Thank you for visiting nature.com. You are using a browser version with limited support for CSS. To obtain the best experience, we recommend you use a more up to date browser (or turn off compatibility mode in Internet Explorer). In the meantime, to ensure continued support, we are displaying the site without styles and JavaScript.
- View all journals
- Explore content
- About the journal
- Sign up for alerts
- ADVERTISEMENT FEATURE Advertiser retains sole responsibility for the content of this article
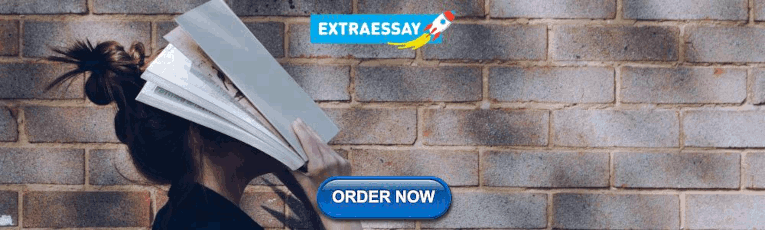
IBM Research uses advanced computing to accelerate therapeutic and biomarker discovery
- IBM Research
Produced by
Over the past decade, artificial intelligence (AI) has emerged as an engine of discovery by helping to unlock information from large repositories of previously inaccessible data. The cloud has expanded computer capacity exponentially by creating a global network of remote and distributed computing resources. And quantum computing has arrived on the scene as a game changer in processing power by harnessing quantum simulation to overcome the scaling and complexity limits of classical computing.
In parallel to these advances in computing, in which IBM is a world leader, the healthcare and life sciences have undergone their own information revolution. There has been an explosion in genomic, proteomic, metabolomic and a plethora of other foundational scientific data, as well as in diagnostic, treatment, outcome and other related clinical data. Paradoxically, however, this unprecedented increase in information volume has resulted in reduced accessibility and a diminished ability to use the knowledge embedded in that information. This reduction is caused by siloing of the data, limitations in existing computing capacity, and processing challenges associated with trying to model the inherent complexity of living systems.
IBM Research is now working on designing and implementing computational architectures that can convert the ever-increasing volume of healthcare and life-sciences data into information that can be used by scientists and industry experts the world over. Through an AI approach powered by high-performance computing (HPC)—a synergy of quantum and classical computing—and implemented in a hybrid cloud that takes advantage of both private and public environments, IBM is poised to lead the way in knowledge integration, AI-enriched simulation, and generative modeling in the healthcare and life sciences. Quantum computing, a rapidly developing technology, offers opportunities to explore and potentially address life-science challenges in entirely new ways.
“The convergence of advances in computation taking place to meet the growing challenges of an ever-shifting world can also be harnessed to help accelerate the rate of discovery in the healthcare and life sciences in unprecedented ways,” said Ajay Royyuru, IBM fellow and CSO for healthcare and life sciences at IBM Research. “At IBM, we are at the forefront of applying these new capabilities for advancing knowledge and solving complex problems to address the most pressing global health challenges.”
Improving the drug discovery value chain
Innovation in the healthcare and life sciences, while overall a linear process leading from identifying drug targets to therapies and outcomes, relies on a complex network of parallel layers of information and feedback loops, each bringing its own challenges (Fig. 1). Success with target identification and validation is highly dependent on factors such as optimized genotype–phenotype linking to enhance target identification, improved predictions of protein structure and function to sharpen target characterization, and refined drug design algorithms for identifying new molecular entities (NMEs). New insights into the nature of disease are further recalibrating the notions of disease staging and of therapeutic endpoints, and this creates new opportunities for improved clinical-trial design, patient selection and monitoring of disease progress that will result in more targeted and effective therapies.
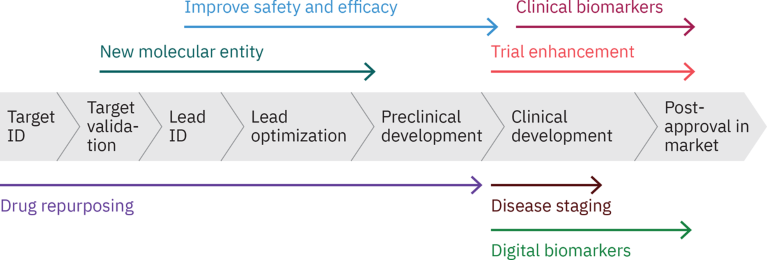
Fig. 1 | Accelerated discovery at a glance. IBM is developing a computing environment for the healthcare and life sciences that integrates the possibilities of next-generation technologies—artificial intelligence, the hybrid cloud, and quantum computing—to accelerate the rate of discovery along the drug discovery and development pipeline.
Powering these advances are several core computing technologies that include AI, quantum computing, classical computing, HPC, and the hybrid cloud. Different combinations of these core technologies provide the foundation for deep knowledge integration, multimodal data fusion, AI-enriched simulations and generative modeling. These efforts are already resulting in rapid advances in the understanding of disease that are beginning to translate into the development of better biomarkers and new therapeutics (Fig. 2).
“Our goal is to maximize what can be achieved with advanced AI, simulation and modeling, powered by a combination of classical and quantum computing on the hybrid cloud,” said Royyuru. “We anticipate that by combining these technologies we will be able to accelerate the pace of discovery in the healthcare and life sciences by up to ten times and yield more successful therapeutics and biomarkers.”
Optimized modeling of NMEs
Developing new drugs hinges on both the identification of new disease targets and the development of NMEs to modulate those targets. Developing NMEs has typically been a one-sided process in which the in silico or in vitro activities of large arrays of ligands would be tested against one target at a time, limiting the number of novel targets explored and resulting in ‘crowding’ of clinical programs around a fraction of validated targets. Recent developments in proteochemometric modeling—machine learning-driven methods to evaluate de novo protein interactions in silico—promise to turn the tide by enabling the simultaneous evaluation of arrays of both ligands and targets, and exponentially reducing the time required to identify potential NMEs.
Proteochemometric modeling relies on the application of deep machine learning tools to determine the combined effect of target and ligand parameter changes on the target–ligand interaction. This bimodal approach is especially powerful for large classes of targets in which active-site similarities and lack of activity data for some of the proteins make the conventional discovery process extremely challenging.
Protein kinases are ubiquitous components of many cellular processes, and their modulation using inhibitors has greatly expanded the toolbox of treatment options for cancer, as well as neurodegenerative and viral diseases. Historically, however, only a small fraction of the kinome has been investigated for its therapeutic potential owing to biological and structural challenges.
Using deep machine learning algorithms, IBM researchers have developed a generative modeling approach to access large target–ligand interaction datasets and leverage the information to simultaneously predict activities for novel kinase–ligand combinations 1 . Importantly, their approach allowed the researchers to determine that reducing the kinase representation from the full protein sequence to just the active-site residues was sufficient to reliably drive their algorithm, introducing an additional time-saving, data-use optimization step.
Machine learning methods capable of handling multimodal datasets and of optimizing information use provide the tools for substantially accelerating NME discovery and harnessing the therapeutic potential of large and sometimes only minimally explored molecular target spaces.
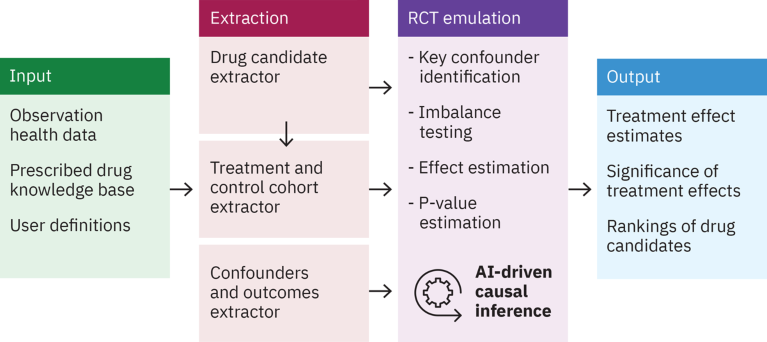
Fig. 2 | Focusing on therapeutics and biomarkers. The identification of new molecular entities or the repurposing potential of existing drugs 2 , together with improved clinical and digital biomarker discovery, as well as disease staging approaches 3 , will substantially accelerate the pace of drug discovery over the next decade. AI, artificial intelligence.
Drug repurposing from real-world data
Electronic health records (EHRs) and insurance claims contain a treasure trove of real-world data about the healthcare history, including medications, of millions of individuals. Such longitudinal datasets hold potential for identifying drugs that could be safely repurposed to treat certain progressive diseases not easily explored with conventional clinical-trial designs because of their long time horizons.
Turning observational medical databases into drug-repurposing engines requires the use of several enabling technologies, including machine learning-driven data extraction from unstructured sources and sophisticated causal inference modeling frameworks.
Parkinson’s disease (PD) is one of the most common neurodegenerative disorders in the world, affecting 1% of the population above 60 years of age. Within ten years of disease onset, an estimated 30–80% of PD patients develop dementia, a debilitating comorbidity that has made developing disease-modifying treatments to slow or stop its progression a high priority.
IBM researchers have now developed an AI-driven, causal inference framework designed to emulate phase 2 clinical trials to identify candidate drugs for repurposing, using real-world data from two PD patient cohorts totaling more than 195,000 individuals 2 . Extracting relevant data from EHRs and claims data, and using dementia onset as a proxy for evaluating PD progression, the team identified two drugs that significantly delayed progression: rasagiline, a drug already in use to treat motor symptoms in PD, and zolpidem, a known psycholeptic used to treat insomnia. Applying advanced causal inference algorithms, the IBM team was able to show that the drugs exert their effects through distinct mechanisms.
Using observational healthcare data to emulate otherwise costly, large and lengthy clinical trials to identify repurposing candidates highlights the potential for applying AI-based approaches to accelerate potential drug leads into prospective registration trials, especially in the context of late-onset progressive diseases for which disease-modifying therapeutic solutions are scarce.
Enhanced clinical-trial design
One of the main bottlenecks in drug discovery is the high failure rate of clinical trials. Among the leading causes for this are shortcomings in identifying relevant patient populations and therapeutic endpoints owing to a fragmented understanding of disease progression.
Using unbiased machine-learning approaches to model large clinical datasets can advance the understanding of disease onset and progression, and help identify biomarkers for enhanced disease monitoring, prognosis, and trial enrichment that could lead to higher rates of trial success.
Huntington’s disease (HD) is an inherited neurodegenerative disease that results in severe motor, cognitive and psychiatric disorders and occurs in about 3 per 100,000 inhabitants worldwide. HD is a fatal condition, and no disease-modifying treatments have been developed to date.
An IBM team has now used a machine-learning approach to build a continuous dynamic probabilistic disease-progression model of HD from data aggregated from multiple disease registries 3 . Based on longitudinal motor, cognitive and functional measures, the researchers were able to identify nine disease states of clinical relevance, including some in the early stages of HD. Retrospective validation of the results with data from past and ongoing clinical studies showed the ability of the new disease-progression model of HD to provide clinically meaningful insights that are likely to markedly improve patient stratification and endpoint definition.
Model-based determination of disease stages and relevant clinical and digital biomarkers that lead to better monitoring of disease progression in individual participants is key to optimizing trial design and boosting trial efficiency and success rates.
A collaborative effort
IBM has established its mission to advance the pace of discovery in healthcare and life sciences through the application of a versatile and configurable collection of accelerator and foundation technologies supported by a backbone of core technologies (Fig. 1). It recognizes that a successful campaign to accelerate discovery for therapeutics and biomarkers to address well-known pain points in the development pipeline requires external, domain-specific partners to co-develop, practice, and scale the concept of technology-based acceleration. The company has already established long-term commitments with strategic collaborators worldwide, including the recently launched joint Cleveland Clinic–IBM Discovery Accelerator, which will house the first private-sector, on-premises IBM Quantum System One in the United States. The program is designed to actively engage with universities, government, industry, startups and other relevant organizations, cultivating, supporting and empowering this community with open-source tools, datasets, technologies and educational resources to help break through long-standing bottlenecks in scientific discovery. IBM is engaging with biopharmaceutical enterprises that share this vision of accelerated discovery.
“Through partnerships with leaders in healthcare and life sciences worldwide, IBM intends to boost the potential of its next-generation technologies to make scientific discovery faster, and the scope of the discoveries larger than ever,” said Royyuru. “We ultimately see accelerated discovery as the core of our contribution to supercharging the scientific method.”
Born, J. et al. J. Chem. Inf. Model. 62 , 240–257 (2022).
Article PubMed Google Scholar
Laifenfeld, D. et al. Front. Pharmacol. 12 , 631584 (2021).
Mohan, A. et al. Mov. Disord. 37 , 553–562 (2022).
Harrer, S. et al. Trends Pharmacol Sci. 40 , 577–591 (2019).
Parikh, J. et al. J. Pharmacokinet. Pharmacodyn. 49 , 51–64 (2022).
Kashyap, A. et al. Trends Biotechnol. 40 , 647–676 (2021).
Norel, R. et al. npj Parkinson’s Dis. 6 , 12 (2020).
Article Google Scholar
Download references
Quick links
- Explore articles by subject
- Guide to authors
- Editorial policies
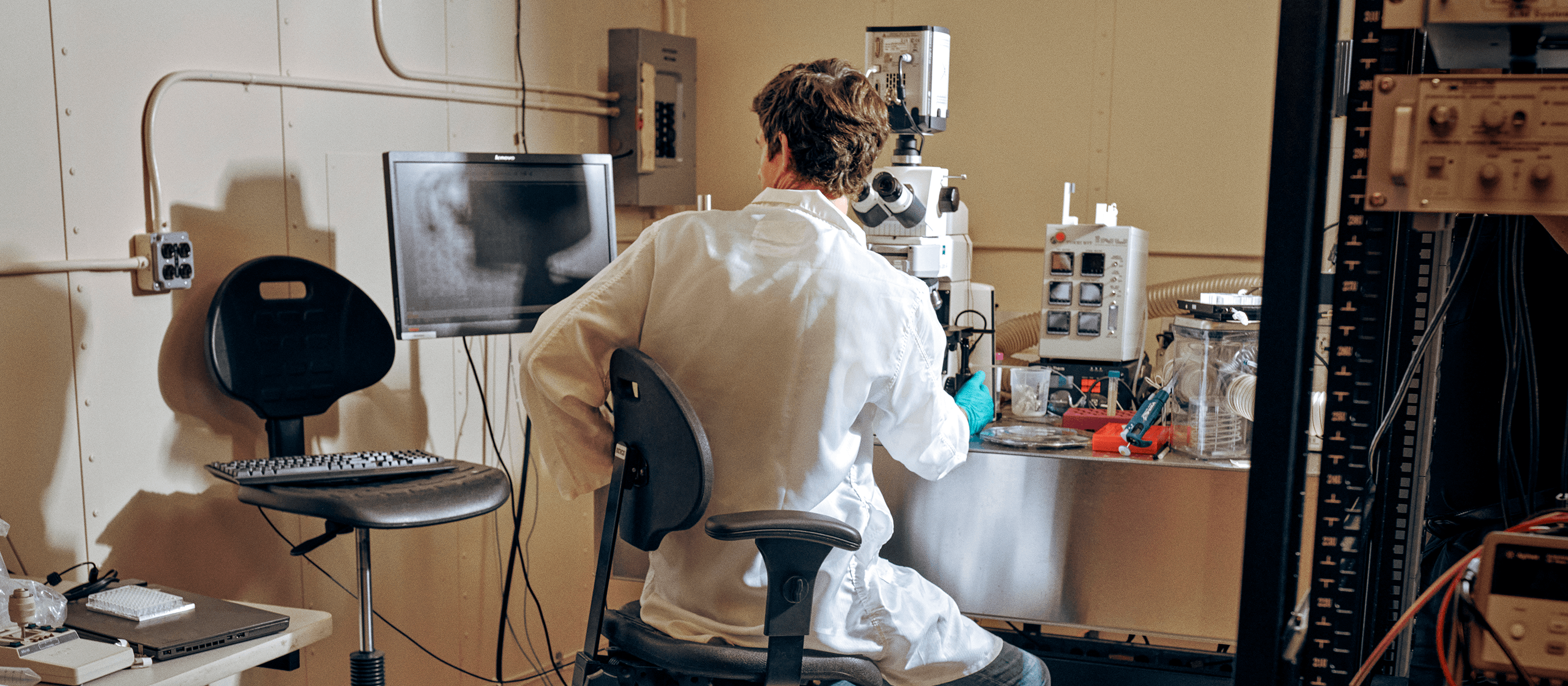
IBM Research Europe – United Kingdom
Working across two locations (Daresbury and Hursley), our teams have been constantly contributing to creating what’s next in computing. Our research is motivated by today’s most pressing challenges both in the global context and specific to UK industries and institutions. We bring cutting-edge computational science and engineering to define the quantum computing of the future, the next generation of artificial intelligence, and the technologies that will help accelerate scientific discovery, for UK and beyond.
Meet the team
How IBM and STFC Hartree Centre are accelerating innovation in the UK
Ibm and nasa team up to spur new discoveries about our planet, ibm’s new open-source toolkit for simulation, new ai research could help find the best treatment for bowel disease, new algorithm accelerates materials screening by working smart, not hard, tapping into the inner rhythm of living organisms with ai and ml, simulation for accelerated discovery.
- Accelerated Discovery
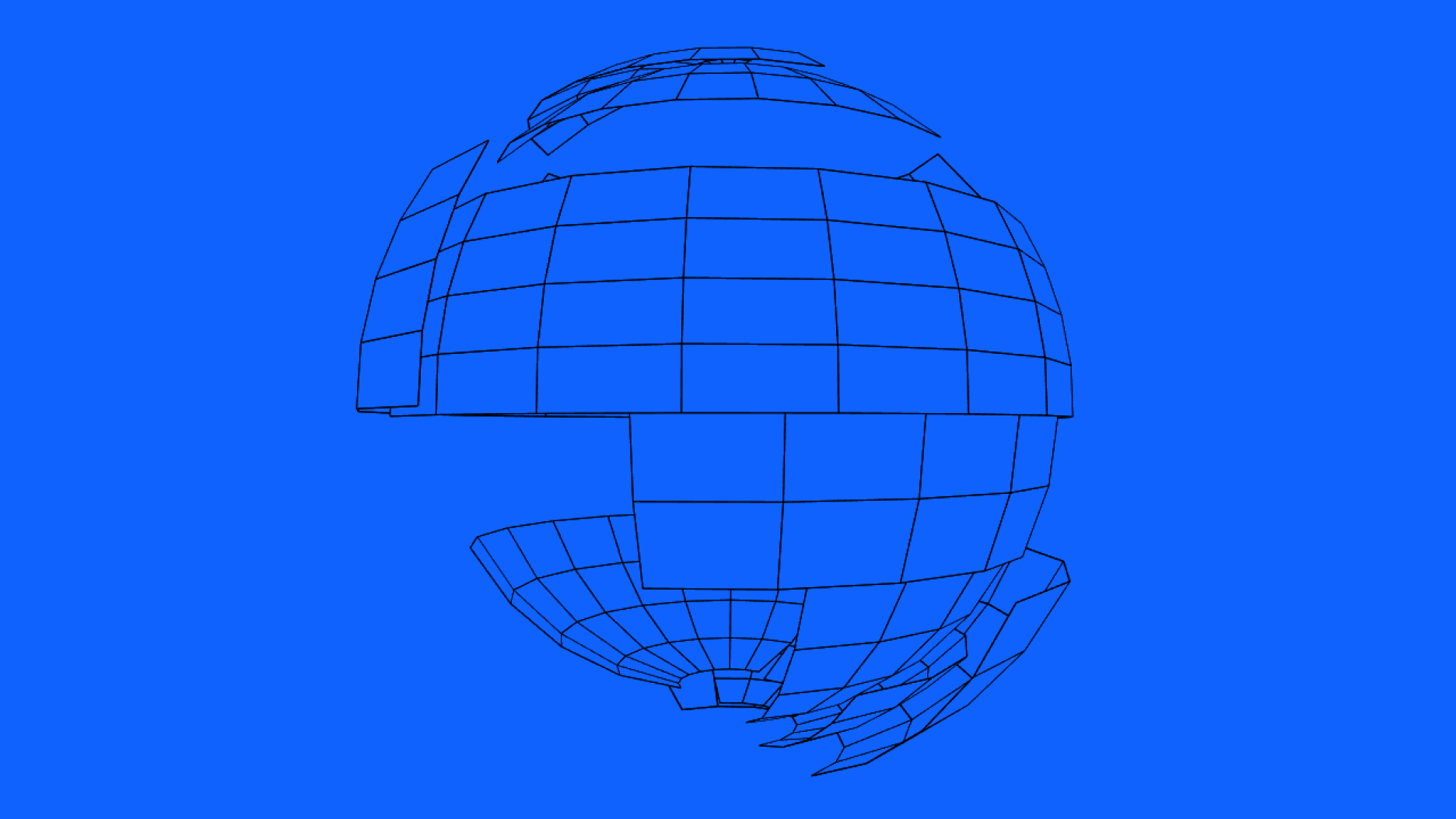
Auto-omics for climate and sustainability
- Explainable AI
- Machine Learning
- Hybrid Cloud HPC
- Life Sciences
- Climate and Sustainability
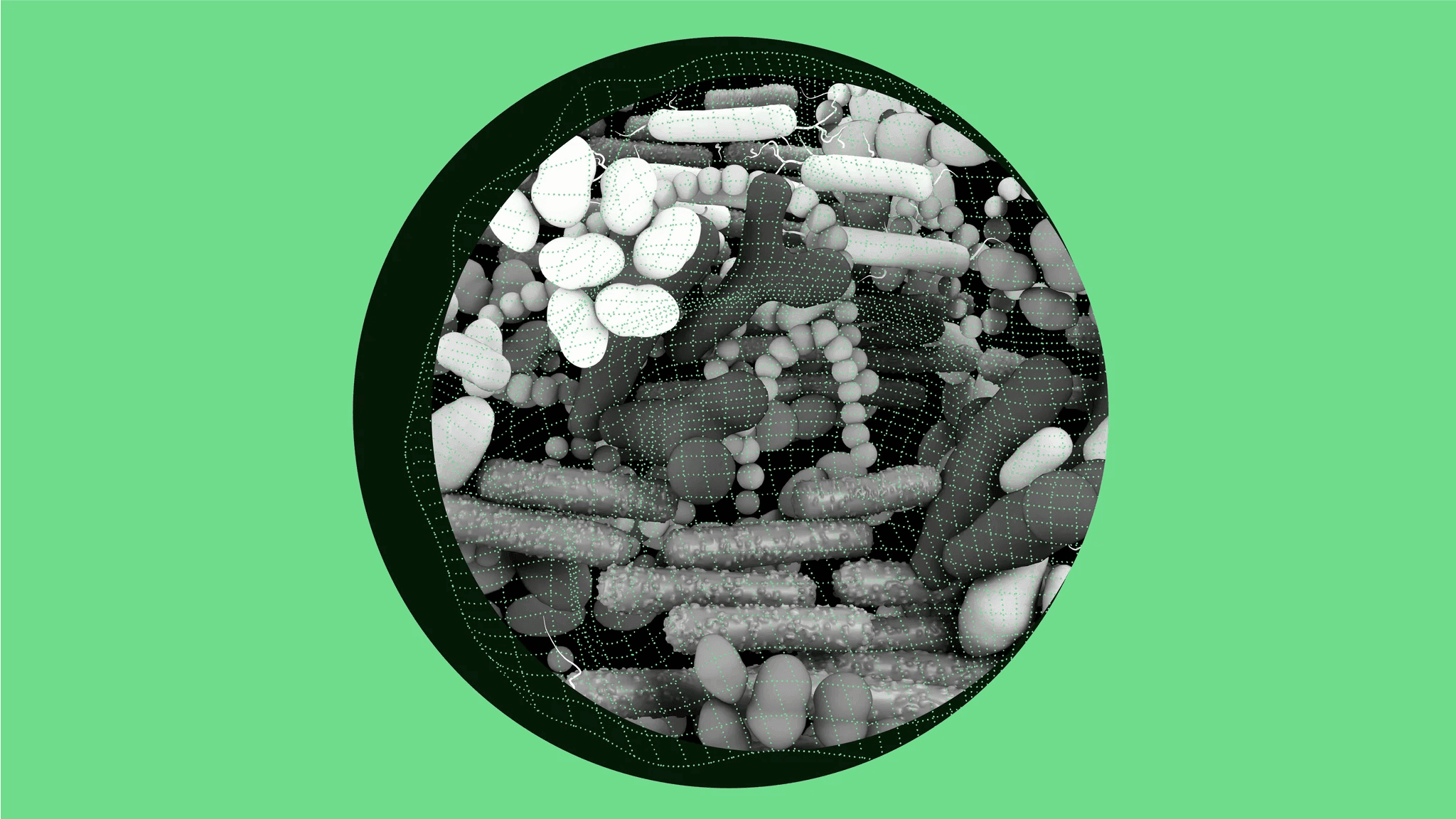
Auto-omics for healthcare and drug discovery
- Automated AI
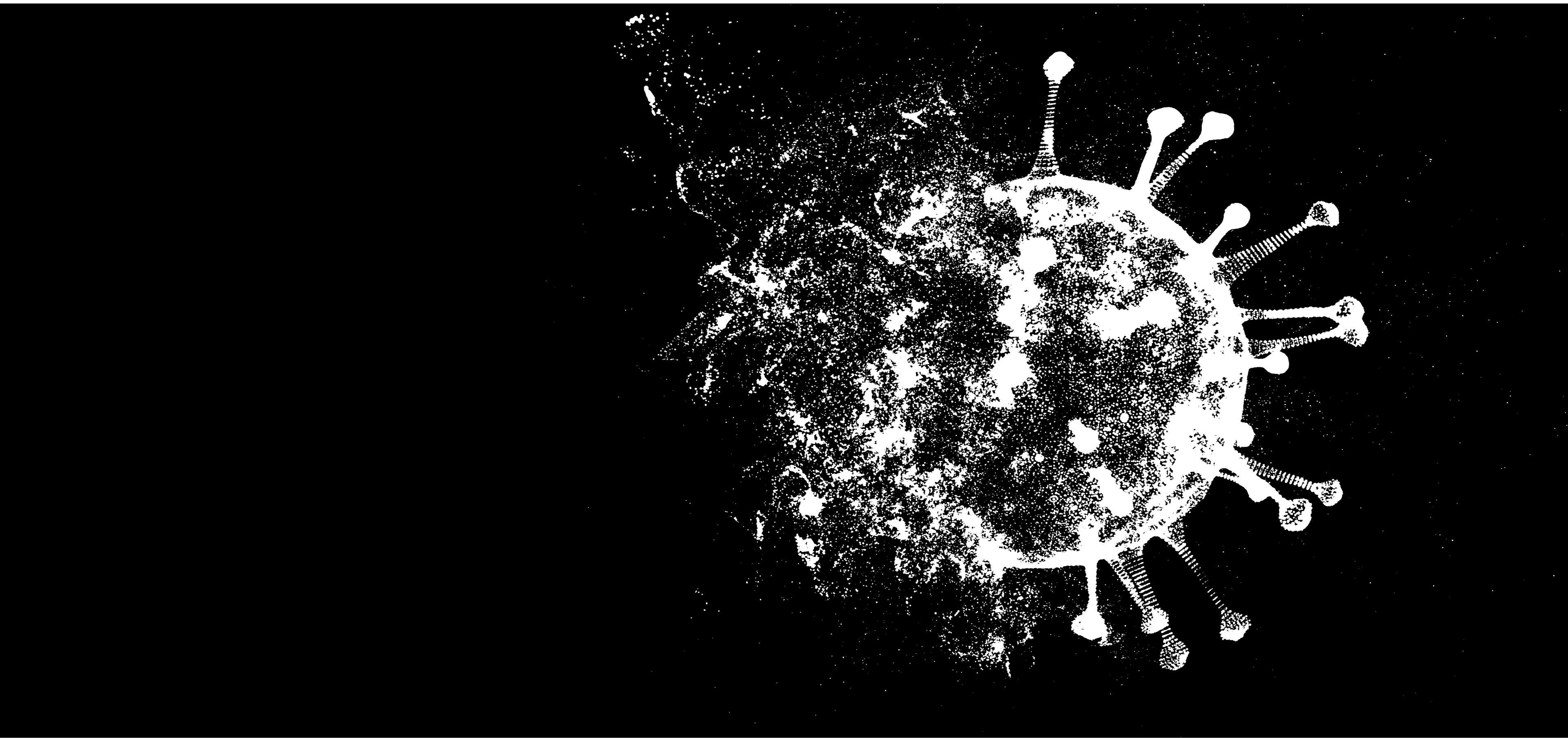
Host-pathogen interactions for healthcare and drug discovery
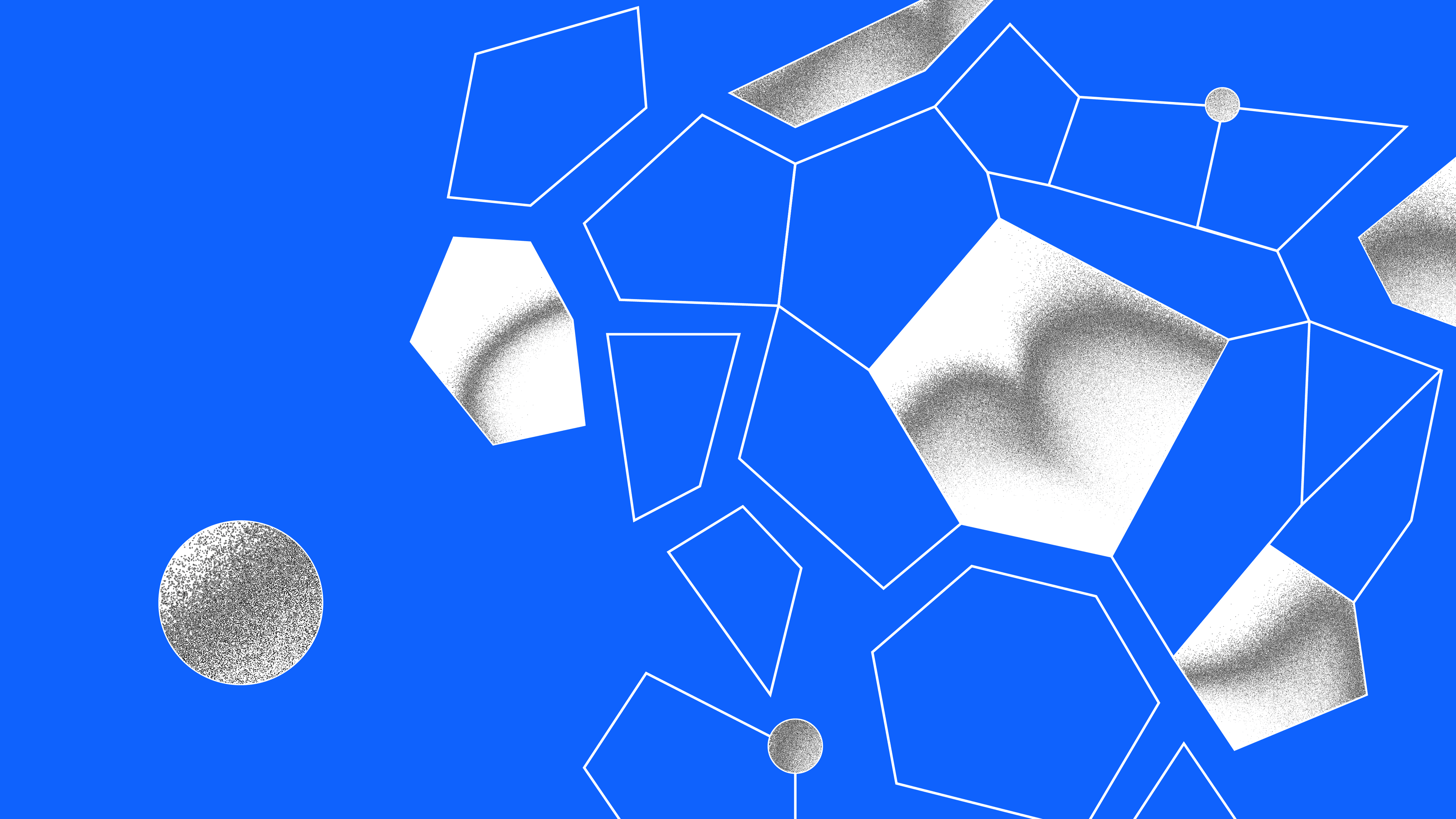
Discovering physics extremes with computing
- Physical Sciences
Location details
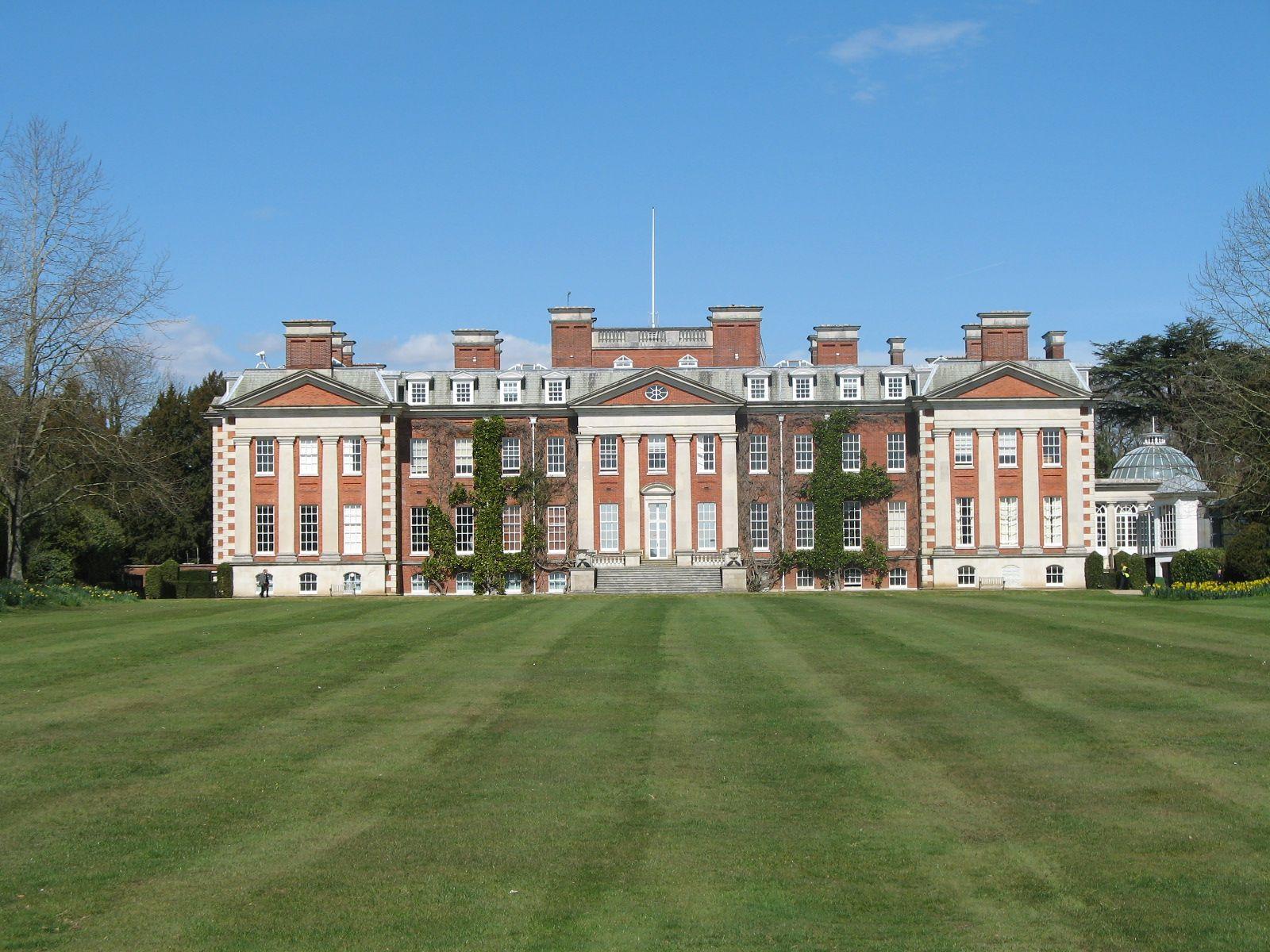
IBM United Kingdom Limited Hursley Park WinchesterHants SO21 2JN UK
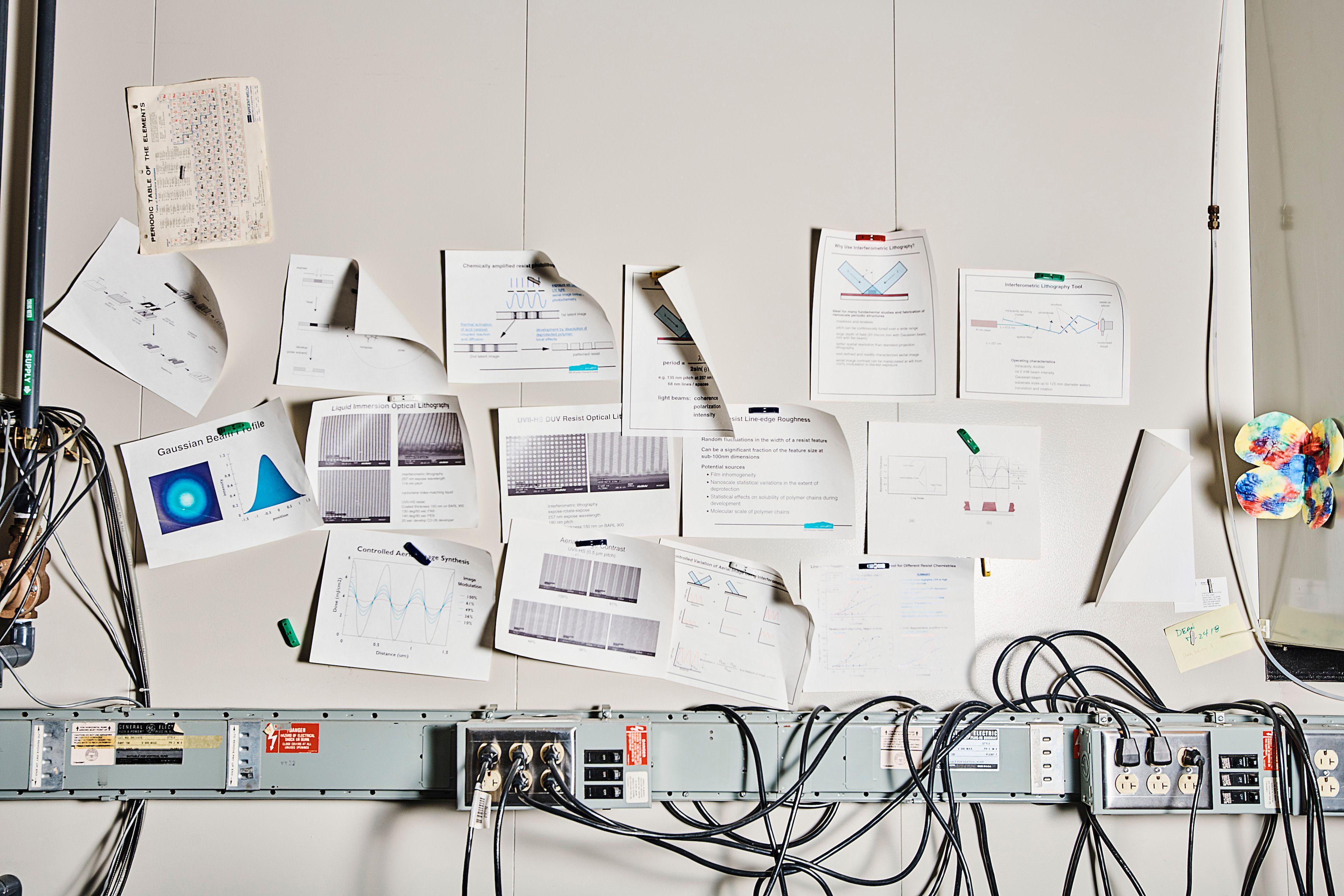
Join our team
We’re always looking for people excited to make a difference. See our open positions and help us invent what’s next.
- See open positions in the UK
All press releases
IBM Study: Security Response Planning on the Rise, But Containing Attacks Remains an Issue
CAMBRIDGE, Mass. , June 30, 2020 / PRNewswire / -- IBM (NYSE: IBM ) Security today announced the results of a global report examining businesses' effectiveness in preparing for and responding to cyberattacks. While organizations surveyed have slowly improved in their ability to plan for, detect and respond to cyberattacks over the past five years, their ability to contain an attack has declined by 13% during this same period. The global survey conducted by Ponemon Institute and sponsored by IBM Security found that respondents' security response efforts were hindered by the use of too many security tools, as well as a lack of specific playbooks for common attack types.
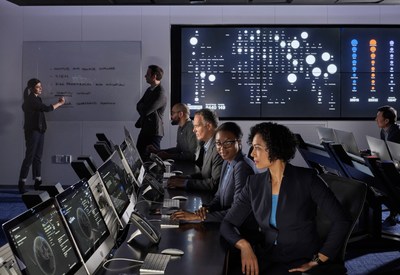
While security response planning is slowly improving, the vast majority of organizations surveyed (74%) are still reporting that their plans are either ad-hoc, applied inconsistently, or that they have no plans at all. This lack of planning can impact the cost of security incidents, as companies that have incident response teams and extensively test their incident response plans spend an average of $1.2 million less on data breaches than those who have both of these cost-saving factors in place. 1
The key findings of those surveyed from the fifth annual Cyber Resilient Organization Report include:
- Slowly Improving: More surveyed organizations have adopted formal, enterprise-wide security response plans over the past 5 years of the study; growing from 18% of respondents in 2015, to 26% in this year's report (a 44% improvement).
- Playbooks Needed: Even amongst those with a formal security response plan, only one third (representing 17% of total respondents) had also developed specific playbooks for common attack types — and plans for emerging attack methods like ransomware lagged even further behind.
- Complexity Hinders Response: The amount of security tools that an organization was using had a negative impact across multiple categories of the threat lifecycle amongst those surveyed. Organizations using 50+ security tools ranked themselves 8% lower in their ability to detect, and 7% lower in their ability to respond to an attack, than those respondents with less tools.
- Better Planning, Less Disruption: Companies with formal security response plans applied across the business were less likely to experience significant disruption as the result of a cyberattack. Over the past two years, only 39% of these companies experienced a disruptive security incident, compared to 62% of those with less formal or consistent plans.
"While more organizations are taking incident response planning seriously, preparing for cyberattacks isn't a one and done activity," said Wendi Whitmore, Vice President of IBM X-Force Threat Intelligence. "Organizations must also focus on testing, practicing and reassessing their response plans regularly. Leveraging interoperable technologies and automation can also help overcome complexity challenges and speed the time it takes to contain an incident."
Updating Playbooks for Emerging Threats The survey found that even amongst organizations with a formal cybersecurity incident response plan (CSIRP), only 33% had playbooks in place for specific types of attacks. Since different breeds of attack require unique response techniques, having pre-defined playbooks provides organizations with consistent and repeatable action plans for the most common attacks they are likely to face.
Amongst the minority of responding organizations who do have attack-specific playbooks, the most common playbooks are for DDoS attacks (64%) and malware (57%). While these methods have historically been top issues for the enterprise, additional attack methods such as ransomware are on the rise. While ransomware attacks have spiked nearly 70% in recent years, 2 only 45% of those in the survey using playbooks had designated plans for ransomware attacks.
Additionally, more than half (52%) of those with security response plans said they have never reviewed or have no set time period for reviewing or testing those plans. With business operations changing rapidly due to an increasingly remote workforce, and new attack techniques constantly being introduced, this data suggests that surveyed businesses may be relying on outdated response plans which don't reflect the current threat and business landscape.
More Tools Led to Worse Response Capabilities The report also found that complexity is negatively impacting incident response capabilities. Those surveyed estimated their organization was using more than 45 different security tools on average, and that each incident they responded to required coordination across around 19 tools on average. However, the study also found that an over-abundance of tools may actually hinder organizations ability to handle attacks. In the survey, those using more than 50 tools ranked themselves 8% lower in their ability to detect an attack (5.83/10 vs. 6.66/10), and around 7% lower when it comes to responding to an attack (5.95/10 vs. 6.72/10).
These findings suggest that adopting more tools didn't necessarily improve security response efforts — in fact, it may have done the opposite. The use of open, interoperable platforms as well as automation technologies can help reduce the complexity of responding across disconnected tools. Amongst high-performing organizations in the report, 63% said the use of interoperable tools helped them improve their response to cyberattacks.
Better Planning Pays Off This year's report suggests that surveyed organizations who invested in formal planning were more successful in responding to incidents. Amongst respondents with a CSIRP applied consistently across the business, only 39% experienced an incident that resulted in a significant disruption to the organization within the past two years compared to 62% of those who didn't have a formal plan in place.
Looking at specific reasons that these organizations cited for their ability to respond to attacks, security workforce skills were found to be a top factor. 61% of those surveyed attributed hiring skilled employees as a top reason for becoming more resilient; amongst those who said their resiliency did not improve, 41% cited the lack of skilled employees as the top reason.
Technology was another differentiator that helped organizations in the report become more cyber resilient, especially when it comes to tools that helped them resolve complexity. Looking at organizations with higher levels of cyber resilience, the top two factors cited for improving their level of cyber resilience were visibility into applications and data (57% selecting) and automation tools (55% selecting). Overall, the data suggests that surveyed organizations that were more mature in their response preparedness relied more heavily on technology innovations to become more resilient.
About the Study Conducted by the Ponemon Institute and sponsored by IBM Security, the 2020 Cyber Resilient Organization Report is the fifth installment covering organizations' ability to properly prepare for and handle cyberattacks. The survey features insight from more than 3,400 security and IT professionals from around the world, including the United States , India , Germany , United Kingdom , Brazil , Japan , Australia , France , Canada , ASEAN, and the Middle East .
Review the full report here: https://www.ibm.com/account/reg/us-en/signup?formid=urx-45839
Sign up for our correlating webinar taking place July 23 at 11:00 AM ET here: https://event.on24.com/wcc/r/2448121/9297B87DE7A378D816846835989BD762
About IBM Security IBM Security offers one of the most advanced and integrated portfolios of enterprise security products and services. The portfolio, supported by world-renowned IBM X-Force® research, enables organizations to effectively manage risk and defend against emerging threats. IBM operates one of the world's broadest security research, development and delivery organizations, monitors 70 billion security events per day in more than 130 countries, and has been granted more than 10,000 security patents worldwide. For more information, please check www.ibm.com/security , follow @ IBMSecurity on Twitter or visit the IBM Security Intelligence blog .
Media Contact: Kim Samra IBM Security [email protected] 510-468-6406
1 IBM Security and Ponemon Institute: 2019 Cost of a Data Breach Report
2 IBM Security, 2020 X-Force Threat Intelligence Index , (2020), p. 15

- IBM Security Command Center
Release Categories
- Artificial intelligence
- Hybrid cloud
- Research and innovation
- Social impact
Additional Assets

Be better prepared for breaches by understanding their causes and the factors that increase or reduce costs. Explore the comprehensive findings from the Cost of a Data Breach Report 2023. Learn from the experiences of more than 550 organizations that were hit by a data breach.
This report provides valuable insights into the threats that you face, along with practical recommendations to upgrade your cybersecurity and minimize losses. Take a deep dive into the report and find out what your organization is up against and how to mitigate the risks.
The global average cost of a data breach in 2023 was USD 4.45 million, a 15% increase over 3 years.
51% of organizations are planning to increase security investments as a result of a breach, including incident response (IR) planning and testing, employee training, and threat detection and response tools.
The average savings for organizations that use security AI and automation extensively is USD 1.76 million compared to organizations that don’t.
Gain insights from IBM X-Force experts
Get the most up-to-date information on the financial implications of data breaches. Learn how to safeguard your organization’s reputation and bottom line.
Check out the recommendations based on the findings of the Cost of a Data Breach Report and learn how to better secure your organization.
Only 28% of organizations used security AI extensively, which reduces costs and speeds up containment.
Innovative technologies such as IBM Security® QRadar® SIEM use AI to rapidly investigate and prioritize high-fidelity alerts based on credibility, relevance and severity of the risk. IBM Security® Guardium® features built-in AI outlier detection that enables organizations to quickly identify abnormalities in data access.
If you need to strengthen your defenses, IBM Security® Managed Detection and Response (MDR) Services use automated and human-initiated actions to provide visibility and stop threats across networks and endpoints. With a unified, AI-powered approach, threat hunters can take decisive actions and respond to threats faster.
Explore QRadar SIEM
Explore Managed Detection and Response Services
82% of breaches involved data stored in the cloud. Organizations must look for solutions that provide visibility across hybrid environments and protect data as it moves across clouds, databases, apps and services. IBM Security Guardium helps you uncover, encrypt, monitor and protect sensitive data across more than 19 hybrid cloud environments to give you a better security posture. IBM data security services provide you with advisory, planning and execution capabilities to secure your data, whether you’re migrating to the cloud or need to secure data already in the cloud. Services include data discovery and classification, data loss prevention, data-centric threat monitoring, encryption services and more.
Explore the Guardium data security portfolio
Learn about data security services
Build security into every stage of software and hardware development. Employing a DevSecOps approach and conducting penetration and application testing are top cost-saving factors in the report. X-Force® Red is a global team of hackers hired to break into organizations and uncover risky vulnerabilities that attackers may use for personal gain. The team's offensive security services—including penetration testing, application testing, vulnerability management and adversary simulation—can help identify, prioritize and remediate security flaws covering your digital and physical ecosystem.
Discover X-Force Red offensive security services
Explore our mobile security solution
Knowing your attack surface isn’t enough. You also need an incident response (IR) plan to protect it. The IBM Security® Randori platform uses a continuous, accurate discovery process to uncover known and unknown IT assets, getting you on target quickly with correlated, factual findings based on adversarial temptation. With X-Force® IR emergency support and proactive services, teams can test your cyberattack readiness plan and minimize the impact of a breach by preparing your IR teams, processes and controls.
Get IBM Security Randori
Explore X-Force for incident response
IBM Security helps protect enterprises with an integrated portfolio of products and services, infused with security AI and automation capabilities. The portfolio enables organizations to predict threats, protect data as it moves, and respond with speed and precision while allowing for innovation.
Success Across Cultures
Understand and minimize cross-cultural issues

Hofstede and IBM: the Beginning of Significant Cross-Cultural Research
Posted on 10. May 2019 2 Comments
If you looked at Geert Hofstede ‘s life, there was nothing particularly remarkable that might make you imagine he’d one day be at the forefront of cross-cultural research.
The Dutch researcher called the Netherlands home. He lived and studied there, after which he entered the military.
He became a management trainer at IBM, as well as the manager of staff research. It was in the latter role that he became entrenched in systematic research which would later hone in on the field of cross-cultural studies.
International Employee Opinion Research Program
In his role as manager of staff research, IBM’s International Employee Opinion Research Program became Hofstede’s brainchild.
Hofstede and his colleagues gathered and analyzed over 116,000 survey questionnaires over six years. The questionnaires were collected from 72 countries and involved 183 questions about the work environment , completed by IBM employees.
Providing a number of options, questionnaires asked employees to choose which option was the most important to them.
An example:
Which is most important to you?
- A job that allows personal/family time
- Challenging work that provides a sense of accomplishment
- Freedom to adapt your approach to work
Employees could choose their preference and, although the word “culture” wasn’t used in any context by IBM staff, and they weren’t charged with researching cross-cultural differences, nevertheless, the data revealed various patterns of cultural opinion and behavior.
Still, no cultural opinions were drawn from the data at the time.
Hofstede’s Findings
Taking a sabbatical from IBM, Hofstede taught at the IMD in Switzerland. It was there that he was allowed the time and academic engagement to analyze the IBM research.
He found that nationality could account for the behavioral differences resultant in the survey.
In order to test his theory, he questioned folks from various countries who didn’t work for IBM.
It became clear that cultural differences were there.
The value of Hofstede’s research was lost on many for a while…it was lost even on him.
He had no idea what a significant gold mine he’d come across, from the standpoint of international business.
At the time, economic success was not dependent on cultural sensitivities . The United States was the number one unchallenged economic power.
As to the matter, Hofstede said:
“In the 1970s I was living in Brussels when I started developing my ideas of culture and I approached the European Commission about this, but found myself initially directed to an official who was responsible for museums! Such was their idea of culture!”
But all this changed in the ‘80s and beyond – a period which we’ll talk more about next week.
Teilen mit:
very interesting read
- Pingback: Wat is bedrijfscultuur? 20 definities met elkaar vergeleken
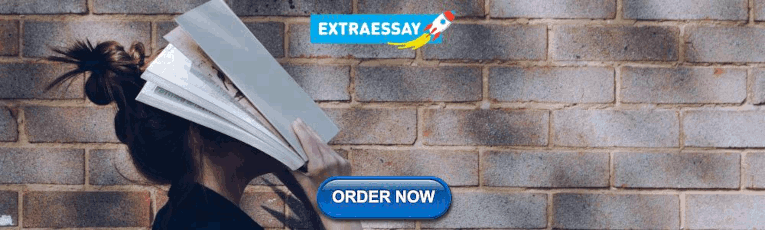
Leave a comment Cancel reply

- Already have a WordPress.com account? Log in now.
- Subscribe Subscribed
- Copy shortlink
- Report this content
- View post in Reader
- Manage subscriptions
- Collapse this bar

An official website of the United States government
The .gov means it’s official. Federal government websites often end in .gov or .mil. Before sharing sensitive information, make sure you’re on a federal government site.
The site is secure. The https:// ensures that you are connecting to the official website and that any information you provide is encrypted and transmitted securely.
- Publications
- Account settings
Preview improvements coming to the PMC website in October 2024. Learn More or Try it out now .
- Advanced Search
- Journal List
- v.13(1); 2021 Jan

Trends in the Usage of Statistical Software and Their Associated Study Designs in Health Sciences Research: A Bibliometric Analysis
Emad masuadi.
1 Research Unit/Biostatistics, King Saud bin Abdulaziz University for Health Sciences, College of Medicine/King Abdullah International Medical Research Centre, Riyadh, SAU
Mohamud Mohamud
2 Research Unit/Epidemiology, King Saud bin Abdulaziz University for Health Sciences, College of Medicine, Riyadh, SAU
Muhannad Almutairi
3 Medicine, King Saud bin Abdulaziz University for Health Sciences, College of Medicine, Riyadh, SAU
Abdulaziz Alsunaidi
Abdulmohsen k alswayed, omar f aldhafeeri.
The development of statistical software in research has transformed the way scientists and researchers conduct their statistical analysis. Despite these advancements, it was not clear which statistical software is mainly used for which research design thereby creating confusion and uncertainty in choosing the right statistical tools. Therefore, this study aimed to review the trend of statistical software usage and their associated study designs in articles published in health sciences research.
This bibliometric analysis study reviewed 10,596 articles published in PubMed in three 10-year intervals (1997, 2007, and 2017). The data were collected through Google sheet and were analyzed using SPSS software. This study described the trend and usage of currently available statistical tools and the different study designs that are associated with them.
Of the statistical software mentioned in the retrieved articles, SPSS was the most common statistical tool used (52.1%) in the three-time periods followed by SAS (12.9%) and Stata (12.6%). WinBugs was the least used statistical software with only 40(0.6%) of the total articles. SPSS was mostly associated with observational (61.1%) and experimental (65.3%) study designs. On the other hand, Review Manager (43.7%) and Stata (38.3%) were the most statistical software associated with systematic reviews and meta-analyses.
In this study, SPSS was found to be the most widely used statistical software in the selected study periods. Observational studies were the most common health science research design. SPSS was associated with observational and experimental studies while Review Manager and Stata were mostly used for systematic reviews and meta-analysis.
Introduction
With the evolution of open access in the publishing world, access to empirical research has never been more widespread than it is now. For most of the researchers, however, the key feature of their articles is the robustness and repeatability of their methods section particularly the design of the study and the type of statistical tests to employ. The emergency of statistical software has transformed the way scientists and researchers conducting their statistical analysis. Therefore, performing complex and at times erroneous statistical analysis manually has become thing of the past [ 1 ].
Statistical software has many useful applications for researchers in the healthcare sciences. Furthermore, the researchers conveniently read their data by representing their data as visual aids using charts and graphs [ 2 ]. It also helps the researchers to easily calculate their results using statistical tests by accounting for their variables either numerical, categorical, or both [ 2 ]. However, in the past few decades, statistical software usage went through different stages based on their development and applications [ 3 ]. Although some software are more dedicated to a specific field, the degree of usage of specific software may depend on the preference of the investigators or the type of study design that is selected in their research.
There are different types of statistical software and each of these is used for a different type of study. In a study of the popularity of statistical software in research, Muenchen R (2016) found that the Statistical Package for Social Sciences (SPSS) is by far the most popular statistical software used in epidemiological studies [ 3 ]. Another example of popular software that is used is SAS (Statistical Analysis Systems), which is statistical software that is considered to be flexible and has better graphical facilities compared to SPSS [ 4 ]. However, it can be difficult for novice users compared to SPSS due to its more advanced commands [ 4 ]. Another statistical software that is used in health sciences research is Stata. It allows users standard and non-standard methods of data analysis due to its ability to implement a powerful programming language in the analysis for a particular use. However, some of these features may be more difficult to use than other applications [ 5 ].
A study conducted in the United States has found that about 61% of original research articles in the Journal of Health Services Research have specified which statistical software they used for the data analysis [ 6 ]. Researchers also found that Stata and SAS were the predominant statistical software used in the reviewed articles. Another study about the use of statistical software and the various ways of measuring the popularity or their market share showed that SAS and SPSS were most popular in the business world. However, SAS was found to be the most popular in job openings followed by SPSS which had just less than double the Minitab, and R-project software had one-quarter of Minitab [ 7 ]. Another similar study was conducted in Pakistan which focused on the type of study design and the statistical software used in two local journals. The investigators found that SPSS was the most commonly used software while cross-sectional study design dominated the articles published [ 8 ].
Despite the development of the different statistical software and the speed with which these tools are produced, it may be very hard for the researchers to choose which statistical software they employ given the type of study design. This is complicated by the fact that all commercially available statistical tools strive to accommodate almost all features researchers need to analyze their data. Regardless of the type of study design, the availability of these statistical software, and the familiarity of the analysts in a particular software may greatly influence which statistical tool to use during the analysis. The type of statistical software and the choice of studies they are used for in health sciences is currently under-researched either because researchers have little knowledge about the applicability of specific statistical tools or the institutional decision on which software to use for analysis. To our knowledge, no study investigated the association between the use of statistical software and the chosen study design in different health sciences fields. Therefore, this study aimed to review the trend of the statistical software usage and their associated study designs among published health sciences articles in three time periods: 1997, 2007, and 2017.
Materials and methods
This bibliometric web-based study covered 14 different statistical software. Because of health sciences being a vast and highly researched area, investigators have chosen to limit their search to one database, PubMed. This database comprises more than 30 million articles of health-related disciplines. The data collection was limited to a 10-year interval (1997, 2007, and 2017), and was accomplished from October 2018 to May 2019. The study employed a semi-structured review process to select the articles for the study. The main inclusion criterion was that the article selected used one of the following statistical software either mentioned in the abstract, methods, or in the full text if available: GraphPad, MedCalc, JMP, LISREL, WinBUGS, Review Manager, Microsoft Excel, Minitab, SAS, Epi Info, Stata, SPSS, Statistica, R Project. However, any article mentioned a similar name but did not mean the statistical software was excluded. The initial search generated 10,596 articles and the process used was as follows: All the 14 statistical software mentioned above were searched together using the Boolean operator “OR” as the connector word. Multiple names were included for the last software (R Project) because it had been mentioned in different articles with different names. Next, articles identified were filtered based on the specified periods which were 1997, 2007, and 2017. No additional filters or other restrictions such as the article language were applied.
For each selected article, the statistical software used and the study design employed were identified by reading the title and abstract. If none of the statistical software was found or the name was an acronym other than the software (e.g., SAS as SATB2-associated syndrome), then the article’s full text was examined. If the full text is not available, that article was excluded. Similarly, the study design was checked in the title and in the abstract and if not evident, the full text was reviewed if available. If two or more study designs were reported in the article, then the main study design was considered. Lastly, the PubMed identifier (PMID) number was added to avoid any errors or article duplications.
Figure Figure1 1 represented the PRISMA flow diagram of the inclusion and exclusion process of the articles. The initial search for the three-time periods specified yielded 10,596 articles. Of those 1,169 articles were excluded because of lack of access to the abstract, the free full text or no software was mentioned. Furthermore, 2,958 articles were excluded because of wrong abbreviations or the acronym had a different meaning. Finally, 6,469 articles were included in the present study. The data were collected through a Google sheet and were analyzed using IBM SPSS Statistics for Windows, Version 24.0 (IBM Corp., Armonk, NY). Categorical data were presented as frequencies and percentages. Bar charts were used to display software usage across the study periods.

Of the 10,596 generated during the literature search, 6,469 articles that were published in the years 1997, 2007, and 2017 were included in the final review. The percentages of the statistical software used in these articles are shown in Figure Figure2. 2 . SPSS was the most commonly used statistical software for data analysis with 3,368 (52.1%) articles, followed by SAS 833 (12.9%), and Stata 815 (12.6%). WinBugs was the least used statistical software with only 40 (0.6%) of the total articles.

The total percentage was 113.3% since some articles used more than one software.
As shown in Figure Figure3, 3 , SPSS was found to be the most commonly used statistical software throughout the study periods 1997 (27.9%), 2007 (59.7%), and 2017 (51.3%). SAS was second to SPSS in the first two periods while in 2017, its use has shifted down to fifth compared to Stata. Other software that have gained popularity included R-project and Review Manager. In the first time period (1997), the articles that used these tools were very few. However, in 2017, their use has shifted up to third (11.4%) and sixth (5.7%), respectively.

Of the 6,469 reviewed articles, 6,342 (98%) had clearly mentioned study designs and the rest 127 (2%) were either not clear or not mentioned in the articles. The study designs were classified into four main types: observational 4,763 (75.1%), experimental 736 (11.6%), systematic review 661 (10.4%), and research support\review article 218 (2.9%) (Figure (Figure4). 4 ). Among the observational studies, cross-sectional was the most frequently used study design with 3,585 (75.3%). In experimental study designs, randomized controlled trials were the most used design with 520 (70.7%) in the reviewed articles. Around three-quarters of the systematic review articles, 506 (76.6%) also included meta-analyses.

Association between statistical software used and study design employed is shown in Table Table1. 1 . The majority of the articles on systematic reviews\meta-analysis designs opted to use Review Manager (43.7%) followed by Stata (38.3%). Two-thirds of experimental studies used SPSS software for data analysis and only SAS software was the other major tool used in these studies. For the observational studies, again SPSS was the predominant statistical software used (61.1%) and the rest of the percentages were distributed among other statistical tools. Most review articles used R-project (60.2%) followed by SAS (27.7%) with only 6.6% of the review articles used SPSS.
Software | Systematic review\meta-analysis (n = 661) | Experimental (n = 736) | Observation (n = 4,763) | Review articles/Research support (n = 218) |
Epi-Info | 0.0% | 0.0% | 5.9% | 0.0% |
Excel | 5.3% | 5.2% | 8.6% | 3.6% |
Graphpad | 0.4% | 1.2% | 0.7% | 0.0% |
JMP | 0.1% | 0.2% | 0.6% | 0.6% |
Lisrel | 0.0% | 0.6% | 1.3% | 0.0% |
Medcalc | 0.6% | 0.2% | 0.5% | 0.6% |
Minitab | 0.0% | 0.4% | 0.0% | 0.0% |
Review Manager | 43.7% | 0.0% | 0.0% | 0.0% |
R-Project | 6.9% | 3.4% | 4.0% | 60.2% |
SAS | 0.9% | 16.5% | 7.5% | 27.7% |
SPSS | 2.7% | 65.3% | 61.1% | 6.6% |
Stata | 38.3% | 5.2% | 8.6% | 0.6% |
Statistica | 0.0% | 0.4% | 1.0% | 0.0% |
Winbugs | 1.1% | 1.2% | 0.2% | 0.0% |
Total | 100.0% | 100.0% | 100.0% | 100.0% |
The relationship between the use of statistical software and the type of research designs in health sciences is not well understood. Therefore, the aim of this study was to describe the trends of the statistical software usage and their associated study designs among published health sciences articles in three time periods. While a five-year interval was possible, the number of articles required to be included would have been overwhelming. However, this study included articles published at a 10-year interval in 1997, 2007, and 2017. With the current search strategy, the amount of data collected exceeded 10,000 articles. One important issue during the data collection was the ambiguity in the abbreviation of names for the statistical software. For example, when typing the abbreviation SAS (Statistical Analysis System) on the PubMed search engine, the search results are sometimes mixed up with the abbreviation of sleep apnea scale or subarachnoid space, there was also a marked difference in software usage across all the years.
Overall, SPSS was found to be the most popular statistical software followed by SAS and Stata. When examined the use of the statistical software, SPSS was found to be the most popular tool in the chosen three time periods. The positions of the other statistical tools fluctuated in terms of their use in the health sciences. Regarding the associated study designs, observational studies and in particular cross-sectional were found to be the predominant when compared to other study designs. This study also found that SPSS was mostly used for observational and experimental studies while Review Manager and Stata were mostly associated with systematic reviews and meta-analysis.
This study included articles in all health sciences regardless of where they were published. However, unlike our study, some articles which reported the use of statistical software have limited their search to a specific region or local journals [ 6 , 8 ]. This study found that SPSS was the most used software worldwide. In contrast, a study conducted in the United States found that Stata was the most commonly used statistical software employed in health services followed by SAS [ 7 ]. Suggesting that there could be geographical variation in the use of statistical software. Another study conducted in Pakistan which included articles published in two local journals found that SPSS was the most commonly used statistical software [ 8 ].
Other reasons that may have caused the variation of the statistical software packages may include the availability of these tools in different parts of the world and the preferences of the researchers. In the US study, for example, close to 50% of US-based researchers used Stata while the percentage of non-US articles that used Stata was only 15% [ 7 ].
For the study design, the current study found that around three-quarters of observational studies were cross-sectional. Our finding agreed with a study conducted in Saudi Arabia which reported almost a similar percentage [ 9 ]. However, the Pakistani study found half the percentage of both studies [ 8 ]. Regarding the other study designs, only 10.4% of the articles were systematic reviews or meta-analyses in this study. This lower percentage found in this study agrees with a study in the United States that investigated the relationship between the type of study design and the chances of citation in the first two years. They reported that only 4% of the 624 articles were meta-analyses or systematic reviews [ 10 ].
Limitations
Because of logistical and personnel issues, the current study only used the PubMed database. Lack of access to the full text of the retrieved titles caused a number of articles to be excluded. This may have introduced bias in reporting the type of statistical software or the chosen study design. This study depended on the reported study designs and did not verify their accuracy, as it was not the main aim of the study.
Conclusions
The purpose of this study was to inform researchers about the usage of the different statistical software packages and their associated study designs in health sciences research. In this study, SPSS was found to be the most widely used statistical software throughout the whole study period. The observational studies were the dominating health science research design with cross-sectional studies being the most common study design. SPSS was associated with observational and experimental studies while Review Manager and Stata were mostly used for systematic reviews and meta-analysis. As this the first wide-ranging study about the statistical software use and the associated study designs, we envisage that it will be of benefit to researchers to choose the most probable statistical software regarding their chosen study design.
The content published in Cureus is the result of clinical experience and/or research by independent individuals or organizations. Cureus is not responsible for the scientific accuracy or reliability of data or conclusions published herein. All content published within Cureus is intended only for educational, research and reference purposes. Additionally, articles published within Cureus should not be deemed a suitable substitute for the advice of a qualified health care professional. Do not disregard or avoid professional medical advice due to content published within Cureus.
The authors have declared that no competing interests exist.
Human Ethics
Consent was obtained by all participants in this study
Animal Ethics
Animal subjects: All authors have confirmed that this study did not involve animal subjects or tissue.
The state of AI in early 2024: Gen AI adoption spikes and starts to generate value
If 2023 was the year the world discovered generative AI (gen AI) , 2024 is the year organizations truly began using—and deriving business value from—this new technology. In the latest McKinsey Global Survey on AI, 65 percent of respondents report that their organizations are regularly using gen AI, nearly double the percentage from our previous survey just ten months ago. Respondents’ expectations for gen AI’s impact remain as high as they were last year , with three-quarters predicting that gen AI will lead to significant or disruptive change in their industries in the years ahead.
About the authors
This article is a collaborative effort by Alex Singla , Alexander Sukharevsky , Lareina Yee , and Michael Chui , with Bryce Hall , representing views from QuantumBlack, AI by McKinsey, and McKinsey Digital.
Organizations are already seeing material benefits from gen AI use, reporting both cost decreases and revenue jumps in the business units deploying the technology. The survey also provides insights into the kinds of risks presented by gen AI—most notably, inaccuracy—as well as the emerging practices of top performers to mitigate those challenges and capture value.
AI adoption surges
Interest in generative AI has also brightened the spotlight on a broader set of AI capabilities. For the past six years, AI adoption by respondents’ organizations has hovered at about 50 percent. This year, the survey finds that adoption has jumped to 72 percent (Exhibit 1). And the interest is truly global in scope. Our 2023 survey found that AI adoption did not reach 66 percent in any region; however, this year more than two-thirds of respondents in nearly every region say their organizations are using AI. 1 Organizations based in Central and South America are the exception, with 58 percent of respondents working for organizations based in Central and South America reporting AI adoption. Looking by industry, the biggest increase in adoption can be found in professional services. 2 Includes respondents working for organizations focused on human resources, legal services, management consulting, market research, R&D, tax preparation, and training.
Also, responses suggest that companies are now using AI in more parts of the business. Half of respondents say their organizations have adopted AI in two or more business functions, up from less than a third of respondents in 2023 (Exhibit 2).
Gen AI adoption is most common in the functions where it can create the most value
Most respondents now report that their organizations—and they as individuals—are using gen AI. Sixty-five percent of respondents say their organizations are regularly using gen AI in at least one business function, up from one-third last year. The average organization using gen AI is doing so in two functions, most often in marketing and sales and in product and service development—two functions in which previous research determined that gen AI adoption could generate the most value 3 “ The economic potential of generative AI: The next productivity frontier ,” McKinsey, June 14, 2023. —as well as in IT (Exhibit 3). The biggest increase from 2023 is found in marketing and sales, where reported adoption has more than doubled. Yet across functions, only two use cases, both within marketing and sales, are reported by 15 percent or more of respondents.
Gen AI also is weaving its way into respondents’ personal lives. Compared with 2023, respondents are much more likely to be using gen AI at work and even more likely to be using gen AI both at work and in their personal lives (Exhibit 4). The survey finds upticks in gen AI use across all regions, with the largest increases in Asia–Pacific and Greater China. Respondents at the highest seniority levels, meanwhile, show larger jumps in the use of gen Al tools for work and outside of work compared with their midlevel-management peers. Looking at specific industries, respondents working in energy and materials and in professional services report the largest increase in gen AI use.
Investments in gen AI and analytical AI are beginning to create value
The latest survey also shows how different industries are budgeting for gen AI. Responses suggest that, in many industries, organizations are about equally as likely to be investing more than 5 percent of their digital budgets in gen AI as they are in nongenerative, analytical-AI solutions (Exhibit 5). Yet in most industries, larger shares of respondents report that their organizations spend more than 20 percent on analytical AI than on gen AI. Looking ahead, most respondents—67 percent—expect their organizations to invest more in AI over the next three years.
Where are those investments paying off? For the first time, our latest survey explored the value created by gen AI use by business function. The function in which the largest share of respondents report seeing cost decreases is human resources. Respondents most commonly report meaningful revenue increases (of more than 5 percent) in supply chain and inventory management (Exhibit 6). For analytical AI, respondents most often report seeing cost benefits in service operations—in line with what we found last year —as well as meaningful revenue increases from AI use in marketing and sales.
Inaccuracy: The most recognized and experienced risk of gen AI use
As businesses begin to see the benefits of gen AI, they’re also recognizing the diverse risks associated with the technology. These can range from data management risks such as data privacy, bias, or intellectual property (IP) infringement to model management risks, which tend to focus on inaccurate output or lack of explainability. A third big risk category is security and incorrect use.
Respondents to the latest survey are more likely than they were last year to say their organizations consider inaccuracy and IP infringement to be relevant to their use of gen AI, and about half continue to view cybersecurity as a risk (Exhibit 7).
Conversely, respondents are less likely than they were last year to say their organizations consider workforce and labor displacement to be relevant risks and are not increasing efforts to mitigate them.
In fact, inaccuracy— which can affect use cases across the gen AI value chain , ranging from customer journeys and summarization to coding and creative content—is the only risk that respondents are significantly more likely than last year to say their organizations are actively working to mitigate.
Some organizations have already experienced negative consequences from the use of gen AI, with 44 percent of respondents saying their organizations have experienced at least one consequence (Exhibit 8). Respondents most often report inaccuracy as a risk that has affected their organizations, followed by cybersecurity and explainability.
Our previous research has found that there are several elements of governance that can help in scaling gen AI use responsibly, yet few respondents report having these risk-related practices in place. 4 “ Implementing generative AI with speed and safety ,” McKinsey Quarterly , March 13, 2024. For example, just 18 percent say their organizations have an enterprise-wide council or board with the authority to make decisions involving responsible AI governance, and only one-third say gen AI risk awareness and risk mitigation controls are required skill sets for technical talent.
Bringing gen AI capabilities to bear
The latest survey also sought to understand how, and how quickly, organizations are deploying these new gen AI tools. We have found three archetypes for implementing gen AI solutions : takers use off-the-shelf, publicly available solutions; shapers customize those tools with proprietary data and systems; and makers develop their own foundation models from scratch. 5 “ Technology’s generational moment with generative AI: A CIO and CTO guide ,” McKinsey, July 11, 2023. Across most industries, the survey results suggest that organizations are finding off-the-shelf offerings applicable to their business needs—though many are pursuing opportunities to customize models or even develop their own (Exhibit 9). About half of reported gen AI uses within respondents’ business functions are utilizing off-the-shelf, publicly available models or tools, with little or no customization. Respondents in energy and materials, technology, and media and telecommunications are more likely to report significant customization or tuning of publicly available models or developing their own proprietary models to address specific business needs.
Respondents most often report that their organizations required one to four months from the start of a project to put gen AI into production, though the time it takes varies by business function (Exhibit 10). It also depends upon the approach for acquiring those capabilities. Not surprisingly, reported uses of highly customized or proprietary models are 1.5 times more likely than off-the-shelf, publicly available models to take five months or more to implement.
Gen AI high performers are excelling despite facing challenges
Gen AI is a new technology, and organizations are still early in the journey of pursuing its opportunities and scaling it across functions. So it’s little surprise that only a small subset of respondents (46 out of 876) report that a meaningful share of their organizations’ EBIT can be attributed to their deployment of gen AI. Still, these gen AI leaders are worth examining closely. These, after all, are the early movers, who already attribute more than 10 percent of their organizations’ EBIT to their use of gen AI. Forty-two percent of these high performers say more than 20 percent of their EBIT is attributable to their use of nongenerative, analytical AI, and they span industries and regions—though most are at organizations with less than $1 billion in annual revenue. The AI-related practices at these organizations can offer guidance to those looking to create value from gen AI adoption at their own organizations.
To start, gen AI high performers are using gen AI in more business functions—an average of three functions, while others average two. They, like other organizations, are most likely to use gen AI in marketing and sales and product or service development, but they’re much more likely than others to use gen AI solutions in risk, legal, and compliance; in strategy and corporate finance; and in supply chain and inventory management. They’re more than three times as likely as others to be using gen AI in activities ranging from processing of accounting documents and risk assessment to R&D testing and pricing and promotions. While, overall, about half of reported gen AI applications within business functions are utilizing publicly available models or tools, gen AI high performers are less likely to use those off-the-shelf options than to either implement significantly customized versions of those tools or to develop their own proprietary foundation models.
What else are these high performers doing differently? For one thing, they are paying more attention to gen-AI-related risks. Perhaps because they are further along on their journeys, they are more likely than others to say their organizations have experienced every negative consequence from gen AI we asked about, from cybersecurity and personal privacy to explainability and IP infringement. Given that, they are more likely than others to report that their organizations consider those risks, as well as regulatory compliance, environmental impacts, and political stability, to be relevant to their gen AI use, and they say they take steps to mitigate more risks than others do.
Gen AI high performers are also much more likely to say their organizations follow a set of risk-related best practices (Exhibit 11). For example, they are nearly twice as likely as others to involve the legal function and embed risk reviews early on in the development of gen AI solutions—that is, to “ shift left .” They’re also much more likely than others to employ a wide range of other best practices, from strategy-related practices to those related to scaling.
In addition to experiencing the risks of gen AI adoption, high performers have encountered other challenges that can serve as warnings to others (Exhibit 12). Seventy percent say they have experienced difficulties with data, including defining processes for data governance, developing the ability to quickly integrate data into AI models, and an insufficient amount of training data, highlighting the essential role that data play in capturing value. High performers are also more likely than others to report experiencing challenges with their operating models, such as implementing agile ways of working and effective sprint performance management.
About the research
The online survey was in the field from February 22 to March 5, 2024, and garnered responses from 1,363 participants representing the full range of regions, industries, company sizes, functional specialties, and tenures. Of those respondents, 981 said their organizations had adopted AI in at least one business function, and 878 said their organizations were regularly using gen AI in at least one function. To adjust for differences in response rates, the data are weighted by the contribution of each respondent’s nation to global GDP.
Alex Singla and Alexander Sukharevsky are global coleaders of QuantumBlack, AI by McKinsey, and senior partners in McKinsey’s Chicago and London offices, respectively; Lareina Yee is a senior partner in the Bay Area office, where Michael Chui , a McKinsey Global Institute partner, is a partner; and Bryce Hall is an associate partner in the Washington, DC, office.
They wish to thank Kaitlin Noe, Larry Kanter, Mallika Jhamb, and Shinjini Srivastava for their contributions to this work.
This article was edited by Heather Hanselman, a senior editor in McKinsey’s Atlanta office.
Explore a career with us
Related articles.

Moving past gen AI’s honeymoon phase: Seven hard truths for CIOs to get from pilot to scale
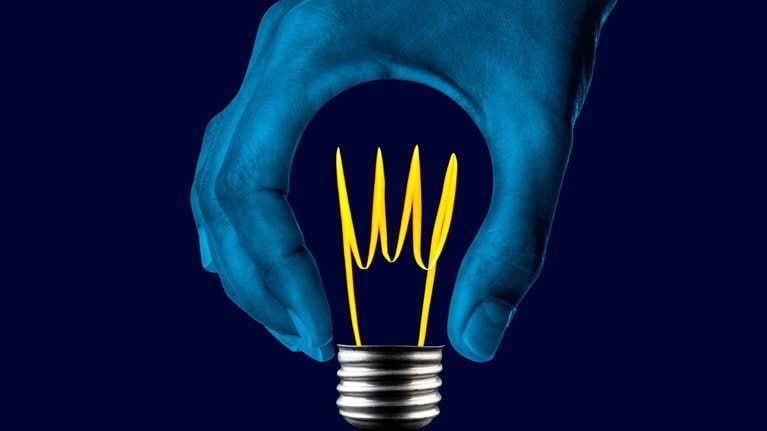
A generative AI reset: Rewiring to turn potential into value in 2024

Implementing generative AI with speed and safety
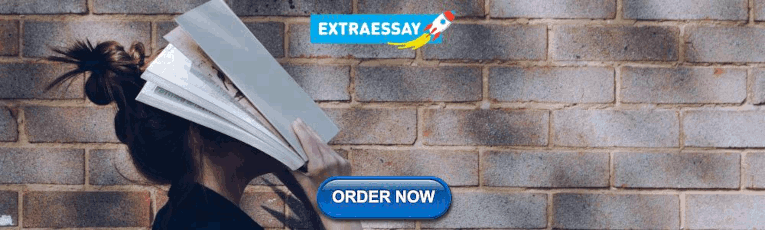
IMAGES
VIDEO
COMMENTS
A large-scale dataset with approximately 14 million code samples, each of which is an intended solution to one of 4000 coding problems. Rich annotation enables research in code search, code completion, code-code translation, and myriad other use cases.
Since our first lab opened in 1945, we've authored more than 110,000 research publications. Our researchers have won six Nobel Prizes, six Turing Awards, and IBM has been granted more than 150,000 patents.
This is our catalog of publications authored by IBM researchers, in collaboration with the global research community. We're currently adding our back catalog of more than 110,000 publications. It's an ever-growing body of work that shows why IBM is one of the most important contributors to modern computing. Filter by.
With over 3,000 researchers across the globe, IBM Research has a long pedigree of turning fundamental research into world-altering technology. Learn more about the ways that we collaborate with businesses and organizations across the globe to help solve their most pressing needs faster. We're inventing what's next in AI research.
Today, IBM Research stands at the forefront of computing. Our semiconductor research is pushing the limits of scaling and redefining the way chips are designed; we're creating new foundation models and hardware for the next generation of enterprise AI; we're a world leader in quantum computing; and we're envisioning a multi-cloud platform ...
The IBM Institute for Business Value uses data-driven research and expert analysis to deliver thought-provoking insights to leaders on the emerging trends that will determine future success.'
A global community. A global. community. We're a group of 3,000 researchers inventing what's next in computing at labs across the world. Learn more about us and our work below. Filter by.
IBM Research is headquartered at the Eero Saarinen-designed Thomas J. Watson Research Center in Yorktown Heights, New York.. IBM Research is the research and development division for IBM, an American multinational information technology company headquartered in Armonk, New York, with operations in over 170 countries.IBM Research is the largest industrial research organization in the world and ...
Focusing on accelerated discovery, IBM Research is leveraging next-generation computing technologies—artificial intelligence, the hybrid cloud, and quantum computing—to streamline and optimize ...
IBM Research is a group of researchers, scientists, technologists, designers, and thinkers inventing what's next in computing. We're relentlessly curious about all the ways that computing can ...
IBM Research Europe - United Kingdom. Working across two locations (Daresbury and Hursley), our teams have been constantly contributing to creating what's next in computing. Our research is motivated by today's most pressing challenges both in the global context and specific to UK industries and institutions. We bring cutting-edge ...
2022 consumer study: Consumers want it all | IBM. Consumer demands are shifting—and retailers and brands need to adapt to deliver. Learn how sustainability expectations and the shopping experience have evolved.
This year, Cleveland Clinic London will expand its clinical research studies and the number of patients enrolled in the BioResource. ... Discovery Accelerator - which combines Cleveland Clinic's renowned expertise in healthcare and biomedical research with IBM's next-generation technologies to accelerate research. Through the partnership ...
Design research guides teams to uncover insights and inform the experiences we create. It begins with the rigorous study of the people we serve and their context. This is the heart of Enterprise Design Thinking. While in the Loop, design research leads teams to continuously build understanding and empathy through observation, prototyping ...
But business leaders are facing a host of talent-related challenges, as a new global study from the IBM Institute for Business Value (IBV) reveals, from the skills gap to shifting employee expectations to the need for new operating models. The global skills gap is real and growing. Executives surveyed estimate that 40% of their workforce will ...
A new global study by the IBM Institute for Business Value found that while a majority of organizations recognize sustainability as important to their business strategy, many C-suite leaders are struggling to fund their sustainability investments. Data collected from 5,000 global C-suite executives highlighted that organizations that embed sustainability within their operations see better ...
Only 23% of CIOs surveyed expect remote workplace changes from the COVID-19 pandemic to become permanent. ARMONK, N.Y., Nov. 9, 2021 /PRNewswire/ -- A new IBM (NYSE: IBM) Institute for Business Value (IBV) study revealed CIOs' influence on business strategy and operations is growing as technology pervades surveyed enterprises. When asked which other C-Suite members will be most critical over ...
Our research-driven insights show how innovative enterprises are taking steps toward hybrid cloud mastery and reaping the benefits.
About the Study Conducted by the ... The portfolio, supported by world-renowned IBM X-Force® research, enables organizations to effectively manage risk and defend against emerging threats. IBM operates one of the world's broadest security research, development and delivery organizations, monitors 70 billion security events per day in more than ...
What is top of mind for global business leaders? Every year, the IBM Institute for Business Value (IBV) interviews C-suite leaders from around the world to discover what outperformers do differently. Since 2003, the IBV has collected data and insights from more than 50,000 executives and academi…
The global average cost of a data breach in 2023 was USD 4.45 million, a 15% increase over 3 years. 51% of organizations are planning to increase security investments as a result of a breach, including incident response (IR) planning and testing, employee training, and threat detection and response tools. The average savings for organizations ...
Every year, IBM Canada Advanced Studies recognizes the most outstanding research projects, students, professors, and IBM product experts that exemplify drive for innovation, collaboration and forward thinking research. These awards are presented at our annual CASCON x EVOKE academic tech conference - a space to engage and learn with a community ...
It was in the latter role that he became entrenched in systematic research which would later hone in on the field of cross-cultural studies. International Employee Opinion Research Program. In his role as manager of staff research, IBM's International Employee Opinion Research Program became Hofstede's brainchild.
A study conducted in the United States has found that about 61% of original research articles in the Journal of Health Services Research have specified which statistical software they used for the data analysis . Researchers also found that Stata and SAS were the predominant statistical software used in the reviewed articles.
About the research. The online survey was in the field from February 22 to March 5, 2024, and garnered responses from 1,363 participants representing the full range of regions, industries, company sizes, functional specialties, and tenures. Of those respondents, 981 said their organizations had adopted AI in at least one business function, and ...