receive the treatment in question, whereas the experimental group does receive the treatment.
When using random assignment, neither the researcher nor the participant can choose the group to which the participant is assigned. This ensures that any differences between and within the groups are not systematic at the onset of the study.
In a study to test the success of a weight-loss program, investigators randomly assigned a pool of participants to one of two groups.
Group A participants participated in the weight-loss program for 10 weeks and took a class where they learned about the benefits of healthy eating and exercise.
Group B participants read a 200-page book that explains the benefits of weight loss. The investigator randomly assigned participants to one of the two groups.
The researchers found that those who participated in the program and took the class were more likely to lose weight than those in the other group that received only the book.
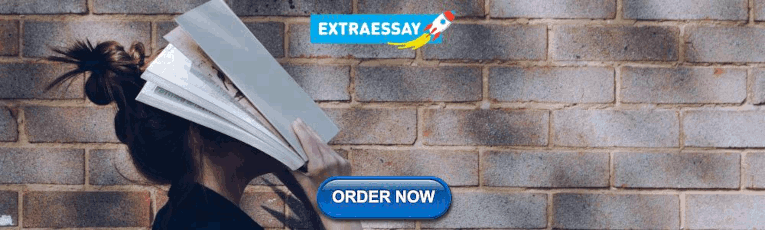
Importance
Random assignment ensures that each group in the experiment is identical before applying the independent variable.
In experiments , researchers will manipulate an independent variable to assess its effect on a dependent variable, while controlling for other variables. Random assignment increases the likelihood that the treatment groups are the same at the onset of a study.
Thus, any changes that result from the independent variable can be assumed to be a result of the treatment of interest. This is particularly important for eliminating sources of bias and strengthening the internal validity of an experiment.
Random assignment is the best method for inferring a causal relationship between a treatment and an outcome.
Random Selection vs. Random Assignment
Random selection (also called probability sampling or random sampling) is a way of randomly selecting members of a population to be included in your study.
On the other hand, random assignment is a way of sorting the sample participants into control and treatment groups.
Random selection ensures that everyone in the population has an equal chance of being selected for the study. Once the pool of participants has been chosen, experimenters use random assignment to assign participants into groups.
Random assignment is only used in between-subjects experimental designs, while random selection can be used in a variety of study designs.
Random Assignment vs Random Sampling
Random sampling refers to selecting participants from a population so that each individual has an equal chance of being chosen. This method enhances the representativeness of the sample.
Random assignment, on the other hand, is used in experimental designs once participants are selected. It involves allocating these participants to different experimental groups or conditions randomly.
This helps ensure that any differences in results across groups are due to manipulating the independent variable, not preexisting differences among participants.
When to Use Random Assignment
Random assignment is used in experiments with a between-groups or independent measures design.
In these research designs, researchers will manipulate an independent variable to assess its effect on a dependent variable, while controlling for other variables.
There is usually a control group and one or more experimental groups. Random assignment helps ensure that the groups are comparable at the onset of the study.
How to Use Random Assignment
There are a variety of ways to assign participants into study groups randomly. Here are a handful of popular methods:
- Random Number Generator : Give each member of the sample a unique number; use a computer program to randomly generate a number from the list for each group.
- Lottery : Give each member of the sample a unique number. Place all numbers in a hat or bucket and draw numbers at random for each group.
- Flipping a Coin : Flip a coin for each participant to decide if they will be in the control group or experimental group (this method can only be used when you have just two groups)
- Roll a Die : For each number on the list, roll a dice to decide which of the groups they will be in. For example, assume that rolling 1, 2, or 3 places them in a control group and rolling 3, 4, 5 lands them in an experimental group.
When is Random Assignment not used?
- When it is not ethically permissible: Randomization is only ethical if the researcher has no evidence that one treatment is superior to the other or that one treatment might have harmful side effects.
- When answering non-causal questions : If the researcher is just interested in predicting the probability of an event, the causal relationship between the variables is not important and observational designs would be more suitable than random assignment.
- When studying the effect of variables that cannot be manipulated: Some risk factors cannot be manipulated and so it would not make any sense to study them in a randomized trial. For example, we cannot randomly assign participants into categories based on age, gender, or genetic factors.
Drawbacks of Random Assignment
While randomization assures an unbiased assignment of participants to groups, it does not guarantee the equality of these groups. There could still be extraneous variables that differ between groups or group differences that arise from chance. Additionally, there is still an element of luck with random assignments.
Thus, researchers can not produce perfectly equal groups for each specific study. Differences between the treatment group and control group might still exist, and the results of a randomized trial may sometimes be wrong, but this is absolutely okay.
Scientific evidence is a long and continuous process, and the groups will tend to be equal in the long run when data is aggregated in a meta-analysis.
Additionally, external validity (i.e., the extent to which the researcher can use the results of the study to generalize to the larger population) is compromised with random assignment.
Random assignment is challenging to implement outside of controlled laboratory conditions and might not represent what would happen in the real world at the population level.
Random assignment can also be more costly than simple observational studies, where an investigator is just observing events without intervening with the population.
Randomization also can be time-consuming and challenging, especially when participants refuse to receive the assigned treatment or do not adhere to recommendations.
What is the difference between random sampling and random assignment?
Random sampling refers to randomly selecting a sample of participants from a population. Random assignment refers to randomly assigning participants to treatment groups from the selected sample.
Does random assignment increase internal validity?
Yes, random assignment ensures that there are no systematic differences between the participants in each group, enhancing the study’s internal validity .
Does random assignment reduce sampling error?
Yes, with random assignment, participants have an equal chance of being assigned to either a control group or an experimental group, resulting in a sample that is, in theory, representative of the population.
Random assignment does not completely eliminate sampling error because a sample only approximates the population from which it is drawn. However, random sampling is a way to minimize sampling errors.
When is random assignment not possible?
Random assignment is not possible when the experimenters cannot control the treatment or independent variable.
For example, if you want to compare how men and women perform on a test, you cannot randomly assign subjects to these groups.
Participants are not randomly assigned to different groups in this study, but instead assigned based on their characteristics.
Does random assignment eliminate confounding variables?
Yes, random assignment eliminates the influence of any confounding variables on the treatment because it distributes them at random among the study groups. Randomization invalidates any relationship between a confounding variable and the treatment.
Why is random assignment of participants to treatment conditions in an experiment used?
Random assignment is used to ensure that all groups are comparable at the start of a study. This allows researchers to conclude that the outcomes of the study can be attributed to the intervention at hand and to rule out alternative explanations for study results.
Further Reading
- Bogomolnaia, A., & Moulin, H. (2001). A new solution to the random assignment problem . Journal of Economic theory , 100 (2), 295-328.
- Krause, M. S., & Howard, K. I. (2003). What random assignment does and does not do . Journal of Clinical Psychology , 59 (7), 751-766.

Related Articles
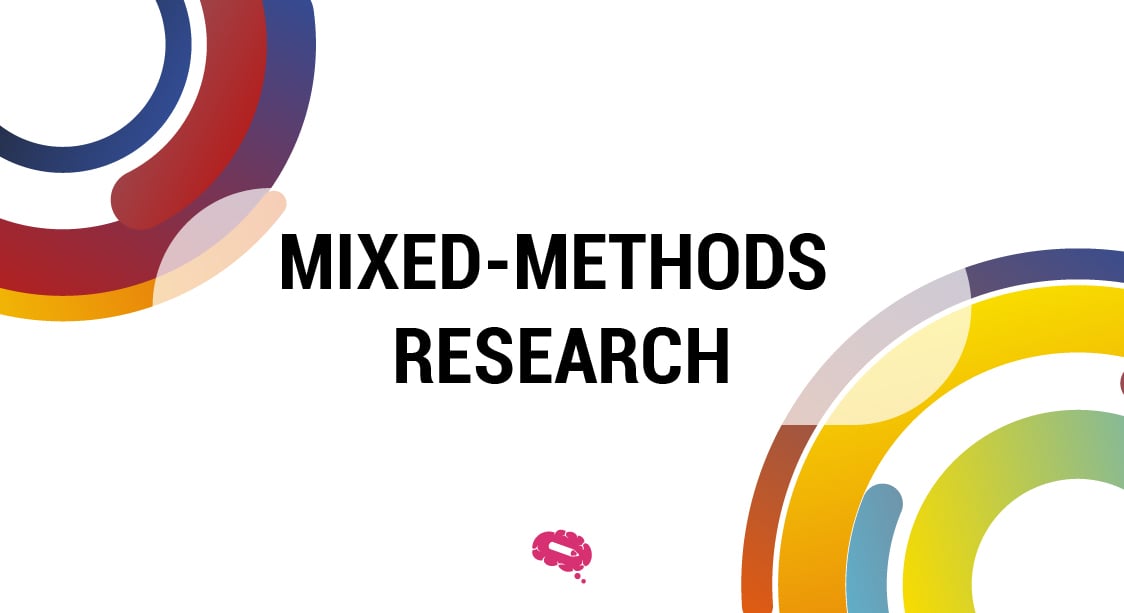
Research Methodology
Mixed Methods Research
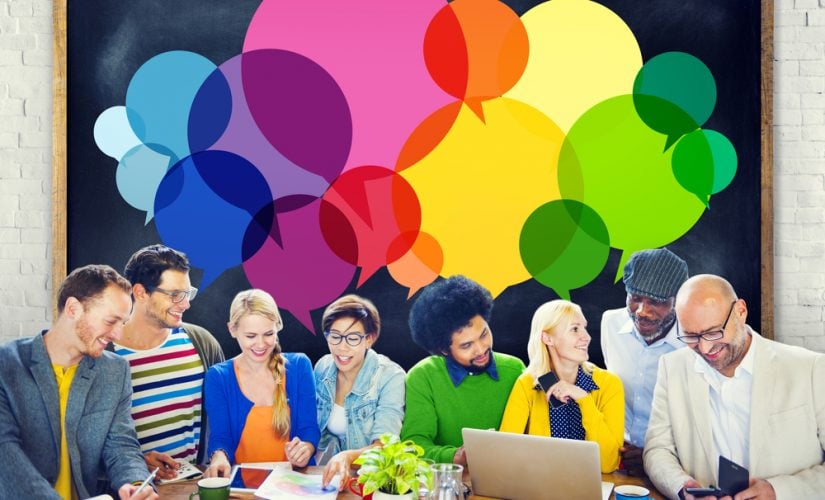
Conversation Analysis
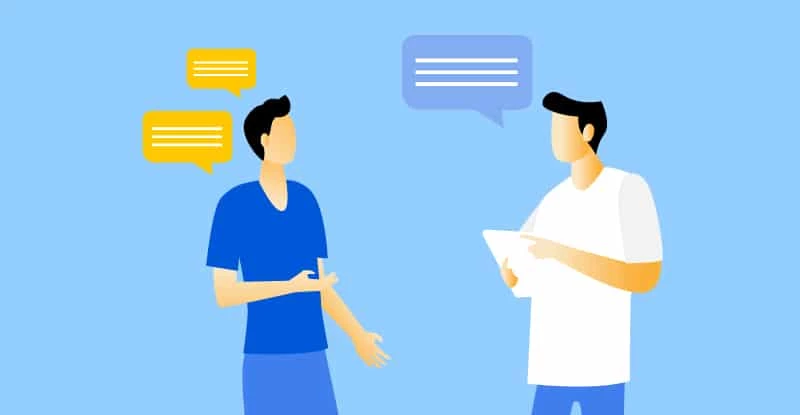
Discourse Analysis
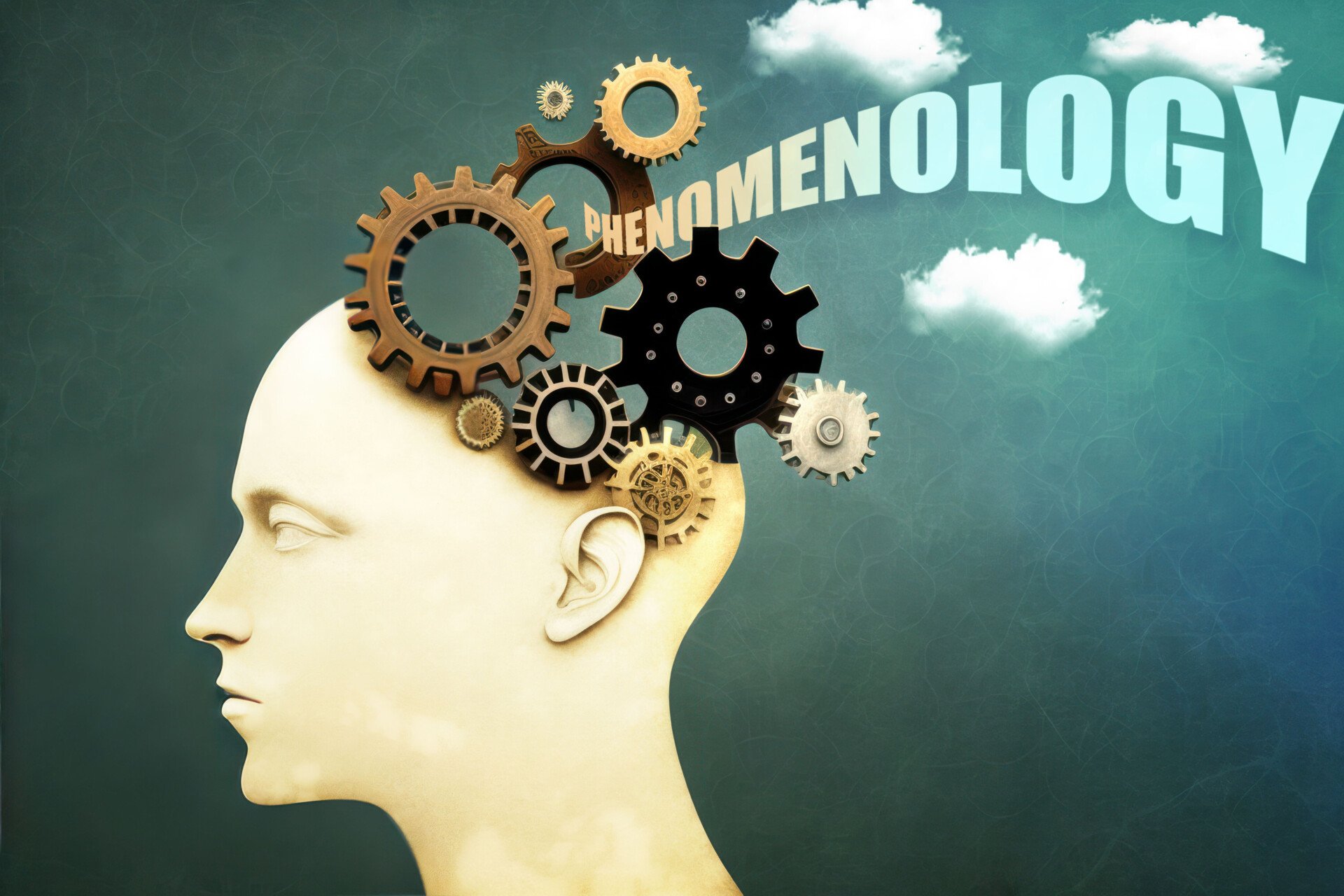
Phenomenology In Qualitative Research
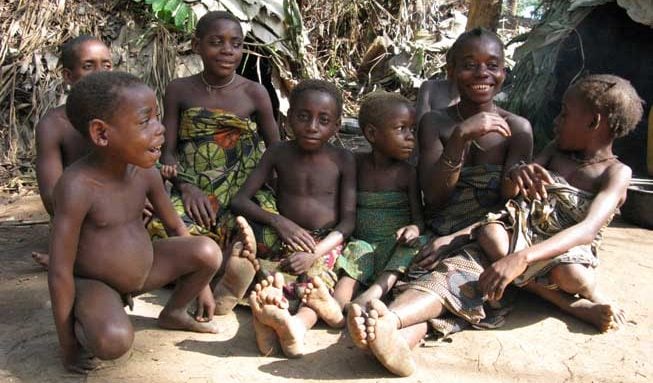
Ethnography In Qualitative Research
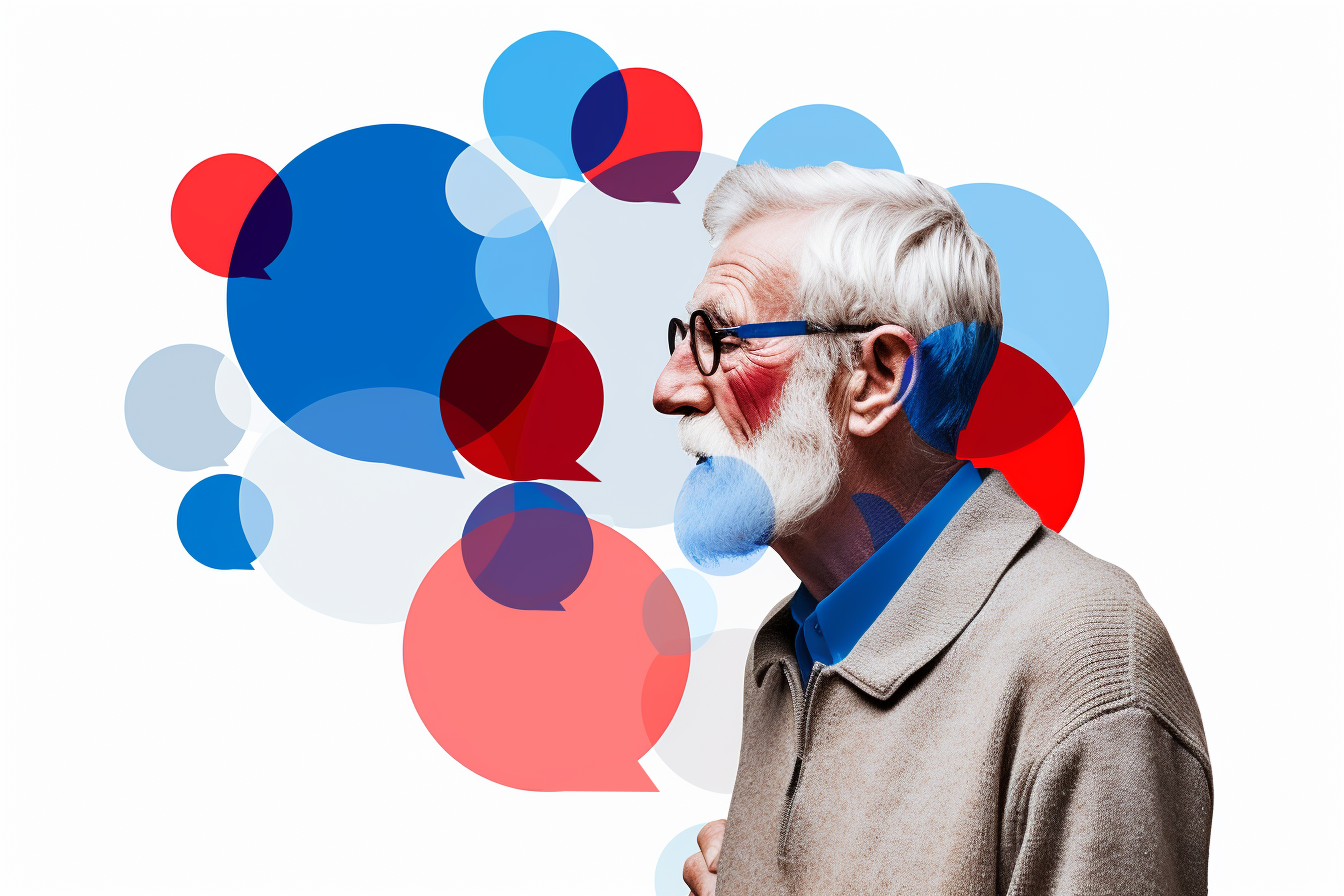
Narrative Analysis In Qualitative Research
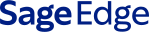
- Request new password
- Create a new account
Political Science Research Methods
Student resources, chapter summary, chapter objectives.
6.1: Distinguish between a causal and spurious relationship. 6.2: Understand the three necessary components in a causal relationship. 6.3: Illustrate how the classical randomized experiment demonstrates how a research design can be used to verify a causal relationship. 6.4: Understand internal and external validity. 6.5: Understand the distinction between qualitative and quantitative research design. 6.6: Understand the difference between effect-of-causes and causes-of-effects approaches to investigating causal relationships.
- All the parts of a research design should work to the same end: drawing sound conclusions supported by observable evidence.
- Spurious relationships arise because two things are both affected by a third factor and this appear to be related.
- When the additional factor has been identified and controlled for, the original relationship weakens or disappears altogether.
- Distinguishing real, causal relations from spurious ones is an important part of scientific research.
- Covariation: the alleged cause varies with the supposed effect.
- Time order: the cause precedes the effect in time.
- Elimination of alternative explanations: the research design should eliminate as many alternative explanations for the observed effect as possible to isolate causation to one factor.
- It begins with a sample.
- A stimulus, or test factor, is a condition applied to participants in an experiment in a way in which the researcher can measure some sort of effect.
- There is at least one experimental group that will have exposure to the treatment and one control group that will not.
- Randomly assigns individuals to each group, avoiding self-selection, and guaranteeing that on average the groups will not differ in any respect.
- Controls the administration of the treatment including the circumstances, under which the experimental group is exposed.
- Establishes and measures a dependent variable before and after the treatment with a pretest and posttest . Because treatment groups are constituted through randomization , any difference between the pre- and posttests can be attributed to the experimental effect of exposure to the treatment.
- Controls the environment of the experiment (time, location, and other physical aspects).
- Events other than the experimental stimulus that occur between the pretest and posttest measurements of the dependent variable.
- Maturation or a change in subjects over time that might produce differences between experiment and control groups.
- Test-subject interaction , the process of measuring the dependent variable prior to the experimental stimulus, may itself affect the posttreatment scores of subjects.
- Selection bias : bias due to the assignment of subjects to experimental and control groups according to some criterion and not randomly.
- Experimental mortality : a differential loss of subjects from experimental and control groups that affects the equivalency of groups.
- Demand characteristics : aspects of the research situation that cause participants to guess at the investigator’s goals and adjust their behavior or opinions accordingly.
- External validity refers to the extent to which the results of an experiment can be generalized across populations, time, and settings.
- The causes-of-effects approach starts with an outcome (e.g., war, election result, passage of a major piece of legislation) and works backward to the causes.
- The effects-of-causes approach starts with a potential cause and works forward to measure its impact on the outcome.
- Qualitative studies are small N , whereas quantitative studies can be small N or large N in scope.
- The classical randomized experiment is an excellent starting point for understanding how a research project can be designed and executed to establish causation through covariation, time order, and eliminate alternative explanations.
|
|
to use a variety of methods in followups? (sometimes called a "natural experiment") and the . is a list of common threats to drawing causal inferences. " neither participants nor the actual administrator (e.g., who hands out the pills) know which treatment participants receive, controlling for everyone's expectations. It can be surprisingly common. (the Federal USA Office of Management and the Budget does Human Subjects review for federal government conducted studies.) |
the groups are different at the very beginning. If we have basic or prior information about those who comprise the individuals or groups in the different treatments we may, at least, try to institute for those variables.
|
even if we assign groups to treatments based on their differences, such as a high ability and low ability group, For example, the high ability group may be more motivated or more confident, on the average, than the low ability group. And it is perhaps those differences in motivation or confidence, instead of the differences in ability (that we originally thought was the true independent variable), that were the true causes of the treatment outcome differences that were observed. |
- Posttest Only, Nonequivalent Control Group Design
- Other types of quasi-experimental designs are also analogous to their experimental counterpasts, such as the Pretest-Postest Design (and additional groups can be added to mimic the Solomon 4 Group).
- A hurricane hits and you assess how well emergency personnel put new training and procedures in place.
- New legislation requires all automobile drivers and passengers to wear seat belts.
- New state legislation mandates new testing for high school students.
- A state eliminates affirmative action considerations in collegiate admissions policies.
- A new therapy is publicized that purports to help autistic children.
- In research several decades ago, Martin Orne found that laboratory subjects would do virtually anything an experimenter asked them to do. They would eat bitter crackers for several minutes, they would throw what they were told was acid at a laboratory assistant, they would pick up snakes or prepare to eat worms.
- Participants may become nervous about being monitored , or exhibit evaluation apprehension . When people become anxious, many things happen. Physiological indicators, such as heart rate or blood pressure, change. If people are slightly anxious, they may do better on tests, performance, or assessments. However if people are very anxious ("flooded") they will almost certainly do worse
- People may try to " fake good ," to appear smarter, more attractive, or more tolerant than they normally are. Paper and pencil questionnaires are especially prone to these effects because often the answers are not checked for their veracity. (And, on online surveys, we may not even correctly know who anyone is .)
- It is not just individuals who have social desirability effects. A century ago, the famous writer Lev Tolstoy wrote about " Potemkin villages ." When the Czar of Russia went on cross-country trips, goverment officials were a little ways ahead of him. In cooperation with local government, they would erect false-fronted buildings (as on a movie set) and the best looking young men and women of the village would stand before these fake structures smiling and throwing flowers. While most groups or organizations will not go to this extent, they may "hide" their more embarassing members, "fudge" or slightly alter records, and claim your procedures were followed when they were not.
- You may need to deceive participants about the true purpose of the study (if you were told the purpose of the study was to measure leadership qualities in sports, might you try to "shape up?")
- Avoid collecting your own data ; don't act as your own experimenter or interviewer. Trade off with another student or apply for a small University or external grant to hire someone to supervise or interview. Try to avoid having your own expectations possibly influence results.
- Don't tell interviewers or experimenters the true purpose of the study and don't tell them (if possible) which participant is in which treatment condition. You might give each person a "generic overview" of the study ("this study is about which movies children like.")
Have a language expert improve your writing
Run a free plagiarism check in 10 minutes, generate accurate citations for free.
- Knowledge Base
Methodology
- Random Assignment in Experiments | Introduction & Examples
Random Assignment in Experiments | Introduction & Examples
Published on March 8, 2021 by Pritha Bhandari . Revised on June 22, 2023.
In experimental research, random assignment is a way of placing participants from your sample into different treatment groups using randomization.
With simple random assignment, every member of the sample has a known or equal chance of being placed in a control group or an experimental group. Studies that use simple random assignment are also called completely randomized designs .
Random assignment is a key part of experimental design . It helps you ensure that all groups are comparable at the start of a study: any differences between them are due to random factors, not research biases like sampling bias or selection bias .
Table of contents
Why does random assignment matter, random sampling vs random assignment, how do you use random assignment, when is random assignment not used, other interesting articles, frequently asked questions about random assignment.
Random assignment is an important part of control in experimental research, because it helps strengthen the internal validity of an experiment and avoid biases.
In experiments, researchers manipulate an independent variable to assess its effect on a dependent variable, while controlling for other variables. To do so, they often use different levels of an independent variable for different groups of participants.
This is called a between-groups or independent measures design.
You use three groups of participants that are each given a different level of the independent variable:
- a control group that’s given a placebo (no dosage, to control for a placebo effect ),
- an experimental group that’s given a low dosage,
- a second experimental group that’s given a high dosage.
Random assignment to helps you make sure that the treatment groups don’t differ in systematic ways at the start of the experiment, as this can seriously affect (and even invalidate) your work.
If you don’t use random assignment, you may not be able to rule out alternative explanations for your results.
- participants recruited from cafes are placed in the control group ,
- participants recruited from local community centers are placed in the low dosage experimental group,
- participants recruited from gyms are placed in the high dosage group.
With this type of assignment, it’s hard to tell whether the participant characteristics are the same across all groups at the start of the study. Gym-users may tend to engage in more healthy behaviors than people who frequent cafes or community centers, and this would introduce a healthy user bias in your study.
Although random assignment helps even out baseline differences between groups, it doesn’t always make them completely equivalent. There may still be extraneous variables that differ between groups, and there will always be some group differences that arise from chance.
Most of the time, the random variation between groups is low, and, therefore, it’s acceptable for further analysis. This is especially true when you have a large sample. In general, you should always use random assignment in experiments when it is ethically possible and makes sense for your study topic.
Prevent plagiarism. Run a free check.
Random sampling and random assignment are both important concepts in research, but it’s important to understand the difference between them.
Random sampling (also called probability sampling or random selection) is a way of selecting members of a population to be included in your study. In contrast, random assignment is a way of sorting the sample participants into control and experimental groups.
While random sampling is used in many types of studies, random assignment is only used in between-subjects experimental designs.
Some studies use both random sampling and random assignment, while others use only one or the other.
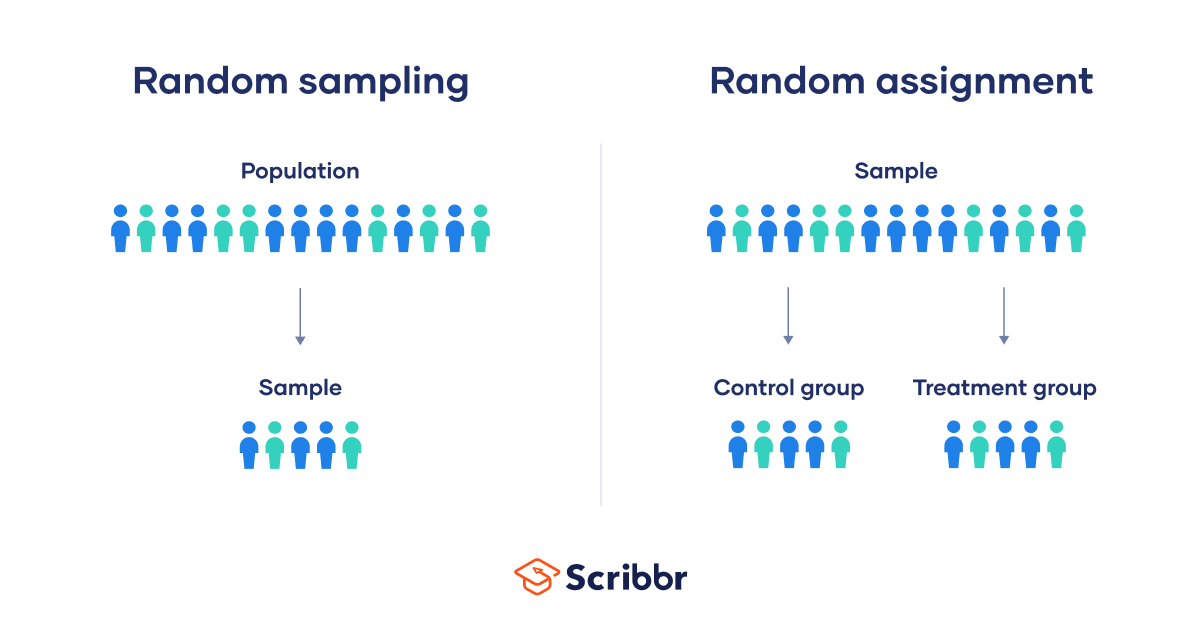
Random sampling enhances the external validity or generalizability of your results, because it helps ensure that your sample is unbiased and representative of the whole population. This allows you to make stronger statistical inferences .
You use a simple random sample to collect data. Because you have access to the whole population (all employees), you can assign all 8000 employees a number and use a random number generator to select 300 employees. These 300 employees are your full sample.
Random assignment enhances the internal validity of the study, because it ensures that there are no systematic differences between the participants in each group. This helps you conclude that the outcomes can be attributed to the independent variable .
- a control group that receives no intervention.
- an experimental group that has a remote team-building intervention every week for a month.
You use random assignment to place participants into the control or experimental group. To do so, you take your list of participants and assign each participant a number. Again, you use a random number generator to place each participant in one of the two groups.
To use simple random assignment, you start by giving every member of the sample a unique number. Then, you can use computer programs or manual methods to randomly assign each participant to a group.
- Random number generator: Use a computer program to generate random numbers from the list for each group.
- Lottery method: Place all numbers individually in a hat or a bucket, and draw numbers at random for each group.
- Flip a coin: When you only have two groups, for each number on the list, flip a coin to decide if they’ll be in the control or the experimental group.
- Use a dice: When you have three groups, for each number on the list, roll a dice to decide which of the groups they will be in. For example, assume that rolling 1 or 2 lands them in a control group; 3 or 4 in an experimental group; and 5 or 6 in a second control or experimental group.
This type of random assignment is the most powerful method of placing participants in conditions, because each individual has an equal chance of being placed in any one of your treatment groups.
Random assignment in block designs
In more complicated experimental designs, random assignment is only used after participants are grouped into blocks based on some characteristic (e.g., test score or demographic variable). These groupings mean that you need a larger sample to achieve high statistical power .
For example, a randomized block design involves placing participants into blocks based on a shared characteristic (e.g., college students versus graduates), and then using random assignment within each block to assign participants to every treatment condition. This helps you assess whether the characteristic affects the outcomes of your treatment.
In an experimental matched design , you use blocking and then match up individual participants from each block based on specific characteristics. Within each matched pair or group, you randomly assign each participant to one of the conditions in the experiment and compare their outcomes.
Sometimes, it’s not relevant or ethical to use simple random assignment, so groups are assigned in a different way.
When comparing different groups
Sometimes, differences between participants are the main focus of a study, for example, when comparing men and women or people with and without health conditions. Participants are not randomly assigned to different groups, but instead assigned based on their characteristics.
In this type of study, the characteristic of interest (e.g., gender) is an independent variable, and the groups differ based on the different levels (e.g., men, women, etc.). All participants are tested the same way, and then their group-level outcomes are compared.
When it’s not ethically permissible
When studying unhealthy or dangerous behaviors, it’s not possible to use random assignment. For example, if you’re studying heavy drinkers and social drinkers, it’s unethical to randomly assign participants to one of the two groups and ask them to drink large amounts of alcohol for your experiment.
When you can’t assign participants to groups, you can also conduct a quasi-experimental study . In a quasi-experiment, you study the outcomes of pre-existing groups who receive treatments that you may not have any control over (e.g., heavy drinkers and social drinkers). These groups aren’t randomly assigned, but may be considered comparable when some other variables (e.g., age or socioeconomic status) are controlled for.
If you want to know more about statistics , methodology , or research bias , make sure to check out some of our other articles with explanations and examples.
- Student’s t -distribution
- Normal distribution
- Null and Alternative Hypotheses
- Chi square tests
- Confidence interval
- Quartiles & Quantiles
- Cluster sampling
- Stratified sampling
- Data cleansing
- Reproducibility vs Replicability
- Peer review
- Prospective cohort study
Research bias
- Implicit bias
- Cognitive bias
- Placebo effect
- Hawthorne effect
- Hindsight bias
- Affect heuristic
- Social desirability bias
In experimental research, random assignment is a way of placing participants from your sample into different groups using randomization. With this method, every member of the sample has a known or equal chance of being placed in a control group or an experimental group.
Random selection, or random sampling , is a way of selecting members of a population for your study’s sample.
In contrast, random assignment is a way of sorting the sample into control and experimental groups.
Random sampling enhances the external validity or generalizability of your results, while random assignment improves the internal validity of your study.
Random assignment is used in experiments with a between-groups or independent measures design. In this research design, there’s usually a control group and one or more experimental groups. Random assignment helps ensure that the groups are comparable.
In general, you should always use random assignment in this type of experimental design when it is ethically possible and makes sense for your study topic.
To implement random assignment , assign a unique number to every member of your study’s sample .
Then, you can use a random number generator or a lottery method to randomly assign each number to a control or experimental group. You can also do so manually, by flipping a coin or rolling a dice to randomly assign participants to groups.
Cite this Scribbr article
If you want to cite this source, you can copy and paste the citation or click the “Cite this Scribbr article” button to automatically add the citation to our free Citation Generator.
Bhandari, P. (2023, June 22). Random Assignment in Experiments | Introduction & Examples. Scribbr. Retrieved June 24, 2024, from https://www.scribbr.com/methodology/random-assignment/
Is this article helpful?
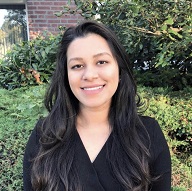
Pritha Bhandari
Other students also liked, guide to experimental design | overview, steps, & examples, confounding variables | definition, examples & controls, control groups and treatment groups | uses & examples, "i thought ai proofreading was useless but..".
I've been using Scribbr for years now and I know it's a service that won't disappoint. It does a good job spotting mistakes”
2.3 Analyzing Findings
Learning objectives.
By the end of this section, you will be able to:
- Explain what a correlation coefficient tells us about the relationship between variables
- Recognize that correlation does not indicate a cause-and-effect relationship between variables
- Discuss our tendency to look for relationships between variables that do not really exist
- Explain random sampling and assignment of participants into experimental and control groups
- Discuss how experimenter or participant bias could affect the results of an experiment
- Identify independent and dependent variables
Did you know that as sales in ice cream increase, so does the overall rate of crime? Is it possible that indulging in your favorite flavor of ice cream could send you on a crime spree? Or, after committing crime do you think you might decide to treat yourself to a cone? There is no question that a relationship exists between ice cream and crime (e.g., Harper, 2013), but it would be pretty foolish to decide that one thing actually caused the other to occur.
It is much more likely that both ice cream sales and crime rates are related to the temperature outside. When the temperature is warm, there are lots of people out of their houses, interacting with each other, getting annoyed with one another, and sometimes committing crimes. Also, when it is warm outside, we are more likely to seek a cool treat like ice cream. How do we determine if there is indeed a relationship between two things? And when there is a relationship, how can we discern whether it is attributable to coincidence or causation?
Correlational Research
Correlation means that there is a relationship between two or more variables (such as ice cream consumption and crime), but this relationship does not necessarily imply cause and effect. When two variables are correlated, it simply means that as one variable changes, so does the other. We can measure correlation by calculating a statistic known as a correlation coefficient. A correlation coefficient is a number from -1 to +1 that indicates the strength and direction of the relationship between variables. The correlation coefficient is usually represented by the letter r .
The number portion of the correlation coefficient indicates the strength of the relationship. The closer the number is to 1 (be it negative or positive), the more strongly related the variables are, and the more predictable changes in one variable will be as the other variable changes. The closer the number is to zero, the weaker the relationship, and the less predictable the relationship between the variables becomes. For instance, a correlation coefficient of 0.9 indicates a far stronger relationship than a correlation coefficient of 0.3. If the variables are not related to one another at all, the correlation coefficient is 0. The example above about ice cream and crime is an example of two variables that we might expect to have no relationship to each other.
The sign—positive or negative—of the correlation coefficient indicates the direction of the relationship ( Figure 2.12 ). A positive correlation means that the variables move in the same direction. Put another way, it means that as one variable increases so does the other, and conversely, when one variable decreases so does the other. A negative correlation means that the variables move in opposite directions. If two variables are negatively correlated, a decrease in one variable is associated with an increase in the other and vice versa.
The example of ice cream and crime rates is a positive correlation because both variables increase when temperatures are warmer. Other examples of positive correlations are the relationship between an individual’s height and weight or the relationship between a person’s age and number of wrinkles. One might expect a negative correlation to exist between someone’s tiredness during the day and the number of hours they slept the previous night: the amount of sleep decreases as the feelings of tiredness increase. In a real-world example of negative correlation, student researchers at the University of Minnesota found a weak negative correlation ( r = -0.29) between the average number of days per week that students got fewer than 5 hours of sleep and their GPA (Lowry, Dean, & Manders, 2010). Keep in mind that a negative correlation is not the same as no correlation. For example, we would probably find no correlation between hours of sleep and shoe size.
As mentioned earlier, correlations have predictive value. Imagine that you are on the admissions committee of a major university. You are faced with a huge number of applications, but you are able to accommodate only a small percentage of the applicant pool. How might you decide who should be admitted? You might try to correlate your current students’ college GPA with their scores on standardized tests like the SAT or ACT. By observing which correlations were strongest for your current students, you could use this information to predict relative success of those students who have applied for admission into the university.
Link to Learning
Manipulate this interactive scatterplot to practice your understanding of positive and negative correlation.
Correlation Does Not Indicate Causation
Correlational research is useful because it allows us to discover the strength and direction of relationships that exist between two variables. However, correlation is limited because establishing the existence of a relationship tells us little about cause and effect . While variables are sometimes correlated because one does cause the other, it could also be that some other factor, a confounding variable , is actually causing the systematic movement in our variables of interest. In the ice cream/crime rate example mentioned earlier, temperature is a confounding variable that could account for the relationship between the two variables.
Even when we cannot point to clear confounding variables, we should not assume that a correlation between two variables implies that one variable causes changes in another. This can be frustrating when a cause-and-effect relationship seems clear and intuitive. Think back to our discussion of the research done by the American Cancer Society and how their research projects were some of the first demonstrations of the link between smoking and cancer. It seems reasonable to assume that smoking causes cancer, but if we were limited to correlational research , we would be overstepping our bounds by making this assumption.
Unfortunately, people mistakenly make claims of causation as a function of correlations all the time. Such claims are especially common in advertisements and news stories. For example, research found that people who eat certain breakfast cereal may have a reduced risk of heart disease (Anderson, Hanna, Peng, & Kryscio, 2000). Cereal companies are likely to share this information in a way that maximizes and perhaps overstates the positive aspects of eating cereal. But does cereal really cause better health, or are there other possible explanations for the health of those who eat cereal? While correlational research is invaluable in identifying relationships among variables, a major limitation is the inability to establish causality. Psychologists want to make statements about cause and effect, but the only way to do that is to conduct an experiment to answer a research question. The next section describes how scientific experiments incorporate methods that eliminate, or control for, alternative explanations, which allow researchers to explore how changes in one variable cause changes in another variable.
Illusory Correlations
The temptation to make erroneous cause-and-effect statements based on correlational research is not the only way we tend to misinterpret data. We also tend to make the mistake of illusory correlations, especially with unsystematic observations. Illusory correlations , or false correlations, occur when people believe that relationships exist between two things when no such relationship exists. One well-known illusory correlation is the supposed effect that the moon’s phases have on human behavior. Many people passionately assert that human behavior is affected by the phase of the moon, and specifically, that people act strangely when the moon is full ( Figure 2.14 ).
There is no denying that the moon exerts a powerful influence on our planet. The ebb and flow of the ocean’s tides are tightly tied to the gravitational forces of the moon. Many people believe, therefore, that it is logical that we are affected by the moon as well. After all, our bodies are largely made up of water. A meta-analysis of nearly 40 studies consistently demonstrated, however, that the relationship between the moon and our behavior does not exist (Rotton & Kelly, 1985). While we may pay more attention to odd behavior during the full phase of the moon, the rates of odd behavior remain constant throughout the lunar cycle.
Why are we so apt to believe in illusory correlations like this? Often we read or hear about them and simply accept the information as valid. Or, we have a hunch about how something works and then look for evidence to support that hunch, ignoring evidence that would tell us our hunch is false; this is known as confirmation bias . Other times, we find illusory correlations based on the information that comes most easily to mind, even if that information is severely limited. And while we may feel confident that we can use these relationships to better understand and predict the world around us, illusory correlations can have significant drawbacks. For example, research suggests that illusory correlations—in which certain behaviors are inaccurately attributed to certain groups—are involved in the formation of prejudicial attitudes that can ultimately lead to discriminatory behavior (Fiedler, 2004).
Causality: Conducting Experiments and Using the Data
As you’ve learned, the only way to establish that there is a cause-and-effect relationship between two variables is to conduct a scientific experiment . Experiment has a different meaning in the scientific context than in everyday life. In everyday conversation, we often use it to describe trying something for the first time, such as experimenting with a new hair style or a new food. However, in the scientific context, an experiment has precise requirements for design and implementation.
The Experimental Hypothesis
In order to conduct an experiment, a researcher must have a specific hypothesis to be tested. As you’ve learned, hypotheses can be formulated either through direct observation of the real world or after careful review of previous research. For example, if you think that the use of technology in the classroom has negative impacts on learning, then you have basically formulated a hypothesis—namely, that the use of technology in the classroom should be limited because it decreases learning. How might you have arrived at this particular hypothesis? You may have noticed that your classmates who take notes on their laptops perform at lower levels on class exams than those who take notes by hand, or those who receive a lesson via a computer program versus via an in-person teacher have different levels of performance when tested ( Figure 2.15 ).
These sorts of personal observations are what often lead us to formulate a specific hypothesis, but we cannot use limited personal observations and anecdotal evidence to rigorously test our hypothesis. Instead, to find out if real-world data supports our hypothesis, we have to conduct an experiment.
Designing an Experiment
The most basic experimental design involves two groups: the experimental group and the control group. The two groups are designed to be the same except for one difference— experimental manipulation. The experimental group gets the experimental manipulation—that is, the treatment or variable being tested (in this case, the use of technology)—and the control group does not. Since experimental manipulation is the only difference between the experimental and control groups, we can be sure that any differences between the two are due to experimental manipulation rather than chance.
In our example of how the use of technology should be limited in the classroom, we have the experimental group learn algebra using a computer program and then test their learning. We measure the learning in our control group after they are taught algebra by a teacher in a traditional classroom. It is important for the control group to be treated similarly to the experimental group, with the exception that the control group does not receive the experimental manipulation.
We also need to precisely define, or operationalize, how we measure learning of algebra. An operational definition is a precise description of our variables, and it is important in allowing others to understand exactly how and what a researcher measures in a particular experiment. In operationalizing learning, we might choose to look at performance on a test covering the material on which the individuals were taught by the teacher or the computer program. We might also ask our participants to summarize the information that was just presented in some way. Whatever we determine, it is important that we operationalize learning in such a way that anyone who hears about our study for the first time knows exactly what we mean by learning. This aids peoples’ ability to interpret our data as well as their capacity to repeat our experiment should they choose to do so.
Once we have operationalized what is considered use of technology and what is considered learning in our experiment participants, we need to establish how we will run our experiment. In this case, we might have participants spend 45 minutes learning algebra (either through a computer program or with an in-person math teacher) and then give them a test on the material covered during the 45 minutes.
Ideally, the people who score the tests are unaware of who was assigned to the experimental or control group, in order to control for experimenter bias. Experimenter bias refers to the possibility that a researcher’s expectations might skew the results of the study. Remember, conducting an experiment requires a lot of planning, and the people involved in the research project have a vested interest in supporting their hypotheses. If the observers knew which child was in which group, it might influence how they interpret ambiguous responses, such as sloppy handwriting or minor computational mistakes. By being blind to which child is in which group, we protect against those biases. This situation is a single-blind study , meaning that one of the groups (participants) are unaware as to which group they are in (experiment or control group) while the researcher who developed the experiment knows which participants are in each group.
In a double-blind study , both the researchers and the participants are blind to group assignments. Why would a researcher want to run a study where no one knows who is in which group? Because by doing so, we can control for both experimenter and participant expectations. If you are familiar with the phrase placebo effect , you already have some idea as to why this is an important consideration. The placebo effect occurs when people's expectations or beliefs influence or determine their experience in a given situation. In other words, simply expecting something to happen can actually make it happen.
The placebo effect is commonly described in terms of testing the effectiveness of a new medication. Imagine that you work in a pharmaceutical company, and you think you have a new drug that is effective in treating depression. To demonstrate that your medication is effective, you run an experiment with two groups: The experimental group receives the medication, and the control group does not. But you don’t want participants to know whether they received the drug or not.
Why is that? Imagine that you are a participant in this study, and you have just taken a pill that you think will improve your mood. Because you expect the pill to have an effect, you might feel better simply because you took the pill and not because of any drug actually contained in the pill—this is the placebo effect.
To make sure that any effects on mood are due to the drug and not due to expectations, the control group receives a placebo (in this case a sugar pill). Now everyone gets a pill, and once again neither the researcher nor the experimental participants know who got the drug and who got the sugar pill. Any differences in mood between the experimental and control groups can now be attributed to the drug itself rather than to experimenter bias or participant expectations ( Figure 2.16 ).
Independent and Dependent Variables
In a research experiment, we strive to study whether changes in one thing cause changes in another. To achieve this, we must pay attention to two important variables, or things that can be changed, in any experimental study: the independent variable and the dependent variable. An independent variable is manipulated or controlled by the experimenter. In a well-designed experimental study, the independent variable is the only important difference between the experimental and control groups. In our example of how technology use in the classroom affects learning, the independent variable is the type of learning by participants in the study ( Figure 2.17 ). A dependent variable is what the researcher measures to see how much effect the independent variable had. In our example, the dependent variable is the learning exhibited by our participants.
We expect that the dependent variable will change as a function of the independent variable. In other words, the dependent variable depends on the independent variable. A good way to think about the relationship between the independent and dependent variables is with this question: What effect does the independent variable have on the dependent variable? Returning to our example, what is the effect of being taught a lesson through a computer program versus through an in-person instructor?
Selecting and Assigning Experimental Participants
Now that our study is designed, we need to obtain a sample of individuals to include in our experiment. Our study involves human participants so we need to determine whom to include. Participants are the subjects of psychological research, and as the name implies, individuals who are involved in psychological research actively participate in the process. Often, psychological research projects rely on college students to serve as participants. In fact, the vast majority of research in psychology subfields has historically involved students as research participants (Sears, 1986; Arnett, 2008). But are college students truly representative of the general population? College students tend to be younger, more educated, more liberal, and less diverse than the general population. Although using students as test subjects is an accepted practice, relying on such a limited pool of research participants can be problematic because it is difficult to generalize findings to the larger population.
Our hypothetical experiment involves high school students, and we must first generate a sample of students. Samples are used because populations are usually too large to reasonably involve every member in our particular experiment ( Figure 2.18 ). If possible, we should use a random sample (there are other types of samples, but for the purposes of this chapter, we will focus on random samples). A random sample is a subset of a larger population in which every member of the population has an equal chance of being selected. Random samples are preferred because if the sample is large enough we can be reasonably sure that the participating individuals are representative of the larger population. This means that the percentages of characteristics in the sample—sex, ethnicity, socioeconomic level, and any other characteristics that might affect the results—are close to those percentages in the larger population.
In our example, let’s say we decide our population of interest is algebra students. But all algebra students is a very large population, so we need to be more specific; instead we might say our population of interest is all algebra students in a particular city. We should include students from various income brackets, family situations, races, ethnicities, religions, and geographic areas of town. With this more manageable population, we can work with the local schools in selecting a random sample of around 200 algebra students who we want to participate in our experiment.
In summary, because we cannot test all of the algebra students in a city, we want to find a group of about 200 that reflects the composition of that city. With a representative group, we can generalize our findings to the larger population without fear of our sample being biased in some way.
Now that we have a sample, the next step of the experimental process is to split the participants into experimental and control groups through random assignment. With random assignment , all participants have an equal chance of being assigned to either group. There is statistical software that will randomly assign each of the algebra students in the sample to either the experimental or the control group.
Random assignment is critical for sound experimental design . With sufficiently large samples, random assignment makes it unlikely that there are systematic differences between the groups. So, for instance, it would be very unlikely that we would get one group composed entirely of males, a given ethnic identity, or a given religious ideology. This is important because if the groups were systematically different before the experiment began, we would not know the origin of any differences we find between the groups: Were the differences preexisting, or were they caused by manipulation of the independent variable? Random assignment allows us to assume that any differences observed between experimental and control groups result from the manipulation of the independent variable.
Use this online random number generator to learn more about random sampling and assignments.
Issues to Consider
While experiments allow scientists to make cause-and-effect claims, they are not without problems. True experiments require the experimenter to manipulate an independent variable, and that can complicate many questions that psychologists might want to address. For instance, imagine that you want to know what effect sex (the independent variable) has on spatial memory (the dependent variable). Although you can certainly look for differences between males and females on a task that taps into spatial memory, you cannot directly control a person’s sex. We categorize this type of research approach as quasi-experimental and recognize that we cannot make cause-and-effect claims in these circumstances.
Experimenters are also limited by ethical constraints. For instance, you would not be able to conduct an experiment designed to determine if experiencing abuse as a child leads to lower levels of self-esteem among adults. To conduct such an experiment, you would need to randomly assign some experimental participants to a group that receives abuse, and that experiment would be unethical.
Interpreting Experimental Findings
Once data is collected from both the experimental and the control groups, a statistical analysis is conducted to find out if there are meaningful differences between the two groups. A statistical analysis determines how likely it is that any difference found is due to chance (and thus not meaningful). For example, if an experiment is done on the effectiveness of a nutritional supplement, and those taking a placebo pill (and not the supplement) have the same result as those taking the supplement, then the experiment has shown that the nutritional supplement is not effective. Generally, psychologists consider differences to be statistically significant if there is less than a five percent chance of observing them if the groups did not actually differ from one another. Stated another way, psychologists want to limit the chances of making “false positive” claims to five percent or less.
The greatest strength of experiments is the ability to assert that any significant differences in the findings are caused by the independent variable. This occurs because random selection, random assignment, and a design that limits the effects of both experimenter bias and participant expectancy should create groups that are similar in composition and treatment. Therefore, any difference between the groups is attributable to the independent variable, and now we can finally make a causal statement. If we find that watching a violent television program results in more violent behavior than watching a nonviolent program, we can safely say that watching violent television programs causes an increase in the display of violent behavior.
Reporting Research
When psychologists complete a research project, they generally want to share their findings with other scientists. The American Psychological Association (APA) publishes a manual detailing how to write a paper for submission to scientific journals. Unlike an article that might be published in a magazine like Psychology Today, which targets a general audience with an interest in psychology, scientific journals generally publish peer-reviewed journal articles aimed at an audience of professionals and scholars who are actively involved in research themselves.
The Online Writing Lab (OWL) at Purdue University can walk you through the APA writing guidelines.
A peer-reviewed journal article is read by several other scientists (generally anonymously) with expertise in the subject matter. These peer reviewers provide feedback—to both the author and the journal editor—regarding the quality of the draft. Peer reviewers look for a strong rationale for the research being described, a clear description of how the research was conducted, and evidence that the research was conducted in an ethical manner. They also look for flaws in the study's design, methods, and statistical analyses. They check that the conclusions drawn by the authors seem reasonable given the observations made during the research. Peer reviewers also comment on how valuable the research is in advancing the discipline’s knowledge. This helps prevent unnecessary duplication of research findings in the scientific literature and, to some extent, ensures that each research article provides new information. Ultimately, the journal editor will compile all of the peer reviewer feedback and determine whether the article will be published in its current state (a rare occurrence), published with revisions, or not accepted for publication.
Peer review provides some degree of quality control for psychological research. Poorly conceived or executed studies can be weeded out, and even well-designed research can be improved by the revisions suggested. Peer review also ensures that the research is described clearly enough to allow other scientists to replicate it, meaning they can repeat the experiment using different samples to determine reliability. Sometimes replications involve additional measures that expand on the original finding. In any case, each replication serves to provide more evidence to support the original research findings. Successful replications of published research make scientists more apt to adopt those findings, while repeated failures tend to cast doubt on the legitimacy of the original article and lead scientists to look elsewhere. For example, it would be a major advancement in the medical field if a published study indicated that taking a new drug helped individuals achieve better health without changing their behavior. But if other scientists could not replicate the results, the original study’s claims would be questioned.
In recent years, there has been increasing concern about a “replication crisis” that has affected a number of scientific fields, including psychology. Some of the most well-known studies and scientists have produced research that has failed to be replicated by others (as discussed in Shrout & Rodgers, 2018). In fact, even a famous Nobel Prize-winning scientist has recently retracted a published paper because she had difficulty replicating her results (Nobel Prize-winning scientist Frances Arnold retracts paper, 2020 January 3). These kinds of outcomes have prompted some scientists to begin to work together and more openly, and some would argue that the current “crisis” is actually improving the ways in which science is conducted and in how its results are shared with others (Aschwanden, 2018).
The Vaccine-Autism Myth and Retraction of Published Studies
Some scientists have claimed that routine childhood vaccines cause some children to develop autism, and, in fact, several peer-reviewed publications published research making these claims. Since the initial reports, large-scale epidemiological research has indicated that vaccinations are not responsible for causing autism and that it is much safer to have your child vaccinated than not. Furthermore, several of the original studies making this claim have since been retracted.
A published piece of work can be rescinded when data is called into question because of falsification, fabrication, or serious research design problems. Once rescinded, the scientific community is informed that there are serious problems with the original publication. Retractions can be initiated by the researcher who led the study, by research collaborators, by the institution that employed the researcher, or by the editorial board of the journal in which the article was originally published. In the vaccine-autism case, the retraction was made because of a significant conflict of interest in which the leading researcher had a financial interest in establishing a link between childhood vaccines and autism (Offit, 2008). Unfortunately, the initial studies received so much media attention that many parents around the world became hesitant to have their children vaccinated ( Figure 2.19 ). Continued reliance on such debunked studies has significant consequences. For instance, between January and October of 2019, there were 22 measles outbreaks across the United States and more than a thousand cases of individuals contracting measles (Patel et al., 2019). This is likely due to the anti-vaccination movements that have risen from the debunked research. For more information about how the vaccine/autism story unfolded, as well as the repercussions of this story, take a look at Paul Offit’s book, Autism’s False Prophets: Bad Science, Risky Medicine, and the Search for a Cure.
Reliability and Validity
Reliability and validity are two important considerations that must be made with any type of data collection. Reliability refers to the ability to consistently produce a given result. In the context of psychological research, this would mean that any instruments or tools used to collect data do so in consistent, reproducible ways. There are a number of different types of reliability. Some of these include inter-rater reliability (the degree to which two or more different observers agree on what has been observed), internal consistency (the degree to which different items on a survey that measure the same thing correlate with one another), and test-retest reliability (the degree to which the outcomes of a particular measure remain consistent over multiple administrations).
Unfortunately, being consistent in measurement does not necessarily mean that you have measured something correctly. To illustrate this concept, consider a kitchen scale that would be used to measure the weight of cereal that you eat in the morning. If the scale is not properly calibrated, it may consistently under- or overestimate the amount of cereal that’s being measured. While the scale is highly reliable in producing consistent results (e.g., the same amount of cereal poured onto the scale produces the same reading each time), those results are incorrect. This is where validity comes into play. Validity refers to the extent to which a given instrument or tool accurately measures what it’s supposed to measure, and once again, there are a number of ways in which validity can be expressed. Ecological validity (the degree to which research results generalize to real-world applications), construct validity (the degree to which a given variable actually captures or measures what it is intended to measure), and face validity (the degree to which a given variable seems valid on the surface) are just a few types that researchers consider. While any valid measure is by necessity reliable, the reverse is not necessarily true. Researchers strive to use instruments that are both highly reliable and valid.
Everyday Connection
How valid are the sat and act.
Standardized tests like the SAT and ACT are supposed to measure an individual’s aptitude for a college education, but how reliable and valid are such tests? Research conducted by the College Board suggests that scores on the SAT have high predictive validity for first-year college students’ GPA (Kobrin, Patterson, Shaw, Mattern, & Barbuti, 2008). In this context, predictive validity refers to the test’s ability to effectively predict the GPA of college freshmen. Given that many institutions of higher education require the SAT or ACT for admission, this high degree of predictive validity might be comforting.
However, the emphasis placed on SAT or ACT scores in college admissions is changing based on a number of factors. For one, some researchers assert that these tests are biased, and students from historically marginalized populations are at a disadvantage that unfairly reduces the likelihood of being admitted into a college (Santelices & Wilson, 2010). Additionally, some research has suggested that the predictive validity of these tests is grossly exaggerated in how well they are able to predict the GPA of first-year college students. In fact, it has been suggested that the SAT’s predictive validity may be overestimated by as much as 150% (Rothstein, 2004). Many institutions of higher education are beginning to consider de-emphasizing the significance of SAT scores in making admission decisions (Rimer, 2008).
Recent examples of high profile cheating scandals both domestically and abroad have only increased the scrutiny being placed on these types of tests, and as of March 2019, more than 1000 institutions of higher education have either relaxed or eliminated the requirements for SAT or ACT testing for admissions (Strauss, 2019, March 19).
As an Amazon Associate we earn from qualifying purchases.
This book may not be used in the training of large language models or otherwise be ingested into large language models or generative AI offerings without OpenStax's permission.
Want to cite, share, or modify this book? This book uses the Creative Commons Attribution License and you must attribute OpenStax.
Access for free at https://openstax.org/books/psychology-2e/pages/1-introduction
- Authors: Rose M. Spielman, William J. Jenkins, Marilyn D. Lovett
- Publisher/website: OpenStax
- Book title: Psychology 2e
- Publication date: Apr 22, 2020
- Location: Houston, Texas
- Book URL: https://openstax.org/books/psychology-2e/pages/1-introduction
- Section URL: https://openstax.org/books/psychology-2e/pages/2-3-analyzing-findings
© Jan 6, 2024 OpenStax. Textbook content produced by OpenStax is licensed under a Creative Commons Attribution License . The OpenStax name, OpenStax logo, OpenStax book covers, OpenStax CNX name, and OpenStax CNX logo are not subject to the Creative Commons license and may not be reproduced without the prior and express written consent of Rice University.
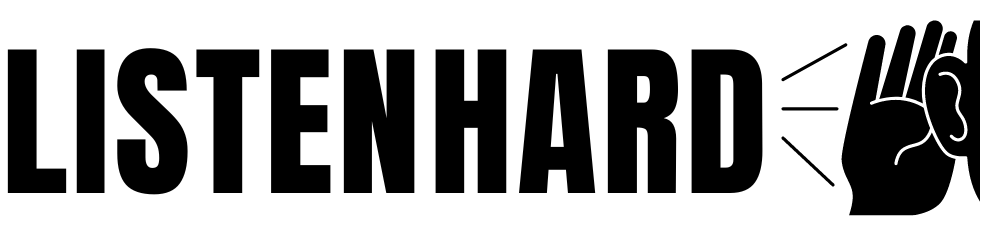
Understanding True Experiments in Psychology: Principles and Applications
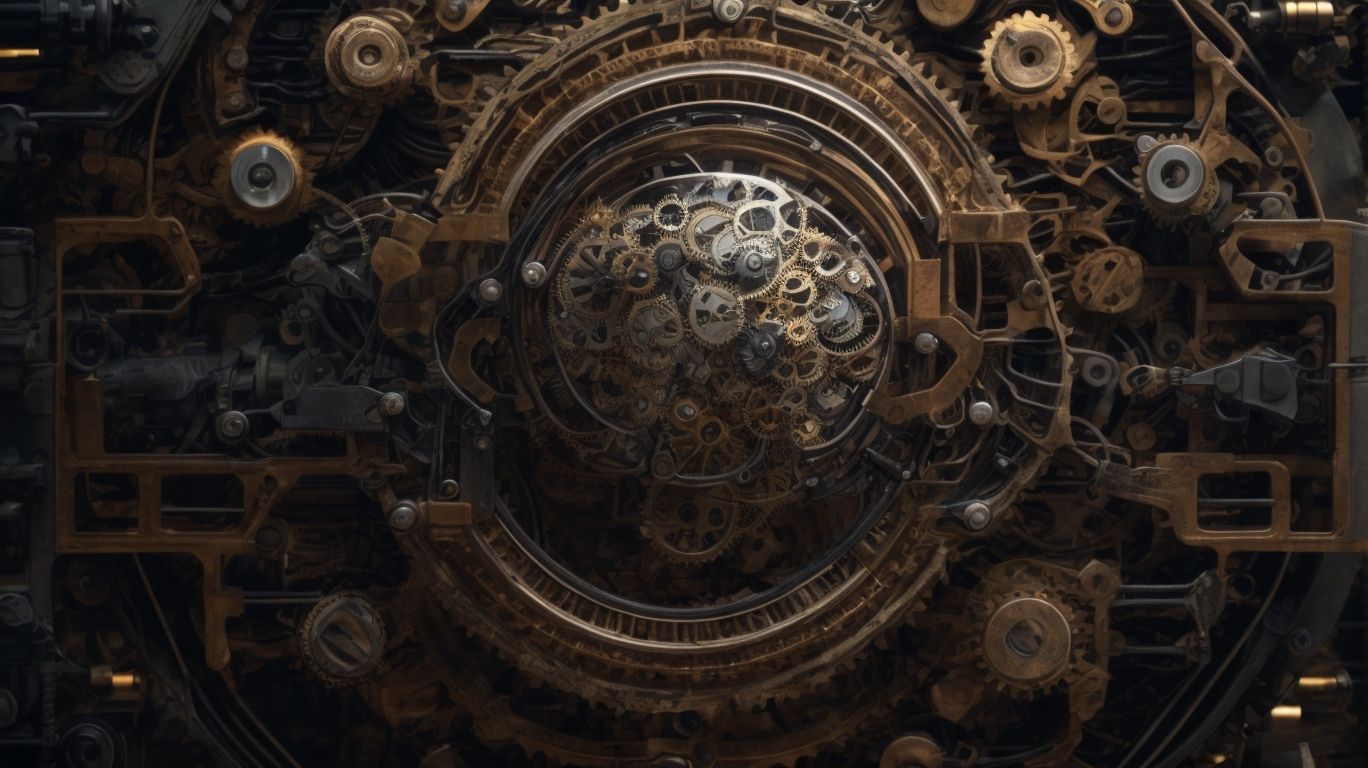
True experiments in psychology are a crucial method used by researchers to test hypotheses, establish cause and effect relationships, and advance scientific knowledge. In this article, we will delve into the principles of true experiments, such as random assignment and manipulation of variables.
We will also explore how true experiments differ from other types of studies, the ethical considerations involved, and the limitations researchers face. Stay tuned to discover how researchers can improve the validity of true experiments and their applications in the field of psychology.
- Random assignment is a crucial principle in true experiments as it ensures that participants are assigned to groups without bias, increasing the validity of the study.
- Manipulation of the independent variable and control group are key elements in establishing cause and effect relationships in true experiments.
- True experiments have various applications such as testing hypotheses, identifying effective treatments, and advancing scientific knowledge in psychology.
- 1 What Are True Experiments in Psychology?
- 2.1 Random Assignment
- 2.2 Control Group
- 2.3 Manipulation of Independent Variable
- 2.4 Measurement of Dependent Variable
- 2.5 Elimination of Confounding Variables
- 3.1 Correlational Studies
- 3.2 Quasi-Experiments
- 3.3 Observational Studies
- 4.1 Testing Hypotheses
- 4.2 Establishing Cause and Effect Relationships
- 4.3 Replicating Previous Findings
- 4.4 Identifying Effective Treatments
- 4.5 Advancing Scientific Knowledge
- 5 What Are the Ethical Considerations in True Experiments?
- 6 What Are the Limitations of True Experiments?
- 7 How Can Researchers Improve the Validity of True Experiments?
- 8.1 What is a true experiment in psychology?
- 8.2 What are the principles of true experiments in psychology?
- 8.3 Why is random assignment important in true experiments?
- 8.4 How does manipulation of an independent variable work in true experiments?
- 8.5 How are extraneous variables controlled in true experiments?
- 8.6 What are some applications of true experiments in psychology?
What Are True Experiments in Psychology?
True experiments in psychology refer to research studies that involve the manipulation of an independent variable to observe the effects on a dependent variable within a controlled environment.
By manipulating the independent variable, researchers aim to determine causality relationships between variables, testing hypotheses and theories. This controlled setting ensures that any changes in the dependent variable are a direct result of the manipulated independent variable, helping to eliminate extraneous factors and increase the internal validity of the study.
Researchers carefully design these experiments, often using random assignment to assign participants to different conditions, minimizing the influence of individual differences. This rigorous methodology allows psychologists to draw reliable conclusions about human behavior and cognition.
What Are the Principles of True Experiments?
The principles of true experiments in psychology encompass key elements such as random assignment , control group implementation, and the manipulation of independent variables.
Random assignment is a crucial aspect of experimental design that involves assigning participants to different groups by chance to reduce biases and ensure that each participant has an equal chance of being in any group. This helps in establishing a cause-and-effect relationship between variables.
Control group practices involve creating a group that does not receive the experimental treatment to serve as a benchmark for comparison, allowing researchers to assess the impact of the treatment.
The manipulation of variables refers to intentionally changing one variable to observe its effect on another, allowing researchers to test hypotheses and draw conclusions based on the results.
Random Assignment
Random assignment is a crucial aspect of true experiments, ensuring that participants are assigned to groups without bias or influence.
By randomly assigning individuals to different experimental groups, researchers can be more confident that any differences observed in the outcomes are due to the manipulation of the independent variable rather than individual characteristics.
This process helps eliminate selection bias, where certain characteristics of participants may influence group allocation, ultimately leading to distorted results.
The importance of random assignment lies in its ability to create comparable groups, making the conclusions drawn from the study more credible and generalizable.
Control Group
The control group in true experiments serves as a baseline for comparison, allowing researchers to assess the impact of the independent variable.
By isolating the variable of interest and comparing it to a group that does not receive the treatment or intervention, researchers can determine whether the observed effects are truly due to the independent variable. This group provides a standard of reference against which the experimental group is measured, ensuring that any changes or outcomes can be attributed accurately. Control groups play a crucial role in reducing bias and ensuring the validity of study results, helping researchers draw meaningful conclusions from their experiments.
Manipulation of Independent Variable
Manipulating the independent variable is a core component of true experiments, enabling researchers to assess its causal impact on the dependent variable.
By systematically varying the independent variable, researchers can observe how changes in the manipulated factor lead to changes in the dependent variable, thereby establishing a cause-and-effect relationship. This manipulation allows for controlling and testing different conditions to study the direct influence of the independent variable on the outcome. Through this process, researchers can deduce the extent to which the independent variable influences the dependent variable, aiding in drawing valid conclusions about the relationship between the two variables.
Measurement of Dependent Variable
The measurement of the dependent variable in true experiments involves assessing the outcome or response that is influenced by the manipulation of the independent variable.
In research studies, the process of measuring the dependent variable plays a crucial role in determining the effectiveness of the experimental manipulation. Researchers use various techniques to quantify and record the responses of participants to different conditions. This measurement step is essential for comparing the outcomes across different groups and evaluating the impact of the independent variable on the dependent variable. Data collection tools such as surveys, questionnaires, observations, and physiological measurements are commonly employed to capture the relevant information accurately.
Elimination of Confounding Variables
Eliminating confounding variables is essential in true experiments to ensure that the observed effects are attributed to the manipulated variables rather than external influences.
To address confounding variables effectively, researchers employ various strategies to control and minimize the impact of extraneous factors.
One common approach is randomization, where participants are assigned to different experimental conditions randomly to distribute potential confounders evenly across groups.
Another method involves matching participants based on certain variables to ensure comparability between groups.
Researchers may use statistical techniques such as regression analysis to control for confounding variables during data analysis.
How Are True Experiments Different from Other Types of Studies?
True experiments in psychology differ from other types of studies such as correlational studies and quasi-experiments by their ability to establish causal relationships through controlled manipulation.
While correlational studies aim to identify relationships between variables without manipulating them, true experiments involve the deliberate manipulation of one or more factors to observe the effect on another variable. This manipulation allows researchers to assess causality effectively by controlling for confounding variables and random assignment of participants to experimental conditions.
With experimental designs, researchers can establish a cause-and-effect relationship, providing stronger evidence of the effect of an independent variable on a dependent variable. This rigorous approach helps in drawing more definitive conclusions compared to observational or correlational studies.
Correlational Studies
Correlational studies in psychology focus on identifying relationships between variables without manipulating them, offering insights into associations and predictive patterns.
These studies are significant in understanding how variables interact in the natural world, providing crucial data for researchers to analyze and draw conclusions from. By examining the relationship between variables, researchers can determine if changes in one variable correspond with changes in another, revealing potential predictive patterns. This type of research design is valuable when it is not possible or ethical to manipulate certain variables, allowing researchers to observe and interpret connections without intervening. Through statistical analysis, correlational studies help establish the strength and direction of relationships between different factors, contributing to a deeper understanding of complex phenomena.
Quasi-Experiments
Quasi-experiments in psychology resemble true experiments but lack random assignment or full control over variables, often utilized when strict experimental conditions are challenging to implement.
This type of research design shares many similarities with traditional experiments, such as having a treatment group and a control group to analyze the effects of an independent variable. Without random assignment, researchers cannot ensure that participants are equally distributed between groups based on relevant factors, potentially impacting the internal validity of the study. The limitation in control and randomization in quasi-experiments leads to difficulties in establishing a cause-and-effect relationship definitively.
Observational Studies
Observational studies in psychology involve the systematic observation of behaviors, perceptions, or phenomena without intervention or manipulation, providing valuable insights into natural settings.
In these studies, researchers carefully examine how people interact with their environment and how certain stimuli influence their actions and reactions. Behavioral analysis plays a crucial role in understanding human nature and decision-making processes. By closely monitoring individuals in their everyday routines and environments, psychologists can uncover patterns, trends, and subconscious influences that might not be apparent in experimental settings.
What Are the Applications of True Experiments in Psychology?
True experiments play a vital role in psychology by testing hypotheses, establishing cause-and-effect relationships, replicating findings, and advancing scientific knowledge through systematic procedures.
One of the key elements of true experiments is hypothesis testing , where researchers formulate a clear statement to be tested through experimentation. This process allows them to investigate and validate theories in a controlled environment.
These experiments are essential for assessing causality , as they help determine if changes in one variable directly cause changes in another. This ability to establish cause-and-effect relationships is crucial for understanding the intricacies of human behavior and mental processes.
By focusing on these rigorous methods, psychologists can push the boundaries of scientific advancement and contribute valuable insights to the field.
Testing Hypotheses
One of the primary applications of true experiments in psychology is testing hypotheses by manipulating variables and analyzing the resulting data for empirical support.
Formulating a hypothesis is the initial step in this process. Researchers conceptualize a testable statement that predicts the relationship between variables. For instance, in a study examining the impact of music on concentration, the hypothesis could be that participants exposed to classical music will perform better on a cognitive task compared to those in a silent environment.
After establishing the hypothesis, the experiment is designed to manipulate the independent variable (in this case, music exposure) and measure the dependent variable (concentration level). Data collection methods, such as observation or surveys, are then employed to gather information.
Establishing Cause and Effect Relationships
True experiments excel in establishing cause-and-effect relationships, particularly in cognitive processing studies, by systematically manipulating variables to determine their impact on outcomes.
In cognitive psychology, researchers use true experiments to delve into the intricate mechanisms of cognitive processes, such as memory, attention, and perception. By carefully manipulating independent variables and observing changes in dependent variables, they can infer causal relationships and better understand the underlying cognitive mechanisms. This method allows researchers to draw reliable conclusions about how specific variables influence mental processes, contributing valuable insights to the field.
Replicating Previous Findings
True experiments contribute to replicating previous findings in psychology, especially in memory research, by confirming the reliability and validity of established results through rigorous experimentation.
In memory-related studies, replicating research findings becomes crucial to strengthen the scientific foundation and ensure the credibility of existing knowledge in cognitive processes. Through meticulous experimental design and methodological precision, researchers aim to validate the consistency of outcomes, providing a solid basis for generalizing findings to broader populations or contexts.
Result validation processes often involve conducting multiple trials, controlling for confounding variables, and utilizing statistical analyses to determine the robustness and significance of the obtained results. By meticulously following these steps, scientists can enhance the overall understanding of memory mechanisms and contribute to the advancement of psychological theories.
Identifying Effective Treatments
True experiments aid in identifying effective treatments and interventions in psychology, enabling researchers to evaluate the efficacy of therapeutic approaches or behavioral modifications through controlled studies.
By establishing control over variables and random assignment of participants, true experiments establish a cause-and-effect relationship between the intervention and outcomes. These experiments often involve an experimental group receiving the treatment and a control group that does not, allowing researchers to compare results objectively.
The results obtained from true experiments provide valuable insights into the effectiveness of different therapeutic techniques and help psychologists tailor interventions to suit individual client needs. This evidence-based approach ensures that psychological interventions are not only well-founded but also have a higher chance of success.
Advancing Scientific Knowledge
True experiments are pivotal in advancing scientific knowledge in psychology, with EEG data studies exemplifying how controlled experimentation enhances our understanding of cognitive processes and brain functions.
Through true experiments, researchers are able to systematically manipulate variables to establish cause-and-effect relationships in their studies, providing valuable insights into complex neural mechanisms. The meticulous process of EEG data analysis in experimental settings allows scientists to observe real-time brain activity, monitor cognitive responses, and pinpoint specific brain regions involved in various tasks.
The applications of cognitive research are vast and impactful, ranging from exploring memory formation, attentional processes, language comprehension, to investigating neurological disorders such as Alzheimer’s disease and schizophrenia. Understanding the intricacies of the brain through empirical research is crucial for developing effective interventions and treatments for cognitive impairments.
What Are the Ethical Considerations in True Experiments?
Ethical considerations in true experiments involve ensuring the well-being and rights of participants, obtaining informed consent, maintaining confidentiality, and adhering to ethical guidelines set by psychological associations.
Central to the ethical framework of true experiments is the fundamental principle of beneficence, where researchers must prioritize the welfare and best interests of those participating in the study. This encompasses not only physical health but also psychological and emotional aspects to ensure a holistic approach to participant protection. Informed consent plays a pivotal role, granting individuals the autonomy to make voluntary decisions regarding their involvement after being provided with all pertinent information. This transparent exchange is crucial for fostering trust and respect.
What Are the Limitations of True Experiments?
The limitations of true experiments in psychology include challenges related to controlling extraneous variables , generalizing findings to real-world scenarios, and addressing ethical constraints in experimental designs.
Extraneous variables, also known as confounding variables, can significantly impact the results of an experiment by introducing unintended influences that distort the relationships being studied. These variables are crucial to be managed effectively to ensure the internal validity of the study. Complete control over all extraneous variables is often impractical, especially in complex human behaviors where numerous factors can interact. This limitation highlights the need for researchers to carefully design experiments and utilize statistical techniques to minimize their effects.
How Can Researchers Improve the Validity of True Experiments?
Researchers can enhance the validity of true experiments in psychology by implementing rigorous scientific methods, conducting thorough data analysis, ensuring result reliability, and adhering to established research protocols.
One of the key strategies for enhancing experiment validity is to prioritize random assignment of participants to experimental groups, which helps control for confounding variables and increases the likelihood of establishing cause and effect relationships.
Researchers should carefully design control conditions to accurately measure the impact of the independent variable. Data validation processes such as peer review and replication studies play a vital role in confirming the robustness of experimental findings.
Frequently Asked Questions
What is a true experiment in psychology.
A true experiment in psychology is a research method in which an independent variable is manipulated to determine its effect on a dependent variable, while controlling for other variables that may influence the results.
What are the principles of true experiments in psychology?
The three main principles of true experiments in psychology are random assignment, manipulation of an independent variable, and control of extraneous variables.
Why is random assignment important in true experiments?
Random assignment helps to ensure that participants are assigned to different experimental groups by chance, reducing the likelihood of pre-existing differences between the groups that could affect the results.
How does manipulation of an independent variable work in true experiments?
The independent variable, often referred to as the treatment, is manipulated by the researcher to observe its effects on the dependent variable. This allows for the establishment of cause-and-effect relationships.
How are extraneous variables controlled in true experiments?
Extraneous variables, which are factors that may influence the results but are not the focus of the study, are controlled through various methods such as random assignment, use of control groups, and experimental design.
What are some applications of true experiments in psychology?
True experiments are commonly used in psychology to study the effects of various interventions or treatments on behavior or mental processes. They can also be used to test theories and hypotheses, and to determine the effectiveness of different strategies or programs.
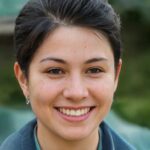
Dr. Emily Tan is a researcher in the field of psychological assessment and testing. Her expertise includes the development and validation of psychological measures, with a particular interest in personality assessment. Dr. Tan’s work aims to improve the accuracy and ethical application of psychological tests in various settings, from clinical diagnostics to organizational hiring processes.
Similar Posts
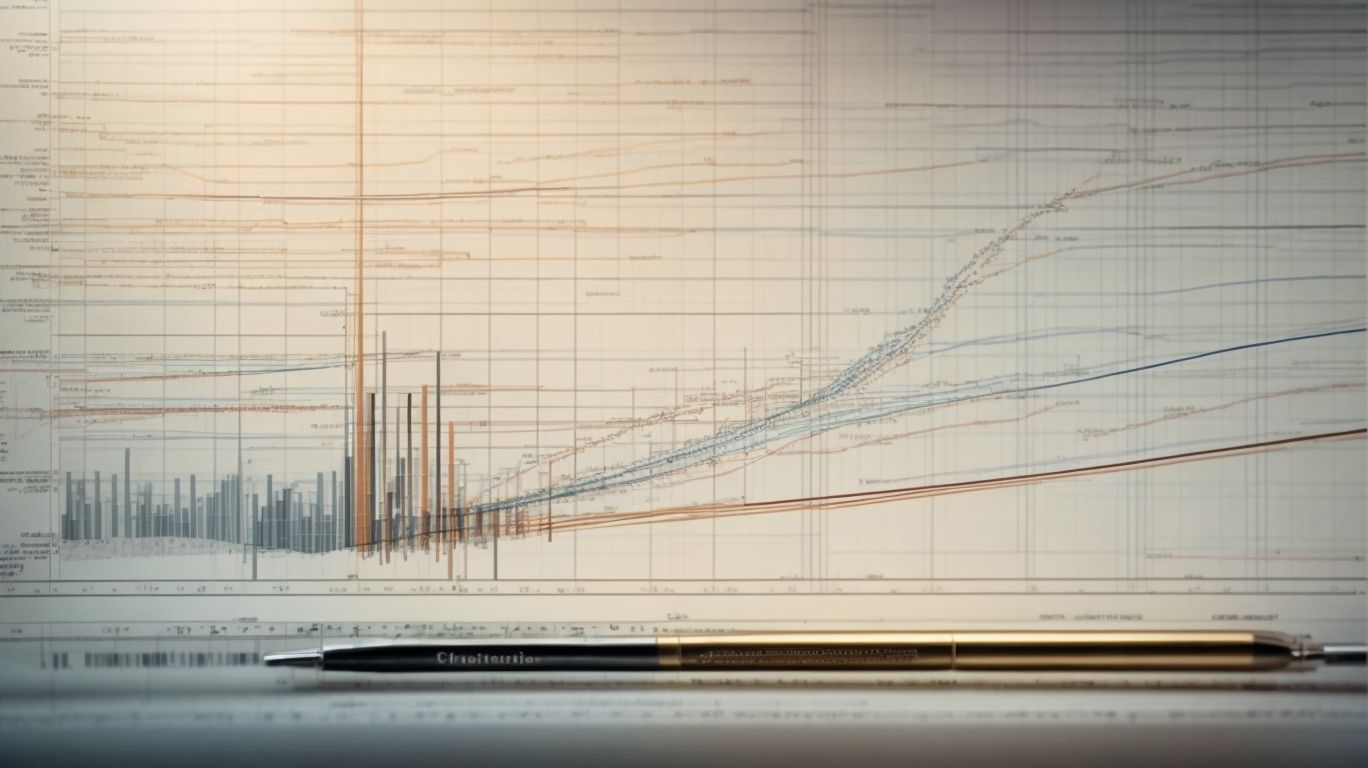
Demystifying Standard Deviation in Psychology
The article was last updated by Samantha Choi on February 5, 2024. Standard deviation is a crucial concept in the field of psychology, helping researchers…
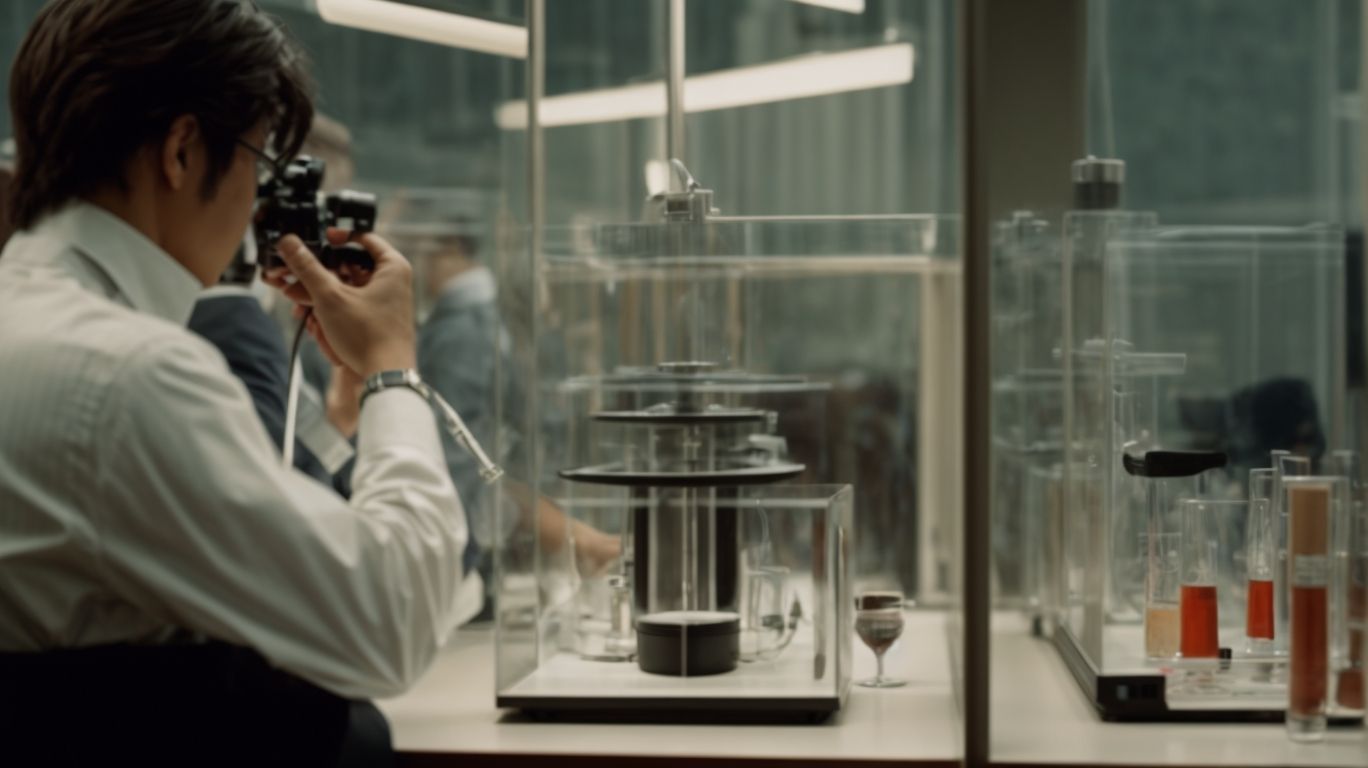
Role of Control Variables in Psychological Experiments
The article was last updated by Dr. Henry Foster on February 9, 2024. Control variables play a crucial role in psychological experiments by helping researchers…
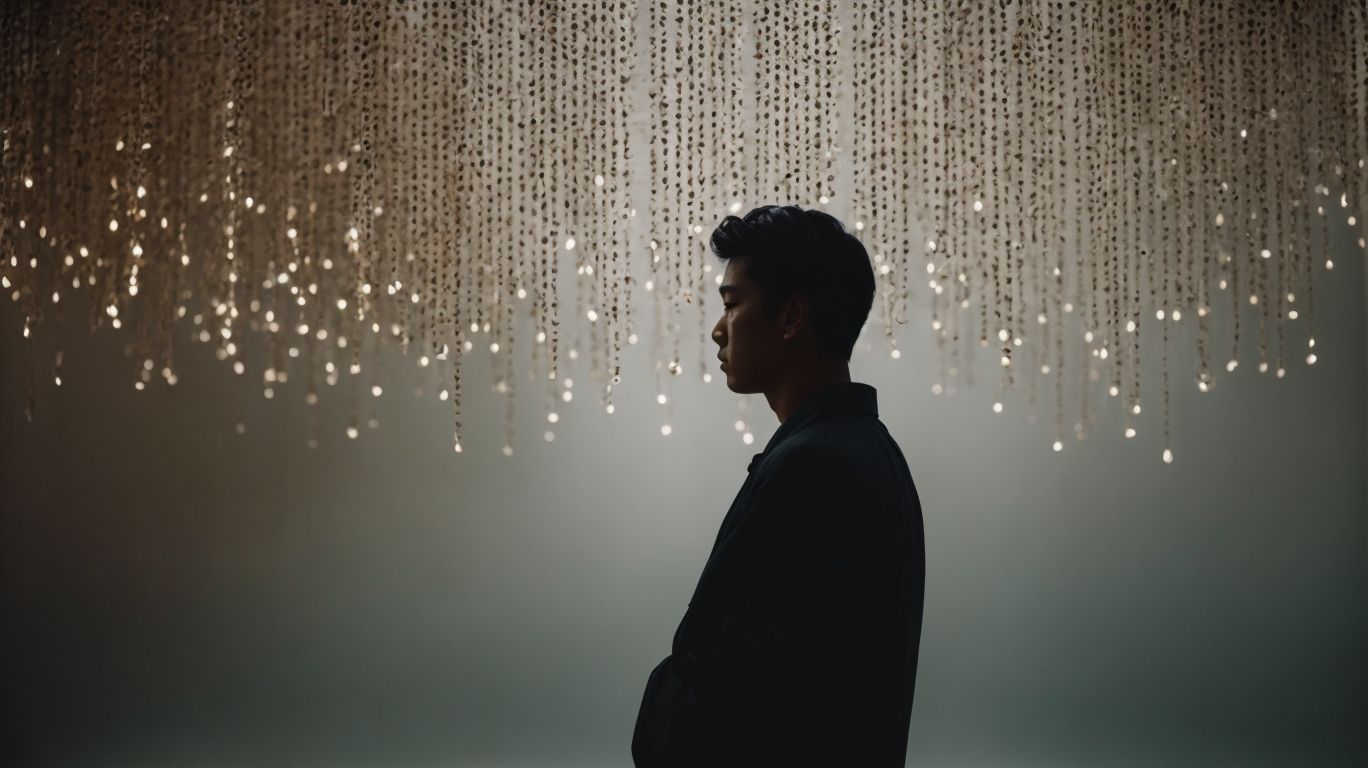
The Power of ‘Why’ Questions in Psychological Inquiry
The article was last updated by Vanessa Patel on February 8, 2024. Have you ever stopped to think about the importance of asking ‘why’ questions…
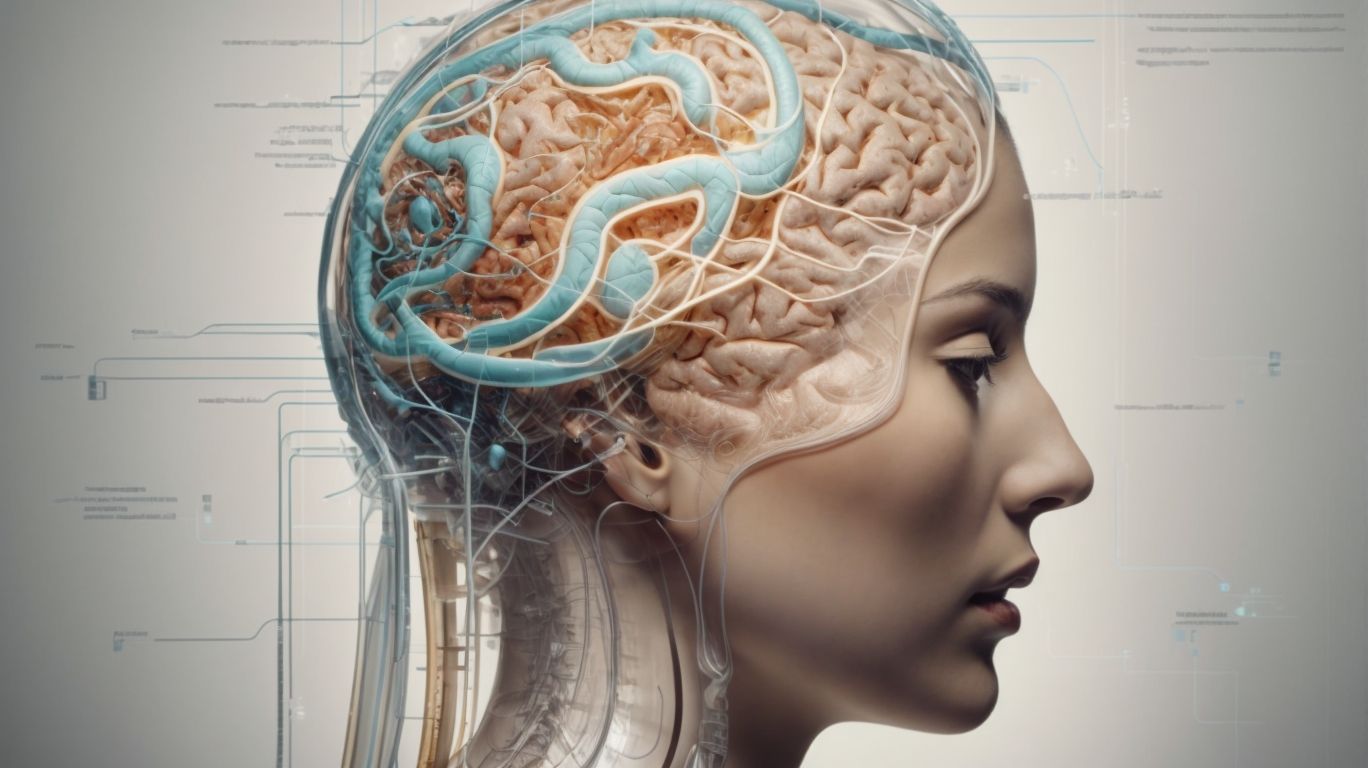
The Basics of Biofeedback in Psychology: Understanding the Mind-Body Connection
The article was last updated by Rachel Liu on February 4, 2024. Have you ever wondered how your mind and body are connected? Biofeedback in…
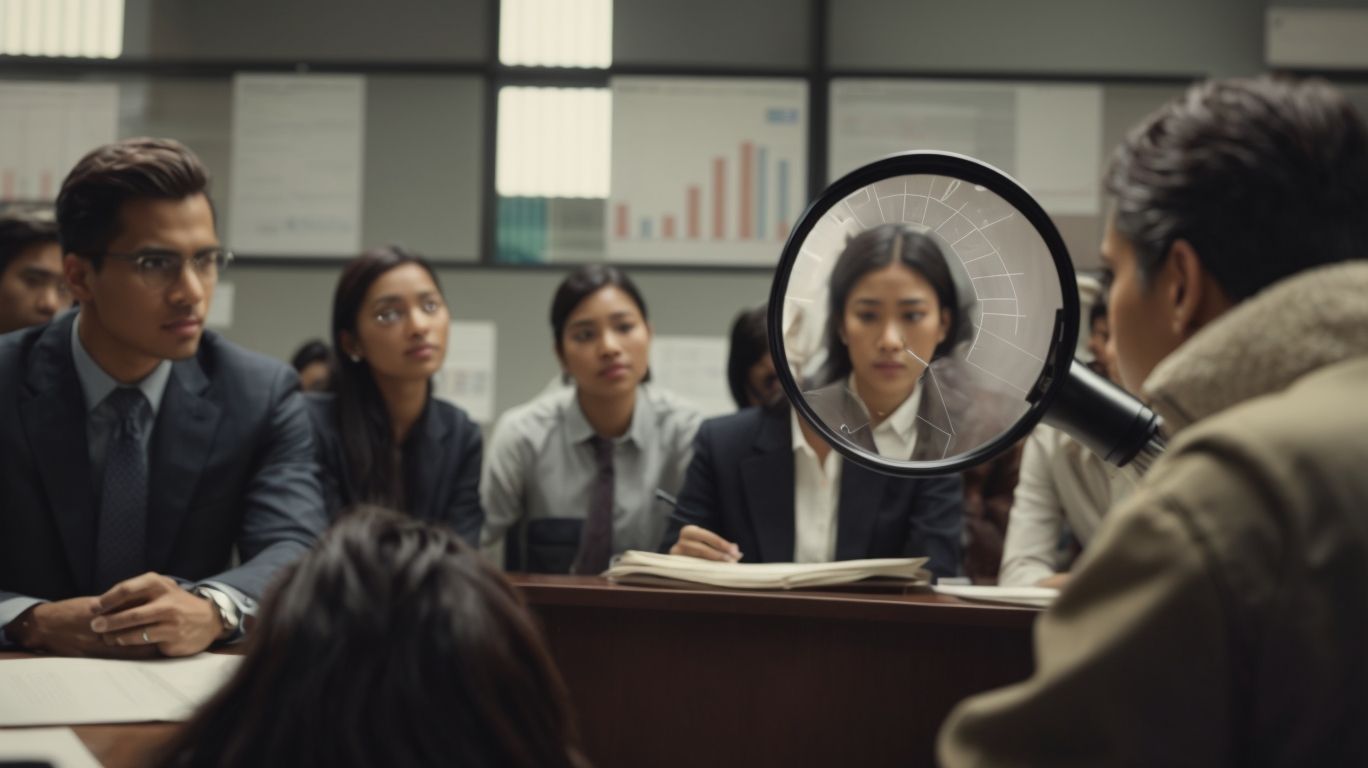
Unveiling the Role of Control Groups in Psychology: Importance, Design, and Applications
The article was last updated by Dr. Emily Tan on February 8, 2024. Control groups play a crucial role in psychology research, serving as a…
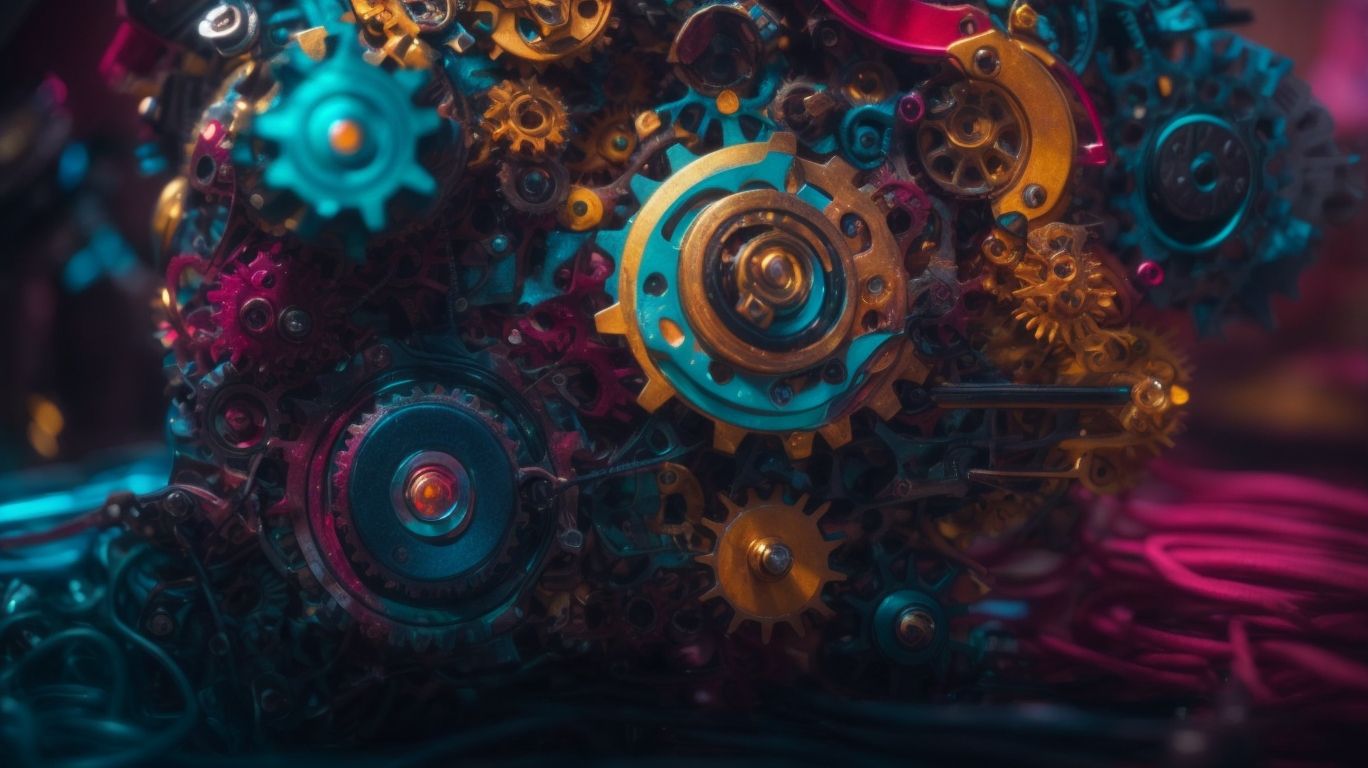
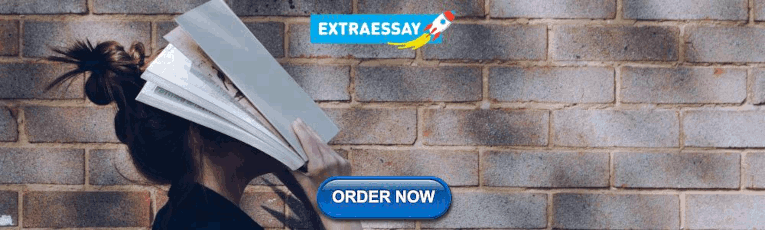
Understanding the Concept of Preregistration in Psychology
The article was last updated by Nicholas Reed on February 8, 2024. Preregistration in psychology is a crucial step in the research process that involves…
Random Assignment in Psychology (Definition + 40 Examples)

Have you ever wondered how researchers discover new ways to help people learn, make decisions, or overcome challenges? A hidden hero in this adventure of discovery is a method called random assignment, a cornerstone in psychological research that helps scientists uncover the truths about the human mind and behavior.
Random Assignment is a process used in research where each participant has an equal chance of being placed in any group within the study. This technique is essential in experiments as it helps to eliminate biases, ensuring that the different groups being compared are similar in all important aspects.
By doing so, researchers can be confident that any differences observed are likely due to the variable being tested, rather than other factors.
In this article, we’ll explore the intriguing world of random assignment, diving into its history, principles, real-world examples, and the impact it has had on the field of psychology.
History of Random Assignment
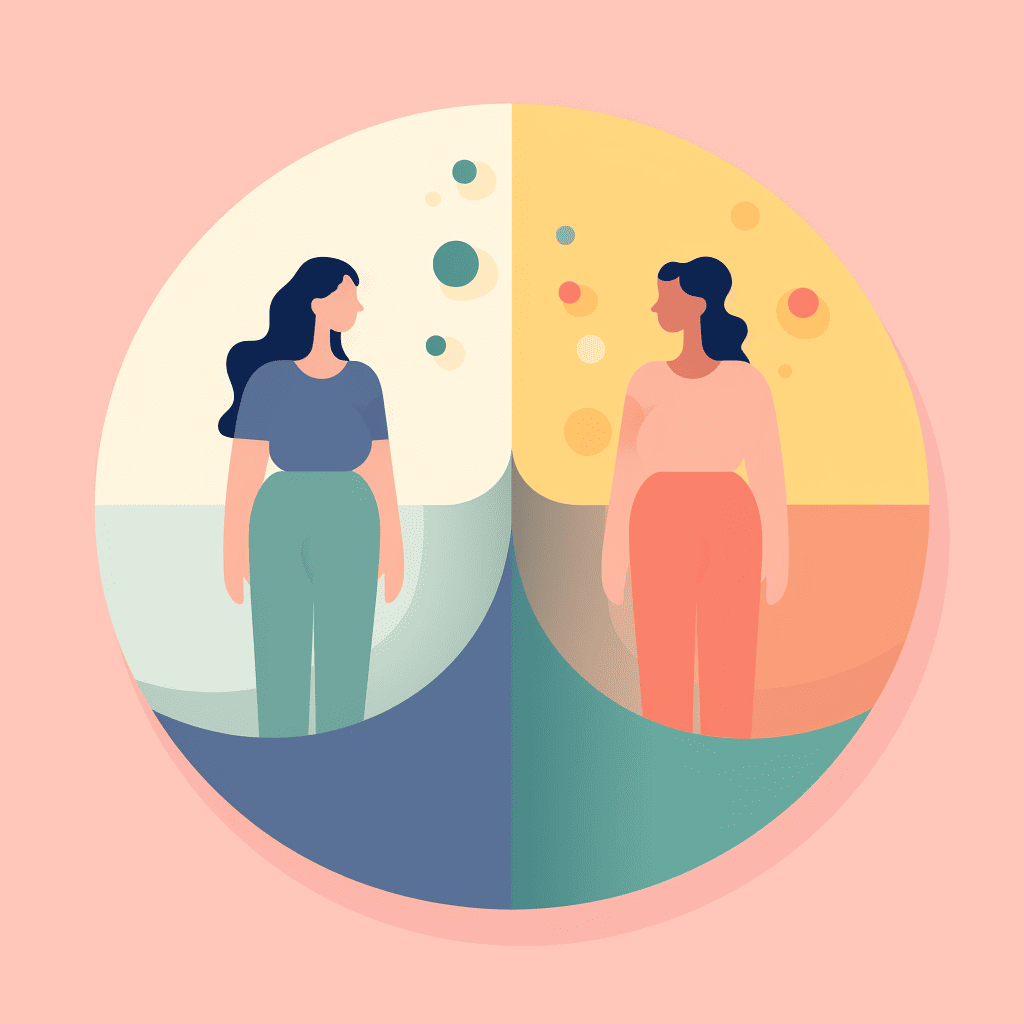
Stepping back in time, we delve into the origins of random assignment, which finds its roots in the early 20th century.
The pioneering mind behind this innovative technique was Sir Ronald A. Fisher , a British statistician and biologist. Fisher introduced the concept of random assignment in the 1920s, aiming to improve the quality and reliability of experimental research .
His contributions laid the groundwork for the method's evolution and its widespread adoption in various fields, particularly in psychology.
Fisher’s groundbreaking work on random assignment was motivated by his desire to control for confounding variables – those pesky factors that could muddy the waters of research findings.
By assigning participants to different groups purely by chance, he realized that the influence of these confounding variables could be minimized, paving the way for more accurate and trustworthy results.
Early Studies Utilizing Random Assignment
Following Fisher's initial development, random assignment started to gain traction in the research community. Early studies adopting this methodology focused on a variety of topics, from agriculture (which was Fisher’s primary field of interest) to medicine and psychology.
The approach allowed researchers to draw stronger conclusions from their experiments, bolstering the development of new theories and practices.
One notable early study utilizing random assignment was conducted in the field of educational psychology. Researchers were keen to understand the impact of different teaching methods on student outcomes.
By randomly assigning students to various instructional approaches, they were able to isolate the effects of the teaching methods, leading to valuable insights and recommendations for educators.
Evolution of the Methodology
As the decades rolled on, random assignment continued to evolve and adapt to the changing landscape of research.
Advances in technology introduced new tools and techniques for implementing randomization, such as computerized random number generators, which offered greater precision and ease of use.
The application of random assignment expanded beyond the confines of the laboratory, finding its way into field studies and large-scale surveys.
Researchers across diverse disciplines embraced the methodology, recognizing its potential to enhance the validity of their findings and contribute to the advancement of knowledge.
From its humble beginnings in the early 20th century to its widespread use today, random assignment has proven to be a cornerstone of scientific inquiry.
Its development and evolution have played a pivotal role in shaping the landscape of psychological research, driving discoveries that have improved lives and deepened our understanding of the human experience.
Principles of Random Assignment
Delving into the heart of random assignment, we uncover the theories and principles that form its foundation.
The method is steeped in the basics of probability theory and statistical inference, ensuring that each participant has an equal chance of being placed in any group, thus fostering fair and unbiased results.
Basic Principles of Random Assignment
Understanding the core principles of random assignment is key to grasping its significance in research. There are three principles: equal probability of selection, reduction of bias, and ensuring representativeness.
The first principle, equal probability of selection , ensures that every participant has an identical chance of being assigned to any group in the study. This randomness is crucial as it mitigates the risk of bias and establishes a level playing field.
The second principle focuses on the reduction of bias . Random assignment acts as a safeguard, ensuring that the groups being compared are alike in all essential aspects before the experiment begins.
This similarity between groups allows researchers to attribute any differences observed in the outcomes directly to the independent variable being studied.
Lastly, ensuring representativeness is a vital principle. When participants are assigned randomly, the resulting groups are more likely to be representative of the larger population.
This characteristic is crucial for the generalizability of the study’s findings, allowing researchers to apply their insights broadly.
Theoretical Foundation
The theoretical foundation of random assignment lies in probability theory and statistical inference .
Probability theory deals with the likelihood of different outcomes, providing a mathematical framework for analyzing random phenomena. In the context of random assignment, it helps in ensuring that each participant has an equal chance of being placed in any group.
Statistical inference, on the other hand, allows researchers to draw conclusions about a population based on a sample of data drawn from that population. It is the mechanism through which the results of a study can be generalized to a broader context.
Random assignment enhances the reliability of statistical inferences by reducing biases and ensuring that the sample is representative.
Differentiating Random Assignment from Random Selection
It’s essential to distinguish between random assignment and random selection, as the two terms, while related, have distinct meanings in the realm of research.
Random assignment refers to how participants are placed into different groups in an experiment, aiming to control for confounding variables and help determine causes.
In contrast, random selection pertains to how individuals are chosen to participate in a study. This method is used to ensure that the sample of participants is representative of the larger population, which is vital for the external validity of the research.
While both methods are rooted in randomness and probability, they serve different purposes in the research process.
Understanding the theories, principles, and distinctions of random assignment illuminates its pivotal role in psychological research.
This method, anchored in probability theory and statistical inference, serves as a beacon of reliability, guiding researchers in their quest for knowledge and ensuring that their findings stand the test of validity and applicability.
Methodology of Random Assignment
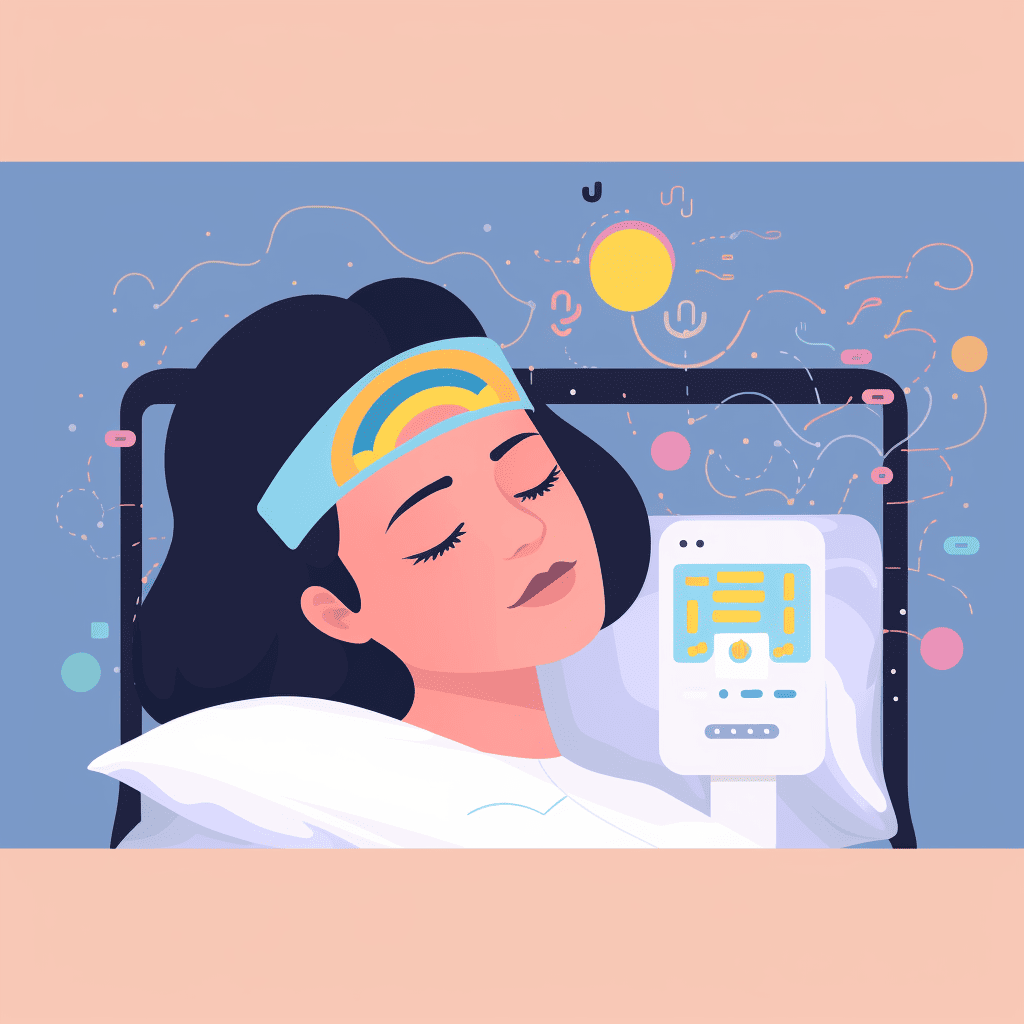
Implementing random assignment in a study is a meticulous process that involves several crucial steps.
The initial step is participant selection, where individuals are chosen to partake in the study. This stage is critical to ensure that the pool of participants is diverse and representative of the population the study aims to generalize to.
Once the pool of participants has been established, the actual assignment process begins. In this step, each participant is allocated randomly to one of the groups in the study.
Researchers use various tools, such as random number generators or computerized methods, to ensure that this assignment is genuinely random and free from biases.
Monitoring and adjusting form the final step in the implementation of random assignment. Researchers need to continuously observe the groups to ensure that they remain comparable in all essential aspects throughout the study.
If any significant discrepancies arise, adjustments might be necessary to maintain the study’s integrity and validity.
Tools and Techniques Used
The evolution of technology has introduced a variety of tools and techniques to facilitate random assignment.
Random number generators, both manual and computerized, are commonly used to assign participants to different groups. These generators ensure that each individual has an equal chance of being placed in any group, upholding the principle of equal probability of selection.
In addition to random number generators, researchers often use specialized computer software designed for statistical analysis and experimental design.
These software programs offer advanced features that allow for precise and efficient random assignment, minimizing the risk of human error and enhancing the study’s reliability.
Ethical Considerations
The implementation of random assignment is not devoid of ethical considerations. Informed consent is a fundamental ethical principle that researchers must uphold.
Informed consent means that every participant should be fully informed about the nature of the study, the procedures involved, and any potential risks or benefits, ensuring that they voluntarily agree to participate.
Beyond informed consent, researchers must conduct a thorough risk and benefit analysis. The potential benefits of the study should outweigh any risks or harms to the participants.
Safeguarding the well-being of participants is paramount, and any study employing random assignment must adhere to established ethical guidelines and standards.
Conclusion of Methodology
The methodology of random assignment, while seemingly straightforward, is a multifaceted process that demands precision, fairness, and ethical integrity. From participant selection to assignment and monitoring, each step is crucial to ensure the validity of the study’s findings.
The tools and techniques employed, coupled with a steadfast commitment to ethical principles, underscore the significance of random assignment as a cornerstone of robust psychological research.
Benefits of Random Assignment in Psychological Research
The impact and importance of random assignment in psychological research cannot be overstated. It is fundamental for ensuring the study is accurate, allowing the researchers to determine if their study actually caused the results they saw, and making sure the findings can be applied to the real world.
Facilitating Causal Inferences
When participants are randomly assigned to different groups, researchers can be more confident that the observed effects are due to the independent variable being changed, and not other factors.
This ability to determine the cause is called causal inference .
This confidence allows for the drawing of causal relationships, which are foundational for theory development and application in psychology.
Ensuring Internal Validity
One of the foremost impacts of random assignment is its ability to enhance the internal validity of an experiment.
Internal validity refers to the extent to which a researcher can assert that changes in the dependent variable are solely due to manipulations of the independent variable , and not due to confounding variables.
By ensuring that each participant has an equal chance of being in any condition of the experiment, random assignment helps control for participant characteristics that could otherwise complicate the results.
Enhancing Generalizability
Beyond internal validity, random assignment also plays a crucial role in enhancing the generalizability of research findings.
When done correctly, it ensures that the sample groups are representative of the larger population, so can allow researchers to apply their findings more broadly.
This representative nature is essential for the practical application of research, impacting policy, interventions, and psychological therapies.
Limitations of Random Assignment
Potential for implementation issues.
While the principles of random assignment are robust, the method can face implementation issues.
One of the most common problems is logistical constraints. Some studies, due to their nature or the specific population being studied, find it challenging to implement random assignment effectively.
For instance, in educational settings, logistical issues such as class schedules and school policies might stop the random allocation of students to different teaching methods .
Ethical Dilemmas
Random assignment, while methodologically sound, can also present ethical dilemmas.
In some cases, withholding a potentially beneficial treatment from one of the groups of participants can raise serious ethical questions, especially in medical or clinical research where participants' well-being might be directly affected.
Researchers must navigate these ethical waters carefully, balancing the pursuit of knowledge with the well-being of participants.
Generalizability Concerns
Even when implemented correctly, random assignment does not always guarantee generalizable results.
The types of people in the participant pool, the specific context of the study, and the nature of the variables being studied can all influence the extent to which the findings can be applied to the broader population.
Researchers must be cautious in making broad generalizations from studies, even those employing strict random assignment.
Practical and Real-World Limitations
In the real world, many variables cannot be manipulated for ethical or practical reasons, limiting the applicability of random assignment.
For instance, researchers cannot randomly assign individuals to different levels of intelligence, socioeconomic status, or cultural backgrounds.
This limitation necessitates the use of other research designs, such as correlational or observational studies , when exploring relationships involving such variables.
Response to Critiques
In response to these critiques, people in favor of random assignment argue that the method, despite its limitations, remains one of the most reliable ways to establish cause and effect in experimental research.
They acknowledge the challenges and ethical considerations but emphasize the rigorous frameworks in place to address them.
The ongoing discussion around the limitations and critiques of random assignment contributes to the evolution of the method, making sure it is continuously relevant and applicable in psychological research.
While random assignment is a powerful tool in experimental research, it is not without its critiques and limitations. Implementation issues, ethical dilemmas, generalizability concerns, and real-world limitations can pose significant challenges.
However, the continued discourse and refinement around these issues underline the method's enduring significance in the pursuit of knowledge in psychology.
By being careful with how we do things and doing what's right, random assignment stays a really important part of studying how people act and think.
Real-World Applications and Examples
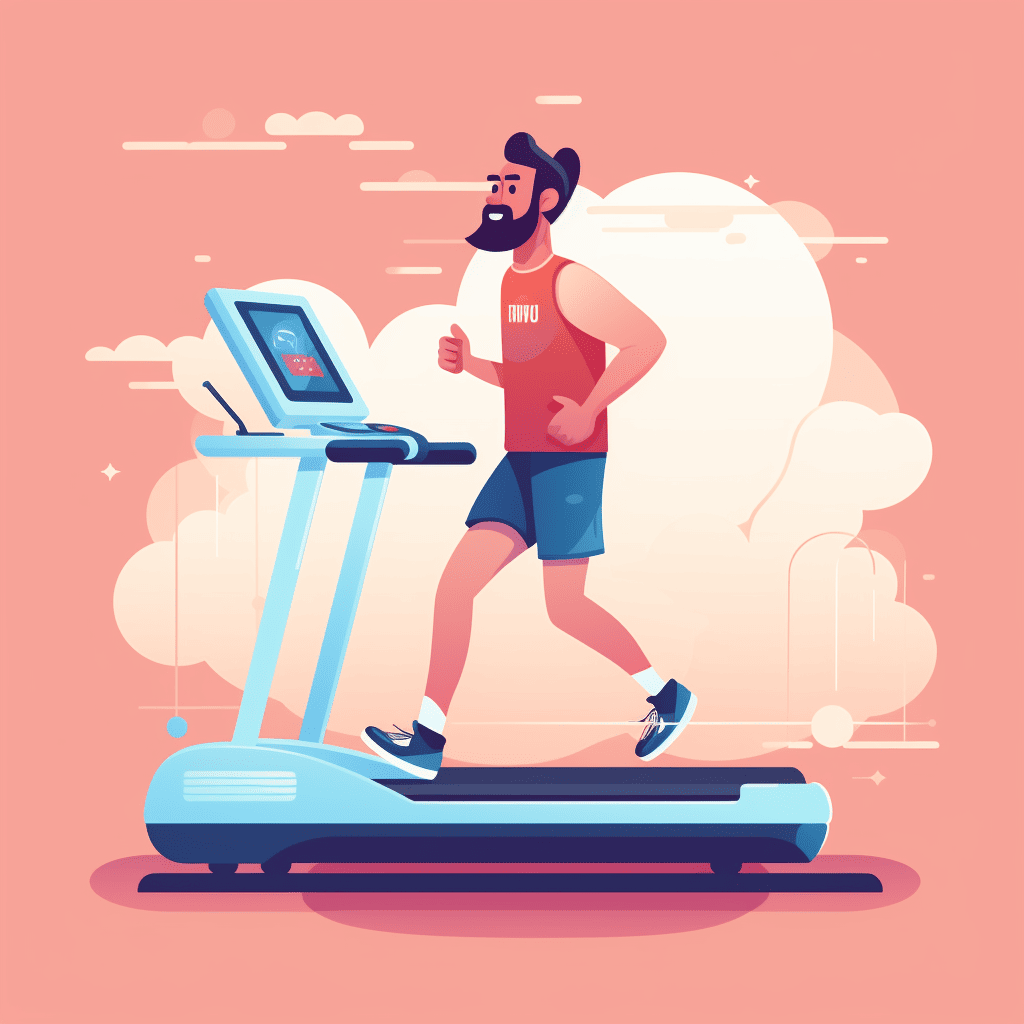
Random assignment has been employed in many studies across various fields of psychology, leading to significant discoveries and advancements.
Here are some real-world applications and examples illustrating the diversity and impact of this method:
- Medicine and Health Psychology: Randomized Controlled Trials (RCTs) are the gold standard in medical research. In these studies, participants are randomly assigned to either the treatment or control group to test the efficacy of new medications or interventions.
- Educational Psychology: Studies in this field have used random assignment to explore the effects of different teaching methods, classroom environments, and educational technologies on student learning and outcomes.
- Cognitive Psychology: Researchers have employed random assignment to investigate various aspects of human cognition, including memory, attention, and problem-solving, leading to a deeper understanding of how the mind works.
- Social Psychology: Random assignment has been instrumental in studying social phenomena, such as conformity, aggression, and prosocial behavior, shedding light on the intricate dynamics of human interaction.
Let's get into some specific examples. You'll need to know one term though, and that is "control group." A control group is a set of participants in a study who do not receive the treatment or intervention being tested , serving as a baseline to compare with the group that does, in order to assess the effectiveness of the treatment.
- Smoking Cessation Study: Researchers used random assignment to put participants into two groups. One group received a new anti-smoking program, while the other did not. This helped determine if the program was effective in helping people quit smoking.
- Math Tutoring Program: A study on students used random assignment to place them into two groups. One group received additional math tutoring, while the other continued with regular classes, to see if the extra help improved their grades.
- Exercise and Mental Health: Adults were randomly assigned to either an exercise group or a control group to study the impact of physical activity on mental health and mood.
- Diet and Weight Loss: A study randomly assigned participants to different diet plans to compare their effectiveness in promoting weight loss and improving health markers.
- Sleep and Learning: Researchers randomly assigned students to either a sleep extension group or a regular sleep group to study the impact of sleep on learning and memory.
- Classroom Seating Arrangement: Teachers used random assignment to place students in different seating arrangements to examine the effect on focus and academic performance.
- Music and Productivity: Employees were randomly assigned to listen to music or work in silence to investigate the effect of music on workplace productivity.
- Medication for ADHD: Children with ADHD were randomly assigned to receive either medication, behavioral therapy, or a placebo to compare treatment effectiveness.
- Mindfulness Meditation for Stress: Adults were randomly assigned to a mindfulness meditation group or a waitlist control group to study the impact on stress levels.
- Video Games and Aggression: A study randomly assigned participants to play either violent or non-violent video games and then measured their aggression levels.
- Online Learning Platforms: Students were randomly assigned to use different online learning platforms to evaluate their effectiveness in enhancing learning outcomes.
- Hand Sanitizers in Schools: Schools were randomly assigned to use hand sanitizers or not to study the impact on student illness and absenteeism.
- Caffeine and Alertness: Participants were randomly assigned to consume caffeinated or decaffeinated beverages to measure the effects on alertness and cognitive performance.
- Green Spaces and Well-being: Neighborhoods were randomly assigned to receive green space interventions to study the impact on residents’ well-being and community connections.
- Pet Therapy for Hospital Patients: Patients were randomly assigned to receive pet therapy or standard care to assess the impact on recovery and mood.
- Yoga for Chronic Pain: Individuals with chronic pain were randomly assigned to a yoga intervention group or a control group to study the effect on pain levels and quality of life.
- Flu Vaccines Effectiveness: Different groups of people were randomly assigned to receive either the flu vaccine or a placebo to determine the vaccine’s effectiveness.
- Reading Strategies for Dyslexia: Children with dyslexia were randomly assigned to different reading intervention strategies to compare their effectiveness.
- Physical Environment and Creativity: Participants were randomly assigned to different room setups to study the impact of physical environment on creative thinking.
- Laughter Therapy for Depression: Individuals with depression were randomly assigned to laughter therapy sessions or control groups to assess the impact on mood.
- Financial Incentives for Exercise: Participants were randomly assigned to receive financial incentives for exercising to study the impact on physical activity levels.
- Art Therapy for Anxiety: Individuals with anxiety were randomly assigned to art therapy sessions or a waitlist control group to measure the effect on anxiety levels.
- Natural Light in Offices: Employees were randomly assigned to workspaces with natural or artificial light to study the impact on productivity and job satisfaction.
- School Start Times and Academic Performance: Schools were randomly assigned different start times to study the effect on student academic performance and well-being.
- Horticulture Therapy for Seniors: Older adults were randomly assigned to participate in horticulture therapy or traditional activities to study the impact on cognitive function and life satisfaction.
- Hydration and Cognitive Function: Participants were randomly assigned to different hydration levels to measure the impact on cognitive function and alertness.
- Intergenerational Programs: Seniors and young people were randomly assigned to intergenerational programs to study the effects on well-being and cross-generational understanding.
- Therapeutic Horseback Riding for Autism: Children with autism were randomly assigned to therapeutic horseback riding or traditional therapy to study the impact on social communication skills.
- Active Commuting and Health: Employees were randomly assigned to active commuting (cycling, walking) or passive commuting to study the effect on physical health.
- Mindful Eating for Weight Management: Individuals were randomly assigned to mindful eating workshops or control groups to study the impact on weight management and eating habits.
- Noise Levels and Learning: Students were randomly assigned to classrooms with different noise levels to study the effect on learning and concentration.
- Bilingual Education Methods: Schools were randomly assigned different bilingual education methods to compare their effectiveness in language acquisition.
- Outdoor Play and Child Development: Children were randomly assigned to different amounts of outdoor playtime to study the impact on physical and cognitive development.
- Social Media Detox: Participants were randomly assigned to a social media detox or regular usage to study the impact on mental health and well-being.
- Therapeutic Writing for Trauma Survivors: Individuals who experienced trauma were randomly assigned to therapeutic writing sessions or control groups to study the impact on psychological well-being.
- Mentoring Programs for At-risk Youth: At-risk youth were randomly assigned to mentoring programs or control groups to assess the impact on academic achievement and behavior.
- Dance Therapy for Parkinson’s Disease: Individuals with Parkinson’s disease were randomly assigned to dance therapy or traditional exercise to study the effect on motor function and quality of life.
- Aquaponics in Schools: Schools were randomly assigned to implement aquaponics programs to study the impact on student engagement and environmental awareness.
- Virtual Reality for Phobia Treatment: Individuals with phobias were randomly assigned to virtual reality exposure therapy or traditional therapy to compare effectiveness.
- Gardening and Mental Health: Participants were randomly assigned to engage in gardening or other leisure activities to study the impact on mental health and stress reduction.
Each of these studies exemplifies how random assignment is utilized in various fields and settings, shedding light on the multitude of ways it can be applied to glean valuable insights and knowledge.
Real-world Impact of Random Assignment
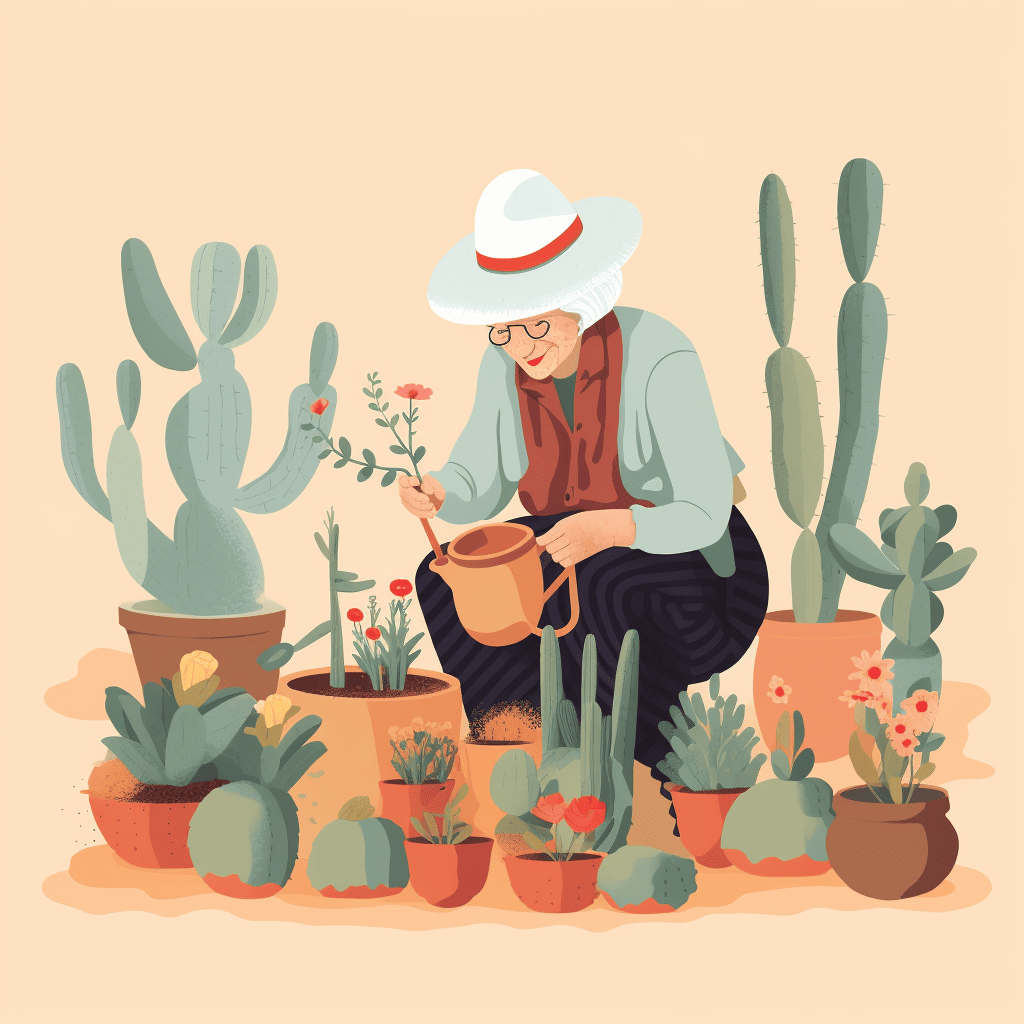
Random assignment is like a key tool in the world of learning about people's minds and behaviors. It’s super important and helps in many different areas of our everyday lives. It helps make better rules, creates new ways to help people, and is used in lots of different fields.
Health and Medicine
In health and medicine, random assignment has helped doctors and scientists make lots of discoveries. It’s a big part of tests that help create new medicines and treatments.
By putting people into different groups by chance, scientists can really see if a medicine works.
This has led to new ways to help people with all sorts of health problems, like diabetes, heart disease, and mental health issues like depression and anxiety.
Schools and education have also learned a lot from random assignment. Researchers have used it to look at different ways of teaching, what kind of classrooms are best, and how technology can help learning.
This knowledge has helped make better school rules, develop what we learn in school, and find the best ways to teach students of all ages and backgrounds.
Workplace and Organizational Behavior
Random assignment helps us understand how people act at work and what makes a workplace good or bad.
Studies have looked at different kinds of workplaces, how bosses should act, and how teams should be put together. This has helped companies make better rules and create places to work that are helpful and make people happy.
Environmental and Social Changes
Random assignment is also used to see how changes in the community and environment affect people. Studies have looked at community projects, changes to the environment, and social programs to see how they help or hurt people’s well-being.
This has led to better community projects, efforts to protect the environment, and programs to help people in society.
Technology and Human Interaction
In our world where technology is always changing, studies with random assignment help us see how tech like social media, virtual reality, and online stuff affect how we act and feel.
This has helped make better and safer technology and rules about using it so that everyone can benefit.
The effects of random assignment go far and wide, way beyond just a science lab. It helps us understand lots of different things, leads to new and improved ways to do things, and really makes a difference in the world around us.
From making healthcare and schools better to creating positive changes in communities and the environment, the real-world impact of random assignment shows just how important it is in helping us learn and make the world a better place.
So, what have we learned? Random assignment is like a super tool in learning about how people think and act. It's like a detective helping us find clues and solve mysteries in many parts of our lives.
From creating new medicines to helping kids learn better in school, and from making workplaces happier to protecting the environment, it’s got a big job!
This method isn’t just something scientists use in labs; it reaches out and touches our everyday lives. It helps make positive changes and teaches us valuable lessons.
Whether we are talking about technology, health, education, or the environment, random assignment is there, working behind the scenes, making things better and safer for all of us.
In the end, the simple act of putting people into groups by chance helps us make big discoveries and improvements. It’s like throwing a small stone into a pond and watching the ripples spread out far and wide.
Thanks to random assignment, we are always learning, growing, and finding new ways to make our world a happier and healthier place for everyone!
Related posts:
- 19+ Experimental Design Examples (Methods + Types)
- Cluster Sampling vs Stratified Sampling
- 41+ White Collar Job Examples (Salary + Path)
- 47+ Blue Collar Job Examples (Salary + Path)
- McDonaldization of Society (Definition + Examples)
Reference this article:
About The Author
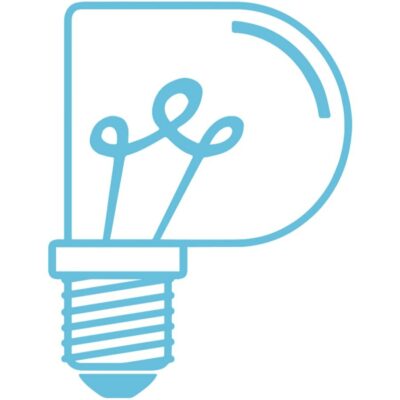
Free Personality Test
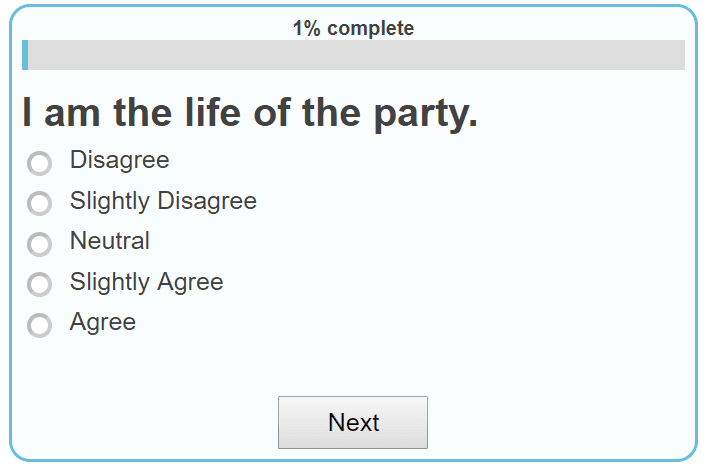
Free Memory Test
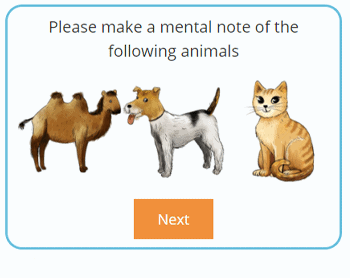
Free IQ Test
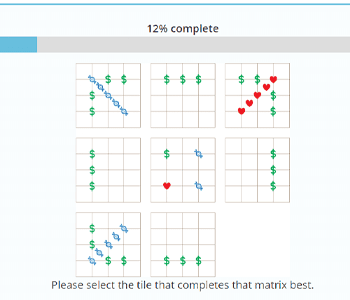
PracticalPie.com is a participant in the Amazon Associates Program. As an Amazon Associate we earn from qualifying purchases.
Follow Us On:
Youtube Facebook Instagram X/Twitter
Psychology Resources
Developmental
Personality
Relationships
Psychologists
Serial Killers
Psychology Tests
Personality Quiz
Memory Test
Depression test
Type A/B Personality Test
© PracticalPsychology. All rights reserved
Privacy Policy | Terms of Use
Pretest-Posttest Control Group Design: An Introduction
The pretest-posttest control group design , also called the pretest-posttest randomized experimental design , is a type of experiment where participants get randomly assigned to either receive an intervention (the treatment group) or not (the control group). The outcome of interest is measured 2 times, once before the treatment group gets the intervention — the pretest — and once after it — the posttest.
The objective is to measure the effect of the intervention which can be:
- A medical treatment
- An education program
- A policy change, etc.
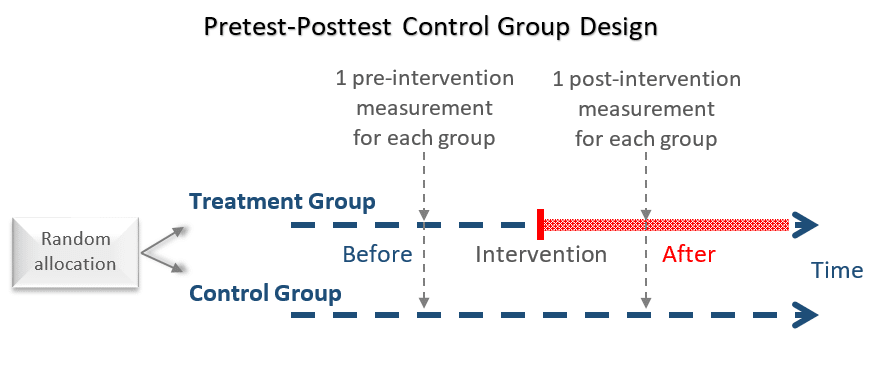
The pretest-posttest control group design has 3 major characteristics:
- The study participants are randomly assigned to either the treatment or the control group (this random assignment can occur either before of after the pretest).
- Both groups are exposed to the same conditions except for the intervention: the treatment group receives the intervention, whereas the control group does not.
- The outcome is measured simultaneously for both groups at 2 points in time — the pretest and the posttest.
The pretest-posttest control group is the most commonly used design in randomized controlled trials.
Advantages of the pretest-posttest control group design
By using a pretest, a control group, and random assignment, this design controls all internal threats to validity.
Advantage of having a pretest measurement
This design is better than the posttest-only control group design because it adds a pretest.
Adding a pretest:
- Increases the power of the design to detect an effect .
- Allows studying the effect of the intervention at different sublevels of the pretest .
- Helps analyzing initial differences between groups (and therefore quantifying their effect on the study outcome).
- Helps controlling attrition bias i.e. the unequal loss to follow-up of participants between the treatment and the control group which can affect the outcome measured at the posttest.
Advantage of using random assignment and having a control group
Random assignment and the control group will both limit the effects of:
- Selection bias : Which happens when participants themselves get to choose if they receive the intervention or not. This may create unequal and incomparable study groups. Randomization allows unbiased assignment of participants to treatment options, and therefore makes the study groups comparable.
- Maturation : Which is the effect of time (between the pretest and the posttest) on study participants (e.g. participants growing older, or getting tired over time) which might influence the outcome, thus becoming a rival explanation for the intervention regarding the study outcome. Participants are subject to maturation both in the treatment and the control group, therefore, any difference between the outcome of these groups will be due to the effect of the treatment alone and will not be affected by maturation.
- History : Which is any event that might co-occur with the intervention and has the potential to influence the outcome. Co-occurring events affect both the treatment and the control group, and therefore any difference between the outcome of these groups will be due to the effect of the treatment alone and will not be affected by history.
- Testing : Which is the effect of taking a pretest on the result of a posttest. For instance, if the pretest sensitizes participants and compels them to behave in a certain way that affects the outcome of the posttest. The presence of a control group protects against testing effects, as these will affect both groups and therefore any difference between the outcome of these groups will be due to the effect of the treatment alone and will not be affected by testing.
- Regression to the mean : When pretest scores are exceptionally good by chance, the posttest scores will naturally regress toward the mean. This happens because an exceptionally good performance is hard to maintain. Regression toward the mean can be mistaken for the effect of the treatment, and therefore is a source of bias. Since participants from both groups are subject to regression, therefore, comparing the outcome of the treatment group with that of the control group will take care of this regression effect.
Limitations of the pretest-posttest control group design
Participants included in any randomized study might not be typical people in the population i.e. they may not represent well the population of interest, this is because:
- Not everyone in the population of interest is eligible for the experiment,
- and not everyone who is eligible can be recruited ,
- and not everyone who is recruited will give us their consent to be included in the study,
- and not everyone who consented will be randomized .
So the outcome of a randomized study may not generalize well to the population .
More specifically, this design:
- Does not allow us to study how the effect of the treatment changes over time: To do so, we need to add more posttest measures.
- Is susceptible for interactions between the intervention and other factors (such as the pretest, history, instrumentation, etc.): One solution for this problem is to use the Solomon four-group design.
Example of a study that used the pretest-posttest control group design
Koenig et al. used a pretest-posttest control group design to study the effect of a yoga program on the classroom behavior of autistic children. These children were randomly assigned to either receive the yoga program or their standard morning routine.
The study concluded that yoga can significantly improve the classroom behavior of autistic children.
But because the researchers used a convenience sample from a particular school and the classrooms that were allowed to participate were hand-picked by administrators, the study outcome may not generalize well to all children with autism.
- Campbell DT, Stanley JC. Experimental and Quasi-Experimental Designs for Research . Wadsworth; 1963.
- Reichardt CS. Quasi-Experimentation: A Guide to Design and Analysis . The Guilford Press; 2019.
- Shadish WR, Cook TD, Campbell DT. Experimental and Quasi-Experimental Designs for Generalized Causal Inference . 2nd edition. Cengage Learning; 2001.
Further reading
- Purpose and Limitations of Random Assignment
- Posttest-Only Control Group Design
- Solomon Four-Group Design
- Matched Pairs Design
- Randomized Block Design
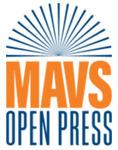
Want to create or adapt books like this? Learn more about how Pressbooks supports open publishing practices.
8.1 Experimental design: What is it and when should it be used?
Learning objectives.
- Define experiment
- Identify the core features of true experimental designs
- Describe the difference between an experimental group and a control group
- Identify and describe the various types of true experimental designs
Experiments are an excellent data collection strategy for social workers wishing to observe the effects of a clinical intervention or social welfare program. Understanding what experiments are and how they are conducted is useful for all social scientists, whether they actually plan to use this methodology or simply aim to understand findings from experimental studies. An experiment is a method of data collection designed to test hypotheses under controlled conditions. In social scientific research, the term experiment has a precise meaning and should not be used to describe all research methodologies.
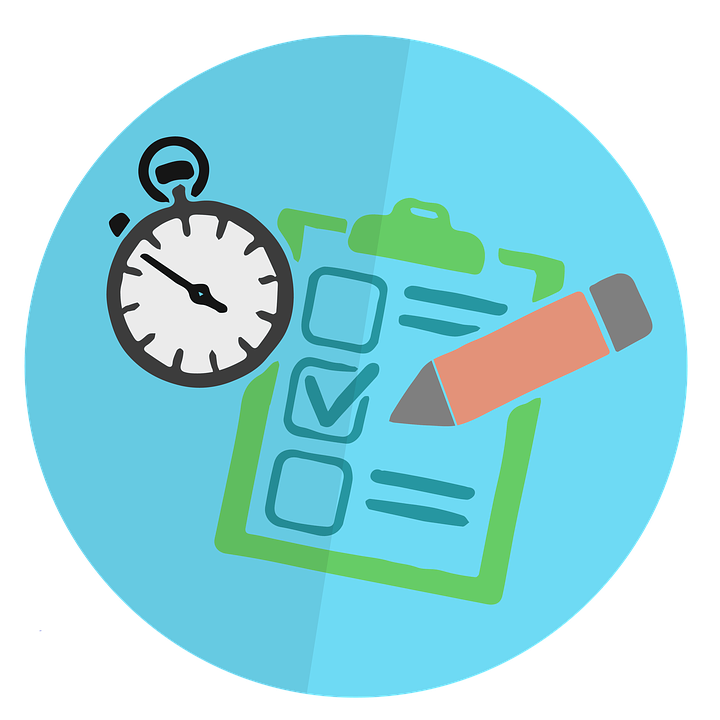
Experiments have a long and important history in social science. Behaviorists such as John Watson, B. F. Skinner, Ivan Pavlov, and Albert Bandura used experimental design to demonstrate the various types of conditioning. Using strictly controlled environments, behaviorists were able to isolate a single stimulus as the cause of measurable differences in behavior or physiological responses. The foundations of social learning theory and behavior modification are found in experimental research projects. Moreover, behaviorist experiments brought psychology and social science away from the abstract world of Freudian analysis and towards empirical inquiry, grounded in real-world observations and objectively-defined variables. Experiments are used at all levels of social work inquiry, including agency-based experiments that test therapeutic interventions and policy experiments that test new programs.
Several kinds of experimental designs exist. In general, designs considered to be true experiments contain three basic key features:
- random assignment of participants into experimental and control groups
- a “treatment” (or intervention) provided to the experimental group
- measurement of the effects of the treatment in a post-test administered to both groups
Some true experiments are more complex. Their designs can also include a pre-test and can have more than two groups, but these are the minimum requirements for a design to be a true experiment.
Experimental and control groups
In a true experiment, the effect of an intervention is tested by comparing two groups: one that is exposed to the intervention (the experimental group , also known as the treatment group) and another that does not receive the intervention (the control group ). Importantly, participants in a true experiment need to be randomly assigned to either the control or experimental groups. Random assignment uses a random number generator or some other random process to assign people into experimental and control groups. Random assignment is important in experimental research because it helps to ensure that the experimental group and control group are comparable and that any differences between the experimental and control groups are due to random chance. We will address more of the logic behind random assignment in the next section.
Treatment or intervention
In an experiment, the independent variable is receiving the intervention being tested—for example, a therapeutic technique, prevention program, or access to some service or support. It is less common in of social work research, but social science research may also have a stimulus, rather than an intervention as the independent variable. For example, an electric shock or a reading about death might be used as a stimulus to provoke a response.
In some cases, it may be immoral to withhold treatment completely from a control group within an experiment. If you recruited two groups of people with severe addiction and only provided treatment to one group, the other group would likely suffer. For these cases, researchers use a control group that receives “treatment as usual.” Experimenters must clearly define what treatment as usual means. For example, a standard treatment in substance abuse recovery is attending Alcoholics Anonymous or Narcotics Anonymous meetings. A substance abuse researcher conducting an experiment may use twelve-step programs in their control group and use their experimental intervention in the experimental group. The results would show whether the experimental intervention worked better than normal treatment, which is useful information.
The dependent variable is usually the intended effect the researcher wants the intervention to have. If the researcher is testing a new therapy for individuals with binge eating disorder, their dependent variable may be the number of binge eating episodes a participant reports. The researcher likely expects her intervention to decrease the number of binge eating episodes reported by participants. Thus, she must, at a minimum, measure the number of episodes that occur after the intervention, which is the post-test . In a classic experimental design, participants are also given a pretest to measure the dependent variable before the experimental treatment begins.
Types of experimental design
Let’s put these concepts in chronological order so we can better understand how an experiment runs from start to finish. Once you’ve collected your sample, you’ll need to randomly assign your participants to the experimental group and control group. In a common type of experimental design, you will then give both groups your pretest, which measures your dependent variable, to see what your participants are like before you start your intervention. Next, you will provide your intervention, or independent variable, to your experimental group, but not to your control group. Many interventions last a few weeks or months to complete, particularly therapeutic treatments. Finally, you will administer your post-test to both groups to observe any changes in your dependent variable. What we’ve just described is known as the classical experimental design and is the simplest type of true experimental design. All of the designs we review in this section are variations on this approach. Figure 8.1 visually represents these steps.

An interesting example of experimental research can be found in Shannon K. McCoy and Brenda Major’s (2003) study of people’s perceptions of prejudice. In one portion of this multifaceted study, all participants were given a pretest to assess their levels of depression. No significant differences in depression were found between the experimental and control groups during the pretest. Participants in the experimental group were then asked to read an article suggesting that prejudice against their own racial group is severe and pervasive, while participants in the control group were asked to read an article suggesting that prejudice against a racial group other than their own is severe and pervasive. Clearly, these were not meant to be interventions or treatments to help depression, but were stimuli designed to elicit changes in people’s depression levels. Upon measuring depression scores during the post-test period, the researchers discovered that those who had received the experimental stimulus (the article citing prejudice against their same racial group) reported greater depression than those in the control group. This is just one of many examples of social scientific experimental research.
In addition to classic experimental design, there are two other ways of designing experiments that are considered to fall within the purview of “true” experiments (Babbie, 2010; Campbell & Stanley, 1963). The posttest-only control group design is almost the same as classic experimental design, except it does not use a pretest. Researchers who use posttest-only designs want to eliminate testing effects , in which participants’ scores on a measure change because they have already been exposed to it. If you took multiple SAT or ACT practice exams before you took the real one you sent to colleges, you’ve taken advantage of testing effects to get a better score. Considering the previous example on racism and depression, participants who are given a pretest about depression before being exposed to the stimulus would likely assume that the intervention is designed to address depression. That knowledge could cause them to answer differently on the post-test than they otherwise would. In theory, as long as the control and experimental groups have been determined randomly and are therefore comparable, no pretest is needed. However, most researchers prefer to use pretests in case randomization did not result in equivalent groups and to help assess change over time within both the experimental and control groups.
Researchers wishing to account for testing effects but also gather pretest data can use a Solomon four-group design. In the Solomon four-group design , the researcher uses four groups. Two groups are treated as they would be in a classic experiment—pretest, experimental group intervention, and post-test. The other two groups do not receive the pretest, though one receives the intervention. All groups are given the post-test. Table 8.1 illustrates the features of each of the four groups in the Solomon four-group design. By having one set of experimental and control groups that complete the pretest (Groups 1 and 2) and another set that does not complete the pretest (Groups 3 and 4), researchers using the Solomon four-group design can account for testing effects in their analysis.
Group 1 | X | X | X |
Group 2 | X | X | |
Group 3 | X | X | |
Group 4 | X |
Solomon four-group designs are challenging to implement in the real world because they are time- and resource-intensive. Researchers must recruit enough participants to create four groups and implement interventions in two of them.
Overall, true experimental designs are sometimes difficult to implement in a real-world practice environment. It may be impossible to withhold treatment from a control group or randomly assign participants in a study. In these cases, pre-experimental and quasi-experimental designs–which we will discuss in the next section–can be used. However, the differences in rigor from true experimental designs leave their conclusions more open to critique.
Experimental design in macro-level research
You can imagine that social work researchers may be limited in their ability to use random assignment when examining the effects of governmental policy on individuals. For example, it is unlikely that a researcher could randomly assign some states to implement decriminalization of recreational marijuana and some states not to in order to assess the effects of the policy change. There are, however, important examples of policy experiments that use random assignment, including the Oregon Medicaid experiment. In the Oregon Medicaid experiment, the wait list for Oregon was so long, state officials conducted a lottery to see who from the wait list would receive Medicaid (Baicker et al., 2013). Researchers used the lottery as a natural experiment that included random assignment. People selected to be a part of Medicaid were the experimental group and those on the wait list were in the control group. There are some practical complications macro-level experiments, just as with other experiments. For example, the ethical concern with using people on a wait list as a control group exists in macro-level research just as it does in micro-level research.
Key Takeaways
- True experimental designs require random assignment.
- Control groups do not receive an intervention, and experimental groups receive an intervention.
- The basic components of a true experiment include a pretest, posttest, control group, and experimental group.
- Testing effects may cause researchers to use variations on the classic experimental design.
- Classic experimental design- uses random assignment, an experimental and control group, as well as pre- and posttesting
- Control group- the group in an experiment that does not receive the intervention
- Experiment- a method of data collection designed to test hypotheses under controlled conditions
- Experimental group- the group in an experiment that receives the intervention
- Posttest- a measurement taken after the intervention
- Posttest-only control group design- a type of experimental design that uses random assignment, and an experimental and control group, but does not use a pretest
- Pretest- a measurement taken prior to the intervention
- Random assignment-using a random process to assign people into experimental and control groups
- Solomon four-group design- uses random assignment, two experimental and two control groups, pretests for half of the groups, and posttests for all
- Testing effects- when a participant’s scores on a measure change because they have already been exposed to it
- True experiments- a group of experimental designs that contain independent and dependent variables, pretesting and post testing, and experimental and control groups
Image attributions
exam scientific experiment by mohamed_hassan CC-0
Foundations of Social Work Research Copyright © 2020 by Rebecca L. Mauldin is licensed under a Creative Commons Attribution-NonCommercial-ShareAlike 4.0 International License , except where otherwise noted.
Share This Book
Chapter 6: Experimental Research
6.2 experimental design, learning objectives.
- Explain the difference between between-subjects and within-subjects experiments, list some of the pros and cons of each approach, and decide which approach to use to answer a particular research question.
- Define random assignment, distinguish it from random sampling, explain its purpose in experimental research, and use some simple strategies to implement it.
- Define what a control condition is, explain its purpose in research on treatment effectiveness, and describe some alternative types of control conditions.
- Define several types of carryover effect, give examples of each, and explain how counterbalancing helps to deal with them.
In this section, we look at some different ways to design an experiment. The primary distinction we will make is between approaches in which each participant experiences one level of the independent variable and approaches in which each participant experiences all levels of the independent variable. The former are called between-subjects experiments and the latter are called within-subjects experiments.
Between-Subjects Experiments
In a between-subjects experiment , each participant is tested in only one condition. For example, a researcher with a sample of 100 college students might assign half of them to write about a traumatic event and the other half write about a neutral event. Or a researcher with a sample of 60 people with severe agoraphobia (fear of open spaces) might assign 20 of them to receive each of three different treatments for that disorder. It is essential in a between-subjects experiment that the researcher assign participants to conditions so that the different groups are, on average, highly similar to each other. Those in a trauma condition and a neutral condition, for example, should include a similar proportion of men and women, and they should have similar average intelligence quotients (IQs), similar average levels of motivation, similar average numbers of health problems, and so on. This is a matter of controlling these extraneous participant variables across conditions so that they do not become confounding variables.
Random Assignment
The primary way that researchers accomplish this kind of control of extraneous variables across conditions is called random assignment , which means using a random process to decide which participants are tested in which conditions. Do not confuse random assignment with random sampling. Random sampling is a method for selecting a sample from a population, and it is rarely used in psychological research. Random assignment is a method for assigning participants in a sample to the different conditions, and it is an important element of all experimental research in psychology and other fields too.
In its strictest sense, random assignment should meet two criteria. One is that each participant has an equal chance of being assigned to each condition (e.g., a 50% chance of being assigned to each of two conditions). The second is that each participant is assigned to a condition independently of other participants. Thus one way to assign participants to two conditions would be to flip a coin for each one. If the coin lands heads, the participant is assigned to Condition A, and if it lands tails, the participant is assigned to Condition B. For three conditions, one could use a computer to generate a random integer from 1 to 3 for each participant. If the integer is 1, the participant is assigned to Condition A; if it is 2, the participant is assigned to Condition B; and if it is 3, the participant is assigned to Condition C. In practice, a full sequence of conditions—one for each participant expected to be in the experiment—is usually created ahead of time, and each new participant is assigned to the next condition in the sequence as he or she is tested. When the procedure is computerized, the computer program often handles the random assignment.
One problem with coin flipping and other strict procedures for random assignment is that they are likely to result in unequal sample sizes in the different conditions. Unequal sample sizes are generally not a serious problem, and you should never throw away data you have already collected to achieve equal sample sizes. However, for a fixed number of participants, it is statistically most efficient to divide them into equal-sized groups. It is standard practice, therefore, to use a kind of modified random assignment that keeps the number of participants in each group as similar as possible. One approach is block randomization . In block randomization, all the conditions occur once in the sequence before any of them is repeated. Then they all occur again before any of them is repeated again. Within each of these “blocks,” the conditions occur in a random order. Again, the sequence of conditions is usually generated before any participants are tested, and each new participant is assigned to the next condition in the sequence. Table 6.2 “Block Randomization Sequence for Assigning Nine Participants to Three Conditions” shows such a sequence for assigning nine participants to three conditions. The Research Randomizer website ( http://www.randomizer.org ) will generate block randomization sequences for any number of participants and conditions. Again, when the procedure is computerized, the computer program often handles the block randomization.
Table 6.2 Block Randomization Sequence for Assigning Nine Participants to Three Conditions
Participant | Condition |
---|---|
4 | B |
5 | C |
6 | A |
Random assignment is not guaranteed to control all extraneous variables across conditions. It is always possible that just by chance, the participants in one condition might turn out to be substantially older, less tired, more motivated, or less depressed on average than the participants in another condition. However, there are some reasons that this is not a major concern. One is that random assignment works better than one might expect, especially for large samples. Another is that the inferential statistics that researchers use to decide whether a difference between groups reflects a difference in the population takes the “fallibility” of random assignment into account. Yet another reason is that even if random assignment does result in a confounding variable and therefore produces misleading results, this is likely to be detected when the experiment is replicated. The upshot is that random assignment to conditions—although not infallible in terms of controlling extraneous variables—is always considered a strength of a research design.
Treatment and Control Conditions
Between-subjects experiments are often used to determine whether a treatment works. In psychological research, a treatment is any intervention meant to change people’s behavior for the better. This includes psychotherapies and medical treatments for psychological disorders but also interventions designed to improve learning, promote conservation, reduce prejudice, and so on. To determine whether a treatment works, participants are randomly assigned to either a treatment condition , in which they receive the treatment, or a control condition , in which they do not receive the treatment. If participants in the treatment condition end up better off than participants in the control condition—for example, they are less depressed, learn faster, conserve more, express less prejudice—then the researcher can conclude that the treatment works. In research on the effectiveness of psychotherapies and medical treatments, this type of experiment is often called a randomized clinical trial .
There are different types of control conditions. In a no-treatment control condition , participants receive no treatment whatsoever. One problem with this approach, however, is the existence of placebo effects. A placebo is a simulated treatment that lacks any active ingredient or element that should make it effective, and a placebo effect is a positive effect of such a treatment. Many folk remedies that seem to work—such as eating chicken soup for a cold or placing soap under the bedsheets to stop nighttime leg cramps—are probably nothing more than placebos. Although placebo effects are not well understood, they are probably driven primarily by people’s expectations that they will improve. Having the expectation to improve can result in reduced stress, anxiety, and depression, which can alter perceptions and even improve immune system functioning (Price, Finniss, & Benedetti, 2008).
Placebo effects are interesting in their own right (see Note 6.28 “The Powerful Placebo” ), but they also pose a serious problem for researchers who want to determine whether a treatment works. Figure 6.2 “Hypothetical Results From a Study Including Treatment, No-Treatment, and Placebo Conditions” shows some hypothetical results in which participants in a treatment condition improved more on average than participants in a no-treatment control condition. If these conditions (the two leftmost bars in Figure 6.2 “Hypothetical Results From a Study Including Treatment, No-Treatment, and Placebo Conditions” ) were the only conditions in this experiment, however, one could not conclude that the treatment worked. It could be instead that participants in the treatment group improved more because they expected to improve, while those in the no-treatment control condition did not.
Figure 6.2 Hypothetical Results From a Study Including Treatment, No-Treatment, and Placebo Conditions
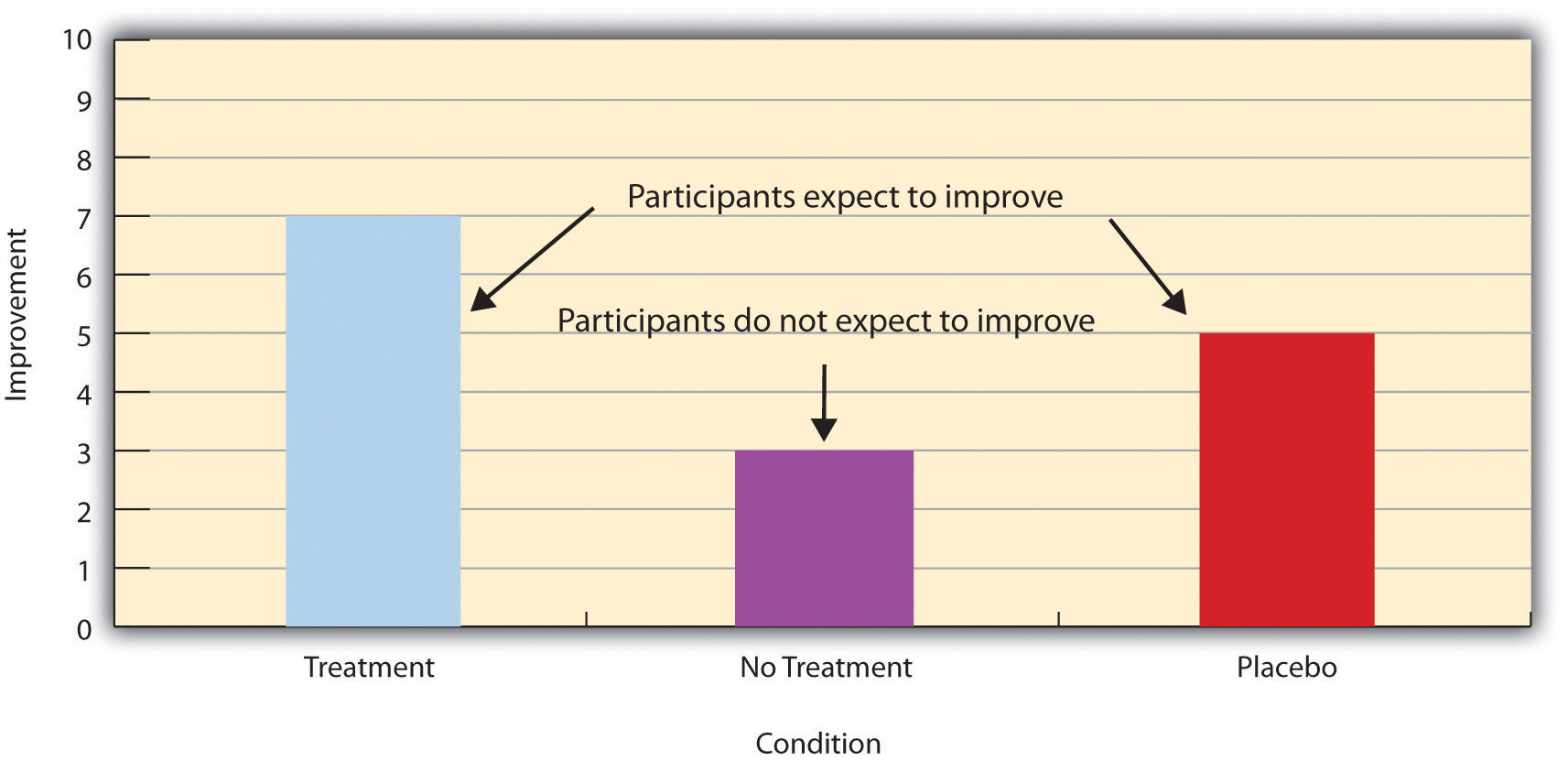
Fortunately, there are several solutions to this problem. One is to include a placebo control condition , in which participants receive a placebo that looks much like the treatment but lacks the active ingredient or element thought to be responsible for the treatment’s effectiveness. When participants in a treatment condition take a pill, for example, then those in a placebo control condition would take an identical-looking pill that lacks the active ingredient in the treatment (a “sugar pill”). In research on psychotherapy effectiveness, the placebo might involve going to a psychotherapist and talking in an unstructured way about one’s problems. The idea is that if participants in both the treatment and the placebo control groups expect to improve, then any improvement in the treatment group over and above that in the placebo control group must have been caused by the treatment and not by participants’ expectations. This is what is shown by a comparison of the two outer bars in Figure 6.2 “Hypothetical Results From a Study Including Treatment, No-Treatment, and Placebo Conditions” .
Of course, the principle of informed consent requires that participants be told that they will be assigned to either a treatment or a placebo control condition—even though they cannot be told which until the experiment ends. In many cases the participants who had been in the control condition are then offered an opportunity to have the real treatment. An alternative approach is to use a waitlist control condition , in which participants are told that they will receive the treatment but must wait until the participants in the treatment condition have already received it. This allows researchers to compare participants who have received the treatment with participants who are not currently receiving it but who still expect to improve (eventually). A final solution to the problem of placebo effects is to leave out the control condition completely and compare any new treatment with the best available alternative treatment. For example, a new treatment for simple phobia could be compared with standard exposure therapy. Because participants in both conditions receive a treatment, their expectations about improvement should be similar. This approach also makes sense because once there is an effective treatment, the interesting question about a new treatment is not simply “Does it work?” but “Does it work better than what is already available?”
The Powerful Placebo
Many people are not surprised that placebos can have a positive effect on disorders that seem fundamentally psychological, including depression, anxiety, and insomnia. However, placebos can also have a positive effect on disorders that most people think of as fundamentally physiological. These include asthma, ulcers, and warts (Shapiro & Shapiro, 1999). There is even evidence that placebo surgery—also called “sham surgery”—can be as effective as actual surgery.
Medical researcher J. Bruce Moseley and his colleagues conducted a study on the effectiveness of two arthroscopic surgery procedures for osteoarthritis of the knee (Moseley et al., 2002). The control participants in this study were prepped for surgery, received a tranquilizer, and even received three small incisions in their knees. But they did not receive the actual arthroscopic surgical procedure. The surprising result was that all participants improved in terms of both knee pain and function, and the sham surgery group improved just as much as the treatment groups. According to the researchers, “This study provides strong evidence that arthroscopic lavage with or without débridement [the surgical procedures used] is not better than and appears to be equivalent to a placebo procedure in improving knee pain and self-reported function” (p. 85).
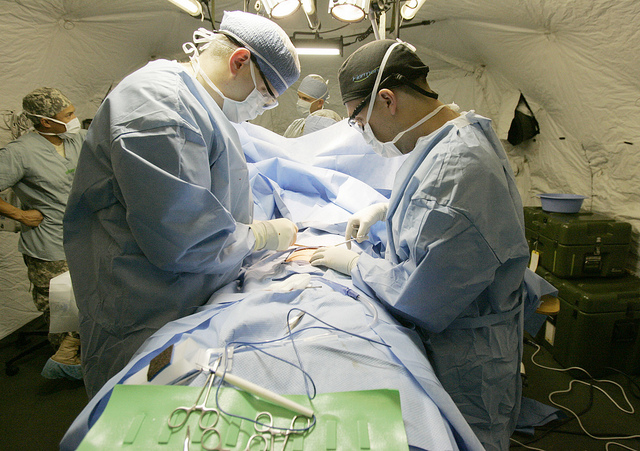
Research has shown that patients with osteoarthritis of the knee who receive a “sham surgery” experience reductions in pain and improvement in knee function similar to those of patients who receive a real surgery.
Army Medicine – Surgery – CC BY 2.0.
Within-Subjects Experiments
In a within-subjects experiment , each participant is tested under all conditions. Consider an experiment on the effect of a defendant’s physical attractiveness on judgments of his guilt. Again, in a between-subjects experiment, one group of participants would be shown an attractive defendant and asked to judge his guilt, and another group of participants would be shown an unattractive defendant and asked to judge his guilt. In a within-subjects experiment, however, the same group of participants would judge the guilt of both an attractive and an unattractive defendant.
The primary advantage of this approach is that it provides maximum control of extraneous participant variables. Participants in all conditions have the same mean IQ, same socioeconomic status, same number of siblings, and so on—because they are the very same people. Within-subjects experiments also make it possible to use statistical procedures that remove the effect of these extraneous participant variables on the dependent variable and therefore make the data less “noisy” and the effect of the independent variable easier to detect. We will look more closely at this idea later in the book.
Carryover Effects and Counterbalancing
The primary disadvantage of within-subjects designs is that they can result in carryover effects. A carryover effect is an effect of being tested in one condition on participants’ behavior in later conditions. One type of carryover effect is a practice effect , where participants perform a task better in later conditions because they have had a chance to practice it. Another type is a fatigue effect , where participants perform a task worse in later conditions because they become tired or bored. Being tested in one condition can also change how participants perceive stimuli or interpret their task in later conditions. This is called a context effect . For example, an average-looking defendant might be judged more harshly when participants have just judged an attractive defendant than when they have just judged an unattractive defendant. Within-subjects experiments also make it easier for participants to guess the hypothesis. For example, a participant who is asked to judge the guilt of an attractive defendant and then is asked to judge the guilt of an unattractive defendant is likely to guess that the hypothesis is that defendant attractiveness affects judgments of guilt. This could lead the participant to judge the unattractive defendant more harshly because he thinks this is what he is expected to do. Or it could make participants judge the two defendants similarly in an effort to be “fair.”
Carryover effects can be interesting in their own right. (Does the attractiveness of one person depend on the attractiveness of other people that we have seen recently?) But when they are not the focus of the research, carryover effects can be problematic. Imagine, for example, that participants judge the guilt of an attractive defendant and then judge the guilt of an unattractive defendant. If they judge the unattractive defendant more harshly, this might be because of his unattractiveness. But it could be instead that they judge him more harshly because they are becoming bored or tired. In other words, the order of the conditions is a confounding variable. The attractive condition is always the first condition and the unattractive condition the second. Thus any difference between the conditions in terms of the dependent variable could be caused by the order of the conditions and not the independent variable itself.
There is a solution to the problem of order effects, however, that can be used in many situations. It is counterbalancing , which means testing different participants in different orders. For example, some participants would be tested in the attractive defendant condition followed by the unattractive defendant condition, and others would be tested in the unattractive condition followed by the attractive condition. With three conditions, there would be six different orders (ABC, ACB, BAC, BCA, CAB, and CBA), so some participants would be tested in each of the six orders. With counterbalancing, participants are assigned to orders randomly, using the techniques we have already discussed. Thus random assignment plays an important role in within-subjects designs just as in between-subjects designs. Here, instead of randomly assigning to conditions, they are randomly assigned to different orders of conditions. In fact, it can safely be said that if a study does not involve random assignment in one form or another, it is not an experiment.
There are two ways to think about what counterbalancing accomplishes. One is that it controls the order of conditions so that it is no longer a confounding variable. Instead of the attractive condition always being first and the unattractive condition always being second, the attractive condition comes first for some participants and second for others. Likewise, the unattractive condition comes first for some participants and second for others. Thus any overall difference in the dependent variable between the two conditions cannot have been caused by the order of conditions. A second way to think about what counterbalancing accomplishes is that if there are carryover effects, it makes it possible to detect them. One can analyze the data separately for each order to see whether it had an effect.
When 9 Is “Larger” Than 221
Researcher Michael Birnbaum has argued that the lack of context provided by between-subjects designs is often a bigger problem than the context effects created by within-subjects designs. To demonstrate this, he asked one group of participants to rate how large the number 9 was on a 1-to-10 rating scale and another group to rate how large the number 221 was on the same 1-to-10 rating scale (Birnbaum, 1999). Participants in this between-subjects design gave the number 9 a mean rating of 5.13 and the number 221 a mean rating of 3.10. In other words, they rated 9 as larger than 221! According to Birnbaum, this is because participants spontaneously compared 9 with other one-digit numbers (in which case it is relatively large) and compared 221 with other three-digit numbers (in which case it is relatively small).
Simultaneous Within-Subjects Designs
So far, we have discussed an approach to within-subjects designs in which participants are tested in one condition at a time. There is another approach, however, that is often used when participants make multiple responses in each condition. Imagine, for example, that participants judge the guilt of 10 attractive defendants and 10 unattractive defendants. Instead of having people make judgments about all 10 defendants of one type followed by all 10 defendants of the other type, the researcher could present all 20 defendants in a sequence that mixed the two types. The researcher could then compute each participant’s mean rating for each type of defendant. Or imagine an experiment designed to see whether people with social anxiety disorder remember negative adjectives (e.g., “stupid,” “incompetent”) better than positive ones (e.g., “happy,” “productive”). The researcher could have participants study a single list that includes both kinds of words and then have them try to recall as many words as possible. The researcher could then count the number of each type of word that was recalled. There are many ways to determine the order in which the stimuli are presented, but one common way is to generate a different random order for each participant.
Between-Subjects or Within-Subjects?
Almost every experiment can be conducted using either a between-subjects design or a within-subjects design. This means that researchers must choose between the two approaches based on their relative merits for the particular situation.
Between-subjects experiments have the advantage of being conceptually simpler and requiring less testing time per participant. They also avoid carryover effects without the need for counterbalancing. Within-subjects experiments have the advantage of controlling extraneous participant variables, which generally reduces noise in the data and makes it easier to detect a relationship between the independent and dependent variables.
A good rule of thumb, then, is that if it is possible to conduct a within-subjects experiment (with proper counterbalancing) in the time that is available per participant—and you have no serious concerns about carryover effects—this is probably the best option. If a within-subjects design would be difficult or impossible to carry out, then you should consider a between-subjects design instead. For example, if you were testing participants in a doctor’s waiting room or shoppers in line at a grocery store, you might not have enough time to test each participant in all conditions and therefore would opt for a between-subjects design. Or imagine you were trying to reduce people’s level of prejudice by having them interact with someone of another race. A within-subjects design with counterbalancing would require testing some participants in the treatment condition first and then in a control condition. But if the treatment works and reduces people’s level of prejudice, then they would no longer be suitable for testing in the control condition. This is true for many designs that involve a treatment meant to produce long-term change in participants’ behavior (e.g., studies testing the effectiveness of psychotherapy). Clearly, a between-subjects design would be necessary here.
Remember also that using one type of design does not preclude using the other type in a different study. There is no reason that a researcher could not use both a between-subjects design and a within-subjects design to answer the same research question. In fact, professional researchers often do exactly this.
Key Takeaways
- Experiments can be conducted using either between-subjects or within-subjects designs. Deciding which to use in a particular situation requires careful consideration of the pros and cons of each approach.
- Random assignment to conditions in between-subjects experiments or to orders of conditions in within-subjects experiments is a fundamental element of experimental research. Its purpose is to control extraneous variables so that they do not become confounding variables.
- Experimental research on the effectiveness of a treatment requires both a treatment condition and a control condition, which can be a no-treatment control condition, a placebo control condition, or a waitlist control condition. Experimental treatments can also be compared with the best available alternative.
Discussion: For each of the following topics, list the pros and cons of a between-subjects and within-subjects design and decide which would be better.
- You want to test the relative effectiveness of two training programs for running a marathon.
- Using photographs of people as stimuli, you want to see if smiling people are perceived as more intelligent than people who are not smiling.
- In a field experiment, you want to see if the way a panhandler is dressed (neatly vs. sloppily) affects whether or not passersby give him any money.
- You want to see if concrete nouns (e.g., dog ) are recalled better than abstract nouns (e.g., truth ).
- Discussion: Imagine that an experiment shows that participants who receive psychodynamic therapy for a dog phobia improve more than participants in a no-treatment control group. Explain a fundamental problem with this research design and at least two ways that it might be corrected.
Birnbaum, M. H. (1999). How to show that 9 > 221: Collect judgments in a between-subjects design. Psychological Methods, 4 , 243–249.
Moseley, J. B., O’Malley, K., Petersen, N. J., Menke, T. J., Brody, B. A., Kuykendall, D. H., … Wray, N. P. (2002). A controlled trial of arthroscopic surgery for osteoarthritis of the knee. The New England Journal of Medicine, 347 , 81–88.
Price, D. D., Finniss, D. G., & Benedetti, F. (2008). A comprehensive review of the placebo effect: Recent advances and current thought. Annual Review of Psychology, 59 , 565–590.
Shapiro, A. K., & Shapiro, E. (1999). The powerful placebo: From ancient priest to modern physician . Baltimore, MD: Johns Hopkins University Press.
- Research Methods in Psychology. Provided by : University of Minnesota Libraries Publishing. Located at : http://open.lib.umn.edu/psychologyresearchmethods . License : CC BY-NC-SA: Attribution-NonCommercial-ShareAlike
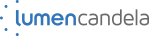
Privacy Policy
The Virtues and Limitations of Randomized Experiments
- Published: 13 October 2021
- Volume 37 , pages 453–470, ( 2022 )
Cite this article
- Tudor M. Baetu ORCID: orcid.org/0000-0002-5544-1773 1
391 Accesses
2 Citations
Explore all metrics
Despite the consensus promoted by the evidence-based medicine framework, many authors continue to express doubts about the superiority of randomized controlled trials. This paper evaluates four objections targeting the legitimacy, feasibility, and extrapolation problems linked to the experimental practice of random allocation. I argue that random allocation is a methodologically sound and feasible practice contributing to the internal validity of controlled experiments dealing with heterogeneous populations. I emphasize, however, that random allocation is solely designed to ensure the validity of causal inferences at the level of groups. By itself, random allocation cannot enhance test precision, doesn’t contribute to external validity, and limits the applicability of causal claims to individuals.
This is a preview of subscription content, log in via an institution to check access.
Access this article
Price includes VAT (Russian Federation)
Instant access to the full article PDF.
Rent this article via DeepDyve
Institutional subscriptions

Similar content being viewed by others
Blinding and Randomization
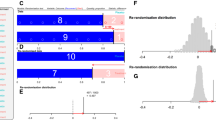
Causal inference in biomedical research
Randomized Trials and Observational Studies: The Current Philosophical Controversy
For the purposes of the present discussion, we may treat the outcome “stress” as an operationalized variable and ignore issues related to its physical interpretation, such as the reality to which the variable ultimately refers and the extent to which the assessment method accurately measures this reality.
“One of the continuing appeals of deterministic methods for case study researchers is the power of the methods. For example, Mill’s Method of Difference can determine causality with only two observations. This power can only be obtained by assuming that the observation with the antecedent of interest, A, B, C and the one without, B, C are exactly alike except for the manipulation of A, and by assuming deterministic causation and the absence of measurement error and interactions among antecedents” (Sekhon 2008 , 286–87).
Jaynes further generalizes the dilemma via an argument from infinite regression: “any specific experiment for which the existence of a physical probability is asserted is subject to physical analysis […] which will lead eventually to an understanding of its mechanism. But as soon as this understanding is reached, then this new experiment will also appear as an exceptional case […] where physical considerations obviate the usual postulates of physical probabilities” (2003, 324).
The intraclass correlation coefficient ρ is the ratio of between-group variance and total, within and between-group variance. Values of ρ range from 0, corresponding to no correlation of outcomes within a group (outcomes within and between the group are independent), to 1, corresponding to a situation when all individual outcomes within a cluster are identical (sample size is reduced to the number of clusters rather than the number of individuals). As ρ increases, the sample size required to detect a significant difference for the variable under investigation also increases.
Altman, D. G. (1985). Comparability of randomized groups. The Statisticia, 341 , 125–136.
Article Google Scholar
Altman, D. G., & Bland, J. M. (1999). Treatment allocation in controlled trials: Why randomise. British Medical Journal, 318 , 1209.
Andersen, H. (2012). Mechanisms: What are they evidence for in evidence-based medicine? Journal of Evaluation in Clinical Practice, 18 (5), 992–999.
Bowers, D. 2014. Medical statistics from scratch: An introduction for health professionals . Hoboken, NJ: Wiley.
Button, K. S., Ioannidis, J. P. A., et al. (2013). Power failure: Why small sample size undermines the reliability of neuroscience. Nature Reviews Neuroscience, 14 , 365–376.
Cartwright, N. (2010). What are randomised controlled trials food for? Philosophical Studies, 147 , 59–70.
Chalmers, T. C., Celano, P., et al. (1983). Bias in treatment assignment in controlled clinical trials. New England Journal of Medicine, 309 (22), 1359–1361.
Deaton, A., & Cartwright, N. (2018). Understanding and misunderstanding randomized controlled trials. Social Science & Medicine, 210 , 2–21.
Diaconis, P., Holmes, S., et al. (2007). Dynamical bias in the coin toss. SIAM Review, 49 (2), 211–235.
Donner, A., & Klar, N. (2004). Pitfalls of and controversies in cluster randomization trials. American Journal of Public Health, 94 (3), 416–422.
Feinstein, A. R., & Horwitz, I. R. (1997). Problems in the “evidence” of “evidence-based medicine.” American Journal of Medicine, 103 , 529–535.
Fisher, R. A. 1947. The design ol experiments . Fourth edition ed. Edinburgh: Oliver and Boyd.
Fuller, J., & Flores, L. (2015). The risk GP model: The standard model of prediction in medicine. Studies in History and Philosophy of Biological and Biomedical Sciences, 54 , 49–61.
Godlee, F. (2007). Milestones on the long road to knowledge. BMJ, 334 (suppl 1), s2–s3.
Godwin, M., L. Ruhland, et al. 2003. “Pragmatic controlled clinical trials in primary care: The struggle between external and internal validity.” BMC Medical Research Methodology 3 (28): https://doi.org/10.1186/471-2288-3-28 .
Greenfield, S., Kravitz, R., et al. (2007). Heterogeneity of treatment effects: Implications for guidelines, payment, and quality assessment. American Journal of Medicine, 120 , S3–S9.
Guyatt, G., and B. Djulbegovic. 2019. “Evidence-based medicine and the theory of knowledge.” In Users’ guides to the medical literature: A manual for evidence-based clinical practice , ed. G. Guyatt, D. Rennie, M. O. Meade and D. J. Cook. New Yotk, NY: JAMA/McGraw-Hill Education.
Guyatt, G., D. Rennie, et al. 2015. Users’ guides to the medical literature: Essentials of evidence-based clinical practice . 3rd ed. New York: McGraw-Hill.
Hernán, M., & Vanderweele, T. (2011). Compound treatments and transportability of causal inference. Epidemiology, 22 (3), 368–377.
Higgins, J. P., Thomas, T. J., et al. (2019). Cochrane handbook for systematic reviews of interventions . John Wiley & Sons.
Hill, A. B. (1952). The clinical trial. New England Journal of Medicine, 247 , 113–119.
Hill, A. B. 1955. Principles of Medical Statistics . 6th ed. New York: Oxford University Press.
Holland, P. W. (1986). Statistics and causal inference. Journal of the American Statistical Association, 81 (396), 945–960.
Howick, J. 2011. The philosophy of evidence-based medicine . Oxford: BMJ Books.
Howick, J., Glaziou, P., et al. (2013). Problems with using mechanisms to solve the problem of extrapolation. Theoretical Medicine and Bioethics, 34 (4), 275–291.
Howson, C., and P. Urbach. 2006. Scientific reasoning, a Bayesian approach . 3rd ed. Chicago: Open Court.
Jaynes, E. T. 2003. Probability theory: the Logic of science . Cambridge University Press.
Kabisch, M., Ruckes, C., et al. (2011). Randomized controlled trials: Part 17 of a series on evaluation of scientific publications. Deutsches Ärzteblatt International, 108 (39), 663–668.
Google Scholar
Kish, L. 1987. Statistical design for research . Wiley.
Koepke, D., and R. Flay. 1989. “Levels of analysis.” In New directions for program evaluation: evaluating health promotion programs , ed. M. T. Braverman. San Francisco: Jossey-Bass.
Landis, S. C., Amara, S. G., et al. (2012). A call for transparent reporting to optimize the predictive value of preclinical research. Nature, 480 , 187–191.
Lavori, P. W., T. A. Louis, et al. 1986. “Designs for experiments: Parallel comparisons of treatment.” In Medical uses of statistics , ed. C. Bailar and F. Mosteller, 61–82. Waltham, MA: New England Journal of Medicine.
Leighton, J. P. 2010. “Internal validity.” In Encyclopedia of research design , ed. N. J. Salkind. Thousand Oaks, CA: SAGE.
Lindley, D. V. (1982). The role of randomization in inference. Philosophy of Science Association, 2 , 431–446.
Mant, M. (1999). Can randomized trials inform clinical decisions about individual patients? Lancet, 353 , 743–746.
Miettinen, O. (1974). Confounding and effect-modification. American Journal of Epidemiology, 100 (5), 350–353.
Musters, A., & Tas, S. W. (2020). Room for improvement in clinical trials for rare diseases. Nature Reviews Rheumatology, 16 , 131–132.
Papineau, D. (1994). The virtues of randomization. British Journal for the Philosophy of Science, 45 , 437–450.
Pearl, J. 2000. Causality, models, reasoning, and inference . Cambridge University Press.
Pearl, J., & Bareinboim, E. (2014). External validity: From do-calculus to transportability across populations. Statistical Science, 29 , 579–595.
Rosenbaum, P. R. (1988). Sensitivity analysis for matching with multiple controls. Biometrika, 75 , 577–581.
Rosenbaum P. R. 1995. Observational studies . Springer.
Rothwell, P. M. (2005). Subgroup analysis in randomized controlled trials: Importance, indications, and interpretation. Lancet, 365 , 76–86.
Rubin, D. (1974). Estimating causal effects of treatments in randomized and nonrandomized studies. Journal of Educational Psychology, 66 (5), 688–701.
Russo, F., & Williamson, J. (2007). Interpreting causality in the health sciences. International Studies in the Philosophy of Science, 21 (2), 157–170.
Russo, F., & Williamson, J. (2011a). Epistemic causality and evidence-based medicine. History and Philosophy of the Life Sciences, 33 (4), 563–581.
Russo, F., & Williamson, J. (2011b). Generic versus single-case causality: The case of autopsy. European Journal for Philosophy of Science, 1 (1), 47–69.
Sekhon, J. S. (2008). The Neyman-Rubin model of causal inference and estimation via matching methods. In J. M. Box-Steffensmeier, H. E. Brady, & D. Collier (Eds.), The Oxford handbook of political methodology (pp. 271–299). Oxford University Press.
Sergent, C., Baillet, S., et al. (2005). Timing of the brain events underlying access to consciousness during the attentional blink. Nature Neuroscience, 8 (10), 1391–1400.
Shadish, W. R., T. D. Cook, et al. 2002. Experimental and quasi-experimental designs for generalized causal inference . Houghton Mifflin.
Sharabiani, M., Aylin, P., et al. (2012). Systematic review of comorbidity indices for administrative data. Medical Care, 50 (12), 1109–1118.
Steel, D. 2008. Across the boundaries: Extrapolation in biology and social science . Oxford University Press.
Upshur, R. E. 2002. If Not Evidence, then What? Or Does Medicine Really Need a Base? Journal of Evaluation in Clinical Practice, 8 , 113–19.
Urbach, P. (1985). Randomization and the design of experiments. Philosophy of Science, 52 , 256–273.
Urbach, P. (1993). The value of randomization and control in clinical trials. Statistics in Medicine, 12 , 1421–1431.
Winch, R. F., & Campbell, D. T. (1969). Proof? No. Evidence? Yes. The significance of tests of significance. The American Sociologist, 4 (2), 140–143.
Woodward, J. 2003. Making things happen: A theory of causal explanation . Oxford: Oxford University Press.
Worrall, J. (2007a). Evidence in medicine and evidence-based medicine. Philosophy Compass, 2 (6), 981–1022.
Worrall, J. (2007b). Why there’s no cause to randomize. British Journal for the Philosophy of Science, 58 , 451–488.
Download references
Author information
Authors and affiliations.
Département de Philosophie Et Des Arts, Université du Québec à Trois-Rivières, 3351, boul. des Forges, Trois-Rivières (Québec), G8Z 4M3, Canada
Tudor M. Baetu
You can also search for this author in PubMed Google Scholar
Corresponding author
Correspondence to Tudor M. Baetu .
Additional information
Publisher's note.
Springer Nature remains neutral with regard to jurisdictional claims in published maps and institutional affiliations.
Rights and permissions
Reprints and permissions
About this article
Baetu, T.M. The Virtues and Limitations of Randomized Experiments. Acta Anal 37 , 453–470 (2022). https://doi.org/10.1007/s12136-021-00497-7
Download citation
Received : 11 March 2021
Accepted : 04 October 2021
Published : 13 October 2021
Issue Date : December 2022
DOI : https://doi.org/10.1007/s12136-021-00497-7
Share this article
Anyone you share the following link with will be able to read this content:
Sorry, a shareable link is not currently available for this article.
Provided by the Springer Nature SharedIt content-sharing initiative
- Find a journal
- Publish with us
- Track your research
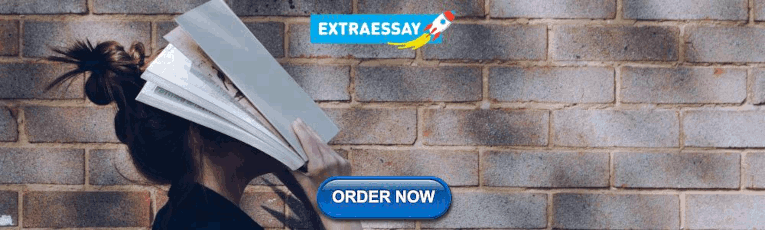
IMAGES
VIDEO
COMMENTS
The classical experiment with random assignment of participants controls for measurement bias. False. An inference is a conclusion that can be logically drawn in light of our research design and other findings. True.
True. When selecting a comparison group in a quasi-experimental design, one should: select a group as similar as possible to the experimental group. The classical experiment with random assignment of participants does NOT necessarily control for: Measurement bias. (T/F) The effectiveness of randomization in experimentation is affected by the ...
The classic experiment with random assignment of participants controls for: Choose matching definition All of these- of a relationship between variables, that one variable precedes the other in time, that a third variable did not cause the changes observed in the first two variables.
See Answer. Question: The "Classic" experimental experiment with random assignment of participants (as shown below) controls for: R O1 X O2 R O1 O2 a. Maturation b. Measurement bias c. History d. a and c only e. All of the above. The "Classic" experimental experiment with random assignment of participants (as shown below) controls for ...
Random selection (also called probability sampling or random sampling) is a way of randomly selecting members of a population to be included in your study. On the other hand, random assignment is a way of sorting the sample participants into control and treatment groups. Random selection ensures that everyone in the population has an equal ...
If random assignment of participants to control and treatment groups is impossible, unethical, or highly difficult, consider an observational study instead. This minimizes several types of research bias, particularly sampling bias, survivorship bias, and attrition bias as time passes.
For trials under 200 participants, additional conditions on the randomization procedure may ensure more equal assignment of participants between the two treatment groups. As discussed below, these conditions in restricted randomization also extend to better balance of prognostic variables between the two treatment groups. Random Assignment
1. Classical Experimental Design a. All designs are variations of the classical experimental design, the type of design discussed so far, which has random assignment, a pretest and a posttest, an experimental group, and a control group. 2. Pre-Experimental Designs a. Some designs lack random assignment and are compromises or shortcuts.
The best method to control for selection bias is random assignment of participants to conditions. A sample of n participants is selected from the target population . This sample is preferably a probability sample from the population (see Sect. 2.4), but is often a nonprobabilty sample (see Sect. 3.1).
Several kinds of experimental designs exist. In general, designs considered to be classic experiments contain three key features: independent and dependent variables, pretesting and posttesting, and experimental and control groups. In the classic experiment, the effect of a stimulus is tested by comparing two groups: one that is exposed to the ...
The classical randomized experiment has basic characteristics. It begins with a sample. The most important design component is the stimulus or test factor. A stimulus, or test factor, is a condition applied to participants in an experiment in a way in which the researcher can measure some sort of effect.
If we could either control bias experimentally (random assignment controls much of it by making experimental treatment groups roughly equivalent at the beginning of a study, thus controlling factors such as self-selection or regression toward the mean effects) or measure the variables we suspect cause bias and thus control them statistically ...
Random sampling (also called probability sampling or random selection) is a way of selecting members of a population to be included in your study. In contrast, random assignment is a way of sorting the sample participants into control and experimental groups. While random sampling is used in many types of studies, random assignment is only used ...
Attrition effects are automatically controlled when participants are assigned randomly to experimental and control groups. F. The classical experiment with random assignment of participants controls for measurement bias. F. Random assignment to experimental and control groups controls for research reactivity. F. About us. About Quizlet;
With random assignment, all participants have an equal chance of being assigned to either group. There is statistical software that will randomly assign each of the algebra students in the sample to either the experimental or the control group. Random assignment is critical for sound experimental design. With sufficiently large samples, random ...
Random assignment is a crucial aspect of true experiments, ensuring that participants are assigned to groups without bias or influence. By randomly assigning individuals to different experimental groups, researchers can be more confident that any differences observed in the outcomes are due to the manipulation of the independent variable rather ...
The second principle focuses on the reduction of bias. Random assignment acts as a safeguard, ensuring that the groups being compared are alike in all essential aspects before the experiment begins. ... Random assignment refers to how participants are placed into different groups in an experiment, aiming to control for confounding variables and ...
The pretest-posttest control group design, also called the pretest-posttest randomized experimental design, is a type of experiment where participants get randomly assigned to either receive an intervention (the treatment group) or not (the control group).The outcome of interest is measured 2 times, once before the treatment group gets the intervention — the pretest — and once after it ...
Posttest-only control group design- a type of experimental design that uses random assignment, and an experimental and control group, but does not use a pretest; Pretest- a measurement taken prior to the intervention; Random assignment-using a random process to assign people into experimental and control groups
Random assignment is a method for assigning participants in a sample to the different conditions, and it is an important element of all experimental research in psychology and other fields too. In its strictest sense, random assignment should meet two criteria. One is that each participant has an equal chance of being assigned to each condition ...
The main feature differentiating RCTs from other types of controlled experiments is the random allocation of individuals to the test and control arms of the experiment, which is thought to facilitate causal inference by eliminating allocation bias, equating groups on the expected value of all variables at pretest, and allowing researchers to ...
The classical experiment with random assignment of participants controls for measurement bias. True. False. 6 of 10. Term. An inference is a conclusion that can be logically drawn in light of our research design and other findings. True. False. 7 of 10.
Study with Quizlet and memorize flashcards containing terms like T/F Case-control designs have a high degree of internal validity because they eliminate the problem of recall bias. , T/F Cross-sectional designs have a high degree of internal validity because they show how causal processes occur over time. , T/F Time-series designs with many measurement points control for maturation. and more.