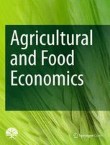
- Search by keyword
- Search by citation
Page 1 of 7
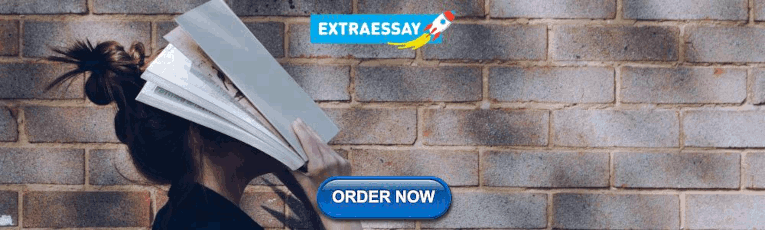
Correction: Has transformation of food systems reached an impasse? Considerations on the role of agri-food research
The original article was published in Agricultural and Food Economics 2024 12 :26
- View Full Text
Structural dynamics and sustainability in the agricultural sector: the case of the European Union
This paper seeks to draw a research agenda for unveiling the underlying bottlenecks and possibilities for improving the agricultural total factor productivity in the European Union (EU). To this aim, the paper...
Nutritional implications of substituting plant-based proteins for meat: evidence from home scan data
There is growing concern among policymakers and researchers about the negative health and climate impacts of meat consumption. Consumers are encouraged to re-evaluate their dietary choices to preserve our ecos...
Safety assessment of agricultural products and the pesticide regulation trend in China
The sustainable development of Chinese agriculture needs to coordinate the quality and quantity of agricultural products. To verify whether government regulation can promote agricultural development by control...

Food security and large-scale land acquisitions by sovereign wealth funds: a systematic review of the literature from 2012 to 2023
Many countries that have large surplus financial resources but contend with limited land and water resources use large-scale land acquisitions (LSLAs) in foreign countries to ensure national food security and ear...
The effect of farm size and farmland use on agricultural diversification: a spatial analysis of Brazilian municipalities
Brazilian agriculture is characterized by the prevalence of small farms and regions with a high degree of rurality and dominance of the agricultural sector in the economy. These two characteristics affect the ...
Has transformation of food systems reached an impasse? Considerations on the role of agri-food research
The Correction to this article has been published in Agricultural and Food Economics 2024 12 :32
Sustainability challenges in the multi-tier crop agri-food sector: a systematic review
This systematic review identifies the potential sustainability challenges lower-tier suppliers and buying firms face in multi-tier crop agri-food supply chains. The first stage applied systematic mapping, and ...
The cereal network: a baseline approach to current configurations of trade communities
This paper attempts to provide insights into the current network configurations of the food-trade system and to study the short-term effects of one of the ongoing and lasting global crises, the Ukraine War, on...
Climate change impact on yield and income of Italian agriculture system: a scoping review
Climate change poses significant challenges to agricultural systems in the Mediterranean region, with Italy being significantly affected. This literature scoping review aims to examine existing research on the...

Theorizing the socio-cultural dynamics of consumer decision-making for participation in community-supported agriculture
In the context of the urgent need to establish sustainable food systems, community-supported agriculture (CSA), in which consumers share risks with producers, has gained increasing attention. Understanding the...
Are university students really hungry for sustainability? A choice experiment on new food products from circular economy
Consumers are demonstrating increasing awareness towards initiatives promoting sustainable practices in the food sector. This is particularly pronounced among university students, a segment known for its recep...
Quantifying sustainability in the agri-food system: a comprehensive methodological framework and expert consensus approach
Assessing the economic, social, and environmental sustainability of food production is imperative for aligning with the Sustainable Development Goals (SDGs) of the 2030 Agenda and adhering to evolving regulato...
Farm animal welfare regulatory preferences and food choice: survey evidence from the US
Public support is critical for the incorporation of farm animal welfare (FAW) standards into national food policies. Multiple pathways, e.g., market-based policies, political mandates, and donations to animal ...
A literature review on the drivers and barriers of organic food consumption in China
Research interest in organic food consumption has increased significantly worldwide, but a systematic analysis of this phenomenon in China is still lacking. The existing studies in this area are wide-ranging a...
Social farming and educational needs: how kindergarten farms could fill a gap
Kindergarten farms are educational initiatives aiming to reinforce or even create relationships between nature and future generations involving them in agricultural settings. They take the form of Outdoor Educ...
The socio-economic issues of agroecology: a scoping review
In recent years, agroecology has gained prominence as one of the innovative approaches to agriculture that could positively contribute to achieving sustainable food systems. As a transdisciplinary science, agr...
Mandatory nutrition attributes labeling and consumer demand: a structural approach analysis of the US soft drink market
While soft drinks stand out as a major cause of obesity and overweight worldwide, the USA is the country most concerned with this problem. To reverse the situation, experts have agreed that labels and taxes re...
Consumers preferences and social sustainability: a discrete choice experiment on ‘Quality Agricultural Work’ ethical label in the Italian fruit sector
The Italian legislator has adopted several instruments to discourage undeclared work and exploitative labour in agriculture, mostly of a penal-repressive nature. Among the direct and indirect policy measures, ...

Pathways towards food sector sustainability: the case of vending
This paper reviews the literature on sustainability in the food vending industry to understand whether: (1) the topic of sustainable development is addressed at the academic level when it comes to the sector; ...
Heterogeneity in farmers’ stage of behavioural change in intercropping adoption: an application of the Transtheoretical Model
Despite its potential economic and environmental benefits, intercropping adoption remains limited in Europe. Drawing upon the Transtheoretical Model, this paper views adoption decision as stages of behavioural...
Sustainability of European agri-food supply chain using MRP-PCI multicriteria analysis method
The need for a more sustainable agri-food system is a topic that has attracted growing interest in recent years. Several international and European policies such as Agenda 2030 and the European Green Deal have...
Sustainability reporting in focus: analysing Spanish transposition of the Non-Financial Reporting European Directive in the agri-food sector
In recent decades, the business world has undergone a paradigm shift, prioritizing social and environmental considerations over the exclusive pursuit of economic profits. Corporate social responsibility has be...
Differing impacts of the COVID-19 pandemic on farmers and intermediaries: insights into the Ecuadorian cocoa value chain
The COVID-19 pandemic generated diverse impacts and responses in agricultural value chains worldwide. Cocoa is a key crop for Ecuadorian exports, and the analysis of effects the pandemic had on value chain act...
From COVID-19 to the war in Ukraine: evidence of a Schumpeterian transformation of food logistics
This study analyzes the changes that have occurred in food logistics in the three years since the emergence of the COVID-19 pandemic and the one year since the war in Ukraine commenced. Food logistics companie...
Examining disparities in common agriculture policy direct payments among farming systems: evidence from Portugal
One of the stated goals of the common agricultural policy reforms has been to provide a fairer distribution of payments across and within member states, but little progress has been accomplished, with about 20...
Exploring the role of blockchain technology in modern high-value food supply chains: global trends and future research directions
Trust, safety, and quality are among the most important factors in the agri-food supply chains. Traceability is a powerful tool to ensure them, but implementing a transparent and effective system is a complex ...
Predicting the differences in food security with and without the Russia–Ukraine conflict scenarios over different regions of the world
The Russia–Ukraine conflict has caused a global food security crisis, impacting sustainable development goals. Predicting the crisis’s impact on food security is crucial for global stability by 2030. From a ma...
Potential unexpected effects of meat reduction in diet: Could educational attainment influence meat substitution strategies?
Regulation of meat consumption appears to be a relevant public policy to limit diet-related greenhouse gas emissions. However, the real impact of such a policy (e.g., tax) on human health and nutrition remains...
Food miles on the shelves: the share of local food products in the Hungarian retail sector
Share of national food products in retail is a frequent subject of policy debates. Local food is often associated with national security, sustainability, and support of local economy, contributing to value-add...
The future of organic certification: potential impacts of the inclusion of Participatory Guarantee Systems in the European organic regulation
Third-party certification (TPC) is the standard approach to quality assurance for organic production, but its administrative burden and cost make it difficult for smallholders to access it. Internal Control Sy...
The implications of governance factors for economic and social upgrading in Ghana’s cocoa value chain
This paper identifies several governance factors that enable economic upgrading and the link between economic and social upgrading /downgrading of smallholders and Abusa sharecroppers in Ghana's cocoa value ch...
The coexistence of psychological drivers and deterrents of consumers’ willingness to try cultured meat hamburger patties: evidence from South Africa
The widespread consumption of alternative meat products, such as plant-based alternatives in the hamburger patty market, has provided insights into the potential of commercially produced cultured meat. Evidenc...
Conventional versus organic olive farming: which has a better economic performance?
The European Green Deal sets a target of at least 25% of the total EU agricultural land under organic farming by 2030. In the case of the Spanish olive sector, organic olive farming accounts for barely 10% of ...
The distortion in the EU feed market due to import constraints on genetically modified soy
Feed importers in some EU member states face constraints on imports of genetically modified (GM) soy, a practice that may compromise the interests of EU livestock farmers. Using the cases of Sweden and Austria...
Challenges of digitization in the social economy in times of pandemic: the evolution of online presence and e-commerce in agri-food cooperatives
Online presence is essential for companies operating in the social economy. The health crisis resulting from Covid-19 has reinforced the need to access the Internet and develop online commerce. This paper anal...
Cultured meat acceptance for global food security: a systematic literature review and future research directions
Cultured meat is a novel technology-based meat alternative with the potential to complement protein supply for a growing world population. An increasing body of consumer research has investigated personal fact...
The role of objective and subjective knowledge on the attitude and intention of Italian consumers to purchase farmed and wild fish
In general, consumers have very little knowledge about production methods employed in the fish sector, and this lack of information contributes to skepticism and confusion when they choose and purchase fish. I...
Customer preferences heterogeneity toward avocado: a latent class approach based on the best–worst scaling choice modeling
Consumption of the avocado fruit and its availability in the retail market has increased in recent decades and with it the desire to learn more about the market and consumer choices. This research aims to expl...
Is it possible to quantify the current resilience level of an agri-food system? A review of the literature
Nowadays being resilient is a requirement of all companies and more in general supply chains, as a consequence of the frequent disruptions which repeatedly affect systems and challenge markets from different s...
The impact of COVID-19 government policy on the international wine trade
To control the health impact of the COVID-19 pandemic, governments implemented various restrictive policies, such as stay-at-home orders and restrictions on internal movement, which had adverse effects on cons...
Unraveling the effects of import bans on domestic poultry production: a case study of Senegal
Senegal banned all imports of uncooked poultry meat in 2006 in response to Avian Influenza outbreaks in many exporting countries. This paper investigates the effects of the import ban on domestic chicken meat ...
Prioritization of e-traceability drivers in the agri-food supply chains
Electronic traceability (e-traceability) is a growing trend in the agri-food industry, offering improved transparency, accountability, and reduced risk of foodborne illnesses through the use of electronic syst...
Traffic light nutrition labeling preferences among children
This study evaluates the effects of traffic light (TL) nutritional label attributes on children’s food choices. Data were collected from a survey of 1179 Ecuadorian students attending public middle and high sc...
Understanding the phenomenon of food waste valorisation from the perspective of supply chain actors engaged in it
The repurposing of surplus food and food processing by-products is a key aspect of the shift towards a circular bioeconomy. In the Netherlands, food supply chain actors are already working towards making bette...
Supporting agriculture in developing countries: new insights on the impact of official development assistance using a climate perspective
Agriculture is a major source of food and income for poor and rural households living in developing countries; yet, agricultural systems are increasingly threatened by changing climate conditions that compromi...
Adaption of the meat attachment scale (MEAS) to Germany: interplay with food neophobia, preference for organic foods, social trust and trust in food technology innovations
Meat-based diets are still the norm, and vegans and vegetarians represent only a small minority of the population. A transition, respectively, behavioural change towards a diet with less meat can only occur by...
Vegetable and fruit consumption during the COVID-19 lockdown: eating habits in Ecuador
This study identifies changes in fruit and vegetable (FV) consumption habits during the COVID-19 pandemic in Quito-Ecuador and observe relationships regarding household income and the relevance of FV nutrition...
Economic effects of food supply chain re-localization on the Croatian economy
Securing the availability of healthy food at affordable prices is of fundamental public interest. The formerly prevailing paradigm of the absolute superiority of free trade in the global food market is changin...
Food, biofuels or cosmetics? Land-use, deforestation and CO 2 emissions embodied in the palm oil consumption of four European countries: a biophysical accounting approach
Around 75% of tropical deforestation in the XXI century has been driven by the expansion of agriculture and forest plantations. Since 1990s, palm oil has been standing for a critical global traded product in t...
- Editorial Board
- Sign up for article alerts and news from this journal
- Follow us on Facebook
- ISSN: 2193-7532 (electronic)
Climate Change and Agriculture
- First Online: 28 August 2024
Cite this chapter
- Latief Ahmad 4 ,
- Gazi Mohammad Shoaib Shah 5 &
- Asim Biswas 6
28 Accesses
Climate change has become one of the major concerns of man in recent decades all over the world. The floods, drought, and desertification seriously threaten the livelihood of farmers and our food supply as well. As the climate continues to change, farm communities around the world will be increasingly challenged. Halfway through the chapter we get to know about the impacts of climate change like greenhouse effect, global warming, nonavailability of water, ozone depletion, etc. Toward the end, the chapter provides apt ways to mitigate climate change and how one can canvass for it, ultimately reversing it.
This is a preview of subscription content, log in via an institution to check access.
Access this chapter
Subscribe and save.
- Get 10 units per month
- Download Article/Chapter or eBook
- 1 Unit = 1 Article or 1 Chapter
- Cancel anytime
- Available as PDF
- Read on any device
- Instant download
- Own it forever
- Available as EPUB and PDF
- Durable hardcover edition
- Dispatched in 3 to 5 business days
- Free shipping worldwide - see info
Tax calculation will be finalised at checkout
Purchases are for personal use only
Institutional subscriptions
5th Assesment Report (AR5) – Intergovernmental Panel on Climate Change (IPCC) (2014) Food security and food production systems 95, 756, 810.
Google Scholar
Chandrasekaran, B., Annadurai, K., & Somasundaram, E. (2018). A textbook of agronomy. 233, 234, 235, 236 - New Age International Private Limited
FAO. (2020). The state of food and agriculture- overcoming water challenges in agriculture, 94.
Mavi, H. S., & Tupper, G. J. (2004). Agrometeorology – Principles and applications of climate studies in agriculture (p. 264).
Book Google Scholar
Mostafazadeh, R. (2018). Re: Dufference between climate change and climate variation? Retrieved from: https://www.researchgate.net/post/Dufference-between-climate-change-and-climate-variation/5a68a70bb0366d23404fff43/citation/download
National Renewable Energy Laboratory. (2012). Renewable electricit futures study. https://www.nrel.gov/docs/fy12osti/52409-1.pdf
USDA – United States Department of Agriculture. (2018) Climate change, global food security, and the U.S. food system. 20.
Download references
Author information
Authors and affiliations.
Division of Agrometeorology, Faculty of Horticulture, Sher-e-Kashmir University of Agricultural Sciences and Technology of Kashmir, Srinagar, Jammu and Kashmir, India
Latief Ahmad
Faculty of Horticulture, Sher-e-Kashmir University of Agricultural Sciences and Technology of Kashmir, Srinagar, Jammu and Kashmir, India
Gazi Mohammad Shoaib Shah
School of Environmental Sciences, University of Guelph, Guelph, ON, Canada
Asim Biswas
You can also search for this author in PubMed Google Scholar
Climate change refers to:
Year to year fluctuations in the weather
Long-term changes in temperature and weather patterns
Short-term changes in temperature and weather patterns
None of the above
Climate change is also called:
Both 1 and 2
Who developed the “greenhouse effects” theory?
J. B. Fourler
Claude Pouillet
The average global temperature of Earth has reportedly risen by roughly
Which of the following is more destructive to ozone?
Nitrous oxide
Global warming leads to:
Habitat destruction
Spread of diseases
All of the above
The ozone layer restricts
Infrared rays
Ultraviolet rays
Visible light
Which of the following is not a greenhouse gas?
Water vapor
Carbon dioxide
Which of the following causes is responsible for climate change?
Change in solar output put
Greenhouse gases
Changes in the earth’s orbit and rotation
The use of fossil fuels is responsible for the increase in the amount of which of the following gases?
Rights and permissions
Reprints and permissions
Copyright information
© 2024 The Author(s), under exclusive license to Springer Nature Switzerland AG
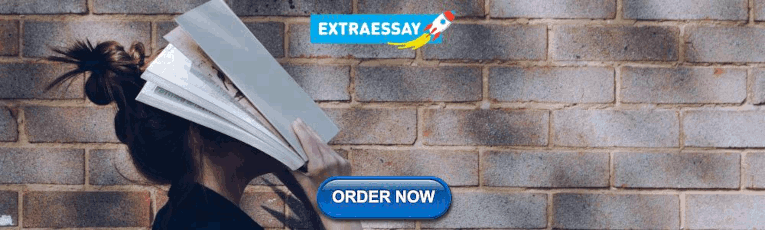
About this chapter
Ahmad, L., Shah, G.M.S., Biswas, A. (2024). Climate Change and Agriculture. In: Fundamentals and Applications of Crop and Climate Science. Springer, Cham. https://doi.org/10.1007/978-3-031-61459-0_12
Download citation
DOI : https://doi.org/10.1007/978-3-031-61459-0_12
Published : 28 August 2024
Publisher Name : Springer, Cham
Print ISBN : 978-3-031-61458-3
Online ISBN : 978-3-031-61459-0
eBook Packages : Biomedical and Life Sciences Biomedical and Life Sciences (R0)
Share this chapter
Anyone you share the following link with will be able to read this content:
Sorry, a shareable link is not currently available for this article.
Provided by the Springer Nature SharedIt content-sharing initiative
- Publish with us
Policies and ethics
- Find a journal
- Track your research
Click through the PLOS taxonomy to find articles in your field.
For more information about PLOS Subject Areas, click here .
Loading metrics
Open Access
Peer-reviewed
Research Article
Climate smart agriculture and global food-crop production
Roles Conceptualization, Data curation, Formal analysis, Investigation, Methodology, Supervision, Writing – original draft, Writing – review & editing
* E-mail: [email protected]
Affiliation Environment and Production Technology Division, International Food Policy Research Institute, Washington, DC, United States of America

Roles Data curation, Formal analysis, Methodology, Validation, Visualization, Writing – original draft, Writing – review & editing
Roles Data curation, Formal analysis, Writing – original draft
Affiliation Energy Systems Division, Argonne National Laboratories, Lemont, IL, United States of America
Roles Data curation, Validation
Roles Data curation
- Alessandro De Pinto,
- Nicola Cenacchi,
- Ho-Young Kwon,
- Jawoo Koo,
- Shahnila Dunston
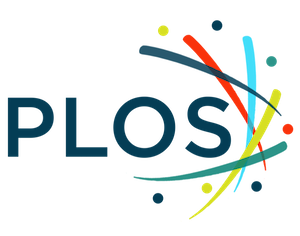
- Published: April 29, 2020
- https://doi.org/10.1371/journal.pone.0231764
- Reader Comments
Most business-as-usual scenarios for farming under changing climate regimes project that the agriculture sector will be significantly impacted from increased temperatures and shifting precipitation patterns. Perhaps ironically, agricultural production contributes substantially to the problem with yearly greenhouse gas (GHG) emissions of about 11% of total anthropogenic GHG emissions, not including land use change. It is partly because of this tension that Climate Smart Agriculture (CSA) has attracted interest given its promise to increase agricultural productivity under a changing climate while reducing emissions. Considerable resources have been mobilized to promote CSA globally even though the potential effects of its widespread adoption have not yet been studied. Here we show that a subset of agronomic practices that are often included under the rubric of CSA can contribute to increasing agricultural production under unfavorable climate regimes while contributing to the reduction of GHG. However, for CSA to make a significant impact important investments and coordination are required and its principles must be implemented widely across the entire sector.
Citation: De Pinto A, Cenacchi N, Kwon H-Y, Koo J, Dunston S (2020) Climate smart agriculture and global food-crop production. PLoS ONE 15(4): e0231764. https://doi.org/10.1371/journal.pone.0231764
Editor: Paolo Agnolucci, University College London, UNITED KINGDOM
Received: September 11, 2019; Accepted: March 31, 2020; Published: April 29, 2020
Copyright: © 2020 De Pinto et al. This is an open access article distributed under the terms of the Creative Commons Attribution License , which permits unrestricted use, distribution, and reproduction in any medium, provided the original author and source are credited.
Data Availability: The data used for this analysis are available for download at https://github.com/IFPRI/Climate-Smart-Ag-PlosOne .
Funding: The authors acknowledge the generous support of the CGIAR Research Program on Policies, Institutions, and Markets (PIM) funded by CGIAR Fund Donors and the CGIAR Research Program on Climate Change, Agriculture, and Food Security, which is carried out with support from CGIAR Fund Donors and through bilateral funding agreements.
Competing interests: The authors have declared that no competing interests exist.
1 Introduction
Uncertainty in projections makes it difficult to determine the precise impact of climate change on future agricultural productivity, but studies have consistently found that under most scenarios significant negative effects should be expected worldwide [ 1 – 6 ] and especially in economically underdeveloped regions [ 7 – 9 ]. Importantly, agricultural production is not only affected by climate change but contributes substantially to the problem with yearly greenhouse gas (GHG) emissions that range from 5.0 to 5.8 Gt CO 2 e or about 11% of total anthropogenic GHG emissions [ 10 ]. Combined with forestry and other land uses, anthropogenic land activities contribute about a quarter of annual GHG emissions, which is the equivalent of 10 to 12 Gt CO 2 e per year [ 10 ].
Recent developments in the United Nations Framework Convention on Climate Change negotiations (i.e. the Paris Agreement in 2015 and the Koronivia joint work on agriculture [ 11 , 12 ]) and the recent Intergovernmental Panel on Climate Change special report [ 13 ] have reinvigorated calls for incentives to reduce GHG emissions, including the pricing of carbon and the levy of a carbon tax. However, the latest analyses on the subject [ 14 , 15 ] indicate that a tax on GHG emissions may lead to significant tradeoffs between emissions abatement and food security.
It is in this environment that the concept of Climate Smart Agriculture (CSA) has become increasingly relevant. CSA proposes a framework that supports decision-making in the agriculture sector by considering three foundational outcomes and by fully accounting for the trade-offs and synergies among them. It is comprised of agricultural systems that contribute to sustainable and equitable increases in agricultural productivity and incomes; greater adaptation and resilience to climate change of food systems from the farm- to the national-level; and reduction, or removal, of greenhouse gas emissions, where possible [ 16 ].
Many operational aspects of CSA are still under investigation as local contexts determine the enabling environment as well as the trade-offs and synergies between productivity, adaptation, and mitigation [ 17 , 18 ]. Farmers must identify what can be considered climate-smart given their biophysical, agricultural, and socio-economic context. As a consequence, the use of the CSA approach is knowledge-intensive and can require considerable institutional support [ 19 , 20 ]. Because of these difficulties, Chandra at al [ 21 ] note that, at this time, CSA is “a popular scholarly solution” experiencing difficulties in translating into smallholder farmer and civil society actions as well as new policy directions. Taylor [ 22 ] illustrates how the failure to incorporate issues related to social justice make the acceptance and implementation of CSA difficult in many communities. The frequent result is that, even when farmers, agrarian organizations, large scale farmers, and policy maker have embraced the concept of CSA, they struggle with the implementation and tend to look for simple protocols to follow.
Despite these unsettled issues, a substantial amount of resources have been mobilized to promote and implement CSA at a scale sufficient to have a global impact [ 23 ]. Even though CSA is more than a set of agricultural practices, it does include some specific technologies and agronomic tools, and these are the focus of this study. Our goal is to provide a first set of boundaries for the global effects of CSA used in food-crop production with a particular attention to its potential to reduce GHG emissions without jeopardizing food security. For this, we focus on some aspects of food-crop production that can be modelled globally with a reasonable level of accuracy given the latest developments in modeling capabilities [ 9 , 24 – 26 ].
Our analysis looks at four major categories of agricultural practices: no-till, integrated soil fertility management, nitrogen use efficiency and alternate wetting and dry. They have been shown to have positive impacts on yields and GHG mitigation across a wide range of conditions, but they all require specific modifications and adjustments on the ground. As a consequence, the modelling work presented here is a stylized representation of a range of many technologies and practices that would be identified using the CSA approach.
Notwithstanding these limitations, the results of our analysis clearly indicate that CSA practices have the potential to increase food production under unfavorable climate regimes and to improve the food security conditions of millions of people while reducing GHG emissions. However, results also indicate that for CSA to make a sufficiently large impact on global GHG emissions, it must be implemented widely across the entire sector and requires a significant amount of support and coordination. Our findings are also suggestive of broader benefits related to the resilience of the production system, to a reduced pressure for expanding cropland area and to reduced soil fertility depletion. While encouraging, these results are the product of the method and of the modeling assumptions used in the analysis and they must be evaluated and refined by additional global and regional analyses.
We performed an ex-ante analysis of the long-term effects on global food security and GHG emissions of adopting of a set of CSA technologies and practices to grow three widely grown crops: maize ( Zea mays ), wheat ( Triticum aestivum ), and rice ( Oryza sativa ). These three crops represent about 41% of the global harvested area and approximately 64% of the estimated 2–3 Gt CO 2 e per year emitted by crop production globally [ 27 ]. The effects of CSA practices on production, food security, and GHG emissions were assessed via comparison with the outcomes of a business-as-usual (BAU) scenario in which farmers retain the current practices during the period 2010–2050.
All scenarios, BAU and alternatives, were created using the IMPACT system of models [ 26 , 28 ] which links crop and climate models to the core economic model in which agricultural production is represented across 320 sub-national regions called “food production units” (FPUs, see Supplementary Discussion S1 in S1 File ). Because of the linkages among these models, IMPACT’s outputs reflect the interactions between biophysical, economic and population trends, the combination of which represents the functioning of the global food market. A stylized representation of the modeling steps and of the information flow is provided in Fig 1 . The process represented in the figure is used to generate each one of the scenarios described in the sections below.
- PPT PowerPoint slide
- PNG larger image
- TIFF original image
Source: Authors.
https://doi.org/10.1371/journal.pone.0231764.g001
2.1 Simulation of production, prices and food security—the core of the modeling system
To simulate the effects of CSA adoption on yields, production, harvested areas, world commodity prices and indicators of food security, we linked the spatially-disaggregated data of three models: the Spatial Production Allocation Model (SPAM) [ 29 ], the Decision Support System for Agrotechnology Transfer (DSSAT) [ 30 ], and the International Model for Policy Analysis of Agricultural Commodities and Trade (IMPACT v3.3) [ 28 ] (Supplementary Discussion S1 and Supplementary Fig 1 in S1 File ).
The SPAM model was used to identify the location of crop production on a global grid in which each grid-cell is of size 30 arc minutes (a square of approximately 56 km by 56 km at the equator). For each grid-cell, SPAM provides a database cataloging the dominant crops, representative cultivars, management practices, and the inputs used. Data about climate, irrigation type, and soil properties were geo-linked to each SPAM grid-cell and were essential to run crop model simulations at the grid-cell level. The DSSAT crop model was used to simulate crop yields, with current and or with CSA practices, as a function of the interaction between biophysical elements of the crop systems (e.g. soil, weather, and crop) and management practices (e.g., tillage, nutrient application, and water availability). After the completion of a calibration step (Supplementary Discussion S2 with Supplementary Table 2 and Supplementary Figs 2 and 3 in S1 File ), the yield responses were aggregated to evaluate agriculture production across IMPACT’s FPUs. IMPACT utilizes the yield responses to agricultural practices as shifters for the crop-specific supply curves and for the yield’s growth rates [ 1 , 9 , 28 , 31 ]. Starting from these changes in growth rates, the systems of equations at the core of the model endogenously determine crop areas, agricultural production, commodity prices and food availability. In turn, taking into consideration population and income growth, changes in food availability translate into changes in the regional availability of kilocalories [ 28 ]. In IMPACT, the share of people at risk of hunger (i.e. suffering from undernourishment) is calculated based on an empirical correlation between the share of undernourished people within the population and the relative availability of food. The calculation is adapted from Fischer et al. [ 28 , 32 ]. The share of undernourished children under the age of five is based on the calculation of the average calorie availability per capita per day, women’s access to secondary education, the ratio of female to male life expectancy at birth, and health and sanitation conditions [ 28 , 33 ]. It is an estimate of undernourishment in terms of weight for age.
2.2 Implementation of CSA technologies in DSSAT
The CSA scenarios simulate the use of four practices that are recurrently identified in the literature for their potential to deliver across the objectives of CSA and to be adopted widely [ 18 , 34 ]. There are several other practices and technologies that are proposed for CSA, the ones selected are among those that can be modeled with higher accuracy. The technologies considered for maize and wheat are no-till and integrated soil fertility management, while those for rice are alternate wetting and drying and nitrogen use efficiency. These practices are already utilized and widely tested due to their promising positive effects on yields, their higher resource use efficiency (e.g., water and nutrients) and their overall effects on GHG emissions ( Table 1 and Supplementary Discussion S3 for a description of the technologies; Supplementary Discussion S4 and Supplementary Table S3 for modeling details and an assessment of our simulation results in S1 File ).
https://doi.org/10.1371/journal.pone.0231764.t001
In order to simulate no-till, the default setting for conventional tillage in DSSAT was removed and a seed planting stick and a deep fertilizer injection were used as planting and fertilizer application methods to minimize soil disturbance (more details in Supplementary Discussion 3 in S1 File ). Six countries (Argentina, Australia, Brazil, New Zealand, Paraguay, and Uruguay) where no-till has already been widely adopted were excluded from the simulations [ 51 , 52 ] because we assume that the potential for expansion in these six countries is low. In other places, especially North America where no till is already present but not widely adopted, we assume that it is not utilized and therefore our analysis may overestimate the impacts of using this practice.
Integrated soil fertility management was implemented by simulating the application of organic amendment, in addition to the inorganic fertilizer applications already defined in the BAU scenario. The site-specific organic manure application quantity was based on Potter et al. (2010; http://www.earthstat.org ) [ 53 ] and was used monthly during the fallow period (after harvesting–before planting). The rate of inorganic fertilizer application is the same as in the BAU scenario; however, the application scheduling is optimized based on the growth stage of each crop to minimize nitrogen stress during flowering and grain filling.
For rice production, enhanced nitrogen use efficiency and alternate wetting and drying (AWD) were simulated. Nitrogen use efficiency was simulated by focusing on enhanced plant uptake of nitrogen fertilizer under both rainfed and irrigated conditions. This was done by turning on in DSSAT the option for nitrogen fertilizer application based on deep placement of urea supergranules at a depth of 10 cm beneath the surface soil. Simulation of AWD was only applied to irrigated rice and was based on a recent study by the International Fund for Agricultural Development-IFPRI Partnership Program that identified alternative agricultural mitigation options for rice production using the DeNitrification-DeComposition model [DNDC; 54 ]. We employed the same modeling approach of DNDC, which assumes that: i) rice paddy is initially flooded to 10 cm, ii) water level is reduced at rate of -0.5 cm/day to -5cm, and iii) then re-flooded at rate of 0.5 cm/day till to 10 cm.
2.3 Calculation of GHG emissions
DSSAT was used to calculate per-hectare GHG emissions which were then multiplied by the harvested areas projected by the IMPACT model to compute total GHG emissions. Specifically, temporal changes in soil carbon stock were simulated in the CENTURY soil organic matter (SOM) module embedded into DSSAT [ 55 ]. Direct N 2 O emissions were simulated by modifying DSSAT’s source codes to model denitrification processes. The modifications ensure that our estimates of direct N 2 O emissions are comparable to those calculated using the 2006 Intergovernmental Panel on Climate Change (IPCC) emission factors [ 56 ], where 1% of N additions from mineral fertilizers, from organic amendments and from crop residues, 1% of N mineralized from soil organic matter, and ~0.7% of N from residue inputs are converted into N 2 O emissions.
For flooded rice soils, we used the IPCC default emission factor of 0.3% of applied N. Methane (CH 4 ) emissions were calculated by combining DSSAT-simulated rice biomass with IPCC Tier 1 method’s emission coefficients proposed by Yan et al. 2009 [ 57 ]. Parameters of the Tier 1 method include baseline emission factor (1.3), scaling factors for continuous flooding (1) and multiple drainage (0.52), simulating effect of rice straw (0.59), and conversion factor of farmyard manure (0.14). These were combined with the simulated outputs of rice yields and straws, days in growing season, soil organic carbon content, and the input data of manure application rate. Finally, all GHG emissions were converted into tons of CO 2 e using global warming potential for 100-yr time horizon of each GHG (Supplementary Table S4 in S1 File ).
2.4 Scenarios
2.4.1 business as usual scenario..
BAU scenario reflects the use of current practices and technologies throughout the 2010–2050 period and assumes that agriculture is developing under climate change conditions. BAU and all the alternative scenarios use the population and income growth assumptions that underly the middle of the road Shared Socioeconomic Pathway 2 (SSP2; [ 37 , 38 ]) from the IPCC Assessment Report 5 (AR5; [ 15 , 24 , 58 ]). Under the SSP2 narrative, global population will reach over 9 billion people by 2050 at an average annual growth rate of 0.6% and per capita GDP grows at just below 2% per year. The climate models used in both DSSAT and IMPACT under a Representative Concentration Pathway 8.5 (RCP 8.5; [ 59 ]) represent two possible future states of the climate. One, the GFDL-ESM2M (GFDL; [ 60 ]), is drier and cooler than the other, the HadGEM2-ES (HadGEM; [ 61 ]) (Supplementary Figs 4 and 5 and Supplementary Table S5 in S1 File ). Given the high uncertainty around the overall effects of carbon fertilization on crop productivity [ 62 , 63 ] we cannot make conclusive statements about how increased concentrations of CO 2 in the atmosphere will impact production (see a detailed treatment of the issue in Supplementary Discussion S5 in S1 File ). Therefore, our modeling approach assumes no additional effects on yields from atmospheric carbon.
2.4.2 Climate smart agriculture scenarios.
These scenarios were constructed by assuming that farmers who are currently using a particular set of practices to grow maize, wheat, and rice are offered a portfolio of alternatives from which to choose (i.e. the four CSA practices considered). We first explored two scenarios with the objective to shed light on the largest possible effects of adopting CSA practices. The scenarios are based on two basic adoption rules implemented at the grid-cell level. The first (Rule 1) requires that CSA practices generate a yield gain compared to BAU in order to be adopted. The second (Rule 2) requires that an alternative practice generates higher yields and a decrease in emission intensity (i.e. the quantity of CO 2 e per unit of product). If none of the alternatives increases yields, it is assumed that farmers retain their current practices. Given our objective, both scenarios assume that when a CSA practice is chosen all farmers in a given area adopt it beginning from the first year (i.e. 100% of the area in which Rule 1 or Rule 2 are satisfied it is assumed to adopt the CSA practices). Admittedly, the chosen adoption rules overestimate the role that yield gains play in farmers’ decisions. We do recognize that there are other benefits that might motivate adoption. However, at this time there are no widely applied financial mechanisms that disincentivize the use of current agricultural practices or that promote CSA [ 64 ]. Furthermore, non-monetary benefits vary greatly with local conditions and with farmers’ idiosyncrasies which cannot be represented in a global modeling exercise. More importantly, it is unlikely that countries would support the wide uptake of practices that reduce yields, given the importance that increased productivity holds for food security. We therefore think that, albeit with limitations, the assumption that adoption requires a yield increase is a pragmatic way to move forward in global analysis such as the one we are undertaking.
We did however test the possible effects of production costs and other barriers to adoption through two additional scenarios. It is well known that production costs and other factors (e.g. farmers’ access to markets and to credit, the characteristics of a particular technology, the quality of extension services, attitudes towards risk and risk exposure) affect the adoption of new practices and technologies [ 65 – 68 ]. Based on these notions, a third scenario was implemented which uses adoption Rule 1 together with the adoption rates used by Rosegrant et al. [ 9 ]. These implicitly include multiple costs related to adoption and were obtained through surveys and interviews with experts. These rates are more realistic than the 100% adoption assumed in the previous scenarios (Supplementary Discussion S6 with Supplementary Table S6 in S1 File ).
The issue of costs of production is particularly important for AWD, a practice that could significantly reduce the use of water without reducing rice yields (see Table 1 ). This means that AWD could be adopted even though there is a reduction in yields. Therefore, the fourth and last simulated scenario uses Rule 2 but expands adoption by including the potential reduction of production costs associated with implementing AWD. A review of the literature reveals that irrigation costs represent from 3 to 36% of production costs [ 56 , 57 , 69 ] and AWD is reported to reduce irrigation cost up to 30% [ 70 , 58 ]. Based on these estimates we calculate that as long as yields decrease less than 9%, AWD is still be more profitable, and therefore preferable, to the current practices (Supplementary Discussion S7 in S1 File ).
The performance of CSA practices depends on tailoring their implementation to the specific local conditions, but the capacity and knowledge to do so varies greatly from farmer to farmer. Therefore, for all scenarios we simulated instances in which farmers perform a poor, average, and optimal tailoring of the technology to their local biophysical circumstances (represented in the model by weather and soil characteristics). To do this, we exploited the fact that each IMPACT FPU contains multiple 30 arc minutes grid-cells. We considered the yield gain distribution in each FPU and used the average of the lower quartile, the average of the distribution, and the average of the upper quartile to represent a poor, average, and optimal tailoring of CSA.
Thus, our evaluation of the effects of widespread adoption of CSA practices is based on a total of 26 simulations ( Table 2 ). Given the amount of output generated, for each scenario we report a single value which is the average of the GFDL and HadGEM results followed in parenthesis by the range of the results (lowest–highest value) obtained using the different climate models and levels of tailoring. The same information is indicated in the whisker bar in the figures.
https://doi.org/10.1371/journal.pone.0231764.t002
3.1 Prices and production
Projections for the BAU scenario indicate that global production of maize, wheat, and rice in 2050 will increase by 47% (36–58%), 42% (40–44%), and 19% (18–20%) respectively, compared to 2010. Prices are projected to increase by 80% (56–103%), 35% (24–46%), and 52% (44–60%) respectively. Therefore, despite the impact of climate change, production of these three main cereals is projected to increase. After economic growth and changing incomes and diets are considered, by 2050 according to BAU projections there will be 47 million (45–48) fewer undernourished children and 385 million (361–410) fewer people at risk of hunger.
We first report in detail the results for the CSA adoption scenarios based on Rules 1 and 2. The sensitivity of these scenarios to barriers to adoption and to costs of production is reported later in the paper. The results show that CSA practices are adopted on a total of approximately 372 million hectares when adoption is based exclusively on yield increase (Rule 1) and on 241 million hectares when adoption is dependent on reduction in emission intensity and increase in yields (Rule 2) (Supplementary Discussion S8 with Supplementary Table S7 in S1 File ). Compared to BAU, by 2050 CSA practices are estimated to increase global production of maize by an additional 4% (1–9%) with Rule 1 and 3% (1–5%) with Rule 2; wheat production is also estimated to increase by about 4% (1–9%) with Rule 1, and 3% (0.4–8%) with Rule 2. CSA practices appear to have the largest effect on rice, for which production is projected to increase by about 9% with both rules (4–16% with Rule 1 and 4–15% with Rule 2) ( Fig 2 ).
Columns indicate the average tailoring of CSA practices; whisker bars identify results for the poor and optimal tailoring. Source: Authors. BAU = business as usual scenario.
https://doi.org/10.1371/journal.pone.0231764.g002
We should note that not all countries experience an increase in production. The wide-scale adoption of CSA practices induces a reorganization of global production because of differences in land suitability. As a result, in some countries the reduction in crop harvested area offsets the gains in yields (Supplementary Table S8 in S1 File ). Nevertheless, overall the changes in production are sufficient to have a sizable impact on world prices (see description of endogenous effects in Supplementary Discussion S9 in S1 File ). Prices are still projected to increase but compared to BAU their growth is reduced by 8% (3–17%) for maize, by 11% (3–25%) for wheat, and by 27% (13–42%) for rice with Rule 1, and by 6% (2–11%) for maize, 8% (2–20%) for wheat and 26% (14–40%) for rice with Rule 2 (Supplementary Table S9 in S1 File ). As a result, the population at risk of hunger decreases more than what is projected by the BAU scenario. The number of people at risk of hunger is reduced by an additional 34 million (10–69 million) by 2050 under Rule 1 and by 29 million (10–59 million) under Rule 2, with the largest improvements in Sub-Saharan Africa, East Asia and Pacific, and South Asia. Similarly, the number of undernourished children decreases by an additional 2 million under both Rules 1 and 2 (a range of 1–5 million with Rule 1 and 1–4 million with Rule 2) with most of the improvements in Sub-Saharan Africa and South Asia (Supplementary Table S10 with Supplementary Figs 6 and 7 in S1 File ).
3.2 GHG emissions
Global GHG emissions decrease under both adoption scenarios, but there are important distinctions between the two. When farmers’ adoption choices are based only on yield increases (Rule 1), the reduction in GHG emissions is estimated to be equivalent to 44 Mt CO 2 e yr -1 (9–77 Mt CO 2 e yr -1 ). The reduction of emissions is significantly higher with Rule 2, 101 Mt CO 2 e yr -1 (84–124 Mt CO 2 e yr -1 ). This shows that there is a substantial amount of area in which CSA practices can increase yields but do not reduce GHG emissions. The higher levels of emissions abatement come at the cost of production. On average across climate scenarios, under Rule 2 total cumulative production for the three crops is reduced by 21 Mt yr -1 (1 and 53) of fresh matter harvest compared to Rule 1. This is equivalent to 1% (0.1 and 2%) of total yearly global production of maize, wheat and rice.
It is important to note that the effects on emissions vary from country to country and that we find instances in which an increase in emissions occurs in some countries ( Fig 3 and Supplementary Table S11 in S1 File ).
Negative values indicate an abatement compared to BAU and positive values an increase. Source: Authors.
https://doi.org/10.1371/journal.pone.0231764.g003
Emissions can increase because of the potentially large changes in countries’ crop harvested areas and yields. Total emissions can increase even when a reduction of emission intensity is achieved if the reduction in emissions per unit of output is offset by increases in yields or in areas (Supplementary Discussion S10 with Supplementary Table S11 in S1 File ).
3.3 Other effects related to emissions
Interestingly, part of the reduction in emissions is due to the effects on soil organic carbon concentration, which is estimated to grow compared to BAU by 0.11 t ha -1 yr -1 (0–0.49 t ha -1 yr -1 ) over the area that adopts the alternative practices based on Rule 1, and by 0.14 t ha -1 yr -1 (0.01–0.53 t ha -1 yr -1 ) based on Rule 2. These changes are beneficial for sustainable production and resilience since higher soil organic carbon concentrations increase soils nutrient availability and soils water retention.
Some potentially important effects on land use must be noted. The combination of higher yields and lower prices reduces producers’ incentives to expand production onto additional land as the demand for wheat and rice can be satisfied with less harvested area (Supplementary Table S12 in S1 File ). Even though global harvested area for maize is projected to expand by 1 million hectares in 2050, on average across Rule 1 and 2 the net effect is a decrease in total harvested areas for the three crops estimated at 10 million hectares (3–27 million hectares) compared to BAU. This result is suggestive of a reduced pressure on forests and other natural areas that might be environmentally significant and rich in carbon. However, the reallocation of harvested area following changes in production and prices causes other crops to take over the land freed by rice and wheat (Supplementary Discussion S11 in S1 File ). Total land allocated to soybeans, vegetables, temperate fruits, sugarcane, and rapeseed is estimated to increase by 2 million hectares in 2050 on average across Rule 1 and 2 (1–4 million hectares) (Supplementary Table 13 in S1 File ). Depending on how they are grown, these crops might have a higher carbon footprint than the crops they replace.
Importantly, simulations show that increases in production of grains reduces the price of livestock feed and increases the number of animals that can be supported globally. The stronger the effect on prices, the greater the increase in the global cattle herd. As a result, emissions from cattle may increase by 5.4 Mt CO 2 e yr -1 (1.2–13.1 Mt CO 2 e yr -1 ) by 2050 and partially offset the reductions in emissions discussed earlier (Supplementary Discussion S12 and Supplementary Table S14 in S1 File ).
3.4 Adaptation and resilience
The effects on production, prices, soil, and land use suggest that CSA practices are a form of adaptation to new climate conditions and make crop-production more resilient. A comparison with a scenario in which the effects of climate change on future crop production are removed (a No Climate Change scenario. See Supplementary Discussion S13 and Supplementary Fig 8 in S1 File ) show that the production gains obtained from CSA practices can offset the negative impacts of climate change on maize and rice production and slow down consequent increases in prices. CSA practices are also successful in reducing the price of wheat which, despite an increase in production, is projected to increase in the BAU scenario. It is however difficult to draw broader conclusions about resilience, as this would require a more specific analysis of the differential effects across multiple social contexts, at different geographical scales, and for different social groups [ 71 , 72 ].
3.5 Effects of adoption rates and production costs
Results for the third scenario, which uses lower adoption rates, and the fourth, which includes AWD production costs, are reported in Fig 4 along with the results from Rules 1 and 2. To provide a comprehensive review of all the simulated scenarios, we consider the cumulative fresh weight harvest for the three crops. The results in Fig 4 focus on production and emissions. Similar results for harvested area are presented in Supplementary Table S15 in S1 File .
Columns indicate the average tailoring of CSA practices; whisker bars identify results for the poor and optimal tailoring Source: Authors.
https://doi.org/10.1371/journal.pone.0231764.g004
As expected, social and cost barriers—heuristically included in the simulations by reducing the rate of adoption of CSA practices as in Rosegrant et al [ 9 ]—significantly affect the results with an overall reduction in benefits. Compared to BAU, yearly production for the three crops increases on average by 60 Mt (22–122 M tons) and GHG yearly emissions are reduced on average by 13 Mt CO 2 e (-2–31 Mt CO 2 e, where the negative number indicates an increase in emissions). Therefore, the reduced adoption lowers the crop production gains observed using Rule 1 by about 40% and reduces the effects on emissions by two thirds or more.
When the reduction in production costs for AWD is accounted for, AWD is adopted on some additional 0.8 million hectares leading to the highest achieved emission reduction. Yearly production is essentially unaffected but yearly abatement reaches on average 105 Mt CO 2 e yr -1 (90–134 Mt CO 2 e yr -1 ).
4 Discussion
The largest positive impacts on production and food security as well as the highest levels in GHG emissions abatement, should be interpreted as aspirational targets and viewed as an upper bound of the possible effects of adopting the CSA practices considered across maize, wheat and rice. These results are predicated on high levels of uptake by farmers and their capacity to tailor the implementation of CSA practices to local conditions. The scenario that simulates lower and more realistic adoption rates and the scenarios that represent a lower proficiency at using the practices, show rapidly diminishing benefits. This stresses the importance of major new investments to overcome long-standing problems such as underperforming extension services, farmers’ lack of credit, risk management and timely information about markets. These barriers are known to prevent the adoption of more productive, more resilient and sustainable agricultural systems.
Even though the focus of CSA is not on mitigation benefits, the pressure on the agriculture sector to reduce GHG emissions is likely to increase as other sectors reduce their share of global emissions. The performance of agricultural practices in terms of their abatement potential is therefore important. According to our assessment, the total maximum abatement obtainable (134 Mt CO 2 e yr -1 ) is about 17% of what is considered the economically achievable mitigation from managing cropland, which is 0.77 Gt CO 2 e yr -1 [ 73 ]. Also, after the indirect effects of cattle emissions are accounted for (approximately 4 Mt CO 2 e yr -1 ), the maximum abatement obtainable is 13% of the 1 Gt CO 2 e yr -1 abatement goal for the agriculture sector to remain below the 2°C global warming [ 74 ]. If one considers that the GHG emissions from the three crops analyzed is in the range of 1.28–1.92 CO 2 e yr -1 [ 25 ], our results point to a 7–10% reduction at best of those emissions. Given these limited abatement levels, the large scale adoption of alternative production systems (e.g. silvopastoral systems, agroforestry practices, precision agriculture) should be considered, and additional opportunities for abatement ought to be found elsewhere along the value chains [ 75 ].
Importantly, results indicate that recent concerns expressed in the literature regarding the negative effects of carbon tax on food security might be misplaced. In actuality, carbon pricing could help internalizing the external costs of GHG emission and steer the agriculture sector towards more carbon-efficient methods of production and distribution. Clearly, one can always impose a sufficiently high carbon tax with detrimental effects on the food security of a significant share of the population. However, our results for population at risk of hunger show that alternative practices and technologies, of which only a sample is explored in this study, can limit these effects if not completely offset them. In our analysis we did not explicitly explore the effects of policies that promote a reduction of GHG emissions. However, the results obtained using adoption Rule 2 show that as the emphasis shifts from yield gains to reducing emission intensity, emission abatement increases albeit at the cost of agricultural output. Resolving the tradeoff between emissions reduction and production in an economically efficient manner depends not only on carbon pricing or emission-reduction incentives but also on a proper pricing of the factors of production. An appropriate pricing of inputs like water and nitrogen fertilizers would promote the adoption of water-saving practices such as AWD with no significant reduction of productivity, and the adoption of practices that increase nitrogen use efficiency, with a consequent reduction in emissions and an increase in productivity.
Results also show the importance of understanding how changes caused by the widespread adoption of CSA practices may play on a global scale. The indirect effects arising from the reorganization of global production can lead to larger agricultural areas being allocated to other crops, and to an expansion in the global livestock herd due to cheaper feedstock prices. These effects can be large enough to limit the emission abatement effectiveness of CSA practices. In addition, the heterogeneous changes in countries’ GHG emissions, even in the presence of a global positive outcome, shows the importance of global coordination. Such coordination should also consider the interaction between agricultural land and carbon-rich environments such as forests and peatlands to avoid emission leakage [ 76 , 77 ].
Beyond the specifics of CSA, our results are strongly suggestive that using alternative practices the agriculture sector can increase its output and reduce its carbon footprint under future climate scenarios. However, many are the assumptions that underly our work and much more research must be undertaken to evaluate the global effects of changes in food production systems. Simulating alternative agricultural futures requires a model representation of the main structural drivers of demand and supply of food products and this requires significant assumptions about producers’ and consumers’ behavior. Scenario analyses based on model simulations, of which this study is an example, are not a prediction of the future. They are a representation of possible alternative futures given our current knowledge and assumptions about trends in climate, technology, population, income and other drivers, and about how they may interact in an economic system. Scenarios also rely heavily on existing global datasets, which come with many limitations. One way to test the robustness of the scenarios produced by models that do not generate confidence intervals or goodness-of-fit metrics, like the one we used, is to undertake a sensitivity analysis for some of the key variables. We have done this for climate, adoption levels, and yield performance but many more could be explored. Results from the sensitivity analysis point to the qualitative robustness of some of the findings (e.g. agriculture can withstand the negative impact of climate change using alternative practices, sustainable increase in production while reducing the carbon food print is possible), others indicate where additional research is necessary (e.g. investigate the multiple pathways that link agricultural output with the food security status of vulnerable people, move beyond the emphasis on yield gains and model the effects of crop rotations). Other results reveal the limits of the modeling environment that was used. A perfect example of this are our findings about land use change. The overall effects of higher yields and lower prices is to reduce the need for additional harvested area to fulfill the increasing demands for maize, wheat and rice. This suggests a reduced pressure to expand cropland and potential land-sparing effects. However, IMPACT does not model explicitly the competing demands of all land uses. It only considers cropland area and its agricultural output. While the idea that agricultural intensification reduces agricultural land’s encroachment into other natural areas is not new, it was famously put forward by Borlaug [ 78 ], whether this happens in reality is the subject of some controversy [ 79 – 81 ] and our model cannot directly resolve these questions. More research in this issue is therefore necessary.
One way to verify and test the validity of these scenarios is also to use alternative models after proper calibration [ 82 ] and compare results across them. Although this requires considerable resources, these types of comparisons have been done to assess global issues related to climate change such as agricultural production under future climate regimes [ 82 ], agricultural production and mitigation [ 83 ], and future nutritional challenges [ 84 ]. It would be advisable that a similar exercise is undertaken to explore the merits and benefits of alternative production systems like the one proposed by CSA.
5 Conclusion
Much has been written about CSA and its potential benefits but most of the existing analyses are based on local experiences and no study has so far attempted to quantify these benefits on a global scale. Our results show that widespread adoption of CSA practices can increase production and lower world prices of wheat, maize, and rice under future unfavorable climatic conditions. The reduction in prices is projected to make food products more accessible to millions of people thereby lowering the number of people at risk of hunger and that of undernourished children. These gains can be obtained while improving soil fertility and with a reduction in GHG emissions. Taken all together, results suggest that CSA practice can deliver benefits across its three foundational pillars on a planetary scale.
However, what clearly transpires from our results is that to make a significant impact, the principles of CSA must be applied widely across production systems and for this to occur significant investments must be made. Ideally, as others have suggested [ 85 ], the same or similar principles should be applied across the whole food system (i.e. trade, stocks, nutrition and social policies). It is also clear that the wide-ranging and multidimensional effects, sometime unintended or unforeseen, must be understood and managed. CSA with its multi-objective approach may provide a useful framework for decision-making ranging from the farm to the policy level.
Supporting information
https://doi.org/10.1371/journal.pone.0231764.s001
Acknowledgments
We would like to thank Jennifer Lieberman, Daniel Mason-D'Croz, Keith Wiebe, and Channing Arndt for their help and useful comments. The authors take sole responsibility for the opinions expressed within this article.
- View Article
- Google Scholar
- PubMed/NCBI
- 8. Bank World. World Development Report 2010: Development and Climate Change. Washington, DC: World Bank; 2009.
- 9. Rosegrant MW, Koo J, Cenacchi N, Ringler C, Robertson R, Fisher M, et al. Food Security in a World of Natural Resource Scarcity—The Role of Agricultural Technologies. Washington, DC: International Food Policy Research Institute (IFPRI); 2014.
- 11. UNFCCC. Paris Agreement. United Nations Treaty Collection, available at https://treaties.un.org/pages/ViewDetails.aspx?src=TREATY&mtdsg_no=XXVII-7-d&chapter=27&clang=_en ; 2015.
- 12. UNFCCC Decision 4/CP.23. Report of the Conference of the Parties on its twenty-third session, held in Bonn from 6 to 18 November 2017. Bonn: United Nations Framework Convention on Climate Change (UNFCCC); 2018.
- 13. IPCC. Summary for Policymakers. In: Masson-Delmotte V, Zhai P, Portner HO, Roberts D, Skea J, Shukla PR, et al., editors. Global warming of 15°C An IPCC Special Report on the impacts of global warming of 15°C above pre-industrial levels and related global greenhouse gas emission pathways, in the context of strengthening the global response to the threat of climate change,. Geneva, Switzerland: World Meteorological Organization; 2018. p. 32.
- 18. FAO. Climate Smart Agriculture Sourcebook [Internet]. 2017 [cited 2018 Jun 20]. Available from: http://www.fao.org/climate-smart-agriculture-sourcebook/en/
- 20. McCarthy N, Lipper L, Branca G. Climate-smart agriculture: smallholder adoption and implications for climate change adaptation and mitigation. Mitigation of Climate Change in Agriculture Series 4. Rome, Italy; 2011.
- 28. Robinson S, Mason d’Croz D, Islam S, Sulser TB, Robertson RD, Zhu T, et al. The International Model for Policy Analysis of Agricultural Commodities and Trade (IMPACT): Model description for version 3. Washington, DC: International Food Policy Research Institute (IFPRI); 2015. (IFPRI Discussion Paper; vol. 1483).
- 29. You LZ, Crespo S, Guo Z, Koo J, Ojo W, Sebastian K, et al. Spatial Production Allocation Model (SPAM) 2000 Version 3, Release 2. http://MapSPAM.info . 2006.
- 33. Smith L, Haddad L. Explaining Child Malnutrition in Developing Countries: A Cross-country Analysis. Washington, D.C.; 2000.
- 34. FAO. Module 1: Why Climate-Smart Agriculture, Forestry and Fisheries. In: Climate Smart Agriculture Sourcebook. Rome, Italy: Food and Agriculture Organization of the United Nations (FAO); 2013.
- 51. Derpsch R, Friedrich T. Global Overview of Conservation Agriculture Adoption. In: Proceedings Lead Papers. New Delhi, India; 2009.
- 56. De Klein C, Novoa RSA, Ogle S, Smith KA, Rochette P, Wirth TC, et al. N2O emissions from managed soils, and CO2 emissions from lime and urea application. In: Eggleston HS, Buendia L, MIwa K, Ngara T, Tanabe K, editors. 2006 IPCC Guidelines for National Greenhouse Gas Inventories—Volume 4. Hayama, Kanagawa, Japan: IGES, Japan; 2006.
- 64. Lipper L, McCarthy B, Zilberman D, Asfaw S, Branca G, editors. Climate smart agriculture: building resilience to climate change. Rome, Italy: FAO; 2018.
- 71. Watts MJ. Resilience as a Way of Life: Biopolitical Security, Catastrophism, and the Food–Climate Change Question. In: Chen N, Sharp L, editors. Bioinsecurity and Vulnerability. SAR Press; 2014. p. 145–72.
- 72. Levine S. Assessing resilience: why quantification misses the point. 2014 [cited 2017 Nov 9]; Available from: http://www.odi.org.uk/hpg
- 73. Smith P, Bustamante M, Ahammad H, Clark H, Dong H, Elsiddig EA, et al. Agriculture, Forestry and Other Land Use (AFOLU). In: Edenhofer O, Pichs-Madruga R, Sokona Y, Farahani E, Kadner S, Seyboth K, et al., editors. Climate Change 2014: Mitigation of Climate Change Contribution of Working Group III to the Fifth Assessment Report of the Intergovernmental Panel on Climate Change. Cambridge, United Kingdom and New York, NY, USA: Cambridge University Press; 2015.
- 81. Wiebe KD, Sulser TB, Pacheco P, De Pinto A, Mason-D’Croz D, Dermawan A, et al. The palm oil dilemma: Policy tensions among higher productivity, rising demand, and deforestation. Washington D.C.; 2019.
Thank you for visiting nature.com. You are using a browser version with limited support for CSS. To obtain the best experience, we recommend you use a more up to date browser (or turn off compatibility mode in Internet Explorer). In the meantime, to ensure continued support, we are displaying the site without styles and JavaScript.
- View all journals
Agriculture articles from across Nature Portfolio
Agriculture is the cultivation of plants, animals, and some other organisms, such as fungi, for the production of food, fibre, fuel, and medicines used by society.
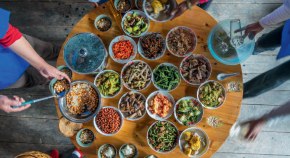
Healthy and sustainable diets must be culturally acceptable too
Dietary shifts are among the most important actions we can take to reduce the environmental impact of our food system and improve human health. However, implementing such changes requires that dietary recommendations be tailored to the cultural heritage, values and preferences of populations.
- Brent Loken
- Nancy Phoebe Rapando
Latest Research and Reviews
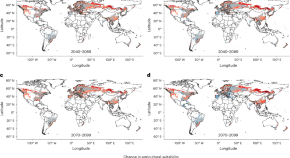
Climate change will exacerbate land conflict between agriculture and timber production
Increases in crop and timber production are necessary to meet rising needs. Here the authors predict how current forestry land is likely to face increased competition from agriculture as climate change shifts land-use suitability.
- Christopher G. Bousfield
- Oscar Morton
- David P. Edwards
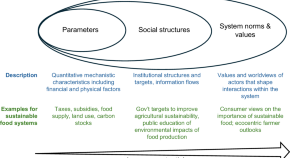
Leverage points for the uptake of organic food production and consumption in the United Kingdom
Short-term economic thinking, landowner engagement with organic farming, and consumers’ willingness to pay for organic food are the most influential factors in organic food production and consumption scale-up, according to an analysis combining data from expert workshops and fuzzy cognitive maps.
- Nicholas Davison
- Laurence G. Smith
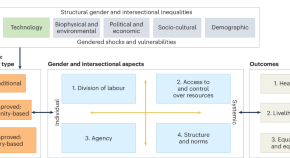
A systematic review of the impact of post-harvest aquatic food processing technology on gender equality and social justice
Fisheries and aquaculture production are key to livelihoods, food and nutrition, yet over one-third of global aquatic harvest is lost or wasted. The post-harvest sector, key to reducing these losses, and dominated by women workers, is not well understood. This systematic review synthesizes evidence on equity and equality outcomes of post-harvest practices and technologies that aim to reduce loss and waste in the fisheries sector.
- Liangzi Zhang
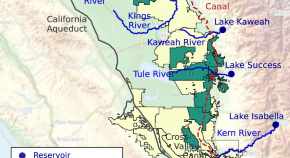
Resilient water infrastructure partnerships in institutionally complex systems face challenging supply and financial risk tradeoffs
Hamilton and colleagues demonstrate critical water supply risks and financial tradeoffs for California utilities investing in cooperative infrastructure projects and develops computational methods for designing more resilient partnerships under uncertainty.
- A. L. Hamilton
- G. W. Characklis
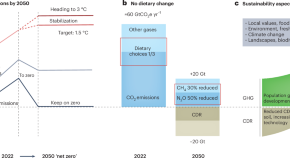
Ruminating on sustainable food systems in a net-zero world
In the midst of debates about climate and environmental impacts of meat, this Perspective uses Norway as a case study to frame a more holistic and nuanced approach to sustainable food production.
- Bob van Oort
- Anne Sophie Daloz
- Nora Svarstad Ytreberg
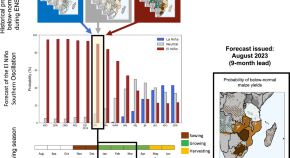
Preseason maize and wheat yield forecasts for early warning of crop failure
Crop failures are potentially predictable much further in advance than previously thought possible. Using multiyear forecasts of the El Niño Southern Oscillation (ENSO), Anderson et al. show that crop failures can be predicted before the planting season even begins in some countries.
- Weston Anderson
- Shraddhanand Shukla
- Amy McNally
News and Comment
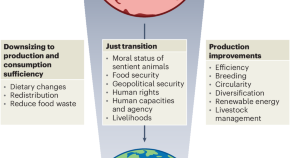
Both downsizing and improvements to livestock systems are needed to stay within planetary boundaries
A focus on improvements to livestock production limits the scope for food systems transformation. Research, policy and industry must adopt measures to downsize livestock production and consumption to meet sustainability targets and facilitate a just transition.
- Irina Herzon
- Rachel Mazac
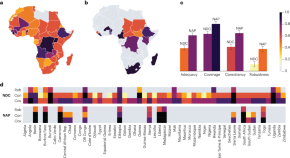
Enhanced policy adequacy facilitates national climate adaptation tracking across Africa
Inadequate information in national adaptation policies limits the ability to track national adaptation progress in Africa. Enhancing coverage, consistency and robustness of these policies offers a clear path to establish effective, nationally led adaptation-tracking infrastructure.
- Andreea C. Nowak
- Lucy Njuguna
- Todd S. Rosenstock
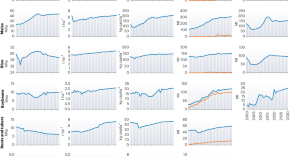
Strategic cropland reserves can strengthen China’s food security
Policies for supporting domestic grain production propose converting large areas of marginal and low-grade arable land into strategic cropland reserves. This process will require advances in science and land engineering, and presents opportunities to revitalize social, economic and ecological systems in rural China.
- Jichang Han
- Brett Anthony Bryan
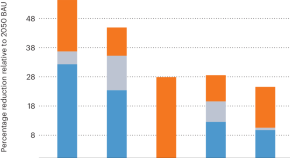
Holistic systems analyses accelerate progress towards Sustainable Development Goals
Food systems innovations — including strategic adoption of crop and livestock breeding technologies, land-use optimization and food waste inhibitors — diminish the need to import protein and avoid greenhouse gas emissions.
- Matthew Tom Harrison
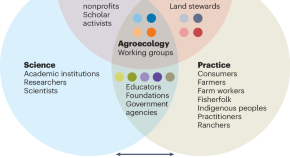
Momentum for agroecology in the USA
Despite decades of resistance in the USA, agroecology is gaining momentum as a catalyst for food systems transformation, calling for coordinated action between science, practice and movement to dismantle the dominant industrial paradigm.
- Theresa W. Ong
- Antonio Roman-Alcalá
- Hannah Duff
Quick links
- Explore articles by subject
- Guide to authors
- Editorial policies

Information
- Author Services
Initiatives
You are accessing a machine-readable page. In order to be human-readable, please install an RSS reader.
All articles published by MDPI are made immediately available worldwide under an open access license. No special permission is required to reuse all or part of the article published by MDPI, including figures and tables. For articles published under an open access Creative Common CC BY license, any part of the article may be reused without permission provided that the original article is clearly cited. For more information, please refer to https://www.mdpi.com/openaccess .
Feature papers represent the most advanced research with significant potential for high impact in the field. A Feature Paper should be a substantial original Article that involves several techniques or approaches, provides an outlook for future research directions and describes possible research applications.
Feature papers are submitted upon individual invitation or recommendation by the scientific editors and must receive positive feedback from the reviewers.
Editor’s Choice articles are based on recommendations by the scientific editors of MDPI journals from around the world. Editors select a small number of articles recently published in the journal that they believe will be particularly interesting to readers, or important in the respective research area. The aim is to provide a snapshot of some of the most exciting work published in the various research areas of the journal.
Original Submission Date Received: .
- Active Journals
- Find a Journal
- Proceedings Series
- For Authors
- For Reviewers
- For Editors
- For Librarians
- For Publishers
- For Societies
- For Conference Organizers
- Open Access Policy
- Institutional Open Access Program
- Special Issues Guidelines
- Editorial Process
- Research and Publication Ethics
- Article Processing Charges
- Testimonials
- Preprints.org
- SciProfiles
- Encyclopedia
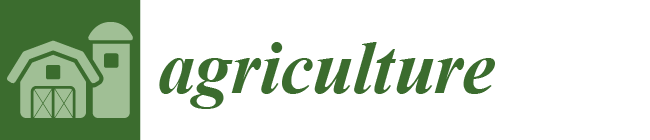
Article Menu
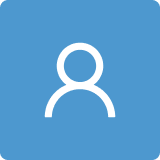
- Subscribe SciFeed
- Recommended Articles
- Google Scholar
- on Google Scholar
- Table of Contents
Find support for a specific problem in the support section of our website.
Please let us know what you think of our products and services.
Visit our dedicated information section to learn more about MDPI.
JSmol Viewer
Research progress on autonomous operation technology for agricultural equipment in large fields.
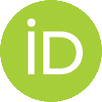
1. Introduction
2. onboard environmental sensing technology, 3. complete-coverage path-planning technology, 3.1. classical path-planning algorithm, 3.2. bionics-based path-planning algorithms, 4. autonomous operation control technology, 5. conclusions and prospection, 5.1. conclusions, 5.2. prospection, author contributions, data availability statement, conflicts of interest.
- Charania, I.; Li, X. Smart farming: Agriculture’s shift from a labor intensive to technology native industry. Internet Things 2020 , 9 , 100142. [ Google Scholar ] [ CrossRef ]
- Ren, C.; Zhou, X.; Wang, C.; Guo, Y.; Diao, Y.; Shen, S.; Reis, S.; Li, W.; Xu, J.; Gu, B. Ageing threatens sustainability of smallholder farming in China. Nature 2023 , 616 , 96–103. [ Google Scholar ] [ CrossRef ]
- Liu, J.; Fang, Y.; Wang, G.; Liu, B.; Wang, R. The aging of farmers and its challenges for labor-intensive agriculture in China: A perspective on farmland transfer plans for farmers’ retirement. J. Rural. Stud. 2023 , 100 , 103013. [ Google Scholar ] [ CrossRef ]
- Chen, R.; Xu, P.; Song, P.; Wang, M.; He, J. China has faster pace than Japan in population aging in next 25 years. Biosci. Trends 2019 , 13 , 287–291. [ Google Scholar ] [ CrossRef ] [ PubMed ]
- Yu, Y.; Zhang, J.; Zhang, K.; Xu, D.; Qi, Y.; Deng, X. The impacts of farmer ageing on farmland ecological restoration technology adoption: Empirical evidence from rural China. J. Clean. Prod. 2023 , 430 , 139648. [ Google Scholar ] [ CrossRef ]
- Cui, Z.; Zhang, H.; Chen, X.; Zhang, C.; Ma, W.; Huang, C.; Zhang, W.; Mi, G.; Miao, Y.; Li, X.; et al. Pursuing sustainable productivity with millions of smallholder farmers. Nature 2018 , 555 , 363–366. [ Google Scholar ] [ CrossRef ] [ PubMed ]
- Zhang, Q.; Chu, Y.; Xue, Y.; Ying, H.; Chen, X.; Zhao, Y.; Ma, W.; Ma, L.; Zhang, J.; Yin, Y.; et al. Outlook of China’s agriculture transforming from smallholder operation to sustainable production. Glob. Food Secur. 2020 , 26 , 100444. [ Google Scholar ] [ CrossRef ]
- Yao, Z.; Zhao, C.; Zhang, T. Agricultural machinery automatic navigation technology. Iscience 2024 , 27 , 108714. [ Google Scholar ] [ CrossRef ] [ PubMed ]
- Shuai, L.; Li, Z.; Chen, Z.; Luo, D.; Mu, J. A research review on deep learning combined with hyperspectral Imaging in multiscale agricultural sensing. Comput. Electron. Agric. 2024 , 217 , 108577. [ Google Scholar ] [ CrossRef ]
- Ariza-Sentís, M.; Vélez, S.; Martínez-Peña, R.; Baja, H.; Valente, J. Object detection and tracking in Precision Farming: A systematic review. Comput. Electron. Agric. 2024 , 219 , 108757. [ Google Scholar ] [ CrossRef ]
- Zhang, S.; Liu, Y.; Xiong, K.; Tian, Y.; Du, Y.; Zhu, Z.; Du, M.; Zhai, Z. A review of vision-based crop row detection method: Focusing on field ground autonomous navigation operations. Comput. Electron. Agric. 2024 , 222 , 109086. [ Google Scholar ] [ CrossRef ]
- Liu, H.; Li, K.; Ma, L.; Meng, Z. Headland Identification and Ranging Method for Autonomous Agricultural Machines. Agriculture 2024 , 14 , 243. [ Google Scholar ] [ CrossRef ]
- Liang, C.; Pan, K.; Zhao, M.; Lu, M. Multi-Node Path Planning of Electric Tractor Based on Improved Whale Optimization Algorithm and Ant Colony Algorithm. Agriculture 2023 , 13 , 586. [ Google Scholar ] [ CrossRef ]
- Wan, H.; Ou, Y.; Guan, X.; Jiang, R.; Zhou, Z.; Luo, X. Review of the perception technologies for unmanned agricultural machinery operating environment. Trans. CSAE 2024 , 40 , 1–18. [ Google Scholar ] [ CrossRef ]
- Bai, Y.; Zhang, B.; Xu, N.; Zhou, J.; Shi, J.; Diao, Z. Vision-based navigation and guidance for agricultural autonomous vehicles and robots: A review. Comput. Electron. Agric. 2023 , 205 , 107584. [ Google Scholar ] [ CrossRef ]
- Barbedo, J.G.A. Data Fusion in Agriculture: Resolving Ambiguities and Closing Data Gaps. Sensors 2022 , 22 , 2285. [ Google Scholar ] [ CrossRef ]
- Höffmann, M.; Patel, S.; Büskens, C. Optimal guidance track generation for precision agriculture: A review of coverage path planning techniques. J. Field Robot. 2024 , 41 , 823–844. [ Google Scholar ] [ CrossRef ]
- Sánchez-Ibáñez, J.R.; Pérez-del-Pulgar, C.J.; García-Cerezo, A. Path Planning for Autonomous Mobile Robots: A Review. Sensors 2021 , 21 , 7898. [ Google Scholar ] [ CrossRef ]
- Zhang, G.; Ji, C.; Wu, Q.; Liu, H.; Zhou, Y.; Fu, J. Study on path planning of mechanized harvesting of ratoon rice in the first season based on the capacitated arc routing problem model. Front. Plant Sci. 2022 , 13 , 963307. [ Google Scholar ] [ CrossRef ]
- Guan, Z.; Li, Y.; Mu, S.; Zhang, M.; Jiang, T.; Li, H.; Wang, G.; Wu, C. Tracing algorithm and control strategy for crawler rice combine harvester auxiliary navigation system. Biosyst. Eng. 2021 , 211 , 50–62. [ Google Scholar ] [ CrossRef ]
- An, G.; Zhong, Z.; Yang, S.; Yang, L.; Jin, C.; Du, J.; Yin, X. EASS: An automatic steering system for agricultural wheeled vehicles using fuzzy control. Comput. Electron. Agric. 2024 , 217 , 108544. [ Google Scholar ] [ CrossRef ]
- Zhao, X.; Lu, E.; Tang, Z.; Luo, C.; Xu, L.; Wang, H. Trajectory prediction method for agricultural tracked robots based on slip parameter estimation. Comput. Electron. Agric. 2024 , 222 , 109057. [ Google Scholar ] [ CrossRef ]
- Wang, Q.; Meng, Z.; Wen, C.; Qin, W.; Wang, F.; Zhang, A.; Zhao, C.; Yin, Y. Grain combine harvester header profiling control system development and testing. Comput. Electron. Agric. 2024 , 223 , 109082. [ Google Scholar ] [ CrossRef ]
- Cui, Z.; Hu, J.; Yu, Y.; Cao, G.; Zhang, H.; Chai, X.; Chen, H.; Xu, L. Automatic grain unloading method for track-driven rice combine harvesters based on stereo vision. Comput. Electron. Agric. 2024 , 220 , 108917. [ Google Scholar ] [ CrossRef ]
- Rovira-Mas, F.; Saiz-Rubio, V.; Cuenca-Cuenca, A. Augmented Perception for Agricultural Robots Navigation. IEEE Sens. J. 2021 , 21 , 11712–11727. [ Google Scholar ] [ CrossRef ]
- Xie, D.; Chen, L.; Liu, L.; Chen, L.; Wang, H. Actuators and Sensors for Application in Agricultural Robots: A Review. Machines 2022 , 10 , 913. [ Google Scholar ] [ CrossRef ]
- Lu, H.; Wang, H.; Ma, Z.; Ren, Y.; Fu, W.; Shan, Y.; Hu, S.; Zhang, G.; Meng, Z. Farmland boundary extraction based on the AttMobile-DeeplabV3+ network and least squares fitting of straight lines. Front. Plant Sci. 2023 , 14 , 1228590. [ Google Scholar ] [ CrossRef ]
- Hong, Q.; Zhu, Y.; Liu, W.; Ren, T.; Shi, C.; Lu, Z.; Yang, Y.; Deng, R.; Qian, J.; Tan, C. A segmentation network for farmland ridge based on encoder-decoder architecture in combined with strip pooling module and ASPP. Front. Plant Sci. 2024 , 15 , 1328075. [ Google Scholar ] [ CrossRef ]
- Yang, Y.; Shen, X.; An, D.; Han, H.; Tang, W.; Wang, Y.; Yang, Y.; Ma, Q.; Chen, L. Crop Row Detection Algorithm Based on 3-D LiDAR: Suitable for Crop Row Detection in Different Periods. IEEE Trans. Instrum. Meas. 2024 , 73 , 8503413. [ Google Scholar ] [ CrossRef ]
- Campos, Y.; Sossa, H.; Pajares, G. Spatio-temporal analysis for obstacle detection in agricultural videos. Appl. Soft Comput. 2016 , 45 , 86–97. [ Google Scholar ] [ CrossRef ]
- Pini, M.; Marucco, G.; Falco, G.; Nicola, M.; De Wilde, W. Experimental Testbed and Methodology for the Assessment of RTK GNSS Receivers Used in Precision Agriculture. IEEE Access 2020 , 8 , 14690–14703. [ Google Scholar ] [ CrossRef ]
- Jiménez-Martínez, M.J.; Farjas-Abadia, M.; Quesada-Olmo, N. An Approach to Improving GNSS Positioning Accuracy Using Several GNSS Devices. Remote Sens. 2021 , 13 , 1149. [ Google Scholar ] [ CrossRef ]
- Perez-Ruiz, M.; Martínez-Guanter, J.; Upadhyaya, S.K. Chapter 15—High-precision GNSS for agricultural operations. In GPS and GNSS Technology in Geosciences ; Elsevier: Amsterdam, The Netherlands, 2021; pp. 299–335. [ Google Scholar ] [ CrossRef ]
- Chen, Y.; Li, G.; Zhang, X.; Jia, J.; Zhou, K.; Wu, C. Identifying field and road modes of agricultural Machinery based on GNSS Recordings: A graph convolutional neural network approach. Comput. Electron. Agric. 2022 , 198 , 107082. [ Google Scholar ] [ CrossRef ]
- Moeller, R.; Deemyad, T.; Sebastian, A. Autonomous Navigation of an Agricultural Robot Using RTK GPS and Pixhawk. In Proceedings of the 2020 Intermountain Engineering, Technology and Computing (IETC), Orem, UT, USA, 2–3 October 2020; IEEE: Piscataway, NJ, USA, 2020; pp. 1–6. [ Google Scholar ] [ CrossRef ]
- Ren, H.; Wu, J.; Lin, T.; Yao, Y.; Liu, C. Research on an Intelligent Agricultural Machinery Unmanned Driving System. Agriculture 2023 , 13 , 1907. [ Google Scholar ] [ CrossRef ]
- Huang, Y.; Fu, J.; Xu, S.; Han, T.; Liu, Y. Research on Integrated Navigation System of Agricultural Machinery Based on RTK-BDS/INS. Agriculture 2022 , 12 , 1169. [ Google Scholar ] [ CrossRef ]
- LeVoir, S.J.; Farley, P.A.; Sun, T.; Xu, C. High-Accuracy Adaptive Low-Cost Location Sensing Subsystems for Autonomous Rover in Precision Agriculture. IEEE Open J. Ind. Appl. 2020 , 1 , 74–94. [ Google Scholar ] [ CrossRef ]
- Liang, X.; Huang, Z.; Qin, H. Differential positioning based on the orthogonal transformation algorithm with GNSS multi-system. GPS Solut. 2018 , 22 , 89. [ Google Scholar ] [ CrossRef ]
- Yue, B.; Zhang, Z.; Zhang, W.; Luo, X.; Zhang, G.; Huang, H.; Wu, X.; Bao, K.; Peng, M. Design of an Automatic Navigation and Operation System for a Crawler-Based Orchard Sprayer Using GNSS Positioning. Agronomy 2024 , 14 , 271. [ Google Scholar ] [ CrossRef ]
- Kaivosoja, J.; Linkolehto, R. GNSS error simulator for farm machinery navigation development. Comput. Electron. Agric. 2015 , 119 , 166–177. [ Google Scholar ] [ CrossRef ]
- Lee, K.; Choi, H.; Kim, J. Development of Path Generation and Algorithm for Autonomous Combine Harvester Using Dual GPS Antenna. Sensors 2023 , 23 , 4944. [ Google Scholar ] [ CrossRef ]
- Vulpi, F.; Marani, R.; Petitti, A.; Reina, G.; Milella, A. An RGB-D multi-view perspective for autonomous agricultural robots. Comput. Electron. Agric. 2022 , 202 , 107419. [ Google Scholar ] [ CrossRef ]
- Galati, R.; Mantriota, G.; Reina, G. RoboNav: An Affordable Yet Highly Accurate Navigation System for Autonomous Agricultural Robots. Robotics 2022 , 11 , 99. [ Google Scholar ] [ CrossRef ]
- Valente, D.S.M.; Momin, A.; Grift, T.; Hansen, A. Accuracy and precision evaluation of two low-cost RTK global navigation satellite systems. Comput. Electron. Agric. 2020 , 168 , 105142. [ Google Scholar ] [ CrossRef ]
- Jing, Y.; Li, Q.; Ye, W.; Liu, G. Development of a GNSS/INS-based automatic navigation land levelling system. Comput. Electron. Agric. 2023 , 213 , 108187. [ Google Scholar ] [ CrossRef ]
- Li, S.; Zhang, M.; Ji, Y.; Zhang, Z.; Cao, R.; Chen, B.; Li, H.; Yin, Y. Agricultural machinery GNSS/IMU-integrated navigation based on fuzzy adaptive finite impulse response Kalman filtering algorithm. Comput. Electron. Agric. 2021 , 191 , 106524. [ Google Scholar ] [ CrossRef ]
- Hong, Z.; Li, Y.; Lin, H.; Gong, L.; Liu, C. Field Boundary Distance Detection Method in Early Stage of Planting Based on Binocular Vision. Trans. CSAM 2022 , 53 , 27–33. [ Google Scholar ] [ CrossRef ]
- Wang, Q.; Liu, H.; Yang, P.; Meng, Z. Detection Method of Headland Boundary Line Based on Machine Vision. Trans. CSAM 2020 , 51 , 18–27. [ Google Scholar ] [ CrossRef ]
- Li, D.; Li, B.; Long, S.; Feng, H.; Wang, Y.; Wang, J. Robust detection of headland boundary in paddy fields from continuous RGB-D images using hybrid deep neural networks. Comput. Electron. Agric. 2023 , 207 , 107713. [ Google Scholar ] [ CrossRef ]
- Yang, L.; Xu, Y.; Li, Y.; Chang, M.; Chen, Z.; Lan, Y.; Wu, C. Real-Time field road freespace extraction for agricultural machinery autonomous driving based on LiDAR. Comput. Electron. Agric. 2023 , 211 , 108028. [ Google Scholar ] [ CrossRef ]
- Turner, R.; Panciera, R.; Tanase, M.A.; Lowell, K.; Hacker, J.M.; Walker, J.P. Estimation of soil surface roughness of agricultural soils using airborne LiDAR. Remote Sens. Environ. 2014 , 140 , 107–117. [ Google Scholar ] [ CrossRef ]
- Wang, X.; Shu, L.; Han, R.; Yang, F.; Gordon, T.; Wang, X.; Xu, H. A Survey of Farmland Boundary Extraction Technology Based on Remote Sensing Images. Electronics 2023 , 12 , 1156. [ Google Scholar ] [ CrossRef ]
- Waldner, F.; Diakogiannis, F.I. Deep learning on edge: Extracting field boundaries from satellite images with a convolutional neural network. Remote Sens. Environ. 2020 , 245 , 111741. [ Google Scholar ] [ CrossRef ]
- Hou, W.; Zhou, C.; Cheng, Y.; Wang, Y.; Liu, L.; Qin, K. Path recognition method of orchard ridges based on lightweight U-Net. Trans. CSAM 2024 , 55 , 16–27. [ Google Scholar ] [ CrossRef ]
- Gong, J.; Wang, X.; Zhang, Y.; Lan, Y.; Mostafa, K. Navigation line extraction based on root and stalk composite locating points. Comput. Electr. Eng. 2021 , 92 , 107115. [ Google Scholar ] [ CrossRef ]
- Diao, Z.; Guo, P.; Zhang, B.; Zhang, D.; Yan, J.; He, Z.; Zhao, S.; Zhao, C.; Zhang, J. Navigation line extraction algorithm for corn spraying robot based on improved YOLOv8s network. Comput. Electron. Agric. 2023 , 212 , 108049. [ Google Scholar ] [ CrossRef ]
- Yang, Y.; Mang, Q.; Chen, Z.; Wen, X.; Zhang, G.; Zhang, T.; Dong, X.; Chen, L. Real-time extraction of the navigation lines between sugarcane ridges using LiDAR. Trans. CSAE 2022 , 38 , 178–185. [ Google Scholar ] [ CrossRef ]
- Zhou, H.; Yang, Y.; Liu, Y.; Ma, R.; Zhang, F.; Zang, Q. Real-time Extraction of Navigation Line Based on LiDAR. Trans. CSAM 2023 , 54 , 9–17. [ Google Scholar ] [ CrossRef ]
- Wang, S.; Song, J.; Qi, P.; Yuan, C.; Wu, H.; Zhang, L.; Liu, W.; Liu, Y.; He, X. Design and development of orchard autonomous navigation spray system. Front. Plant Sci. 2022 , 13 , 960686. [ Google Scholar ] [ CrossRef ] [ PubMed ]
- Liu, X.; Zhang, C.; Zhang, H.; Yang, S.; Jiang, S.; Zheng, Y.; Su, D.; Wan, C. Inter-row automatic navigation method by combining least square and SVM in forestry. Trans. CSAE 2021 , 37 , 157–164. [ Google Scholar ] [ CrossRef ]
- He, Y.; Jiang, H.; Fang, H.; Wang, Y.; Liu, Y. Research progress of intelligent obstacle detection methods of vehicles and their application on agriculture. Trans. Chin. Soc. Agric. Eng. 2018 , 34 , 21–32. [ Google Scholar ] [ CrossRef ]
- Li, Y.; Li, M.; Qi, J.; Zhou, D.; Zou, Z.; Liu, K. Detection of typical obstacles in orchards based on deep convolutional neural network. Comput. Electron. Agric. 2021 , 181 , 105932. [ Google Scholar ] [ CrossRef ]
- Xue, J.; Cheng, F.; Li, Y.; Song, Y.; Mao, T. Detection of Farmland Obstacles Based on an Improved YOLOv5s Algorithm by Using CIoU and Anchor Box Scale Clustering. Sensors 2022 , 22 , 1790. [ Google Scholar ] [ CrossRef ] [ PubMed ]
- Xu, H.; Li, S.; Ji, Y.; Cao, R.; Zhang, M. Dynamic obstacle detection based on panoramic vision in the moving state of agricultural machineries. Comput. Electron. Agric. 2021 , 184 , 106104. [ Google Scholar ] [ CrossRef ]
- Du, X.; Li, Z.; Ma, Z.; Yang, Z.; Wang, D. UAV Field Obstacle Detection Based on Spatial Attention and Deformable Convolution. Trans. CSAM 2023 , 54 , 275–283. [ Google Scholar ] [ CrossRef ]
- Shang, Y.; Zhang, G.; Meng, Z.; Wang, H.; Su, C.; Song, Z. Field Obstacle Detection Method of 3D LiDAR Point Cloud Based on Euclidean Clustering. Trans. CSAM 2022 , 53 , 23–32. [ Google Scholar ] [ CrossRef ]
- Wang, T.; Wang, N.; Xiao, J.; Miao, Y.; Sun, Y.; Li, H.; Zhang, M. One-shot domain adaptive real-time 3D obstacle detection in farmland based on semantic-geometry-intensity fusion strategy. Comput. Electron. Agric. 2023 , 214 , 108264. [ Google Scholar ] [ CrossRef ]
- Xue, J.; Cheng, F.; Wang, B.; Li, Y.; Ma, Z.; Chu, Y. Method for Millimeter Wave Radar Farm Obstacle Detection Based on Invalid Target Filtering. Trans. CSAM 2023 , 54 , 233–240. [ Google Scholar ] [ CrossRef ]
- Ball, D.; Upcroft, B.; Wyeth, G.; Corke, P.; English, A.; Ross, P.; Patten, T.; Fitch, R.; Sukkarieh, S.; Bate, A. Vision-based Obstacle Detection and Navigation for an Agricultural Robot. J. Field Robot. 2016 , 33 , 1107–1130. [ Google Scholar ] [ CrossRef ]
- Lv, P.; Wang, B.; Cheng, F.; Xue, J. Multi-Objective Association Detection of Farmland Obstacles Based on Information Fusion of Millimeter Wave Radar and Camera. Sensors 2022 , 23 , 230. [ Google Scholar ] [ CrossRef ]
- Cai, D. Research on Autonomous Operation Perception Technology in Unstructured Farmland Environment. Master’s Thesis, Shanghai Jiao Tong University, Shanghai, China, 2020. [ Google Scholar ]
- Kragh, M.; Underwood, J. Multimodal obstacle detection in unstructured environments with conditional random fields. J. Field Robot. 2020 , 37 , 53–72. [ Google Scholar ] [ CrossRef ]
- Liu, L.; Wang, X.; Liu, H.; Li, J.; Wang, P.; Yang, X. A Full-Coverage Path Planning Method for an Orchard Mower Based on the Dung Beetle Optimization Algorithm. Agriculture 2024 , 14 , 865. [ Google Scholar ] [ CrossRef ]
- Shen, Y.; Liu, Z.; Liu, H.; Du, W. Orchard Spray Robot Planning Algorithm Based on Multiple Constraints. Trans. CSAM 2023 , 54 , 56–67. [ Google Scholar ] [ CrossRef ]
- Xu, X.; Zeng, J.; Zhao, Y.; Lü, X. Research on global path planning algorithm for mobile robots based on improved A*. Expert Syst. Appl. 2024 , 243 , 122922. [ Google Scholar ] [ CrossRef ]
- Feng, Z.; Zhou, L.; Qi, J.; Hong, S. DBVS-APF-RRT*: A global path planning algorithm with ultra-high speed generation of initial paths and high optimal path quality. Expert Syst. Appl. 2024 , 249 , 123571. [ Google Scholar ] [ CrossRef ]
- Kong, F.; Liu, B.; Han, X.; Yi, L.; Sun, H.; Liu, J.; Liu, L.; Lan, Y. Path Planning Algorithm of Orchard Fertilization Robot Based on Multi-Constrained Bessel Curve. Agriculture 2024 , 14 , 979. [ Google Scholar ] [ CrossRef ]
- Wu, Z.; Dai, J.; Jiang, B.; Karimi, H.R. Robot Path Planning Based on Artificial Potential Field with Deterministic Annealing. ISA Trans. 2023 , 138 , 74–87. [ Google Scholar ] [ CrossRef ] [ PubMed ]
- Cui, J.; Zhang, X.; Fan, X.; Feng, W.; Li, P.; Wu, Y. Path planning of autonomous agricultural machineries in complex rural road. J. Eng. 2020 , 6 , 239–245. [ Google Scholar ] [ CrossRef ]
- Boryga, M.; Kołodziej, P.; Gołacki, K. Application of Polynomial Transition Curves for Trajectory Planning on the Headlands. Agriculture 2020 , 10 , 144. [ Google Scholar ] [ CrossRef ]
- He, Y.; Zhou, J.; Sun, J.; Jia, H.; Gemechu, T.-T. Traveling control method adapted to different paddy ground conditions with feedforward compensation for crawler combine harvester based on online tracking error prediction. Comput. Electron. Agric. 2024 , 220 , 108853. [ Google Scholar ] [ CrossRef ]
- He, Y.; Zhou, J.; Yuan, L.; Zheng, P.; Liang, Z. Local Tracking Path Planning Based on Steering Characteristics of Crawler-type Combine Harvester. Trans. CSAM 2022 , 53 , 13–21. [ Google Scholar ] [ CrossRef ]
- He, Y.; Zhou, J.; Sun, J.; Jia, H.; Liang, Z.; Awuah, E. An adaptive control system for path tracking of crawler combine harvester based on paddy ground conditions identification. Comput. Electron. Agric. 2023 , 210 , 107948. [ Google Scholar ] [ CrossRef ]
- Qi, Z.; Zhou, J.; Ye, Z.; Zhou, X.; Yang, H. Local Path Planning Method in Automatic Walking of Single-side Brake Steering Track Chassis. Trans. CSAM 2024 , 55 , 52–59. [ Google Scholar ] [ CrossRef ]
- Yang, Y.; Li, Y.; Wen, X.; Zhang, G.; Ma, Q.; Cheng, S.; Qi, J.; Xu, L.; Chen, L. An optimal goal point determination algorithm for automatic navigation of agricultural machinery: Improving the tracking accuracy of the Pure Pursuit algorithm. Comput. Electron. Agric. 2022 , 194 , 106760. [ Google Scholar ] [ CrossRef ]
- Liu, L.; Wang, X.; Yang, X.; Liu, H.; Li, J.; Wang, P. Path planning techniques for mobile robots: Review and prospect. Expert Syst. Appl. 2023 , 227 , 120254. [ Google Scholar ] [ CrossRef ]
- Kala, R. Multi-robot path planning using co-evolutionary genetic programming. Expert Syst. Appl. 2012 , 39 , 3817–3831. [ Google Scholar ] [ CrossRef ]
- Ab Wahab, M.N.; Nazir, A.; Khalil, A.; Ho, W.J.; Akbar, M.F.; Noor, M.H.M.; Mohamed, A.S.A. Improved genetic algorithm for mobile robot path planning in static environments. Expert Syst. Appl. 2024 , 249 , 123762. [ Google Scholar ] [ CrossRef ]
- Shang, Y.; Shen, J.; Wei, W.; Zheng, B. Optimization of Ball Mill Cylinder Structure Based on Response Surface Optimization Module and Multi-objective Genetic Algorithm. J. Mech. Sci. Technol. 2024 , 38 , 3631–3640. [ Google Scholar ] [ CrossRef ]
- Hilli, A.A.; Al-Ibadi, M.; Alfadhel, A.M.; Abdulshaheed, S.H.; Hadi, A.H. Optimal path finding in stochastic quasi-dynamic environments using particle swarm optimization. Expert Syst. Appl. 2021 , 186 , 115706. [ Google Scholar ] [ CrossRef ]
- Linker, R.; Blass, T. Path-planning algorithm for vehicles operating in orchards. Biosyst. Eng. 2008 , 101 , 152–160. [ Google Scholar ] [ CrossRef ]
- Reda, M.; Onsy, A.; Haikal, A.Y.; Ghanbari, A. Path planning algorithms in the autonomous driving system: A comprehensive review. Robot. Auton. Syst. 2024 , 174 , 104630. [ Google Scholar ] [ CrossRef ]
- Zhou, L.; Liu, T.; Lu, J. Traversal Path Planning for Farmland in Hilly Areas based on Floyd and Im-proved Genetic Algorithm. Smart Agric. 2023 , 4 , 45–57. [ Google Scholar ] [ CrossRef ]
- Xu, L.; You, J.; Yuan, H. Real-Time Parametric Path Planning Algorithm for Agricultural Machinery Kinematics Model Based on Particle Swarm Optimization. Agriculture 2023 , 13 , 1960. [ Google Scholar ] [ CrossRef ]
- Zhao, Z.; Liu, S.; Wei, J.; Qin, F. Improved biological neural network approach for path planning of differential drive agricultural robots with arbitrary shape. Comput. Electron. Agric. 2024 , 216 , 108525. [ Google Scholar ] [ CrossRef ]
- Zhang, X.; Guo, Y.; Yang, J.; Li, D.; Wang, Y.; Zhao, R. Many-objective evolutionary algorithm based agricultural mobile robot route planning. Comput. Electron. Agric. 2022 , 200 , 107274. [ Google Scholar ] [ CrossRef ]
- Wu, L.; Huang, X.; Cui, J.; Liu, C.; Xiao, W. Modified adaptive ant colony optimization algorithm and its application for solving path planning of mobile robot. Expert Syst. Appl. 2023 , 215 , 119410. [ Google Scholar ] [ CrossRef ]
- Wang, Z.; Liu, Z.; Bai, X.; Gao, L. Longitudinal Acceleration Tracking Control of Tractor Cruise System. Trans. CSAM 2018 , 49 , 21–28. [ Google Scholar ] [ CrossRef ]
- Miao, H.; Diao, P.; Xu, G.; Yao, W.; Song, Z.; Wang, W. Research on decoupling control for the longitudinal and lateral dynamics of a tractor considering steering delay. Sci. Rep. 2022 , 12 , 13997. [ Google Scholar ] [ CrossRef ]
- Wang, F.; Yang, Q.; Zhang, Z.; Li, A.; Xu, H. Path Tracking and Turning Control Algorithm of Tracked Vehicle Based on ICR. Trans. CSAM 2024 , 55 , 386–395. [ Google Scholar ] [ CrossRef ]
- Shan, Y.; Zheng, B.; Chen, L.; Chen, L.S.; Chen, D. A Reinforcement Learning-Based Adaptive Path Tracking Approach for Autonomous Driving. IEEE T Veh. Technol. 2020 , 69 , 10581–10595. [ Google Scholar ] [ CrossRef ]
- Zhang, W.; Gai, J.; Zhang, Z.; Tang, L.; Liao, Q.; Ding, Y. Double-DQN based path smoothing and tracking control method for robotic vehicle navigation. Comput. Electron. Agric. 2019 , 166 , 104985. [ Google Scholar ] [ CrossRef ]
- Wang, Q.; Wang, X.; Wang, W.; Song, Y.; Cui, Y. Joint control method based on speed and slip rate switching in plowing operation of wheeled electric tractor equipped with sliding battery pack. Comput. Electron. Agric. 2023 , 215 , 108426. [ Google Scholar ] [ CrossRef ]
- Tan, H.; Wang, G.; Zhou, S.; Jia, H.; Qu, M.; Xiang, M.; Gao, X.; Zhou, Z.; Li, H.; Zou, Z. Design and Experiment of Header Height Adaptive Adjustment System for Maize ( Zea mays L.) Harvester. Sustainability 2023 , 15 , 14137. [ Google Scholar ] [ CrossRef ]
- Xue, B.; Zhou, L.; Niu, K.; Zheng, Y.; Bai, S.; Gui, L. Sowing Depth Control System of Wheat Planter Based on Adaptive Fuzzy PID. Trans. CSAM 2023 , 54 , 93–102. [ Google Scholar ] [ CrossRef ]
- Jing, Y.; Liu, G.; Jin, Z. Adaptive Guidance Control Method with Sideslip Estimation for Land Leveler Pulling by Tractor. Trans. CSAM 2020 , 51 , 26–33. [ Google Scholar ] [ CrossRef ]
Click here to enlarge figure
Method | Sensor Type | Characteristics | Sensing Task |
---|---|---|---|
Vision Sensors | Monocular Camera | Monocular cameras are low cost and provide rich image information, but lack depth data and are susceptible to environmental influences. | Farmland boundary detection, navigation line extraction |
Binocular Camera | Binocular cameras can provide rich image information and highly reliable depth information, but the configuration and calibration are more complicated; the computation is large, and parallax calculation depends on computing resources. | Farmland boundary detection, navigation line extraction | |
RGB-D Camera | RGB-D camera can provide an RGB map and a depth map, and the calculation amount is small. However, the measurement range is narrow, the noise level is high, the field of view is small, and it is easily interfered with by daylight. | Farmland boundary detection | |
Radar Sensor | Lidar | LIDAR is highly accurate, stable, and reliable. However, it has a high cost, is susceptible to dust interference with the limited detection range, and cannot recognize color and texture in farmland boundary identification and navigation line extraction. | Navigation line extraction |
Camera Type | Features | Advantages | Common Cameras |
---|---|---|---|
RGB Cameras | A standard color camera that captures images in the red, green, and blue color channels. | RGB cameras provide rich color and texture information that helps distinguish between different types of obstacles, are low cost, and are easy to integrate and deploy. | Logitech C920, Sony Alpha Series (Logitech, Lausanne, Switzerland) |
Depth Camera | In addition to capturing RGB images, it also acquires depth information for each pixel. | Combining depth information and RGB images improves the accuracy and reliability of obstacle detection, providing more precise obstacle localization, especially in complex environments. | Intel RealSense (Intel, Santa Clara, CA, USA), Microsoft Kinect 360 (Microsoft 360, Washington, DC, USA) |
Stereo Camera | Captures stereo images through two cameras and uses parallax to calculate depth information. | Provides high-precision depth perception for fine obstacle detection tasks and is more reliable than a single depth camera in terms of detection accuracy and range. | ZED Series (ZED Series, San Francisco, America), Bumblebee2 (Teledyne FLIR, Washington, DC, USA) |
Panoramic Camera | Capable of capturing images or videos with a 360-degree field of view. | In obstacle detection, it provides a comprehensive view of the environment, reduces blind spots, and improves the coverage and accuracy of obstacle detection. | Ricoh Theta (RICOH, Tōkyō, Japan), Insta360 Pro (insta360, Shenzhen, China) |
Classification | Common Algorithms | Common Application Areas |
---|---|---|
Algorithms based on graph search | Dijkstra, A *, D * | Global path planning |
Algorithm based on sampling | RRT | Global path planning |
Algorithms based on artificial potential fields | Artificial potential field method | Local path planning |
Algorithms based on curve fitting | Arcs and straight lines, polynomial curves, spline curves, Bessel curves, differential flatness | Local path planning |
Algorithms based on numerical optimization | Describing and solving planning problems using objective functions and constraints | Local path planning |
Intelligent algorithms based on bionics | Genetic algorithms, particle swarm optimization algorithms, ant colony algorithms | Global path planning, local path planning |
Step | GA | PSO | ACO |
---|---|---|---|
Initialization | Initialize population | Initialize particles | Initialize ants |
Fitness Eval. | Evaluate fitness | Evaluate fitness | Evaluate fitness |
Selection | Roulette wheel selection | N/A | Select next node based on probability |
Crossover | Single-point crossover | N/A | N/A |
Mutation | Swap mutation | N/A | N/A |
Update Ind. | Replace individual | Update velocity and position | Update pheromone |
Update Best | Find best individual | Update global best | Find global best path |
Iteration Loop | Repeat for max generations | Repeat for max iterations | Repeat for max iterations |
Return Result | Return best individual | Return global best | Return global best path |
Algorithm Category | Global Search Ability | Convergence Speed | Computational Complexity | Adaptability | Scalability |
---|---|---|---|---|---|
Genetic algorithm | ★★★★ | ★★ | ★★★★ | ★★★★ | ★★★ |
Particle swarm optimization | ★★★★ | ★★★★★ | ★★★ | ★★★ | ★★★★ |
Ant colony algorithm | ★★★★★ | ★★★ | ★★★★ | ★★★★ | ★★★★ |
The statements, opinions and data contained in all publications are solely those of the individual author(s) and contributor(s) and not of MDPI and/or the editor(s). MDPI and/or the editor(s) disclaim responsibility for any injury to people or property resulting from any ideas, methods, instructions or products referred to in the content. |
Share and Cite
Wei, W.; Xiao, M.; Duan, W.; Wang, H.; Zhu, Y.; Zhai, C.; Geng, G. Research Progress on Autonomous Operation Technology for Agricultural Equipment in Large Fields. Agriculture 2024 , 14 , 1473. https://doi.org/10.3390/agriculture14091473
Wei W, Xiao M, Duan W, Wang H, Zhu Y, Zhai C, Geng G. Research Progress on Autonomous Operation Technology for Agricultural Equipment in Large Fields. Agriculture . 2024; 14(9):1473. https://doi.org/10.3390/agriculture14091473
Wei, Wenbo, Maohua Xiao, Weiwei Duan, Hui Wang, Yejun Zhu, Cheng Zhai, and Guosheng Geng. 2024. "Research Progress on Autonomous Operation Technology for Agricultural Equipment in Large Fields" Agriculture 14, no. 9: 1473. https://doi.org/10.3390/agriculture14091473
Article Metrics
Article access statistics, further information, mdpi initiatives, follow mdpi.
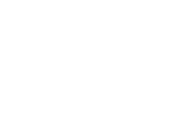
Subscribe to receive issue release notifications and newsletters from MDPI journals
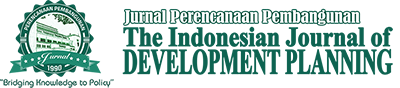
Enhancing Agricultural Practices through ICT: The Diffusion and Impact of the Among Tani Application in Batu City, Indonesia
- Syahirul Alim Communication Science Department, University of Brawijaya
- Angga Sukmara Christian Permadi Government Science Department, University of Brawijaya
- Verdy Firmantoro Communication Science Department, University of Brawijaya
This study examines the implementation and impact of the Among Tani application, a digital platform designed to support farmers in Batu City, Indonesia, as part of a broader smart city initiative. Utilizing a qualitative approach, the research investigates the diffusion process of the application, its role as a virtual communication medium, and its effects on organizational structures within farmer groups. The study reveals a staged adoption process influenced by factors such as farmer age and crop vulnerability. While the application has enhanced access to agricultural information and improved pest management, challenges including the digital divide and integration with traditional practices persist. The findings highlight the complex interplay between digital innovation and existing agricultural systems, emphasizing the need for context-sensitive approaches in implementing agricultural technologies. This research contributes to the understanding of digital agriculture's potential in supporting sustainable rural development and provides insights for future smart city initiatives in agricultural contexts.
Abduh, M. (2023). Indonesia Agricultural Transformation: How Far? Where Would It Go?. Jurnal Perencanaan Pembangunan: The Indonesian Journal of Development Planning, 7(1), 48-82.
Adesipo, A., Fadeyi, O., Kuca, K., Krejcar, O., Maresova, P., Selamat, A., & Adenola, M. (2020). Smart and climate-smart agricultural trends as core aspects of smart village functions. Sensors, 20(21), 5977.
Alim, S. (2024). Komunikasi Lingkungan: Konsep Kunci dan Studi Kasus Terkini di Asia dan Indonesia. Universitas Brawijaya Press.
Alim, S., Nisa, A. K., & Hair, A. (2019). New Public Service In Bandung Through The Concept Of Smart City. Journal of Politics and Policy, 95–108. https://doi.org/10.21776/ub.jppol.2019.002.01.5
Amankwah, A. (2023). Climate variability, agricultural technologies adoption, and productivity in rural Nigeria: a plot-level analysis. Agriculture & Food Security, 12(1), 7.
Andriani, I., & Yuniarsih, E. T. (2020). Inovasi Teknologi pada budidaya cabai di Sulawesi Barat. Jurnal Agercolere, 2(2), 37-44.
Bala, K., & Kaur, P. D. Information and communication technology in smart agriculture: A scientometric review. Concurrency and Computation: Practice and Experience, e8136.
Baltac, V. (2019). Smart cities—A view of societal aspects. Smart Cities, 2(4), 538-548.
Barzola Iza, C. L., Dentoni, D., & Omta, O. S. (2020). The influence of multi-stakeholder platforms on farmers' innovation and rural development in emerging economies: a systematic literature review. Journal of Agribusiness in Developing and Emerging Economies, 10(1), 13-39.
Bhat, P. P., Prasad, R., Anil, K., Jadhav, A., Manohar, K. N., Rajesh, C. M., & Reddy, S. L. (2024). The Role of Information and Communication Technology in Enhancing the Effectiveness of Agricultural Extension Programs Worldwide: A Review. Journal of Scientific Research and Reports, 30(7), 963-976.
Biviji, R., Vest, J., Dixon, B., Cullen, T., & Harle, C. (2018, June). Factors Related to User Ratings and User Downloads of Mobile Apps for Maternal and Child Health (MCH). In 2018 Annual Research Meeting. AcademyHealth.
Cawley, A., Heanue, K., Hilliard, R., O’Donoghue, C., & Sheehan, M. (2023). How knowledge transfer impact happens at the farm level: Insights from advisers and farmers in the Irish agricultural sector. Sustainability, 15(4), 3226.
Cernisevs, O., Surmach, A., & Buka, S. (2024). Smart agriculture for urban regions: Digital transformation strategies in the agro-industrial sector for enhanced compliance and economic growth.
Chandra, A., Games, D., & Sari, D. K. S. (2023). Organizational Structure, Governance, Leader Characteristics and Organizational Performance: Case Study of Sinergy Tani and Harapan Gumanti Farmers Group in Gumanti Valley, Solok Regency. Business and Investment Review, 1(5), 146-155.
Chentouf, F. Z., & Bouchkaren, S. (2023). Security and privacy in smart city: a secure e-voting system based on blockchain. International Journal of Electrical and Computer Engineering, 13(2), 1848.
Choudhary, R., Mantri, S. P., Barse, M. V. A., & Chitnis, S. (2024). Leveraging AI in Smart Agro-Informatics: A Review of Data Science Applications. International Research Journal on Advanced Engineering and Management (IRJAEM), 2(06), 1964-1975.
E-Elahi, Q. M., Habiba, S. U., & Talukder, M. S. A. Revolutionizing Urban Life: Smart Mobility and Smart Cities in Bangladesh.
El Bilali, H., Hassen, T. B., Bottalico, F., Berjan, S., & Capone, R. (2021). Acceptance and adoption of technologies in agriculture. AGROFOR, 6(1).
Fibrianingtyas, A., & Rayesa, N. F. (2023). Development Model for Sustainable Utilization of Among Tani Application (A Study in Batu Smart City). In IOP Conference Series: Earth and Environmental Science (Vol. 1131, No. 1, p. 012009). IOP Publishing.
Heriaty, A., & AR, A. T. (2021). Adopsi Penerapan Pengelolaan Tanaman Terpadu (PTT) Padi Sawah di Kelompok Tani Bolie Kelurahan Salokaraja Kecamatan Lalabata Kabupaten Soppeng. Jurnal Ilmiah Agrotani, 3(2), 36-41.
Jabbar, A., Liu, W., Zhang, J., Wang, Y., Wu, Q., Peng, J., & Liu, J. (2023). Enhancing adaptation to climate change by fostering collective action groups among smallholders in Punjab, Pakistan. Frontiers in Sustainable Food Systems, 7, 1235726.
Khalil, Z. F., Zamharira, C., Zalikha, S. N., Dian, W., & Wulandari, D. (2024). Governance Analysis in Driving Smart City Policy in Aceh. KnE Social Sciences, 121-129.
Khan, N., Siddiqui, B. N., Khan, N., Khan, F., Ullah, N., Ihtisham, M., ... & Muhammad, S. (2020). Analyzing mobile phone usage in agricultural modernization and rural development. International Journal of Agricultural Extension, 8(2), 139-147.
Khurshid, F., Ullah, A., & Manan, S. (2022). Adoption of agricultural innovations: Does the awareness raising through information communication technologies (ICTs) is functional. Sarhad Journal of Agriculture, 38(5), 335-340.
Kusumastuti, R. D., & Rouli, J. (2021, December). Smart city implementation and citizen engagement in Indonesia. In IOP Conference Series: Earth and Environmental Science (Vol. 940, No. 1, p. 012076). IOP Publishing.
Laiyinah, F. N., Ningsih, G. M., & Amir, N. O. (2021). Farmers’ Perception of Among Tani Application. SOCA: Jurnal Sosial Ekonomi Pertanian, 15(1), 19-29.
Laso Bayas, J. C., Gardeazabal, A., Karner, M., Folberth, C., Vargas, L., Skalský, R., ... & Govaerts, B. (2020). AgroTutor: a mobile phone application supporting sustainable agricultural intensification. Sustainability, 12(22), 9309.
Levis, L. R., Malle, I., Bureni, E., & Luruk, M. O. (2024). Difusi Inovasi Sistem Tanam Double Track Dalam Meningkatkan Produksi Jagung di Kabupaten Kupang. AGRIMOR, 9(1), 28-36.
Michels, M., Bonke, V., & Musshoff, O. (2019). Understanding the adoption of smartphone apps in dairy herd management. Journal of dairy science, 102(10), 9422-9434.
Molina-Maturano, J., Verhulst, N., Tur-Cardona, J., Güereña, D. T., Gardeazábal-Monsalve, A., Govaerts, B., & Speelman, S. (2021). Understanding smallholder farmers’ intention to adopt agricultural apps: The role of mastery approach and innovation hubs in Mexico. Agronomy, 11(2), 194.
Montes de Oca Munguia, O., Pannell, D. J., & Llewellyn, R. (2021). Understanding the adoption of innovations in agriculture: A review of selected conceptual models. Agronomy, 11(1), 139.
Muli, E. K. (2021). Adoption of Agricultural Innovations: The Effectiveness of Communication Channels Used in the Diffusion of Zero Grazing among Dairy Farmers in Bureti Sub County, Kericho County, Kenya. International Journal of Research and Innovation in Social Science, 5(07), 296-299.
Murti, H. T., Puspita, V., & Ratih, P. (2021). Pemanfaatan Teknologi Informasi dan Manajemen Perubahan Organisasi dalam Mendukung Bisnis Berkelanjutan Pasca Covid-19 pada UMKM di Kota Bengkulu. Jurnal Bisnis Dan Pemasaran Digital, 1(1), 33-41.
Ni'mah, S., Lestari, N. C., & Adawiyah, R. (2018). Pengembangan dan uji validasi perangkat pembelajaran SMA berbasis Kurikulum 2013 pada konsep sistem pencernaan. Jurnal Pendidikan Hayati, 4(1).
Nugraha, I. S. (2023). The Influence of Online Shopping Attributes on Customer Satisfaction and Loyalty from the Bukalapak Online Shopping Experience. Jurnal Multidisiplin Indonesia, 2(9), 2539-2552.
Nwafor, C. U., Ogundeji, A. A., & van Der Westhuizen, C. (2020). Adoption of ICT-based information sources and market participation among smallholder livestock farmers in South Africa. Agriculture, 10(2), 44.
Olumide, A. (2024). Agricultural innovation and food security in Sub-Saharan Africa in Nigeria. Journal of Developing Country Studies, 8(1), 62-73.
Osabohien, R. (2023). ICT adoption and youth employment in Nigeria's agricultural sector. African Journal of Economic and Management Studies, (ahead-of-print).
Pambudi, D. (2024). Harvesting opportunities: Transforming Indonesia's agribusiness through smart city planning in the digital age. Academic Society for Appropriate Technology, 10(1), 9-19.
Pang, Y., Marinello, F., Tang, P., Li, H., & Liang, Q. (2023). Bibliometric analysis of trends in smart irrigation for smart agriculture. Sustainability, 15(23), 16420.
Permadi, A. S. C., & Wardani, S. B. E. (2018). Jatibaru Raya Road Closure: Jakarta Governor's Incremental Policy in Managing the Tanah Abang Market Area. Journal of Public Administration and Local Governance, 2 (2), 16-29.
Quarshie, P. T., Antwi-Agyei, P., Suh, N. N., & Fraser, E. D. (2022). Tackling post-COVID-19 pandemic food crises through the adoption of improved maize seeds and technologies by smallholder farmers: The case of Ejura Sekyeredumase in Ghana. Frontiers in Sustainable Food Systems, 6, 804984.
Rachmawati, R., Rachmadani, P., Anifa, V. N., & Lutfiana, F. (2020). Various ICT-based applications and their uses to support smart city implementation in the Regency of Blora. In E3S Web of Conferences (Vol. 200, p. 07004). EDP Sciences.
Raeesi, A., Khajouei, R., & Ahmadian, L. (2022). Evaluating and rating HIV/AIDS mobile apps using the feature-based application rating method and mobile app rating scale. BMC Medical Informatics and Decision Making, 22(1), 281.
Rendra, R., Jamaluddin, J., & Sativa, F. (2019). Pelatihan PenggunaanMedia Aplikasi Penyuluhan Berbasis Android bagi PPL dan Ketua Kelompok Tani di Balai Penyuluh Pertanian (BPP) Kec. Hamparan Rawang Kota Sungai Penuh. Jurnal Karya Abdi Masyarakat, 3(2), 278-285.
Roni, R., Sundari, R. S., & Umbara, D. S. (2023). Any Correlation between Agricultural Extension with Participation Farmer Groups in Guava Farming?. Jurnal Agrinika: Jurnal Agroteknologi Dan Agribisnis, 7(2), 155-162.
Sahin, C. (2024). Do popular apps have issues regarding energy efficiency?. PeerJ Computer Science, 10, e1891.
Saker, A. (2023). Nurturing Sustainable Urban Space: Integrating Smart City Innovations and Earthship Design Principles for Eco-Friendly Futures. International Journal of Architectural Engineering and Urban Research, 6(2), 376-400.
Sangeetha, S., Yamunarani, K., & Dhanushkodi, V. (2023). Assessing the Effectiveness of different Mobile Apps in Terms of Knowledge Gain and Adoption Level. Asian Journal of Agricultural Extension, Economics & Sociology, 41(12), 279-283.
Simões, A. R. P., Bueno, N. P., Almeida, F. M. D. S., Nicholson, C. F., Reis, J. D. D., & Leonel, F. D. P. (2020). Public policies for enhancing diffusion of technology: a network analysis for a dairy farmer community in Minas Gerais, Brazil. Revista Brasileira de Zootecnia, 49, e20190207.
Somantri, A., & Rijayanti, R. (2022). Pelatihan Penggunaan Aplikasi Mobile Pertanian Untuk Pelaku Usaha Tani Di Desa Panyocokan. Jurnal Pengabdian Pada Masyarakat, 7(3), 730-738.
Sood, A., Sharma, R. K., & Bhardwaj, A. K. (2022). Artificial intelligence research in agriculture: A review. Online Information Review, 46(6), 1054-1075.
Sun, X., Lyu, J., & Ge, C. (2022). Knowledge and farmers’ adoption of green production technologies: an empirical study on IPM adoption intention in major Indica-Rice-Producing areas in the Anhui province of China. International Journal of Environmental Research and Public Health, 19(21), 14292.
Ulfa, A., Widiyanto, W., Nurhidayati, I., et al. (2021). Transformasi kelembagaan petani menjadi badan usaha milik petani (BUMP): Studi kasus di BUMP PT. Pengayom Tani Sejagad. Jurnal Sosio Agribisnis, 6(2).
Vanya, N. L., & Suherman, M. (2022, January). Sosialisasi Aplikasi Among Tani oleh Dinas Pertanian dan Ketahanan Pangan untuk Mewujudkan Kota Batu sebagai Smart City. In Bandung Conference Series: Public Relations (Vol. 2, No. 1, pp. 190-198).
Wole, B. D., Sasmito, C., & Purwaningsih, A. (2021). Analisis Kebijakan Smart City Pada Among Tani untuk Menumbuhkan Partisipasi Masyarakat dalam Rangka Mewujudkan Good Governance. Jurnal Inovasi Ilmu Sosial dan Politik (JISoP), 3(1), 58-67.
Yang, C., Zeng, H., & Zhang, Y. (2022). Are socialized services of agricultural green production conducive to the reduction in fertilizer input? Empirical evidence from rural China. International Journal of Environmental Research and Public Health, 19(22), 14856.
- Download PDF
How to Cite
- Endnote/Zotero/Mendeley (RIS)
Copyright (c) 2024 Jurnal Perencanaan Pembangunan: The Indonesian Journal of Development Planning

This work is licensed under a Creative Commons Attribution-NonCommercial-ShareAlike 4.0 International License .
This is an open-access article distributed under the terms of the Creative Commons Attribution-NonCommercial-ShareAlike 4.0 International License. Copyright © Kementerian PPN/Bappenas RI
Most read articles by the same author(s)
- Abdul Malik Sadat Idris, Angga Sukmara Christian Permadi, Aozora Insan Kamil, Bintang Rahmat Wananda, Aditya Riski Taufani, Citarum Harum Project: A Restoration Model of River Basin , Jurnal Perencanaan Pembangunan: The Indonesian Journal of Development Planning: Vol. 3 No. 3 (2019): December 2019
make_submission
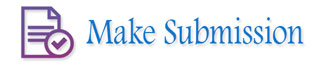
sidebar_menu
Focus & scopes
Publication ethics
Editorial Business Process
Publication Fee
Open Access Statement
License Term
Author Guideline
Manuscript template
Peer Review Process
Plagiarism Check
Reviewers
Reviewer Guideline
MOU Association Planner
Statistics
custom-link
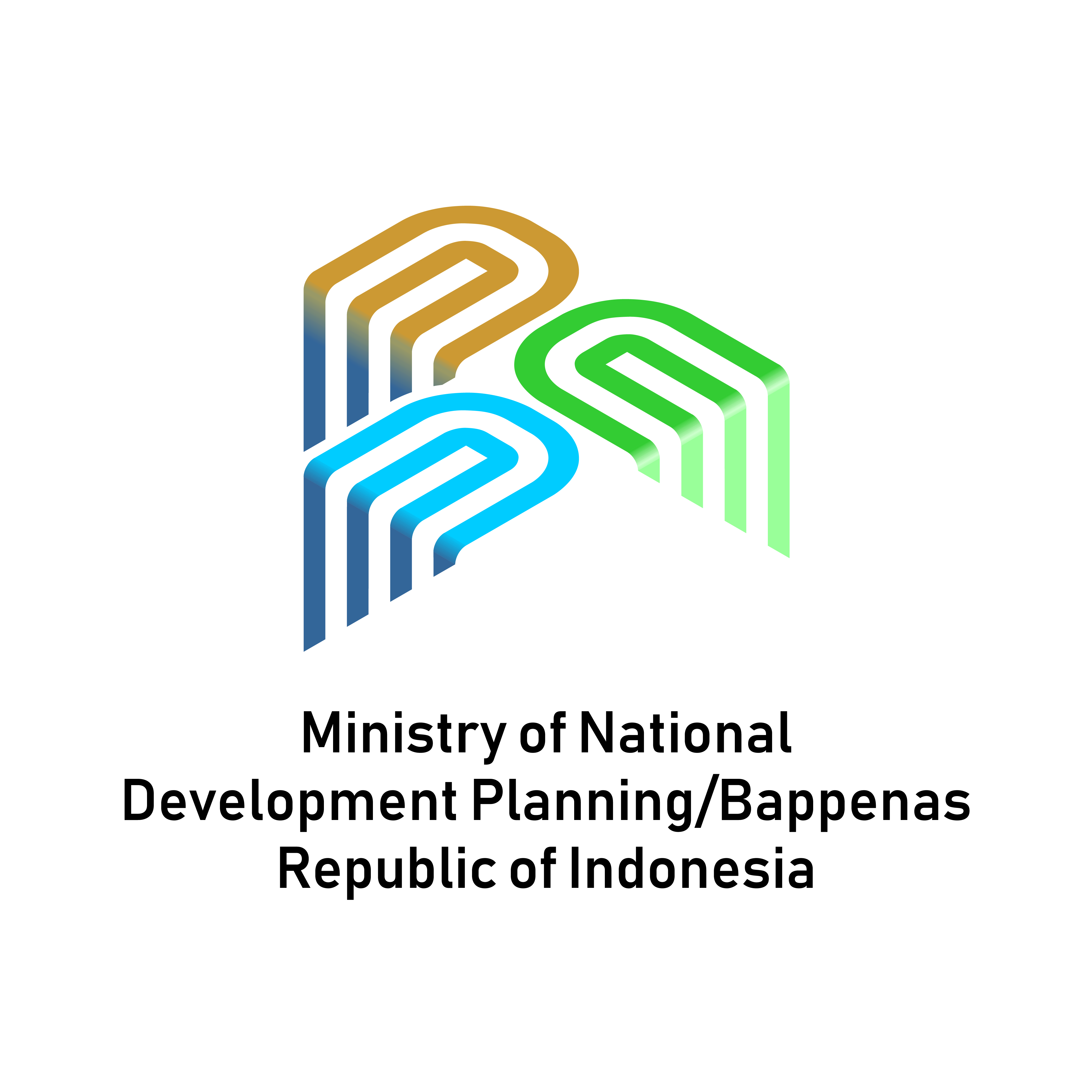
flag-counter
ADVERTORIAL
sertivicate
Accreditation, contact info.
- Agricultural Science
- Urban Agriculture
Urban Agriculture: Opportunities and Challenges for Sustainable Development
- January 2020
- In book: Zero Hunger (pp.1-14)

- The University of Sheffield
- This person is not on ResearchGate, or hasn't claimed this research yet.

Discover the world's research
- 25+ million members
- 160+ million publication pages
- 2.3+ billion citations

- John Choptiany
- Samuel Ledermann

- Mohamad Zaid

- Karim Daoud

- Mariam Shehata

- Giuseppe Calore

- Meshal J. Abdullah

- Margarita Fernandez
- Justine Williams

- Fernando Funes Aguilar

- Nicholas Clinton

- INT J HEALTH GEOGR

- BIOL CONSERV

- LANDSCAPE URBAN PLAN

- Recruit researchers
- Join for free
- Login Email Tip: Most researchers use their institutional email address as their ResearchGate login Password Forgot password? Keep me logged in Log in or Continue with Google Welcome back! Please log in. Email · Hint Tip: Most researchers use their institutional email address as their ResearchGate login Password Forgot password? Keep me logged in Log in or Continue with Google No account? Sign up
- State Boards
PSEB 8th Agriculture Sample Paper 2024-25 (PDF): Punjab Board Class 8th Model Paper Free PDF Download!
Punjab board pseb class 8th agriculture sample paper 2024-25: the punjab board has made available the sample papers for classes 8 for the current academic year 2024-25. students and teachers can download the free pdf of sample papers from here. .
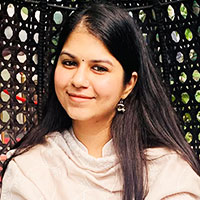
PSEB Punjab Board Class 8th Agriculture Sample Paper 2024-25
We are providing the sample paper here. You can take a look at the sample paper for class 8 Agriculture.
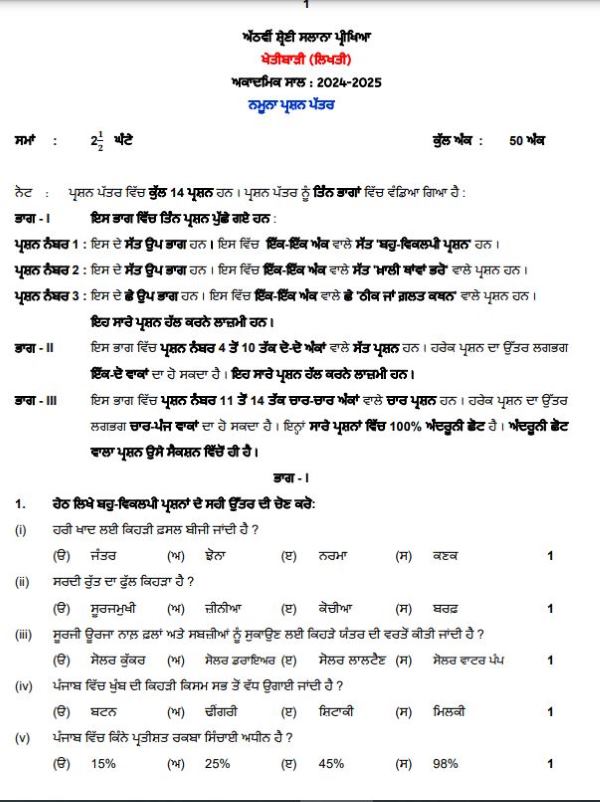
Now, that the students have the sample paper with them, they can start preparing for the exam to score well. We are also providing the link below to download the sample paper PDF for free.
CHECK: Punjab Board PSEB Class 8 Agrucluture Sample Paper 2024-25 Free PDF Download
- PSEB Class 8 Science Model Test Paper 2024-25: Download FREE PDF!
- Punjab Board PSEB Class 8 Model Papers 2024-25: Download Model Test Papers Of All Subjects In PDF!
Get here latest School , CBSE and Govt Jobs notification and articles in English and Hindi for Sarkari Naukari , Sarkari Result and Exam Preparation . Download the Jagran Josh Sarkari Naukri App .
- India Post GDS Merit List 2024
- NDA Question Paper 2024
- RBI Grade B Admit Card 2024
- UP Police Constable Admit Card 2024
- SSC CGL Admit Card 2024
- UP Police Constable Question Paper 2024 PDF
- CDS Question Paper 2024
- RRB NTPC Recruitment 2024
- Teachers Day Speech
- Teachers Day Gift
Latest Education News
Paris Paralympics 2024 India Medals list: किन भारतीयों ने जीते मेडल,पढ़ें सबके नाम
उत्तर प्रदेश के 8 रेलवे स्टेशनों को मिले नए नाम, यहां देखें नई लिस्ट
Jasdeep Singh Gill Story: कौन हैं जसदीप सिंह गिल? केमिकल इंजीनियर से धार्मिक गुरु बनने तक की कहानी
बिना पासपोर्ट और वीजा के विदेश की सैर, बस यह डॉक्यूमेंट रखें साथ
Brain Teaser: This High IQ Logic Puzzle Will Separate the Smart From the Smarter
Teacher’s Day 2024: डॉ. सर्वपल्ली राधाकृष्णन, जो राष्ट्रपति के रूप में दान कर देते थे आधे से अधिक वेतन
Picture Puzzle IQ Test: Find the mistake in the picture in 5 seconds!
Maharashtra Police SRPF Result 2022-23 OUT at mahapolice.gov.in, Download Here
Kerala SET Result 2024 OUT at lbsedp.lbscentre.in: Download KSET Marks Link
UP Scholarship 2024: Direct Link to Apply Online for Pre-Matric and Post-Matric Scheme, Check Eligibility, Other Details
Dr MGR Medical University Result 2024 OUT at tnmgrmu.ac.in; Direct Link to Download UG and PG Marksheet
Teachers Day 2024: How Dr. Sarvepalli Radhakrishnan's Birthday Became Teachers' Day in India? Details Here
MGSU Result 2024 OUT at mgsubikaner.ac.in, Direct Link to Download UG and PG Marksheet PDF
SSC CGL Admit Card 2024 Out at ssc.gov.in: Direct Link to Download Region Wise Tier 1 Hall Ticket PDF Here
Delhi Home Guard Admit Card 2024 OUT at dghgenrollment.in: Download Link Here
SSC CGL Reasoning Questions With Solution, Download PDF Here
Synonyms and Antonyms: 100+ Synonyms and Antonyms List with Examples and Meaning for SSC; Download PDF
SSC CGL Previous Year Question Paper PDF Download, Tier 1 & 2 PDFs with Solutions
Visual Skill Test: Find the odd woman face in the picture in 3 seconds!
KTET Result 2024 OUT at ktet.kerala.gov.in: Download Kerala TET Marks

An official website of the United States government
Official websites use .gov A .gov website belongs to an official government organization in the United States.
Secure .gov websites use HTTPS A lock ( Lock A locked padlock ) or https:// means you’ve safely connected to the .gov website. Share sensitive information only on official, secure websites.
USDA Releases Updated Guideline to Strengthen Substantiation of Animal-Raising and Environment-Related Claims on Meat and Poultry Labels
WASHINGTON, August 28, 2024 – The U.S. Department of Agriculture (USDA) announced today the availability of an updated guideline that makes recommendations to strengthen the documentation that supports animal-raising or environment-related claims on meat or poultry product labeling . Today’s action builds on the significant work USDA has already undertaken to protect consumers from false and misleading labels and to implement President Biden’s Executive Order on Promoting Competition in the American economy.
“USDA continues to deliver on its commitment to fairness and choice for both farmers and consumers, and that means supporting transparency and high-quality standards,” said Agriculture Secretary Tom Vilsack. “These updates will help to level the playing field for businesses who are truthfully using these claims and ensure people can trust the labels when they purchase meat and poultry products.”
Animal-raising claims, such as “Raised Without Antibiotics,” “Grass-Fed” and “Free-Range,” and environment-related claims, such as “Raised using Regenerative Agriculture Practices” and “Climate-Friendly,” are voluntary marketing claims that highlight certain aspects of how the source animals for meat and poultry products are raised or how the producer maintains or improves the land or otherwise implements environmentally sustainable practices. The documentation submitted by companies to support these claims is reviewed by USDA’s Food Safety and Inspection Service (FSIS) and the claims can only be included on the labels of meat and poultry products sold to consumers after they are approved by the agency.
FSIS last updated its guideline on these claims in 2019.
In the updated guideline, FSIS strongly encourages the use of third-party certification to substantiate animal-raising or environment-related claims. Third-party certification of animal-raising or environment-related claims helps ensure that such claims are truthful and not misleading by having an independent organization verify that their standards are being met on the farm for the raising of animals and for environmental stewardship. The revised guideline also emphasizes more robust documentation for environment-related and animal-raising claims.
Additionally, the updated guideline recommends that establishments using “negative” antibiotic claims (e.g., “Raised Without Antibiotics” or “No Antibiotics Ever”) implement routine sampling and testing programs to detect antibiotic use in animals prior to slaughter or obtain third-party certification that includes testing. The revisions were informed by sampling data, petitions, public comments to those petitions and feedback received from a wide range of stakeholders.
In light of concerns about negative antibiotic claims, FSIS announced last year that the agency would be conducting a study in partnership with USDA’s Agricultural Research Service (ARS) to assess the veracity of these claims. FSIS collected liver and kidney samples from 196 eligible cattle at 84 slaughter establishments in 34 states, and ARS analyzed the samples using a method that targeted more than 180 veterinary drugs including various major classes of antibiotics. The study found antibiotic residues in approximately 20% of samples tested from the “Raised Without Antibiotics” market.
The action FSIS is taking through the publishing of this guidance today addresses these concerning findings and makes clear that FSIS will take enforcement action against any establishments found to be making false or misleading negative antibiotic claims. Additionally, FSIS has informed the establishments with positive results from the ARS-FSIS study and advised them to conduct a root cause analysis and implement corrective actions. FSIS has also advised these establishments to determine how antibiotics were introduced into the animal and to take appropriate measures to ensure that future products are not misbranded. Further information on the study can be found in the Federal Register Notice announcing the availability of the updated guideline.
FSIS and ARS will be publishing a peer-reviewed paper with complete results from the study in the near future. The study findings underscore the need for more rigorous substantiation of such claims. These sampling results may lead to additional testing by the agency. FSIS has the authority to collect samples any time it believes a product is mislabeled with any claim covered by the guidance. Moreover, FSIS may consider future additional actions, including random sampling and rulemaking, to further strengthen the substantiation of animal-raising and environment-related claims.
USDA is committed to ongoing stakeholder engagement. For more information, please view the Federal Register notice . The updated guideline will be open for public comment for 60 days after publishing in the Federal Register.
USDA touches the lives of all Americans each day in so many positive ways. In the Biden-Harris Administration, USDA is transforming America’s food system with a greater focus on more resilient local and regional food production, fairer markets for all producers, ensuring access to safe, healthy, and nutritious food in all communities, building new markets and streams of income for farmers and producers using climate smart food and forestry practices, making historic investments in infrastructure and clean energy capabilities in rural America, and committing to equity across the Department by removing systemic barriers and building a workforce more representative of America. To learn more, visit www.usda.gov .
USDA is an equal opportunity provider, employer, and lender.
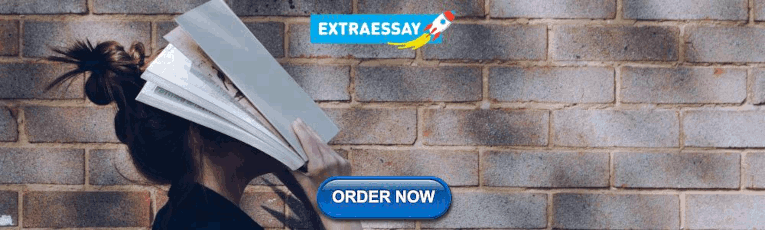
COMMENTS
The Oxford English Dictiona ry (1971) defines agriculture. very broadly as "The science and art of cultivating the soil, including the allied pursuits of. gathering in the crops and rearing live ...
Table 2.1 summarizes the main drivers and mechanisms of climate impact on cropping systems, which were reviewed by Bongaarts (1994), Rosenzweig et al. (2001), Boote et al. (2010), Kimball (2010), and Porter et al. (2014). Notably, direct climate impacts include both damage and benefits as well as opportunities for farm-level adaptations.
Metrics. A technological revolution in farming led by advances in robotics and sensing technologies looks set to disrupt modern practice. Over the centuries, as farmers have adopted more ...
This review article aims to provide an overview of the recent innovations, challenges, and future prospects of precision agriculture and smart farming. It presents an analysis of the current state of precision agriculture, including the most recent innovations in technology, such as drones, sensors, and machine learning.
For this purpose, we. conducted a structured literature review in combination with a cluster analysis in order to. (1) identify the overall ideas and aspects associated with sustainable ...
An interconnected combination of steps could help ensure that the most vulnerable countries and people get the nutrition they need. 1 The modest ambition of this paper is to review the economic literature on agriculture, focusing on the issues that are critical for agricultural productivity and poverty reduction.
Abstract: Agriculture associated with one third o f global land use and it has a si gnificant importance in. land use change globally. i.e., food security and nutrition, water and soil quality ...
Sustainable agriculture. Nature Sustainability 1, 531 (2018) Cite this article. Achieving food security is possible, if we better understand the complexity of the agricultural system and re-design ...
Raising agricultural productivity has been essential for global food security and conserving land. Now, research quantifies how climate change has slowed agricultural productivity growth around ...
View PDF Structural dynamics and sustainability in the agricultural sector: the case of the European Union. This paper seeks to draw a research agenda for unveiling the underlying bottlenecks and possibilities for improving the agricultural total factor productivity in the European Union (EU).
A more sustainable agriculture, therefore, is any system of food or fibre production that systematically pursues the goals in Box 1. Box 1. A more sustainable agriculture pursues: a thorough incorporation of natural processes such as nutrient cycling, nitrogen fixation, and pest-predator relationships;
While the use of the adjective regenerative is expanding among activists, civil society groups and corporations as they call for renewal, transformation and revitalization of the global food system (Duncan et al., 2021), in this paper we explore the calls for Regenerative Agriculture from an agronomic perspective.By this we mean a perspective steeped in the use of plant, soil, ecological and ...
By systematically reviewing the literature across India, this study intends to provide a generalized and standard overview of farmers' perception and adaptation measures, identify the key research gaps in the current empirical literature, and bridge the gaps between research practitioners and farmers for robust policymaking. 2.
agriculture remains fundamental to economic growth, poverty alleviation, and environmental sustainability. The World Bank's Agriculture and Rural Development publication series presents recent analyses of issues that affect agriculture's role as a source of economic development, rural livelihoods, and environmental services. The
Journal of Agricultural Education 249 Volume 61, Issue 2, 2020 Elevating the Impacts of Research in Agricultural Education James R. Lindner,1 Amy Harder2, and T. Grady Roberts3 Abstract Dissemination of research is a critical part of the research process. Researchers in agricultural education have long embraced this process.
Research by (Saleh et al., Citation 2016) points out that the training of extension workers in agriculture is an essential step in the general farming production course. Training of trainers is the prerequisite for effective implementation of technical solutions in the field and an important step for their dissemination (Braga et al., Citation ...
Along with the subsystem development, numerous research articles have been published highlighting technology developments in the optimum growing conditions for indoor vertical farming. The plant grown in vertical farming is primarily based on artificial lights such as light-emitting diodes (LEDs) sources instead of natural sunlight (Figure 2).
The following section discusses the impact of climate change including greenhouse effect, effect on water resources, agriculture, food, etc. 12.5.1 Greenhouse Effect. J.B. Fourler developed the "greenhouse effects" theory more than a century ago. Tyndall's research on the heat absorption by gases backed it up.
Most business-as-usual scenarios for farming under changing climate regimes project that the agriculture sector will be significantly impacted from increased temperatures and shifting precipitation patterns. Perhaps ironically, agricultural production contributes substantially to the problem with yearly greenhouse gas (GHG) emissions of about 11% of total anthropogenic GHG emissions, not ...
This article explores the key trends shaping the future of Indian agriculture, including sustainable practices, technological innovations, vertical farming, genetic engineering, data-driven ...
Agriculture is the cultivation of plants, animals, and some other organisms, such as fungi, for the production of food, fibre, fuel, and medicines used by society. Dietary shifts are among the ...
View PDF View article View in Scopus Google Scholar [3] Sekar M., Praveen Kumar T.R., ... Research on the Impact of Digital Agriculture Development on Agricultural Green Total Factor Productivity. Land, 12 (2023), p. 195, 10.3390/land12010195. View PDF View article View in Scopus Google Scholar
Agriculture is a labor-intensive industry. However, with the demographic shift toward an aging population, agriculture is increasingly confronted with a labor shortage. The technology for autonomous operation of agricultural equipment in large fields can improve productivity and reduce labor intensity, which can help alleviate the impact of population aging on agriculture. Nevertheless ...
Introduction. It is widely accepted that agriculture in sub-Saharan Africa (SSA) has been under-performing since independence (Food and Agriculture Organization [FAO], Citation 2009).SSA had modest growth in agricultural production per capita from the early 1960s to the 1970s, and occasional short periods of growth in the mid-1970s and 1980s (Figure 1).
This study examines the implementation and impact of the Among Tani application, a digital platform designed to support farmers in Batu City, Indonesia, as part of a broader smart city initiative. Utilizing a qualitative approach, the research investigates the diffusion process of the application, its role as a virtual communication medium, and its effects on organizational structures within ...
Urban agriculture (UA) can be used as a mechanis m to contribute to food security in each of the four. dimensions. The FAO (2007) has defined urban agriculture as "the growing of plants and the ...
Punjab Board Class 8 Agriculture Model Test Paper 2023-2024: Get the direct link to Download a FREE PDF for the PSEB 8th Agriculture sample question paper here.
Considering that more than half of the world's population relies on N fertilizer for food production, research on N fertilizer application in agricultural systems has garnered considerable attention. The appropriate application of N fertilizer can enhance crop yields by 30-50%, but inappropriate N scheduling leads to poor synchronization ...
WASHINGTON, August 28, 2024 - The U.S. Department of Agriculture (USDA) announced today the availability of an updated guideline that makes recommendations to strengthen the documentation that supports animal-raising or environment-related claims on meat or poultry product labeling.Today's action builds on the significant work USDA has already undertaken to protect consumers from false and ...
Background Podcasts have become an increasingly popular method of communicating information in medicine, including in radiology. However, the effect of podcasts on the reach of journal articles remains unclear. Purpose To evaluate the influence of Radiology podcasts on the performance metrics, including downloads, citations, and Altmetric Attention Score (AAS), of Radiology articles. Materials ...