- Sampling Techniques
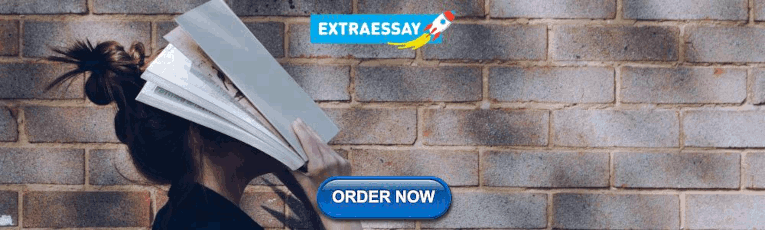
Understanding Sampling Techniques in Experimental Research: A Comprehensive Guide
- August 6, 2024

Experimental research is a crucial aspect of scientific investigation, allowing researchers to test hypotheses and draw conclusions about various phenomena. However, the success of experimental research depends heavily on the quality of the sampling technique used. Sampling techniques refer to the methods used to select participants or observations for a study. In this comprehensive guide, we will explore the various sampling techniques used in experimental research, their advantages and disadvantages, and how to choose the right sampling technique for your study. By understanding the principles of sampling techniques, you can ensure that your experimental research is valid, reliable, and provides meaningful insights. So, let’s dive in and explore the world of sampling techniques in experimental research!
Importance of Sampling Techniques in Experimental Research
Definition of sampling techniques.
Sampling techniques refer to the methods used to select a subset of individuals or units from a larger population for the purpose of research. These techniques are crucial in experimental research as they determine the representativeness and generalizability of the findings to the larger population.
Sampling techniques can be broadly classified into two categories: probability sampling and non-probability sampling.
Probability sampling involves selecting samples based on known probabilities or random selection from the population. Examples of probability sampling techniques include simple random sampling, stratified random sampling, and cluster sampling.
Non-probability sampling involves selecting samples based on non-random criteria, such as convenience or purposeful sampling. Examples of non-probability sampling techniques include snowball sampling, quota sampling, and purposive sampling.
The choice of sampling technique depends on the research question, the size and characteristics of the population, and the resources available for the study. The sampling technique should be representative of the population to ensure that the findings can be generalized to the larger population.
The Significance of Proper Sampling in Experimental Research
Proper sampling is a critical aspect of experimental research, as it determines the representativeness and generalizability of the findings. The significance of proper sampling lies in its ability to ensure that the sample accurately reflects the population of interest, thereby minimizing bias and maximizing the validity of the results. In addition, proper sampling techniques help researchers to draw meaningful conclusions and generalize their findings to the larger population.
Types of Sampling Techniques
Random sampling.
Random sampling is a technique used in experimental research to select participants or samples from a population in a way that ensures a representative and unbiased sample. In this method, every member of the population has an equal chance of being selected, and the selection is made using a randomization process. This process can be done using various methods such as random number generators, tables of random numbers, or algorithms.
Random sampling is widely used in experimental research as it helps to eliminate selection bias, which occurs when the sample is not representative of the population. This technique is also efficient as it allows for a larger sample size to be drawn from a larger population. Additionally, it ensures that the sample is a fair representation of the population, and the results obtained can be generalized to the population.
There are several types of random sampling techniques, including simple random sampling, stratified random sampling, and cluster sampling. Simple random sampling involves selecting participants or samples randomly from the population. Stratified random sampling involves dividing the population into groups or strata and then selecting participants or samples randomly from each stratum. Cluster sampling involves dividing the population into clusters and then selecting clusters randomly for inclusion in the sample.
In conclusion, random sampling is a widely used technique in experimental research as it ensures a representative and unbiased sample. It eliminates selection bias and allows for a larger sample size to be drawn from a larger population. Additionally, there are several types of random sampling techniques that can be used depending on the research design and sample size.
Stratified Sampling
Stratified sampling is a type of sampling technique used in experimental research to ensure that the sample is representative of the population . This technique involves dividing the population into smaller groups or strata based on specific characteristics and then selecting a sample from each stratum.
The process of stratified sampling involves the following steps:
- Identify the population: The first step is to identify the population that you want to study. This population can be defined by demographic characteristics such as age, gender, ethnicity, or geographic location.
- Define the strata: Once the population has been identified, the next step is to define the strata or subgroups within the population. These strata are defined based on specific characteristics that are relevant to the research question.
- Select the sample: After defining the strata, a sample is selected from each stratum. The sample size for each stratum is determined based on the research question and the desired level of precision.
- Ensure adequate representation: Stratified sampling ensures that each stratum is adequately represented in the sample. This means that the sample should reflect the characteristics of the population in each stratum.
Stratified sampling is particularly useful when the population is heterogeneous and the research question requires a representative sample. It is also useful when the researcher wants to ensure that the sample is proportionate to the population.
However, stratified sampling can be time-consuming and resource-intensive, particularly when the population is large and complex. Additionally, the researcher must have a clear understanding of the population and the relevant characteristics to define the strata effectively.
Overall, stratified sampling is a useful sampling technique in experimental research that ensures a representative sample and helps to minimize bias.
Cluster Sampling
Cluster sampling is a technique that involves dividing a population into smaller groups or clusters and selecting a subset of these clusters for study. This method is particularly useful when it is difficult or expensive to access the entire population. The selection of clusters is random, and each cluster is treated as a single unit.
Here are some key points to consider when using cluster sampling:
- Advantages: Cluster sampling can be more efficient and cost-effective than other sampling methods, especially when studying large populations. It also allows for the study of groups that may be difficult to access individually.
- Disadvantages: The main disadvantage of cluster sampling is that it may not be as representative of the entire population as other sampling methods. Additionally, there may be variability within clusters that can affect the results of the study.
- Considerations: When using cluster sampling, it is important to ensure that the clusters are selected randomly and that the sample size is sufficient to produce meaningful results. Additionally, it is important to consider the size and homogeneity of the clusters and to account for any variability within them.
Overall, cluster sampling can be a useful sampling technique in experimental research, but it is important to carefully consider its advantages and disadvantages and to select the appropriate sampling method based on the research question and population being studied.
Convenience Sampling
Convenience sampling is a non-probability sampling technique that involves selecting participants based on their availability and accessibility. This method is often used when the population is difficult to identify or when time and resources are limited. The main advantage of convenience sampling is its speed and ease of implementation. However, the main disadvantage is that the sample may not be representative of the population, which can lead to biased results.
To ensure that the sample is as representative as possible, researchers should take steps to ensure that the sample is diverse and includes individuals from different backgrounds and demographics. This can be achieved by recruiting participants from different locations, using multiple sources to recruit participants, and actively seeking out underrepresented groups. Additionally, researchers should aim to collect a sufficient sample size to ensure that the results are reliable and accurate.
It is important to note that convenience sampling should only be used when other sampling techniques are not feasible or practical. Researchers should carefully consider the advantages and disadvantages of this method before deciding to use it. In general, convenience sampling is best suited for exploratory research or pilot studies, where the aim is to gather preliminary data to inform future research.
Snowball Sampling
Snowball sampling is a non-probability sampling technique that is often used in studies where the population is difficult to identify or recruit. It is particularly useful in studying hard-to-reach populations or those that are sensitive to researchers. The method involves recruiting a small number of initial participants and then asking them to recruit additional participants who fit the study criteria. This process continues until a sufficient sample size is reached.
Snowball sampling has several advantages over other sampling techniques. It is often more efficient and cost-effective than other methods, as it relies on the existing social networks of the initial participants to recruit additional participants. It also allows for a more diverse sample, as participants are not limited by pre-defined criteria and can recruit others who may not have otherwise been included in the study.
However, snowball sampling also has some limitations. It is not a random sampling technique, so there is a risk of bias in the sample. Additionally, the process of recruiting participants can be time-consuming and may require a significant amount of effort from the researcher. It is important to carefully consider the potential benefits and limitations of snowball sampling before deciding to use it in a study.
Sampling Techniques in Clinical Research
Clinical research involves the study of drugs, devices, and biologics in human subjects to determine their safety and efficacy. In clinical research, sampling techniques play a crucial role in selecting the right participants for the study. Here are some commonly used sampling techniques in clinical research:
Randomization
Randomization is a sampling technique used to assign participants to different treatment groups. Participants are randomly selected from the eligible population and assigned to different treatment groups using a predetermined algorithm. Randomization helps to reduce bias and ensure that each treatment group is comparable.
Stratified Randomization
Stratified randomization is a variation of randomization in which participants are divided into strata based on certain characteristics, such as age, gender, or disease severity. Participants within each stratum are then randomly assigned to treatment groups. Stratified randomization helps to ensure that each treatment group has a similar distribution of the stratifying factors.
Convenience sampling is a non-probability sampling technique in which participants are selected based on their availability and accessibility. Participants are typically recruited from hospitals, clinics, or other healthcare facilities. Convenience sampling is often used when it is difficult or expensive to recruit a representative sample from the general population.
Purposive Sampling
Purposive sampling is a non-probability sampling technique in which participants are selected based on specific criteria or characteristics. Participants are typically recruited based on their knowledge, experience, or expertise related to the research topic. Purposive sampling is often used in qualitative research to obtain in-depth insights from experts or stakeholders.
Cluster sampling is a sampling technique in which participants are selected from clusters or groups rather than from the entire population. Participants within each cluster are then randomly selected for the study. Cluster sampling is often used in clinical research when it is difficult or impractical to recruit participants from the entire population.
Overall, sampling techniques in clinical research play a critical role in ensuring the validity and reliability of study results. Researchers must carefully consider the appropriate sampling technique based on the research question, study design, and population characteristics.
Factors to Consider When Selecting Sampling Techniques
Sample size.
The sample size is a crucial factor to consider when selecting a sampling technique in experimental research. It refers to the number of participants or observations that will be included in the study. The sample size determines the statistical power of the study, which is the probability of detecting a true effect if it exists. A larger sample size increases the statistical power of the study, making it more likely to detect a true effect, even if it is small.
Importance of Sample Size
The sample size is important for several reasons. First, it affects the precision and accuracy of the results. A larger sample size increases the precision of the results, making them more reliable. Second, it affects the statistical power of the study, which determines the likelihood of detecting a true effect. A smaller sample size decreases the statistical power of the study, making it less likely to detect a true effect, even if it is large. Third, it affects the generalizability of the results, as a larger sample size increases the likelihood that the results will be representative of the population.
Determining Sample Size
The sample size should be determined based on several factors, including the research question, the level of precision required, the expected effect size, and the variability of the data. A power analysis can be used to determine the appropriate sample size for the study. A power analysis considers the research question, the expected effect size, the variability of the data, and the level of precision required to determine the appropriate sample size.
Implications of Sample Size
The sample size has several implications for the study design and data analysis. A larger sample size may require more resources, such as time and money, to collect and analyze the data. A smaller sample size may require a larger effect size to be detected, making it more difficult to detect a true effect. Additionally, a smaller sample size may require a larger variability in the data to detect a true effect, making it more difficult to detect a true effect.
Overall, the sample size is a critical factor to consider when selecting a sampling technique in experimental research. It affects the precision, accuracy, and generalizability of the results, and should be determined based on several factors, including the research question, the level of precision required, the expected effect size, and the variability of the data.
Diversity and Inclusion
When selecting a sampling technique, it is important to consider diversity and inclusion. Diversity refers to the representation of different groups within the sample, while inclusion refers to the extent to which the sample reflects the population of interest. Both diversity and inclusion are important to ensure that the sample accurately represents the population being studied and to avoid bias in the results.
There are several strategies that can be used to increase diversity and inclusion in the sample. One approach is to use random sampling techniques, such as simple random sampling or stratified random sampling, to ensure that the sample is representative of the population . Another approach is to oversample certain groups, such as underrepresented populations, to ensure that they are adequately represented in the sample.
It is also important to consider the potential for self-selection bias when using certain sampling techniques, such as convenience sampling or snowball sampling. Self-selection bias occurs when individuals who are more likely to have certain characteristics or opinions are more likely to participate in the study, leading to biased results. To mitigate this bias, researchers can use methods such as random assignment or controlled recruitment to ensure that the sample is representative of the population .
In addition to diversity and inclusion, researchers should also consider other factors when selecting a sampling technique, such as cost, time, and the nature of the research question. By carefully selecting the appropriate sampling technique, researchers can ensure that their study produces valid and reliable results.
Cost and Time Constraints
When it comes to selecting sampling techniques, it is important to consider the costs and time constraints associated with each method. In some cases, certain sampling techniques may be more expensive or time-consuming than others, which can have a significant impact on the overall feasibility of a research project.
One factor to consider is the cost of data collection. For example, some sampling techniques may require specialized equipment or software that can be expensive to obtain or maintain. Additionally, some methods may require a larger sample size in order to be statistically valid, which can increase the cost of the study.
Another factor to consider is the time required to conduct the study. Some sampling techniques may be faster to implement than others, which can be important if a researcher is working with a tight deadline. However, it is important to note that some methods may require more time for data analysis and interpretation, which can impact the overall timeline of the study.
It is important to carefully weigh the costs and time constraints associated with each sampling technique in order to select the most appropriate method for a given research project. By considering these factors, researchers can ensure that they are able to conduct high-quality studies that are both feasible and practical.
Ethical Considerations
When selecting sampling techniques, it is important to consider ethical considerations. These considerations are essential to ensure that the research process is conducted in a manner that is respectful of human rights and dignity.
- Informed Consent: Informed consent is a crucial ethical consideration in experimental research. It involves obtaining permission from participants before they take part in the study. Participants should be provided with all relevant information about the study, including its purpose, procedures, risks, benefits, and confidentiality measures.
- Voluntary Participation: Participation in experimental research should be voluntary, and participants should be free to withdraw from the study at any time without any negative consequences.
- Deception: Deception is a common ethical issue in experimental research. It occurs when participants are misled or deceived about the nature or purpose of the study. Researchers should avoid deception and if it is necessary, they should take steps to minimize harm and provide appropriate debriefing after the study.
- Risk of Harm: Experimental research may involve some risks of harm to participants, such as physical or psychological harm. Researchers should take all necessary precautions to minimize the risk of harm and provide appropriate care if harm occurs.
- Confidentiality: Confidentiality is an essential ethical consideration in experimental research. Researchers should ensure that participants’ personal information is kept confidential and only used for the intended purpose of the study.
- Fairness: Experimental research should be conducted in a fair manner. Participants should be selected randomly or based on specific criteria that are relevant to the study. Researchers should avoid any form of discrimination or bias in the selection process.
In summary, ethical considerations are crucial in experimental research. Researchers should obtain informed consent, ensure voluntary participation, avoid deception, minimize the risk of harm, maintain confidentiality, and conduct the study in a fair manner.
Sampling Techniques in Practice
Case study: random sampling in a psychology experiment.
Random sampling is a widely used technique in experimental research, particularly in psychology. It involves selecting participants from a population in a way that ensures that each participant has an equal chance of being selected. In this section, we will examine a case study that demonstrates the use of random sampling in a psychology experiment.
Participants
In this case study, the researcher selected 100 participants from a pool of undergraduate students at a large university. The researcher used a random number generator to select the participants, ensuring that each participant had an equal chance of being selected.
The researcher designed an experiment to investigate the effects of stress on memory performance. The experiment consisted of two phases: a stress induction phase and a memory recall phase.
During the stress induction phase, the participants were asked to give a brief impromptu speech in front of a video camera. This was designed to induce stress in the participants. The participants were then randomly assigned to one of two groups: a stress group or a control group. The stress group was asked to solve a difficult math problem, while the control group was asked to solve an easy math problem.
During the memory recall phase, the participants were asked to recall as many words as they could from a list of 20 words. The researcher measured the number of words recalled by each participant and compared the results between the stress group and the control group.
Data Analysis
The researcher analyzed the data using statistical tests to determine whether there was a significant difference in memory recall between the stress group and the control group. The results showed that the stress group recalled significantly fewer words than the control group.
This case study demonstrates the use of random sampling in a psychology experiment. The researcher used random sampling to select participants from a population and ensured that each participant had an equal chance of being selected. The results of the experiment suggest that stress can have a negative impact on memory performance.
Random sampling is a useful technique in experimental research as it ensures that the sample is representative of the population and reduces the risk of bias. However, it is important to ensure that the sample size is large enough to provide accurate results and that the participants are selected using a fair and unbiased method.
Case Study: Stratified Sampling in a Public Health Study
In this case study, we will explore the use of stratified sampling in a public health study. Stratified sampling is a technique where the population is divided into subgroups or strata based on specific criteria, and then a random sample is drawn from each stratum. This method is particularly useful when the researcher wants to ensure that the sample is representative of the population and that each stratum is proportionally represented in the sample.
Objectives of the Public Health Study
The primary objective of this public health study was to investigate the prevalence of a particular disease in a specific population and identify any potential risk factors associated with the disease.
Population and Sampling Frame
The population of interest in this study was the adult population living in a specific geographic area. The sampling frame was a list of all adults living in the area, which was obtained from the local government.
Stratification Criteria
The population was stratified based on age, gender, and socioeconomic status. The rationale behind this stratification was to ensure that the sample was representative of the population in terms of these key demographic factors.
Sampling Procedure
A random sample of 1000 adults was drawn from the sampling frame. The sample was stratified based on the three criteria mentioned above, and a random sample of 100 adults was drawn from each stratum.
Data Collection and Analysis
Data was collected through a combination of self-reported surveys and medical examinations. The data was analyzed using statistical software to identify any patterns or associations between the disease and the stratified factors.
Case Study: Cluster Sampling in a Sociology Study
Cluster sampling is a technique that involves dividing a population into smaller groups or clusters and selecting a sample from each cluster. This method is often used in sociology studies to examine social phenomena at the community level. In this case study, we will explore how cluster sampling was used in a sociology study to investigate the impact of a community-based program on crime rates.
Study Design
The study was a quasi-experimental design, where the researchers compared crime rates in a community that received a community-based program aimed at reducing crime with a control community that did not receive the program. The study used cluster sampling to select the communities for the study.
The study used a two-stage sampling process. In the first stage, the researchers identified 20 clusters of census tracts based on their crime rates. Each cluster consisted of contiguous census tracts with similar crime rates. In the second stage, the researchers randomly selected one community from each cluster to participate in the study. The final sample consisted of 10 communities, with five in the treatment group and five in the control group.
Advantages and Disadvantages
Cluster sampling has several advantages, including the ability to collect data from large populations, reduce costs, and increase the generalizability of the findings. However, cluster sampling also has some disadvantages, such as the potential for selection bias and the loss of within-cluster variation.
In this study, the researchers used cluster sampling to address the issue of limited resources and time, as it allowed them to collect data from a large number of communities with limited resources. However, the study was also subject to selection bias, as the researchers chose communities based on their crime rates, which could have influenced the results. Additionally, the use of clusters may have led to a loss of within-cluster variation, as the researchers may have missed important differences between communities within the same cluster.
Cluster sampling is a useful technique in sociology studies when the population is large and diverse, and resources are limited. However, researchers must be aware of the potential for selection bias and the loss of within-cluster variation when using this method. In this case study, the researchers used cluster sampling to investigate the impact of a community-based program on crime rates, but they were subject to selection bias and the loss of within-cluster variation.
Best Practices for Sampling Techniques
1. defining the study population.
Before selecting a sample, it is crucial to define the study population. This involves identifying the individuals or units that meet the criteria for inclusion in the study. For instance, if the study is focused on college students, the study population would include all the college students who meet the inclusion criteria. Defining the study population helps to ensure that the sample is representative of the population of interest.
2. Determining Sample Size
Another best practice is to determine the appropriate sample size for the study. Sample size determination involves estimating the number of participants needed to achieve the desired level of statistical power and precision. Researchers can use sample size calculators or consult statistical experts to determine the appropriate sample size. It is important to note that underpowered samples can lead to incorrect conclusions, while overpowered samples can result in wasted resources.
3. Randomization
Randomization is a critical aspect of sampling techniques in experimental research. It involves assigning participants to treatment groups randomly to minimize selection bias. Randomization can be achieved using various methods, such as simple random sampling, stratified random sampling, or blocked random sampling. Randomization ensures that each participant has an equal chance of being assigned to a particular treatment group, thereby reducing the impact of selection bias.
4. Control of Confounding Variables
Sampling techniques in experimental research should also control for confounding variables. Confounding variables are factors that can influence the outcome of the study and may cause misleading results. Researchers should take steps to control for confounding variables by matching participants or adjusting for potential confounders in the analysis. Failure to control for confounding variables can lead to biased results and incorrect conclusions.
5. Replication and Replication Bias
Finally, best practices for sampling techniques in experimental research should address the issue of replication and replication bias. Replication refers to the process of repeating a study to confirm the results. Replication bias occurs when researchers only report positive findings or selectively publish studies that support their hypotheses. To avoid replication bias, researchers should aim to publish all their findings, regardless of whether they are positive or negative. Replication studies can also help to confirm the validity of previous findings and reduce the impact of sampling bias.
Limitations and Future Directions
Despite the numerous advantages of sampling techniques in experimental research, there are limitations that researchers should be aware of when planning and conducting studies. Moreover, there are areas for future exploration to further enhance the accuracy and validity of experimental findings.
- Sampling Errors: Sampling errors occur when the sample does not accurately represent the population of interest. This can lead to biased results and incorrect conclusions. Researchers should ensure that their sampling technique is appropriate for the research question and population of interest.
- Generalizability: The generalizability of experimental findings depends on the representativeness of the sample. If the sample is not representative of the population, the results may not be generalizable to other settings or groups. Future research should focus on developing more inclusive sampling techniques to improve generalizability.
- Sample Size: The sample size is an important consideration in experimental research. Small samples may not provide sufficient statistical power to detect significant effects, while large samples may be impractical or expensive to obtain. Researchers should consider the trade-offs between sample size and other factors, such as cost and time.
- Non-Response Bias: Non-response bias occurs when non-responders differ systematically from responders. This can lead to biased results and incorrect conclusions. Future research should explore methods to reduce non-response bias, such as incentives for participation or follow-up strategies to encourage response.
- Technological Advancements: Technological advancements offer new opportunities for sampling techniques in experimental research . For example, online surveys and social media platforms provide new avenues for recruiting diverse and representative samples. Future research should explore the potential of these new technologies to improve sampling techniques and enhance experimental validity.
Overall, while sampling techniques have proven to be an essential component of experimental research, it is important to be aware of their limitations and potential biases. Future research should focus on developing new and innovative sampling techniques to overcome these limitations and improve the accuracy and validity of experimental findings.
Resources for Further Learning
If you are interested in learning more about sampling techniques in experimental research , there are a variety of resources available to you. Some useful places to start include:
- “Experimental Design and Analysis: An Introduction” by David Blaxter
- “The Practice of Statistics in the Sciences” by Geoff Cumming and Chris Wallace
- “Design of Experiments: A Practical Perspective” by Richard J. St. Anne
Online Courses
- “Sampling and Sample Size Calculations for Clinical Research” offered by the University of Florida
- “Experimental Design and Analysis” offered by the University of Illinois at Urbana-Champaign
- “Introduction to Statistical Methods for Clinical Research” offered by the University of California, San Diego
- The Statistical Methods for Practice website ( https://www.stats4stem.com/ ) offers a variety of resources for learning about experimental design and sampling techniques.
- The Experimental Design webpage ( https://www.ncsu.edu/statistics/examples/experimental-design/ ) provides an overview of the different types of experimental designs and sampling techniques.
By taking advantage of these resources, you can deepen your understanding of sampling techniques in experimental research and improve your ability to design and analyze experiments.
1. What is sampling in experimental research?
Sampling is the process of selecting a subset of individuals or cases from a larger population for the purpose of studying particular characteristics or behaviors. It is an essential part of experimental research as it helps to ensure that the results obtained are representative of the population being studied.
2. What are the different types of sampling techniques in experimental research?
There are several types of sampling techniques used in experimental research, including random sampling, stratified sampling, cluster sampling, and oversampling/undersampling. Each technique has its own advantages and disadvantages, and the choice of technique depends on the research question, sample size, and population characteristics.
3. What is random sampling in experimental research?
Random sampling is a technique where every individual or case in the population has an equal chance of being selected for the sample. It is considered the most representative and unbiased sampling technique, as it ensures that the sample is a true reflection of the population.
4. What is stratified sampling in experimental research?
Stratified sampling is a technique where the population is divided into smaller groups or strata based on certain characteristics, and a sample is then selected from each stratum. This technique is useful when the population is heterogeneous and the researcher wants to ensure that the sample is representative of each stratum.
5. What is cluster sampling in experimental research?
Cluster sampling is a technique where groups or clusters of individuals or cases are selected for the sample, rather than individuals or cases being selected randomly. This technique is useful when it is difficult or expensive to reach all individuals or cases in the population.
6. What is oversampling/undersampling in experimental research?
Oversampling and undersampling are techniques where the sample size is increased or decreased, respectively, to ensure that certain groups or characteristics are adequately represented in the sample. These techniques are useful when the population is imbalanced or when certain groups or characteristics are underrepresented in the population.
7. How do sampling techniques affect experimental research results?
Sampling techniques can have a significant impact on the results of experimental research. If the sample is not representative of the population, the results may not be generalizable to the population of interest. Therefore, it is essential to carefully consider the sampling technique and ensure that it is appropriate for the research question and population characteristics.
How to Choose a Sampling Technique for Research | Sampling Methods in Research Methodology
Leave a Reply Cancel reply
Your email address will not be published. Required fields are marked *
Save my name, email, and website in this browser for the next time I comment.
Related Articles

Understanding the Role of Sampling Techniques in Research Studies

Understanding the 5 Sampling Techniques and Their Meanings in Research

Understanding the Four Sampling Techniques Used in Research Studies
Educational resources and simple solutions for your research journey
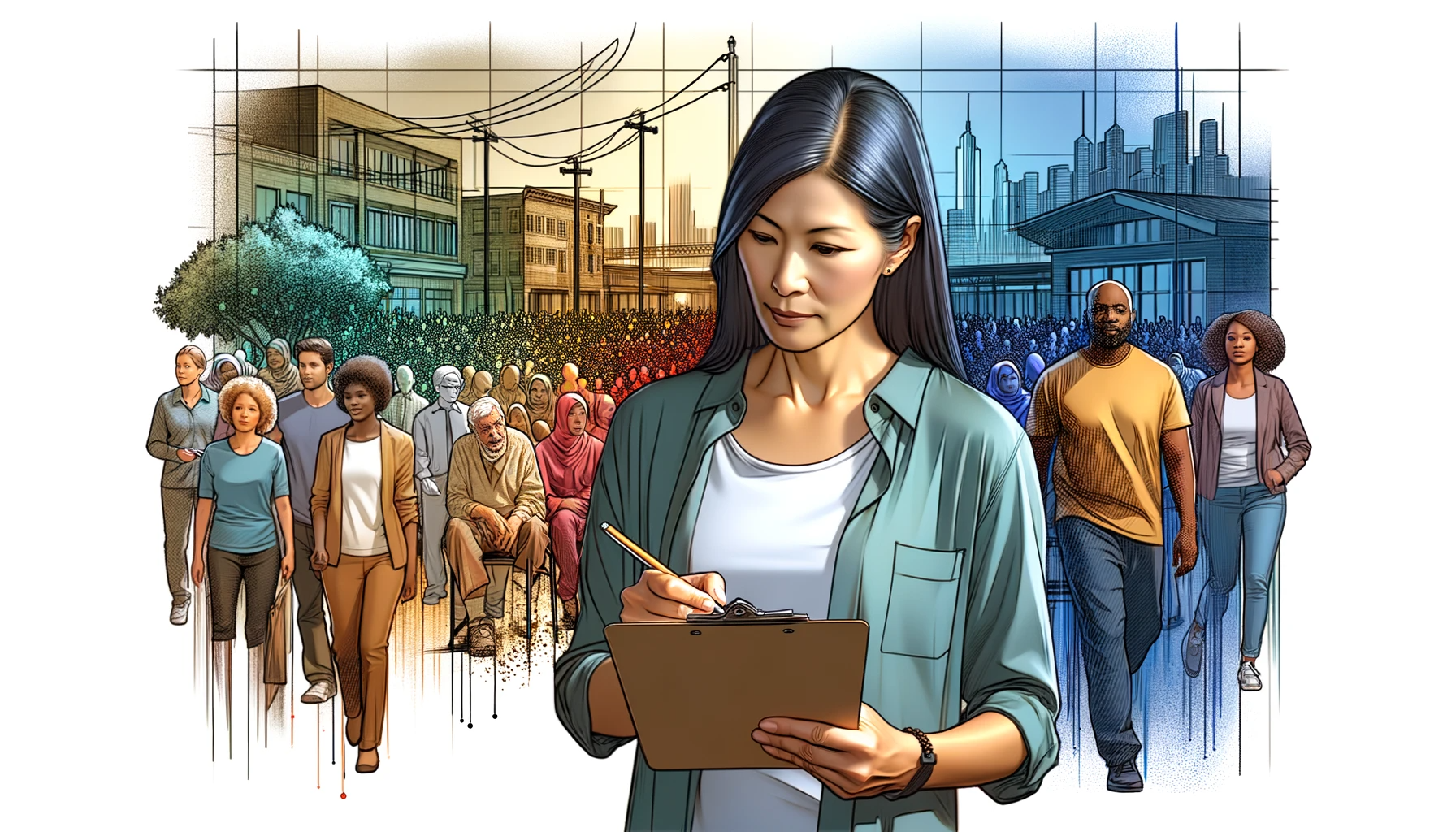
What are Sampling Methods? Techniques, Types, and Examples
Every type of research includes samples from which inferences are drawn. The sample could be biological specimens or a subset of a specific group or population selected for analysis. The goal is often to conclude the entire population based on the characteristics observed in the sample. Now, the question comes to mind: how does one collect the samples? Answer: Using sampling methods. Various sampling strategies are available to researchers to define and collect samples that will form the basis of their research study.
In a study focusing on individuals experiencing anxiety, gathering data from the entire population is practically impossible due to the widespread prevalence of anxiety. Consequently, a sample is carefully selected—a subset of individuals meant to represent (or not in some cases accurately) the demographics of those experiencing anxiety. The study’s outcomes hinge significantly on the chosen sample, emphasizing the critical importance of a thoughtful and precise selection process. The conclusions drawn about the broader population rely heavily on the selected sample’s characteristics and diversity.
Table of Contents
What is sampling?
Sampling involves the strategic selection of individuals or a subset from a population, aiming to derive statistical inferences and predict the characteristics of the entire population. It offers a pragmatic and practical approach to examining the features of the whole population, which would otherwise be difficult to achieve because studying the total population is expensive, time-consuming, and often impossible. Market researchers use various sampling methods to collect samples from a large population to acquire relevant insights. The best sampling strategy for research is determined by criteria such as the purpose of the study, available resources (time and money), and research hypothesis.
For example, if a pet food manufacturer wants to investigate the positive impact of a new cat food on feline growth, studying all the cats in the country is impractical. In such cases, employing an appropriate sampling technique from the extensive dataset allows the researcher to focus on a manageable subset. This enables the researcher to study the growth-promoting effects of the new pet food. This article will delve into the standard sampling methods and explore the situations in which each is most appropriately applied.
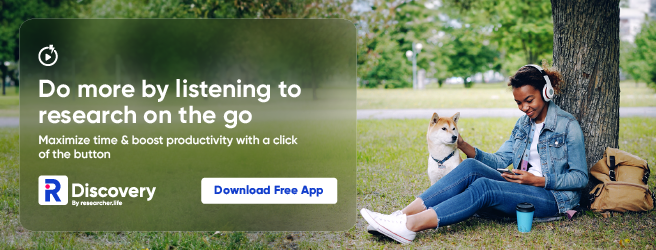
What are sampling methods or sampling techniques?
Sampling methods or sampling techniques in research are statistical methods for selecting a sample representative of the whole population to study the population’s characteristics. Sampling methods serve as invaluable tools for researchers, enabling the collection of meaningful data and facilitating analysis to identify distinctive features of the people. Different sampling strategies can be used based on the characteristics of the population, the study purpose, and the available resources. Now that we understand why sampling methods are essential in research, we review the various sample methods in the following sections.
Types of sampling methods
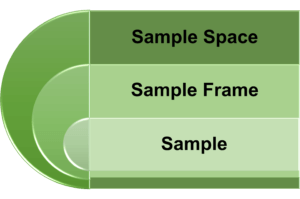
Before we go into the specifics of each sampling method, it’s vital to understand terms like sample, sample frame, and sample space. In probability theory, the sample space comprises all possible outcomes of a random experiment, while the sample frame is the list or source guiding sample selection in statistical research. The sample represents the group of individuals participating in the study, forming the basis for the research findings. Selecting the correct sample is critical to ensuring the validity and reliability of any research; the sample should be representative of the population.
There are two most common sampling methods:
- Probability sampling: A sampling method in which each unit or element in the population has an equal chance of being selected in the final sample. This is called random sampling, emphasizing the random and non-zero probability nature of selecting samples. Such a sampling technique ensures a more representative and unbiased sample, enabling robust inferences about the entire population.
- Non-probability sampling: Another sampling method is non-probability sampling, which involves collecting data conveniently through a non-random selection based on predefined criteria. This offers a straightforward way to gather data, although the resulting sample may or may not accurately represent the entire population.
Irrespective of the research method you opt for, it is essential to explicitly state the chosen sampling technique in the methodology section of your research article. Now, we will explore the different characteristics of both sampling methods, along with various subtypes falling under these categories.
What is probability sampling?
The probability sampling method is based on the probability theory, which means that the sample selection criteria involve some random selection. The probability sampling method provides an equal opportunity for all elements or units within the entire sample space to be chosen. While it can be labor-intensive and expensive, the advantage lies in its ability to offer a more accurate representation of the population, thereby enhancing confidence in the inferences drawn in the research.
Types of probability sampling
Various probability sampling methods exist, such as simple random sampling, systematic sampling, stratified sampling, and clustered sampling. Here, we provide detailed discussions and illustrative examples for each of these sampling methods:
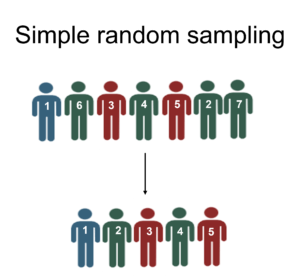
- Simple random sampling: In simple random sampling, each individual has an equal probability of being chosen, and each selection is independent of the others. Because the choice is entirely based on chance, this is also known as the method of chance selection. In the simple random sampling method, the sample frame comprises the entire population.
For example, A fitness sports brand is launching a new protein drink and aims to select 20 individuals from a 200-person fitness center to try it. Employing a simple random sampling approach, each of the 200 people is assigned a unique identifier. Of these, 20 individuals are then chosen by generating random numbers between 1 and 200, either manually or through a computer program. Matching these numbers with the individuals creates a randomly selected group of 20 people. This method minimizes sampling bias and ensures a representative subset of the entire population under study.
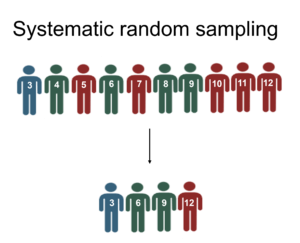
- Systematic sampling: The systematic sampling approach involves selecting units or elements at regular intervals from an ordered list of the population. Because the starting point of this sampling method is chosen at random, it is more convenient than essential random sampling. For a better understanding, consider the following example.
For example, considering the previous model, individuals at the fitness facility are arranged alphabetically. The manufacturer then initiates the process by randomly selecting a starting point from the first ten positions, let’s say 8. Starting from the 8th position, every tenth person on the list is then chosen (e.g., 8, 18, 28, 38, and so forth) until a sample of 20 individuals is obtained.
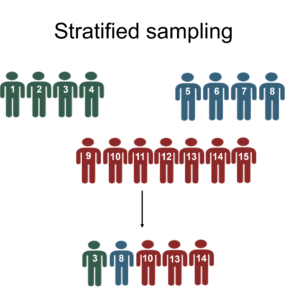
- Stratified sampling: Stratified sampling divides the population into subgroups (strata), and random samples are drawn from each stratum in proportion to its size in the population. Stratified sampling provides improved representation because each subgroup that differs in significant ways is included in the final sample.
For example, Expanding on the previous simple random sampling example, suppose the manufacturer aims for a more comprehensive representation of genders in a sample of 200 people, consisting of 90 males, 80 females, and 30 others. The manufacturer categorizes the population into three gender strata (Male, Female, and Others). Within each group, random sampling is employed to select nine males, eight females, and three individuals from the others category, resulting in a well-rounded and representative sample of 200 individuals.
- Clustered sampling: In this sampling method, the population is divided into clusters, and then a random sample of clusters is included in the final sample. Clustered sampling, distinct from stratified sampling, involves subgroups (clusters) that exhibit characteristics similar to the whole sample. In the case of small clusters, all members can be included in the final sample, whereas for larger clusters, individuals within each cluster may be sampled using the sampling above methods. This approach is referred to as multistage sampling. This sampling method is well-suited for large and widely distributed populations; however, there is a potential risk of sample error because ensuring that the sampled clusters truly represent the entire population can be challenging.
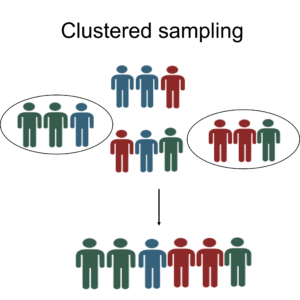
For example, Researchers conducting a nationwide health study can select specific geographic clusters, like cities or regions, instead of trying to survey the entire population individually. Within each chosen cluster, they sample individuals, providing a representative subset without the logistical challenges of attempting a nationwide survey.
Use s of probability sampling
Probability sampling methods find widespread use across diverse research disciplines because of their ability to yield representative and unbiased samples. The advantages of employing probability sampling include the following:
- Representativeness
Probability sampling assures that every element in the population has a non-zero chance of being included in the sample, ensuring representativeness of the entire population and decreasing research bias to minimal to non-existent levels. The researcher can acquire higher-quality data via probability sampling, increasing confidence in the conclusions.
- Statistical inference
Statistical methods, like confidence intervals and hypothesis testing, depend on probability sampling to generalize findings from a sample to the broader population. Probability sampling methods ensure unbiased representation, allowing inferences about the population based on the characteristics of the sample.
- Precision and reliability
The use of probability sampling improves the precision and reliability of study results. Because the probability of selecting any single element/individual is known, the chance variations that may occur in non-probability sampling methods are reduced, resulting in more dependable and precise estimations.
- Generalizability
Probability sampling enables the researcher to generalize study findings to the entire population from which they were derived. The results produced through probability sampling methods are more likely to be applicable to the larger population, laying the foundation for making broad predictions or recommendations.
- Minimization of Selection Bias
By ensuring that each member of the population has an equal chance of being selected in the sample, probability sampling lowers the possibility of selection bias. This reduces the impact of systematic errors that may occur in non-probability sampling methods, where data may be skewed toward a specific demographic due to inadequate representation of each segment of the population.
What is non-probability sampling?
Non-probability sampling methods involve selecting individuals based on non-random criteria, often relying on the researcher’s judgment or predefined criteria. While it is easier and more economical, it tends to introduce sampling bias, resulting in weaker inferences compared to probability sampling techniques in research.
Types of Non-probability Sampling
Non-probability sampling methods are further classified as convenience sampling, consecutive sampling, quota sampling, purposive or judgmental sampling, and snowball sampling. Let’s explore these types of sampling methods in detail.
- Convenience sampling: In convenience sampling, individuals are recruited directly from the population based on the accessibility and proximity to the researcher. It is a simple, inexpensive, and practical method of sample selection, yet convenience sampling suffers from both sampling and selection bias due to a lack of appropriate population representation.
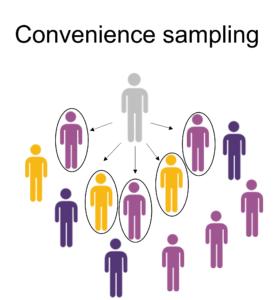
For example, imagine you’re a researcher investigating smartphone usage patterns in your city. The most convenient way to select participants is by approaching people in a shopping mall on a weekday afternoon. However, this convenience sampling method may not be an accurate representation of the city’s overall smartphone usage patterns as the sample is limited to individuals present at the mall during weekdays, excluding those who visit on other days or never visit the mall.
- Consecutive sampling: Participants in consecutive sampling (or sequential sampling) are chosen based on their availability and desire to participate in the study as they become available. This strategy entails sequentially recruiting individuals who fulfill the researcher’s requirements.
For example, In researching the prevalence of stroke in a hospital, instead of randomly selecting patients from the entire population, the researcher can opt to include all eligible patients admitted over three months. Participants are then consecutively recruited upon admission during that timeframe, forming the study sample.
- Quota sampling: The selection of individuals in quota sampling is based on non-random selection criteria in which only participants with certain traits or proportions that are representative of the population are included. Quota sampling involves setting predetermined quotas for specific subgroups based on key demographics or other relevant characteristics. This sampling method employs dividing the population into mutually exclusive subgroups and then selecting sample units until the set quota is reached.
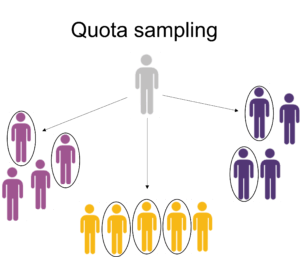
For example, In a survey on a college campus to assess student interest in a new policy, the researcher should establish quotas aligned with the distribution of student majors, ensuring representation from various academic disciplines. If the campus has 20% biology majors, 30% engineering majors, 20% business majors, and 30% liberal arts majors, participants should be recruited to mirror these proportions.
- Purposive or judgmental sampling: In purposive sampling, the researcher leverages expertise to select a sample relevant to the study’s specific questions. This sampling method is commonly applied in qualitative research, mainly when aiming to understand a particular phenomenon, and is suitable for smaller population sizes.
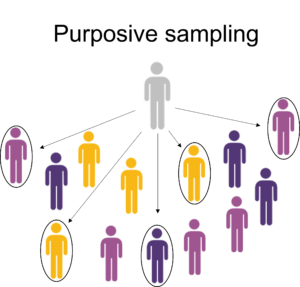
For example, imagine a researcher who wants to study public policy issues for a focus group. The researcher might purposely select participants with expertise in economics, law, and public administration to take advantage of their knowledge and ensure a depth of understanding.
- Snowball sampling: This sampling method is used when accessing the population is challenging. It involves collecting the sample through a chain-referral process, where each recruited candidate aids in finding others. These candidates share common traits, representing the targeted population. This method is often used in qualitative research, particularly when studying phenomena related to stigmatized or hidden populations.

For example, In a study focusing on understanding the experiences and challenges of individuals in hidden or stigmatized communities (e.g., LGBTQ+ individuals in specific cultural contexts), the snowball sampling technique can be employed. The researcher initiates contact with one community member, who then assists in identifying additional candidates until the desired sample size is achieved.
Uses of non-probability sampling
Non-probability sampling approaches are employed in qualitative or exploratory research where the goal is to investigate underlying population traits rather than generalizability. Non-probability sampling methods are also helpful for the following purposes:
- Generating a hypothesis
In the initial stages of exploratory research, non-probability methods such as purposive or convenience allow researchers to quickly gather information and generate hypothesis that helps build a future research plan.
- Qualitative research
Qualitative research is usually focused on understanding the depth and complexity of human experiences, behaviors, and perspectives. Non-probability methods like purposive or snowball sampling are commonly used to select participants with specific traits that are relevant to the research question.
- Convenience and pragmatism
Non-probability sampling methods are valuable when resource and time are limited or when preliminary data is required to test the pilot study. For example, conducting a survey at a local shopping mall to gather opinions on a consumer product due to the ease of access to potential participants.
Probability vs Non-probability Sampling Methods
Selection of participants | Random selection of participants from the population using randomization methods | Non-random selection of participants from the population based on convenience or criteria |
Representativeness | Likely to yield a representative sample of the whole population allowing for generalizations | May not yield a representative sample of the whole population; poor generalizability |
Precision and accuracy | Provides more precise and accurate estimates of population characteristics | May have less precision and accuracy due to non-random selection |
Bias | Minimizes selection bias | May introduce selection bias if criteria are subjective and not well-defined |
Statistical inference | Suited for statistical inference and hypothesis testing and for making generalization to the population | Less suited for statistical inference and hypothesis testing on the population |
Application | Useful for quantitative research where generalizability is crucial | Commonly used in qualitative and exploratory research where in-depth insights are the goal |
Frequently asked questions
- What is multistage sampling ? Multistage sampling is a form of probability sampling approach that involves the progressive selection of samples in stages, going from larger clusters to a small number of participants, making it suited for large-scale research with enormous population lists.
- What are the methods of probability sampling? Probability sampling methods are simple random sampling, stratified random sampling, systematic sampling, cluster sampling, and multistage sampling.
- How to decide which type of sampling method to use? Choose a sampling method based on the goals, population, and resources. Probability for statistics and non-probability for efficiency or qualitative insights can be considered . Also, consider the population characteristics, size, and alignment with study objectives.
- What are the methods of non-probability sampling? Non-probability sampling methods are convenience sampling, consecutive sampling, purposive sampling, snowball sampling, and quota sampling.
- Why are sampling methods used in research? Sampling methods in research are employed to efficiently gather representative data from a subset of a larger population, enabling valid conclusions and generalizations while minimizing costs and time.
R Discovery is a literature search and research reading platform that accelerates your research discovery journey by keeping you updated on the latest, most relevant scholarly content. With 250M+ research articles sourced from trusted aggregators like CrossRef, Unpaywall, PubMed, PubMed Central, Open Alex and top publishing houses like Springer Nature, JAMA, IOP, Taylor & Francis, NEJM, BMJ, Karger, SAGE, Emerald Publishing and more, R Discovery puts a world of research at your fingertips.
Try R Discovery Prime FREE for 1 week or upgrade at just US$72 a year to access premium features that let you listen to research on the go, read in your language, collaborate with peers, auto sync with reference managers, and much more. Choose a simpler, smarter way to find and read research – Download the app and start your free 7-day trial today !
Related Posts

What are the Best Research Funding Sources

What are Experimental Groups in Research
Have a language expert improve your writing
Run a free plagiarism check in 10 minutes, automatically generate references for free.
- Knowledge Base
- Methodology
- Sampling Methods | Types, Techniques, & Examples
Sampling Methods | Types, Techniques, & Examples
Published on 3 May 2022 by Shona McCombes . Revised on 10 October 2022.
When you conduct research about a group of people, it’s rarely possible to collect data from every person in that group. Instead, you select a sample. The sample is the group of individuals who will actually participate in the research.
To draw valid conclusions from your results, you have to carefully decide how you will select a sample that is representative of the group as a whole. There are two types of sampling methods:
- Probability sampling involves random selection, allowing you to make strong statistical inferences about the whole group. It minimises the risk of selection bias .
- Non-probability sampling involves non-random selection based on convenience or other criteria, allowing you to easily collect data.
You should clearly explain how you selected your sample in the methodology section of your paper or thesis.
Table of contents
Population vs sample, probability sampling methods, non-probability sampling methods, frequently asked questions about sampling.
First, you need to understand the difference between a population and a sample , and identify the target population of your research.
- The population is the entire group that you want to draw conclusions about.
- The sample is the specific group of individuals that you will collect data from.
The population can be defined in terms of geographical location, age, income, and many other characteristics.
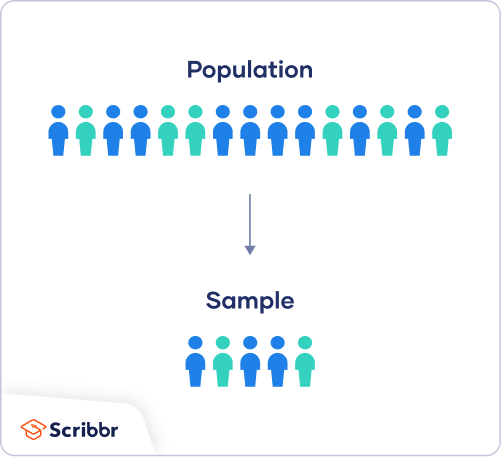
It is important to carefully define your target population according to the purpose and practicalities of your project.
If the population is very large, demographically mixed, and geographically dispersed, it might be difficult to gain access to a representative sample.
Sampling frame
The sampling frame is the actual list of individuals that the sample will be drawn from. Ideally, it should include the entire target population (and nobody who is not part of that population).
You are doing research on working conditions at Company X. Your population is all 1,000 employees of the company. Your sampling frame is the company’s HR database, which lists the names and contact details of every employee.
Sample size
The number of individuals you should include in your sample depends on various factors, including the size and variability of the population and your research design. There are different sample size calculators and formulas depending on what you want to achieve with statistical analysis .
Prevent plagiarism, run a free check.
Probability sampling means that every member of the population has a chance of being selected. It is mainly used in quantitative research . If you want to produce results that are representative of the whole population, probability sampling techniques are the most valid choice.
There are four main types of probability sample.
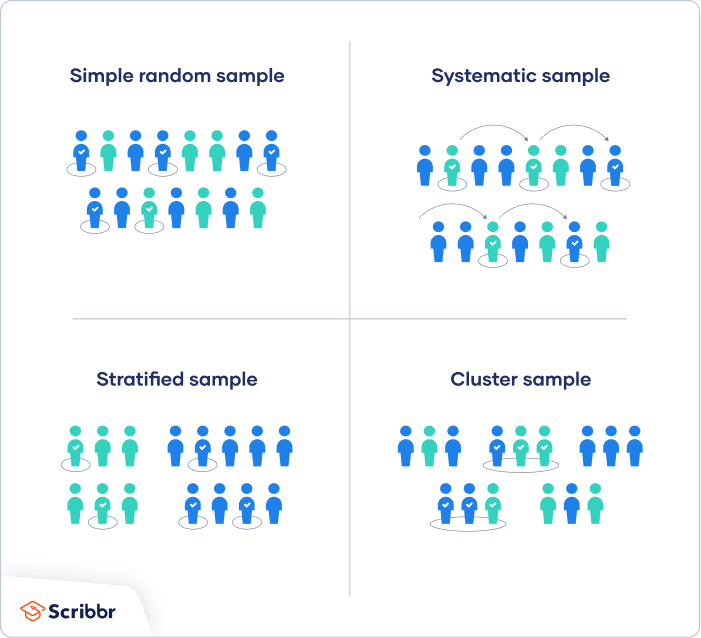
1. Simple random sampling
In a simple random sample , every member of the population has an equal chance of being selected. Your sampling frame should include the whole population.
To conduct this type of sampling, you can use tools like random number generators or other techniques that are based entirely on chance.
You want to select a simple random sample of 100 employees of Company X. You assign a number to every employee in the company database from 1 to 1000, and use a random number generator to select 100 numbers.
2. Systematic sampling
Systematic sampling is similar to simple random sampling, but it is usually slightly easier to conduct. Every member of the population is listed with a number, but instead of randomly generating numbers, individuals are chosen at regular intervals.
All employees of the company are listed in alphabetical order. From the first 10 numbers, you randomly select a starting point: number 6. From number 6 onwards, every 10th person on the list is selected (6, 16, 26, 36, and so on), and you end up with a sample of 100 people.
If you use this technique, it is important to make sure that there is no hidden pattern in the list that might skew the sample. For example, if the HR database groups employees by team, and team members are listed in order of seniority, there is a risk that your interval might skip over people in junior roles, resulting in a sample that is skewed towards senior employees.
3. Stratified sampling
Stratified sampling involves dividing the population into subpopulations that may differ in important ways. It allows you draw more precise conclusions by ensuring that every subgroup is properly represented in the sample.
To use this sampling method, you divide the population into subgroups (called strata) based on the relevant characteristic (e.g., gender, age range, income bracket, job role).
Based on the overall proportions of the population, you calculate how many people should be sampled from each subgroup. Then you use random or systematic sampling to select a sample from each subgroup.
The company has 800 female employees and 200 male employees. You want to ensure that the sample reflects the gender balance of the company, so you sort the population into two strata based on gender. Then you use random sampling on each group, selecting 80 women and 20 men, which gives you a representative sample of 100 people.
4. Cluster sampling
Cluster sampling also involves dividing the population into subgroups, but each subgroup should have similar characteristics to the whole sample. Instead of sampling individuals from each subgroup, you randomly select entire subgroups.
If it is practically possible, you might include every individual from each sampled cluster. If the clusters themselves are large, you can also sample individuals from within each cluster using one of the techniques above. This is called multistage sampling .
This method is good for dealing with large and dispersed populations, but there is more risk of error in the sample, as there could be substantial differences between clusters. It’s difficult to guarantee that the sampled clusters are really representative of the whole population.
The company has offices in 10 cities across the country (all with roughly the same number of employees in similar roles). You don’t have the capacity to travel to every office to collect your data, so you use random sampling to select 3 offices – these are your clusters.
In a non-probability sample , individuals are selected based on non-random criteria, and not every individual has a chance of being included.
This type of sample is easier and cheaper to access, but it has a higher risk of sampling bias . That means the inferences you can make about the population are weaker than with probability samples, and your conclusions may be more limited. If you use a non-probability sample, you should still aim to make it as representative of the population as possible.
Non-probability sampling techniques are often used in exploratory and qualitative research . In these types of research, the aim is not to test a hypothesis about a broad population, but to develop an initial understanding of a small or under-researched population.
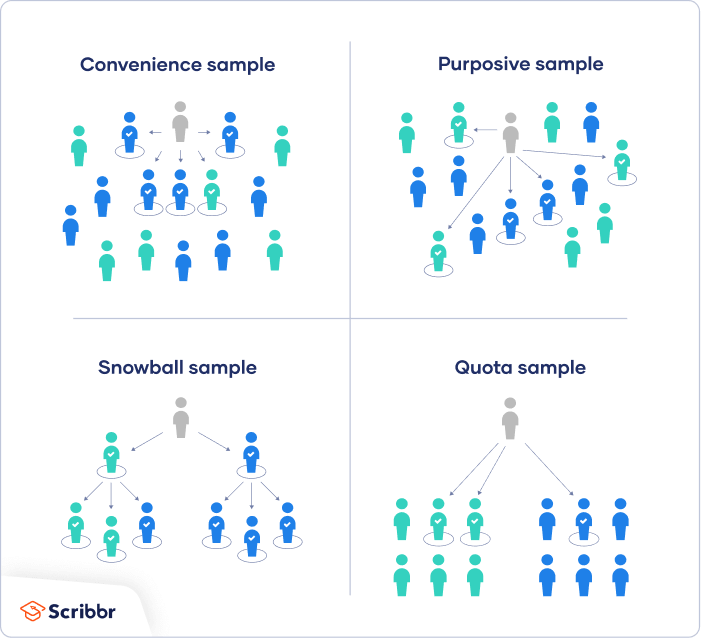
1. Convenience sampling
A convenience sample simply includes the individuals who happen to be most accessible to the researcher.
This is an easy and inexpensive way to gather initial data, but there is no way to tell if the sample is representative of the population, so it can’t produce generalisable results.
You are researching opinions about student support services in your university, so after each of your classes, you ask your fellow students to complete a survey on the topic. This is a convenient way to gather data, but as you only surveyed students taking the same classes as you at the same level, the sample is not representative of all the students at your university.
2. Voluntary response sampling
Similar to a convenience sample, a voluntary response sample is mainly based on ease of access. Instead of the researcher choosing participants and directly contacting them, people volunteer themselves (e.g., by responding to a public online survey).
Voluntary response samples are always at least somewhat biased, as some people will inherently be more likely to volunteer than others.
You send out the survey to all students at your university and many students decide to complete it. This can certainly give you some insight into the topic, but the people who responded are more likely to be those who have strong opinions about the student support services, so you can’t be sure that their opinions are representative of all students.
3. Purposive sampling
Purposive sampling , also known as judgement sampling, involves the researcher using their expertise to select a sample that is most useful to the purposes of the research.
It is often used in qualitative research , where the researcher wants to gain detailed knowledge about a specific phenomenon rather than make statistical inferences, or where the population is very small and specific. An effective purposive sample must have clear criteria and rationale for inclusion.
You want to know more about the opinions and experiences of students with a disability at your university, so you purposely select a number of students with different support needs in order to gather a varied range of data on their experiences with student services.
4. Snowball sampling
If the population is hard to access, snowball sampling can be used to recruit participants via other participants. The number of people you have access to ‘snowballs’ as you get in contact with more people.
You are researching experiences of homelessness in your city. Since there is no list of all homeless people in the city, probability sampling isn’t possible. You meet one person who agrees to participate in the research, and she puts you in contact with other homeless people she knows in the area.
A sample is a subset of individuals from a larger population. Sampling means selecting the group that you will actually collect data from in your research.
For example, if you are researching the opinions of students in your university, you could survey a sample of 100 students.
Statistical sampling allows you to test a hypothesis about the characteristics of a population. There are various sampling methods you can use to ensure that your sample is representative of the population as a whole.
Samples are used to make inferences about populations . Samples are easier to collect data from because they are practical, cost-effective, convenient, and manageable.
Probability sampling means that every member of the target population has a known chance of being included in the sample.
Probability sampling methods include simple random sampling , systematic sampling , stratified sampling , and cluster sampling .
In non-probability sampling , the sample is selected based on non-random criteria, and not every member of the population has a chance of being included.
Common non-probability sampling methods include convenience sampling , voluntary response sampling, purposive sampling , snowball sampling , and quota sampling .
Sampling bias occurs when some members of a population are systematically more likely to be selected in a sample than others.
Cite this Scribbr article
If you want to cite this source, you can copy and paste the citation or click the ‘Cite this Scribbr article’ button to automatically add the citation to our free Reference Generator.
McCombes, S. (2022, October 10). Sampling Methods | Types, Techniques, & Examples. Scribbr. Retrieved 12 August 2024, from https://www.scribbr.co.uk/research-methods/sampling/
Is this article helpful?
Shona McCombes
Other students also liked, what is quantitative research | definition & methods, a quick guide to experimental design | 5 steps & examples, controlled experiments | methods & examples of control.
- En español – ExME
- Em português – EME
What are sampling methods and how do you choose the best one?
Posted on 18th November 2020 by Mohamed Khalifa
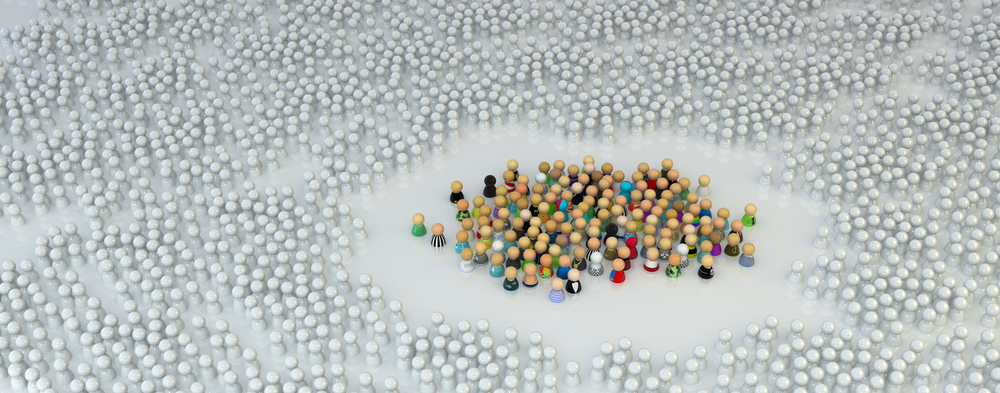
This tutorial will introduce sampling methods and potential sampling errors to avoid when conducting medical research.
Introduction to sampling methods
Examples of different sampling methods, choosing the best sampling method.
It is important to understand why we sample the population; for example, studies are built to investigate the relationships between risk factors and disease. In other words, we want to find out if this is a true association, while still aiming for the minimum risk for errors such as: chance, bias or confounding .
However, it would not be feasible to experiment on the whole population, we would need to take a good sample and aim to reduce the risk of having errors by proper sampling technique.
What is a sampling frame?
A sampling frame is a record of the target population containing all participants of interest. In other words, it is a list from which we can extract a sample.
What makes a good sample?
A good sample should be a representative subset of the population we are interested in studying, therefore, with each participant having equal chance of being randomly selected into the study.
We could choose a sampling method based on whether we want to account for sampling bias; a random sampling method is often preferred over a non-random method for this reason. Random sampling examples include: simple, systematic, stratified, and cluster sampling. Non-random sampling methods are liable to bias, and common examples include: convenience, purposive, snowballing, and quota sampling. For the purposes of this blog we will be focusing on random sampling methods .
Example: We want to conduct an experimental trial in a small population such as: employees in a company, or students in a college. We include everyone in a list and use a random number generator to select the participants
Advantages: Generalisable results possible, random sampling, the sampling frame is the whole population, every participant has an equal probability of being selected
Disadvantages: Less precise than stratified method, less representative than the systematic method
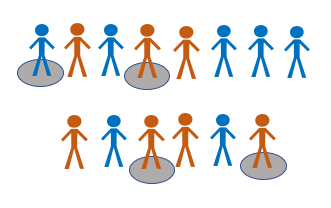
Example: Every nth patient entering the out-patient clinic is selected and included in our sample
Advantages: More feasible than simple or stratified methods, sampling frame is not always required
Disadvantages: Generalisability may decrease if baseline characteristics repeat across every nth participant
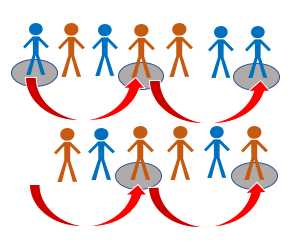
Example: We have a big population (a city) and we want to ensure representativeness of all groups with a pre-determined characteristic such as: age groups, ethnic origin, and gender
Advantages: Inclusive of strata (subgroups), reliable and generalisable results
Disadvantages: Does not work well with multiple variables
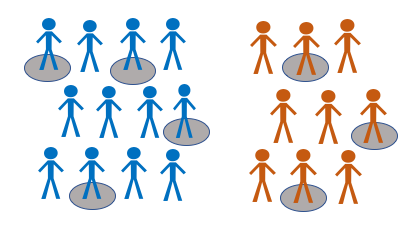
Example: 10 schools have the same number of students across the county. We can randomly select 3 out of 10 schools as our clusters
Advantages: Readily doable with most budgets, does not require a sampling frame
Disadvantages: Results may not be reliable nor generalisable
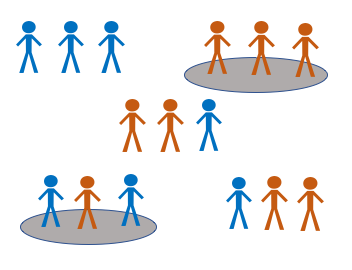
How can you identify sampling errors?
Non-random selection increases the probability of sampling (selection) bias if the sample does not represent the population we want to study. We could avoid this by random sampling and ensuring representativeness of our sample with regards to sample size.
An inadequate sample size decreases the confidence in our results as we may think there is no significant difference when actually there is. This type two error results from having a small sample size, or from participants dropping out of the sample.
In medical research of disease, if we select people with certain diseases while strictly excluding participants with other co-morbidities, we run the risk of diagnostic purity bias where important sub-groups of the population are not represented.
Furthermore, measurement bias may occur during re-collection of risk factors by participants (recall bias) or assessment of outcome where people who live longer are associated with treatment success, when in fact people who died were not included in the sample or data analysis (survivors bias).
By following the steps below we could choose the best sampling method for our study in an orderly fashion.
Research objectiveness
Firstly, a refined research question and goal would help us define our population of interest. If our calculated sample size is small then it would be easier to get a random sample. If, however, the sample size is large, then we should check if our budget and resources can handle a random sampling method.
Sampling frame availability
Secondly, we need to check for availability of a sampling frame (Simple), if not, could we make a list of our own (Stratified). If neither option is possible, we could still use other random sampling methods, for instance, systematic or cluster sampling.
Study design
Moreover, we could consider the prevalence of the topic (exposure or outcome) in the population, and what would be the suitable study design. In addition, checking if our target population is widely varied in its baseline characteristics. For example, a population with large ethnic subgroups could best be studied using a stratified sampling method.
Random sampling
Finally, the best sampling method is always the one that could best answer our research question while also allowing for others to make use of our results (generalisability of results). When we cannot afford a random sampling method, we can always choose from the non-random sampling methods.
To sum up, we now understand that choosing between random or non-random sampling methods is multifactorial. We might often be tempted to choose a convenience sample from the start, but that would not only decrease precision of our results, and would make us miss out on producing research that is more robust and reliable.
References (pdf)
Mohamed Khalifa
Leave a reply cancel reply.
Your email address will not be published. Required fields are marked *
Save my name, email, and website in this browser for the next time I comment.
No Comments on What are sampling methods and how do you choose the best one?
Thank you for this overview. A concise approach for research.
really helps! am an ecology student preparing to write my lab report for sampling.
I learned a lot to the given presentation.. It’s very comprehensive… Thanks for sharing…
Very informative and useful for my study. Thank you
Oversimplified info on sampling methods. Probabilistic of the sampling and sampling of samples by chance does rest solely on the random methods. Factors such as the random visits or presentation of the potential participants at clinics or sites could be sufficiently random in nature and should be used for the sake of efficiency and feasibility. Nevertheless, this approach has to be taken only after careful thoughts. Representativeness of the study samples have to be checked at the end or during reporting by comparing it to the published larger studies or register of some kind in/from the local population.
Thank you so much Mr.mohamed very useful and informative article
Subscribe to our newsletter
You will receive our monthly newsletter and free access to Trip Premium.
Related Articles
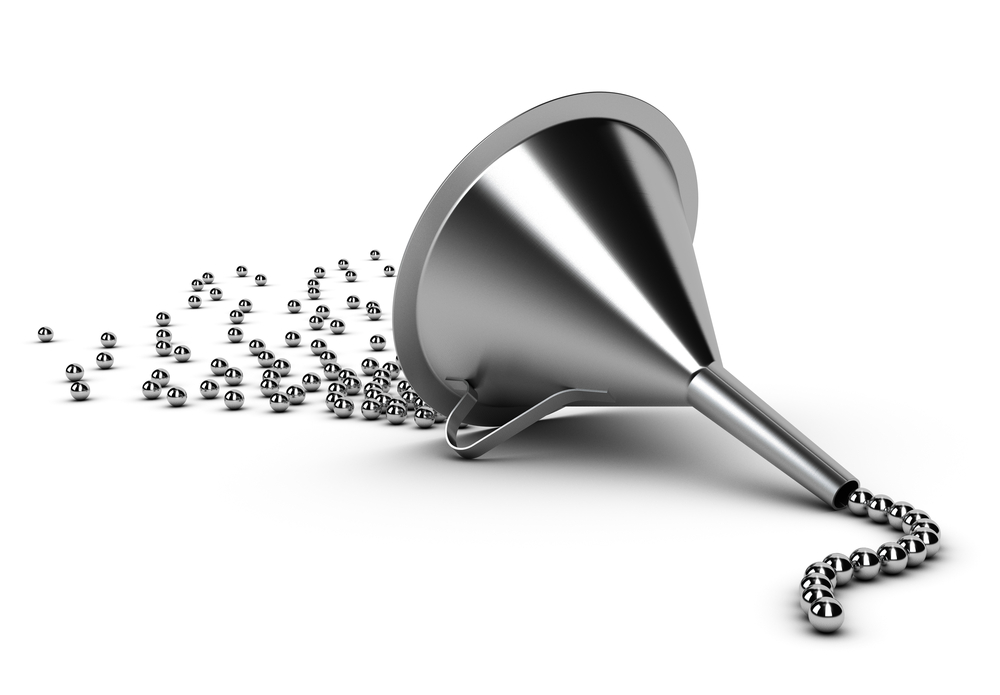
How to read a funnel plot
This blog introduces you to funnel plots, guiding you through how to read them and what may cause them to look asymmetrical.
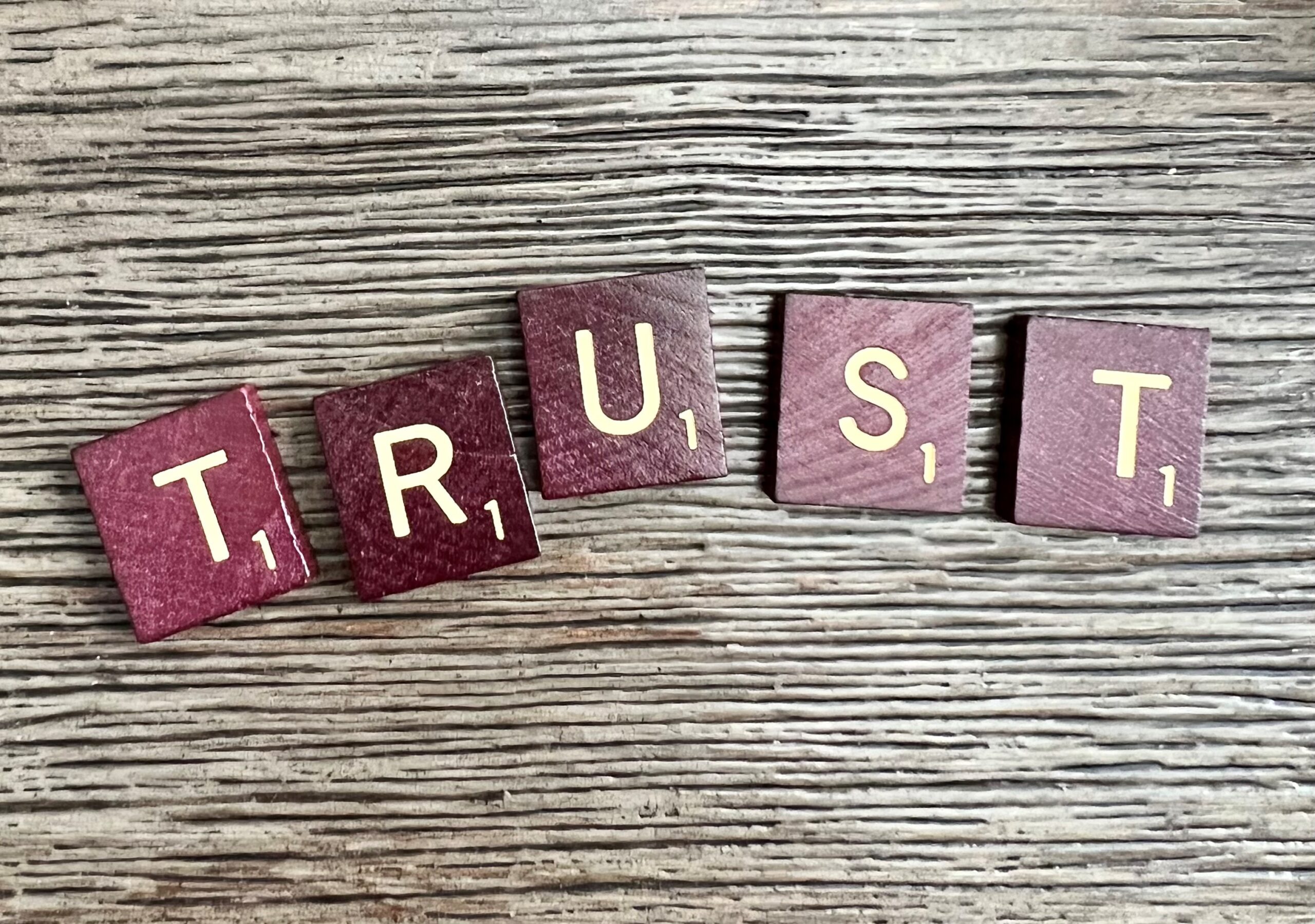
Internal and external validity: what are they and how do they differ?
Is this study valid? Can I trust this study’s methods and design? Can I apply the results of this study to other contexts? Learn more about internal and external validity in research to help you answer these questions when you next look at a paper.
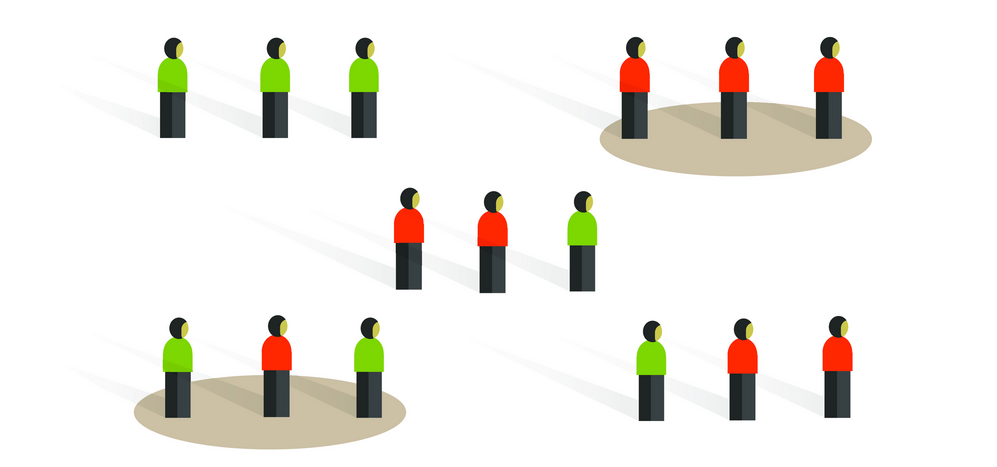
Cluster Randomized Trials: Concepts
This blog summarizes the concepts of cluster randomization, and the logistical and statistical considerations while designing a cluster randomized controlled trial.
Have a language expert improve your writing
Run a free plagiarism check in 10 minutes, generate accurate citations for free.
- Knowledge Base
Methodology
- What Is a Research Design | Types, Guide & Examples
What Is a Research Design | Types, Guide & Examples
Published on June 7, 2021 by Shona McCombes . Revised on November 20, 2023 by Pritha Bhandari.
A research design is a strategy for answering your research question using empirical data. Creating a research design means making decisions about:
- Your overall research objectives and approach
- Whether you’ll rely on primary research or secondary research
- Your sampling methods or criteria for selecting subjects
- Your data collection methods
- The procedures you’ll follow to collect data
- Your data analysis methods
A well-planned research design helps ensure that your methods match your research objectives and that you use the right kind of analysis for your data.
Table of contents
Step 1: consider your aims and approach, step 2: choose a type of research design, step 3: identify your population and sampling method, step 4: choose your data collection methods, step 5: plan your data collection procedures, step 6: decide on your data analysis strategies, other interesting articles, frequently asked questions about research design.
- Introduction
Before you can start designing your research, you should already have a clear idea of the research question you want to investigate.
There are many different ways you could go about answering this question. Your research design choices should be driven by your aims and priorities—start by thinking carefully about what you want to achieve.
The first choice you need to make is whether you’ll take a qualitative or quantitative approach.
Qualitative approach | Quantitative approach |
---|---|
and describe frequencies, averages, and correlations about relationships between variables |
Qualitative research designs tend to be more flexible and inductive , allowing you to adjust your approach based on what you find throughout the research process.
Quantitative research designs tend to be more fixed and deductive , with variables and hypotheses clearly defined in advance of data collection.
It’s also possible to use a mixed-methods design that integrates aspects of both approaches. By combining qualitative and quantitative insights, you can gain a more complete picture of the problem you’re studying and strengthen the credibility of your conclusions.
Practical and ethical considerations when designing research
As well as scientific considerations, you need to think practically when designing your research. If your research involves people or animals, you also need to consider research ethics .
- How much time do you have to collect data and write up the research?
- Will you be able to gain access to the data you need (e.g., by travelling to a specific location or contacting specific people)?
- Do you have the necessary research skills (e.g., statistical analysis or interview techniques)?
- Will you need ethical approval ?
At each stage of the research design process, make sure that your choices are practically feasible.
Receive feedback on language, structure, and formatting
Professional editors proofread and edit your paper by focusing on:
- Academic style
- Vague sentences
- Style consistency
See an example
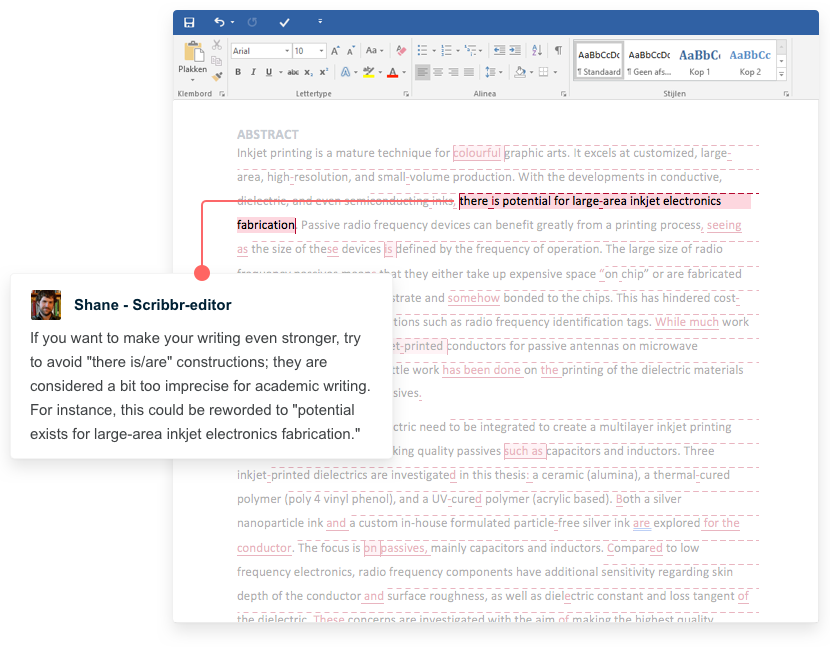
Within both qualitative and quantitative approaches, there are several types of research design to choose from. Each type provides a framework for the overall shape of your research.
Types of quantitative research designs
Quantitative designs can be split into four main types.
- Experimental and quasi-experimental designs allow you to test cause-and-effect relationships
- Descriptive and correlational designs allow you to measure variables and describe relationships between them.
Type of design | Purpose and characteristics |
---|---|
Experimental | relationships effect on a |
Quasi-experimental | ) |
Correlational | |
Descriptive |
With descriptive and correlational designs, you can get a clear picture of characteristics, trends and relationships as they exist in the real world. However, you can’t draw conclusions about cause and effect (because correlation doesn’t imply causation ).
Experiments are the strongest way to test cause-and-effect relationships without the risk of other variables influencing the results. However, their controlled conditions may not always reflect how things work in the real world. They’re often also more difficult and expensive to implement.
Types of qualitative research designs
Qualitative designs are less strictly defined. This approach is about gaining a rich, detailed understanding of a specific context or phenomenon, and you can often be more creative and flexible in designing your research.
The table below shows some common types of qualitative design. They often have similar approaches in terms of data collection, but focus on different aspects when analyzing the data.
Type of design | Purpose and characteristics |
---|---|
Grounded theory | |
Phenomenology |
Your research design should clearly define who or what your research will focus on, and how you’ll go about choosing your participants or subjects.
In research, a population is the entire group that you want to draw conclusions about, while a sample is the smaller group of individuals you’ll actually collect data from.
Defining the population
A population can be made up of anything you want to study—plants, animals, organizations, texts, countries, etc. In the social sciences, it most often refers to a group of people.
For example, will you focus on people from a specific demographic, region or background? Are you interested in people with a certain job or medical condition, or users of a particular product?
The more precisely you define your population, the easier it will be to gather a representative sample.
- Sampling methods
Even with a narrowly defined population, it’s rarely possible to collect data from every individual. Instead, you’ll collect data from a sample.
To select a sample, there are two main approaches: probability sampling and non-probability sampling . The sampling method you use affects how confidently you can generalize your results to the population as a whole.
Probability sampling | Non-probability sampling |
---|---|
Probability sampling is the most statistically valid option, but it’s often difficult to achieve unless you’re dealing with a very small and accessible population.
For practical reasons, many studies use non-probability sampling, but it’s important to be aware of the limitations and carefully consider potential biases. You should always make an effort to gather a sample that’s as representative as possible of the population.
Case selection in qualitative research
In some types of qualitative designs, sampling may not be relevant.
For example, in an ethnography or a case study , your aim is to deeply understand a specific context, not to generalize to a population. Instead of sampling, you may simply aim to collect as much data as possible about the context you are studying.
In these types of design, you still have to carefully consider your choice of case or community. You should have a clear rationale for why this particular case is suitable for answering your research question .
For example, you might choose a case study that reveals an unusual or neglected aspect of your research problem, or you might choose several very similar or very different cases in order to compare them.
Data collection methods are ways of directly measuring variables and gathering information. They allow you to gain first-hand knowledge and original insights into your research problem.
You can choose just one data collection method, or use several methods in the same study.
Survey methods
Surveys allow you to collect data about opinions, behaviors, experiences, and characteristics by asking people directly. There are two main survey methods to choose from: questionnaires and interviews .
Questionnaires | Interviews |
---|---|
) |
Observation methods
Observational studies allow you to collect data unobtrusively, observing characteristics, behaviors or social interactions without relying on self-reporting.
Observations may be conducted in real time, taking notes as you observe, or you might make audiovisual recordings for later analysis. They can be qualitative or quantitative.
Quantitative observation | |
---|---|
Other methods of data collection
There are many other ways you might collect data depending on your field and topic.
Field | Examples of data collection methods |
---|---|
Media & communication | Collecting a sample of texts (e.g., speeches, articles, or social media posts) for data on cultural norms and narratives |
Psychology | Using technologies like neuroimaging, eye-tracking, or computer-based tasks to collect data on things like attention, emotional response, or reaction time |
Education | Using tests or assignments to collect data on knowledge and skills |
Physical sciences | Using scientific instruments to collect data on things like weight, blood pressure, or chemical composition |
If you’re not sure which methods will work best for your research design, try reading some papers in your field to see what kinds of data collection methods they used.
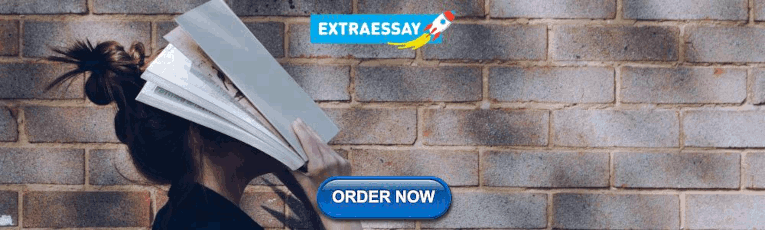
Secondary data
If you don’t have the time or resources to collect data from the population you’re interested in, you can also choose to use secondary data that other researchers already collected—for example, datasets from government surveys or previous studies on your topic.
With this raw data, you can do your own analysis to answer new research questions that weren’t addressed by the original study.
Using secondary data can expand the scope of your research, as you may be able to access much larger and more varied samples than you could collect yourself.
However, it also means you don’t have any control over which variables to measure or how to measure them, so the conclusions you can draw may be limited.
Here's why students love Scribbr's proofreading services
Discover proofreading & editing
As well as deciding on your methods, you need to plan exactly how you’ll use these methods to collect data that’s consistent, accurate, and unbiased.
Planning systematic procedures is especially important in quantitative research, where you need to precisely define your variables and ensure your measurements are high in reliability and validity.
Operationalization
Some variables, like height or age, are easily measured. But often you’ll be dealing with more abstract concepts, like satisfaction, anxiety, or competence. Operationalization means turning these fuzzy ideas into measurable indicators.
If you’re using observations , which events or actions will you count?
If you’re using surveys , which questions will you ask and what range of responses will be offered?
You may also choose to use or adapt existing materials designed to measure the concept you’re interested in—for example, questionnaires or inventories whose reliability and validity has already been established.
Reliability and validity
Reliability means your results can be consistently reproduced, while validity means that you’re actually measuring the concept you’re interested in.
Reliability | Validity |
---|---|
) ) |
For valid and reliable results, your measurement materials should be thoroughly researched and carefully designed. Plan your procedures to make sure you carry out the same steps in the same way for each participant.
If you’re developing a new questionnaire or other instrument to measure a specific concept, running a pilot study allows you to check its validity and reliability in advance.
Sampling procedures
As well as choosing an appropriate sampling method , you need a concrete plan for how you’ll actually contact and recruit your selected sample.
That means making decisions about things like:
- How many participants do you need for an adequate sample size?
- What inclusion and exclusion criteria will you use to identify eligible participants?
- How will you contact your sample—by mail, online, by phone, or in person?
If you’re using a probability sampling method , it’s important that everyone who is randomly selected actually participates in the study. How will you ensure a high response rate?
If you’re using a non-probability method , how will you avoid research bias and ensure a representative sample?
Data management
It’s also important to create a data management plan for organizing and storing your data.
Will you need to transcribe interviews or perform data entry for observations? You should anonymize and safeguard any sensitive data, and make sure it’s backed up regularly.
Keeping your data well-organized will save time when it comes to analyzing it. It can also help other researchers validate and add to your findings (high replicability ).
On its own, raw data can’t answer your research question. The last step of designing your research is planning how you’ll analyze the data.
Quantitative data analysis
In quantitative research, you’ll most likely use some form of statistical analysis . With statistics, you can summarize your sample data, make estimates, and test hypotheses.
Using descriptive statistics , you can summarize your sample data in terms of:
- The distribution of the data (e.g., the frequency of each score on a test)
- The central tendency of the data (e.g., the mean to describe the average score)
- The variability of the data (e.g., the standard deviation to describe how spread out the scores are)
The specific calculations you can do depend on the level of measurement of your variables.
Using inferential statistics , you can:
- Make estimates about the population based on your sample data.
- Test hypotheses about a relationship between variables.
Regression and correlation tests look for associations between two or more variables, while comparison tests (such as t tests and ANOVAs ) look for differences in the outcomes of different groups.
Your choice of statistical test depends on various aspects of your research design, including the types of variables you’re dealing with and the distribution of your data.
Qualitative data analysis
In qualitative research, your data will usually be very dense with information and ideas. Instead of summing it up in numbers, you’ll need to comb through the data in detail, interpret its meanings, identify patterns, and extract the parts that are most relevant to your research question.
Two of the most common approaches to doing this are thematic analysis and discourse analysis .
Approach | Characteristics |
---|---|
Thematic analysis | |
Discourse analysis |
There are many other ways of analyzing qualitative data depending on the aims of your research. To get a sense of potential approaches, try reading some qualitative research papers in your field.
If you want to know more about the research process , methodology , research bias , or statistics , make sure to check out some of our other articles with explanations and examples.
- Simple random sampling
- Stratified sampling
- Cluster sampling
- Likert scales
- Reproducibility
Statistics
- Null hypothesis
- Statistical power
- Probability distribution
- Effect size
- Poisson distribution
Research bias
- Optimism bias
- Cognitive bias
- Implicit bias
- Hawthorne effect
- Anchoring bias
- Explicit bias
A research design is a strategy for answering your research question . It defines your overall approach and determines how you will collect and analyze data.
A well-planned research design helps ensure that your methods match your research aims, that you collect high-quality data, and that you use the right kind of analysis to answer your questions, utilizing credible sources . This allows you to draw valid , trustworthy conclusions.
Quantitative research designs can be divided into two main categories:
- Correlational and descriptive designs are used to investigate characteristics, averages, trends, and associations between variables.
- Experimental and quasi-experimental designs are used to test causal relationships .
Qualitative research designs tend to be more flexible. Common types of qualitative design include case study , ethnography , and grounded theory designs.
The priorities of a research design can vary depending on the field, but you usually have to specify:
- Your research questions and/or hypotheses
- Your overall approach (e.g., qualitative or quantitative )
- The type of design you’re using (e.g., a survey , experiment , or case study )
- Your data collection methods (e.g., questionnaires , observations)
- Your data collection procedures (e.g., operationalization , timing and data management)
- Your data analysis methods (e.g., statistical tests or thematic analysis )
A sample is a subset of individuals from a larger population . Sampling means selecting the group that you will actually collect data from in your research. For example, if you are researching the opinions of students in your university, you could survey a sample of 100 students.
In statistics, sampling allows you to test a hypothesis about the characteristics of a population.
Operationalization means turning abstract conceptual ideas into measurable observations.
For example, the concept of social anxiety isn’t directly observable, but it can be operationally defined in terms of self-rating scores, behavioral avoidance of crowded places, or physical anxiety symptoms in social situations.
Before collecting data , it’s important to consider how you will operationalize the variables that you want to measure.
A research project is an academic, scientific, or professional undertaking to answer a research question . Research projects can take many forms, such as qualitative or quantitative , descriptive , longitudinal , experimental , or correlational . What kind of research approach you choose will depend on your topic.
Cite this Scribbr article
If you want to cite this source, you can copy and paste the citation or click the “Cite this Scribbr article” button to automatically add the citation to our free Citation Generator.
McCombes, S. (2023, November 20). What Is a Research Design | Types, Guide & Examples. Scribbr. Retrieved August 12, 2024, from https://www.scribbr.com/methodology/research-design/
Is this article helpful?
Shona McCombes
Other students also liked, guide to experimental design | overview, steps, & examples, how to write a research proposal | examples & templates, ethical considerations in research | types & examples, what is your plagiarism score.
Statistical Design and Analysis of Biological Experiments
Chapter 1 principles of experimental design, 1.1 introduction.
The validity of conclusions drawn from a statistical analysis crucially hinges on the manner in which the data are acquired, and even the most sophisticated analysis will not rescue a flawed experiment. Planning an experiment and thinking about the details of data acquisition is so important for a successful analysis that R. A. Fisher—who single-handedly invented many of the experimental design techniques we are about to discuss—famously wrote
To call in the statistician after the experiment is done may be no more than asking him to perform a post-mortem examination: he may be able to say what the experiment died of. ( Fisher 1938 )
(Statistical) design of experiments provides the principles and methods for planning experiments and tailoring the data acquisition to an intended analysis. Design and analysis of an experiment are best considered as two aspects of the same enterprise: the goals of the analysis strongly inform an appropriate design, and the implemented design determines the possible analyses.
The primary aim of designing experiments is to ensure that valid statistical and scientific conclusions can be drawn that withstand the scrutiny of a determined skeptic. Good experimental design also considers that resources are used efficiently, and that estimates are sufficiently precise and hypothesis tests adequately powered. It protects our conclusions by excluding alternative interpretations or rendering them implausible. Three main pillars of experimental design are randomization , replication , and blocking , and we will flesh out their effects on the subsequent analysis as well as their implementation in an experimental design.
An experimental design is always tailored towards predefined (primary) analyses and an efficient analysis and unambiguous interpretation of the experimental data is often straightforward from a good design. This does not prevent us from doing additional analyses of interesting observations after the data are acquired, but these analyses can be subjected to more severe criticisms and conclusions are more tentative.
In this chapter, we provide the wider context for using experiments in a larger research enterprise and informally introduce the main statistical ideas of experimental design. We use a comparison of two samples as our main example to study how design choices affect an analysis, but postpone a formal quantitative analysis to the next chapters.
1.2 A Cautionary Tale
For illustrating some of the issues arising in the interplay of experimental design and analysis, we consider a simple example. We are interested in comparing the enzyme levels measured in processed blood samples from laboratory mice, when the sample processing is done either with a kit from a vendor A, or a kit from a competitor B. For this, we take 20 mice and randomly select 10 of them for sample preparation with kit A, while the blood samples of the remaining 10 mice are prepared with kit B. The experiment is illustrated in Figure 1.1 A and the resulting data are given in Table 1.1 .
A | 8.96 | 8.95 | 11.37 | 12.63 | 11.38 | 8.36 | 6.87 | 12.35 | 10.32 | 11.99 |
B | 12.68 | 11.37 | 12.00 | 9.81 | 10.35 | 11.76 | 9.01 | 10.83 | 8.76 | 9.99 |
One option for comparing the two kits is to look at the difference in average enzyme levels, and we find an average level of 10.32 for vendor A and 10.66 for vendor B. We would like to interpret their difference of -0.34 as the difference due to the two preparation kits and conclude whether the two kits give equal results or if measurements based on one kit are systematically different from those based on the other kit.
Such interpretation, however, is only valid if the two groups of mice and their measurements are identical in all aspects except the sample preparation kit. If we use one strain of mice for kit A and another strain for kit B, any difference might also be attributed to inherent differences between the strains. Similarly, if the measurements using kit B were conducted much later than those using kit A, any observed difference might be attributed to changes in, e.g., mice selected, batches of chemicals used, device calibration, or any number of other influences. None of these competing explanations for an observed difference can be excluded from the given data alone, but good experimental design allows us to render them (almost) arbitrarily implausible.
A second aspect for our analysis is the inherent uncertainty in our calculated difference: if we repeat the experiment, the observed difference will change each time, and this will be more pronounced for a smaller number of mice, among others. If we do not use a sufficient number of mice in our experiment, the uncertainty associated with the observed difference might be too large, such that random fluctuations become a plausible explanation for the observed difference. Systematic differences between the two kits, of practically relevant magnitude in either direction, might then be compatible with the data, and we can draw no reliable conclusions from our experiment.
In each case, the statistical analysis—no matter how clever—was doomed before the experiment was even started, while simple ideas from statistical design of experiments would have provided correct and robust results with interpretable conclusions.
1.3 The Language of Experimental Design
By an experiment we understand an investigation where the researcher has full control over selecting and altering the experimental conditions of interest, and we only consider investigations of this type. The selected experimental conditions are called treatments . An experiment is comparative if the responses to several treatments are to be compared or contrasted. The experimental units are the smallest subdivision of the experimental material to which a treatment can be assigned. All experimental units given the same treatment constitute a treatment group . Especially in biology, we often compare treatments to a control group to which some standard experimental conditions are applied; a typical example is using a placebo for the control group, and different drugs for the other treatment groups.
The values observed are called responses and are measured on the response units ; these are often identical to the experimental units but need not be. Multiple experimental units are sometimes combined into groupings or blocks , such as mice grouped by litter, or samples grouped by batches of chemicals used for their preparation. More generally, we call any grouping of the experimental material (even with group size one) a unit .
In our example, we selected the mice, used a single sample per mouse, deliberately chose the two specific vendors, and had full control over which kit to assign to which mouse. In other words, the two kits are the treatments and the mice are the experimental units. We took the measured enzyme level of a single sample from a mouse as our response, and samples are therefore the response units. The resulting experiment is comparative, because we contrast the enzyme levels between the two treatment groups.

Figure 1.1: Three designs to determine the difference between two preparation kits A and B based on four mice. A: One sample per mouse. Comparison between averages of samples with same kit. B: Two samples per mouse treated with the same kit. Comparison between averages of mice with same kit requires averaging responses for each mouse first. C: Two samples per mouse each treated with different kit. Comparison between two samples of each mouse, with differences averaged.
In this example, we can coalesce experimental and response units, because we have a single response per mouse and cannot distinguish a sample from a mouse in the analysis, as illustrated in Figure 1.1 A for four mice. Responses from mice with the same kit are averaged, and the kit difference is the difference between these two averages.
By contrast, if we take two samples per mouse and use the same kit for both samples, then the mice are still the experimental units, but each mouse now groups the two response units associated with it. Now, responses from the same mouse are first averaged, and these averages are used to calculate the difference between kits; even though eight measurements are available, this difference is still based on only four mice (Figure 1.1 B).
If we take two samples per mouse, but apply each kit to one of the two samples, then the samples are both the experimental and response units, while the mice are blocks that group the samples. Now, we calculate the difference between kits for each mouse, and then average these differences (Figure 1.1 C).
If we only use one kit and determine the average enzyme level, then this investigation is still an experiment, but is not comparative.
To summarize, the design of an experiment determines the logical structure of the experiment ; it consists of (i) a set of treatments (the two kits); (ii) a specification of the experimental units (animals, cell lines, samples) (the mice in Figure 1.1 A,B and the samples in Figure 1.1 C); (iii) a procedure for assigning treatments to units; and (iv) a specification of the response units and the quantity to be measured as a response (the samples and associated enzyme levels).
1.4 Experiment Validity
Before we embark on the more technical aspects of experimental design, we discuss three components for evaluating an experiment’s validity: construct validity , internal validity , and external validity . These criteria are well-established in areas such as educational and psychological research, and have more recently been discussed for animal research ( Würbel 2017 ) where experiments are increasingly scrutinized for their scientific rationale and their design and intended analyses.
1.4.1 Construct Validity
Construct validity concerns the choice of the experimental system for answering our research question. Is the system even capable of providing a relevant answer to the question?
Studying the mechanisms of a particular disease, for example, might require careful choice of an appropriate animal model that shows a disease phenotype and is accessible to experimental interventions. If the animal model is a proxy for drug development for humans, biological mechanisms must be sufficiently similar between animal and human physiologies.
Another important aspect of the construct is the quantity that we intend to measure (the measurand ), and its relation to the quantity or property we are interested in. For example, we might measure the concentration of the same chemical compound once in a blood sample and once in a highly purified sample, and these constitute two different measurands, whose values might not be comparable. Often, the quantity of interest (e.g., liver function) is not directly measurable (or even quantifiable) and we measure a biomarker instead. For example, pre-clinical and clinical investigations may use concentrations of proteins or counts of specific cell types from blood samples, such as the CD4+ cell count used as a biomarker for immune system function.
1.4.2 Internal Validity
The internal validity of an experiment concerns the soundness of the scientific rationale, statistical properties such as precision of estimates, and the measures taken against risk of bias. It refers to the validity of claims within the context of the experiment. Statistical design of experiments plays a prominent role in ensuring internal validity, and we briefly discuss the main ideas before providing the technical details and an application to our example in the subsequent sections.
Scientific Rationale and Research Question
The scientific rationale of a study is (usually) not immediately a statistical question. Translating a scientific question into a quantitative comparison amenable to statistical analysis is no small task and often requires careful consideration. It is a substantial, if non-statistical, benefit of using experimental design that we are forced to formulate a precise-enough research question and decide on the main analyses required for answering it before we conduct the experiment. For example, the question: is there a difference between placebo and drug? is insufficiently precise for planning a statistical analysis and determine an adequate experimental design. What exactly is the drug treatment? What should the drug’s concentration be and how is it administered? How do we make sure that the placebo group is comparable to the drug group in all other aspects? What do we measure and what do we mean by “difference?” A shift in average response, a fold-change, change in response before and after treatment?
The scientific rationale also enters the choice of a potential control group to which we compare responses. The quote
The deep, fundamental question in statistical analysis is ‘Compared to what?’ ( Tufte 1997 )
highlights the importance of this choice.
There are almost never enough resources to answer all relevant scientific questions. We therefore define a few questions of highest interest, and the main purpose of the experiment is answering these questions in the primary analysis . This intended analysis drives the experimental design to ensure relevant estimates can be calculated and have sufficient precision, and tests are adequately powered. This does not preclude us from conducting additional secondary analyses and exploratory analyses , but we are not willing to enlarge the experiment to ensure that strong conclusions can also be drawn from these analyses.
Risk of Bias
Experimental bias is a systematic difference in response between experimental units in addition to the difference caused by the treatments. The experimental units in the different groups are then not equal in all aspects other than the treatment applied to them. We saw several examples in Section 1.2 .
Minimizing the risk of bias is crucial for internal validity and we look at some common measures to eliminate or reduce different types of bias in Section 1.5 .
Precision and Effect Size
Another aspect of internal validity is the precision of estimates and the expected effect sizes. Is the experimental setup, in principle, able to detect a difference of relevant magnitude? Experimental design offers several methods for answering this question based on the expected heterogeneity of samples, the measurement error, and other sources of variation: power analysis is a technique for determining the number of samples required to reliably detect a relevant effect size and provide estimates of sufficient precision. More samples yield more precision and more power, but we have to be careful that replication is done at the right level: simply measuring a biological sample multiple times as in Figure 1.1 B yields more measured values, but is pseudo-replication for analyses. Replication should also ensure that the statistical uncertainties of estimates can be gauged from the data of the experiment itself, without additional untestable assumptions. Finally, the technique of blocking , shown in Figure 1.1 C, can remove a substantial proportion of the variation and thereby increase power and precision if we find a way to apply it.
1.4.3 External Validity
The external validity of an experiment concerns its replicability and the generalizability of inferences. An experiment is replicable if its results can be confirmed by an independent new experiment, preferably by a different lab and researcher. Experimental conditions in the replicate experiment usually differ from the original experiment, which provides evidence that the observed effects are robust to such changes. A much weaker condition on an experiment is reproducibility , the property that an independent researcher draws equivalent conclusions based on the data from this particular experiment, using the same analysis techniques. Reproducibility requires publishing the raw data, details on the experimental protocol, and a description of the statistical analyses, preferably with accompanying source code. Many scientific journals subscribe to reporting guidelines to ensure reproducibility and these are also helpful for planning an experiment.
A main threat to replicability and generalizability are too tightly controlled experimental conditions, when inferences only hold for a specific lab under the very specific conditions of the original experiment. Introducing systematic heterogeneity and using multi-center studies effectively broadens the experimental conditions and therefore the inferences for which internal validity is available.
For systematic heterogeneity , experimental conditions are systematically altered in addition to the treatments, and treatment differences estimated for each condition. For example, we might split the experimental material into several batches and use a different day of analysis, sample preparation, batch of buffer, measurement device, and lab technician for each batch. A more general inference is then possible if effect size, effect direction, and precision are comparable between the batches, indicating that the treatment differences are stable over the different conditions.
In multi-center experiments , the same experiment is conducted in several different labs and the results compared and merged. Multi-center approaches are very common in clinical trials and often necessary to reach the required number of patient enrollments.
Generalizability of randomized controlled trials in medicine and animal studies can suffer from overly restrictive eligibility criteria. In clinical trials, patients are often included or excluded based on co-medications and co-morbidities, and the resulting sample of eligible patients might no longer be representative of the patient population. For example, Travers et al. ( 2007 ) used the eligibility criteria of 17 random controlled trials of asthma treatments and found that out of 749 patients, only a median of 6% (45 patients) would be eligible for an asthma-related randomized controlled trial. This puts a question mark on the relevance of the trials’ findings for asthma patients in general.
1.5 Reducing the Risk of Bias
1.5.1 randomization of treatment allocation.
If systematic differences other than the treatment exist between our treatment groups, then the effect of the treatment is confounded with these other differences and our estimates of treatment effects might be biased.
We remove such unwanted systematic differences from our treatment comparisons by randomizing the allocation of treatments to experimental units. In a completely randomized design , each experimental unit has the same chance of being subjected to any of the treatments, and any differences between the experimental units other than the treatments are distributed over the treatment groups. Importantly, randomization is the only method that also protects our experiment against unknown sources of bias: we do not need to know all or even any of the potential differences and yet their impact is eliminated from the treatment comparisons by random treatment allocation.
Randomization has two effects: (i) differences unrelated to treatment become part of the ‘statistical noise’ rendering the treatment groups more similar; and (ii) the systematic differences are thereby eliminated as sources of bias from the treatment comparison.
Randomization transforms systematic variation into random variation.
In our example, a proper randomization would select 10 out of our 20 mice fully at random, such that the probability of any one mouse being picked is 1/20. These ten mice are then assigned to kit A, and the remaining mice to kit B. This allocation is entirely independent of the treatments and of any properties of the mice.
To ensure random treatment allocation, some kind of random process needs to be employed. This can be as simple as shuffling a pack of 10 red and 10 black cards or using a software-based random number generator. Randomization is slightly more difficult if the number of experimental units is not known at the start of the experiment, such as when patients are recruited for an ongoing clinical trial (sometimes called rolling recruitment ), and we want to have reasonable balance between the treatment groups at each stage of the trial.
Seemingly random assignments “by hand” are usually no less complicated than fully random assignments, but are always inferior. If surprising results ensue from the experiment, such assignments are subject to unanswerable criticism and suspicion of unwanted bias. Even worse are systematic allocations; they can only remove bias from known causes, and immediately raise red flags under the slightest scrutiny.
The Problem of Undesired Assignments
Even with a fully random treatment allocation procedure, we might end up with an undesirable allocation. For our example, the treatment group of kit A might—just by chance—contain mice that are all bigger or more active than those in the other treatment group. Statistical orthodoxy recommends using the design nevertheless, because only full randomization guarantees valid estimates of residual variance and unbiased estimates of effects. This argument, however, concerns the long-run properties of the procedure and seems of little help in this specific situation. Why should we care if the randomization yields correct estimates under replication of the experiment, if the particular experiment is jeopardized?
Another solution is to create a list of all possible allocations that we would accept and randomly choose one of these allocations for our experiment. The analysis should then reflect this restriction in the possible randomizations, which often renders this approach difficult to implement.
The most pragmatic method is to reject highly undesirable designs and compute a new randomization ( Cox 1958 ) . Undesirable allocations are unlikely to arise for large sample sizes, and we might accept a small bias in estimation for small sample sizes, when uncertainty in the estimated treatment effect is already high. In this approach, whenever we reject a particular outcome, we must also be willing to reject the outcome if we permute the treatment level labels. If we reject eight big and two small mice for kit A, then we must also reject two big and eight small mice. We must also be transparent and report a rejected allocation, so that critics may come to their own conclusions about potential biases and their remedies.
1.5.2 Blinding
Bias in treatment comparisons is also introduced if treatment allocation is random, but responses cannot be measured entirely objectively, or if knowledge of the assigned treatment affects the response. In clinical trials, for example, patients might react differently when they know to be on a placebo treatment, an effect known as cognitive bias . In animal experiments, caretakers might report more abnormal behavior for animals on a more severe treatment. Cognitive bias can be eliminated by concealing the treatment allocation from technicians or participants of a clinical trial, a technique called single-blinding .
If response measures are partially based on professional judgement (such as a clinical scale), patient or physician might unconsciously report lower scores for a placebo treatment, a phenomenon known as observer bias . Its removal requires double blinding , where treatment allocations are additionally concealed from the experimentalist.
Blinding requires randomized treatment allocation to begin with and substantial effort might be needed to implement it. Drug companies, for example, have to go to great lengths to ensure that a placebo looks, tastes, and feels similar enough to the actual drug. Additionally, blinding is often done by coding the treatment conditions and samples, and effect sizes and statistical significance are calculated before the code is revealed.
In clinical trials, double-blinding creates a conflict of interest. The attending physicians do not know which patient received which treatment, and thus accumulation of side-effects cannot be linked to any treatment. For this reason, clinical trials have a data monitoring committee not involved in the final analysis, that performs intermediate analyses of efficacy and safety at predefined intervals. If severe problems are detected, the committee might recommend altering or aborting the trial. The same might happen if one treatment already shows overwhelming evidence of superiority, such that it becomes unethical to withhold this treatment from the other patients.
1.5.3 Analysis Plan and Registration
An often overlooked source of bias has been termed the researcher degrees of freedom or garden of forking paths in the data analysis. For any set of data, there are many different options for its analysis: some results might be considered outliers and discarded, assumptions are made on error distributions and appropriate test statistics, different covariates might be included into a regression model. Often, multiple hypotheses are investigated and tested, and analyses are done separately on various (overlapping) subgroups. Hypotheses formed after looking at the data require additional care in their interpretation; almost never will \(p\) -values for these ad hoc or post hoc hypotheses be statistically justifiable. Many different measured response variables invite fishing expeditions , where patterns in the data are sought without an underlying hypothesis. Only reporting those sub-analyses that gave ‘interesting’ findings invariably leads to biased conclusions and is called cherry-picking or \(p\) -hacking (or much less flattering names).
The statistical analysis is always part of a larger scientific argument and we should consider the necessary computations in relation to building our scientific argument about the interpretation of the data. In addition to the statistical calculations, this interpretation requires substantial subject-matter knowledge and includes (many) non-statistical arguments. Two quotes highlight that experiment and analysis are a means to an end and not the end in itself.
There is a boundary in data interpretation beyond which formulas and quantitative decision procedures do not go, where judgment and style enter. ( Abelson 1995 )
Often, perfectly reasonable people come to perfectly reasonable decisions or conclusions based on nonstatistical evidence. Statistical analysis is a tool with which we support reasoning. It is not a goal in itself. ( Bailar III 1981 )
There is often a grey area between exploiting researcher degrees of freedom to arrive at a desired conclusion, and creative yet informed analyses of data. One way to navigate this area is to distinguish between exploratory studies and confirmatory studies . The former have no clearly stated scientific question, but are used to generate interesting hypotheses by identifying potential associations or effects that are then further investigated. Conclusions from these studies are very tentative and must be reported honestly as such. In contrast, standards are much higher for confirmatory studies, which investigate a specific predefined scientific question. Analysis plans and pre-registration of an experiment are accepted means for demonstrating lack of bias due to researcher degrees of freedom, and separating primary from secondary analyses allows emphasizing the main goals of the study.
Analysis Plan
The analysis plan is written before conducting the experiment and details the measurands and estimands, the hypotheses to be tested together with a power and sample size calculation, a discussion of relevant effect sizes, detection and handling of outliers and missing data, as well as steps for data normalization such as transformations and baseline corrections. If a regression model is required, its factors and covariates are outlined. Particularly in biology, handling measurements below the limit of quantification and saturation effects require careful consideration.
In the context of clinical trials, the problem of estimands has become a recent focus of attention. An estimand is the target of a statistical estimation procedure, for example the true average difference in enzyme levels between the two preparation kits. A main problem in many studies are post-randomization events that can change the estimand, even if the estimation procedure remains the same. For example, if kit B fails to produce usable samples for measurement in five out of ten cases because the enzyme level was too low, while kit A could handle these enzyme levels perfectly fine, then this might severely exaggerate the observed difference between the two kits. Similar problems arise in drug trials, when some patients stop taking one of the drugs due to side-effects or other complications.
Registration
Registration of experiments is an even more severe measure used in conjunction with an analysis plan and is becoming standard in clinical trials. Here, information about the trial, including the analysis plan, procedure to recruit patients, and stopping criteria, are registered in a public database. Publications based on the trial then refer to this registration, such that reviewers and readers can compare what the researchers intended to do and what they actually did. Similar portals for pre-clinical and translational research are also available.
1.6 Notes and Summary
The problem of measurements and measurands is further discussed for statistics in Hand ( 1996 ) and specifically for biological experiments in Coxon, Longstaff, and Burns ( 2019 ) . A general review of methods for handling missing data is Dong and Peng ( 2013 ) . The different roles of randomization are emphasized in Cox ( 2009 ) .
Two well-known reporting guidelines are the ARRIVE guidelines for animal research ( Kilkenny et al. 2010 ) and the CONSORT guidelines for clinical trials ( Moher et al. 2010 ) . Guidelines describing the minimal information required for reproducing experimental results have been developed for many types of experimental techniques, including microarrays (MIAME), RNA sequencing (MINSEQE), metabolomics (MSI) and proteomics (MIAPE) experiments; the FAIRSHARE initiative provides a more comprehensive collection ( Sansone et al. 2019 ) .
The problems of experimental design in animal experiments and particularly translation research are discussed in Couzin-Frankel ( 2013 ) . Multi-center studies are now considered for these investigations, and using a second laboratory already increases reproducibility substantially ( Richter et al. 2010 ; Richter 2017 ; Voelkl et al. 2018 ; Karp 2018 ) and allows standardizing the treatment effects ( Kafkafi et al. 2017 ) . First attempts are reported of using designs similar to clinical trials ( Llovera and Liesz 2016 ) . Exploratory-confirmatory research and external validity for animal studies is discussed in Kimmelman, Mogil, and Dirnagl ( 2014 ) and Pound and Ritskes-Hoitinga ( 2018 ) . Further information on pilot studies is found in Moore et al. ( 2011 ) , Sim ( 2019 ) , and Thabane et al. ( 2010 ) .
The deliberate use of statistical analyses and their interpretation for supporting a larger argument was called statistics as principled argument ( Abelson 1995 ) . Employing useless statistical analysis without reference to the actual scientific question is surrogate science ( Gigerenzer and Marewski 2014 ) and adaptive thinking is integral to meaningful statistical analysis ( Gigerenzer 2002 ) .
In an experiment, the investigator has full control over the experimental conditions applied to the experiment material. The experimental design gives the logical structure of an experiment: the units describing the organization of the experimental material, the treatments and their allocation to units, and the response. Statistical design of experiments includes techniques to ensure internal validity of an experiment, and methods to make inference from experimental data efficient.
- Scroll to top
- Light Dark Light Dark
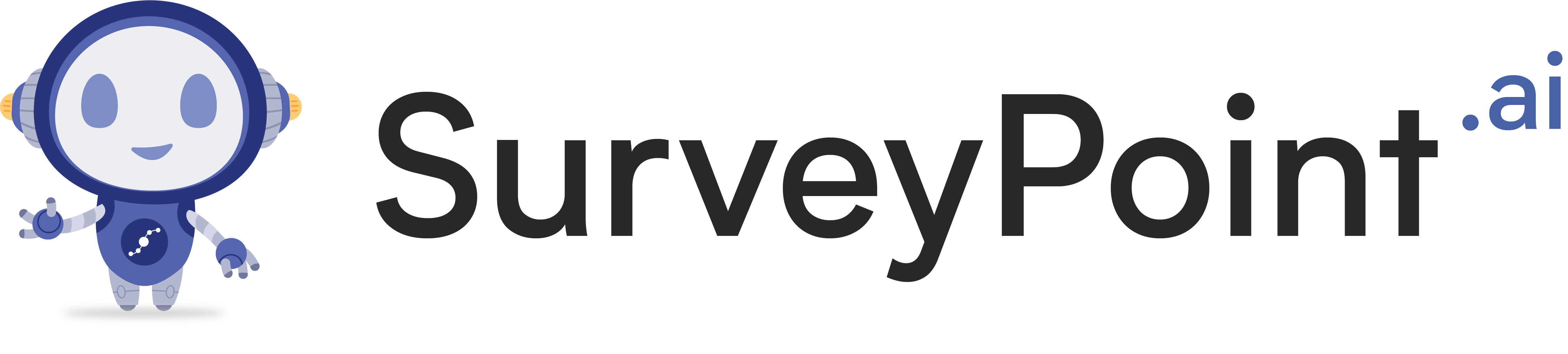
Cart review
No products in the cart.
7 Powerful Steps in Sampling Design for Effective Research
- Author Survey Point Team
- Published January 3, 2024
Unlock the secrets of effective research with these 7 powerful steps in sampling design. Elevate your research game and ensure precision from the outset. Dive into a world of insights and methodology that guarantees meaningful results.
Research forms the foundation of knowledge and understanding in any field. The quality and validity of research depend largely on the sampling design used. An effective sampling design ensures unbiased and reliable results that can be generalized to the entire population. In this article, we will explore seven powerful steps in sampling design that researchers can follow to conduct effective research.
Table of Contents
1. Define the Research Objectives
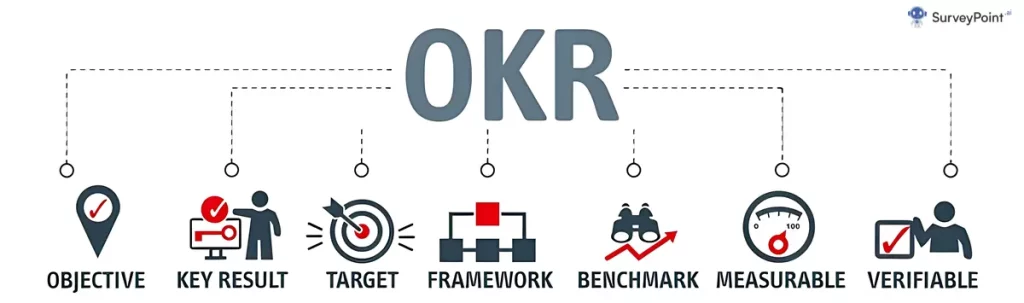
Before diving into the sampling design process, it is vital to define the research objectives. Clearly determining what you aim to achieve through the research will guide the entire sampling design. Whether it is to study consumer behavior, analyze market trends, or explore the impact of a specific intervention, outlining the research objectives provides a clear roadmap for sampling.
Example: Without a clear research objective, sampling becomes directionless, leading to inaccurate results that do not contribute to meaningful insights.
2. Identify the Target Population
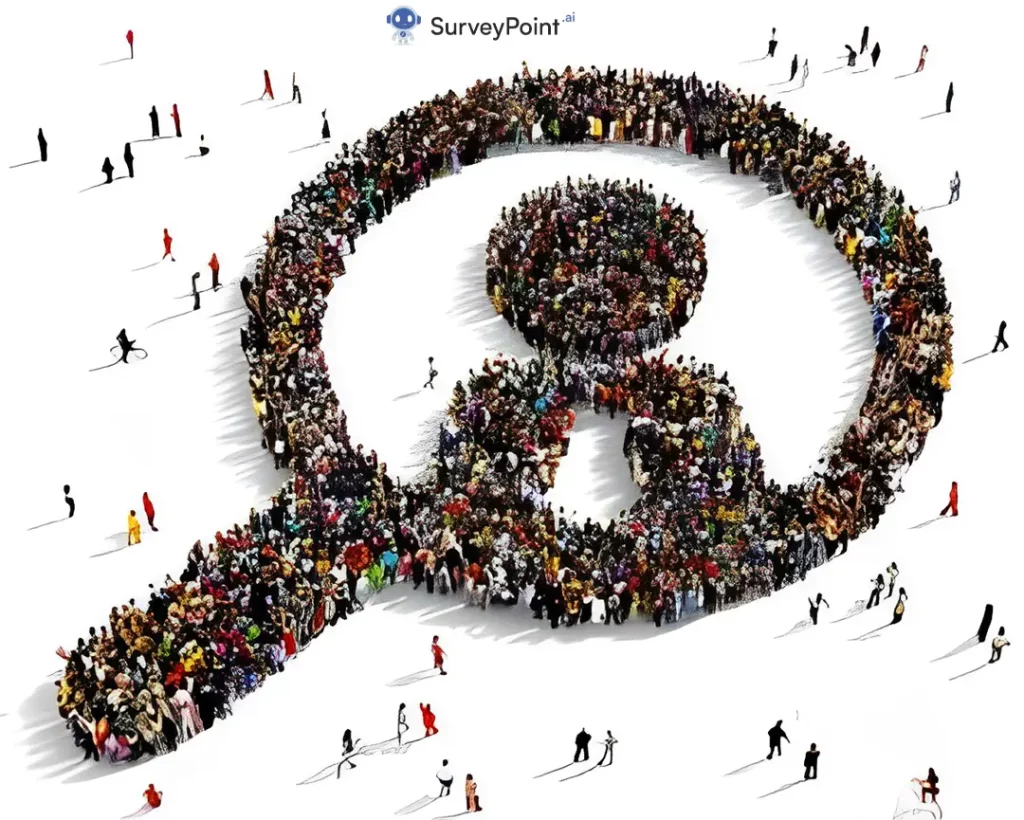
After defining the research objectives, identifying the target population is the next crucial step. The target population represents the group of individuals or elements that the research aims to generalize the findings to. It is essential to clearly define and understand the demographics, characteristics, and parameters of the target population before moving forward with sampling.
Example: Identifying the target population allows researchers to ensure that the sampled individuals represent the broader group accurately, increasing the external validity of the study.
3. Determine the Sample Size
Determining the appropriate sample size is a critical factor in sampling design. A sample size that is too small may not accurately represent the target population, while a sample size that is too large may result in unnecessary costs and resources. Determining the sample size requires considering various factors, such as desired level of precision, variability within the population, and available resources.
Example: The sample size should strike a balance between statistical reliability and practical feasibility. A larger sample size increases the precision of the estimates, while a smaller sample size may result in wider confidence intervals.
4. Select the Sampling Technique
Various sampling techniques exist, each catering to different research scenarios. The choice of sampling technique depends on the nature of the research, available resources, and the level of precision required. Common sampling techniques include simple random sampling, stratified sampling, cluster sampling, and systematic sampling.
Example: Understanding the different sampling techniques allows researchers to choose the most appropriate method for their specific research, ensuring representative and reliable results.
5. Implement the Sampling Strategy
Once the sampling technique is selected, it is time to implement the sampling strategy. This involves identifying the potential sampling units and selecting the actual sample elements from the target population. Researchers must avoid any biases and ensure randomness in the selection process to maintain the integrity of the research findings.
Example: Implementing the sampling strategy meticulously enables researchers to minimize potential biases and increase the chances of obtaining accurate results that can be generalized to the larger population.
6. Collect Data from the Sample: Steps in Sampling Design
With the sample selected, data collection becomes the next crucial step. Researchers can use various methods, such as surveys, interviews, observations, or experiments, to collect the necessary data. It is essential to follow the research design and consider data quality measures to ensure the reliability and validity of the collected information.
Example: Collecting data from the sample involves establishing effective communication channels, designing appropriate data collection instruments, and capturing the information accurately to minimize measurement errors.
7. Analyze and Interpret the Findings
Once the data is collected, it is time to analyze and interpret the findings. This involves applying statistical techniques, conducting hypothesis testing, and drawing meaningful conclusions. Researchers should ensure they have the necessary analytical skills or collaborate with experts in data analysis to derive accurate and insightful results.
Example: Analyzing and interpreting the findings allows researchers to draw meaningful conclusions and make informed decisions based on the evidence obtained through the research process.
Top 10 Sampling Techniques along with their respective Pros and Cons :
Simple Random Sampling | – Easy to implement | – May not represent specific subgroups adequately |
Stratified Sampling | – Ensures representation of subgroups | – Requires accurate classification of units |
Systematic Sampling | – Simple and easy to implement | – Susceptible to periodic patterns in the data |
Cluster Sampling | – Reduces costs and resources | – Potential for increased sampling error |
Convenience Sampling | – Quick and cost-effective | – Lack of representativeness |
Snowball Sampling | – Useful for hard-to-reach populations | – Potential for bias due to network connections |
Purposive Sampling | – Allows for targeted inclusion of specific cases | – Limited generalizability |
Quota Sampling | – Ensures representation of specific characteristics | – Potential for bias if quotas are not accurately defined |
Multi-Stage Sampling | – Allows for more complex and detailed studies | – Increased complexity and potential for errors |
Time-Location Sampling | – Useful for studying behaviors at specific times and locations | – Limited generalizability outside specified times and locations |
This table provides a quick overview of the strengths and weaknesses of each sampling technique, aiding researchers in selecting the most appropriate method for their specific research objectives.
Frequently Asked Questions (FAQs)
Q: Why is defining research objectives the first step in sampling design?
A: Defining research objectives sets a clear direction for the study, ensuring focus and purpose in the subsequent steps.
Q: How does the selection of a sampling frame impact research outcomes?
A: The sampling frame defines the accessible population, influencing the generalizability of results to the broader context.
Q: What factors influence the choice of a sampling technique?
A: Research objectives and the nature of the study guide the choice of a sampling technique, ensuring alignment with the research goals.
Q: Why is determining the sample size crucial in sampling design?
A: The sample size strikes a delicate balance, ensuring accuracy in representation while maintaining manageability.
Q: How do data collection methods align with the chosen sampling design?
A: The sampling design informs the selection of data collection methods, ensuring synergy for a comprehensive research approach.
Q: Why is analysis and interpretation the culmination of the sampling design process?
A: Analysis and interpretation transform raw data into actionable knowledge, realizing the objectives set at the beginning of the research journey.
Sampling design plays a fundamental role in conducting effective research. By following the seven powerful steps outlined in this article – defining research objectives, identifying the target population, determining the sample size, selecting the sampling technique, implementing the sampling strategy, collecting data from the sample, and analyzing and interpreting the findings – researchers can ensure reliable, valid, and generalizable results. Adopting a systematic and rigorous approach to sampling design will ultimately enhance the impact of research across various fields.
Remember, a solid sampling design empowers researchers to capture the essence of a larger population, revealing valuable insights that drive progress and innovation.
Survey Point Team
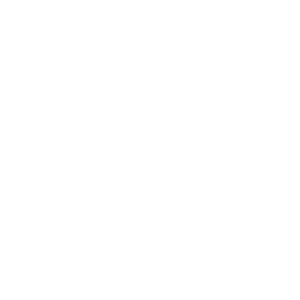
Sampling Methods In Reseach: Types, Techniques, & Examples
Saul McLeod, PhD
Editor-in-Chief for Simply Psychology
BSc (Hons) Psychology, MRes, PhD, University of Manchester
Saul McLeod, PhD., is a qualified psychology teacher with over 18 years of experience in further and higher education. He has been published in peer-reviewed journals, including the Journal of Clinical Psychology.
Learn about our Editorial Process
Olivia Guy-Evans, MSc
Associate Editor for Simply Psychology
BSc (Hons) Psychology, MSc Psychology of Education
Olivia Guy-Evans is a writer and associate editor for Simply Psychology. She has previously worked in healthcare and educational sectors.
On This Page:
Sampling methods in psychology refer to strategies used to select a subset of individuals (a sample) from a larger population, to study and draw inferences about the entire population. Common methods include random sampling, stratified sampling, cluster sampling, and convenience sampling. Proper sampling ensures representative, generalizable, and valid research results.
- Sampling : the process of selecting a representative group from the population under study.
- Target population : the total group of individuals from which the sample might be drawn.
- Sample: a subset of individuals selected from a larger population for study or investigation. Those included in the sample are termed “participants.”
- Generalizability : the ability to apply research findings from a sample to the broader target population, contingent on the sample being representative of that population.
For instance, if the advert for volunteers is published in the New York Times, this limits how much the study’s findings can be generalized to the whole population, because NYT readers may not represent the entire population in certain respects (e.g., politically, socio-economically).
The Purpose of Sampling
We are interested in learning about large groups of people with something in common in psychological research. We call the group interested in studying our “target population.”
In some types of research, the target population might be as broad as all humans. Still, in other types of research, the target population might be a smaller group, such as teenagers, preschool children, or people who misuse drugs.
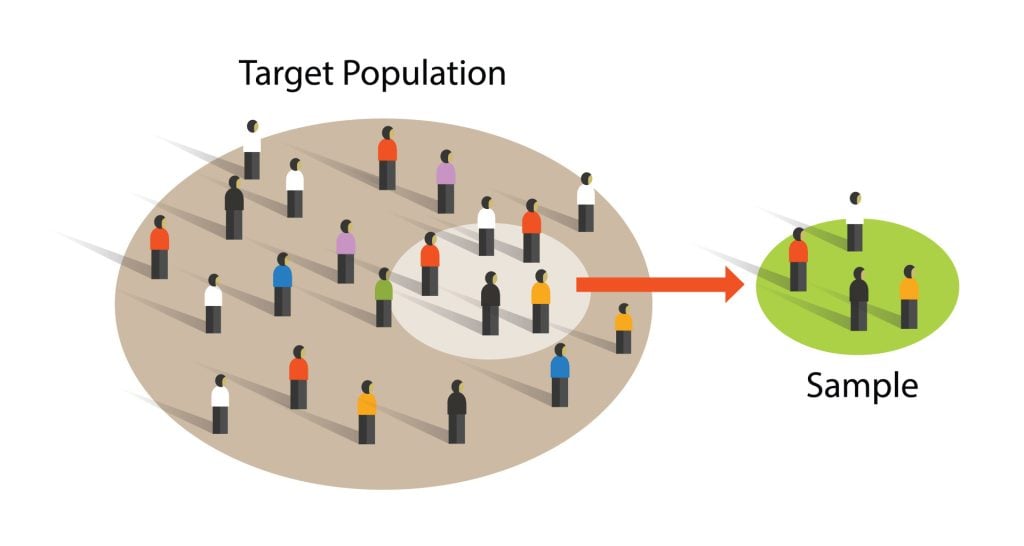
Studying every person in a target population is more or less impossible. Hence, psychologists select a sample or sub-group of the population that is likely to be representative of the target population we are interested in.
This is important because we want to generalize from the sample to the target population. The more representative the sample, the more confident the researcher can be that the results can be generalized to the target population.
One of the problems that can occur when selecting a sample from a target population is sampling bias. Sampling bias refers to situations where the sample does not reflect the characteristics of the target population.
Many psychology studies have a biased sample because they have used an opportunity sample that comprises university students as their participants (e.g., Asch ).
OK, so you’ve thought up this brilliant psychological study and designed it perfectly. But who will you try it out on, and how will you select your participants?
There are various sampling methods. The one chosen will depend on a number of factors (such as time, money, etc.).
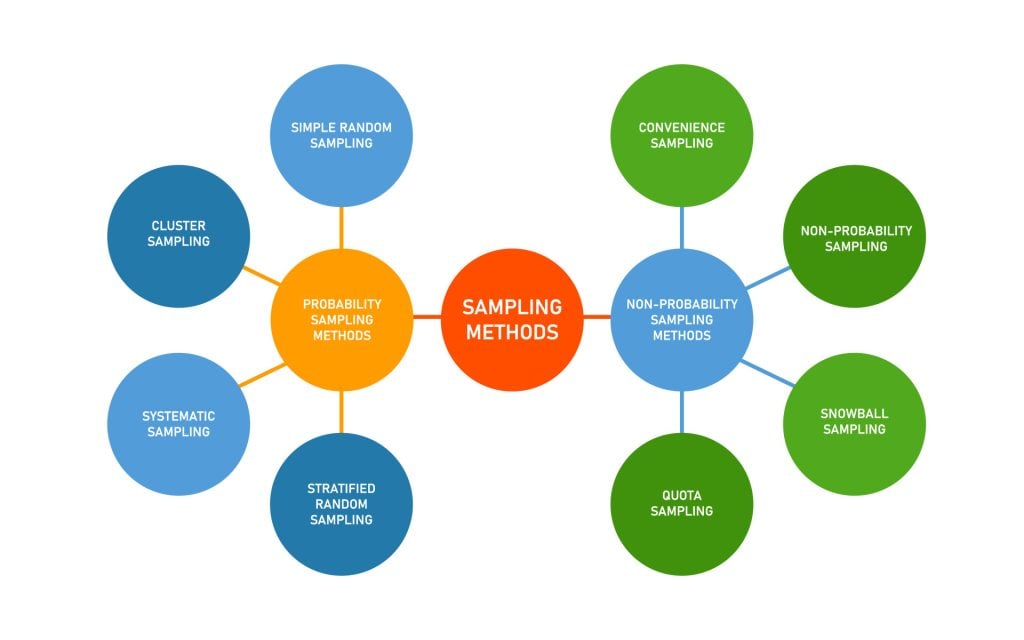
Random Sampling
Random sampling is a type of probability sampling where everyone in the entire target population has an equal chance of being selected.
This is similar to the national lottery. If the “population” is everyone who bought a lottery ticket, then everyone has an equal chance of winning the lottery (assuming they all have one ticket each).
Random samples require naming or numbering the target population and then using some raffle method to choose those to make up the sample. Random samples are the best method of selecting your sample from the population of interest.
- The advantages are that your sample should represent the target population and eliminate sampling bias.
- The disadvantage is that it is very difficult to achieve (i.e., time, effort, and money).
Stratified Sampling
During stratified sampling , the researcher identifies the different types of people that make up the target population and works out the proportions needed for the sample to be representative.
A list is made of each variable (e.g., IQ, gender, etc.) that might have an effect on the research. For example, if we are interested in the money spent on books by undergraduates, then the main subject studied may be an important variable.
For example, students studying English Literature may spend more money on books than engineering students, so if we use a large percentage of English students or engineering students, our results will not be accurate.
We have to determine the relative percentage of each group at a university, e.g., Engineering 10%, Social Sciences 15%, English 20%, Sciences 25%, Languages 10%, Law 5%, and Medicine 15%. The sample must then contain all these groups in the same proportion as the target population (university students).
- The disadvantage of stratified sampling is that gathering such a sample would be extremely time-consuming and difficult to do. This method is rarely used in Psychology.
- However, the advantage is that the sample should be highly representative of the target population, and therefore we can generalize from the results obtained.
Opportunity Sampling
Opportunity sampling is a method in which participants are chosen based on their ease of availability and proximity to the researcher, rather than using random or systematic criteria. It’s a type of convenience sampling .
An opportunity sample is obtained by asking members of the population of interest if they would participate in your research. An example would be selecting a sample of students from those coming out of the library.
- This is a quick and easy way of choosing participants (advantage)
- It may not provide a representative sample and could be biased (disadvantage).
Systematic Sampling
Systematic sampling is a method where every nth individual is selected from a list or sequence to form a sample, ensuring even and regular intervals between chosen subjects.
Participants are systematically selected (i.e., orderly/logical) from the target population, like every nth participant on a list of names.
To take a systematic sample, you list all the population members and then decide upon a sample you would like. By dividing the number of people in the population by the number of people you want in your sample, you get a number we will call n.
If you take every nth name, you will get a systematic sample of the correct size. If, for example, you wanted to sample 150 children from a school of 1,500, you would take every 10th name.
- The advantage of this method is that it should provide a representative sample.
Sample size
The sample size is a critical factor in determining the reliability and validity of a study’s findings. While increasing the sample size can enhance the generalizability of results, it’s also essential to balance practical considerations, such as resource constraints and diminishing returns from ever-larger samples.
Reliability and Validity
Reliability refers to the consistency and reproducibility of research findings across different occasions, researchers, or instruments. A small sample size may lead to inconsistent results due to increased susceptibility to random error or the influence of outliers. In contrast, a larger sample minimizes these errors, promoting more reliable results.
Validity pertains to the accuracy and truthfulness of research findings. For a study to be valid, it should accurately measure what it intends to do. A small, unrepresentative sample can compromise external validity, meaning the results don’t generalize well to the larger population. A larger sample captures more variability, ensuring that specific subgroups or anomalies don’t overly influence results.
Practical Considerations
Resource Constraints : Larger samples demand more time, money, and resources. Data collection becomes more extensive, data analysis more complex, and logistics more challenging.
Diminishing Returns : While increasing the sample size generally leads to improved accuracy and precision, there’s a point where adding more participants yields only marginal benefits. For instance, going from 50 to 500 participants might significantly boost a study’s robustness, but jumping from 10,000 to 10,500 might not offer a comparable advantage, especially considering the added costs.

- Privacy Policy

Home » Sampling Methods – Types, Techniques and Examples
Sampling Methods – Types, Techniques and Examples
Table of Contents

Sampling refers to the process of selecting a subset of data from a larger population or dataset in order to analyze or make inferences about the whole population.
In other words, sampling involves taking a representative sample of data from a larger group or dataset in order to gain insights or draw conclusions about the entire group.
Sampling Methods
Sampling methods refer to the techniques used to select a subset of individuals or units from a larger population for the purpose of conducting statistical analysis or research.
Sampling is an essential part of the Research because it allows researchers to draw conclusions about a population without having to collect data from every member of that population, which can be time-consuming, expensive, or even impossible.
Types of Sampling Methods
Sampling can be broadly categorized into two main categories:
Probability Sampling
This type of sampling is based on the principles of random selection, and it involves selecting samples in a way that every member of the population has an equal chance of being included in the sample.. Probability sampling is commonly used in scientific research and statistical analysis, as it provides a representative sample that can be generalized to the larger population.
Type of Probability Sampling :
- Simple Random Sampling: In this method, every member of the population has an equal chance of being selected for the sample. This can be done using a random number generator or by drawing names out of a hat, for example.
- Systematic Sampling: In this method, the population is first divided into a list or sequence, and then every nth member is selected for the sample. For example, if every 10th person is selected from a list of 100 people, the sample would include 10 people.
- Stratified Sampling: In this method, the population is divided into subgroups or strata based on certain characteristics, and then a random sample is taken from each stratum. This is often used to ensure that the sample is representative of the population as a whole.
- Cluster Sampling: In this method, the population is divided into clusters or groups, and then a random sample of clusters is selected. Then, all members of the selected clusters are included in the sample.
- Multi-Stage Sampling : This method combines two or more sampling techniques. For example, a researcher may use stratified sampling to select clusters, and then use simple random sampling to select members within each cluster.
Non-probability Sampling
This type of sampling does not rely on random selection, and it involves selecting samples in a way that does not give every member of the population an equal chance of being included in the sample. Non-probability sampling is often used in qualitative research, where the aim is not to generalize findings to a larger population, but to gain an in-depth understanding of a particular phenomenon or group. Non-probability sampling methods can be quicker and more cost-effective than probability sampling methods, but they may also be subject to bias and may not be representative of the larger population.
Types of Non-probability Sampling :
- Convenience Sampling: In this method, participants are chosen based on their availability or willingness to participate. This method is easy and convenient but may not be representative of the population.
- Purposive Sampling: In this method, participants are selected based on specific criteria, such as their expertise or knowledge on a particular topic. This method is often used in qualitative research, but may not be representative of the population.
- Snowball Sampling: In this method, participants are recruited through referrals from other participants. This method is often used when the population is hard to reach, but may not be representative of the population.
- Quota Sampling: In this method, a predetermined number of participants are selected based on specific criteria, such as age or gender. This method is often used in market research, but may not be representative of the population.
- Volunteer Sampling: In this method, participants volunteer to participate in the study. This method is often used in research where participants are motivated by personal interest or altruism, but may not be representative of the population.
Applications of Sampling Methods
Applications of Sampling Methods from different fields:
- Psychology : Sampling methods are used in psychology research to study various aspects of human behavior and mental processes. For example, researchers may use stratified sampling to select a sample of participants that is representative of the population based on factors such as age, gender, and ethnicity. Random sampling may also be used to select participants for experimental studies.
- Sociology : Sampling methods are commonly used in sociological research to study social phenomena and relationships between individuals and groups. For example, researchers may use cluster sampling to select a sample of neighborhoods to study the effects of economic inequality on health outcomes. Stratified sampling may also be used to select a sample of participants that is representative of the population based on factors such as income, education, and occupation.
- Social sciences: Sampling methods are commonly used in social sciences to study human behavior and attitudes. For example, researchers may use stratified sampling to select a sample of participants that is representative of the population based on factors such as age, gender, and income.
- Marketing : Sampling methods are used in marketing research to collect data on consumer preferences, behavior, and attitudes. For example, researchers may use random sampling to select a sample of consumers to participate in a survey about a new product.
- Healthcare : Sampling methods are used in healthcare research to study the prevalence of diseases and risk factors, and to evaluate interventions. For example, researchers may use cluster sampling to select a sample of health clinics to participate in a study of the effectiveness of a new treatment.
- Environmental science: Sampling methods are used in environmental science to collect data on environmental variables such as water quality, air pollution, and soil composition. For example, researchers may use systematic sampling to collect soil samples at regular intervals across a field.
- Education : Sampling methods are used in education research to study student learning and achievement. For example, researchers may use stratified sampling to select a sample of schools that is representative of the population based on factors such as demographics and academic performance.
Examples of Sampling Methods
Probability Sampling Methods Examples:
- Simple random sampling Example : A researcher randomly selects participants from the population using a random number generator or drawing names from a hat.
- Stratified random sampling Example : A researcher divides the population into subgroups (strata) based on a characteristic of interest (e.g. age or income) and then randomly selects participants from each subgroup.
- Systematic sampling Example : A researcher selects participants at regular intervals from a list of the population.
Non-probability Sampling Methods Examples:
- Convenience sampling Example: A researcher selects participants who are conveniently available, such as students in a particular class or visitors to a shopping mall.
- Purposive sampling Example : A researcher selects participants who meet specific criteria, such as individuals who have been diagnosed with a particular medical condition.
- Snowball sampling Example : A researcher selects participants who are referred to them by other participants, such as friends or acquaintances.
How to Conduct Sampling Methods
some general steps to conduct sampling methods:
- Define the population: Identify the population of interest and clearly define its boundaries.
- Choose the sampling method: Select an appropriate sampling method based on the research question, characteristics of the population, and available resources.
- Determine the sample size: Determine the desired sample size based on statistical considerations such as margin of error, confidence level, or power analysis.
- Create a sampling frame: Develop a list of all individuals or elements in the population from which the sample will be drawn. The sampling frame should be comprehensive, accurate, and up-to-date.
- Select the sample: Use the chosen sampling method to select the sample from the sampling frame. The sample should be selected randomly, or if using a non-random method, every effort should be made to minimize bias and ensure that the sample is representative of the population.
- Collect data: Once the sample has been selected, collect data from each member of the sample using appropriate research methods (e.g., surveys, interviews, observations).
- Analyze the data: Analyze the data collected from the sample to draw conclusions about the population of interest.
When to use Sampling Methods
Sampling methods are used in research when it is not feasible or practical to study the entire population of interest. Sampling allows researchers to study a smaller group of individuals, known as a sample, and use the findings from the sample to make inferences about the larger population.
Sampling methods are particularly useful when:
- The population of interest is too large to study in its entirety.
- The cost and time required to study the entire population are prohibitive.
- The population is geographically dispersed or difficult to access.
- The research question requires specialized or hard-to-find individuals.
- The data collected is quantitative and statistical analyses are used to draw conclusions.
Purpose of Sampling Methods
The main purpose of sampling methods in research is to obtain a representative sample of individuals or elements from a larger population of interest, in order to make inferences about the population as a whole. By studying a smaller group of individuals, known as a sample, researchers can gather information about the population that would be difficult or impossible to obtain from studying the entire population.
Sampling methods allow researchers to:
- Study a smaller, more manageable group of individuals, which is typically less time-consuming and less expensive than studying the entire population.
- Reduce the potential for data collection errors and improve the accuracy of the results by minimizing sampling bias.
- Make inferences about the larger population with a certain degree of confidence, using statistical analyses of the data collected from the sample.
- Improve the generalizability and external validity of the findings by ensuring that the sample is representative of the population of interest.
Characteristics of Sampling Methods
Here are some characteristics of sampling methods:
- Randomness : Probability sampling methods are based on random selection, meaning that every member of the population has an equal chance of being selected. This helps to minimize bias and ensure that the sample is representative of the population.
- Representativeness : The goal of sampling is to obtain a sample that is representative of the larger population of interest. This means that the sample should reflect the characteristics of the population in terms of key demographic, behavioral, or other relevant variables.
- Size : The size of the sample should be large enough to provide sufficient statistical power for the research question at hand. The sample size should also be appropriate for the chosen sampling method and the level of precision desired.
- Efficiency : Sampling methods should be efficient in terms of time, cost, and resources required. The method chosen should be feasible given the available resources and time constraints.
- Bias : Sampling methods should aim to minimize bias and ensure that the sample is representative of the population of interest. Bias can be introduced through non-random selection or non-response, and can affect the validity and generalizability of the findings.
- Precision : Sampling methods should be precise in terms of providing estimates of the population parameters of interest. Precision is influenced by sample size, sampling method, and level of variability in the population.
- Validity : The validity of the sampling method is important for ensuring that the results obtained from the sample are accurate and can be generalized to the population of interest. Validity can be affected by sampling method, sample size, and the representativeness of the sample.
Advantages of Sampling Methods
Sampling methods have several advantages, including:
- Cost-Effective : Sampling methods are often much cheaper and less time-consuming than studying an entire population. By studying only a small subset of the population, researchers can gather valuable data without incurring the costs associated with studying the entire population.
- Convenience : Sampling methods are often more convenient than studying an entire population. For example, if a researcher wants to study the eating habits of people in a city, it would be very difficult and time-consuming to study every single person in the city. By using sampling methods, the researcher can obtain data from a smaller subset of people, making the study more feasible.
- Accuracy: When done correctly, sampling methods can be very accurate. By using appropriate sampling techniques, researchers can obtain a sample that is representative of the entire population. This allows them to make accurate generalizations about the population as a whole based on the data collected from the sample.
- Time-Saving: Sampling methods can save a lot of time compared to studying the entire population. By studying a smaller sample, researchers can collect data much more quickly than they could if they studied every single person in the population.
- Less Bias : Sampling methods can reduce bias in a study. If a researcher were to study the entire population, it would be very difficult to eliminate all sources of bias. However, by using appropriate sampling techniques, researchers can reduce bias and obtain a sample that is more representative of the entire population.
Limitations of Sampling Methods
- Sampling Error : Sampling error is the difference between the sample statistic and the population parameter. It is the result of selecting a sample rather than the entire population. The larger the sample, the lower the sampling error. However, no matter how large the sample size, there will always be some degree of sampling error.
- Selection Bias: Selection bias occurs when the sample is not representative of the population. This can happen if the sample is not selected randomly or if some groups are underrepresented in the sample. Selection bias can lead to inaccurate conclusions about the population.
- Non-response Bias : Non-response bias occurs when some members of the sample do not respond to the survey or study. This can result in a biased sample if the non-respondents differ from the respondents in important ways.
- Time and Cost : While sampling can be cost-effective, it can still be expensive and time-consuming to select a sample that is representative of the population. Depending on the sampling method used, it may take a long time to obtain a sample that is large enough and representative enough to be useful.
- Limited Information : Sampling can only provide information about the variables that are measured. It may not provide information about other variables that are relevant to the research question but were not measured.
- Generalization : The extent to which the findings from a sample can be generalized to the population depends on the representativeness of the sample. If the sample is not representative of the population, it may not be possible to generalize the findings to the population as a whole.
About the author
Muhammad Hassan
Researcher, Academic Writer, Web developer
You may also like

Simple Random Sampling – Types, Method and...

Systematic Sampling – Types, Method and Examples

Volunteer Sampling – Definition, Methods and...

Convenience Sampling – Method, Types and Examples

Non-probability Sampling – Types, Methods and...

Probability Sampling – Methods, Types and...

Want to create or adapt books like this? Learn more about how Pressbooks supports open publishing practices.
Part 3: Using quantitative methods
13. Experimental design
Chapter outline.
- What is an experiment and when should you use one? (8 minute read)
- True experimental designs (7 minute read)
- Quasi-experimental designs (8 minute read)
- Non-experimental designs (5 minute read)
- Critical, ethical, and critical considerations (5 minute read)
Content warning : examples in this chapter contain references to non-consensual research in Western history, including experiments conducted during the Holocaust and on African Americans (section 13.6).
13.1 What is an experiment and when should you use one?
Learning objectives.
Learners will be able to…
- Identify the characteristics of a basic experiment
- Describe causality in experimental design
- Discuss the relationship between dependent and independent variables in experiments
- Explain the links between experiments and generalizability of results
- Describe advantages and disadvantages of experimental designs
The basics of experiments
The first experiment I can remember using was for my fourth grade science fair. I wondered if latex- or oil-based paint would hold up to sunlight better. So, I went to the hardware store and got a few small cans of paint and two sets of wooden paint sticks. I painted one with oil-based paint and the other with latex-based paint of different colors and put them in a sunny spot in the back yard. My hypothesis was that the oil-based paint would fade the most and that more fading would happen the longer I left the paint sticks out. (I know, it’s obvious, but I was only 10.)
I checked in on the paint sticks every few days for a month and wrote down my observations. The first part of my hypothesis ended up being wrong—it was actually the latex-based paint that faded the most. But the second part was right, and the paint faded more and more over time. This is a simple example, of course—experiments get a heck of a lot more complex than this when we’re talking about real research.
Merriam-Webster defines an experiment as “an operation or procedure carried out under controlled conditions in order to discover an unknown effect or law, to test or establish a hypothesis, or to illustrate a known law.” Each of these three components of the definition will come in handy as we go through the different types of experimental design in this chapter. Most of us probably think of the physical sciences when we think of experiments, and for good reason—these experiments can be pretty flashy! But social science and psychological research follow the same scientific methods, as we’ve discussed in this book.
As the video discusses, experiments can be used in social sciences just like they can in physical sciences. It makes sense to use an experiment when you want to determine the cause of a phenomenon with as much accuracy as possible. Some types of experimental designs do this more precisely than others, as we’ll see throughout the chapter. If you’ll remember back to Chapter 11 and the discussion of validity, experiments are the best way to ensure internal validity, or the extent to which a change in your independent variable causes a change in your dependent variable.
Experimental designs for research projects are most appropriate when trying to uncover or test a hypothesis about the cause of a phenomenon, so they are best for explanatory research questions. As we’ll learn throughout this chapter, different circumstances are appropriate for different types of experimental designs. Each type of experimental design has advantages and disadvantages, and some are better at controlling the effect of extraneous variables —those variables and characteristics that have an effect on your dependent variable, but aren’t the primary variable whose influence you’re interested in testing. For example, in a study that tries to determine whether aspirin lowers a person’s risk of a fatal heart attack, a person’s race would likely be an extraneous variable because you primarily want to know the effect of aspirin.
In practice, many types of experimental designs can be logistically challenging and resource-intensive. As practitioners, the likelihood that we will be involved in some of the types of experimental designs discussed in this chapter is fairly low. However, it’s important to learn about these methods, even if we might not ever use them, so that we can be thoughtful consumers of research that uses experimental designs.
While we might not use all of these types of experimental designs, many of us will engage in evidence-based practice during our time as social workers. A lot of research developing evidence-based practice, which has a strong emphasis on generalizability, will use experimental designs. You’ve undoubtedly seen one or two in your literature search so far.
The logic of experimental design
How do we know that one phenomenon causes another? The complexity of the social world in which we practice and conduct research means that causes of social problems are rarely cut and dry. Uncovering explanations for social problems is key to helping clients address them, and experimental research designs are one road to finding answers.
As you read about in Chapter 8 (and as we’ll discuss again in Chapter 15 ), just because two phenomena are related in some way doesn’t mean that one causes the other. Ice cream sales increase in the summer, and so does the rate of violent crime; does that mean that eating ice cream is going to make me murder someone? Obviously not, because ice cream is great. The reality of that relationship is far more complex—it could be that hot weather makes people more irritable and, at times, violent, while also making people want ice cream. More likely, though, there are other social factors not accounted for in the way we just described this relationship.
Experimental designs can help clear up at least some of this fog by allowing researchers to isolate the effect of interventions on dependent variables by controlling extraneous variables . In true experimental design (discussed in the next section) and some quasi-experimental designs, researchers accomplish this w ith the control group and the experimental group . (The experimental group is sometimes called the “treatment group,” but we will call it the experimental group in this chapter.) The control group does not receive the intervention you are testing (they may receive no intervention or what is known as “treatment as usual”), while the experimental group does. (You will hopefully remember our earlier discussion of control variables in Chapter 8 —conceptually, the use of the word “control” here is the same.)
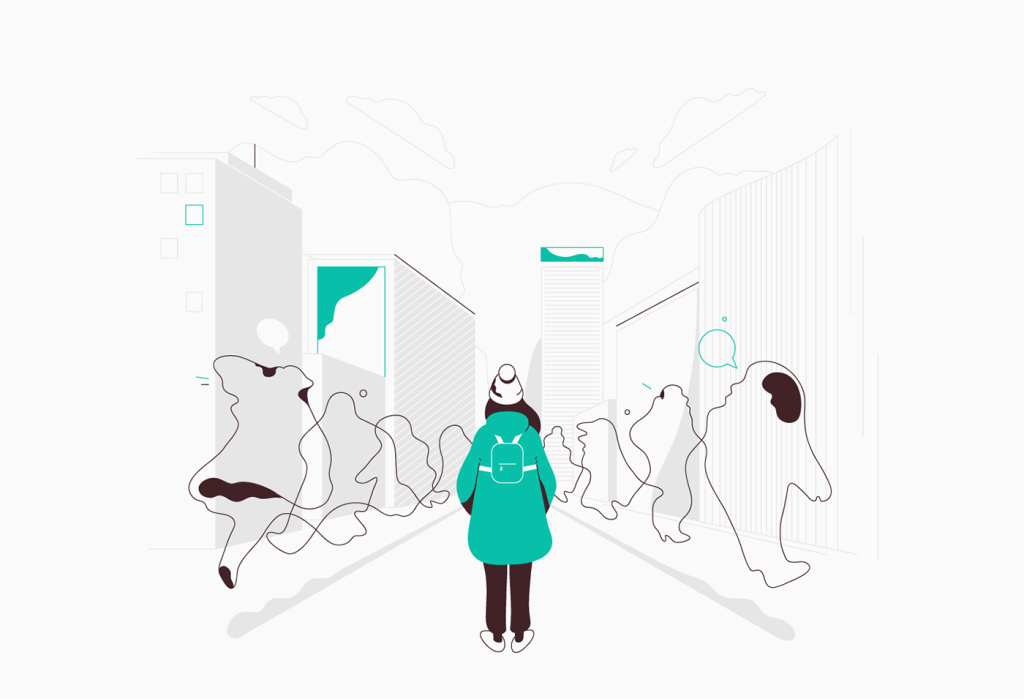
In a well-designed experiment, your control group should look almost identical to your experimental group in terms of demographics and other relevant factors. What if we want to know the effect of CBT on social anxiety, but we have learned in prior research that men tend to have a more difficult time overcoming social anxiety? We would want our control and experimental groups to have a similar gender mix because it would limit the effect of gender on our results, since ostensibly, both groups’ results would be affected by gender in the same way. If your control group has 5 women, 6 men, and 4 non-binary people, then your experimental group should be made up of roughly the same gender balance to help control for the influence of gender on the outcome of your intervention. (In reality, the groups should be similar along other dimensions, as well, and your group will likely be much larger.) The researcher will use the same outcome measures for both groups and compare them, and assuming the experiment was designed correctly, get a pretty good answer about whether the intervention had an effect on social anxiety.
You will also hear people talk about comparison groups , which are similar to control groups. The primary difference between the two is that a control group is populated using random assignment, but a comparison group is not. Random assignment entails using a random process to decide which participants are put into the control or experimental group (which participants receive an intervention and which do not). By randomly assigning participants to a group, you can reduce the effect of extraneous variables on your research because there won’t be a systematic difference between the groups.
Do not confuse random assignment with random sampling. Random sampling is a method for selecting a sample from a population, and is rarely used in psychological research. Random assignment is a method for assigning participants in a sample to the different conditions, and it is an important element of all experimental research in psychology and other related fields. Random sampling also helps a great deal with generalizability , whereas random assignment increases internal validity .
We have already learned about internal validity in Chapter 11 . The use of an experimental design will bolster internal validity since it works to isolate causal relationships. As we will see in the coming sections, some types of experimental design do this more effectively than others. It’s also worth considering that true experiments, which most effectively show causality , are often difficult and expensive to implement. Although other experimental designs aren’t perfect, they still produce useful, valid evidence and may be more feasible to carry out.
Key Takeaways
- Experimental designs are useful for establishing causality, but some types of experimental design do this better than others.
- Experiments help researchers isolate the effect of the independent variable on the dependent variable by controlling for the effect of extraneous variables .
- Experiments use a control/comparison group and an experimental group to test the effects of interventions. These groups should be as similar to each other as possible in terms of demographics and other relevant factors.
- True experiments have control groups with randomly assigned participants, while other types of experiments have comparison groups to which participants are not randomly assigned.
- Think about the research project you’ve been designing so far. How might you use a basic experiment to answer your question? If your question isn’t explanatory, try to formulate a new explanatory question and consider the usefulness of an experiment.
- Why is establishing a simple relationship between two variables not indicative of one causing the other?
13.2 True experimental design
- Describe a true experimental design in social work research
- Understand the different types of true experimental designs
- Determine what kinds of research questions true experimental designs are suited for
- Discuss advantages and disadvantages of true experimental designs
True experimental design , often considered to be the “gold standard” in research designs, is thought of as one of the most rigorous of all research designs. In this design, one or more independent variables are manipulated by the researcher (as treatments), subjects are randomly assigned to different treatment levels (random assignment), and the results of the treatments on outcomes (dependent variables) are observed. The unique strength of experimental research is its internal validity and its ability to establish ( causality ) through treatment manipulation, while controlling for the effects of extraneous variable. Sometimes the treatment level is no treatment, while other times it is simply a different treatment than that which we are trying to evaluate. For example, we might have a control group that is made up of people who will not receive any treatment for a particular condition. Or, a control group could consist of people who consent to treatment with DBT when we are testing the effectiveness of CBT.
As we discussed in the previous section, a true experiment has a control group with participants randomly assigned , and an experimental group . This is the most basic element of a true experiment. The next decision a researcher must make is when they need to gather data during their experiment. Do they take a baseline measurement and then a measurement after treatment, or just a measurement after treatment, or do they handle measurement another way? Below, we’ll discuss the three main types of true experimental designs. There are sub-types of each of these designs, but here, we just want to get you started with some of the basics.
Using a true experiment in social work research is often pretty difficult, since as I mentioned earlier, true experiments can be quite resource intensive. True experiments work best with relatively large sample sizes, and random assignment, a key criterion for a true experimental design, is hard (and unethical) to execute in practice when you have people in dire need of an intervention. Nonetheless, some of the strongest evidence bases are built on true experiments.
For the purposes of this section, let’s bring back the example of CBT for the treatment of social anxiety. We have a group of 500 individuals who have agreed to participate in our study, and we have randomly assigned them to the control and experimental groups. The folks in the experimental group will receive CBT, while the folks in the control group will receive more unstructured, basic talk therapy. These designs, as we talked about above, are best suited for explanatory research questions.
Before we get started, take a look at the table below. When explaining experimental research designs, we often use diagrams with abbreviations to visually represent the experiment. Table 13.1 starts us off by laying out what each of the abbreviations mean.
R | Randomly assigned group (control/comparison or experimental) |
O | Observation/measurement taken of dependent variable |
X | Intervention or treatment |
X | Experimental or new intervention |
X | Typical intervention/treatment as usual |
A, B, C, etc. | Denotes different groups (control/comparison and experimental) |
Pretest and post-test control group design
In pretest and post-test control group design , participants are given a pretest of some kind to measure their baseline state before their participation in an intervention. In our social anxiety experiment, we would have participants in both the experimental and control groups complete some measure of social anxiety—most likely an established scale and/or a structured interview—before they start their treatment. As part of the experiment, we would have a defined time period during which the treatment would take place (let’s say 12 weeks, just for illustration). At the end of 12 weeks, we would give both groups the same measure as a post-test .
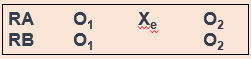
In the diagram, RA (random assignment group A) is the experimental group and RB is the control group. O 1 denotes the pre-test, X e denotes the experimental intervention, and O 2 denotes the post-test. Let’s look at this diagram another way, using the example of CBT for social anxiety that we’ve been talking about.

In a situation where the control group received treatment as usual instead of no intervention, the diagram would look this way, with X i denoting treatment as usual (Figure 13.3).
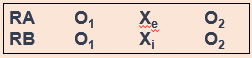
Hopefully, these diagrams provide you a visualization of how this type of experiment establishes time order , a key component of a causal relationship. Did the change occur after the intervention? Assuming there is a change in the scores between the pretest and post-test, we would be able to say that yes, the change did occur after the intervention. Causality can’t exist if the change happened before the intervention—this would mean that something else led to the change, not our intervention.
Post-test only control group design
Post-test only control group design involves only giving participants a post-test, just like it sounds (Figure 13.4).
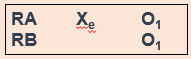
But why would you use this design instead of using a pretest/post-test design? One reason could be the testing effect that can happen when research participants take a pretest. In research, the testing effect refers to “measurement error related to how a test is given; the conditions of the testing, including environmental conditions; and acclimation to the test itself” (Engel & Schutt, 2017, p. 444) [1] (When we say “measurement error,” all we mean is the accuracy of the way we measure the dependent variable.) Figure 13.4 is a visualization of this type of experiment. The testing effect isn’t always bad in practice—our initial assessments might help clients identify or put into words feelings or experiences they are having when they haven’t been able to do that before. In research, however, we might want to control its effects to isolate a cleaner causal relationship between intervention and outcome.
Going back to our CBT for social anxiety example, we might be concerned that participants would learn about social anxiety symptoms by virtue of taking a pretest. They might then identify that they have those symptoms on the post-test, even though they are not new symptoms for them. That could make our intervention look less effective than it actually is.
However, without a baseline measurement establishing causality can be more difficult. If we don’t know someone’s state of mind before our intervention, how do we know our intervention did anything at all? Establishing time order is thus a little more difficult. You must balance this consideration with the benefits of this type of design.
Solomon four group design
One way we can possibly measure how much the testing effect might change the results of the experiment is with the Solomon four group design. Basically, as part of this experiment, you have two control groups and two experimental groups. The first pair of groups receives both a pretest and a post-test. The other pair of groups receives only a post-test (Figure 13.5). This design helps address the problem of establishing time order in post-test only control group designs.
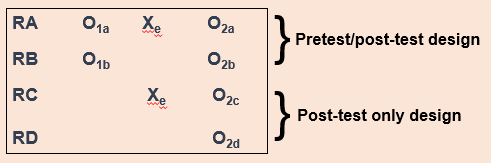
For our CBT project, we would randomly assign people to four different groups instead of just two. Groups A and B would take our pretest measures and our post-test measures, and groups C and D would take only our post-test measures. We could then compare the results among these groups and see if they’re significantly different between the folks in A and B, and C and D. If they are, we may have identified some kind of testing effect, which enables us to put our results into full context. We don’t want to draw a strong causal conclusion about our intervention when we have major concerns about testing effects without trying to determine the extent of those effects.
Solomon four group designs are less common in social work research, primarily because of the logistics and resource needs involved. Nonetheless, this is an important experimental design to consider when we want to address major concerns about testing effects.
- True experimental design is best suited for explanatory research questions.
- True experiments require random assignment of participants to control and experimental groups.
- Pretest/post-test research design involves two points of measurement—one pre-intervention and one post-intervention.
- Post-test only research design involves only one point of measurement—post-intervention. It is a useful design to minimize the effect of testing effects on our results.
- Solomon four group research design involves both of the above types of designs, using 2 pairs of control and experimental groups. One group receives both a pretest and a post-test, while the other receives only a post-test. This can help uncover the influence of testing effects.
- Think about a true experiment you might conduct for your research project. Which design would be best for your research, and why?
- What challenges or limitations might make it unrealistic (or at least very complicated!) for you to carry your true experimental design in the real-world as a student researcher?
- What hypothesis(es) would you test using this true experiment?
13.4 Quasi-experimental designs
- Describe a quasi-experimental design in social work research
- Understand the different types of quasi-experimental designs
- Determine what kinds of research questions quasi-experimental designs are suited for
- Discuss advantages and disadvantages of quasi-experimental designs
Quasi-experimental designs are a lot more common in social work research than true experimental designs. Although quasi-experiments don’t do as good a job of giving us robust proof of causality , they still allow us to establish time order , which is a key element of causality. The prefix quasi means “resembling,” so quasi-experimental research is research that resembles experimental research, but is not true experimental research. Nonetheless, given proper research design, quasi-experiments can still provide extremely rigorous and useful results.
There are a few key differences between true experimental and quasi-experimental research. The primary difference between quasi-experimental research and true experimental research is that quasi-experimental research does not involve random assignment to control and experimental groups. Instead, we talk about comparison groups in quasi-experimental research instead. As a result, these types of experiments don’t control the effect of extraneous variables as well as a true experiment.
Quasi-experiments are most likely to be conducted in field settings in which random assignment is difficult or impossible. They are often conducted to evaluate the effectiveness of a treatment—perhaps a type of psychotherapy or an educational intervention. We’re able to eliminate some threats to internal validity, but we can’t do this as effectively as we can with a true experiment. Realistically, our CBT-social anxiety project is likely to be a quasi experiment, based on the resources and participant pool we’re likely to have available.
It’s important to note that not all quasi-experimental designs have a comparison group. There are many different kinds of quasi-experiments, but we will discuss the three main types below: nonequivalent comparison group designs, time series designs, and ex post facto comparison group designs.
Nonequivalent comparison group design
You will notice that this type of design looks extremely similar to the pretest/post-test design that we discussed in section 13.3. But instead of random assignment to control and experimental groups, researchers use other methods to construct their comparison and experimental groups. A diagram of this design will also look very similar to pretest/post-test design, but you’ll notice we’ve removed the “R” from our groups, since they are not randomly assigned (Figure 13.6).
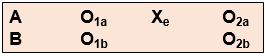
Researchers using this design select a comparison group that’s as close as possible based on relevant factors to their experimental group. Engel and Schutt (2017) [2] identify two different selection methods:
- Individual matching : Researchers take the time to match individual cases in the experimental group to similar cases in the comparison group. It can be difficult, however, to match participants on all the variables you want to control for.
- Aggregate matching : Instead of trying to match individual participants to each other, researchers try to match the population profile of the comparison and experimental groups. For example, researchers would try to match the groups on average age, gender balance, or median income. This is a less resource-intensive matching method, but researchers have to ensure that participants aren’t choosing which group (comparison or experimental) they are a part of.
As we’ve already talked about, this kind of design provides weaker evidence that the intervention itself leads to a change in outcome. Nonetheless, we are still able to establish time order using this method, and can thereby show an association between the intervention and the outcome. Like true experimental designs, this type of quasi-experimental design is useful for explanatory research questions.
What might this look like in a practice setting? Let’s say you’re working at an agency that provides CBT and other types of interventions, and you have identified a group of clients who are seeking help for social anxiety, as in our earlier example. Once you’ve obtained consent from your clients, you can create a comparison group using one of the matching methods we just discussed. If the group is small, you might match using individual matching, but if it’s larger, you’ll probably sort people by demographics to try to get similar population profiles. (You can do aggregate matching more easily when your agency has some kind of electronic records or database, but it’s still possible to do manually.)
Time series design
Another type of quasi-experimental design is a time series design. Unlike other types of experimental design, time series designs do not have a comparison group. A time series is a set of measurements taken at intervals over a period of time (Figure 13.7). Proper time series design should include at least three pre- and post-intervention measurement points. While there are a few types of time series designs, we’re going to focus on the most common: interrupted time series design.

But why use this method? Here’s an example. Let’s think about elementary student behavior throughout the school year. As anyone with children or who is a teacher knows, kids get very excited and animated around holidays, days off, or even just on a Friday afternoon. This fact might mean that around those times of year, there are more reports of disruptive behavior in classrooms. What if we took our one and only measurement in mid-December? It’s possible we’d see a higher-than-average rate of disruptive behavior reports, which could bias our results if our next measurement is around a time of year students are in a different, less excitable frame of mind. When we take multiple measurements throughout the first half of the school year, we can establish a more accurate baseline for the rate of these reports by looking at the trend over time.
We may want to test the effect of extended recess times in elementary school on reports of disruptive behavior in classrooms. When students come back after the winter break, the school extends recess by 10 minutes each day (the intervention), and the researchers start tracking the monthly reports of disruptive behavior again. These reports could be subject to the same fluctuations as the pre-intervention reports, and so we once again take multiple measurements over time to try to control for those fluctuations.
This method improves the extent to which we can establish causality because we are accounting for a major extraneous variable in the equation—the passage of time. On its own, it does not allow us to account for other extraneous variables, but it does establish time order and association between the intervention and the trend in reports of disruptive behavior. Finding a stable condition before the treatment that changes after the treatment is evidence for causality between treatment and outcome.
Ex post facto comparison group design
Ex post facto (Latin for “after the fact”) designs are extremely similar to nonequivalent comparison group designs. There are still comparison and experimental groups, pretest and post-test measurements, and an intervention. But in ex post facto designs, participants are assigned to the comparison and experimental groups once the intervention has already happened. This type of design often occurs when interventions are already up and running at an agency and the agency wants to assess effectiveness based on people who have already completed treatment.
In most clinical agency environments, social workers conduct both initial and exit assessments, so there are usually some kind of pretest and post-test measures available. We also typically collect demographic information about our clients, which could allow us to try to use some kind of matching to construct comparison and experimental groups.
In terms of internal validity and establishing causality, ex post facto designs are a bit of a mixed bag. The ability to establish causality depends partially on the ability to construct comparison and experimental groups that are demographically similar so we can control for these extraneous variables .
Quasi-experimental designs are common in social work intervention research because, when designed correctly, they balance the intense resource needs of true experiments with the realities of research in practice. They still offer researchers tools to gather robust evidence about whether interventions are having positive effects for clients.
- Quasi-experimental designs are similar to true experiments, but do not require random assignment to experimental and control groups.
- In quasi-experimental projects, the group not receiving the treatment is called the comparison group, not the control group.
- Nonequivalent comparison group design is nearly identical to pretest/post-test experimental design, but participants are not randomly assigned to the experimental and control groups. As a result, this design provides slightly less robust evidence for causality.
- Nonequivalent groups can be constructed by individual matching or aggregate matching .
- Time series design does not have a control or experimental group, and instead compares the condition of participants before and after the intervention by measuring relevant factors at multiple points in time. This allows researchers to mitigate the error introduced by the passage of time.
- Ex post facto comparison group designs are also similar to true experiments, but experimental and comparison groups are constructed after the intervention is over. This makes it more difficult to control for the effect of extraneous variables, but still provides useful evidence for causality because it maintains the time order of the experiment.
- Think back to the experiment you considered for your research project in Section 13.3. Now that you know more about quasi-experimental designs, do you still think it’s a true experiment? Why or why not?
- What should you consider when deciding whether an experimental or quasi-experimental design would be more feasible or fit your research question better?
13.5 Non-experimental designs
- Describe non-experimental designs in social work research
- Discuss how non-experimental research differs from true and quasi-experimental research
- Demonstrate an understanding the different types of non-experimental designs
- Determine what kinds of research questions non-experimental designs are suited for
- Discuss advantages and disadvantages of non-experimental designs
The previous sections have laid out the basics of some rigorous approaches to establish that an intervention is responsible for changes we observe in research participants. This type of evidence is extremely important to build an evidence base for social work interventions, but it’s not the only type of evidence to consider. We will discuss qualitative methods, which provide us with rich, contextual information, in Part 4 of this text. The designs we’ll talk about in this section are sometimes used in qualitative research but in keeping with our discussion of experimental design so far, we’re going to stay in the quantitative research realm for now. Non-experimental is also often a stepping stone for more rigorous experimental design in the future, as it can help test the feasibility of your research.
In general, non-experimental designs do not strongly support causality and don’t address threats to internal validity. However, that’s not really what they’re intended for. Non-experimental designs are useful for a few different types of research, including explanatory questions in program evaluation. Certain types of non-experimental design are also helpful for researchers when they are trying to develop a new assessment or scale. Other times, researchers or agency staff did not get a chance to gather any assessment information before an intervention began, so a pretest/post-test design is not possible.
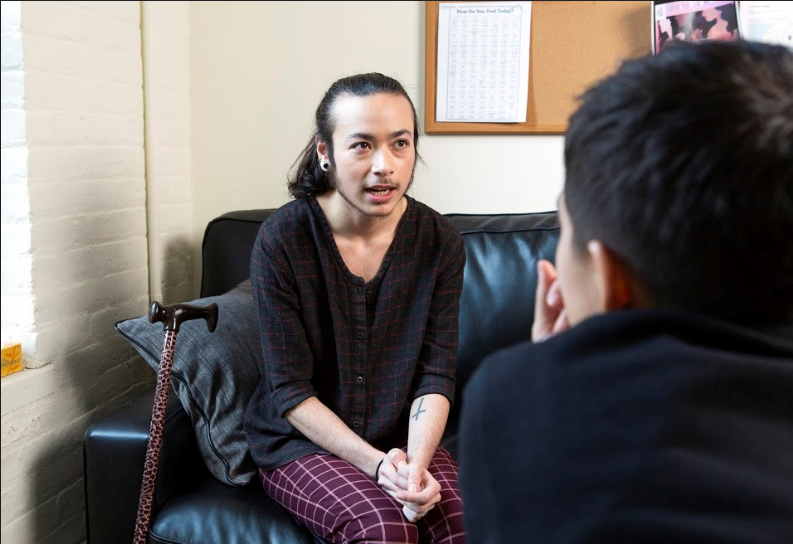
A significant benefit of these types of designs is that they’re pretty easy to execute in a practice or agency setting. They don’t require a comparison or control group, and as Engel and Schutt (2017) [3] point out, they “flow from a typical practice model of assessment, intervention, and evaluating the impact of the intervention” (p. 177). Thus, these designs are fairly intuitive for social workers, even when they aren’t expert researchers. Below, we will go into some detail about the different types of non-experimental design.
One group pretest/post-test design
Also known as a before-after one-group design, this type of research design does not have a comparison group and everyone who participates in the research receives the intervention (Figure 13.8). This is a common type of design in program evaluation in the practice world. Controlling for extraneous variables is difficult or impossible in this design, but given that it is still possible to establish some measure of time order, it does provide weak support for causality.

Imagine, for example, a researcher who is interested in the effectiveness of an anti-drug education program on elementary school students’ attitudes toward illegal drugs. The researcher could assess students’ attitudes about illegal drugs (O 1 ), implement the anti-drug program (X), and then immediately after the program ends, the researcher could once again measure students’ attitudes toward illegal drugs (O 2 ). You can see how this would be relatively simple to do in practice, and have probably been involved in this type of research design yourself, even if informally. But hopefully, you can also see that this design would not provide us with much evidence for causality because we have no way of controlling for the effect of extraneous variables. A lot of things could have affected any change in students’ attitudes—maybe girls already had different attitudes about illegal drugs than children of other genders, and when we look at the class’s results as a whole, we couldn’t account for that influence using this design.
All of that doesn’t mean these results aren’t useful, however. If we find that children’s attitudes didn’t change at all after the drug education program, then we need to think seriously about how to make it more effective or whether we should be using it at all. (This immediate, practical application of our results highlights a key difference between program evaluation and research, which we will discuss in Chapter 23 .)
After-only design
As the name suggests, this type of non-experimental design involves measurement only after an intervention. There is no comparison or control group, and everyone receives the intervention. I have seen this design repeatedly in my time as a program evaluation consultant for nonprofit organizations, because often these organizations realize too late that they would like to or need to have some sort of measure of what effect their programs are having.
Because there is no pretest and no comparison group, this design is not useful for supporting causality since we can’t establish the time order and we can’t control for extraneous variables. However, that doesn’t mean it’s not useful at all! Sometimes, agencies need to gather information about how their programs are functioning. A classic example of this design is satisfaction surveys—realistically, these can only be administered after a program or intervention. Questions regarding satisfaction, ease of use or engagement, or other questions that don’t involve comparisons are best suited for this type of design.
Static-group design
A final type of non-experimental research is the static-group design. In this type of research, there are both comparison and experimental groups, which are not randomly assigned. There is no pretest, only a post-test, and the comparison group has to be constructed by the researcher. Sometimes, researchers will use matching techniques to construct the groups, but often, the groups are constructed by convenience of who is being served at the agency.
Non-experimental research designs are easy to execute in practice, but we must be cautious about drawing causal conclusions from the results. A positive result may still suggest that we should continue using a particular intervention (and no result or a negative result should make us reconsider whether we should use that intervention at all). You have likely seen non-experimental research in your daily life or at your agency, and knowing the basics of how to structure such a project will help you ensure you are providing clients with the best care possible.
- Non-experimental designs are useful for describing phenomena, but cannot demonstrate causality.
- After-only designs are often used in agency and practice settings because practitioners are often not able to set up pre-test/post-test designs.
- Non-experimental designs are useful for explanatory questions in program evaluation and are helpful for researchers when they are trying to develop a new assessment or scale.
- Non-experimental designs are well-suited to qualitative methods.
- If you were to use a non-experimental design for your research project, which would you choose? Why?
- Have you conducted non-experimental research in your practice or professional life? Which type of non-experimental design was it?
13.6 Critical, ethical, and cultural considerations
- Describe critiques of experimental design
- Identify ethical issues in the design and execution of experiments
- Identify cultural considerations in experimental design
As I said at the outset, experiments, and especially true experiments, have long been seen as the gold standard to gather scientific evidence. When it comes to research in the biomedical field and other physical sciences, true experiments are subject to far less nuance than experiments in the social world. This doesn’t mean they are easier—just subject to different forces. However, as a society, we have placed the most value on quantitative evidence obtained through empirical observation and especially experimentation.
Major critiques of experimental designs tend to focus on true experiments, especially randomized controlled trials (RCTs), but many of these critiques can be applied to quasi-experimental designs, too. Some researchers, even in the biomedical sciences, question the view that RCTs are inherently superior to other types of quantitative research designs. RCTs are far less flexible and have much more stringent requirements than other types of research. One seemingly small issue, like incorrect information about a research participant, can derail an entire RCT. RCTs also cost a great deal of money to implement and don’t reflect “real world” conditions. The cost of true experimental research or RCTs also means that some communities are unlikely to ever have access to these research methods. It is then easy for people to dismiss their research findings because their methods are seen as “not rigorous.”
Obviously, controlling outside influences is important for researchers to draw strong conclusions, but what if those outside influences are actually important for how an intervention works? Are we missing really important information by focusing solely on control in our research? Is a treatment going to work the same for white women as it does for indigenous women? With the myriad effects of our societal structures, you should be very careful ever assuming this will be the case. This doesn’t mean that cultural differences will negate the effect of an intervention; instead, it means that you should remember to practice cultural humility implementing all interventions, even when we “know” they work.
How we build evidence through experimental research reveals a lot about our values and biases, and historically, much experimental research has been conducted on white people, and especially white men. [4] This makes sense when we consider the extent to which the sciences and academia have historically been dominated by white patriarchy. This is especially important for marginalized groups that have long been ignored in research literature, meaning they have also been ignored in the development of interventions and treatments that are accepted as “effective.” There are examples of marginalized groups being experimented on without their consent, like the Tuskegee Experiment or Nazi experiments on Jewish people during World War II. We cannot ignore the collective consciousness situations like this can create about experimental research for marginalized groups.
None of this is to say that experimental research is inherently bad or that you shouldn’t use it. Quite the opposite—use it when you can, because there are a lot of benefits, as we learned throughout this chapter. As a social work researcher, you are uniquely positioned to conduct experimental research while applying social work values and ethics to the process and be a leader for others to conduct research in the same framework. It can conflict with our professional ethics, especially respect for persons and beneficence, if we do not engage in experimental research with our eyes wide open. We also have the benefit of a great deal of practice knowledge that researchers in other fields have not had the opportunity to get. As with all your research, always be sure you are fully exploring the limitations of the research.
- While true experimental research gathers strong evidence, it can also be inflexible, expensive, and overly simplistic in terms of important social forces that affect the resources.
- Marginalized communities’ past experiences with experimental research can affect how they respond to research participation.
- Social work researchers should use both their values and ethics, and their practice experiences, to inform research and push other researchers to do the same.
- Think back to the true experiment you sketched out in the exercises for Section 13.3. Are there cultural or historical considerations you hadn’t thought of with your participant group? What are they? Does this change the type of experiment you would want to do?
- How can you as a social work researcher encourage researchers in other fields to consider social work ethics and values in their experimental research?
Media Attributions
- Being kinder to yourself © Evgenia Makarova is licensed under a CC BY-NC-ND (Attribution NonCommercial NoDerivatives) license
- Original by author is licensed under a CC BY-NC-SA (Attribution NonCommercial ShareAlike) license
- Original by author. is licensed under a CC BY-NC-SA (Attribution NonCommercial ShareAlike) license
- Orginal by author. is licensed under a CC BY-NC-SA (Attribution NonCommercial ShareAlike) license
- therapist © Zackary Drucker is licensed under a CC BY-NC-ND (Attribution NonCommercial NoDerivatives) license
- nonexper-pretest-posttest is licensed under a CC BY-NC-SA (Attribution NonCommercial ShareAlike) license
- Engel, R. & Schutt, R. (2016). The practice of research in social work. Thousand Oaks, CA: SAGE Publications, Inc. ↵
- Sullivan, G. M. (2011). Getting off the “gold standard”: Randomized controlled trials and education research. Journal of Graduate Medical Education , 3 (3), 285-289. ↵
an operation or procedure carried out under controlled conditions in order to discover an unknown effect or law, to test or establish a hypothesis, or to illustrate a known law.
explains why particular phenomena work in the way that they do; answers “why” questions
variables and characteristics that have an effect on your outcome, but aren't the primary variable whose influence you're interested in testing.
the group of participants in our study who do not receive the intervention we are researching in experiments with random assignment
in experimental design, the group of participants in our study who do receive the intervention we are researching
the group of participants in our study who do not receive the intervention we are researching in experiments without random assignment
using a random process to decide which participants are tested in which conditions
The ability to apply research findings beyond the study sample to some broader population,
Ability to say that one variable "causes" something to happen to another variable. Very important to assess when thinking about studies that examine causation such as experimental or quasi-experimental designs.
the idea that one event, behavior, or belief will result in the occurrence of another, subsequent event, behavior, or belief
An experimental design in which one or more independent variables are manipulated by the researcher (as treatments), subjects are randomly assigned to different treatment levels (random assignment), and the results of the treatments on outcomes (dependent variables) are observed
a type of experimental design in which participants are randomly assigned to control and experimental groups, one group receives an intervention, and both groups receive pre- and post-test assessments
A measure of a participant's condition before they receive an intervention or treatment.
A measure of a participant's condition after an intervention or, if they are part of the control/comparison group, at the end of an experiment.
A demonstration that a change occurred after an intervention. An important criterion for establishing causality.
an experimental design in which participants are randomly assigned to control and treatment groups, one group receives an intervention, and both groups receive only a post-test assessment
The measurement error related to how a test is given; the conditions of the testing, including environmental conditions; and acclimation to the test itself
a subtype of experimental design that is similar to a true experiment, but does not have randomly assigned control and treatment groups
In nonequivalent comparison group designs, the process by which researchers match individual cases in the experimental group to similar cases in the comparison group.
In nonequivalent comparison group designs, the process in which researchers match the population profile of the comparison and experimental groups.
a set of measurements taken at intervals over a period of time
Research that involves the use of data that represents human expression through words, pictures, movies, performance and other artifacts.
Graduate research methods in social work Copyright © 2021 by Matthew DeCarlo, Cory Cummings, Kate Agnelli is licensed under a Creative Commons Attribution-NonCommercial-ShareAlike 4.0 International License , except where otherwise noted.
Share This Book
Click through the PLOS taxonomy to find articles in your field.
For more information about PLOS Subject Areas, click here .
Loading metrics
Open Access
Peer-reviewed
Research Article
Archaeology in space: The Sampling Quadrangle Assemblages Research Experiment (SQuARE) on the International Space Station. Report 1: Squares 03 and 05
Roles Conceptualization, Data curation, Formal analysis, Funding acquisition, Investigation, Methodology, Project administration, Resources, Supervision, Visualization, Writing – original draft, Writing – review & editing
* E-mail: [email protected]
Affiliations Department of Art, Chapman University, Orange, CA, United States of America, Space Engineering Research Center, University of Southern California, Marina del Rey, CA, United States of America

Roles Data curation, Formal analysis, Investigation, Methodology, Resources, Software, Supervision, Validation, Visualization, Writing – original draft, Writing – review & editing
Affiliation Department of History, Carleton University, Ottawa, ON, United States of America
Roles Conceptualization, Data curation, Methodology, Project administration, Supervision, Writing – review & editing
Affiliation College of Humanities, Arts and Social Sciences, Flinders University, Adelaide, Australia
Roles Software, Writing – original draft
Roles Investigation, Writing – original draft
Affiliation Archaeology Research Center, University of Southern California, Los Angeles, CA, United States of America
- Justin St. P. Walsh,
- Shawn Graham,
- Alice C. Gorman,
- Chantal Brousseau,
- Salma Abdullah
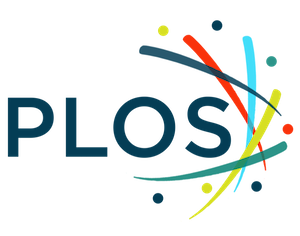
- Published: August 7, 2024
- https://doi.org/10.1371/journal.pone.0304229
- Reader Comments
Between January and March 2022, crew aboard the International Space Station (ISS) performed the first archaeological fieldwork in space, the Sampling Quadrangle Assemblages Research Experiment (SQuARE). The experiment aimed to: (1) develop a new understanding of how humans adapt to life in an environmental context for which we are not evolutionarily adapted, using evidence from the observation of material culture; (2) identify disjunctions between planned and actual usage of facilities on a space station; (3) develop and test techniques that enable archaeological research at a distance; and (4) demonstrate the relevance of social science methods and perspectives for improving life in space. In this article, we describe our methodology, which involves a creative re-imagining of a long-standing sampling practice for the characterization of a site, the shovel test pit. The ISS crew marked out six sample locations (“squares”) around the ISS and documented them through daily photography over a 60-day period. Here we present the results from two of the six squares: an equipment maintenance area, and an area near exercise equipment and the latrine. Using the photographs and an innovative webtool, we identified 5,438 instances of items, labeling them by type and function. We then performed chronological analyses to determine how the documented areas were actually used. Our results show differences between intended and actual use, with storage the most common function of the maintenance area, and personal hygiene activities most common in an undesignated area near locations for exercise and waste.
Citation: Walsh JSP, Graham S, Gorman AC, Brousseau C, Abdullah S (2024) Archaeology in space: The Sampling Quadrangle Assemblages Research Experiment (SQuARE) on the International Space Station. Report 1: Squares 03 and 05. PLoS ONE 19(8): e0304229. https://doi.org/10.1371/journal.pone.0304229
Editor: Peter F. Biehl, University of California Santa Cruz, UNITED STATES OF AMERICA
Received: March 9, 2024; Accepted: May 7, 2024; Published: August 7, 2024
Copyright: © 2024 Walsh et al. This is an open access article distributed under the terms of the Creative Commons Attribution License , which permits unrestricted use, distribution, and reproduction in any medium, provided the original author and source are credited.
Data Availability: All relevant data are within the paper and its Supporting Information files.
Funding: JW was the recipient of funding from Chapman University’s Office of Research and Sponsored Programs to support the activities of Axiom Space as implementation partner for the research presented in this article. There are no associated grant numbers for this financial support. Axiom Space served in the role of a contractor hired by Chapman University for the purpose of overseeing logistics relating to our research. In-kind support in the form of ISS crew time and access to the space station’s facilities, also awarded to JW from the ISS National Laboratory, resulted from an unsolicited proposal, and therefore there is no opportunity title or number associated with our work. No salary was received by any of the investigators as a result of the grant support. No additional external funding was received for this study.
Competing interests: The authors have declared that no competing interests exist.
Introduction
The International Space Station Archaeological Project (ISSAP) aims to fill a gap in social science investigation into the human experience of long-duration spaceflight [ 1 – 3 ]. As the largest, most intensively inhabited space station to date, with over 270 visitors from 23 countries during more than 23 years of continuous habitation, the International Space Station (ISS) is the ideal example of a new kind of spacefaring community—“a microsociety in a miniworld” [ 4 ]. While it is possible to interview crew members about their experiences, the value of an approach focused on material culture is that it allows identification of longer-term patterns of behaviors and associations that interlocutors are unable or even unwilling to articulate. In this respect, we are inspired by previous examples of contemporary archaeology such as the Tucson Garbage Project and the Undocumented Migration Project [ 5 – 7 ]. We also follow previous discussions of material culture in space contexts that highlight the social and cultural features of space technology [ 8 , 9 ].
Our primary goal is to identify how humans adapt to life in a new environment for which our species has not evolved, one characterized by isolation, confinement, and especially microgravity. Microgravity introduces opportunities, such as the ability to move and work in 360 degrees, and to carry out experiments impossible in full Earth gravity, but also limitations, as unrestrained objects float away. The most routine activities carried out on Earth become the focus of intense planning and technological intervention in microgravity. By extension, our project also seeks to develop archaeological techniques that permit the study of other habitats in remote, extreme, or dangerous environments [ 10 , 11 ]. Since it is too costly and difficult to visit our archaeological site in person, we have to creatively re-imagine traditional archaeological methods to answer key questions. To date, our team has studied crew-created visual displays [ 12 , 13 ], meanings and processes associated with items returned to Earth [ 14 ], distribution of different population groups around the various modules [ 15 ], and the development of machine learning (ML) computational techniques to extract data about people and places, all from historic photographs of life on the ISS [ 16 ].
From January to March 2022, we developed a new dataset through the first archaeological work conducted off-Earth. We documented material culture in six locations around the ISS habitat, using daily photography taken by the crew which we then annotated and studied as evidence for changes in archaeological assemblages of material culture over time. This was the first time such data had been captured in a way that allowed statistical analysis. Here, we present the data and results from Squares 03 and 05, the first two sample locations to be completed.
Materials and methods
Square concept and planning.
Gorman proposed the concept behind the investigation, deriving it from one of the most traditional terrestrial archaeological techniques, the shovel test pit. This method is used to understand the overall characteristics of a site quickly through sampling. A site is mapped with a grid of one-meter squares. Some of the squares are selected for initial excavation to understand the likely spatial and chronological distribution of features across the entire site. In effect, the technique is a way to sample a known percentage of the entire site systematically. In the ISS application of this method, we documented a notional stratigraphy through daily photography, rather than excavation.
Historic photography is a key dataset for the International Space Station Archaeological Project. Tens of thousands of images have been made available to us, either through publication [ 17 ], or through an arrangement with the ISS Research Integration Office, which supplied previously unpublished images from the first eight years of the station’s habitation. These photographs are informative about the relationships between people, places, and objects over time in the ISS. However, they were taken randomly (from an archaeological perspective) and released only according to NASA’s priorities and rules. Most significantly, they were not made with the purpose of answering archaeological questions. By contrast, the photographs taken during the present investigation were systematic, representative of a defined proportion of the habitat’s area, and targeted towards capturing archaeology’s primary evidence: material culture. We were interested in how objects move around individual spaces and the station, what these movements revealed about crew adherence to terrestrial planning, and the creative use of material culture to make the laboratory-like interior of the ISS more habitable.
Access to the field site was gained through approval of a proposal submitted to the Center for the Advancement of Science in Space (also known as the ISS National Laboratory [ISS NL]). Upon acceptance, Axiom Space was assigned as the Implementation Partner for carriage of the experiment according to standard procedure. No other permits were required for this work.
Experiment design
Since our work envisioned one-meter sample squares, and recognizing the use of acronyms as a persistent element of spacefaring culture, we named our payload the Sampling Quadrangle Assemblages Research Experiment (SQuARE). Permission from the ISS NL to conduct SQuARE was contingent on using equipment that was already on board the space station. SQuARE required only five items: a camera, a wide-angle lens, adhesive tape (for marking the boundaries of the sample locations), a ruler (for scale), and a color calibration card (for post-processing of the images). All of these were already present on the ISS.
Walsh performed tests on the walls of a terrestrial art gallery to assess the feasibility of creating perfect one-meter squares in microgravity. He worked on a vertical surface, using the Pythagorean theorem to determine where the corners should be located. The only additional items used for these tests were two metric measuring tapes and a pencil for marking the wall (these were also already on the ISS). While it was possible to make a square this way, it also became clear that at least two people were needed to manage holding the tape measures in position while marking the points for the corners. This was not possible in the ISS context.
Walsh and Gorman identified seven locations for the placement of squares. Five of these were in the US Orbital Segment (USOS, consisting of American, European, and Japanese modules) and two in the Russian Orbital Segment. Unfortunately, tense relations between the US and Russian governments meant we could only document areas in the USOS. The five locations were (with their SQuARE designations):
- 01—an experimental rack on the forward wall, starboard end, of the Japanese Experiment Module
- 02—an experimental rack on the forward wall, port end, of the European laboratory module Columbus
- 03—the starboard Maintenance Work Area (workstation) in the US Node 2 module
- 04—the wall area “above” (according to typical crew body orientation) the galley table in the US Node 1 module
- 05—the aft wall, center location, of the US Node 3 module
Our square selection encompassed different modules and activities, including work and leisure. We also asked the crew to select a sixth sample location based on their understanding of the experiment and what they thought would be interesting to document. They chose a workstation on the port wall of the US laboratory module, at the aft end, which they described in a debriefing following their return to Earth in June 2022 as “our central command post, like our shared office situation in the lab.” Results from the four squares not included here will appear in future publications.
Walsh worked with NASA staff to determine payload procedures, including precise locations for the placement of the tape that would mark the square boundaries. The squares could not obstruct other facilities or experiments, so (unlike in terrestrial excavations, where string is typically used to demarcate trench boundaries) only the corners of each square were marked, not the entire perimeter. We used Kapton tape due to its bright yellow-orange color, which aided visibility for the crew taking photographs and for us when cropping the images. In practice, due to space constraints, the procedures that could actually be performed by crew in the ISS context, and the need to avoid interfering with other ongoing experiments, none of the locations actually measured one square meter or had precise 90° corners like a trench on Earth.
On January 14, 2022, NASA astronaut Kayla Barron set up the sample locations, marking the beginning of archaeological work in space ( S1 Movie ). For 30 days, starting on January 21, a crew member took photos of the sample locations at approximately the same time each day; the process was repeated at a random time each day for a second 30-day period to eliminate biases. Photography ended on March 21, 2022. The crew were instructed not to move any items prior to taking the photographs. Walsh led image management, including color and barrel distortion correction, fixing the alignment of each image, and cropping them to the boundaries of the taped corners.
Data processing—Item tagging, statistics, visualizations
We refer to each day’s photo as a “context” by analogy with chronologically-linked assemblages of artifacts and installations at terrestrial archaeological sites ( S1 and S2 Datasets). As previously noted, each context represented a moment roughly 24 hours distant from the previous one, showing evidence of changes in that time. ISS mission planners attempted to schedule the activity at the same time in the first month, but there were inevitable changes due to contingencies. Remarkably, the average time between contexts in Phase 1 was an almost-perfect 24h 0m 13s. Most of the Phase 1 photos were taken between 1200 and 1300 GMT (the time zone in which life on the ISS is organized). In Phase 2, the times were much more variable, but the average time between contexts during this period was still 23h 31m 45s. The earliest Phase 2 photo was taken at 0815 GMT, and the latest at 2101. We did not identify any meaningful differences between results from the two phases.
Since the “test pits” were formed of images rather than soil matrices, we needed a tool to capture information about the identity, nature, and location of every object. An open-source image annotator platform [ 18 ] mostly suited our needs. Brousseau rebuilt the platform to work within the constraints of our access to the imagery (turning it into a desktop tool with secure access to our private server), to permit a greater range of metadata to be added to each item or be imported, to autosave, and to export the resulting annotations. The tool also had to respect privacy and security limitations required by NASA.
The platform Brousseau developed and iterated was rechristened “Rocket-Anno” ( S1 File ). For each context photograph, the user draws an outline around every object, creating a polygon; each polygon is assigned a unique ID and the user provides the relevant descriptive information, using a controlled vocabulary developed for ISS material culture by Walsh and Gorman. Walsh and Abdullah used Rocket-Anno to tag the items in each context for Squares 03 and 05. Once all the objects were outlined for every context’s photograph, the tool exported a JSON file with all of the metadata for both the images themselves and all of the annotations, including the coordinate points for every polygon ( S3 Dataset ). We then developed Python code using Jupyter “notebooks” (an interactive development environment) that ingests the JSON file and generates dataframes for various facets of the data. Graham created a “core” notebook that exports summary statistics, calculates Brainerd-Robinson coefficients of similarity, and visualizes the changing use of the square over time by indicating use-areas based on artifact types and subtypes ( S2 File ). Walsh and Abdullah also wrote detailed square notes with context-by-context discussions and interpretations of features and patterns.
We asked NASA for access to the ISS Crew Planner, a computer system that shows each astronaut’s tasks in five-minute increments, to aid with our interpretation of contexts, but were denied. As a proxy, we use another, less detailed source: the ISS Daily Summary Reports (DSRs), published on a semi-regular basis by NASA on its website [ 19 ]. Any activities mentioned in the DSRs often must be connected with a context by inference. Therefore, our conclusions are likely less precise than if we had seen the Crew Planner, but they also more clearly represent the result of simply observing and interpreting the material culture record.
The crew during our sample period formed ISS Expedition 66 (October 2021-March 2022). They were responsible for the movement of objects in the sample squares as they carried out their daily tasks. The group consisted of two Russians affiliated with Roscosmos (the Russian space agency, 26%), one German belonging to the European Space Agency (ESA, 14%), and four Americans employed by NASA (57%). There were six men (86%) and one woman (14%), approximately equivalent to the historic proportions in the ISS population (84% and 16%, respectively). The Russian crew had their sleeping quarters at the aft end of the station, in the Zvezda module. The ESA astronaut slept in the European Columbus laboratory module. The four NASA crew slept in the US Node 2 module (see below). These arrangements emphasize the national character of discrete spaces around the ISS, also evident in our previous study of population distributions [ 15 ]. Both of the sample areas in this study were located in US modules.
Square 03 was placed in the starboard Maintenance Work Area (MWA, Fig 1 ), one of a pair of workstations located opposite one another in the center of the Node 2 module, with four crew berths towards the aft and a series of five ports for the docking of visiting crew/cargo vehicles and two modules on the forward end ( Fig 2 ). Node 2 (sometimes called “Harmony”) is a connector that links the US, Japanese, and European lab modules. According to prevailing design standards when the workstation was developed, an MWA “shall serve as the primary location for servicing and repair of maximum sized replacement unit/system components” [ 20 ]. Historic images published by NASA showing its use suggested that its primary function was maintenance of equipment and also scientific work that did not require a specific facility such as a centrifuge or furnace.
- PPT PowerPoint slide
- PNG larger image
- TIFF original image
An open crew berth is visible at right. The yellow dotted line indicates the boundaries of the sample area. Credit: NASA/ISSAP.
https://doi.org/10.1371/journal.pone.0304229.g001
Credit: Tor Finseth, by permission, modified by Justin Walsh.
https://doi.org/10.1371/journal.pone.0304229.g002
Square 03 measured 90.3 cm (top) x 87.8 (left) x 89.4 (bottom) x 87.6 (right), for an area of approximately 0.79 m 2 . Its primary feature was a blue metal panel with 40 square loop-type Velcro patches arranged in four rows of ten. During daily photography, many items were attached to the Velcro patches (or held by a clip or in a resealable bag which had its own hook-type Velcro). Above and below the blue panel were additional Velcro patches placed directly on the white plastic wall surface. These patches were white, in different sizes and shapes and irregularly arranged, indicating that they had been placed on the wall in response to different needs. Some were dirty, indicating long use. The patches below the blue panel were rarely used during the sample period, but the patches above were used frequently to hold packages of wet wipes, as well as resealable bags with electrostatic dispersion kits and other items. Outside the sample area, the primary features were a crew berth to the right, and a blue metal table attached to the wall below. This table, the primary component of the MWA, “provides a rigid surface on which to perform maintenance tasks,” according to NASA [ 21 ]. It is modular and can be oriented in several configurations, from flat against the wall to horizontal ( i . e ., perpendicular to the wall). A laptop to the left of the square occasionally showed information about work happening in the area.
In the 60 context photos of Square 03, we recorded 3,608 instances of items, an average of 60.1 (median = 60.5) per context. The lowest count was 24 in context 2 (where most of the wall was hidden from view behind an opaque storage bag), and the highest was 75 in both contexts 20 and 21. For comparison between squares, we can also calculate the item densities per m 2 . The average count was 76.1/m 2 (minimum = 30, maximum = 95). The count per context ( Fig 3(A)) began much lower than average in the first three contexts because of a portable glovebag and a stowage bag that obscured much of the sample square. It rose to an above-average level which was sustained (with the exception of contexts 11 and 12, which involved the appearance of another portable glovebag) until about context 43, when the count dipped again and the area seemed to show less use. Contexts 42–59 showed below-average numbers, as much as 20% lower than previously.
(a) Count of artifacts in Square 03 over time. (b) Proportions of artifacts by function in Square 03. Credit: Rao Hamza Ali.
https://doi.org/10.1371/journal.pone.0304229.g003
74 types of items appeared at least once here, belonging to six categories: equipment (41%), office supplies (31%), electronic (17%), stowage (9%), media (1%), and food (<1%). To better understand the significance of various items in the archaeological record, we assigned them to functional categories ( Table 1 , Fig 3(B)) . 35% of artifacts were restraints, or items used for holding other things in place; 12% for tools; 9% for containers; 9% for writing items; 6% for audiovisual items; 6% for experimental items; 4% for lights; 4% for safety items; 4% for body maintenance; 4% for power items; 3% for computing items; 1% for labels; and less than 1% drinks. We could not identify a function for two percent of the items.
https://doi.org/10.1371/journal.pone.0304229.t001
One of the project goals is understanding cultural adaptations to the microgravity environment. We placed special attention on “gravity surrogates,” pieces of (often simple) technology that are used in space to replicate the terrestrial experience of things staying where they are placed. Gravity surrogates include restraints and containers. It is quite noticeable that gravity surrogates comprise close to half of all items (44%) in Square 03, while the tools category, which might have been expected to be most prominent in an area designated for maintenance, is less than one-third as large (12%). Adding other groups associated with work, such as “experiment” and “light,” only brings the total to 22%.
Square 05 (Figs 2 and 4 ) was placed in a central location on the aft wall of the multipurpose Node 3 (“Tranquility”) module. This module does not include any specific science facilities. Instead, there are two large pieces of exercise equipment, the TVIS (Treadmill with Vibration Isolation Stabilization System, on the forward wall at the starboard end), and the ARED (Advanced Resistive Exercise Device, on the overhead wall at the port end). Use of the machines forms a significant part of crew activities, as they are required to exercise for two hours each day to counteract loss of muscle mass and bone density, and enable readjustment to terrestrial gravity on their return. The Waste and Hygiene Compartment (WHC), which includes the USOS latrine, is also here, on the forward wall in the center of the module, opposite Square 05. Finally, three modules are docked at Node 3’s port end. Most notable is the Cupola, a kind of miniature module on the nadir side with a panoramic window looking at Earth. This is the most popular leisure space for the crew, who often describe the hours they spend there. The Permanent Multipurpose Module (PMM) is docked on the forward side, storing equipment, food, and trash. In previous expeditions, some crew described installing a curtain in the PMM to create a private space for changing clothes and performing body maintenance activities such as cleaning oneself [ 22 , 23 ], but it was unclear whether that continued to be its function during the expedition we observed. One crew member during our sample period posted a video on Instagram showing the PMM interior and their efforts to re-stow equipment in a bag [ 24 ]. The last space attached to Node 3 is an experimental inflatable module docked on the aft side, called the Bigelow Expandable Activity Module (BEAM), which is used for storage of equipment.
The yellow dotted line indicates the boundaries of the sample area. The ARED machine is at the far upper right, on the overhead wall. The TVIS treadmill is outside this image to the left, on the forward wall. The WHC is directly behind the photographer. Credit: NASA/ISSAP.
https://doi.org/10.1371/journal.pone.0304229.g004
Square 05 was on a mostly featureless wall, with a vertical handrail in the middle. Handrails are metal bars located throughout the ISS that are used by the crew to hold themselves in place or provide a point from which to propel oneself to another location. NASA’s most recent design standards acknowledge that “[t]hey also serve as convenient locations for temporary mounting, affixing, or restraint of loose equipment and as attachment points for equipment” [ 25 ]. The handrail in Square 05 was used as an impromptu object restraint when a resealable bag filled with other bags was squeezed between the handrail and the wall.
The Brine Processing Assembly (BPA), a white plastic box which separates water from other components of urine for treatment and re-introduction to the station’s drinkable water supply [ 26 ], was fixed to the wall outside the square boundaries at lower left. A bungee cord was attached to both sides of the box; the one on the right was connected at its other end to the handrail attachment bracket. Numerous items were attached to or wedged into this bungee cord during the survey, bringing “gravity” into being. A red plastic duct ran through the square from top center into the BPA. This duct led from the latrine via the overhead wall. About halfway through the survey period, in context 32, the duct was wrapped in Kapton tape. According to the DSR for that day, “the crew used duct tape [ sic ] to make a seal around the BPA exhaust to prevent odor permeation in the cabin” [ 27 ], revealing an aspect of the crew’s experience of this area that is captured only indirectly in the context photograph. Permanently attached to the wall were approximately 20 loop-type Velcro patches in many shapes and sizes, placed in a seemingly random pattern that likely indicates that they were put there at different times and for different reasons.
Other common items in Square 05 were a mirror, a laptop computer, and an experimental item belonging to the German space agency DLR called the Touch Array Assembly [ 28 ]. The laptop moved just three times, and only by a few centimeters each time, during the sample period. The Touch Array was a black frame enclosing three metal surfaces which were being tested for their bacterial resistance; members of the crew touched the surfaces at various moments during the sample period. Finally, and most prominent due to its size, frequency of appearance, and use (judged by its movement between context photos) was an unidentified crew member’s toiletry kit.
By contrast with Square 03, 05 was the most irregular sample location, roughly twice as wide as it was tall. Its dimensions were 111 cm (top) x 61.9 (left) x 111.4 (bottom) x 64.6 (right), for an area of approximately 0.7 m 2 , about 89% of Square 03. We identified 1,830 instances of items in the 60 contexts, an average of 30.5 (median = 32) per context. The minimum was 18 items in context 5, and the maximum was 39 in contexts 24, 51, and 52. The average item density was 43.6/m 2 (minimum = 26, maximum = 56), 57% of Square 03.
The number of items trended upward throughout the sample period ( Fig 5(A)) . The largest spike occurred in context 6 with the appearance of the toiletry kit, which stored (and revealed) a number of related items. The kit can also be linked to one of the largest dips in item count, seen from contexts 52 to 53, when it was closed (but remained in the square). Other major changes can often be attributed to the addition and removal of bungee cords, which had other items such as carabiners and brackets attached. For example, the dip seen in context 25 correlates with the removal of a bungee cord with four carabiners.
(a) Count of artifacts and average count in Square 05 over time. (b) Proportions of artifacts by function in Square 05. Credit: Rao Hamza Ali.
https://doi.org/10.1371/journal.pone.0304229.g005
41 different item types were found in Square 05, about 55% as many as in Square 03. These belonged to five different categories: equipment (63%), electronic (17%), stowage (10%), office supplies (5%), and food (2%). The distribution of function proportions was quite different in this sample location ( Table 2 and Fig 5(B)) . Even though restraints were still most prominent, making up 32% of all items, body maintenance was almost as high (30%), indicating how strongly this area was associated with the activity of cleaning and caring for oneself. Computing (8%, represented by the laptop, which seems not to have been used), power (8%, from various cables), container (7%, resealable bags and Cargo Transfer Bags), and hygiene (6%, primarily the BPA duct) were the next most common items. Experiment was the function of 4% of the items, mostly the Touch Array, which appeared in every context, followed by drink (2%) and life support (1%). Safety, audiovisual, food, and light each made up less than 1% of the functional categories.
https://doi.org/10.1371/journal.pone.0304229.t002
Tracking changes over time is critical to understanding the activity happening in each area. We now explore how the assemblages change by calculating the Brainerd-Robinson Coefficient of Similarity [ 29 , 30 ] as operationalized by Peeples [ 31 , 32 ]. This metric is used in archaeology for comparing all pairs of the contexts by the proportions of categorical artifact data, here functional type. Applying the coefficient to the SQuARE contexts enables identification of time periods for distinct activities using artifact function and frequency alone, independent of documentary or oral evidence.
Multiple phases of activities took place in the square. Moments of connected activity are visible as red clusters in contexts 0–2, 11–12, 28–32, and 41 ( Fig 6(A)) . Combining this visualization with close observation of the photos themselves, we argue that there are actually eight distinct chronological periods.
- Contexts 0–2: Period 1 (S1 Fig in S3 File ) is a three-day period of work involving a portable glovebag (contexts 0–1) and a large blue stowage bag (context 2). It is difficult to describe trends in functional types because the glovebag and stowage bag obstruct the view of many objects. Items which appear at the top of the sample area, such as audiovisual and body maintenance items, are overemphasized in the data as a result. It appears that some kind of science is happening here, perhaps medical sample collection due to the presence of several small resealable bags visible in the glovebag. The work appears particularly intense in context 1, with the positioning of the video camera and light to point into the glovebag. These items indicate observation and oversight of crew activities by ground control. A white cargo transfer bag for storage and the stowage bag for holding packing materials in the context 2 photo likely relate to the packing of a Cargo Dragon vehicle that was docked to Node 2. The Dragon departed from the ISS for Earth, full of scientific samples, equipment, and crew personal items, a little more than three hours after the context 2 photo was taken [ 33 ].
- Contexts 3–10: Period 2 (S2 Fig in S3 File ) was a “stable” eight-day period in the sample, when little activity is apparent, few objects were moved or transferred in or out the square, and the primary function of the area seems to be storage rather than work. In context 6, a large Post-It notepad appeared in the center of the metal panel with a phone number written on it. This number belonged to another astronaut, presumably indicating that someone on the ISS had been told to call that colleague on the ground (for reasons of privacy, and in accordance with NASA rules for disseminating imagery, we have blurred the number in the relevant images). In context 8, the same notepad sheet had new writing appear on it, this time reading “COL A1 L1,” the location of an experimental rack in the European lab module.
- Contexts 11–12: Period 3 (S3 Fig in S3 File ) involves a second appearance of a portable glovebag (a different one from that used in contexts 0–1, according to its serial number), this time for a known activity, a concrete hardening experiment belonging to the European Space Agency [ 34 , 35 ]. This two-day phase indicates how the MWA space can be shared with non-US agencies when required. It also demonstrates the utility of this flexible area for work beyond biology/medicine, such as material science. Oversight of the crew’s activities by ground staff is evident from the positioning of the video camera and LED light pointing into the glovebag.
- Contexts 13–27: Period 4 (S4 Fig in S3 File ) is another stable fifteen-day period, similar to Period 2. Many items continued to be stored on the aluminum panel. The LED light’s presence is a trace of the activity in Period 3 that persists throughout this phase. Only in context 25 can a movement of the lamp potentially be connected to an activity relating to one of the stored items on the wall: at least one nitrile glove was removed from a resealable bag behind the lamp. In general, the primary identifiable activity during Period 4 is storage.
- Contexts 28–32: Period 5 (S5 Fig in S3 File ), by contrast, represents a short period of five days of relatively high and diverse activity. In context 28, a Microsoft Hololens augmented reality headset appeared. According to the DSR for the previous day, a training activity called Sidekick was carried out using the headset [ 36 ]. The following day, a Saturday, showed no change in the quantity or type of objects, but many were moved around and grouped by function—adhesive tape rolls were placed together, tools were moved from Velcro patches into pouches or straightened, and writing implements were placed in a vertical orientation when previously they were tilted. Context 29 represents a cleaning and re-organization of the sample area, which is a common activity for the crew on Saturdays [ 37 ]. Finally, in context 32, an optical coherence tomography scanner—a large piece of equipment for medical research involving crew members’ eyes—appeared [ 38 ]. This device was used previously during the sample period, but on the same day as the ESA concrete experiment, so that earlier work seems to have happened elsewhere [ 39 ].
- Contexts 33–40: Period 6 (S6 Fig in S3 File ) is the third stable period, in which almost no changes are visible over eight days. The only sign of activity is a digital timer which was started six hours before the context 39 image was made and continued to run at least through context 42.
- Context 41: Period 7 (S7 Fig in S3 File ) is a single context in which medical sample collection may have occurred. Resealable bags (some holding others) appeared in the center of the image and at lower right. One of the bags at lower right had a printed label reading “Reservoir Containers.” We were not able to discern which type of reservoir containers the label refers to, although the DSR for the day mentions “[Human Research Facility] Generic Saliva Collection,” without stating the location for this work [ 40 ]. Evidence from photos of other squares shows that labeled bags could be re-used for other purposes, so our interpretation of medical activity for this context is not conclusive.
- Contexts 42–60: Period 8 (S8 Fig in S3 File ) is the last and longest period of stability and low activity—eighteen days in which no specific activity other than the storage of items can be detected. The most notable change is the appearance for the first time of a foil water pouch in the central part of the blue panel.
Visualization of Brainerd-Robinson similarity, compared context-by-context by item function, for (a) Square 03 and (b) Square 05. The more alike a pair of contexts is, the higher the coefficient value, with a context compared against itself where a value of 200 equals perfect similarity. The resulting matrix of coefficients is visualized on a scale from blue to red where blue is lowest and red is highest similarity. The dark red diagonal line indicates complete similarity, where each context is compared to itself. Dark blue represents a complete difference. Credit: Shawn Graham.
https://doi.org/10.1371/journal.pone.0304229.g006
In the standards used at the time of installation, “stowage space” was the sixth design requirement listed for the MWA after accessibility; equipment size capability; scratch-resistant surfaces; capabilities for electrical, mechanical, vacuum, and fluid support during maintenance; and the accommodation of diagnostic equipment [ 20 ]. Only capabilities for fabrication were listed lower than stowage. Yet 50 of the 60 contexts (83%) fell within stable periods where little or no activity is identifiable in Square 03. According to the sample results, therefore, this area seems to exist not for “maintenance,” but primarily for the storage and arrangement of items. The most recent update of the design standards does not mention the MWA, but states, “Stowage location of tool kits should be optimized for accessibility to workstations and/or maintenance workbenches” [ 25 ]. Our observation confirms the importance of this suggestion.
The MWA was also a flexible location for certain science work, like the concrete study or crew health monitoring. Actual maintenance of equipment was hardly in evidence in the sample (possibly contexts 25, 39, and 44), and may not even have happened at all in this location. Some training did happen here, such as review of procedures for the Electromagnetic Levitator camera (instructions for changing settings on a high-speed camera appeared on the laptop screen; the day’s DSR shows that this camera is part of the Electromagnetic Levitator facility, located in the Columbus module [ 41 ]. The training required the use of the Hololens system (context 28 DSR, cited above).
Although many item types were represented in Square 03, it became clear during data capture how many things were basically static, unmoving and therefore unused, especially certain tools, writing implements, and body maintenance items. The MWA was seen as an appropriate place to store these items. It may be the case that their presence here also indicates that their function was seen as an appropriate one for this space, but the function(s) may not be carried out—or perhaps not in this location. Actualization of object function was only visible to us when the state of the item changed—it appeared, it moved, it changed orientation, it disappeared, or, in the case of artifacts that were grouped in collections rather than found as singletons, its shape changed or it became visibly smaller/lesser. We therefore have the opportunity to explore not only actuality of object use, but also potentiality of use or function, and the meaning of that quality for archaeological interpretation [ 42 , 43 ]. This possibility is particularly intriguing in light of the archaeological turn towards recognizing the agency of objects to impact human activity [ 44 , 45 ]. We will explore these implications in a future publication.
We performed the same chronological analysis for Square 05. Fig 6(B) represents the analysis for both item types and for item functions. We identified three major phases of activity, corresponding to contexts 0–5, 6–52, and 53–59 (S9-S11 Figs in S3 File ). The primary characteristics of these phases relate to an early period of unclear associations (0–5) marked by the presence of rolls of adhesive tape and a few body maintenance items (toothpaste and toothbrush, wet wipes); the appearance of a toiletry kit on the right side of the sample area, fully open with clear views of many of the items contained within (6–52); and finally, the closure of the toiletry kit so that its contents can no longer be seen (53–59). We interpret the phases as follows:
- Contexts 0–5: In Period 1 (six days, S9 Fig in S3 File ), while items such as a mirror, dental floss picks, wet wipes, and a toothbrush held in the end of a toothpaste tube were visible, the presence of various other kinds of items confounds easy interpretation. Two rolls of duct tape were stored on the handrail in the center of the sample area, and the Touch Array and laptop appeared in the center. Little movement can be identified, apart from a blue nitrile glove that appeared in context 1 and moved left across the area until it was wedged into the bungee cord for contexts 3 and 4. The tape rolls were removed prior to context 5. A collection of resealable bags was wedged behind the handrail in context 3, remaining there until context 9. Overall, this appears to be a period characterized by eclectic associations, showing an area without a clear designated function.
- Contexts 6–52: Period 2 (S10 Fig in S3 File ) is clearly the most significant one for this location due to its duration (47 days). It was dominated by the number of body maintenance items located in and around the toiletry kit, especially a white hand towel (on which a brown stain was visible from context 11, allowing us to confirm that the same towel was present until context 46). A second towel appeared alongside the toiletry kit in context 47, and the first one was fixed at the same time to the handrail, where it remained through the end of the sample period. A third towel appeared in context 52, attached to the handrail together with the first one by a bungee cord, continuing to the end of the sample period. Individual body maintenance items moved frequently from one context to the next, showing the importance of this type of activity for this part of Node 3. For reasons that are unclear, the mirror shifted orientation from vertical to diagonal in context 22, and then was put back in a vertical orientation in context 31 (a Monday, a day which is not traditionally associated with cleaning and organization). Collections of resealable bags appeared at various times, including a large one labeled “KYNAR BAG OF ZIPLOCKS” in green marker at the upper left part of the sample area beginning of context 12 (Kynar is a non-flammable plastic material that NASA prefers for resealable bags to the generic commercial off-the-shelf variety because it is non-flammable; however, its resistance to heat makes it less desirable for creating custom sizes, so bags made from traditional but flammable low-density polyethylene still dominate on the ISS [ 14 ]). The Kynar bag contained varying numbers of bags within it over time; occasionally, it appeared to be empty. The Touch Array changed orientation on seven of 47 days in period 2, or 15% of the time (12% of all days in the survey), showing activity associated with scientific research in this area. In context 49, a life-support item, the Airborne Particulate Monitor (APM) was installed [ 46 ]. This device, which measures “real-time particulate data” to assess hazards to crew health [ 47 ], persisted through the end of the sample period.
- Contexts 53–59: Period 3 (S11 Fig in S3 File ) appears as a seven-day phase marked by low activity. Visually, the most notable feature is the closure of the toiletry kit, which led to much lower counts of body maintenance items. Hardly any of the items on the wall moved at all during this period.
While body maintenance in the form of cleaning and caring for oneself could be an expected function for an area with exercise and excretion facilities, it is worth noting that the ISS provides, at most, minimal accommodation for this activity. A description of the WHC stated, “To provide privacy…an enclosure was added to the front of the rack. This enclosure, referred to as the Cabin, is approximately the size of a typical bathroom stall and provides room for system consumables and hygiene item stowage. Space is available to also support limited hygiene functions such as hand and body washing” [ 48 ]. A diagram of the WHC in the same publication shows the Cabin without a scale but suggests that it measures roughly 2 m (h) x .75 (w) x .75 (d), a volume of approximately 1.125 m 3 . NASA’s current design standards state that the body volume of a 95th percentile male astronaut is 0.99 m 3 [ 20 ], meaning that a person of that size would take up 88% of the space of the Cabin, leaving little room for performing cleaning functions—especially if the Cabin is used as apparently intended, to also hold “system consumables and hygiene item[s]” that would further diminish the usable volume. This situation explains why crews try to adapt other spaces, such as storage areas like the PMM, for these activities instead. According to the crew debriefing statement, only one of them used the WHC for body maintenance purposes; it is not clear whether the toiletry kit belonged to that individual. But the appearance of the toiletry kit in Square 05—outside of the WHC, in a public space where others frequently pass by—may have been a response to the limitations of the WHC Cabin. It suggests a need for designers to re-evaluate affordances for body maintenance practices and storage for related items.
Although Square 03 and 05 were different sizes and shapes, comparing the density of items by function shows evidence of their usage ( Table 3 ). The typical context in Square 03 had twice as many restraints and containers, but less than one-quarter as many body maintenance items as Square 05. 03 also had many tools, lights, audiovisual equipment, and writing implements, while there were none of any of these types in 05. 05 had life support and hygiene items which were missing from 03. It appears that flexibility and multifunctionality were key elements for 03, while in 05 there was emphasis on one primary function (albeit an improvised one, designated by the crew rather than architects or ground control), cleaning and caring for one’s body, with a secondary function of housing static equipment for crew hygiene and life support.
https://doi.org/10.1371/journal.pone.0304229.t003
As this is the first time such an analysis has been performed, it is not yet possible to say how typical or unusual these squares are regarding the types of activities taking place; but they provide a baseline for eventual comparison with the other four squares and future work on ISS or other space habitats.
Some general characteristics are revealed by archaeological analysis of a space station’s material culture. First, even in a small, enclosed site, occupied by only a few people over a relatively short sample period, we can observe divergent patterns for different locations and activity phases. Second, while distinct functions are apparent for these two squares, they are not the functions that we expected prior to this research. As a result, our work fulfills the promise of the archaeological approach to understanding life in a space station by revealing new, previously unrecognized phenomena relating to life and work on the ISS. There is now systematically recorded archaeological data for a space habitat.
Squares 03 and 05 served quite different purposes. The reasons for this fact are their respective affordances and their locations relative to activity areas designated for science and exercise. Their national associations, especially the manifestation of the control wielded by NASA over its modules, also played a role in the use of certain materials, the placement of facilities, and the organization of work. How each area was used was also the result of an interplay between the original plans developed by mission planners and habitat designers (or the lack of such plans), the utility of the equipment and architecture in each location, and the contingent needs of the crew as they lived in the station. This interplay became visible in the station’s material culture, as certain areas were associated with particular behaviors, over time and through tradition—over the long duration across many crews (Node 2, location of Square 03, docked with the ISS in 2007, and Node 3, location of Square 05, docked in 2010), and during the specific period of this survey, from January to March 2022. During the crew debriefing, one astronaut said, “We were a pretty organized crew who was also pretty much on the same page about how to do things…. As time went on…we organized the lab and kind of got on the same page about where we put things and how we’re going to do things.” This statement shows how functional associations can become linked to different areas of the ISS through usage and mutual agreement. At the same time, the station is not frozen in time. Different people have divergent ideas about how and where to do things. It seems from the appearance of just one Russian item—a packet of generic wipes ( salfetky sukhiye ) stored in the toiletry kit throughout the sample period—that the people who used these spaces and carried out their functions did not typically include the ISS’s Russian crew. Enabling greater flexibility to define how spaces can be used could have a significant impact on improving crew autonomy over their lives, such as how and where to work. It could also lead to opening of all spaces within a habitat to the entire crew, which seems likely to improve general well-being.
An apparent disjunction between planned and actual usage appeared in Square 03. It is intended for maintenance as well as other kinds of work. But much of the time, there was nobody working here—a fact that is not captured by historic photos of the area, precisely because nothing is happening. The space has instead become the equivalent of a pegboard mounted on a wall in a home garage or shed, convenient for storage for all kinds of items—not necessarily items being used there—because it has an enormous number of attachment points. Storage has become its primary function. Designers of future workstations in space should consider that they might need to optimize for functions other than work, because most of the time, there might not be any work happening there. They could optimize for quick storage, considering whether to impose a system of organization, or allow users to organize as they want.
We expected from previous (though unsystematic) observation of historic photos and other research, that resealable plastic bags (combined with Velcro patches on the bags and walls) would be the primary means for creating gravity surrogates to control items in microgravity. They only comprise 7% of all items in Square 03 (256 instances). There are more than twice as many clips (572—more than 9 per context) in the sample. There were 193 instances of adhesive tape rolls, and more than 100 cable ties, but these were latent (not holding anything), representing potentiality of restraint rather than actualization. The squares showed different approaches to managing “gravity.” While Square 03 had a pre-existing structured array of Velcro patches, Square 05 showed a more expedient strategy with Velcro added in response to particular activities. Different needs require different affordances; creating “gravity” is a more nuanced endeavor than it initially appears. More work remains to be done to optimize gravity surrogates for future space habitats, because this is evidently one of the most critical adaptations that crews have to make in microgravity (44% of all items in Square 03, 39% in 05).
Square 05 is an empty space, seemingly just one side of a passageway for people going to use the lifting machine or the latrine, to look out of the Cupola, or get something out of deep storage in one of the ISS’s closets. In our survey, this square was a storage place for toiletries, resealable bags, and a computer that never (or almost never) gets used. It was associated with computing and hygiene simply by virtue of its location, rather than due to any particular facilities it possessed. It has no affordances for storage. There are no cabinets or drawers, as would be appropriate for organizing and holding crew personal items. A crew member decided that this was an appropriate place to leave their toiletry kit for almost two months. Whether this choice was appreciated or resented by fellow crew members cannot be discerned based on our evidence, but it seems to have been tolerated, given its long duration. The location of the other four USOS crew members’ toiletry kits during the sample period is unknown. A question raised by our observations is: how might a function be more clearly defined by designers for this area, perhaps by providing lockers for individual crew members to store their toiletries and towels? This would have a benefit not only for reducing clutter, but also for reducing exposure of toiletry kits and the items stored in them to flying sweat from the exercise equipment or other waste particles from the latrine. A larger compartment providing privacy for body maintenance and a greater range of motion would also be desirable.
As the first systematic collection of archaeological data from a space site outside Earth, this analysis of two areas on the ISS as part of the SQuARE payload has shown that novel insights into material culture use can be obtained, such as the use of wall areas as storage or staging posts between activities, the accretion of objects associated with different functions, and the complexity of using material replacements for gravity. These results enable better space station design and raise new questions that will be addressed through analysis of the remaining four squares.
Supporting information
S1 movie. nasa astronaut kayla barron installs the first square for the sampling quadrangle assemblages research experiment in the japanese experiment module (also known as kibo) on the international space station, january 14, 2022..
She places Kapton tape to mark the square’s upper right corner. Credit: NASA.
https://doi.org/10.1371/journal.pone.0304229.s001
S1 Dataset.
https://doi.org/10.1371/journal.pone.0304229.s002
S2 Dataset.
https://doi.org/10.1371/journal.pone.0304229.s003
S3 Dataset. The image annotations are represented according to sample square in json formatted text files.
The data is available in the ‘SQuARE-notebooks’ repository on Github.com in the ‘data’ subfolder at https://github.com/issarchaeologicalproject/SQuARE-notebooks/tree/main ; archived version of the repository is at Zenodo, DOI: 10.5281/zenodo.10654812 .
https://doi.org/10.1371/journal.pone.0304229.s004
S1 File. The ‘Rocket-Anno’ image annotation software is available on Github at https://github.com/issarchaeologicalproject/MRE-RocketAnno .
The archived version of the repository is at Zenodo, DOI: 10.5281/zenodo.10648399 .
https://doi.org/10.1371/journal.pone.0304229.s005
S2 File. The computational notebooks that process the data json files to reshape the data suitable for basic statistics as well as the computation of the Brainerd-Robinson coefficients of similarity are in the.ipynb notebook format.
The code is available in the ‘SQuARE-notebooks’ repository on Github.com in the ‘notebooks’ subfolder at https://github.com/issarchaeologicalproject/SQuARE-notebooks/tree/main ; archived version of the repository is at Zenodo, DOI: 10.5281/zenodo.10654812 . The software can be run online in the Google Colab environment ( https://colab.research.google.com ) or any system running Jupyter Notebooks ( https://jupyter.org/ ).
https://doi.org/10.1371/journal.pone.0304229.s006
https://doi.org/10.1371/journal.pone.0304229.s007
Acknowledgments
We thank Chapman University’s Office of Research and Sponsored Programs, and especially Dr. Thomas Piechota and Dr. Janeen Hill, for funding the Implementation Partner costs associated with the SQuARE payload. Chapman’s Leatherby Libraries’ Supporting Open Access Research and Scholarship (SOARS) program funded the article processing fee for this publication. Ken Savin and Ken Shields at the ISS National Laboratory gave major support by agreeing to sponsor SQuARE and providing access to ISS NL’s allocation of crew time. David Zuniga and Kryn Ambs at Axiom Space were key collaborators in managing payload logistics. NASA staff and contractors were critical to the experiment’s success, especially Kristen Fortson, Jay Weber, Crissy Canerday, Sierra Wolbert, and Jade Conway. We also gratefully acknowledge the help and resources provided by Dr. Erik Linstead, director of the Machine Learning and Affiliated Technology Lab at Chapman University. Aidan St. P. Walsh corrected the color and lens barrel distortion in all of the SQuARE imagery. Rao Hamza Ali produced charts using accessible color combinations for Figs 3 and 5 . And finally, of course, we are extremely appreciative of the efforts of the five USOS members of the Expedition 66 crew on the ISS—Kayla Barron, Raja Chari, Thomas Marshburn, Matthias Maurer, and Mark Vande Hei—who were the first archaeologists in space.
- 1. Buchli V. Extraterrestrial methods: Towards an ethnography of the ISS. In: Carroll T, Walford A, Walton S, editors. Lineages and advancements in material culture studies: Perspectives from UCL anthropology. London: Routledge; 2021, pp. 17–32.
- 2. Gorman A, Walsh J. Archaeology in a vacuum: obstacles to and solutions for developing a real space archaeology. In: Barnard H, editor. Archaeology outside the box: investigations at the edge of the discipline. Los Angeles, Cotsen Institute of Archaeology Press; 2023. pp. 131–123.
- 3. Walsh J. Adapting to space: The International Space Station Archaeological Project. In: Salazar Sutil JF, Gorman A, editors. Routledge handbook of social studies of outer space. London, Routledge; 2023. pp. 400–412. https://doi.org/10.4324/9781003280507-37
- View Article
- Google Scholar
- 6. Rathje W, Murphy C. Rubbish! The archaeology of garbage Tucson: University of Arizona Press; 2001.
- 7. De León J. The land of open graves: living and dying on the migrant trail. Berkeley, University of California Press; 2015.
- 8. Garrison Darrin A, O’Leary B, editors. Handbook of space engineering, archaeology, and heritage. Boca Raton, CRC Press; 2009.
- 9. Capelotti PJ. The human archaeology of space: Lunar, planetary, and interstellar relics of exploration. Jefferson, NC, McFarland Press; 2010.
- 11. Gorman A. Space and time through material culture: An account of space archaeology. In: Salazar Sutil JF, Gorman A, editors. Routledge handbook of social studies of outer space. London, Routledge; 2023. pp. 44–56. https://doi.org/10.4324/9781003280507-5
- 17. NASA. NASA Johnson. 2008 Aug [cited May 12 2024]. In: Flickr [Internet]. San Francisco. Available from https://www.flickr.com/photos/nasa2explore/
- 19. NASA. ISS Daily Status Reports. 2012 Mar 1 [Cited May 12 2024]. Available from: https://blogs.nasa.gov/stationreport/
- 20. NASA. Man-systems integration. STD-3000 Vol. 1. Houston, NASA Johnson; 1995, pp. 9–15, 78
- 21. NASA. Maintenance Work Area | Glenn Research Center. 2020 Mar 6 [cited May 12 2024]. Available from: https://www1.grc.nasa.gov/space/iss-research/mwa/
- 22. Cristoforetti S. Diario di un’apprendista astronauta. Milan, Le Polene; 2018. pp. 379.
- 23. Kelly S. Endurance: A year in space, a lifetime of discovery. New York, Knopf; 2017. pp. 175, 285–86.
- 24. Barron K. Instagram post, 2022 Feb 12 [cited 2024 May 12]. Available from: https://www.instagram.com/tv/CZ4pW9HJ2Wg/?igsh=ZDE1MWVjZGVmZQ==
- 25. NASA. NASA space flight human-system standard. STD-3001 Volume 1: Human integration design handbook. Rev. 1 Houston, NASA Johnson; 2014. pp. 814, 829–833.
- 27. Keeter B. ISS daily summary report– 2/21/2022. 2022 Feb 21 [cited May 12 2024]. In: NASA ISS On-Orbit Status Report blog [Internet]. Houston. Available from: https://blogs.nasa.gov/stationreport/2022/02/page/6/
- 28. DLR. Fingerprint research to combat harmful bacteria. 2022 Jan 18 [cited May 12 2024]. Available from: https://www.dlr.de/en/latest/news/2022/01/20220118_fingerprint-research-to-combat-harmful-bacteria
- 31. Peeples MA. R script for calculating the Brainerd-Robinson coefficient of similarity and assessing sampling error. 2011 [cited May 12 2024]. Available from: http://www.mattpeeples.net/br.html .
- 33. Garcia M. Cargo Dragon Splashes Down Ending SpaceX CRS-24 Mission. 2022 Jan 24 [cited May 12 2024]. NASA Space Station blog [Internet]. Available from: https://blogs.nasa.gov/spacestation/2022/01/24/cargo-dragon-splashes-down-ending-spacex-crs-24-mission/
- 34. ESA. Concrete Hardening | Cosmic Kiss 360°. 2022 Mar 5 [cited May 12 2024]. Available from: https://www.esa.int/ESA_Multimedia/Videos/2022/05/Concrete_Hardening_Cosmic_Kiss_360
- 35. Keeter B. ISS daily summary report– 2/01/2022. 2022 Feb 1 [cited May 12 2024]. In: NASA ISS On-Orbit Status Report blog [Internet]. Houston. Available from: https://blogs.nasa.gov/stationreport/2022/02/page/19/
- 36. Keeter B. ISS daily summary report– 2/17/2022. 2022 Feb 17 [cited May 12 2024]. In: NASA ISS On-Orbit Status Report blog [Internet]. Houston. Available from: https://blogs.nasa.gov/stationreport/2022/02/page/8/
- 37. T. Pultarova, How Do You Clean a Space Station? Astronaut Thomas Pesquet Shares Orbital Spring Cleaning Tips, Space.com, May 6, 2021. Online at https://www.space.com/space-station-cleaning-tips-astronaut-thomas-pesquet
- 38. Keeter B. ISS daily summary report– 2/22/2022. 2022 Feb 22 [cited May 12 2024]. In: NASA ISS On-Orbit Status Report blog [Internet]. Houston. Available from: https://blogs.nasa.gov/stationreport/2022/02/page/5/
- 39. Keeter B. ISS daily summary report– 2/02/2022. 2022 Feb 2 [cited May 12 2024]. NASA ISS On-Orbit Status Report blog [Internet]. Houston. Online at https://blogs.nasa.gov/stationreport/2022/02/page/18/
- 40. Keeter B. ISS daily summary report– 3/03/2022. 2022 Mar 3 [cited May 12 2024]. In: NASA ISS On-Orbit Status Report blog [Internet]. Houston. Available from: https://blogs.nasa.gov/stationreport/2022/03/page/21/
- 41. Keeter B. ISS daily summary report– 2/08/2022. 2022 Feb 8 [cited May 12 2024]. NASA ISS On-Orbit Status Report blog [Internet]. Houston. Available from: https://blogs.nasa.gov/stationreport/2022/02/page/15/
- 42. Aristotle of Stageira. Metaphysics, Volume I: Books 1–9, Tredennick H, translator. Loeb Classical Library 271. Cambridge, MA, Harvard University Press; 1933. pp. 429–473.
- 44. Hodder I. Entangled: An archaeology of the relationships between humans and things. Hoboken. NJ, Wiley-Blackwell; 2012.
- 45. Malafouris L., How Things Shape the Mind: A Theory of Material Engagement (MIT Press, 2016).
- 46. Keeter B. ISS daily summary report– 3/11/2022. 2022 Mar 11 [cited May 12 2024]. NASA ISS On-Orbit Status Report blog [Internet]. Houston. Available from: https://blogs.nasa.gov/stationreport/2022/03/page/15/

Title Page Setup
A title page is required for all APA Style papers. There are both student and professional versions of the title page. Students should use the student version of the title page unless their instructor or institution has requested they use the professional version. APA provides a student title page guide (PDF, 199KB) to assist students in creating their title pages.
Student title page
The student title page includes the paper title, author names (the byline), author affiliation, course number and name for which the paper is being submitted, instructor name, assignment due date, and page number, as shown in this example.
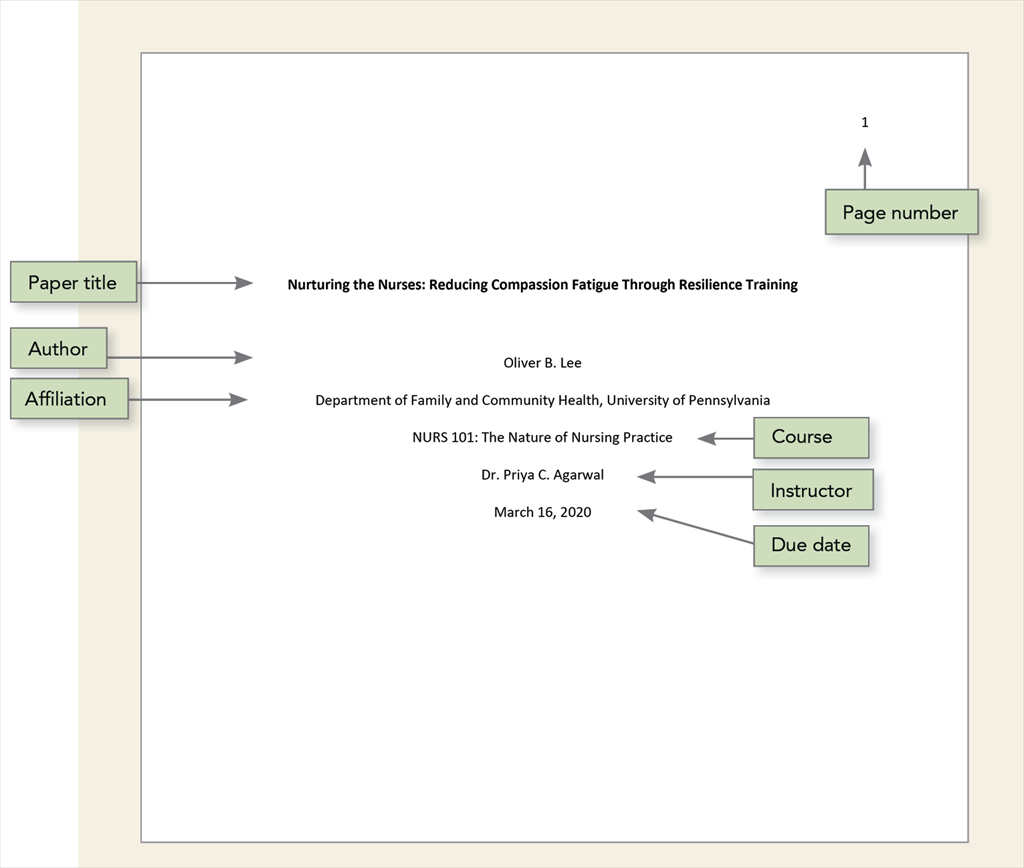
Title page setup is covered in the seventh edition APA Style manuals in the Publication Manual Section 2.3 and the Concise Guide Section 1.6

Related handouts
- Student Title Page Guide (PDF, 263KB)
- Student Paper Setup Guide (PDF, 3MB)
Student papers do not include a running head unless requested by the instructor or institution.
Follow the guidelines described next to format each element of the student title page.
|
|
|
---|---|---|
Paper title | Place the title three to four lines down from the top of the title page. Center it and type it in bold font. Capitalize of the title. Place the main title and any subtitle on separate double-spaced lines if desired. There is no maximum length for titles; however, keep titles focused and include key terms. |
|
Author names | Place one double-spaced blank line between the paper title and the author names. Center author names on their own line. If there are two authors, use the word “and” between authors; if there are three or more authors, place a comma between author names and use the word “and” before the final author name. | Cecily J. Sinclair and Adam Gonzaga |
Author affiliation | For a student paper, the affiliation is the institution where the student attends school. Include both the name of any department and the name of the college, university, or other institution, separated by a comma. Center the affiliation on the next double-spaced line after the author name(s). | Department of Psychology, University of Georgia |
Course number and name | Provide the course number as shown on instructional materials, followed by a colon and the course name. Center the course number and name on the next double-spaced line after the author affiliation. | PSY 201: Introduction to Psychology |
Instructor name | Provide the name of the instructor for the course using the format shown on instructional materials. Center the instructor name on the next double-spaced line after the course number and name. | Dr. Rowan J. Estes |
Assignment due date | Provide the due date for the assignment. Center the due date on the next double-spaced line after the instructor name. Use the date format commonly used in your country. | October 18, 2020 |
| Use the page number 1 on the title page. Use the automatic page-numbering function of your word processing program to insert page numbers in the top right corner of the page header. | 1 |
Professional title page
The professional title page includes the paper title, author names (the byline), author affiliation(s), author note, running head, and page number, as shown in the following example.
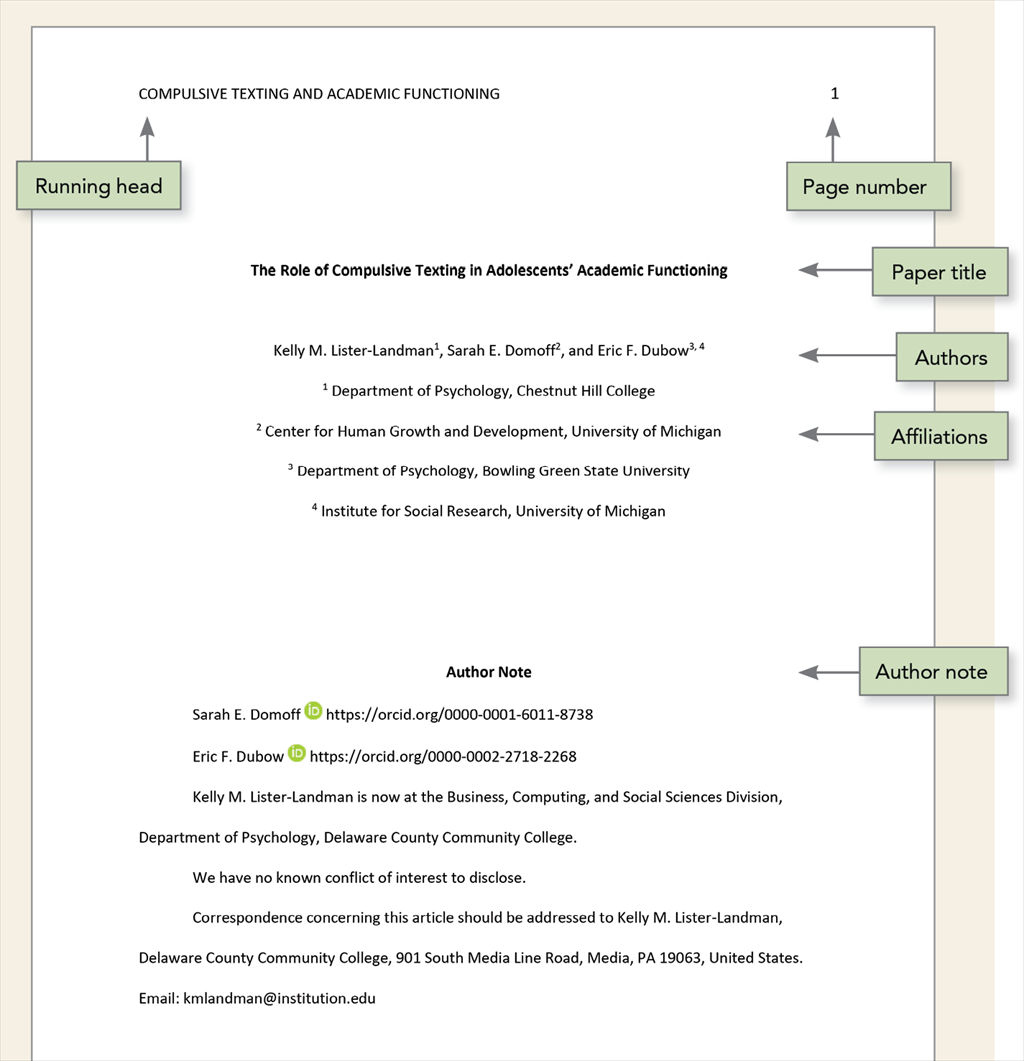
Follow the guidelines described next to format each element of the professional title page.
|
|
|
---|---|---|
Paper title | Place the title three to four lines down from the top of the title page. Center it and type it in bold font. Capitalize of the title. Place the main title and any subtitle on separate double-spaced lines if desired. There is no maximum length for titles; however, keep titles focused and include key terms. |
|
Author names
| Place one double-spaced blank line between the paper title and the author names. Center author names on their own line. If there are two authors, use the word “and” between authors; if there are three or more authors, place a comma between author names and use the word “and” before the final author name. | Francesca Humboldt |
When different authors have different affiliations, use superscript numerals after author names to connect the names to the appropriate affiliation(s). If all authors have the same affiliation, superscript numerals are not used (see Section 2.3 of the for more on how to set up bylines and affiliations). | Tracy Reuter , Arielle Borovsky , and Casey Lew-Williams | |
Author affiliation
| For a professional paper, the affiliation is the institution at which the research was conducted. Include both the name of any department and the name of the college, university, or other institution, separated by a comma. Center the affiliation on the next double-spaced line after the author names; when there are multiple affiliations, center each affiliation on its own line.
| Department of Nursing, Morrigan University |
When different authors have different affiliations, use superscript numerals before affiliations to connect the affiliations to the appropriate author(s). Do not use superscript numerals if all authors share the same affiliations (see Section 2.3 of the for more). | Department of Psychology, Princeton University | |
Author note | Place the author note in the bottom half of the title page. Center and bold the label “Author Note.” Align the paragraphs of the author note to the left. For further information on the contents of the author note, see Section 2.7 of the . | n/a |
| The running head appears in all-capital letters in the page header of all pages, including the title page. Align the running head to the left margin. Do not use the label “Running head:” before the running head. | Prediction errors support children’s word learning |
| Use the page number 1 on the title page. Use the automatic page-numbering function of your word processing program to insert page numbers in the top right corner of the page header. | 1 |
This paper is in the following e-collection/theme issue:
Published on 12.8.2024 in Vol 26 (2024)
Investigating Best Practices for Ecological Momentary Assessment: Nationwide Factorial Experiment
Authors of this article:


Results of the Protein Engineering Tournament: An Open Science Benchmark for Protein Modeling and Design
- Find this author on Google Scholar
- Find this author on PubMed
- Search for this author on this site
- ORCID record for Chase Armer
- ORCID record for Hassan Kane
- ORCID record for Dana L. Cortade
- ORCID record for Henning Redestig
- ORCID record for David A. Estell
- ORCID record for Adil Yusuf
- ORCID record for Nathan Rollins
- ORCID record for Hansen Spinner
- ORCID record for Debora Marks
- ORCID record for TJ Brunette
- ORCID record for Erika DeBenedictis
- For correspondence: [email protected]
- Info/History
- Supplementary material
- Preview PDF
The grand challenge of protein engineering is the development of computational models to characterize and generate protein sequences for arbitrary functions. Progress is limited by lack of 1) benchmarking opportunities, 2) large protein function datasets, and 3) access to experimental protein characterization. We introduce the Protein Engineering Tournament—a fully-remote competition designed to foster the development and evaluation of computational approaches in protein engineering. The tournament consists of an in silico round, predicting biophysical properties from protein sequences, followed by an in vitro round where novel protein sequences are designed, expressed and characterized using automated methods. Upon completion, all datasets, experimental protocols, and methods are made publicly available. We detail the structure and outcomes of a pilot Tournament involving seven protein design teams, powered by six multi-objective datasets, with experimental characterization by our partner, International Flavors and Fragrances. Forthcoming Protein Engineering Tournaments aim to mobilize the scientific community towards transparent evaluation of progress in the field.
- Download figure
- Open in new tab
Competing Interest Statement
The authors have declared no competing interest.
https://github.com/the-protein-engineering-tournament/pet-pilot-2023
View the discussion thread.
Supplementary Material
Thank you for your interest in spreading the word about bioRxiv.
NOTE: Your email address is requested solely to identify you as the sender of this article.

Citation Manager Formats
- EndNote (tagged)
- EndNote 8 (xml)
- RefWorks Tagged
- Ref Manager
- Tweet Widget
- Facebook Like
- Google Plus One
Subject Area
- Bioengineering
- Animal Behavior and Cognition (5518)
- Biochemistry (12552)
- Bioengineering (9417)
- Bioinformatics (30790)
- Biophysics (15833)
- Cancer Biology (12904)
- Cell Biology (18494)
- Clinical Trials (138)
- Developmental Biology (9992)
- Ecology (14947)
- Epidemiology (2067)
- Evolutionary Biology (19142)
- Genetics (12727)
- Genomics (17523)
- Immunology (12664)
- Microbiology (29683)
- Molecular Biology (12359)
- Neuroscience (64654)
- Paleontology (479)
- Pathology (2000)
- Pharmacology and Toxicology (3449)
- Physiology (5322)
- Plant Biology (11070)
- Scientific Communication and Education (1728)
- Synthetic Biology (3061)
- Systems Biology (7681)
- Zoology (1728)
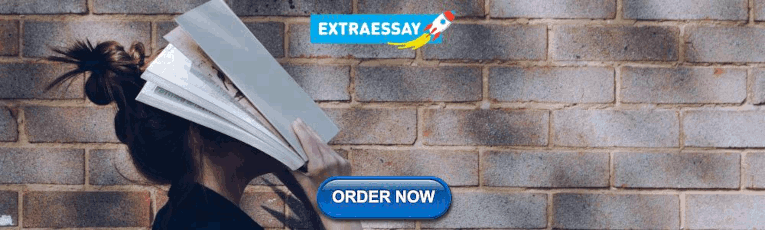
IMAGES
COMMENTS
Table of contents. Step 1: Define your variables. Step 2: Write your hypothesis. Step 3: Design your experimental treatments. Step 4: Assign your subjects to treatment groups. Step 5: Measure your dependent variable. Other interesting articles. Frequently asked questions about experiments.
2. Systematic sampling. Systematic sampling is similar to simple random sampling, but it is usually slightly easier to conduct. Every member of the population is listed with a number, but instead of randomly generating numbers, individuals are chosen at regular intervals. Example: Systematic sampling.
If you are interested in learning more about sampling techniques in experimental research, there are a variety of resources available to you. Some useful places to start include: Books "Experimental Design and Analysis: An Introduction" by David Blaxter "The Practice of Statistics in the Sciences" by Geoff Cumming and Chris Wallace
Chapter 1 - Sampling and Experimental Design Read sections 1.3 - 1.5 Sampling (1.3.3 and 1.4.2) Sampling Plans: methods of selecting individuals from a population. We are interested in sampling plans such that results from the sample can be used to make conclusions about the population.
Types of probability sampling. Various probability sampling methods exist, such as simple random sampling, systematic sampling, stratified sampling, and clustered sampling. Here, we provide detailed discussions and illustrative examples for each of these sampling methods: Simple random sampling: In simple random sampling, each individual has an ...
We offer a structured process for sample development and present eight key sampling considerations. The paper contributes to research method selection, development, and use, as well as extending discussions surrounding knowledge construction, standards of reporting, and design research impact. research methods. design science.
8 Five Sampling Methods • (Simple) Random Sampling • Stratified (Random) sampling • Systematic sampling • Cluster sampling • Convenience sampling Simple Random Sampling • Every element in the population is equally likely to be in sample. And •Every possible sample of size N has the same chance of being chosen. Stratified Sampling
Sampling Methods | Types, Techniques, & Examples. Published on 3 May 2022 by Shona McCombes. Revised on 10 October 2022. ... Experimental design is the process of planning an experiment to test a hypothesis. 33. Controlled Experiments | Methods & Examples of Control In a controlled experiment, all variables other than the independent variable ...
We could choose a sampling method based on whether we want to account for sampling bias; a random sampling method is often preferred over a non-random method for this reason. Random sampling examples include: simple, systematic, stratified, and cluster sampling. Non-random sampling methods are liable to bias, and common examples include ...
Abstract. Knowledge of sampling methods is essential to design quality research. Critical questions are provided to help researchers choose a sampling method. This article reviews probability and non-probability sampling methods, lists and defines specific sampling techniques, and provides pros and cons for consideration.
Three types of experimental designs are commonly used: 1. Independent Measures. Independent measures design, also known as between-groups, is an experimental design where different participants are used in each condition of the independent variable. This means that each condition of the experiment includes a different group of participants.
Cluster sampling- she puts 50 into random groups of 5 so we get 10 groups then randomly selects 5 of them and interviews everyone in those groups --> 25 people are asked. 2. Stratified sampling- she puts 50 into categories: high achieving smart kids, decently achieving kids, mediumly achieving kids, lower poorer achieving kids and clueless ...
Step 1: Consider your aims and approach. Step 2: Choose a type of research design. Step 3: Identify your population and sampling method. Step 4: Choose your data collection methods. Step 5: Plan your data collection procedures. Step 6: Decide on your data analysis strategies. Other interesting articles.
1.2 A Cautionary Tale. For illustrating some of the issues arising in the interplay of experimental design and analysis, we consider a simple example. We are interested in comparing the enzyme levels measured in processed blood samples from laboratory mice, when the sample processing is done either with a kit from a vendor A, or a kit from a competitor B.
sampling; and (2) design and analysis of experiments. More advanced topics will be covered in Stat-454: Sampling Theory and Practice and Stat-430: Experimental Design. 1.1 Population Statisticians are preoccupied with tasks of modeling random phenomena in the real world. The randomness as most of us understood, generally points to
Design of experiments (DOE) is defined as a branch of applied statistics that deals with planning, conducting, analyzing, and interpreting controlled tests to evaluate the factors that control the value of a parameter or group of parameters. DOE is a powerful data collection and analysis tool that can be used in a variety of experimental ...
The use of a sequence of experiments, where the design of each may depend on the results of previous experiments, including the possible decision to stop experimenting, is within the scope of sequential analysis, a field that was pioneered [12] by Abraham Wald in the context of sequential tests of statistical hypotheses. [13] Herman Chernoff wrote an overview of optimal sequential designs, [14 ...
Common sampling techniques include simple random sampling, stratified sampling, cluster sampling, and systematic sampling. Example: Understanding the different sampling techniques allows researchers to choose the most appropriate method for their specific research, ensuring representative and reliable results. 5. Implement the Sampling Strategy
Design of experiment means how to design an experiment in the sense that how the observations or measurements should be obtained to answer a query in a valid, efficient and economical way. The designing of the experiment and the analysis of obtained data are inseparable. If the experiment is designed properly keeping in mind the question, then ...
You use blocking to minimize the potential variables (also known as extraneous variables) from influencing your experimental result. Let's use the experiment example that Mr.Khan used in the video. To verify the effect of the pill, we need to make sure that the person's gender, health, or other personal traits don't affect the result.
Opportunity Sampling. Systematic Sampling. Sample size. Sampling methods in psychology refer to strategies used to select a subset of individuals (a sample) from a larger population, to study and draw inferences about the entire population. Common methods include random sampling, stratified sampling, cluster sampling, and convenience sampling.
This is often used to ensure that the sample is representative of the population as a whole. Cluster Sampling: In this method, the population is divided into clusters or groups, and then a random sample of clusters is selected. Then, all members of the selected clusters are included in the sample. Multi-Stage Sampling: This method combines two ...
Key Takeaways. Experimental designs are useful for establishing causality, but some types of experimental design do this better than others. Experiments help researchers isolate the effect of the independent variable on the dependent variable by controlling for the effect of extraneous variables.; Experiments use a control/comparison group and an experimental group to test the effects of ...
Between January and March 2022, crew aboard the International Space Station (ISS) performed the first archaeological fieldwork in space, the Sampling Quadrangle Assemblages Research Experiment (SQuARE). The experiment aimed to: (1) develop a new understanding of how humans adapt to life in an environmental context for which we are not evolutionarily adapted, using evidence from the observation ...
Machine learning methods, particularly neural networks trained on large datasets, are transforming how scientists approach scientific discovery and experimental design. However, current state-of-the-art neural networks are limited by their uninterpretability: Despite their excellent accuracy, they cannot describe how they arrived at their ...
While bioinformatics reveals patterns in protein sequences and structural biology methods elucidate atomic details of protein structures, it is difficult to attain equally high-resolution energetic information about protein conformational ensembles. We present PIGEON-FEATHER, a method for calculating free energies of opening (∆Gop) at single- or near-single-amino acid resolution for protein ...
The transport experiments were carried out at pH 7.4 in both the apical and basolateral chamber. The experiments were performed at 37°C and with a stirring rate of 500 rpm. The receiver compartment was sampled at 15, 30, and 60 min, and, at 60 min, also a final sample from the donor chamber was taken to calculate the mass balance of the compound.
The student title page includes the paper title, author names (the byline), author affiliation, course number and name for which the paper is being submitted, instructor name, assignment due date, and page number, as shown in this example.
Background: Ecological momentary assessment (EMA) is a measurement methodology that involves the repeated collection of real-time data on participants' behavior and experience in their natural environment. While EMA allows researchers to gain valuable insights into dynamic behavioral processes, the need for frequent self-reporting can be burdensome and disruptive.
The grand challenge of protein engineering is the development of computational models to characterize and generate protein sequences for arbitrary functions. Progress is limited by lack of 1) benchmarking opportunities, 2) large protein function datasets, and 3) access to experimental protein characterization. We introduce the Protein Engineering Tournament, a fully-remote competition designed ...