Faculty - January 2, 2024
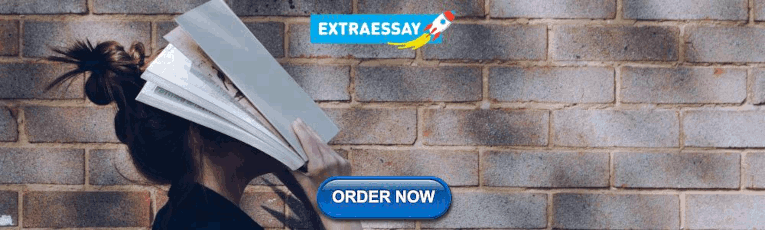
The Real Impact of Fake News: The Rise of Political Misinformation—and How We Can Combat Its Influence
- Political Analytics
- Strategic Communication
What is the difference between misinformation and disinformation?
That was among the key questions considered at the recent Strategies for Combating Political Misinformation panel hosted by the Columbia University School of Professional Studies (SPS) Strategic Communication and Political Analytics graduate programs. The discussion centered on the varying factors that determine the influence of misinformation on beliefs, and what strategies can be used to combat it effectively.
Dr. Kristine Billmyer, program director of the M.S. in Strategic Communication, and Dr. Gregory Wawro, director and founder of the M.S. in Political Analytics program, welcomed attendees and introduced the panel’s moderator, Josie Cox, author of Women Money Power and an associate faculty member at SPS.
Jennifer Counter, vice president of cyber security solutions company Orbis Operations and an associate faculty member at SPS, shared a succinct distinction between misinformation and disinformation. Misinformation is like a game of telephone among family members who try to relay information accurately, she said, but don’t necessarily get the facts entirely right. Disinformation, on the other hand, is the intentional dissemination of false information.
Cox asked panelists how concerned they are about the state of misinformation and disinformation, the power and influence it has in our lives and communities today, and where they see things going over the next few years.
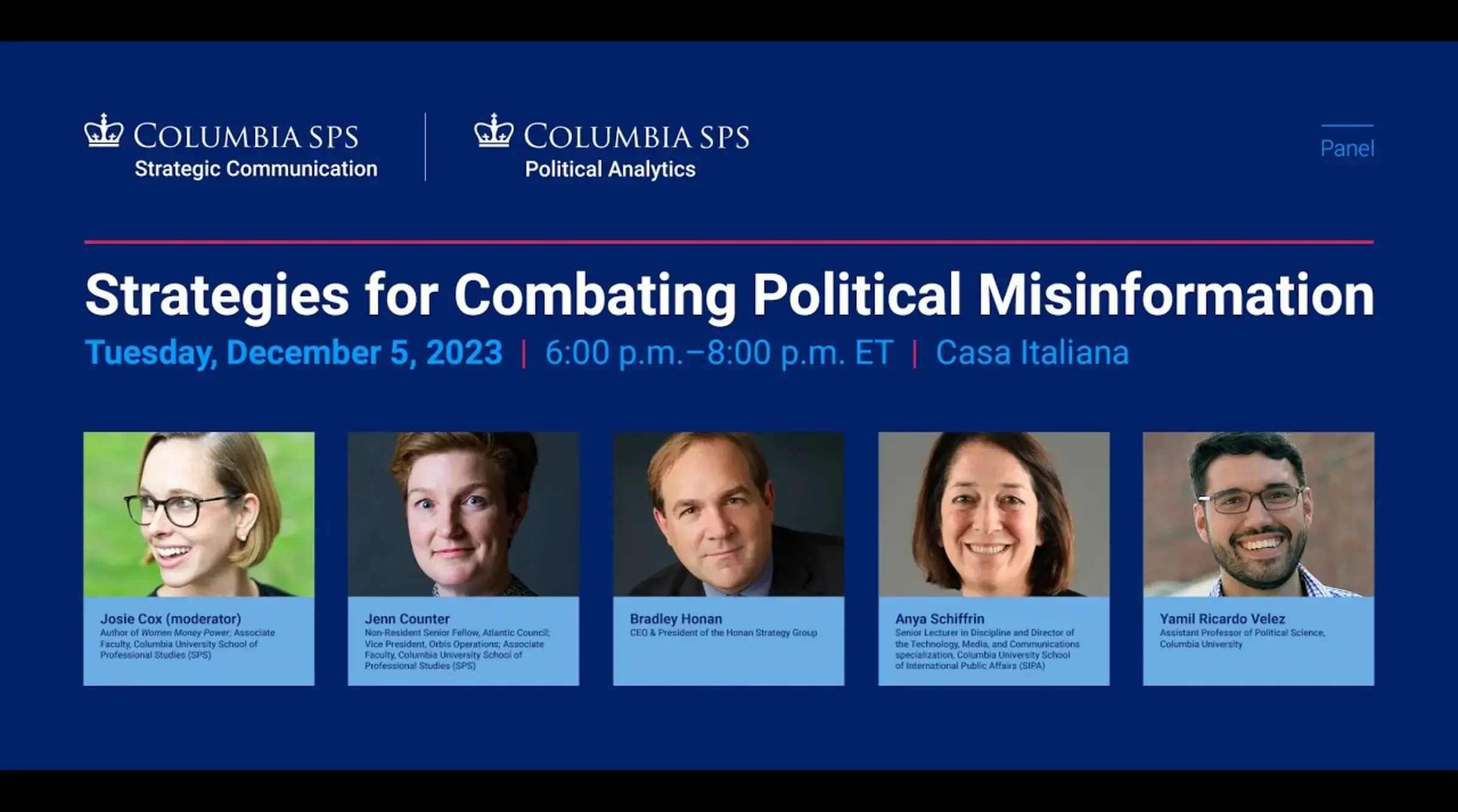
Panelist Anya Schiffrin, director of the Technology, Media, and Communications specialization at Columbia University School of International Public Affairs (SIPA), noted that she is highly concerned, pointing to the spread of misinformation currently occurring in the Middle East. Yamil Ricardo Velez, assistant professor of Political Science at Columbia, shared that while he acknowledged Schiffrin’s concern, he harbors a level of optimism for our ability to limit the negative impact of misinformation, pointing out that professionals and academics are working on solutions to the problems presented by misinformation.
But the scope of the problem isn’t to be underestimated, countered panelist Bradley Honan, CEO and president of Honan Strategy Group. “There’s been disinformation as long as humans have been talking to each other,” Honan said. “Having so many different channels and platforms allows information now to move as never before. Disinformation is becoming more persuasive because there’s so much of it.”
Honan also pointed out that misinformation could wind up being the decisive issue of the 2024 presidential election. In America, there is no regulation when it comes to fact-checking and misinformation, and the fact-checking that does exist has an English language bias, which means that large groups of people who don’t speak the language aren’t able to access the highest quality information because it simply isn’t made available to them.
The panel also discussed the role that local news plays in small, underserved communities known as “news deserts.” While the role of trusted local journalists is critical in combating misinformation, sufficient funding is unfortunately not in place to help grow those smaller newsrooms. Schiffrin mentioned that these local news teams ought to be funded by major tech companies like Google or Meta who have been profiting off these publishers’ work for years without compensation.
In addition to a call for more regulation, the panelists agreed that media literacy is a critical solution to solving the misinformation problem. Schiffrin pointed out that New Jersey just passed bipartisan legislation establishing K-12 information literacy education to help students evaluate and understand the news they encounter—the first curriculum of its kind in the country.
Counter spoke to the potentially problematic nature of AI-generated content. “There’s been content pushed out on social media inflaming things,” she said. “It’s going to democratize disinformation even more than it already does. People will not know what to believe.”
However, generative AI’s impact isn’t necessarily all negative when it comes to misinformation. Panelist Yamil Velez shared that, in isolated instances, generative AI can increase access for minority groups, citing the example of New York City Mayor Eric Adams, who used AI to communicate with non-English-speaking New Yorkers. However, both Velez and Counter added that even when used appropriately, content that uses AI should always be flagged.
The dissemination of misinformation is a problem that’s not going away any time soon, the panelists agreed, though they shared the hope that its impact could be limited through educational channels like media literacy—and insightful discussions such as this one.
About the Political Analytics Program
The Columbia University M.S. in Political Analytics program provides students quantitative skills in an explicitly political context, facilitating crosswalk with nontechnical professionals and decision-makers—and empowers students to become decision-makers themselves. The 36-point credit program is available part-time and full-time. Please complete this form more information.
About the Strategic Communication Program
The business world’s around-the-clock communications challenges are demanding a new level of strategic thinking. Columbia University’s Master of Science in Strategic Communication graduates emerge equipped with all the essential skills and tools for a successful career in a wide range of communication fields. Applications are open now for fall 2024 enrollment.
Related News
The growth of renewable energy and the need for decentralized sources of power the transition to renewable energy must be built on a business case: the financial benefits of a modernized energy system. faculty decoding political communications: insights on the 2024 election cycle strategic communication lecturer andrew whitehouse discusses evolving campaign tactics in the current presidential election. faculty fifteen years of columbia’s master of science in sustainability management program the ms in sustainability management program is welcoming its 15th class this tuesday. all news footer social links.
203 Lewisohn Hall 2970 Broadway, MC 4119 New York, NY, 10027
© Copyright 2019 Columbia University School of Professional Studies. Privacy Policy
Numbers, Facts and Trends Shaping Your World
Read our research on:
Full Topic List
Regions & Countries
- Publications
- Our Methods
- Short Reads
- Tools & Resources
Read Our Research On:
- How Americans Navigated the News in 2020: A Tumultuous Year in Review
- 3. Misinformation and competing views of reality abounded throughout 2020
Table of Contents
- 1. About a quarter of Republicans, Democrats consistently turned only to news outlets whose audiences aligned with them politically in 2020
- 2. Republicans who relied on Trump for news in 2020 diverged from others in GOP in views of COVID-19, election
- 4. Americans who mainly got news via social media knew less about politics and current events, heard more about some unproven stories
- 5. Republicans’ views on COVID-19 shifted over course of 2020; Democrats’ hardly budged
- Appendix: Measuring news sources used during the 2020 presidential election
- Acknowledgments
- Methodology
Unprecedented national news events, a sharp and sometimes hostile political divide, and polarized news streams created a ripe environment for misinformation and made-up news in 2020. The truth surrounding the two intense, yearlong storylines – the coronavirus pandemic and the presidential election – was often a matter of dispute, whether due to genuine confusion or the intentional distortion of reality.
Pew Research Center’s American News Pathways project revealed consistent differences in what parts of the population – including political partisans and consumers of particular news outlets – heard and believed about the developments involving COVID-19 and the election. For example, news consumers who consistently turned only to outlets with right-leaning audiences were more likely to hear about and believe in certain false or unproven claims . In some cases, the study also showed that made-up news and misinformation have become labels applied to pieces of news and information that do not fit into people’s preferred worldview or narrative – regardless of whether the information was actually made up.
Of course, differences in political party or news diet are not always linked with differences in perceptions of misinformation, nor are they the only factors that have an impact. As explained in Chapter 2 , using Donald Trump himself as a news source connects closely to beliefs about certain false claims and exposure to misinformation. So, too, does the reliance on social media as the primary pathway to one’s news, as discussed in Chapter 4 .
The Pathways project, then, revealed the degree to which the spread of misinformation is pervasive, but not uniform. Americans’ exposure to – and belief in – misinformation differs by both the specific news outlets and more general pathways they rely on most. Certain types of misinformation emerge more or less strongly within each of these. For example, Americans who rely most on social media for their news (and who also pay less attention to news generally and are less knowledgeable about it) get exposed to different misinformation threads than those who turn only to sources with right-leaning audiences, or to Trump. Both of these latter groups are also more ideologically united and pay very close attention to news.
Takeaway #1: Most Americans said they saw made-up news and expressed concern about it
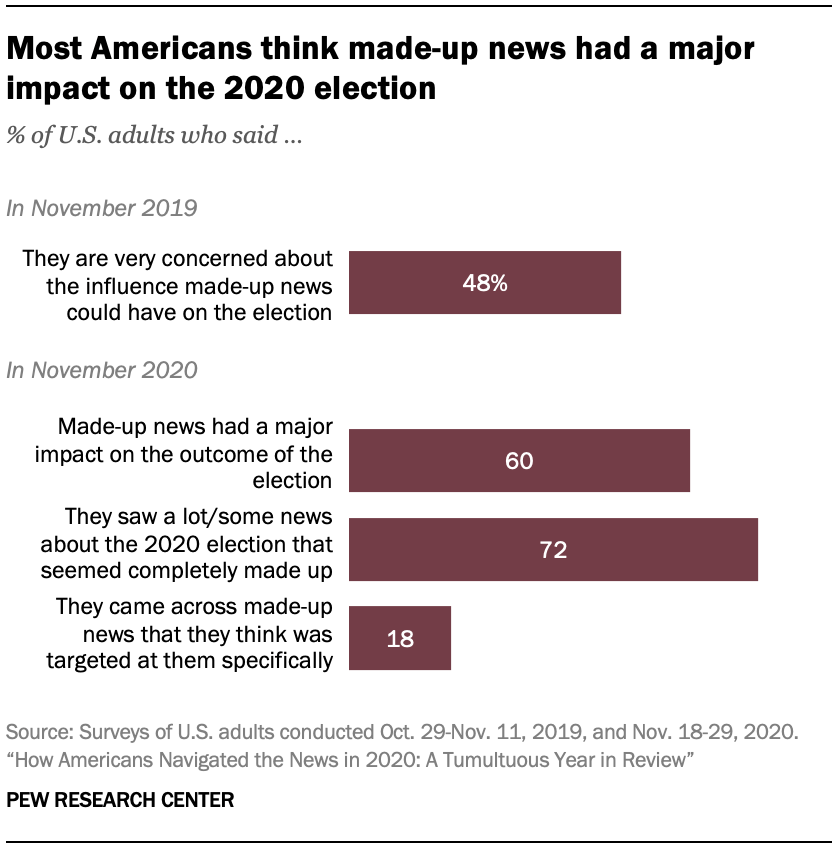
Even a year before the 2020 election, in November 2019, the vast majority of Americans said they were either “very” (48%) or “somewhat” (34%) concerned about the impact made-up news could have on the election. This concern cut across party lines, with almost identical shares of Democrats (including independents who lean toward the Democratic Party) and Republicans (including GOP leaners) expressing these views. But on both sides of the aisle, people were far more concerned that made-up news would be targeted at members of their own party rather than the other party.
A year later, in the weeks following the election, Americans said these fears were borne out: 60% of U.S. adults overall said they felt made-up news had a major impact on the outcome of the election, and an additional 26% said it had a minor impact. Republicans were more likely than Democrats to say it had a major impact (69% vs. 54%). In addition, nearly three-quarters of U.S. adults overall (72%) said they had come across at least “some” election news that seemed completely made up, though far fewer – 18% – felt the made-up news they saw was aimed directly at them.
During the year, many Americans also felt exposed to made-up news related to the coronavirus pandemic, a phenomenon that grew over time. As of mid-March 20 20, 48% of Americans said they had seen at least some news related to COVID-19 that seemed completely made up. By mid-April, that figure had risen to 64%.
Overall, older Americans, those who paid more attention to news and those who showed higher levels of knowledge on a range of core political questions expressed greater concern about the impact of made-up news. Republicans also expressed more concern and said it’s harder to identify what is true when it comes to COVID-19 news . Meanwhile, those who relied most on social media for political news tended to express less concern about made-up news.
Takeaway #2: What Americans categorized as made-up news varied widely – and often aligned with partisan views
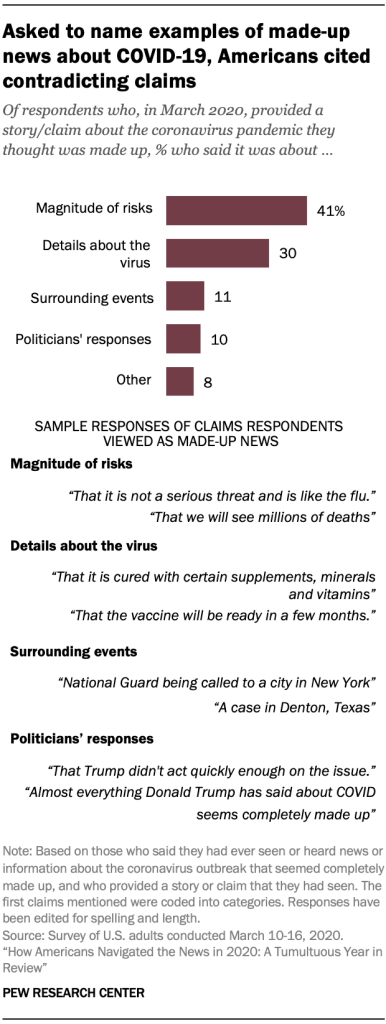
Especially in America’s polarized political environment, just because people say that something seemed made up doesn’t mean it was. Without a doubt, many Americans who report encountering made-up news actually did, while others likely came across real, fact-based news that did not fit into their perceptions of what is true. Indeed, open-ended survey responses show that people’s examples of made-up news they saw run the gamut – often connected with partisan divides about reality.
In March of 2020, after asking whether people had come across made-up news related to COVID-19, the American News Pathways project asked respondents to write in an example of something they came across that was made up . The responses were revealing, and sometimes contradictory: Roughly four-in-ten (41%) among those who provided an example named something related to the level of risk associated with the outbreak. Within this category, 22% said the “made-up” information falsely elevated the risks (Republicans were more likely to say this than Democrats), and 15% felt the made-up information was falsely downplaying the risks (Democrats were more likely to give these examples).
Respondents’ examples of made-up news that exaggerated the severity of the pandemic included such claims as numbers of COVID-19 deaths that seemed higher than possible, and the idea that risks had been overplayed by investors so they could make “gobs of money.” Some of these respondents said it was the media overhyping the risk, including one respondent who objected to a front-page newspaper photo designed to equate the coronavirus with the 1918 Spanish flu.
On the flip side, respondents’ examples of made-up news that underplayed COVID-19’s significance included references to statements made by Trump or his administration, including the then-president predicting an early end to the crisis and suggesting that the number of cases in the U.S. would remain low.
Three-in-ten respondents pointed to details about the virus itself. This included some truly made-up claims, such as that it could be “cured with certain supplements, minerals and vitamins,” and others that were perceived by respondents as made up but were not. For example, some respondents listed “wearing a mask for the general public” as an example of a misleading claim. Finally, 10% identified purely political statements as examples of misinformation, such as “That Trump didn’t act quickly enough,” or, by contrast, that “Almost everything Donald Trump has said” about the coronavirus has constituted made-up news.
Takeaway #3: While political divides were a big part of the equation, news diet within party has been a consistent factor in what Americans believe, whether true or untrue
In addition to wholly made-up claims, another finding to emerge from the Pathways project was the degree to which news diet also plays into the storylines – both true and untrue – that people get exposed to, how that feeds into perceptions about those events and, ultimately, different views of reality.
This phenomenon appears more strongly among Republicans than among Democrats, in large part due to the smaller mix of outlets Republicans tend to rely on – and within that, the outsize role of Fox News . (This is in addition to differences in perceptions and beliefs between Republicans who relied on Trump for news and those who didn’t, written about in Chapter 2 .)
Trump’s first impeachment
Consider one of the first news topics covered by the project: the 2019 impeachment of Donald Trump, which involved Trump’s behavior and motives in withholding military aid to Ukraine , as well as actions there by Democratic presidential candidate Joe Biden (whom Trump had asked Ukraine’s government to investigate).
A Pathways survey conducted in November 2019 found that Americans’ sense of the impeachment story connected closely with where they got their news. For instance, about half (52%) of Republicans who, among 30 outlets asked about in that survey, got political news only from outlets with right-leaning audiences had heard a lot about Biden’s efforts to remove a prosecutor in Ukraine in 2016. That is more than double the percentage of Democrats who got news only from outlets with left-leaning audiences (20%) who heard a lot. The gap is similar on Biden’s son (Hunter Biden) work with a Ukraine-based natural gas company: 64% of these Republicans had heard a lot about this, compared with 33% of these Democrats. (Details of the news outlet groupings and audience profiles can be found here .)

These patterns also play out in views about Joe Biden’s motivations. When asked, based on what they had heard in the news, whether they thought Biden called for the prosecutor’s removal in order to advance a U.S. government position to reduce corruption in Ukraine or to protect his son from being investigated, 81% of Republicans who got news only from outlets with right-leaning audiences said he wanted to protect his son. Only 2% of these Republicans thought it was part of a U.S. anti-corruption campaign.
Democrats who got news only from outlets with left-leaning audiences were much more inclined to attribute Biden’s actions to anti-corruption efforts (44%) than to a desire to protect his son (13%) – though that 44% is nearly matched by 42% who said they were not sure why Biden called for the prosecutor’s removal.
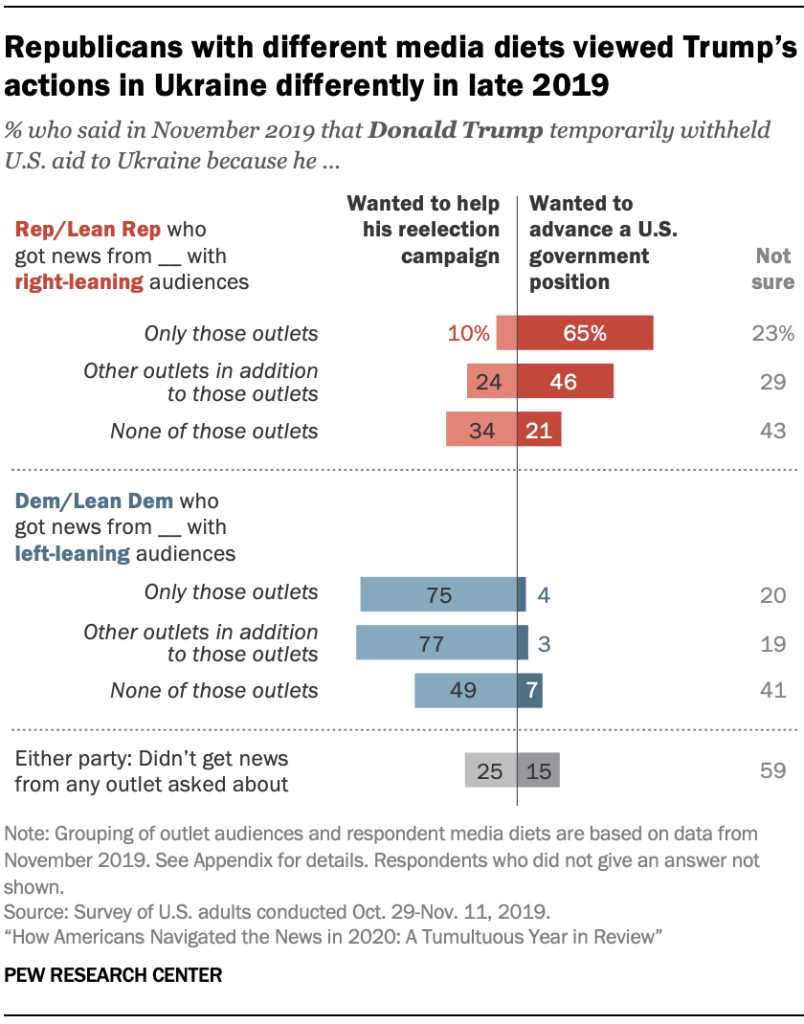
A similar gap is evident when it comes to views about Trump’s role in the Ukraine affair.
About two-thirds of Republicans and Republican leaners who got their political news only from media outlets with right-leaning audiences (65%) said he did it to advance a U.S. policy to reduce corruption in Ukraine. Just 10% of these Republicans said Trump withheld the aid to help his reelection campaign (23% said they weren’t sure).
Among Republicans who got political news from a combination of outlet types – some of which have right-leaning audiences and some which have mixed and/or left-leaning audiences – that gap narrows significantly. About half (46%) cited the advancement of U.S. policy, and 24% cited political gain. What’s more, Republicans who did not get news from any sources with right-leaning audiences (but did get news from outlets with mixed and/or left-leaning audiences) were more likely to say it was for political gain than to advance U.S. policy (34% vs. 21%), while 43% of Republicans in this group were not sure why he did it.
Among Democrats and Democratic leaners, those who got political news only on outlets with left-leaning audiences and those who got news from outlets with left-leaning audiences plus others that have mixed and/or right-leaning audiences responded similarly. Roughly three-quarters of Democrats in each of these groups (75% and 77%, respectively) said Trump withheld aid to help his reelection effort, while very small minorities of these Democrats (4% and 3%, respectively) cited reducing corruption as the president’s intent.
The coronavirus pandemic
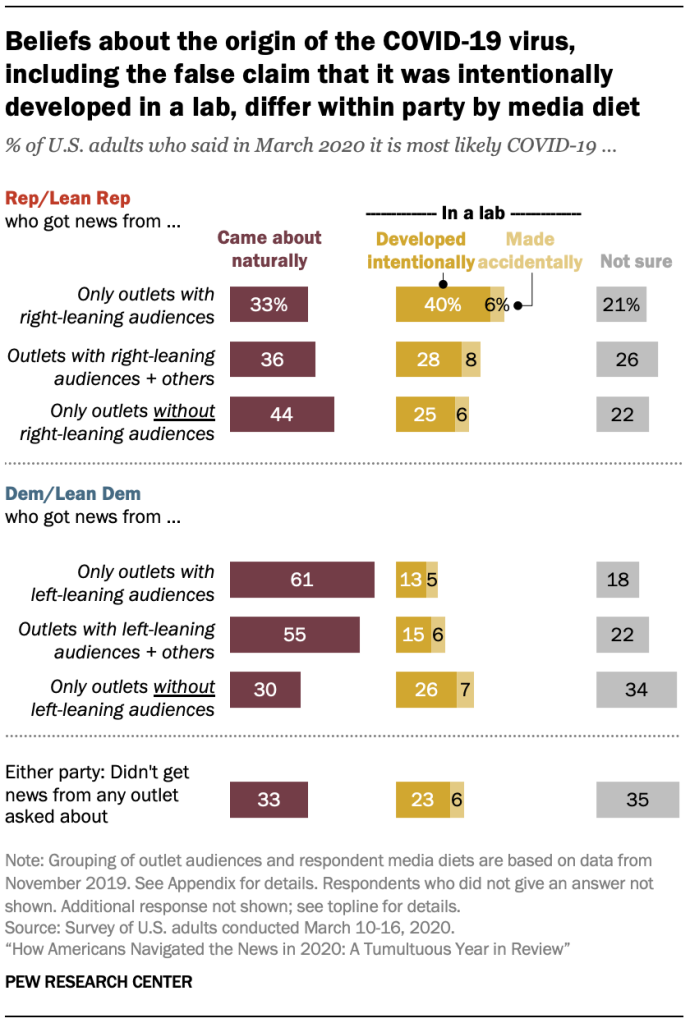
Several false claims related to the pandemic emerged over the course of the study. Not only did Republicans who turned to Trump for news about the pandemic express higher levels of belief in some of these claims (discussed in Chapter 2 ), but those who only relied on outlets with right-leaning audiences also stood out in this way (from that same initial group of 30).
One early claim, made without evidence, was that COVID-19 was created intentionally in a lab . (Scientists have determined that the virus almost certainly came about naturally , but some authorities, while saying it’s unlikely, have not ruled out the possibility that a lab played a role in its release.) When asked in March 2020 what they thought was the most likely way the current strain came about based on what they had seen or heard in the news, 40% of Republicans who only got news from outlets with right-leaning audiences said COVID-19 was most likely created intentionally in a lab, far higher than the 28% of Republicans who got political news from outlets with both right-leaning and mixed audiences and 25% of Republicans who get political news only from outlets without right-leaning audiences.
Among Democrats, those who got political news only from outlets with left-leaning audiences stood out less. They were slightly more likely than Democrats whose news diet included outlets with both left-leaning and non-left-leaning audiences to say the virus strain came about naturally (61% and 55%, respectively). Instead, it was Democrats who didn’t get news from any outlets with left-leaning audiences who stood apart. They were more likely to say COVID-19 was most likely created intentionally in a lab (26%), less likely than other Democrats to say it came about naturally (30%) and more likely to express uncertainty over the virus’ origin (34%).
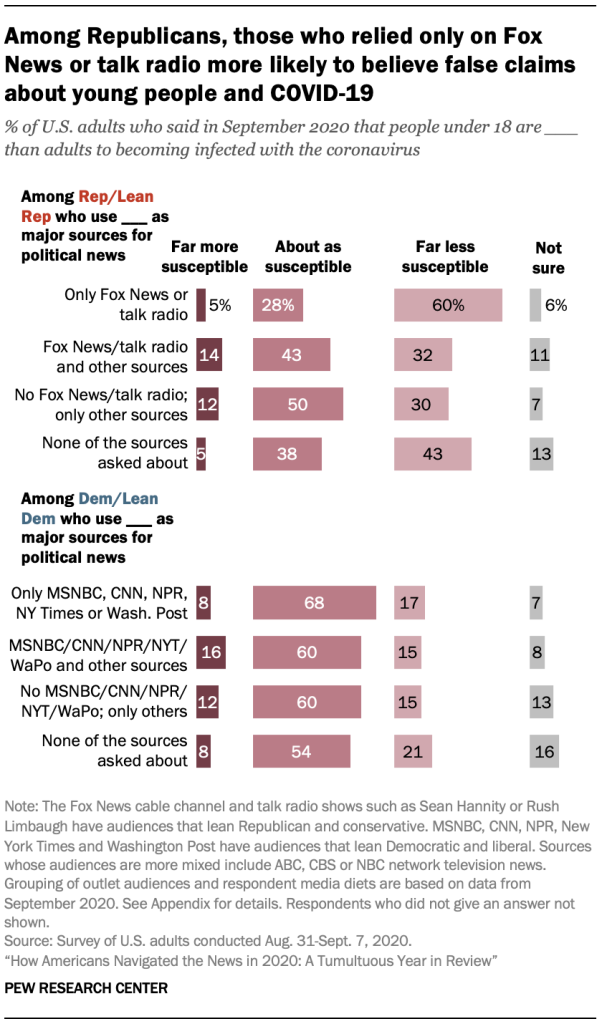
In another area of false claims, Republicans who turned only to outlets with right-leaning audiences (according to whether they used eight sources in September 2020 ) also stood apart. As of September 2020, they were more likely than other Republicans to believe a much-touted (but false) claim that young people are far less susceptible to catching COVID-19 than older adults. (Young people have much lower rates of severe illness and death from COVID-19, but there is no strong evidence that they are less likely to contract the virus.)
Looking at media diet within party, there were only small differences in responses to this question among Democrats who used different major sources for political news. But among Republicans who used only outlets with right-leaning audiences (in this case among eight asked about), a majority (60%) said that minors under 18 are far less susceptible, compared with far fewer among Republicans who used a mixed media diet (32%) or only major sources without conservative-leaning audiences (30%).
- Election 2020
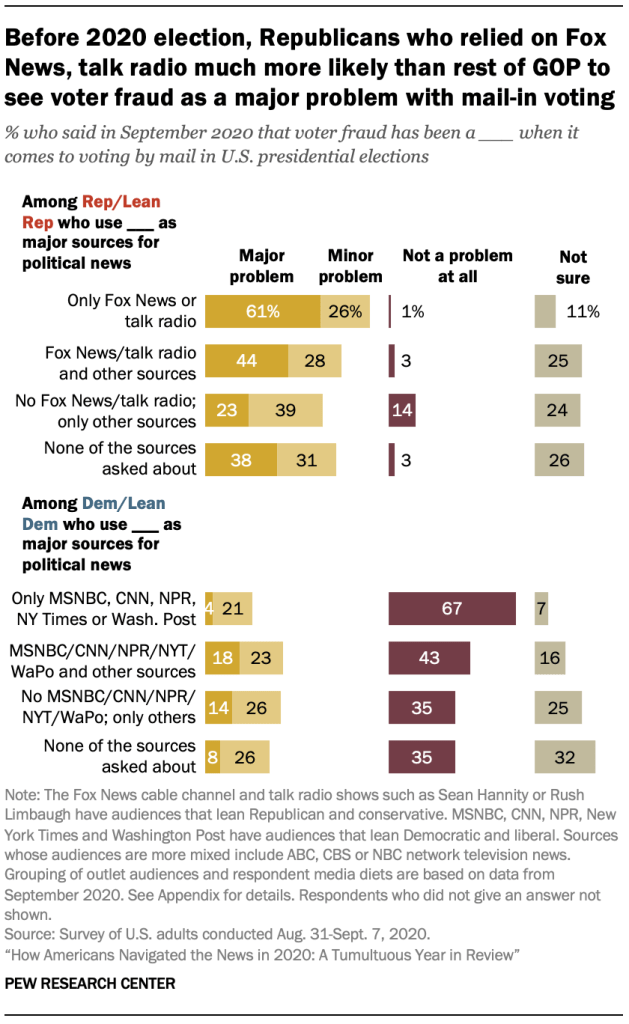
The study also explored the impact of false and unproven claims made prior to Election Day about the potential of voter fraud tied to mail-in ballots (though experts say there is almost no meaningful fraud associated with mail ballots ), and then after the fact, whether voter fraud was getting too much or too little attention.
In September, fully 61% of Republicans who only cited Fox News and/or talk radio shows as key news sources said fraud has been a major problem when mail-in ballots are used. That figure drops to 44% for Republicans who cited other outlets alongside Fox News and/or talk radio as major sources, then down to about a quarter (23%) among Republicans who didn’t rely on Fox News or talk radio (but selected at least one of the six other sources mentioned in the survey).
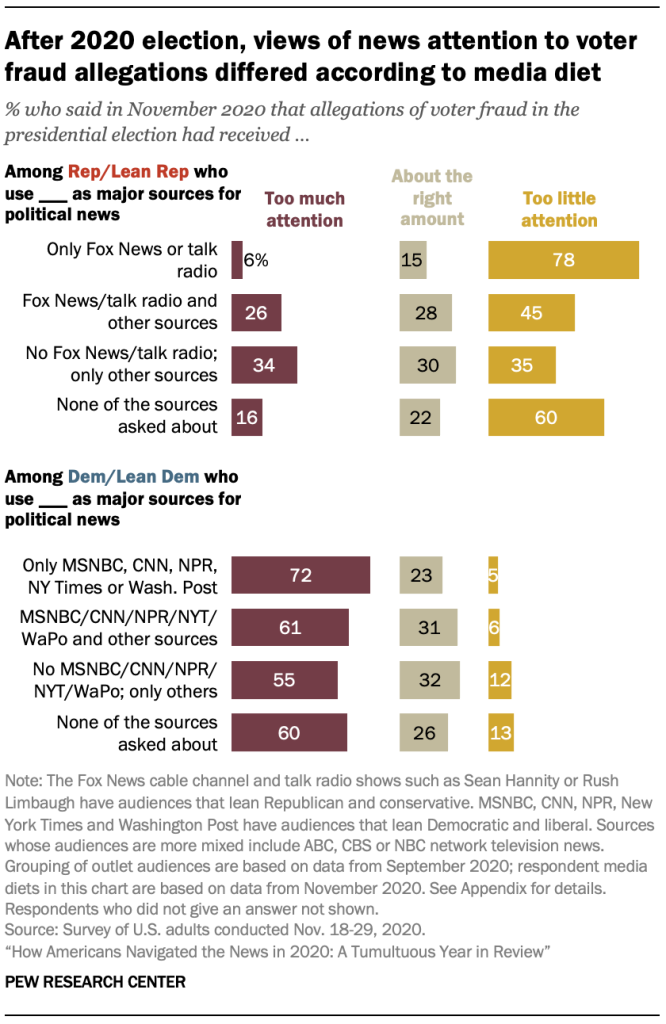
Democrats who cited only outlets with left-leaning audiences as key sources of political news were by far the most likely to say that voter fraud has not been a problem associated with mail-in ballots: 67% said this, compared with 43% of those who relied on some of these sources but also others. Democrats who didn’t rely on any of the outlets with left-leaning audiences (or, in some cases, any of the eight major news sources mentioned in the survey) expressed greater uncertainty on this issue than other Democrats.
Similarly, after the election, Republicans who turned only to outlets with conservative-leaning audiences were much more likely than those who turned to other outlets to say allegations of voter fraud were getting “too little attention.” Just 6% of Republicans who only used Fox News or talk radio as major sources for post-election news said there had been too much attention paid to the fraud allegations, compared with 78% who said there had been too little attention. In the group that used other sources in addition to Fox News and/or talk radio, 26% said there had been too much attention, while 45% said there had been too little. And Republicans who didn’t rely on Fox News or talk radio at all and only relied on other sources for their post-election news were pretty evenly divided between the two responses.
Sign up for our weekly newsletter
Fresh data delivery Saturday mornings
Sign up for The Briefing
Weekly updates on the world of news & information
- American News Pathways 2020 Project
- Media Polarization
- Politics & Media
- Politics Online
Republicans who relied on Trump for news more concerned than other Republicans about election fraud
As cdc warned against holiday travel, 57% of americans say they changed thanksgiving plans due to covid-19, 5 facts about the qanon conspiracy theories, two-thirds of u.s. adults say they’ve seen their own news sources report facts meant to favor one side, americans blame unfair news coverage on media outlets, not the journalists who work for them, most popular, report materials.
901 E St. NW, Suite 300 Washington, DC 20004 USA (+1) 202-419-4300 | Main (+1) 202-857-8562 | Fax (+1) 202-419-4372 | Media Inquiries
Research Topics
- Email Newsletters
ABOUT PEW RESEARCH CENTER Pew Research Center is a nonpartisan fact tank that informs the public about the issues, attitudes and trends shaping the world. It conducts public opinion polling, demographic research, media content analysis and other empirical social science research. Pew Research Center does not take policy positions. It is a subsidiary of The Pew Charitable Trusts .
© 2024 Pew Research Center
- A-Z Publications
Annual Review of Political Science
Volume 23, 2020, review article, open access, political misinformation.
- Jennifer Jerit 1 , and Yangzi Zhao 1
- View Affiliations Hide Affiliations Affiliations: Department of Political Science, Stony Brook University, Stony Brook, New York 11794, USA; email: [email protected] [email protected]
- Vol. 23:77-94 (Volume publication date May 2020) https://doi.org/10.1146/annurev-polisci-050718-032814
- Copyright © 2020 by Annual Reviews. This work is licensed under a Creative Commons Attribution 4.0 International License, which permits unrestricted use, distribution, and reproduction in any medium, provided the original author and source are credited. See credit lines of images or other third party material in this article for license information.
Misinformation occurs when people hold incorrect factual beliefs and do so confidently. The problem, first conceptualized by Kuklinski and colleagues in 2000, plagues political systems and is exceedingly difficult to correct. In this review, we assess the empirical literature on political misinformation in the United States and consider what scholars have learned since the publication of that early study. We conclude that research on this topic has developed unevenly. Over time, scholars have elaborated on the psychological origins of political misinformation, and this work has cumulated in a productive way. By contrast, although there is an extensive body of research on how to correct misinformation, this literature is less coherent in its recommendations. Finally, a nascent line of research asks whether people's reports of their factual beliefs are genuine or are instead a form of partisan cheerleading. Overall, scholarly research on political misinformation illustrates the many challenges inherent in representative democracy.
Article metrics loading...
Full text loading...
Literature Cited
- Ahler DJ , Sood G. 2018 . The parties in our heads: misperceptions about party composition and their consequences. J. Politics 80 : 964– 81 [Google Scholar]
- Althaus SL. 2003 . Collective Preferences in Democratic Politics: Opinion Surveys and the Will of the People Cambridge, UK: Cambridge Univ. Press [Google Scholar]
- Arceneaux K , Vander Wielen RJ 2017 . Taming Intuition: How Reflection Minimizes Partisan Reasoning and Promotes Democratic Accountability Cambridge, UK: Cambridge Univ. Press [Google Scholar]
- Benegal SD , Scruggs LA. 2018 . Correcting misinformation about climate change: the impact of partisanship in an experimental setting. Climatic Change 148 : 61– 80 [Google Scholar]
- Berinsky AJ. 2017 . Rumors and health care reform: experiments in political misinformation. Br. J. Political Sci. 47 : 241– 62 [Google Scholar]
- Berinsky AJ. 2018 . Telling the truth about believing the lies? Evidence for the limited prevalence of expressive survey responding. J. Politics 80 : 211– 24 [Google Scholar]
- Bisgaard M. 2015 . Bias will find a way: economic perceptions, attributions of blame, and partisan-motivated reasoning during crisis. J. Politics 77 : 849– 60 [Google Scholar]
- Bode L , Vraga EK. 2015 . In related news, that was wrong: the correction of misinformation through related stories functionality in social media. J. Commun. 65 : 619– 38 [Google Scholar]
- Bolsen T , Druckman JN , Cook FL 2014 . The influence of partisan motivated reasoning on public opinion. Political Behav 36 : 235– 62 [Google Scholar]
- Brotherton R , French CC , Pickering AD 2013 . Measuring belief in conspiracy theories: the generic conspiracist beliefs scale. Front. Psychol. 4 : 279 [Google Scholar]
- Bullock JG. 2007 . Experiments on partisanship and public opinion: party cues, false beliefs, and Bayesian updating PhD Thesis, Stanford University Stanford, CA: [Google Scholar]
- Bullock JG. 2009 . Partisan bias and the Bayesian ideal in the study of public opinion. J. Politics 71 : 1109– 24 [Google Scholar]
- Bullock JG. 2011 . Elite influence on public opinion in an informed electorate. Am. Political Sci. Rev. 105 : 496– 515 [Google Scholar]
- Bullock JG , Gerber AS , Hill SJ , Huber GA 2015 . Partisan bias in factual beliefs about politics. Q. J. Political Sci. 10 : 519– 78 [Google Scholar]
- Bullock JG , Lenz G. 2019 . Partisan bias in surveys. Annu. Rev. Political Sci. 22 : 325– 42 [Google Scholar]
- Cacioppo JT , Petty RE. 1982 . The need for cognition. J. Personal. Soc. Psychol. 42 : 116– 31 [Google Scholar]
- Chan MS , Jones CR , Hall Jamieson K , Albarracin D 2017 . Debunking: a meta-analysis of the psychological efficacy of messages countering misinformation. Psychol. Sci. 28 : 1531– 46 [Google Scholar]
- Clifford S , Thorson E. 2017 . Encouraging information search reduces factual misperceptions Paper presented at the Annual Meeting of the Midwest Political Science Association Chicago, IL: [Google Scholar]
- Cobb M , Nyhan B , Reifler J 2013 . Beliefs don't always preserve: how political figures are punished when positive information about them is discredited. Political Psychol 34 : 307– 26 [Google Scholar]
- Cook J , Ecker U , Lewandowsky S 2015 . Misinformation and how to correct it. Emerging Trends in the Social and Behavioral Sciences RA Scott, MC Buchmann, SM Kosslyn New York: Wiley https://doi.org/10.1002/9781118900772.etrds0222 [Crossref] [Google Scholar]
- Delli Carpini MX , Keeter S 1993 . Measuring political knowledge: putting first things first. Am. J. Political Sci. 37 : 1179– 206 [Google Scholar]
- Delli Carpini MX , Keeter S 1996 . What Americans Know About Politics and Why It Matters New Haven, CT: Yale Univ. Press [Google Scholar]
- Ditto PH , Lopez DF. 1992 . Motivated skepticism: use of differential decision criteria for preferred and nonpreferred conclusions. J. Personal. Soc. Psychol. 63 : 568– 84 [Google Scholar]
- Druckman JN 2012 . The politics of motivation. Crit. Rev. J. Politics Soc. 24 : 199– 216 [Google Scholar]
- Druckman JN , McGrath MC. 2019 . The evidence for motivated reasoning in climate change preference formation. Nat. Climate Change 9 : 111– 19 [Google Scholar]
- Druckman JN , Peterson E , Slothuus R 2013 . How elite partisan polarization affects public opinion formation. Am. Political Sci. Rev. 107 : 57– 79 [Google Scholar]
- Duran ND , Nicholson SP , Dale R 2017 . The hidden appeal and aversion to political conspiracies as revealed in the response dynamics of partisans. J. Exp. Soc. Psychol. 73 : 268– 78 [Google Scholar]
- Ecker UK , Ang LC. 2019 . Political attitudes and the processing of misinformation corrections. Political Psychol 40 : 241– 60 [Google Scholar]
- Ecker UK , Lewandowsky S , Apai J 2011 . Terrorists brought down the plane!—No, actually it was a technical fault: processing corrections of emotive information. Q. J. Exp. Psychol. 64 : 283– 310 [Google Scholar]
- Ecker UK , Lewandowsky S , Chang EP , Pillai R 2014a . The effects of subtle misinformation in news headlines. J. Exp. Psychol. Appl. 20 : 323– 35 [Google Scholar]
- Ecker UK , Lewandowsky S , Fenton O , Martin K 2014b . Do people keep believing because they want to? Preexisting attitudes and the continued influence of misinformation. Mem. Cogn. 42 : 292– 304 [Google Scholar]
- Ecker UK , Lewandowsky S , Tang DT 2010 . Explicit warnings reduce but do not eliminate the continued influence of misinformation. Mem. Cogn. 38 : 1087– 100 [Google Scholar]
- Feldman S. 1995 . Answering survey questions: the measurement and meaning of public opinion. Political Judgment: Structure and Process M Lodge, KM McGraw 249– 70 Ann Arbor: Univ. Mich. Press [Google Scholar]
- Feldman S , Huddy L , Marcus GE 2015 . Going to War in Iraq: When Citizens and the Press Matter Chicago: Univ. Chicago Press [Google Scholar]
- Flynn D. 2016 . The scope and correlates of political misperceptions in the mass public Paper presented at the Annual Meeting of the American Political Science Association Philadelphia, PA: [Google Scholar]
- Flynn D , Nyhan B , Reifler J 2017 . The nature and origins of misperceptions: understanding false and unsupported beliefs about politics. Adv. Political Psychol 38 : 127– 50 [Google Scholar]
- Gaines BJ , Kuklinski JH , Quirk PJ , Peyton B , Verkuilen J 2007 . Same facts, different interpretations: partisan motivation and opinion on Iraq. J. Politics 69 : 957– 74 [Google Scholar]
- Gal D , Rucker DD. 2010 . When in doubt, shout! Paradoxical influences of doubt on proselytizing. Psychol. Sci. 21 : 1701– 7 [Google Scholar]
- Garrett RK , Nisbet EC , Lynch EK 2013 . Undermining the corrective effects of media-based political fact checking? The role of contextual cues and naïve theory. J. Commun. 63 : 617– 37 [Google Scholar]
- Gershkoff A , Kushner S. 2005 . Shaping public opinion: the 9/11-Iraq connection in the Bush administration's rhetoric. Perspect. Politics 3 : 525– 37 [Google Scholar]
- Gilens M. 2001 . Political ignorance and collective policy preferences. Am. Political Sci. Rev. 95 : 379– 96 [Google Scholar]
- Graham MH. 2018 . Self-awareness of political knowledge. Political Behav https://doi.org/10.1007/s11109-018-9499-8 [Crossref] [Google Scholar]
- Guillory JJ , Geraci L. 2013 . Correcting erroneous inferences in memory: the role of source credibility. J. Appl. Res. Mem. Cogn. 2 : 201– 9 [Google Scholar]
- Haglin K. 2017 . The limitations of the backfire effect. Res. Politics 4 : 3 1– 5 [Google Scholar]
- Hart PS , Nisbet EC. 2012 . Boomerang effects in science communication: how motivated reasoning and identity cues amplify opinion polarization about climate mitigation policies. Commun. Res. 39 : 701– 23 [Google Scholar]
- Hill SJ. 2017 . Learning together slowly: Bayesian learning about political facts. J. Politics 79 : 1403– 18 [Google Scholar]
- Hochschild JL. 2001 . Where you stand depends on what you see: connections among values, perceptions of fact, and political prescriptions. Citizens and Politics: Perspectives from Political Psychology JH Kuklinski 313– 40 Cambridge, UK: Cambridge Univ. Press [Google Scholar]
- Hochschild JL , Einstein KL. 2015 . Do Facts Matter? Information and Misinformation in American Politics Norman: Univ. Okla. Press [Google Scholar]
- Hopkins D , Sides J , Citrin J 2018 . The muted consequences of correct information about immigration. J. Politics 81 : 315– 20 [Google Scholar]
- Jerit J , Barabas J. 2006 . Bankrupt rhetoric: how misleading information affects knowledge about social security. Public Opin. Q. 70 : 278– 303 [Google Scholar]
- Jerit J , Barabas J. 2012 . Partisan perceptual bias and the information environment. J. Politics 74 : 672– 84 [Google Scholar]
- Johnson HM , Seifert CM. 1994 . Sources of the continued influence effect: when misinformation in memory affects later inferences. J. Exp. Psychol. Learn. Mem. Cogn. 20 : 1420– 36 [Google Scholar]
- Jost JT , Glaser J , Kruglanski AW , Sulloway FJ 2003 . Political conservatism as motivated social cognition. Psychol. Bull. 129 : 3 339– 75 [Google Scholar]
- Kahan DM. 2016 . The politically motivated reasoning paradigm, part 1: what politically motivated reasoning is and how to measure it. Emerging Trends in the Social and Behavioral Sciences RA Scott, SM Kosslyn 1– 16 New York: Wiley https://doi.org/10.1002/9781118900772.etrds0417 [Crossref] [Google Scholar]
- Kahan DM , Landrum A , Carpenter K , Helft L , Hall Jamieson K 2017a . Science curiosity and political information processing. Political Psychol 38 : 179– 99 [Google Scholar]
- Kahan DM , Peters E , Dawson EC , Slovic P 2017b . Motivated numeracy and enlightened self-government. Behav. Public Policy 1 : 54– 86 [Google Scholar]
- Khanna K , Sood G. 2018 . Motivated responding in studies of factual learning. Political Behav 40 : 79– 101 [Google Scholar]
- Kuklinski JH , Quirk PJ , Jerit J , Schwieder D , Rich RF 2000 . Misinformation and the currency of democratic citizenship. J. Politics 62 : 790– 816 [Google Scholar]
- Kunda Z. 1990 . The case for motivated reasoning. Psychol. Bull. 108 : 480– 98 [Google Scholar]
- Lavine HG , Johnston CD , Steenbergen MR 2012 . The Ambivalent Partisan: How Critical Loyalty Promotes Democracy Oxford, UK: Oxford Univ. Press [Google Scholar]
- Lee S , Matsuo A. 2018 . Decomposing political knowledge: what is confidence in knowledge and why it matters. Electoral Stud 51 : 1– 13 [Google Scholar]
- Leeper TJ , Slothuus R. 2014 . Political parties, motivated reasoning, and public opinion formation. Political Psychol 35 : 129– 56 [Google Scholar]
- Lewandowsky S , Ecker UK , Cook J 2017 . Beyond misinformation: understanding and coping with the “post-truth” era. J. Appl. Res. Mem. Cogn. 6 : 353– 69 [Google Scholar]
- Lewandowsky S , Ecker UK , Seifert CM , Schwarz N , Cook J 2012 . Misinformation and its correction: continued influence and successful debiasing. Psychol. Sci. Public Interest 13 : 106– 31 [Google Scholar]
- Lewandowsky S , Stritzke WGK , Oberauer K , Morales M 2005 . Memory for fact, fiction, and misinformation: the Iraq War 2003. Psychol. Sci. 16 : 190– 95 [Google Scholar]
- Lodge M , Taber CS. 2013 . The Rationalizing Voter Cambridge, UK: Cambridge Univ. Press [Google Scholar]
- Maio GR , Esses VM. 2001 . The need for affect: individual differences in the motivation to approach or avoid emotions. J. Personal. 69 : 583– 614 [Google Scholar]
- Miller JM , Saunders KL , Farhart CE 2016 . Conspiracy endorsement as motivated reasoning: the moderating roles of political knowledge and trust. Am. J. Political Sci. 60 : 824– 44 [Google Scholar]
- Muirhead R , Rosenblum NL. 2019 . A Lot of People Are Saying: The New Conspiracism and the Assault on Democracy Princeton, NJ: Princeton Univ. Press [Google Scholar]
- Murphy ST , Zajonc RB. 1993 . Affect, cognition, and awareness: affective priming with optimal and suboptimal stimulus exposures. J. Personal. Soc. Psychol. 64 : 723– 39 [Google Scholar]
- Nir L. 2011 . Motivated reasoning and public opinion perception. Public Opin. Q. 75 : 504– 32 [Google Scholar]
- Nisbet EC , Cooper KE , Garrett RK 2015 . The partisan brain: how dissonant science messages lead conservatives and liberals to (dis)trust science. Ann. Am. Acad. Political Soc. Sci. 658 : 36– 66 [Google Scholar]
- Nyhan B. 2010 . Why the “death panel” myth wouldn't die: misinformation in the health care reform debate. Forum 8 : 1 5 [Google Scholar]
- Nyhan B , Porter E , Reifler J , Wood TJ 2019 . Taking fact-checks literally but not seriously? The effects of journalistic fact-checking on factual beliefs and candidate favorability. Political Behav https://doi.org/10.1007/s11109-019-09528-x [Crossref] [Google Scholar]
- Nyhan B , Reifler J. 2010 . When corrections fail: the persistence of political misperceptions. Political Behav 32 : 303– 30 [Google Scholar]
- Nyhan B , Reifler J. 2015a . Displacing misinformation about events: an experimental test of causal corrections. J. Exp. Political Sci. 2 : 81– 93 [Google Scholar]
- Nyhan B , Reifler J. 2015b . Does correcting myths about the flu vaccine work? An experimental evaluation of the effects of corrective information. Vaccine 33 : 459– 64 [Google Scholar]
- Oliver JE , Wood TJ. 2014 . Conspiracy theories and the paranoid style(s) of mass opinion. Am. J. Political Sci. 58 : 952– 66 [Google Scholar]
- Page BI , Shapiro RY. 1992 . The Rational Public: Fifty Years of Trends in Americans’ Policy Preferences Chicago: Univ. Chicago Press [Google Scholar]
- Parker-Stephen E. 2013 . Tides of disagreement: how reality facilitates (and inhibits) partisan public opinion. J. Politics 75 : 1077– 88 [Google Scholar]
- Pasek J , Sood G , Krosnick JA 2015 . Misinformed about the Affordable Care Act? Leveraging certainty to assess the prevalence of misperceptions. J. Commun. 65 : 660– 73 [Google Scholar]
- Pennycook G , Rand DG. 2019 . Cognitive reflection and the 2016 US Presidential election. Personal. Soc. Psychol. Bull. 45 : 224– 39 [Google Scholar]
- Peter C , Koch T. 2016 . When debunking scientific myths fails (and when it does not): the backfire effect in the context of journalistic coverage and immediate judgments as prevention strategy. Sci. Commun. 38 : 3– 25 [Google Scholar]
- Pornpitakpan C. 2004 . The persuasiveness of source credibility: A critical review of five decades’ evidence. J. Appl. Soc. Psychol. 34 : 243– 81 [Google Scholar]
- Prasad M , Perrin AJ , Bezila K , Hoffman SG , Kindleberger K et al. 2009 . “There must be a reason”: Osama, Saddam, and inferred justification. Sociol. Inq. 79 : 142– 62 [Google Scholar]
- Prior M , Sood G , Khanna K 2015 . You cannot be serious: the impact of accuracy incentives on partisan bias in reports of economic perceptions. Q. J. Political Sci. 10 : 489– 518 [Google Scholar]
- Redlawsk DP , Civettini AJ , Emmerson KM 2010 . The affective tipping point: Do motivated reasoners ever “get it”?. Political Psychol 31 : 563– 93 [Google Scholar]
- Rojecki R , Meraz S. 2016 . Rumors and factitious information blends: the role of the web in speculative politics. New Media Soc 18 : 25– 43 [Google Scholar]
- Sangalang A , Ophir Y , Cappella JN 2019 . The potential for narrative correctives to combat misinformation. J. Commun. 69 : 298– 319 [Google Scholar]
- Schaffner BF , Luks S. 2018 . Misinformation or expressive responding? What an inauguration crowd can tell us about the source of political misinformation in surveys. Public Opin. Q. 82 : 135– 47 [Google Scholar]
- Schaffner BF , Roche C. 2017 . Misinformation and motivated reasoning. Public Opin. Q. 81 : 86– 110 [Google Scholar]
- Shapiro RY , Bloch‐Elkon Y. 2008 . Do the facts speak for themselves? Partisan disagreement as a challenge to democratic competence. Crit. Rev. 20 : 115– 39 [Google Scholar]
- Shin J , Jian L , Driscoll K , Bar F 2017 . Political rumoring on Twitter during the 2012 US presidential election: rumor diffusion and correction. New Media Soc 19 : 1214– 35 [Google Scholar]
- Shin J , Jian L , Driscoll K , Bar F 2018 . The diffusion of misinformation on social media: temporal pattern, message, and source. Comput. Hum. Behav. 83 : 278– 87 [Google Scholar]
- Sunstein CR. 2009 . On Rumors: How Falsehoods Spread, Why We Believe Them, and What Can Be Done Princeton, NJ: Princeton Univ. Press [Google Scholar]
- Sunstein CR , Vermeule A. 2009 . Conspiracy theories: causes and cures. J. Political Philos. 17 : 202– 27 [Google Scholar]
- Swire B , Berinsky AJ , Lewandowsky S , Ecker UK 2017 . Processing political misinformation: comprehending the Trump phenomenon. R. Soc. Open Sci. 4 : 160802 [Google Scholar]
- Swire B , Ecker U. 2018 . Misinformation and its correction: cognitive mechanisms and recommendations for mass communication. Misinformation and Mass Audiences BG Southwell, EA Thorson, L Sheble 195– 211 Austin: Univ. Texas Press [Google Scholar]
- Thorson E. 2016 . Belief echoes: the persistent effects of corrected misinformation. Political Commun 33 : 460– 80 [Google Scholar]
- Trevors GJ , Muis KR , Pekrun R , Sinatra GM , Winne PH 2016 . Identity and epistemic emotions during knowledge revision: a potential account for the backfire effect. Discourse Process 53 : 339– 70 [Google Scholar]
- Vraga EK , Bode L. 2017 . Using expert sources to correct health misinformation in social media. Sci. Commun. 39 : 621– 45 [Google Scholar]
- Walter N , Murphy ST. 2018 . How to unring the bell: a meta-analytic approach to correction of misinformation. Commun. Monogr. 85 : 423– 41 [Google Scholar]
- Weeks BE. 2015 . Emotions, partisanship, and misperceptions: how anger and anxiety moderate the effect of partisan bias on susceptibility to political misinformation. J. Commun. 65 : 699– 719 [Google Scholar]
- Weeks BE. 2018 . Media and political misperceptions. Misinformation and Mass Audiences BG Southwell, EA Thorson, L Sheble 140– 56 Austin: Univ. Texas Press [Google Scholar]
- Weeks BE , Garrett RK. 2014 . Electoral consequences of political rumors: motivated reasoning, candidate rumors, and vote choice during the 2008 US presidential election. Int. J. Public Opin. Res. 26 : 401– 22 [Google Scholar]
- Wilkes A , Leatherbarrow M. 1988 . Editing episodic memory following the identification of error. Q. J. Exp. Psychol. 40 : 361– 87 [Google Scholar]
- Wood T , Porter E. 2019 . The elusive backfire effect: mass attitudes’ steadfast factual adherence. Political Behav 41 : 135– 63 [Google Scholar]
- Yair O , Huber GA. 2018 . How robust is evidence of partisan perceptual bias in survey responses? A new approach for studying expressive responding Paper presented at the Annual Meeting of the Midwest Political Science Association Chicago, IL: [Google Scholar]
- Young DG , Jamieson KH , Poulsen S , Goldring A 2018 . Fact-checking effectiveness as a function of format and tone: evaluating FactCheck.org and FlackCheck.org. J. Mass Commun. Q. 95 : 49– 75 [Google Scholar]
- Zaller JR. 1992 . The Nature and Origins of Mass Opinion Cambridge, UK: Cambridge Univ. Press [Google Scholar]
- Article Type: Review Article
Most Read This Month
Most cited most cited rss feed, framing theory, discursive institutionalism: the explanatory power of ideas and discourse, historical institutionalism in comparative politics, the origins and consequences of affective polarization in the united states, political trust and trustworthiness, public attitudes toward immigration, what do we know about democratization after twenty years, what have we learned about the causes of corruption from ten years of cross-national empirical research, economic determinants of electoral outcomes, public deliberation, discursive participation, and citizen engagement: a review of the empirical literature.
Fake Claims of Fake News: Political Misinformation, Warnings, and the Tainted Truth Effect
- Original Paper
- Open access
- Published: 05 February 2020
- Volume 43 , pages 1433–1465, ( 2021 )
Cite this article
You have full access to this open access article
- Melanie Freeze ORCID: orcid.org/0000-0003-3193-5178 1 ,
- Mary Baumgartner 2 ,
- Peter Bruno 3 ,
- Jacob R. Gunderson 4 ,
- Joshua Olin 5 ,
- Morgan Quinn Ross 6 &
- Justine Szafran 7
72k Accesses
38 Citations
98 Altmetric
Explore all metrics
“O, what a tangled web we weave when first we practice to deceive.” Walter Scott, Marmion
Fact-checking and warnings of misinformation are increasingly salient and prevalent components of modern news media and political communications. While many warnings about political misinformation are valid and enable people to reject misleading information, the quality and validity of misinformation warnings can vary widely. Replicating and extending research from the fields of social cognition and forensic psychology, we find evidence that valid retrospective warnings of misleading news can help individuals discard erroneous information, although the corrections are weak. However, when informative news is wrongly labeled as inaccurate, these false warnings reduce the news’ credibility. Invalid misinformation warnings taint the truth, lead individuals to discard authentic information, and impede political memory. As only a few studies on the tainted truth effect exist, our research helps to illuminate the less explored dark side of misinformation warnings. Our findings suggest general warnings of misinformation should be avoided as indiscriminate use can reduce the credibility of valid news sources and lead individuals to discard useful information.
Similar content being viewed by others
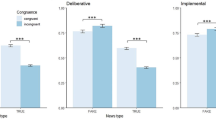
Mindsets and politically motivated reasoning about fake news
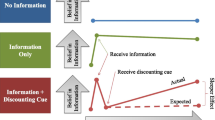
Nevertheless, partisanship persisted: fake news warnings help briefly, but bias returns with time
The Context of Fake News, Disinformation, and Manipulation
Explore related subjects.
- Artificial Intelligence
Avoid common mistakes on your manuscript.
Introduction
Warnings of misinformation are an increasingly common feature of American political communication. The spread of misleading news through social media platforms during the 2016 U.S. election season provoked widespread discussions of and warnings about political misinformation (Allcott and Gentzkow 2017 ; Frankovic 2016 ; Guess et al. 2018a , b ; Nyhan 2019 ; Silverman 2016 ; Silverman et al. 2016 ; Silverman and Singer-Vine 2016 ). In the months prior to the 2016 general election, one in four Americans read a fact-checking article from a national fact-checking website (Guess et al. 2018b , p. 10). Fact-checking organization growth accelerated in the early 2000s, and the number of fact-checking outlets continues to increase in the U.S. and around the world (Graves 2016 ; Graves et al. 2016 ; Spivak 2010 ; Stencel 2019 ). Due to the increased salience of political misinformation and rise of fact-checking organizations, people often encounter warnings regarding misinformation, but the quality and veracity of these warnings can vary considerably. In this article, we evaluate how invalid warnings of misinformation can lead people to distrust the information’s source, cause people to discard accurate information, and ultimately impede memory.
Valid warnings of misinformation tend to originate from professional third-party organizations, target information that is actually misleading, and reduce the spread and acceptance of misinformation. For example, FactCheck.org, PolitiFact, and the Washington Post’s Fact Checker are all organizations that investigate the veracity of claims made by political figures and news organizations, operate year-round, and view themselves as a distinct professional cohort within journalism guided by rules and norms (Graves 2016 ). Warnings originating from these organizations tend to be precise and issued neutrally. Footnote 1 Other institutions, such as Facebook, also devote resources to counteract false news through critical changes to algorithms and various policies. Working to retain users’ trust and confidence in their site, Facebook’s warnings of misinformation often seek to correctly identify and reduce the spread of actual misinformation, although these efforts have recently excluded the direct speech of politicians (Funke 2019 ; Kang 2019 ; Mosseri 2017 ). Footnote 2 Irrespective of the source of a warning, the main criterion of whether or not a warning is valid is if it correctly targets misinformation and efficiently counters the effects of misinformation.
In contrast, less valid or invalid misinformation warnings are biased and inefficient. First, warnings of misinformation are biased when they target factual information rather than misinformation. Bias may be inadvertent but some misinformation warnings are intentionally designed to discredit information. Strategic elites may issue warnings of misinformation against news that is factually correct but unfavorable. Recently, the term “fake news,” has been used by politicians and pundits around the world to discount news reports and organizations they find disagreeable in order to control political news and shape public opinion (Tandoc Jr. et al. 2018 ; Wardle and Derakhshan 2017 ; Wong 2019 ).
Second, warnings of misinformation may be less valid because their effects are inefficient and imprecise. In the U.S., President Donald Trump frequently uses the term “fake news” in tweets referencing the mainstream news media, especially in reaction to critical coverage or investigative reporting (Sugars 2019 ). These and other warnings of misinformation employed by President Trump are often so broadly construed that they could potentially target both misleading and accurate news (Grynbaum 2019a , b ). For example, on March 28, 2019, President Donald Trump wrote “The Fake News Media is going Crazy! They are suffering a major “breakdown,” have ZERO credibility or respect, & must be thinking about going legit. I have learned to live with Fake News, which has never been more corrupt than it is right now. Someday, I will tell you the secret!” Footnote 3
While clumsy warnings may be able to counter misinformation, they are less valid because they often incur high unintended casualties. For example, in contrast to warnings that identify specific misleading facts, Clayton et al. ( 2019 ) find that general warnings of misinformation shown to people before news exposure reduce the perceived accuracy of both real and false news headlines. Mistrust and rejection of news is beneficial when that news is misleading, but when the mistrust and rejection spills over to real news, the potential drawbacks of misinformation warnings become apparent.
Pennycook and Rand ( 2017 ) also uncover other drawbacks of misinformation warnings. An “implied truth effect” emerges when some, but not all, false stories are tagged as misinformation. Those false stories which fail to get tagged are considered validated and seen as more accurate. Even legitimate misinformation warnings, if not fully deployed, can enhance the effects of misinformation in the larger system. Sophisticated organizations seek to employ nuanced and specific fact-checking techniques, but less valid warnings of misinformation continue to be used by both political elites and in broad public conversations on misinformation and the news media. Consequently, it is very important that we continue to investigate both the positive and negative effects of misinformation warnings in the realm of news media and political communications.
In this study, we investigate the potentially negative side effects of invalid, retrospective Footnote 4 misinformation warnings. To do this, we replicate and expand a relatively understudied area of research traditionally applied to the area of eyewitness testimony in the field of social cognition. Specifically, we investigate the tainted truth effect , which proposes that misdirected warnings of post-event misinformation can disadvantage memory of an original event by discrediting factual information and causing it to be discarded at the time of memory assessment (Echterhoff et al. 2007 ; Szpitalak and Polczyk 2011 ).
Drawing on Szpitalak and Polczyk’s ( 2011 ) study on the tainted truth effect, we replicate and extend their three primary research questions to a political context. We first ask, after viewing a political event, how does later exposure to information and misinformation in a news article describing the event alter individuals’ memory and recognition of the details from the original event? Second, when individuals are retrospectively exposed to a valid warning that the news article contained misinformation, are they able to discard the misinformation and remember the correct original event information? Third, do people discard accurate data when exposed to an invalid warning of misinformation? While all three research questions work together to build a picture of individual memory and information processing, the third question regarding the potential drawbacks of misinformation warnings, formally referred to as the tainted truth effect, is the focus of our research. Finally, building on Szpitalak and Polczyk’s three primary questions, we also consider the mechanisms and nuances of misinformation warnings, that is, how these warnings influence the credibility of the warning’s target and the certainty of memory.
From these questions, we derive a series of particular expectations. First, in the absence of a misinformation warning, we expect that individuals’ memories of the original event will be strongly influenced by a post-event description, that is, a related news article. Receiving misleading (or accurate) post-event descriptions in a news article will decrease (or increase) respondents’ ability to recognize original event details.
Hypothesis 1a (Misinformation Effect)
Exposure to misleading information in a post-event description is expected to reduce memory recognition of the original event.
Hypotheses 1b (Information Effect)
Exposure to accurate information in the post-event description is expected to increase the memory recognition of the original event details.
Second, respondents who are exposed to misinformation in the news article but are later warned about misleading information should recognize original event details and misinformation better than respondents who were exposed to misinformation without a warning.
Hypothesis 2a (Warning and the Memory Performance)
Exposure to a valid retrospective misinformation warning will increase the ability to correctly recognize original event details.
Hypothesis 2b (Warning and the Misinformation Recognition)
Exposure to a valid retrospective misinformation warning will reduce the incorrect recognition of misinformation as original event information.
Third, warnings of misinformation are expected to taint all information that is associated with the news article. Therefore, misinformation warnings, even when completely invalid (in the case where no misinformation is in the post-event description), should lead individuals to also reject accurate information that is associated with the news article and result in reduced memory accuracy compared to individuals who are not warned.
Hypothesis 3 (Tainted Truth Effect)
Exposure to an invalid or imprecise retrospective misinformation warning will reduce the ability to correctly recognize original event details.
Finally, we expect trust to be fundamentally damaged by misinformation warnings. First, when warned of misinformation, individuals should be less trusting of their own memory and feel more uncertain about their responses. Second, warnings of misinformation should erode trust in the origins of the information and should lead people to view the news source as less credible.
Hypothesis 4a (Warning and Information Uncertainty)
Exposure to a misinformation warning will increase memory uncertainty.
Hypothesis 4b (Perceived Credibility)
Exposure to a misinformation warning will reduce the perceived credibility of the post-event description that is targeted by the warning.
We find evidence that retrospective, invalid misinformation warnings taint news and lead individuals to view the news as less credible. Increased skepticism produced by invalid misinformation warnings leads individuals to discard information that was in fact accurate, as predicted by the tainted truth hypothesis, and these invalid warnings are also associated with more memory uncertainty. In addition to the tainted truth effect, we find valid warnings help people reject misleading information, but we do not find that individuals are able to fully overcome the effect of misinformation and remember all of the correct information. Our findings generally align with the few studies that have previously examined this topic. However, our use of a diverse subject pool and political context reveals more muted effects and insights into the influence of misinformation warnings on memory, memory uncertainty, and the perceived credibility of news that has been discounted by misinformation warnings.
Post-event Misinformation
Misinformation is broadly defined as “false or misleading information” (Lazer et al. 2018 , p. 1094). Terms such as disinformation, fake or false news, and post-event misinformation refer to specific types of misinformation. Footnote 5 Disinformation is misinformation that is intentionally produced and spread to deceive people (Lazer et al. 2018 ; Wardle and Derakhshan 2017 ). Footnote 6 Often classified as a type of disinformation, fake or false news is fabricated information that assumes the guise of traditional news media but only in form, eschewing the organizational process or intent designed to produce accurate and credible information (Lazer et al. 2018 ; Wardle 2017 , p. 1094). Finally, post-event misinformation is false information in the specific case where individuals have direct experience with an event but are later presented with misleading information about that original event. The post-event misinformation effect occurs when information inconsistent with an event and originating from another source enters an observer’s recollection of that event (Szpitalak and Polczyk 2011 , p. 140). While all types of misinformation are important to understand, our research focuses specifically on post-event misinformation in the context of political news to explore how retrospective warnings moderate post-event misinformation’s effect on memory.
Historically, social cognition researchers have studied the post-event misinformation effect for the purpose of understanding eyewitness testimonies and criminal trials (e.g., Wyler and Oswald 2016 ). However, the post-event approach to misinformation can also be applied to political information and communication. While most of the political information received by the average individual is reprocessed through intermediaries (e.g., acquaintances, political elites, or media and journalistic sources), individuals often have existing knowledge of or experience with many of these reprocessed political events or issues. For example, people may watch a presidential debate and then read or watch commentary that summarizes and expands upon the debate.
Similarly, with the rise of video streaming and sharing on social media platforms, people can experience a political event almost directly and then later encounter the same event reprocessed through a post-event description, such as a news article. Moreover, the pluralistic nature of political communication often results in people seeing multiple presentations of the same event, roughly mirroring the original event and post-event description paradigm. Whether the result of calculation or error, any reprocessing of information increases the likelihood that the information will be biased and misleading, thus opening individuals to the misinformation effect in the realm of political information.
Hundreds of studies over several decades have tackled the topic of the post-event misinformation effect (Ayers and Reder 1998 ; Blank and Launay 2014 ; Loftus 2005 ). In the 1970s, Elizabeth Loftus and colleagues were among the first to explore how eyewitness stories could be distorted by suggestive forensic interview practices (Loftus 1975 ; Loftus et al. 1978 ). Loftus et al. ( 1978 ) discovered that exposing people to misinformation about an event they had previously witnessed altered their ability to recognize details from the original event. This finding, referred to as the misinformation effect, was replicated in many studies under a wide range of conditions (for reviews see Ayers and Reder 1998 ; Chrobak and Zaragoza 2013 ; Loftus 2005 ; Frenda et al. 2011 ). Generally, a three-stage paradigm is used to investigate the misinformation effect. Participants are first shown an original event, then exposed to misleading information, and finally have their memory of the original event assessed, through either recognition or recall memory tests.
Misinformation Warnings and the Tainted Truth Effect
A subset of research on the misinformation effect explores whether the negative effects of misinformation on memory can be reversed, or at least minimized (e.g., Blank and Launay 2014 ; Chambers and Zaragoza 2001 ; Christiaansen and Ochalek 1983 ; Eakin et al. 2003 ; Echterhoff et al. 2005 ; Ecker et al. 2010 ; Wright 1993 ). For example, one of the earliest studies on the effects of misinformation warnings conducted by Dodd and Bradshaw ( 1980 ) found identifying the source of the misinformation as biased dramatically reduced the effect of misleading information on eyewitness memory. In the field of political science, a related body of literature also scrutinizes the causes, implications, and difficulty of countering political misinformation for topics, including the 2010 health care reform (Berinsky 2015 ; Nyhan 2010 ); climate change (van der Linden et al. 2017 ); campaign advertisements and political candidates (Amazeen et al. 2018 ; Cappella and Jamieson 1994 ; Pfau and Louden 1994 ; Thorson 2016 ; Wintersieck et al. 2018 ); political news (Clayton et al. 2019 ); and governmental policies, actions, and politically relevant data (Pennycook et al. 2018 ; Weeks 2015 ). Footnote 7 Under some conditions, warnings of misinformation can help individuals counter the effects of misinformation on attitudes and memory, but the corrections are often only partial, with long-lasting negative effects on trust (Cook and Lewandowsky 2011 ; Huang 2015 ; Lewandowsky et al. 2012 ; Nyhan and Reifler 2012 ). Warnings may even produce a boomerang or backfire effect and lead to misinformation becoming more deeply entrenched in memory when corrections conflict with personal worldview or ideology (Nyhan and Reifler 2010 ). In a meta-analysis of 25 studies on retrospective warnings and post-event misinformation, Blank and Launay ( 2014 ) found retrospective warnings were only somewhat effective, on average reducing the post-event misinformation effect by half.
In addition to imperfectly counteracting misperceptions, misinformation warnings can produce other, often unintended, consequences. Although few in number, some studies outside of political science have investigated how misinformation warnings can extend beyond the intended target of misinformation and negatively influence surrounding information and memories. For example, Greene et al. ( 1982 ) discovered participants who were warned that post-event information came from an untrustworthy source were less likely to recognize events that were correctly described in the post-event description, compared to a no warning condition. Similarly, Meade and Roediger ( 2002 ) found warnings of an unreliable co-witness reduced recall of correct items reported by the co-witness.
Green et al. ( 1982 ) and Meade and Roediger ( 2002 ) noted the negative effects of warnings on memory, but these findings were not the primary focus of their research. Drawing on the research of Greene et al. ( 1982 ) and Meade and Roediger ( 2002 ), Echterhoff et al. ( 2007 ) deliberately began to study misinformation warnings’ potentially adverse influence on correct memories, which they defined as the tainted truth effect . They found that when warned about misinformation, participants were less likely to recognize events that were accurately described in a post-event description, especially when the items were somewhat peripheral or difficult to remember.
In their investigation of the tainted truth effect, Echterhoff et al. ( 2007 ) considered various proposed mechanisms that could drive the misinformation and tainted truth effects. Footnote 8 Echterhoff et al. argued that under certain circumstances, misinformation warnings will reduce the ability to remember original events because warned individuals are more likely to monitor information from a source that has been discredited by a warning. Increased skepticism leads any information that is associated with the untrustworthy source to be tainted and rejected in retrospect, regardless of whether it is true or false. We also propose that retrospective warnings fundamentally alter how people reconstruct memory. In the absence of misinformation warnings, individuals should naturally rely more on post-event descriptions of an event as they are more recent and accessible (Wyler and Oswald 2016 ; Zaller 1992 ). However, when these post-event descriptions become tainted by misinformation warnings, individuals will feel more uncertainty and engage in a memory reconstruction process that discounts and rejects more recent data that comes from the post-event description, including both misinformation and accurate information.
Only a few studies on the tainted truth effect emerged after the initial formal consideration of the phenomenon by Echterhoff et al. ( 2007 ). In a series of related experiments, Szpitalak and Polczyk ( 2010 , 2011 , 2012 ) drew on Polish high school and university student subject pools to replicate and test the misinformation and the tainted truth effects in the contexts of a radio debate on education reform and a historical lecture on Christopher Columbus. Clayton et al. ( 2019 ) also recently identified the need for further research on the tainted truth effect in the area of political misinformation warnings. While the tainted truth effect was not the central hypothesis motivating their research, Clayton et al. ( 2019 ) found general warnings shown to participants before they read a set of headlines reduced the credibility of both truthful and untruthful headlines.
Our experiment contributes to the relatively understudied topic of the tainted truth effect by replicating and extending Szpitalak and Polczyk’s ( 2011 ) study of misinformation, retrospective warnings of misinformation, and memory. Figure 1 illustrates a flow chart of the experimental design employed by Szpitalak and Polczyk ( 2011 ) to investigate the tainted truth effect. In Szpitalak and Polczyk’s study, participants experienced an event (audio lecture on Christopher Columbus’ expedition), read a description of the event following a lapse in time, and were tested on their memory of the original event. Footnote 9 Within this general design, participants were exposed to two main experimental manipulations: the first manipulation varied the content of the post-event description, and the second varied the presence of a retrospective warning of misinformation.
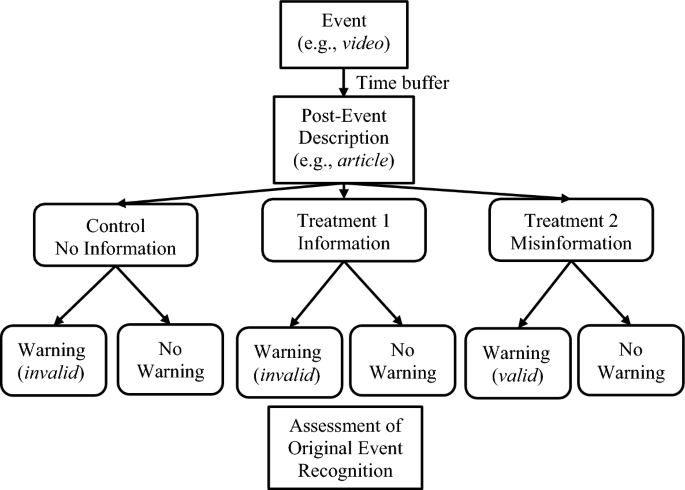
Overview of Szpitalak and Polczyk’s ( 2011 ) experimental approach
While all participants observed the exact same original event, the informational content of the written post-event description differed across three experimental description conditions. In the Control Condition, the post-event description was a vague summary of the original event with no review of the specific facts on which they were later tested. In the Information Condition, the post-event description provided an accurate review of precise facts seen in the original event that were also included in the final memory test. Finally, in the Misinformation Condition, the same set of detailed facts were presented to the participant in the post-event description, but a proportion of these facts were changed so they no longer accurately described the original event. The final manipulation altered whether a warning of misinformation followed the post-event description (Warning Condition and No Warning Condition).
Our study replicates the study design of Szpitalak and Polczyk ( 2011 ) but expands upon their research by examining the misinformation effect and post-warnings in the context of political news and testing the experiment through an online survey experiment with a more diverse subject pool.
Participants
Our online survey experiment was conducted from April 26 to 28, 2017 among adult U.S. participants recruited through Amazon Mechanical Turk (MTurk). Footnote 10 While the MTurk user population is not a representative sample of U.S. citizens, there is ample research suggesting it is a viable setting for survey experiments (Berinsky et al. 2012 ; Casler et al. 2013 ; Coppock 2018 ; Horton et al. 2011 ; Mullinix et al. 2015 ), and it is at least more diverse than the traditional experimental subject pool based on college students (Buhrmester et al. 2011 ).The sample of 434 participants used in our analyses is relatively diverse and comparable to the U.S. population (median age group is 35–54), although the sample we use is significantly more female (65% female) and more educated (52.1% have a bachelor’s degree or greater). Footnote 11
To reduce respondent confirmation bias, the study was presented to participants under a cover story of “Color and Memory” (Podsakoff et al. 2012 ). Participants were told the purpose of the research was to “advance our understanding of the role of color in processing video material.” Following brief instructions, participants were presented with the original event, a four minute CSPAN video recording of three U.S. House Representatives giving short speeches on the repeal of the Affordable Care Act, the UN resolution condemning Jewish settlement of the West Bank, and on the opening of the New York City subway. Footnote 12 These one-minute speeches were selected because they covered a range of political issues (health care, foreign policy, and a regional infrastructure issue) presented by congressional members of both parties. To create a buffer period between the original event and post-event description, participants were asked to answer a set of 22 unrelated questions about their personal political positions and other basic demographic information after they viewed the video.
Participants were then randomly assigned to one of six conditions: a post-event description condition was crossed with exposure to a retrospective misinformation warning condition in a 3 × 2 between-subjects design (three description conditions: Control, Misinformation, Information by two warning conditions: No Warning, Misinformation Warning). Following the buffer period, participants were randomly exposed to one of three possible post-event descriptions (fabricated news articles) that had the same basic format but differed slightly in their content. In the Control Condition, the news article provided only a vague description of the original event/CSPAN video. In the Information Condition, specific facts from the floor speeches were inserted into the news article. In the Misinformation Condition, a subset of the specific facts was altered so the details no longer correctly reflected the original CSPAN video content. Each news article was formatted to look like a real article with a vague but plausible source: Jane Ross, a staff member the Globe . See the online supplementary materials for the entire news article transcript used in the description conditions.
Only a subset, rather than all facts, were manipulated in the Misinformation Condition to ensure the misinformation treatment was subtle and unlikely to lead people to reject the misinformation without any specific warning. After reading the news article, people were randomly assigned to one of two conditions. In the Warning Condition, participants saw a misinformation warning, “warning: some of the information presented in the news article you read was inaccurate.” Footnote 13 Participants in the No Warning Condition did not receive this warning. All survey questions and treatment materials are available in the online supplementary information.
After exposure to the post-event description and warning experimental materials, participants completed a recognition memory test of the 20 facts that were drawn from the CSPAN clip and described in the treatment conditions’ news article. Eleven of the 20 factual questions corresponded to the 11 experimental facts that were altered to be misleading in the Misinformation Condition. The other 9 questions asked about the 9 fixed facts that were held constant in the news articles across all description conditions.
In the memory test, participants were asked to identify which one of four response options corresponded most closely to the information seen in the CSPAN video clip. Each question provided the accurate response option, two inaccurate options, and a “none of the answers are correct” option. Footnote 14 For the 11 experimental fact questions, one of the inaccurate options was the misinformation seen by participants in the Misinformation Condition. Following the memory test, participants were asked to rate the credibility of both the CSPAN video clip (original event) and the news article (post-event description) using an 11-item credibility measure. The full question and response wording and study material details can be found in the supplementary information.
Measures and Design-Specific Expectations
The primary dependent variable examined in this study is memory score : the ability to recognize information seen in the video (original event). Original event recognition memory scores are calculated as the percentage of test questions for which the participant correctly identified the response that corresponded to the original event information. Memory scores for both the 9 fixed fact subset and 11 experimental fact subset were calculated.
In alignment with the expectations of Szpitalak and Polczyk ( 2011 ), we anticipate that exposure to misinformation should lower memory score. Footnote 15 However, when respondents view accurate information in the news article, their ability to recognize the accurate information from the video should increase. Footnote 16 To investigate the effect of the news article on memory, we only consider respondents in conditions without any misinformation warnings. Memory scores of respondents in the treatment conditions are compared to the memory scores of respondents in the Control Condition who only read vague post-event description news article. Formally, these expectations constitute the following two hypotheses as applied to our particular experimental design and measures:
Exposure to misleading information in the post-event description news article is expected to reduce memory recognition of the original event video details: Memory scores (experimental fact subset) are expected to be lower in the Misinformation & No Warning Condition compared to the Control & No Warning Condition.
Hypothesis 1b (Information Effect)
Exposure to accurate information in the post-event description news article is expected to increase the memory recognition of the original event video details: Memory scores (experimental and fixed fact subset) are expected to be higher in the Information & No Warning Condition compared to the Control & No Warning Condition. Memory scores (fixed fact subset) are expected to be higher in the Misinformation & No Warning Condition compared to the Control & No Warning Condition.
Assuming misinformation negatively affects memory score, we also expect warnings of misinformation will improve original event memory as warned individuals try and reject misleading information. First, memory scores should be higher for respondents who were exposed to misleading information in the news article and then later presented with a misinformation warning. Second, these more valid warnings of misinformation should also reduce the selection of the memory test response option that corresponds to the misleading information they were shown. The misinformation score is the percentage of experimental facts questions for which the participant selected the answer that corresponds with the misleading fact shown in the news article rather than the accurate information that was presented in the CSPAN video. If warnings make it easier to discard inaccurate information, respondents receiving valid warnings should have lower misinformation scores than individuals receiving the misinformation condition but no warning.
Exposure to a valid retrospective misinformation warning will increase the ability to correctly recognize original event details: Memory scores (experimental subset) are expected to be higher in the Misinformation & Warning Condition compared to the scores in the Misinformation & No Warning Condition.
Exposure to a valid retrospective misinformation warning will reduce the incorrect recognition of misinformation as original event information: Misinformation scores (experimental subset) are expected to be lower in the Misinformation & Warning Condition compared to the scores in the Misinformation & No Warning Condition.
Because we expect misinformation warnings can contaminate accurate information, warnings should lead to the tainted truth effect even when they are invalid and no misinformation is present in the news article. When individuals are warned of misinformation, we anticipate worse memory scores as accurate information is rejected.
Given the design and fact subset structure of our study, the tainted truth effect hypothesis can be examined from multiple angles. Specifically, the tainted truth effect hypothesis logically leads us to test how misinformation warnings moderate all three components of the Information Effect considered in Hypothesis 1b. In the Information Condition, the post-event description is completely accurate, so the warning is invalid for both the fixed and experimental memory score subsets. In the Misinformation Condition, the warnings, while valid given the presence of misinformation, are still not completely valid due to their general, imprecise wording and potential for spillover. Therefore, the fixed facts (i.e., accurate information) have the potential to be tainted by the misinformation warning and rejected by respondents. A decrease in the memory score for the experimental fact subset (Information Condition) or the fixed fact subset (Information and Misinformation Conditions) will provide evidence that biased and inefficient warnings make it more difficult for respondents to recognize accurate information.
Exposure to an invalid retrospective misinformation warning will reduce the ability to correctly recognize original event details: Memory scores (experimental and fixed subset) are expected to be lower in the Information & Warning Condition compared to the scores in the Information & No Warning Condition. Memory scores (fixed subset) are expected to be lower in the Misinformation & Warning Condition compared to the scores in the Misinformation & No Warning Condition.
While warnings aim to enable people to discard misinformation and correctly recognize original event material, warnings of misinformation may simply lead people to feel more uncertain about their memories. Specifically, people who are exposed to misinformation warnings may gravitate toward the response option, “none of the answers are correct,” as they deal with more recognition confusion. An uncertainty score is calculated as the percent of the experimental and fixed fact subsets for which the participant selected the “none of the answers are correct” option. If warnings caused individuals to feel more confused and uncertain about their memory, we should see larger uncertainty scores in the Warning Conditions relative to the No Warning Conditions for all fact subsets and in all conditions.
Exposure to a misinformation warning will increase memory uncertainty: Uncertainty scores (i.e., frequencies of selecting the “none of the answers are correct” response option) are expected to be higher in the Warning Conditions compared to the No Warning Conditions in all post-event description conditions.
Finally, participants were asked to evaluate how well eleven adjectives described the news article on a five-point Likert scale from “Not very well” to “Extremely well.” A credibility score was calculated as the average of a participant’s response to these eleven items (believable, accurate, trustworthy, biased [reverse coded], reliable, authoritative, honest, valuable, informative, professional, interesting). Even when the news article’s source is not specifically mentioned in the misinformation warning, we expect participants to hold the source responsible for the veracity of the information. Warnings are expected to always reduce the perceived credibility of the news article (Hypothesis 4b). Footnote 17
Exposure to a misinformation warning will reduce the perceived credibility of the post-event description that is targeted by the warning: News article credibility scores are expected to be lower in the Warning Conditions compared to the No Warning Conditions in all post-event description conditions.
The tainted truth and warning effect expectations are critically tied to how misinformation warnings alter perceptions of the source. All information associated with a source connected to misinformation allegations become tainted, leading to the possibility that accurate information will be cast out with the false.
Before formally testing each hypothesis, it is useful to consider the size of the treatment effects through summary statistics broken down by experimental condition for each relevant variable. Figure 2 shows the average memory scores within each description and warning condition for both the fixed and experimental subsets. Footnote 18 In the Control Conditions, the average participant is able to correctly recognize 59% of the original event items for both fixed and experimental question subsets shown in panels a and b. In panel b of Fig. 2 , we see, on average, people who read misleading information in the news article only recognize 46% of the experimental subset’s memory questions. This negative effect of misinformation on memory is also reflected in misinformation scores as shown in Fig. 3 . On average, individuals who were exposed to misinformation but not warned about it incorrectly reported the misleading information as what they had seen in the original event video for 33% of the experimental subset questions (compared to 18% for people in the pure control condition). The valid warning of misinformation does seem to improve memory but only slightly, with the experimental subset mean memory score for people in the Warning & Misinformation Condition increasing to 52% (from 46% in the No Warning & Misinformation Condition) and the misinformation score decreasing to 25% (from 33%).
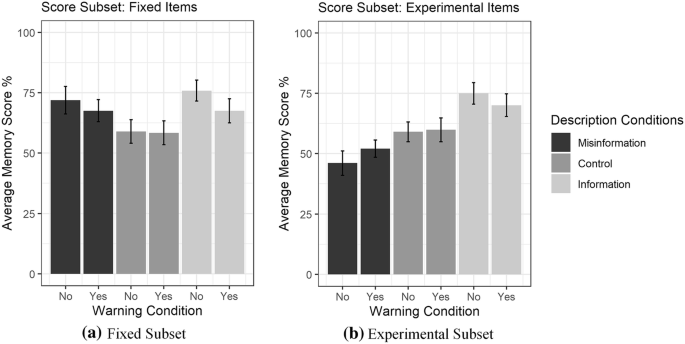
Average memory score by condition
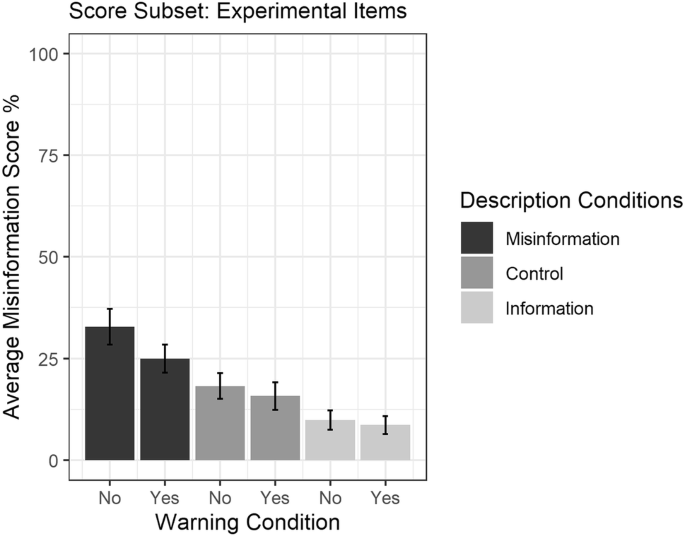
Average misinformation score by condition, experimental subset
Conversely, exposure to accurate information in the news article boosts recognition memory. When people are exposed to accurate information in a news article (e.g., the memory scores for fixed experimental subsets in the Information Condition and fixed subset for the Misinformation Condition), average memory score jumps to around 72–76%. However, in these cases where the information in the news article was correct, subsequent warnings that there was misleading information in the news article suppress the memory scores down to 68–70%. This downward move in memory performance aligns with the expected direction of the tainted truth hypothesis, but the shift is marginal and the informed but warned memory scores are still higher than the 59% accuracy obtained in the Control Condition. At first glance, it looks like warnings of misinformation do not completely eradicate the benefits of accurate post-event information.
To formally test whether information, misinformation, and warnings of misinformation move memory in the expected directions, we interact warning and description condition indicators in three OLS regression models for the fixed and experimental memory score subset dependent variables. The first three models in Table 1 present the estimates used to test our three main hypotheses. In these models, participants in the Control & No Warning Condition serve as the baseline comparison group. Consistent with Hypothesis 1a, misinformation exposure reduces recognition accuracy as seen in the negative effect of misinformation on memory score in Model 1 ( β misinformation = − 12.98, se = 3.14, p < 0.001) and positive effect on misinformation score in Model 3 ( β misinformation = 14.61, se = 2.25, p < 0.001). Hypothesis 1b, the prediction that accurate information in the news article increases original event recognition memory, is also supported by the positive and significant effect of information on memory score in Model 1 ( β information = 15.94, se = 3.11, p < 0.001) and Model 2 ( β information = 16.94, se = 3.44, p < 0.01).
The insignificant interaction terms in Models 1–3 of Table 1 reveal that the effects of warning on memory in the Information and Misinformation Conditions are not significantly different from the null effect found in the Control Condition. Footnote 19 However, while the warning effects in the Information and Misinformation Conditions are not statistically different from the effect established in the Control Condition, warning effects do emerge within the post-event description treatment conditions.
Columns 1–3 of Table 2 present the marginal effects of warning on memory and misinformation scores calculated from the estimates of Table 1 . The marginal effects of warning on memory and misinformation scores are visually displayed in Fig. 4 . The effects of valid warnings on memory performance (Hypothesis 2a) and misinformation endorsement (Hypothesis 2b) are visible in the green/triangular treatment marginal estimates. We see in the right panel of Fig. 4 , compared to participants in the misinformation condition who received no warning, those who were warned that they had been exposed to misleading information in the news article were significantly less likely to select the misleading information ( \({\beta }_{warning}+{\beta }_{misinformation\times warning}\) = − 7.90, se = 2.32, p < 0.01). Footnote 20 However, rejection of misinformation does not fully translate to correct identification of original event information. A complete correction would require a 13 point change to bring the memory score up to the level found in the control conditions (see Table SI-5 in the supplementary information for memory scores across the conditions). Our results find that while warned individuals reject the news article misinformation, they still struggle to remember the correct details they saw earlier in the video. On the left panel of Fig. 4 , we see memory scores for individuals exposed to misleading information and then warned improve only 6 percentage points. This is consistent with Blank and Launay’s ( 2014 ) finding that retrospective warnings usually only reduce the post-event misinformation effect by half. Even though the correction effect is not quite significant at the 0.05 level, it is positive and substantively large, indicating participants seek to counter misinformation when they have been alerted to its presence ( \({\beta }_{warning}+{\beta }_{misinformation\times warning}\) = 6.00, se = 3.23, p = 0.06). Footnote 21 These results suggest that misinformation may have a more persistent influence on memory as correction attempts often fall short.
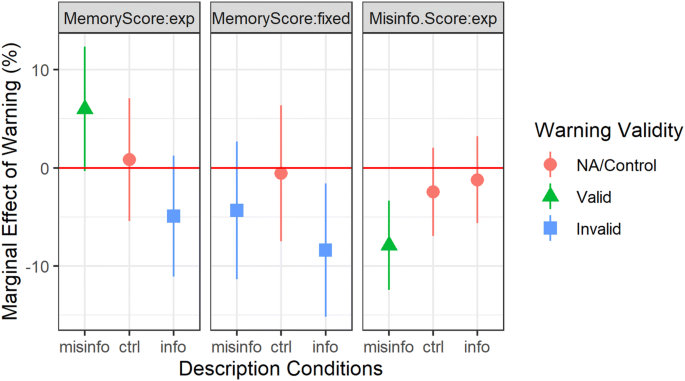
Marginal effect of warning on memory by description condition
The effects of invalid warnings and tests of the tainted truth effect (Hypothesis 3) are presented in the left and middle panels of Fig. 4 by the blue/square symbols that plot the marginal effects of warning on memory score. Of the three possible tests of the tainted truth effect, a significant finding only emerges for the fixed fact subset for individuals in the Information Condition (middle panel; ( \({\beta }_{warning}+{\beta }_{misinformation\times warning}\) = − 8.39, se = 3.46, p = 0.02). For these same individuals in the Information Condition, warnings still suppress memory score for the experimental fact subset, but the effect size is not large enough to reach statistical significance ( \({\beta }_{warning}+{\beta }_{misinformation\times warning}\) = − 4.91, se = 3.13, p = 0.12). Footnote 22 Similarly, participants in the Misinformation Condition are more likely to reject the valid information (fixed fact subset) when they are warned of misinformation, but the size of rejection is too small to be significantly different from those people in the No Warning & Misinformation Condition ( \({\beta }_{warning}+{\beta }_{misinformation\times warning}\) = − 4.35, se = 3.57, p = 0.22). Footnote 23
The final aspect of memory responses that our experimental design and data allows us to examine is uncertainty. Figure 5 presents the average uncertainty scores over the six conditions. Contrary to our expectations in Hypothesis 4a, warnings of misinformation do not appear to consistently alter uncertainty. Uncertainty increases slightly in all warning conditions, but warnings only significantly alter uncertainty in the Information Condition. When the information in the news article is completely correct as is found in the Information Condition, participants appear more confident in their memory compared to those who are informed but then exposed to an invalid warning. Informed and not warned individuals select the “none of the answers are correct” option for only around 7% of the questions, but when warned this number rises to 15%.
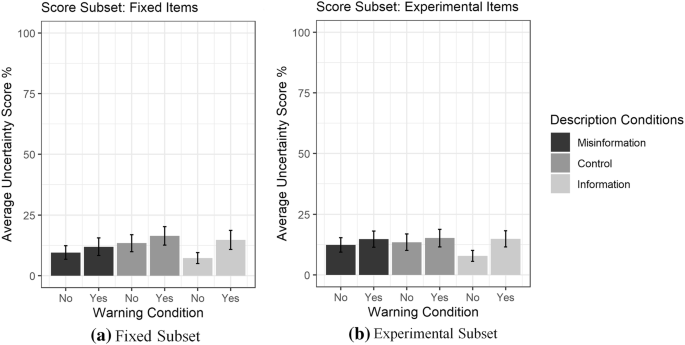
Average memory uncertainty score by condition
Models 4 and 5 in Table 1 estimate the effect of information, misinformation, and warnings on uncertainty scores. The corresponding marginal effects of warnings are presented in columns 4 and 5 in Table 2 and graphically displayed Fig. 6 . In both models 4 and 5 of Table 1 , while the level of uncertainty in the No Warning & Information Condition is significantly different from the uncertainty in the No Warning & Control Condition for both experimental (β information = − 5.64, se = 2, 23, p = 0.012) and fixed (β information = − 6.11, se = 2.36, p = 0.01) fact subsets, the upward shift in uncertainty produced by warnings is not significantly different from that found in the Control Condition (exp: β information × warning = 5.35, se = 3.20, p = 0.094; fixed: β information × warning = 4.50, se = 3.39, p = 0.185). Footnote 24 However, as shown by the marginal effect estimates in Fig. 6 , within the Information Condition, warnings significantly move the uncertainty score around 7 percentage points. In the absence of warning, individuals presented with an accurate news article in the Information Condition were more likely to be certain about their memory compared to those in the Control or Misinformation Conditions. But once exposed to a misinformation warning, individuals doubt their memory and response uncertainty jumps to average levels seen in the other condition.
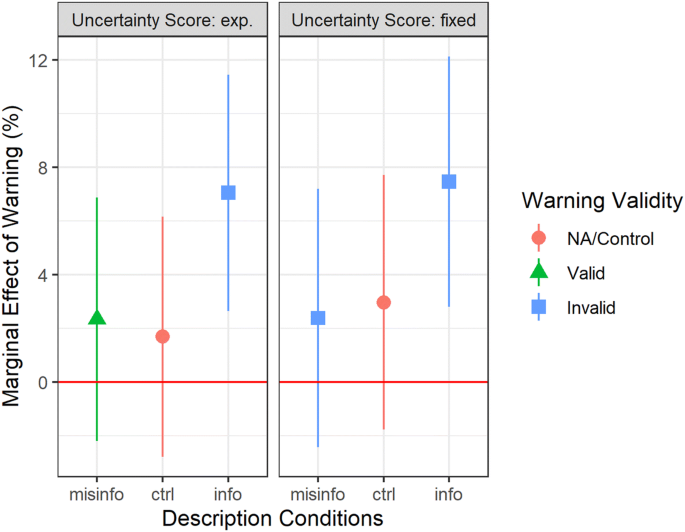
Marginal effect of warning for memory uncertainty by description condition
Having considered all possible aspects of memory as influenced by information, misinformation, and misinformation warnings, we now turn to the primary mechanism proposed by Echterhoff et al. ( 2007 ): source monitoring. The rejection of misinformation, rejection of accurate information, and increase in memory uncertainty occur as general warnings taint all information, good and bad, that people associate with the allegedly misleading source. And, as seen in panel b in Fig. 7 , average levels of the news article’s credibility clearly decrease under warning conditions for all information conditions. For example, in the condition where the news article should have the most credibility (No Warning & Information Condition), we see the article has the same average credibility score as the CSPAN video displayed in panel b. Furthermore, as expected since the video content was held constant across all conditions, the credibility of the video does not significantly change across the conditions (See Model 6 of Table 1 and marginal effects in column 6 of Table 2 ).
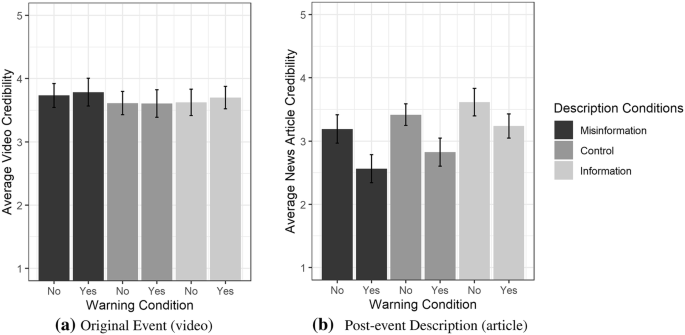
Average credibility of original event (video) and post-event description (article) by condition
In contrast to their perceptions of the video, respondents’ perceptions of the news article credibility do significantly respond to the experimental treatments. Looking at the estimated effect of warning in Model 7 of Table 1 , we see that a misinformation warning leads individuals in the Control Condition to view the news article as 0.59 points less credible (out of five points). The insignificant warning and description conditions interactions reveals that the significant negative effect of the misinformation warning on article credibility found in the Control Condition also occurs in the Information and Misinformation Conditions.
It is important to note that while warnings did reduce the credibility of the news article in all conditions, the news article credibility is not completely identical across all the baseline (No Warning) description conditions. The accurate news article in the No Warning & Information Condition is significantly more credible than the misleading article in the No Warning & Misinformation Condition ( \({\beta }_{information}-{\beta }_{misinformation}\) = 0.43, se = 0.15, p = 0.004). Participants were probably somewhat aware of the misinformation even without being exposed to a retrospective warning. While our design sought to keep the misleading information subtle by changing only a subset (the experimental facts) to be false, the lower levels of credibility in the unwarned Misinformation Conditions suggests the manipulation might have not been subtle enough. Even though overall credibility is significantly higher in the Information Condition compared to the Misinformation Condition, within each description condition the warnings still produced significant drops, as seen in the statistically significant marginal effects of warning presented in column 7 of Table 2 and in the right panel of Fig. 8 . Footnote 25
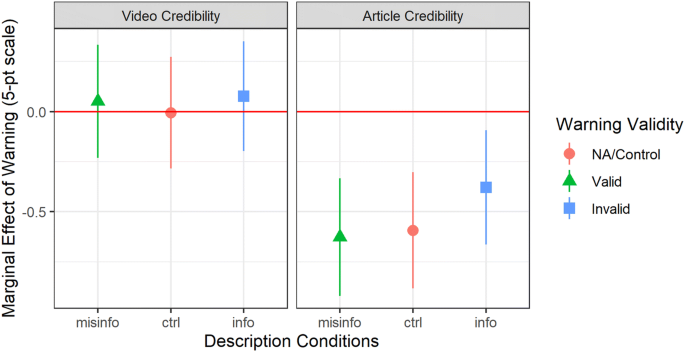
Marginal effects of warning on source credibility by description conditions
Our research replicates the relatively unexplored tainted truth effect and provides useful insights into how efforts to prevent misinformation can have unintended and negative consequences for memory. We find that invalid misinformation warnings can damage source credibility and cause people to reject accurate information that is associated with the tainted source. Warnings of misinformation can also cause people to feel more uncertain about their memory, especially when they were in fact not exposed to any information and the warnings are completely invalid. While valid warnings of misinformation enable people to reject false information, misdirected and imprecise warnings may counter the positive influence of misinformation warnings on memory.
In addition to extending the tainted truth effect to the domain of political communication, our research also provides an interesting launching point for exploring the complexity of invalid warnings of political misinformation. In a February 19, 2019 Twitter post, President Donald Trump alleged the existence of invalid misinformation warnings by saying: “The Washington Post is a Fact Checker only for Democrats. For Republicans, and for your all time favorite President, it is a Fake Fact Checker!” Although this reasoning may feel as though we are being pulled down the rabbit hole with Alice, it does broach several interesting questions: can misinformation warnings be countered and how does political and ideological congruence with the sources moderate these attempts? A recent public opinion study identified Republicans as more likely than Democrats to say fact-checking efforts by news organizations favor one side (Walker and Gottfried 2019 ). If the source of a misinformation warning is perceived as less credible, does it alter the effect of warnings and potential tainted truth effects? Our study begins to address the varied potential effects of misinformation warnings and we suggest this is a topic of inquiry ripe for exploration.
One clear practical implication for political psychology that stems from our tainted truth effect research is the recognition that misinformation warnings may have a dark side as they can lead people to feel more uncertain about, less trusting of, and more likely to reject accurate information. If invalid misinformation warnings have the potential to impede political knowledge, we need to more clearly identify what constitutes an invalid warning and when spillover effects are likely to occur. The quality of misinformation warnings needs to become a part of the dialogue surrounding investigation of misinformation in the realm of politics. Just as there has been an explosion of fact-checking organizations in the past decade as misinformation has become more salient, there may be a demand for comparable efforts that enhance the integrity of valid fact-checkers. In line with this is the need for further research on trust of fact-checking organizations and other sources of misinformation warnings to better understand when misinformation may be more or less effective.
Several design choices made in our study could also be revisited in subsequent research. First, the original event and post-event description form and content may influence how information is processed and whether the tainted truth effect is amplified or minimized. In the study conducted by Szpitalak and Polczyk ( 2011 ), participants experienced the original event information in audio form while the post-event description was read. Our study presented a video original event and written post-event description. In the field of social cognition, misinformation has been introduced through various forms including direct personal interaction, written, and, sometimes, audio. When misinformation encounters are classified as direct (e.g., face-to-face, co-witness, social) versus indirect (e.g., written reports, non-social), Blank et al. ( 2013 ) found no clear difference in misinformation retention in the area of eyewitness reports. However, these findings may not hold in the area of warning effects and political communication. For example, warnings of misinformation may be less likely to taint good information if the post-event description comes in the form of a written news article compared to a radio or television news program if information is encoded more strongly through reading versus listening or watching.
Second, careful considerations of the type of (mis)information accepted or rejected in the face of retrospective warnings should be addressed in future studies of the tainted truth effect. While our design sought to incorporate a wide range of political topics including health care, foreign policy, and distributive politics, the facts used in the memory test were mostly novel and moderately peripheral. We chose to test recognition memory of these details for several reasons. First, details were relatively obscure (e.g., how many jobs a new subway generated), thus minimizing the likelihood participants would have prior exposure and heterogeneous ability to remember them (Pennycook et al. 2018 ). Second, we chose to examine memory of moderately peripheral information to minimize the likelihood of participants independently identifying our misinformation manipulations in the post-event description. However, the lower source credibility in the No Warning Misinformation Condition compared to the No Warning Information Condition leads us to doubt whether our details and misinformation manipulations were peripheral enough.
Furthermore, how easy or difficult it to remember information can alter the power of a warning. For very difficult, or peripheral, misinformation details where no memory exits, individuals engage in “best-guess” strategies in recognition memory tests and warnings make no difference (Wyler and Oswald 2016 ). On the other hand, when the information is very easy to remember, retrospective warnings may also have little influence as individuals are able to identify and correct for the misinformation at the time of exposure (Putnam et al. 2017 ). The misinformation effect and impact of subsequent warnings tend to be largest for moderately peripheral information. For details that are somewhat difficult to remember, misinformation is often undetected and recognition tasks are more prone to recency or familiarity bias which warnings can later mitigate (Wyler and Oswald 2016 ).
Our findings may have been muted because we chose to examine information that was too memorable or too peripheral. Table SI-4 in the online supplementary information suggests most items were only moderately difficult and the difficulty for the experimental and fixed subsets similar, but further research could specifically examine the effect of information difficulty and the tainted truth effect. Future extensions of our research could also examine how ideological congruence with either the original event information, source of the post-event description, or source of the retrospective warning alters the tainted truth effect. While the warnings in our study came from the researcher (warning source was not clearly specified), it would be interesting to see if motivated reasoning alters the tainted truth effect if Trump or some other source presents the misinformation warnings.
While we were able to test this effect on a sample that was reasonably diverse, our results may be limited by the substantial number of participants we had to exclude due to insufficient exposure to our experimental manipulations. Future research using a nationally representative sample and an experimental design that reduces attrition may reveal different effect sizes. Finally, our design examined recognition memory within a relatively short experiment. Participants took 16 min on average to complete the study. While the inclusion of a set of unrelated questions after the original event provided some buffer period between the original event and post-event description, a design that considers the tainted truth effect over longer time intervals between the original event, post-event description, warning, and memory test could shed greater understanding of the cognitive processes underlying the tainted truth effect. Altering the format of the memory test could also help provide more understanding of foundational mechanisms. For example, changing the memory test to forced choice and adding an additional question that measures memory uncertainty could identify the whether or not correction attempts are produced by confusion or enlightenment.
One of the basic assumptions of a well-functioning democracy is the presence of an educated and well-informed citizenry (Lewandowsky et al. 2012 ). At face value, misinformation threatens democratic proceedings if it can influence and shape public opinion and social decisions. Consequently, numerous studies and efforts have emerged to identify and counteract the effects of misinformation in journalistic settings and broader areas of political communication. Our research takes a step back from this fundamental problem to consider whether the efforts to combat misinformation in themselves may have negative side effects.
Our research replicates the tainted truth effect and extends it to the area of political news. Our findings cast much needed light on this phenomenon that has gathered only a little attention in the field of social cognition and even less in the area of political news and communication. Drawing on a relatively diverse sample, we reproduce the general results of prior studies of misinformation and warnings. We find clear evidence that post-event descriptions of prior events shape memory. When original events are twisted by misinformation in a subsequent news article, people are more likely to recognize the false information as the original event data and less likely to identify the correct facts. Conversely, exposure to a news article that provides an accurate retelling of an event experienced earlier boosts individuals’ abilities to correctly remember original event items. When these news articles are then followed by statements warning individuals that the news articles contained some misleading information, we find several interesting developments in recognition memory. Although people try to correct for the misinformation, these efforts are often inadequate. Valid warnings lead people to try and discard the false data seen in the news article, but they still struggle to correctly remember the original event details.
Warnings of misinformation potentially hold other negative consequences for an informed citizenry. When the allegations of misinformation in the news article are invalid, people reject the accurate information, leading to the tainted truth effect. False warnings of misinformation reduce the credibility of legitimate news, decrease acceptance of useful news data, increase memory uncertainty, and impede original event memory. However, these negative effects of misinformation warnings on memory are constrained as the decrease is substantively small. We find the tainted truth effect does not completely erode the positive benefits of factual news on memory.
Our research finds that both valid and invalid retrospective warnings reduce news credibility and alter how news information is processed. Given the potential for misinformation warnings to impede the credibility and acceptance of real news, more attention and research on the tainted truth effect and other unforeseen negative consequences of general warnings of misinformation is needed. We join (Clayton et al. 2019 ) and others’ recommendations that fact-checkers, news media, and political elites tread carefully when deploying general allegations and warnings of fake news and misinformation. While misinformation warnings are critical in combatting the negative effects of misinformation, it is important to be cognizant of the many possible spillover effects from general warnings which may unintentionally damage real news institutions that support critical democratic processes.
Data Availability
Replication materials can be found on Harvard Dataverse at: https://doi.org/10.7910/DVN/TRR0DK
For examples of high quality misinformation warnings see Cook and Lewandowsky ( 2011 ) and Nyhan and Reifler ( 2012 ).
It is arguable that the validity of Facebook and other organizations’ fact-checking efforts also vary, especially in early stages of development. In the immediate months following the 2016 election, Facebook collaborated with fact-checking organizations, flagged false news as “Disputed,” and warned people of the status before they attempted to share the article. These “Disputed” tags were later replaced by a policy in which people viewing popular links were instead shown a series of “Related Articles” that included both misinformation and third-party fact-checker articles (Allcott et al. 2019 , Appendix 4). Since 2016, Facebook’s general strategy has been to “remove, reduce, and inform” (Lyons 2018a ), and the organization continues to update and revise their approach to misinformation, drawing on machine learning tools and expanding fact-check efforts to photos and videos (Lyons 2018b ). However, Facebook has recently taken a more hands-off approach to claims or statements made by politicians on their Facebook Page, an ad, or their website. These statements are considered direct speech and ineligible for third-party fact checking program (Kang 2019 ).
Accessed June 8, 2019 at https://twitter.com/realdonaldtrump/status/1111209625825640448 .
Retrospective warnings are warnings presented to an individual after misinformation exposure [see Blank and Launay ( 2014 ) for a review]. Echterhoff et al. ( 2007 ) recommend research on retrospective warnings of misinformation. These scholars argue retrospective warnings are more likely to mirror real life situations given the difficulty in identifying misinformation and warning people prior to exposure.
With the politicization of the term “fake news,” some scholars and organizations prefer to use the term “false news” (Lazer et al. 2018 ; Wardle and Derakhshan 2017 ; Tandoc Jr. et al. 2018 ). We use the terms interchangeably in this article.
Tucker et al. ( 2018 ) define disinformation as encompassing an even wider range of information types found online including “fake news,” rumors, factual information that is purposely misleading, inadvertently incorrect information, politically slanted information, and “hyperpartisan” news. We prefer a more precise terminology that separates purposive from inadvertent deception.
See Flynn et al. ( 2017 ); Tucker et al. ( 2018 ) for a more comprehensive review of political misinformation research.
See Loftus ( 1975 ) for the initial memory impairment theory that theorized original event detail memory as being overwritten by misinformation. Other proposed mechanisms developed as subsequent research found the misinformation effect could be reduced through non-informative warnings (e.g., Blank and Launay 2014 ; Belli and Loftus 1996 ; Hell et al. 1988 , Loftus 1991 ; Mazzoni and Vannucci 2007 ; McCloskey and Zaragoza 1985 ; Zaragoza et al. 2006 ). More recent proposed mechanisms model memory as a reconstruction process. When memory is assessed, a variety of construction strategies may be used, many of which are subject to different cognitive biases (Mazzoni and Vannucci 2007 ; Wyler and Oswald 2016 ).
Although the results are applied to forensic science, the actual content examined was historical in nature.
In order to be eligible for the study, MTurk workers had to use a U.S. IP address, be over the age of 18, have a 95% or high approval rating for previous MTurk projects (HITs), and completed at least 50 other projects via Mturk. On April 26, 2017, eighty-three individuals participated in our study for a compensation of $.30 per subject. Realizing we underestimated the study’s completion time, the compensation was raised to $.50 per subject the next two days while the study was open. Our substantive results remain when participation date/compensation amount is included as a control variable in the respective models.
One difficulty of conducting experiments through online surveys is ensuring that participants actually receive the experimental treatments. Anticipating a degree of technical problems and insufficient exposure to experimental materials, our study measured both the technical experience of participants and time they spent on critical materials. A total of 549 participants entered the study, but 115 participants were dropped due to non-response or insufficient exposure to the main experimental treatments. Three participants entered the study but then exited immediately after reading the initial instructions, sixty-nine participants had technical problems viewing or did not view the entire video containing the original event materials, and forty-three people spent only ten seconds or less reading the post-event description. While these individuals cannot be included in the analysis, their failure to participate could introduce selection bias if they would have responded differently to the information and warning manipulations. The excluded participants did differ significantly from those who remained in the sample. Excluded participants were more likely to be younger males who had graduated from college but who had a lower need for cognition, read the news fewer days in a week, and knew less about politics. A more detailed analysis of the excluded participants and comparisons between age, education, and gender characteristics for the sample and census populations can be found in the supplementary information Tables SI-1–SI-3.
The three January 4, 2017 one-minute U.S. House floor speeches for Representatives Bustos (D-Illinois, 17th District), Poe (R-Texas 2nd District) and Maloney (D-New York, 12th District) can be viewed here: https://www.c-span.org/video/standalone/?c4666248 . For the full transcripts and more information on the original event materials, see the online supplementary information.
The word “warning” was presented in red font to help draw the readers’ attention.
The presentation order of questions and response options was randomized.
This expectation applies only to the experimental subset memory scores which correspond to the subset of details that were manipulated to be misleading in the Misinformation Condition.
In the Information Condition, all news article details were accurate and the corresponding memory scores measured for both the fixed and experimental subset reflect exposure to accurate information. For respondents in the Misinformation Condition, the fixed fact subset news article details also correctly reflected the original video details. Therefore, the expectation that accurate information will improve memory score can also be considered for the fixed subset memory scores of individuals in the Misinformation Condition.
The credibility of the original event CSPAN video was also measured and the index calculated. We do not expect the original event credibility to be significantly different over the description and warning conditions.
Complete descriptive statistic for all measures are available in the online supplementary information.
Because there is no information to discard in the Control Condition where the news article offered only a vague description of the video, we did not expect the misinformation warning to alter memory scores in the Control Condition. However, it is possible that warning could heighten attention and thus improve the quality of memory reconstruction. This possibility that warnings affect memory through increased attentiveness does not hold in the data. Being warned about misinformation does not significantly alter memory performance (as seen in the insignificant βwarning in Models 1 and 2) or misinformation memory (Model 3) for people in the Control Condition.
While the insignificant interaction term in Model 3 of Table 1 ( \({\beta }_{misinformation\times warning}\) = -5.43, se = 3.26, p = 0.10) suggests this negative effect of warning on misinformation endorsement is not significantly different from the null effect of warning found in the Control Condition, the marginal effect of warning in the Misinformation Condition is significantly different from the null effect of warning found in the Information Condition ( \({\beta }_{information\times warning}{- \beta }_{misinformation\times warning}\) = 6.68, se = 3.23, p = 0.04).
Just as we should be cautious about over-interpreting 0.04 < p < 0.05, we should not over-interpret a p = 0.06 in light of the substantively large findings. Additionally, the insignificant interaction term in Model 1 of Table 1 ( \({\beta }_{misinformation\times warning}\) = -5.16, se = 4.54, p = 0.26) suggests this positive effect of warning on memory is not significantly different from the null effect of warning found in the Control Condition, but the positive marginal effect of warning in the Misinformation Condition is significantly different from the negative effect of warning found in the Information Condition ( \({\beta }_{information\times warning}{- \beta }_{misinformation\times warning}\) = -10.91, se = 4.50, p = 0.01). The treatment conditions’ marginal effects of warning on memory as displayed in the left panel of Fig. 4 are not different from the Control, but they are different from each other.
The insignificant interaction terms in Model 2 of Table 1 ( \({\beta }_{misinformation\times warning}\) = − 3.77, se = 5.01, p = 0.45) and ( \({\beta }_{information\times warning}\) = − 7.83, se = 4.94, p = 0.11) suggest this negative effect of warning on memory (fixed subset) is not significantly different from the null effect of warning found in the Control Condition. If the warnings lead to accurate information being rejected, we would expect to see a negative effect of warning on fixed subset memory in both of the Description treatment conditions. As expected, the negative effect of warning is not significantly different between the Misinformation and Information Conditions ( \({\beta }_{information\times warning}{- \beta }_{misinformation\times warning}\) = − 4.05, se = 4.97, p = 0.42).
While these multiple tests allow us to consider the tainted truth effect in different aspects of the design, as noted by Gelman and Stern ( 2006 ), these tests do not identify whether the differences between the tests are significant. Even though only one test reached statistical significance, their collective alignment in direction and substance builds a stronger case for the tainted truth effect. Also, the tainted truth effect remains statistically significant in a comparison of warned and not warned respondents in the Information Condition when fixed and experimental subsets are combined to create an overall memory score.
The positive marginal effect of warning on uncertainty in the Information Condition is also not significantly different from the marginal effect in the Misinformation Condition; exp: β information × warning − β imisnformation × warning = 4.71, se = 3.22, p = 0.14; fixed: β information × warning − β imisnformation × warning = 5.09, se = 3.41, p = 0.14.
The negative marginal effect of warning on news credibility does not differ significantly between any of the Description Conditions as is seen in the insignificant interaction terms in Model 7 of Table 1 and the insignificant linear combination test that compares the interaction terms: β informationXwarning —β imisnformationXwarning = 0.25, se = 0.21, p = 0.24,
Allcott, H., & Gentzkow, M. (2017). Social media and fake news in the 2016 election. Journal of Economic Perspectives, 31 (2), 211–236.
Article Google Scholar
Allcott, H., Gentzkow, M., Yu, C. (2019). Trends in the diffusion of misinformation on social media. Technical report . National Bureau of Economic Research. Retrieved April 23, 2019, from https://www.nber.org/papers/w25500.pdf .
Amazeen, M. A., Thorson, E., Muddiman, A., & Graves, L. (2018). Correcting political and consumer misperceptions: The effectiveness and effects of rating scale versus contextual correction formats. Journalism & Mass Communication Quarterly, 95 (1), 28–48.
Ayers, M. S., & Reder, & L. M. (1998). A theoretical review of the misinformation effect: Predictions from an activation-based memory model. Psychonomic Bulletin & Review, 5 (1), 1–21.
Belli, R. F., & Loftus, E. F. (1996). The pliability of autobiographical memory: Misinformation and the false memory problem. In D. C. Rubin (Ed.), Remembering our past: Studies in autobiographical memory (pp. 157–179). New York: Cambridge University Press.
Chapter Google Scholar
Berinsky, A. J. (2015). Rumors and health care reform: Experiments in political misinformation. British Journal of Political Science, 47 (2), 241–262.
Berinsky, A. J., Huber, G. A., & Lenz, G. S. (2012). Evaluating online labor markets for experimental research: Amazon.com’s Mechanical Turk. Political Analysis, 20 , 351–368.
Blank, H., & Launay, C. (2014). How to protect eyewitness memory against the misinformation effect: A meta-analysis of post-warning studies. Journal of Applied Research in Memory and Cognition, 3 (2), 77–88.
Blank, H., Ost, J., Davies, J., Jones, G., Lambert, K., & Salmon, K. (2013). Comparing the influence of directly vs indirectly encountered post-event misinformation on eyewitness remembering. Acta Psychologica, 144 (3), 635–641.
Buhrmester, M., Kwang, T., & Gosling, S. D. (2011). Amazon’s Mechanical Turk: A new source of inexpensive, yet high-quality, data? Perspectives on Psychological Science, 6 , 3–5.
Cappella, J. N., & Jamieson, K. H. (1994). Broadcast adwatch effects: A field experiment. Communication Research, 21 (3), 342–365.
Casler, K., Bickel, L., & Hackett, E. (2013). Separate but equal? A comparison of participants and data gathered via Amazon’s MTurk, social media, and face-to-face behavioral testing. Computers in Human Behavior, 29 , 2156–2160.
Chambers, K. L., & Zaragoza, M. S. (2001). Intended and unintended effects of explicit warnings on eyewitness suggestibility: Evidence from source identification tests. Memory & Cognition, 29 (8), 1120–1129.
Christiaansen, R. E., & Ochalek, K. (1983). Editing misleading information from memory: Evidence for the coexistence of original and postevent information. Memory & Cognition, 11 (5), 467–475.
Chrobak, Q. M., & Zaragoza, M. S. (2013). The misinformation effect: Past research and recent advances. In A. M. Ridley, F. Gabbert, & D. J. Rooy (Eds.), Suggestibility in legal contexts: Psychological research and forensic implications (pp. 21–44). West Sussex, UK: Wiley-Blackwell.
Google Scholar
Clayton, K., Blair, S., Busam, J. A., Forstner, S., Glance, J., Green, G., et al. (2019). Real solutions for fake news? Measuring the effectiveness of generalwarnings and fact-check tags in reducing belief in false stories on social media. Political Behavior. doi: 10.1007/s11109-019-09533-0.
Cook, J., & Lewandowsky, S. (2011). The debunking handbook . St. Lucia: University of Queensland.
Coppock, A. (2018). Generalizing from survey experiments conducted on Mechanical Turk: A replication approach. Political Science Research and Methods, 7 (3), 1–16.
Dodd, D. H., & Bradshaw, J. M. (1980). Leading questions and memory: Pragmatic constraints. Journal of Verbal Learning and Verbal Behavior, 19 (6), 695–704.
Eakin, D. K., Schreiber, T. A., & Sergent-Marshall, S. (2003). Misinformation effects in eyewitness memory: The presence and absence of memory impairment as a function of warning and misinformation accessibility. Journal of Experimental Psychology: Learning, Memory, and Cognition, 29 (5), 813.
Echterhoff, G., Hirst, W., & Hussy, W. (2005). How eyewitnesses resist misinformation: Social postwarnings and the monitoring of memory characteristics. Memory & Cognition, 33 (5), 770–782.
Echterhoff, G., Groll, S., & Hirst, W. (2007). Tainted truth: Overcorrection for misinformation influence on eyewitness memory. Social Cognition, 25 (3), 367–409.
Ecker, U. K. H., Lewandowsky, S., & Tang, D. T. W. (2010). Explicit warnings reduce but do not eliminate the continued influence of misinformation. Memory & Cognition, 38 (8), 1087–1100.
Flynn, D. J., Nyhan, B., & Reifler, J. (2017). The nature and origins of misperceptions: Understanding false and unsupported beliefs about politics. Political Psychology, Supplement: Advances in Political Psychology, 38 (S1), 127–150.
Frankovic, K. (2016). Belief in conspiracies largely depends on political identity. YouGov . Retrieved April 22, 2019, from https://today.yougov.com/topics/politics/articles-reports/2016/12/27/belief-conspiracies-largely-depends-political-iden .
Frenda, S. J., Nichols, R. M., & Loftus, E. F. (2011). Current issues and advances in misinformation research. Current Directions in Psychological Science, 20 (1), 20–23.
Funke, D. (2019). Facebook announces sweeping changes to its anti-misinformation policies. Poynter , April 10. Retrieved April 22, 2019, from https://www.poynter.org/fact-checking/2019/facebook-announces-sweeping-changes-to-its-anti-misinformation-policies/ .
Gelman, A., & Stern, H. (2006). The difference between “significant” and “not significant” is not itself statistically significant. The American Statistician, 60 (4), 328–331.
Graves, L. (2016). Deciding what’s true: The rise of political fact-checking in American journalism . New York: Columbia University Press.
Book Google Scholar
Graves, L., Nyhan, B., & Reifler, J. (2016). Field experiment examining motivations for fact-checking. Journal of Communication, 66 (1), 102–138.
Greene, E., Flynn, M. S., & Loftus, E. F. (1982). Inducing resistance to misleading information. Journal of Verbal Learning and Verbal Behavior, 21 (2), 207–219.
Grynbaum, M. M. (2019a). Buzzfeed news faces scrutiny after Mueller denies a dramatic Trump report. The New York Times , January 19. Retrieved June 8, 2019, from https://www.nytimes.com/2019/01/19/business/media/buzzfeed-news-trump-michael-cohen-mueller.html .
Grynbaum, M. M. (2019b). Trump discusses claims of ‘fake news,’ and their impact with New York Times publisher. The New York Times , February 1. Retrieved April 22, 2019, from https://nyti.ms/2DMIXwq .
Guess, A., Lyons, B., Montgomery, J. M., Nyhan, B., & Reifler, J. (2018a). Fake news, Facebook ads, and misperceptions: Assessing information quality in the 2018 U.S. midterm election campaign. Retrieved April 22, 2019, from https://www-personal.umich.edu/ bnyhan/fake-news-2018.pdf.
Guess, A., Nyhan, B., & Reifler, J. (2018b). Selective exposure to misinformation: Evidence from the consumption of fake news during the 2016 us presidential campaign. European Research Council , January 9. Retrieved April 22, 2019, from https://www.dartmouth.edu/~nyhan/fake-news-2016.pdf .
Hell, W., Gigerenzer, G., Gauggel, S., Mall, M., & Müller, M. (1988). Hindsight bias: An interaction of automatic and motivational factors? Memory & Cognition, 16 (6), 533–538.
Horton, J. J., Rand, D. G., & Zeckhauser, R. J. (2011). The online laboratory: Conducting experiments in a real labor market. Experimental Economics, 14 (3), 399–425.
Huang, H. (2015). A war of (mis)information: The political effects of rumors and rumor rebuttals in an authoritarian country. British Journal of Political Science, 47 (2), 283–311.
Kang, C. (2019) Facebook’s Hands-Off Approach to Political Speech Gets Impeachment Test. The New York Times , October 8. Retrieved January 3, 2019, from https://www.nytimes.com/2019/10/08/technology/facebook-trump-biden-ad.html .
Lazer, D. M. J., Baum, M. A., Benkler, Y., Berinsky, A. J., Greenhill, K. M., Menczer, F., et al. (2018). The science of fake news: Addressing fake news requires a multidisciplinary effort. Science, 369 (6380), 1094–1096.
Lewandowsky, S., Ecker, U. K. H., Seifert, C. M., Schwarz, N., & Cook, J. (2012). Misinformation and its correction: Continued influence and successful debiasing. Psychological Science in the Public Interest, 13 (3), 106–131.
Loftus, E. F. (1975). Leading questions and the eyewitness report. Cognitive Psychology, 7 (4), 560–572.
Loftus, E. F. (1991). Made in memory: Distortions in recollection after misleading information. In G. H. Bower (Ed.), Psychology of learning and motivation (Vol. 27, pp. 187–215). Cambridge: Academic Press.
Loftus, E. F. (2005). Planting misinformation in the human mind: A 30-year investigation of the malleability of memory. Learning & Memory, 12 (4), 361–366.
Loftus, E. F., Miller, D. G., & Burns, H. J. (1978). Semantic integration of verbal information into a visual memory. Journal of Experimental Psychology: Human Learning and Memory, 4 (1), 19.
Lyons, T. (2018a). Hard questions: What’s Facebook’s strategy for stopping false news? Facebook Newsroom , May 23. Retrieved April 22, 2019, from https://newsroom.fb.com/news/2018/05/hard-questions-false-news/ .
Lyons, T. (2018b). Increasing our efforts to fight false news. Facebook Newsroom , June 21. Retrieved June 23, 2019, from https://newsroom.fb.com/news/2018/06/increasing-our-efforts-to-fight-false-news/ .
Mazzoni, G., & Vannucci, M. (2007). Hindsight bias, the misinformation effect, and false autobiographical memories. Social Cognition, 25 (1), 203–220.
McCloskey, M., & Zaragoza, M. (1985). Misleading postevent information and memory for events: Arguments and evidence against memory impairment hypothesis. Journal of Experimental Psychology: General, 114 , 1–16.
Meade, M. L., & Roediger, H. L. (2002). Explorations in the social contagion of memory. Memory & cognition, 30 (7), 995–1009.
Mosseri, A. (2017). Working to stop misinformation and false news. Facebook for Media , April 7. Retrieved April 22, 2019, from https://newsroom.fb.com/news/2017/04/working-to-stop-misinformation-and-false-news/ .
Mullinix, K. J., Leeper, T. J., Druckman, J. N., & Freese, J. (2015). The generalizability of survey experiments. Journal of Experimental Political Science, 2 (2), 109–138.
Nyhan, B. (2010). Why the “death panel” myth wouldn’t die: Misinformation in the health care reform debate. The Forum, 8 (1), 1–24.
Nyhan, B. (2019). Why fears of fake news are overhyped, February 22. Medium . Retrieved April 22, 2019, from https://medium.com/s/reasonable-doubt/why-fears-of-fake-news-are-overhyped-2ed9ca0a52c9 .
Nyhan, B., & Reifler, J. (2010). When corrections fail: The persistence of political misperceptions. Political Behavior, 32 (2), 303–330.
Nyhan, B., & Reifler, J. (2012). Misinformation and fact-checking: Research findings from social science. Media Policy Initiative, New America Foundation , February 28. Retrieved April 22, 2019, from https://www.newamerica.org/oti/policy-papers/misinformation-and-fact-checking/ .
Pennycook, G., & Rand, D. G. (2017). The implied truth effect: Attaching warnings to a subset of fake news stories increases perceived accuracy of stories without warnings. Retrieved May 8, 2017, from https://tinyurl.com/y25rxlmc .
Pennycook, G., Cannon, T. D., & Rand, D. G. (2018). Prior exposure increases perceived accuracy of fake news. Journal of experimental psychology, 147 (12), 1865–1880.
Pfau, M., & Louden, A. (1994). Effectiveness of adwatch formats in deflecting political attack ads. Communication Research, 21 (3), 325–341.
Podsakoff, P. M., MacKenzie, S. B., & Podsakoff, N. P. (2012). Sources of method bias in social science research and recommendations on how to control it. Annual Review of Psychology, 63 , 539–569.
Putnam, A. L., Sungkhasettee, V. W., & Roediger, H. L., III. (2017). When misinformation improves memory: The effects of recollecting change. Psychological Science, 28 (1), 36–46.
Silverman, C. (2016). This analysis shows how fake election news stories outperformed real news on facebook. Buzzfeed News , November 16. Retrieved April 19, 2019, from https://www.buzzfeednews.com/article/craigsilverman/viral-fake-election-news-outperformed-real-news-on-facebook .
Silverman, C., & Singer-Vine, J. (2016). Most Americans who see fake news believe it, new survey says. Buzzfeed News , December 6. Retrieved April 22, 2019, from https://www.buzzfeednews.com/article/craigsilverman/fake-news-survey .
Silverman, C., Strapagiel, L., Shaban, H., Hall, E., & Singer-Vine, J. (2016). Hyperpartisan Facebook pages are publishing false and misleading information at an alarming rate. Buzzfeed News , October 20. Retrieved April 22, 2019, from https://www.buzzfeednews.com/article/craigsilverman/partisan-fb-pages-analysis .
Spivak, C. (2010). The fact-checking explosion: In a bitter political landscape marked by rampant allegations of questionable credibility, more and more news outlets are launching truth-squad operations. American Journalism Review, 32 (4), 38–44.
Stencel, M. (2019). Number of fact-checking outlets surges to 188 in more than 60 countries. Poynter . Retrieved August 6, 2019, from https://www.poynter.org/fact-checking/2019/number-of-fact-checking-outlets-surges-to-188-in-more-than-60-countries/ .
Sugars, S. (2019). From fake news to enemy of the people: An anatomy of Trump’s tweets. Committee to Protect Journalists , January 30. Retrieved June 8, 2019, from https://cpj.org/blog/2019/01/trump-twitter-press-fake-news-enemy-people.php .
Szpitalak, M., & Polczyk, R. (2010). Warning against warnings: Alerted subjects may perform worse misinformation, involvement and warning as determinants of witness testimony. Polish Psychological Bulletin, 41 (3), 105–112.
Szpitalak, M., & Polczyk, R. (2011). Can warning harm memory? The impact of warning on eyewitness testimony. Problems of Forensic Sciences, 86 , 140–150.
Szpitalak, M., & Polczyk, R. (2012). When does warning help and when does it harm? The impact of warning on eyewitness testimony. Roczniki Psychologiczne/Annals of Psychology, 15 (4), 51–72.
Tandoc, E. C., Jr., Lim, Z. W., & Ling, R. (2018). Defining “fake news” a typology of scholarly definitions. Digital Journalism, 6 (2), 137–153.
Thorson, E. (2016). Belief echoes: The persistent effects of corrected misinformation. Political Communication, 33 (3), 460–480.
Tucker, J., Guess, A., Barberá, P., Vaccari, C., Siegel, A., Sanovich, S., et al. (2018). Social media, political polarization, and political disinformation: A review of the scientific literature. Report. William and Flora Hewlett Foundation . Retrieved December 17, 2019, from https://eprints.lse.ac.uk/87402/1/Social-Media-Political-Polarization-and-Political-Disinformation-Literature-Review.pdf .
van der Linden, S., Leiserowitz, A., Rosenthal, S., & Maibach, E. (2017). Inoculating the public against misinformation about climate change. Global Challenges, 1 (2), 1–7.
Walker, M., & Gotttfried, J. (2019). Republicans far more likely than democrats to say fact-checkers tend to favor one side. Pew Research Center , June 27. Retrieved July 9, 2019, from https://www.pewresearch.org/fact-tank/2019/06/27/republicans-far-more-likely-than-democrats-to-say-fact-checkers-tend-to-favor-one-side/ .
Wardle, C. (2017). Fake news. It’s complicated. FirstDraft , February 16. Retrieved April 22, 2019, from https://medium.com/1st-draft/fake-news-its-complicated-d0f773766c79 .
Wardle, C., & Derakhshan, H. (2017). Information disorder: Toward an interdisciplinary framework for research and policy making (p. 9). DGI: Council of Europe report.
Weeks, B. E. (2015). Emotions, partisanship, and misperceptions: How anger and anxiety moderate the effect of partisan bias on susceptibility to political misinformation. Journal of Communication, 65 (4), 699–719.
Wintersieck, A., Fridkin, K., & Kenney, P. (2018). The message matters: The influence of fact-checking on evaluations of political messages. Journal of Political Marketing . https://doi.org/10.1080/15377857.2018.1457591 .
Wong, T. (2019). Singapore fake news law polices chats and online platforms. BBC News , May 9. Retrieved June 8, 2019, from https://www.bbc.com/news/world-asia-48196985 .
Wright, D. B. (1993). Misinformation and warnings in eyewitness testimony: A new testing procedure to differentiate explanations. Memory, 1 (2), 153–166.
Wyler, H., & Oswald, M. E. (2016). Why misinformation is reported: Evidence from a warning and a source-monitoring task. Memory, 24 (10), 1419–1434.
Zaller, J. R. (1992). The Nature and Origins of Mass Opinion . Cambridge, UK: Cambridge University Press.
Zaragoza, M. S., Belli, Robert F., & Payment, K. E. (2006). Misinformation effects and the suggestibility of eyewitness memory. In Garry, M. and Hayne, H. (Eds.), Do justice and let the sky fall: Elizabeth F. Loftus and her contributions to science, law, and academic freedom (pp. 35–63). Hillsdale, NJ: Lawrence Erlbaum Associates.
Download references
Acknowledgements
Other co-authors are or were undergraduate students at Carleton College (Yoichiro Ashida, Mitch Bermel, Sharaka Berry, Jeremy Brog, Ursula Clausing, Eveline Dowling, Maximilian Esslinger, M. Forsyth, Malcom Fox, Shayna Gleason, Lea Gould, Boluwatife Johnson, Schuyler, Kapnick, Mark Leedy, Calypso Leonard, Malekai Mischke, Isabel Storey, Oliver Wolyniec, Sol Yanuck), Spring 2017 POSC 226 course. We thank Carleton College, Department of Political Science and Dean of the College for generous funding support and the Headley Travel Fund Grant. We also thank the Department of Political Science at Brigham Young University-Provo for workshop travel support. We are grateful for the advice of Brendan Nyhan, Jessica Preece, Kent Freeze and two anonymous reviewers.
Author information
Authors and affiliations.
Department of Political Science, Carleton College, One North College Street, Northfield, MN, 55057, USA
Melanie Freeze
Minneapolis, USA
Mary Baumgartner
Washington, USA
Peter Bruno
Department of Political Science, University of North Carolina at Chapel Hill, 361 Hamilton Hall, CB 3265, Chapel Hill, 27599-3265, North Carolina, USA
Jacob R. Gunderson
New York, USA
Joshua Olin
School of Communication, Ohio State University, 3016 Derby Hall, 154 N Oval Mall, Columbus, 43210, Ohio, USA
Morgan Quinn Ross
Wilmette, USA
Justine Szafran
You can also search for this author in PubMed Google Scholar
Corresponding author
Correspondence to Melanie Freeze .
Additional information
Publisher's note.
Springer Nature remains neutral with regard to jurisdictional claims in published maps and institutional affiliations.
Electronic supplementary material
Below is the link to the electronic supplementary material.
Supplementary file1 (DOCX 1995 kb)
Supplementary file2 (docx 340 kb), rights and permissions.
Open Access This article is licensed under a Creative Commons Attribution 4.0 International License, which permits use, sharing, adaptation, distribution and reproduction in any medium or format, as long as you give appropriate credit to the original author(s) and the source, provide a link to the Creative Commons licence, and indicate if changes were made. The images or other third party material in this article are included in the article's Creative Commons licence, unless indicated otherwise in a credit line to the material. If material is not included in the article's Creative Commons licence and your intended use is not permitted by statutory regulation or exceeds the permitted use, you will need to obtain permission directly from the copyright holder. To view a copy of this licence, visit http://creativecommons.org/licenses/by/4.0/ .
Reprints and permissions
About this article
Freeze, M., Baumgartner, M., Bruno, P. et al. Fake Claims of Fake News: Political Misinformation, Warnings, and the Tainted Truth Effect. Polit Behav 43 , 1433–1465 (2021). https://doi.org/10.1007/s11109-020-09597-3
Download citation
Published : 05 February 2020
Issue Date : December 2021
DOI : https://doi.org/10.1007/s11109-020-09597-3
Share this article
Anyone you share the following link with will be able to read this content:
Sorry, a shareable link is not currently available for this article.
Provided by the Springer Nature SharedIt content-sharing initiative
- Tainted truth effect
- Misinformation
- Find a journal
- Publish with us
- Track your research
- DOI: 10.1146/annurev-polisci-050718-032814
- Corpus ID: 212733536
Political Misinformation
- Jennifer Jerit , Yangzi Zhao
- Published 2020
- Political Science
123 Citations
Issue importance and the correction of misinformation, misinformation, disinformation, and fake news: lessons from an interdisciplinary, systematic literature review, the liar’s dividend: can politicians claim misinformation to evade accountability, the importance of epistemology for the study of misinformation., measuring misperceptions, misperceptions about immigration: reviewing their nature, motivations and determinants, acquiescence bias inflates estimates of conspiratorial beliefs and political misperceptions, political fact-checking and its effects on public attitudes: experimental evidence from china, how news coverage of misinformation shapes perceptions and trust, research note: lies and presidential debates: how political misinformation spread across media streams during the 2020 election, 105 references, misinformation and the currency of democratic citizenship.
- Highly Influential
- 10 Excerpts
Misinformation and How to Correct It
Political attitudes and the processing of misinformation corrections, when corrections fail: the persistence of political misperceptions, the nature and origins of misperceptions: understanding false and unsupported beliefs about politics, processing political misinformation: comprehending the trump phenomenon, partisan bias in surveys, beliefs don't always persevere: how political figures are punished when positive information about them is discredited, bankrupt rhetoric how misleading information affects knowledge about social security, belief echoes: the persistent effects of corrected misinformation, related papers.
Showing 1 through 3 of 0 Related Papers
- About The Journalist’s Resource
- Follow us on Facebook
- Follow us on Twitter
- Criminal Justice
- Environment
- Politics & Government
- Race & Gender
Expert Commentary
Fake news and the spread of misinformation: A research roundup
This collection of research offers insights into the impacts of fake news and other forms of misinformation, including fake Twitter images, and how people use the internet to spread rumors and misinformation.
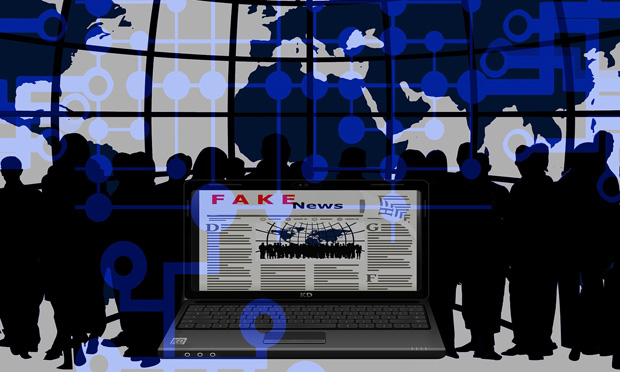
Republish this article

This work is licensed under a Creative Commons Attribution-NoDerivatives 4.0 International License .
by Denise-Marie Ordway, The Journalist's Resource September 1, 2017
This <a target="_blank" href="https://journalistsresource.org/politics-and-government/fake-news-conspiracy-theories-journalism-research/">article</a> first appeared on <a target="_blank" href="https://journalistsresource.org">The Journalist's Resource</a> and is republished here under a Creative Commons license.<img src="https://journalistsresource.org/wp-content/uploads/2020/11/cropped-jr-favicon-150x150.png" style="width:1em;height:1em;margin-left:10px;">
It’s too soon to say whether Google ’s and Facebook ’s attempts to clamp down on fake news will have a significant impact. But fabricated stories posing as serious journalism are not likely to go away as they have become a means for some writers to make money and potentially influence public opinion. Even as Americans recognize that fake news causes confusion about current issues and events, they continue to circulate it. A December 2016 survey by the Pew Research Center suggests that 23 percent of U.S. adults have shared fake news, knowingly or unknowingly, with friends and others.
“Fake news” is a term that can mean different things, depending on the context. News satire is often called fake news as are parodies such as the “Saturday Night Live” mock newscast Weekend Update. Much of the fake news that flooded the internet during the 2016 election season consisted of written pieces and recorded segments promoting false information or perpetuating conspiracy theories. Some news organizations published reports spotlighting examples of hoaxes, fake news and misinformation on Election Day 2016.
The news media has written a lot about fake news and other forms of misinformation, but scholars are still trying to understand it — for example, how it travels and why some people believe it and even seek it out. Below, Journalist’s Resource has pulled together academic studies to help newsrooms better understand the problem and its impacts. Two other resources that may be helpful are the Poynter Institute’s tips on debunking fake news stories and the First Draft Partner Network , a global collaboration of newsrooms, social media platforms and fact-checking organizations that was launched in September 2016 to battle fake news. In mid-2018, JR ‘s managing editor, Denise-Marie Ordway, wrote an article for Harvard Business Review explaining what researchers know to date about the amount of misinformation people consume, why they believe it and the best ways to fight it.
—————————
“The Science of Fake News” Lazer, David M. J.; et al. Science , March 2018. DOI: 10.1126/science.aao2998.
Summary: “The rise of fake news highlights the erosion of long-standing institutional bulwarks against misinformation in the internet age. Concern over the problem is global. However, much remains unknown regarding the vulnerabilities of individuals, institutions, and society to manipulations by malicious actors. A new system of safeguards is needed. Below, we discuss extant social and computer science research regarding belief in fake news and the mechanisms by which it spreads. Fake news has a long history, but we focus on unanswered scientific questions raised by the proliferation of its most recent, politically oriented incarnation. Beyond selected references in the text, suggested further reading can be found in the supplementary materials.”
“Who Falls for Fake News? The Roles of Bullshit Receptivity, Overclaiming, Familiarity, and Analytical Thinking” Pennycook, Gordon; Rand, David G. May 2018. Available at SSRN. DOI: 10.2139/ssrn.3023545.
Abstract: “Inaccurate beliefs pose a threat to democracy and fake news represents a particularly egregious and direct avenue by which inaccurate beliefs have been propagated via social media. Here we present three studies (MTurk, N = 1,606) investigating the cognitive psychological profile of individuals who fall prey to fake news. We find consistent evidence that the tendency to ascribe profundity to randomly generated sentences — pseudo-profound bullshit receptivity — correlates positively with perceptions of fake news accuracy, and negatively with the ability to differentiate between fake and real news (media truth discernment). Relatedly, individuals who overclaim regarding their level of knowledge (i.e. who produce bullshit) also perceive fake news as more accurate. Conversely, the tendency to ascribe profundity to prototypically profound (non-bullshit) quotations is not associated with media truth discernment; and both profundity measures are positively correlated with willingness to share both fake and real news on social media. We also replicate prior results regarding analytic thinking — which correlates negatively with perceived accuracy of fake news and positively with media truth discernment — and shed further light on this relationship by showing that it is not moderated by the presence versus absence of information about the new headline’s source (which has no effect on perceived accuracy), or by prior familiarity with the news headlines (which correlates positively with perceived accuracy of fake and real news). Our results suggest that belief in fake news has similar cognitive properties to other forms of bullshit receptivity, and reinforce the important role that analytic thinking plays in the recognition of misinformation.”
“Social Media and Fake News in the 2016 Election” Allcott, Hunt; Gentzkow, Matthew. Working paper for the National Bureau of Economic Research, No. 23089, 2017.
Abstract: “We present new evidence on the role of false stories circulated on social media prior to the 2016 U.S. presidential election. Drawing on audience data, archives of fact-checking websites, and results from a new online survey, we find: (i) social media was an important but not dominant source of news in the run-up to the election, with 14 percent of Americans calling social media their “most important” source of election news; (ii) of the known false news stories that appeared in the three months before the election, those favoring Trump were shared a total of 30 million times on Facebook, while those favoring Clinton were shared eight million times; (iii) the average American saw and remembered 0.92 pro-Trump fake news stories and 0.23 pro-Clinton fake news stories, with just over half of those who recalled seeing fake news stories believing them; (iv) for fake news to have changed the outcome of the election, a single fake article would need to have had the same persuasive effect as 36 television campaign ads.”
“Debunking: A Meta-Analysis of the Psychological Efficacy of Messages Countering Misinformation” Chan, Man-pui Sally; Jones, Christopher R.; Jamieson, Kathleen Hall; Albarracín, Dolores. Psychological Science , September 2017. DOI: 10.1177/0956797617714579.
Abstract: “This meta-analysis investigated the factors underlying effective messages to counter attitudes and beliefs based on misinformation. Because misinformation can lead to poor decisions about consequential matters and is persistent and difficult to correct, debunking it is an important scientific and public-policy goal. This meta-analysis (k = 52, N = 6,878) revealed large effects for presenting misinformation (ds = 2.41–3.08), debunking (ds = 1.14–1.33), and the persistence of misinformation in the face of debunking (ds = 0.75–1.06). Persistence was stronger and the debunking effect was weaker when audiences generated reasons in support of the initial misinformation. A detailed debunking message correlated positively with the debunking effect. Surprisingly, however, a detailed debunking message also correlated positively with the misinformation-persistence effect.”
“Displacing Misinformation about Events: An Experimental Test of Causal Corrections” Nyhan, Brendan; Reifler, Jason. Journal of Experimental Political Science , 2015. doi: 10.1017/XPS.2014.22.
Abstract: “Misinformation can be very difficult to correct and may have lasting effects even after it is discredited. One reason for this persistence is the manner in which people make causal inferences based on available information about a given event or outcome. As a result, false information may continue to influence beliefs and attitudes even after being debunked if it is not replaced by an alternate causal explanation. We test this hypothesis using an experimental paradigm adapted from the psychology literature on the continued influence effect and find that a causal explanation for an unexplained event is significantly more effective than a denial even when the denial is backed by unusually strong evidence. This result has significant implications for how to most effectively counter misinformation about controversial political events and outcomes.”
“Rumors and Health Care Reform: Experiments in Political Misinformation” Berinsky, Adam J. British Journal of Political Science , 2015. doi: 10.1017/S0007123415000186.
Abstract: “This article explores belief in political rumors surrounding the health care reforms enacted by Congress in 2010. Refuting rumors with statements from unlikely sources can, under certain circumstances, increase the willingness of citizens to reject rumors regardless of their own political predilections. Such source credibility effects, while well known in the political persuasion literature, have not been applied to the study of rumor. Though source credibility appears to be an effective tool for debunking political rumors, risks remain. Drawing upon research from psychology on ‘fluency’ — the ease of information recall — this article argues that rumors acquire power through familiarity. Attempting to quash rumors through direct refutation may facilitate their diffusion by increasing fluency. The empirical results find that merely repeating a rumor increases its power.”
“Rumors and Factitious Informational Blends: The Role of the Web in Speculative Politics” Rojecki, Andrew; Meraz, Sharon. New Media & Society , 2016. doi: 10.1177/1461444814535724.
Abstract: “The World Wide Web has changed the dynamics of information transmission and agenda-setting. Facts mingle with half-truths and untruths to create factitious informational blends (FIBs) that drive speculative politics. We specify an information environment that mirrors and contributes to a polarized political system and develop a methodology that measures the interaction of the two. We do so by examining the evolution of two comparable claims during the 2004 presidential campaign in three streams of data: (1) web pages, (2) Google searches, and (3) media coverage. We find that the web is not sufficient alone for spreading misinformation, but it leads the agenda for traditional media. We find no evidence for equality of influence in network actors.”
“Analyzing How People Orient to and Spread Rumors in Social Media by Looking at Conversational Threads” Zubiaga, Arkaitz; et al. PLOS ONE, 2016. doi: 10.1371/journal.pone.0150989.
Abstract: “As breaking news unfolds people increasingly rely on social media to stay abreast of the latest updates. The use of social media in such situations comes with the caveat that new information being released piecemeal may encourage rumors, many of which remain unverified long after their point of release. Little is known, however, about the dynamics of the life cycle of a social media rumor. In this paper we present a methodology that has enabled us to collect, identify and annotate a dataset of 330 rumor threads (4,842 tweets) associated with 9 newsworthy events. We analyze this dataset to understand how users spread, support, or deny rumors that are later proven true or false, by distinguishing two levels of status in a rumor life cycle i.e., before and after its veracity status is resolved. The identification of rumors associated with each event, as well as the tweet that resolved each rumor as true or false, was performed by journalist members of the research team who tracked the events in real time. Our study shows that rumors that are ultimately proven true tend to be resolved faster than those that turn out to be false. Whilst one can readily see users denying rumors once they have been debunked, users appear to be less capable of distinguishing true from false rumors when their veracity remains in question. In fact, we show that the prevalent tendency for users is to support every unverified rumor. We also analyze the role of different types of users, finding that highly reputable users such as news organizations endeavor to post well-grounded statements, which appear to be certain and accompanied by evidence. Nevertheless, these often prove to be unverified pieces of information that give rise to false rumors. Our study reinforces the need for developing robust machine learning techniques that can provide assistance in real time for assessing the veracity of rumors. The findings of our study provide useful insights for achieving this aim.”
“Miley, CNN and The Onion” Berkowitz, Dan; Schwartz, David Asa. Journalism Practice , 2016. doi: 10.1080/17512786.2015.1006933.
Abstract: “Following a twerk-heavy performance by Miley Cyrus on the Video Music Awards program, CNN featured the story on the top of its website. The Onion — a fake-news organization — then ran a satirical column purporting to be by CNN’s Web editor explaining this decision. Through textual analysis, this paper demonstrates how a Fifth Estate comprised of bloggers, columnists and fake news organizations worked to relocate mainstream journalism back to within its professional boundaries.”
“Emotions, Partisanship, and Misperceptions: How Anger and Anxiety Moderate the Effect of Partisan Bias on Susceptibility to Political Misinformation”
Weeks, Brian E. Journal of Communication , 2015. doi: 10.1111/jcom.12164.
Abstract: “Citizens are frequently misinformed about political issues and candidates but the circumstances under which inaccurate beliefs emerge are not fully understood. This experimental study demonstrates that the independent experience of two emotions, anger and anxiety, in part determines whether citizens consider misinformation in a partisan or open-minded fashion. Anger encourages partisan, motivated evaluation of uncorrected misinformation that results in beliefs consistent with the supported political party, while anxiety at times promotes initial beliefs based less on partisanship and more on the information environment. However, exposure to corrections improves belief accuracy, regardless of emotion or partisanship. The results indicate that the unique experience of anger and anxiety can affect the accuracy of political beliefs by strengthening or attenuating the influence of partisanship.”
“Deception Detection for News: Three Types of Fakes” Rubin, Victoria L.; Chen, Yimin; Conroy, Niall J. Proceedings of the Association for Information Science and Technology , 2015, Vol. 52. doi: 10.1002/pra2.2015.145052010083.
Abstract: “A fake news detection system aims to assist users in detecting and filtering out varieties of potentially deceptive news. The prediction of the chances that a particular news item is intentionally deceptive is based on the analysis of previously seen truthful and deceptive news. A scarcity of deceptive news, available as corpora for predictive modeling, is a major stumbling block in this field of natural language processing (NLP) and deception detection. This paper discusses three types of fake news, each in contrast to genuine serious reporting, and weighs their pros and cons as a corpus for text analytics and predictive modeling. Filtering, vetting, and verifying online information continues to be essential in library and information science (LIS), as the lines between traditional news and online information are blurring.”
“When Fake News Becomes Real: Combined Exposure to Multiple News Sources and Political Attitudes of Inefficacy, Alienation, and Cynicism” Balmas, Meital. Communication Research , 2014, Vol. 41. doi: 10.1177/0093650212453600.
Abstract: “This research assesses possible associations between viewing fake news (i.e., political satire) and attitudes of inefficacy, alienation, and cynicism toward political candidates. Using survey data collected during the 2006 Israeli election campaign, the study provides evidence for an indirect positive effect of fake news viewing in fostering the feelings of inefficacy, alienation, and cynicism, through the mediator variable of perceived realism of fake news. Within this process, hard news viewing serves as a moderator of the association between viewing fake news and their perceived realism. It was also demonstrated that perceived realism of fake news is stronger among individuals with high exposure to fake news and low exposure to hard news than among those with high exposure to both fake and hard news. Overall, this study contributes to the scientific knowledge regarding the influence of the interaction between various types of media use on political effects.”
“Faking Sandy: Characterizing and Identifying Fake Images on Twitter During Hurricane Sandy” Gupta, Aditi; Lamba, Hemank; Kumaraguru, Ponnurangam; Joshi, Anupam. Proceedings of the 22nd International Conference on World Wide Web , 2013. doi: 10.1145/2487788.2488033.
Abstract: “In today’s world, online social media plays a vital role during real world events, especially crisis events. There are both positive and negative effects of social media coverage of events. It can be used by authorities for effective disaster management or by malicious entities to spread rumors and fake news. The aim of this paper is to highlight the role of Twitter during Hurricane Sandy (2012) to spread fake images about the disaster. We identified 10,350 unique tweets containing fake images that were circulated on Twitter during Hurricane Sandy. We performed a characterization analysis, to understand the temporal, social reputation and influence patterns for the spread of fake images. Eighty-six percent of tweets spreading the fake images were retweets, hence very few were original tweets. Our results showed that the top 30 users out of 10,215 users (0.3 percent) resulted in 90 percent of the retweets of fake images; also network links such as follower relationships of Twitter, contributed very little (only 11 percent) to the spread of these fake photos URLs. Next, we used classification models, to distinguish fake images from real images of Hurricane Sandy. Best results were obtained from Decision Tree classifier, we got 97 percent accuracy in predicting fake images from real. Also, tweet-based features were very effective in distinguishing fake images tweets from real, while the performance of user-based features was very poor. Our results showed that automated techniques can be used in identifying real images from fake images posted on Twitter.”
“The Impact of Real News about ‘Fake News’: Intertextual Processes and Political Satire” Brewer, Paul R.; Young, Dannagal Goldthwaite; Morreale, Michelle. International Journal of Public Opinion Research , 2013. doi: 10.1093/ijpor/edt015.
Abstract: “This study builds on research about political humor, press meta-coverage, and intertextuality to examine the effects of news coverage about political satire on audience members. The analysis uses experimental data to test whether news coverage of Stephen Colbert’s Super PAC influenced knowledge and opinion regarding Citizens United, as well as political trust and internal political efficacy. It also tests whether such effects depended on previous exposure to The Colbert Report (Colbert’s satirical television show) and traditional news. Results indicate that exposure to news coverage of satire can influence knowledge, opinion, and political trust. Additionally, regular satire viewers may experience stronger effects on opinion, as well as increased internal efficacy, when consuming news coverage about issues previously highlighted in satire programming.”
“With Facebook, Blogs, and Fake News, Teens Reject Journalistic ‘Objectivity’” Marchi, Regina. Journal of Communication Inquiry , 2012. doi: 10.1177/0196859912458700.
Abstract: “This article examines the news behaviors and attitudes of teenagers, an understudied demographic in the research on youth and news media. Based on interviews with 61 racially diverse high school students, it discusses how adolescents become informed about current events and why they prefer certain news formats to others. The results reveal changing ways news information is being accessed, new attitudes about what it means to be informed, and a youth preference for opinionated rather than objective news. This does not indicate that young people disregard the basic ideals of professional journalism but, rather, that they desire more authentic renderings of them.”
Keywords: alt-right, credibility, truth discovery, post-truth era, fact checking, news sharing, news literacy, misinformation, disinformation
5 fascinating digital media studies from fall 2018
Facebook and the newsroom: 6 questions for Siva Vaidhyanathan
About The Author
Denise-Marie Ordway
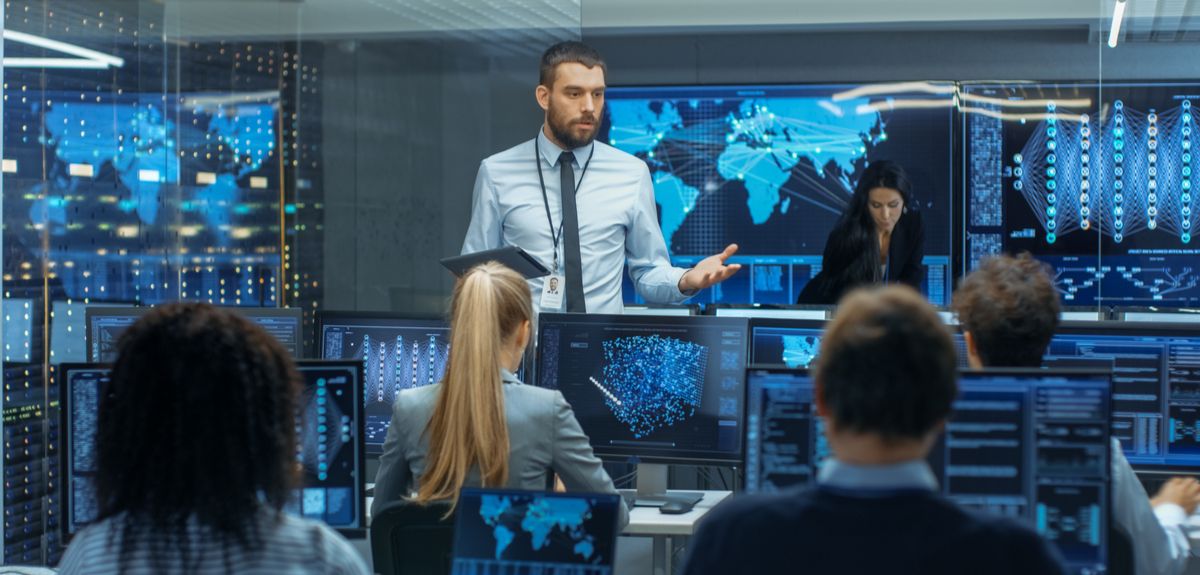
Social media manipulation by political actors an industrial scale problem - Oxford report
Social media manipulation of public opinion is a growing threat to democracies around the world, according to the 2020 media manipulation survey from the Oxford Internet Institute , which found evidence in every one of the 80+ countries surveyed.
Organised social media manipulation campaigns were found in each of the 81 surveyed countries, up 15% in one year, from 70 countries in 2019. Governments, public relations firms and political parties are producing misinformation on an industrial scale, according to the report. It shows disinformation has become a common strategy, with more than 93% of the countries (76 out of 81) seeing disinformation deployed as part of political communication.
Social media manipulation of public opinion is a growing threat to democracies around the world
Professor Philip Howard , Director of the Oxford Internet Institute, and the report’s co-author says, ‘Our report shows misinformation has become more professionalised and is now produced on an industrial scale. Now, more than ever, the public needs to be able to rely on trustworthy information about government policy and activity. Social media companies need to raise their game by increasing their efforts to flag misinformation and close fake accounts without the need for government intervention, so the public has access to high-quality information.’
Social media companies need to raise their game by increasing their efforts to flag misinformation and close fake accounts without the need for government intervention, so the public has access to high-quality information Professor Philip Howard
The OII team warns the level of social media manipulation has soared, with governments and political parties spending millions on private sector ‘cyber troops’, who drown out other voices on social media. Citizen influencers are used to spread manipulated messages. These include volunteers, youth groups and civil society organisations, who support their ideologies.
OII alumna, Dr Samantha Bradshaw, the report’s lead author says, ‘Our 2020 report highlights the way in which government agencies, political parties and private firms continue to use social media to spread political propaganda, polluting the digital information ecosystem and suppressing freedom of speech and freedom of the press. A large part of this activity has become professionalised, with private firms offering disinformation-for-hire services.’
Key findings the OII researchers identified include:
- Private ‘strategic communications’ firms are playing an increasing role in spreading computational propaganda, with researchers identifying state actors working with such firms in 48 countries.
- Almost $60 million has been spent on firms who use bots and other amplification strategies to create the impression of trending political messaging.
- Social media has become a major battleground, with firms such as Facebook and Twitter taking steps to combat ‘cyber troops’, with some $10 million has been spent on social media political advertisements. The platforms removed more than 317,000 accounts and pages from ‘cyber troops’ actors between January 2019 and November 2020.
This activity has become professionalised, with private firms offering disinformation-for-hire services Dr Samantha Bradshaw
Cyber troops are frequently directly linked to state agencies. According to the report, ‘In 62 countries, we found evidence of a government agency using computational propaganda to shape public attitudes.’
Established political parties were also found to be using social media to ‘spread disinformation, suppress political participation, and undermine oppositional parties’, say the Oxford researchers.
According to the report, ‘In 61 countries, we found evidence of political parties or politicians running for office who have used the tools and techniques of computational propaganda as part of their political campaigns. Indeed, social media has become a critical component of digital campaigning.’
We found evidence of political parties or politicians running for office who have used the tools and techniques of computational propaganda as part of their political campaigns....social media has become a critical component of digital campaigning
Dr Bradshaw adds, ‘Cyber troop activity can look different in democracies compared to authoritarian regimes. Electoral authorities need to consider the broader ecosystem of disinformation and computational propaganda, including private firms and paid influencers, who are increasingly prominent actors in this space.’
The report explores the tools and techniques of computational propaganda, including the use of fake accounts – bots, humans and hacked accounts – to spread disinformation. It finds:
- 79 countries used human accounts,
- 57 counties used bot accounts, and
- 14 countries used hacked or stolen accounts.
Researchers examined how cyber troops use different communication strategies to manipulate public opinion, such as creating disinformation or manipulated media, data-driven targeting and employing abusive strategies such as mounting smear campaigns or online harassment. The report finds:
- 76 countries used disinformation and media manipulation as part of their campaigns,
- 30 countries used data-drive strategies to target specific users with political advertisements,
- 59 countries used state sponsored trolls to attack political opponents or activists in 2019, up from 47 countries in 2019.
The 2020 report draws upon a four-step methodology employed by Oxford researchers to identify evidence of globally organised manipulation campaigns. This includes a systematic content analysis of news articles on cyber troop activity, a secondary literature review of public archives and scientific reports, generating country specific case studies and expert consultations.
The research work was carried out by Oxford researchers between 2019 and 2020. Computational Propaganda project research studies are published at https://demtech.oii.ox.ac.uk/research/posts/industrialized-disinformation/

DISCOVER MORE
- Support Oxford's research
- Partner with Oxford on research
- Study at Oxford
- Research jobs at Oxford
You can view all news or browse by category
How to combat fake news and disinformation
Subscribe to the center for technology innovation newsletter, darrell m. west darrell m. west senior fellow - center for technology innovation , douglas dillon chair in governmental studies.
December 18, 2017
Executive summary
Journalism is in a state of considerable flux. New digital platforms have unleashed innovative journalistic practices that enable novel forms of communication and greater global reach than at any point in human history. But on the other hand, disinformation and hoaxes that are popularly referred to as “fake news” are accelerating and affecting the way individuals interpret daily developments. Driven by foreign actors, citizen journalism, and the proliferation of talk radio and cable news, many information systems have become more polarized and contentious, and there has been a precipitous decline in public trust in traditional journalism.
Fake news and sophisticated disinformation campaigns are especially problematic in democratic systems, and there is growing debate on how to address these issues without undermining the benefits of digital media. In order to maintain an open, democratic system, it is important that government, business, and consumers work together to solve these problems. Governments should promote news literacy and strong professional journalism in their societies. The news industry must provide high-quality journalism in order to build public trust and correct fake news and disinformation without legitimizing them. Technology companies should invest in tools that identify fake news, reduce financial incentives for those who profit from disinformation, and improve online accountability. Educational institutions should make informing people about news literacy a high priority. Finally, individuals should follow a diversity of news sources, and be skeptical of what they read and watch.
The state of the news media
The news media landscape has changed dramatically over the past decades. Through digital sources, there has been a tremendous increase in the reach of journalism, social media, and public engagement. Checking for news online—whether through Google, Twitter, Facebook, major newspapers, or local media websites—has become ubiquitous, and smartphone alerts and mobile applications bring the latest developments to people instantaneously around the world. As of 2017, 93 percent of Americans say they receive news online. 1 When asked where they got online news in the last two hours, 36 percent named a news organization website or app; 35 percent said social media (which typically means a post from a news organization, but can be a friend’s commentary); 20 percent recalled a search engine; 15 percent indicated a news organization email, text, or alert; 9 percent said it was another source; and 7 percent named a family member email or text (see Figure 1). 2
In general, young people are most likely to get their news through online sources, relying heavily on mobile devices for their communications. According to the Pew Research Center, 55 percent of smartphone users receive news alerts on their devices. And about 47 percent of those receiving alerts click through to read the story. 3 Increasingly, people can customize information delivery to their personal preferences. For example, it is possible to sign up for news alerts from many organizations so that people hear news relevant to their particular interests.
There have been changes overtime in sources of news overall. Figure 2 shows the results for 2012 to 2017. It demonstrates that the biggest gain has been in reliance upon social media. In 2012-2013, 27 percent relied upon social media sites, compared to 51 percent who did so in 2017. 4 In contrast, the percentage of Americans relying upon print news has dropped from 38 to 22 percent.
A number of research organizations have found significant improvements in digital access around the world. For example, the Pew Research Center has documented through surveys in 21 emerging nations that internet usage has risen from 45 percent in 2013 to 54 percent in 2015. That number still trails the 87 percent usage figure seen in 11 developed countries, but there clearly have been major gains in many places around the world. 5
Social media sites are very popular in the developing world. As shown in Figure 3, 86 percent of Middle Eastern internet users rely upon social networks, compared to 82 percent in Latin America, 76 percent in Africa, 71 percent in the United States, 66 percent in Asia and the Pacific, and 65 percent in Europe.
In addition, the Reuters Institute for the Study of Journalism has demonstrated important trends in news consumption. It has shown major gains in reliance upon mobile news notifications. The percentage of people in the United States making use of this source has risen by 8 percentage points, while there have been gains of 7 percentage points in South Korea and 4 percentage points in Australia. There also have been increases in the use of news aggregators, digital news sources, and voice-activated digital assistants. 6
Declining trust in the news media
In the United States, there is a declining public trust in traditional journalism. The Gallup Poll asked a number of Americans over the past two decades how much trust and confidence they have in mass media reporting the news fully, accurately, and fairly. As shown in Figure 4, the percentage saying they had a great deal or fair amount of trust dropped from 53 percent in 1997 to 32 percent in 2016. 7
Between news coverage they don’t like and fake news that is manipulative in nature, many Americans question the accuracy of their news. A recent Gallup poll found that only 37 percent believe “news organizations generally get the facts straight.” This is down from about half of the country who felt that way in 1998. There is also a startling partisan divide in public assessments. Only 14 percent of Republicans believe the media report the news accurately, compared to 62 percent for Democrats. Even more disturbingly, “a solid majority of the country believes major news organizations routinely produce false information.” 8
This decline in public trust in media is dangerous for democracies. With the current political situation in a state of great flux in the U.S. and around the world, there are questions concerning the quality of the information available to the general public and the impact of marginal media organizations on voter assessments. These developments have complicated the manner in which people hold leaders accountable and the way in which our political system operates.
Challenges facing the digital media landscape
As the overall media landscape has changed, there have been several ominous developments. Rather than using digital tools to inform people and elevate civic discussion, some individuals have taken advantage of social and digital platforms to deceive, mislead, or harm others through creating or disseminating fake news and disinformation.
Fake news is generated by outlets that masquerade as actual media sites but promulgate false or misleading accounts designed to deceive the public. When these activities move from sporadic and haphazard to organized and systematic efforts, they become disinformation campaigns with the potential to disrupt campaigns and governance in entire countries. 9
As an illustration, the United States saw apparently organized efforts to disseminate false material in the 2016 presidential election. A Buzzfeed analysis found that the most widely shared fake news stories in 2016 were about “Pope Francis endorsing Donald Trump, Hillary Clinton selling weapons to ISIS, Hillary Clinton being disqualified from holding federal office, and the FBI director receiving millions from the Clinton Foundation.” 10 Using a social media assessment, it claimed that the 20 largest fake stories generated 8.7 million shares, reactions, and comments, compared to 7.4 million generated by the top 20 stories from 19 major news sites.
When [fake news] activities move from sporadic and haphazard to organized and systematic efforts, they become disinformation campaigns with the potential to disrupt campaigns and governance in entire countries.
Fake content was widespread during the presidential campaign. Facebook has estimated that 126 million of its platform users saw articles and posts promulgated by Russian sources. Twitter has found 2,752 accounts established by Russian groups that tweeted 1.4 million times in 2016. 11 The widespread nature of these disinformation efforts led Columbia Law School Professor Tim Wu to ask: “Did Twitter kill the First Amendment?” 12
A specific example of disinformation was the so-called “Pizzagate” conspiracy, which started on Twitter. The story falsely alleged that sexually abused children were hidden at Comet Ping Pong, a Washington, D.C. pizza parlor, and that Hillary Clinton knew about the sex ring. It seemed so realistic to some that a North Carolina man named Edgar Welch drove to the capital city with an assault weapon to personally search for the abused kids. After being arrested by the police, Welch said “that he had read online that the Comet restaurant was harboring child sex slaves and that he wanted to see for himself if they were there. [Welch] stated that he was armed.” 13
A post-election survey of 3,015 American adults suggested that it is difficult for news consumers to distinguish fake from real news. Chris Jackson of Ipsos Public Affairs undertook a survey that found “fake news headlines fool American adults about 75 percent of the time” and “‘fake news’ was remembered by a significant portion of the electorate and those stories were seen as credible.” 14 Another online survey of 1,200 individuals after the election by Hunt Allcott and Matthew Gentzkow found that half of those who saw these fake stories believed their content. 15
False news stories are not just a problem in the United States, but afflict other countries around the world. For example, India has been plagued by fake news concerning cyclones, public health, and child abuse. When intertwined with religious or caste issues, the combination can be explosive and lead to violence. People have been killed when false rumors have spread through digital media about child abductions. 16
Sometimes, fake news stories are amplified and disseminated quickly through false accounts, or automated “bots.” Most bots are benign in nature, and some major sites like Facebook ban bots and seek to remove them, but there are social bots that are “malicious entities designed specifically with the purpose to harm. These bots mislead, exploit, and manipulate social media discourse with rumors, spam, malware, misinformation, slander, or even just noise.” 17
This information can distort election campaigns, affect public perceptions, or shape human emotions. Recent research has found that “elusive bots could easily infiltrate a population of unaware humans and manipulate them to affect their perception of reality, with unpredictable results.” 18 In some cases, they can “engage in more complex types of interactions, such as entertaining conversations with other people, commenting on their posts, and answering their questions.” Through designated keywords and interactions with influential posters, they can magnify their influence and affect national or global conversations, especially resonating with like-minded clusters of people. 19
An analysis after the 2016 election found that automated bots played a major role in disseminating false information on Twitter. According to Jonathan Albright, an assistant professor of media analytics at Elon University, “what bots are doing is really getting this thing trending on Twitter. These bots are providing the online crowds that are providing legitimacy.” 20 With digital content, the more posts that are shared or liked, the more traffic they generate. Through these means, it becomes relatively easy to spread fake information over the internet. For example, as graphic content spreads, often with inflammatory comments attached, it can go viral and be seen as credible information by people far from the original post.
Everyone has a responsibility to combat the scourge of fake news. This ranges from supporting investigative journalism, reducing financial incentives for fake news, and improving digital literacy among the general public.
False information is dangerous because of its ability to affect public opinion and electoral discourse. According to David Lazer, “such situations can enable discriminatory and inflammatory ideas to enter public discourse and be treated as fact. Once embedded, such ideas can in turn be used to create scapegoats, to normalize prejudices, to harden us-versus-them mentalities and even, in extreme cases, to catalyze and justify violence.” 21 As he points out, factors such as source credibility, repetition, and social pressure affect information flows and the extent to which misinformation is taken seriously. When viewers see trusted sources repeat certain points, they are more likely to be influenced by that material.
Recent polling data demonstrate how harmful these practices have become to the reputations of reputable platforms. According to the Reuters Institute for the Study of Journalism, only 24 percent of Americans today believe social media sites “do a good job separating fact from fiction, compared to 40 percent for the news media.” 22 That demonstrates how much these developments have hurt public discourse.
The risks of regulation
Government harassment of journalists is a serious problem in many parts of the world. United Nations Human Rights Council Special Rapporteur David Kaye notes that “all too many leaders see journalism as the enemy, reporters as rogue actors, tweeps as terrorists, and bloggers as blasphemers.” 23 In Freedom House’s most recent report on global press freedoms, researchers found that media freedom was at its lowest point in 13 years and there were “unprecedented threats to journalists and media outlets in major democracies and new moves by authoritarian states to control the media, including beyond their borders.” 24
Journalists can often be accused of generating fake news and there have been numerous cases of legitimate journalists being arrested or their work being subject to official scrutiny. In Egypt, an Al-Jazeera producer was arrested on charges of “incitement against state institutions and broadcasting fake news with the aim of spreading chaos.” 25 This was after the network broadcast a documentary criticizing Egyptian military conscription.
Some governments have also moved to create government regulations to control information flows and censor content on social media platforms. Indonesia has established a government agency to “monitor news circulating online” and “tackle fake news.” 26 In the Philippines, Senator Joel Villanueva has introduced a bill that would impose up to a five-year prison term for those who publish or distribute “fake news,” which the legislation defined as activities that “cause panic, division, chaos, violence, and hate, or those which exhibit a propaganda to blacken or discredit one’s reputation.” 27
Critics have condemned the bill’s definition of social networks, misinformation, hate speech, and illegal speech as too broad, and believe that it risks criminalizing investigative journalism and limiting freedom of expression. Newspaper columnist Jarius Bondoc noted “the bill is prone to abuse. A bigot administration can apply it to suppress the opposition. By prosecuting critics as news fakers, the government can stifle legitimate dissent. Whistleblowers, not the grafters, would be imprisoned and fined for daring to talk. Investigative journalists would cram the jails.” 28
In a situation of false information, it is tempting for legal authorities to deal with offensive content and false news by forbidding or regulating it. For example, in Germany, legislation was passed in June 2017 that forces digital platforms to delete hate speech and misinformation. It requires large social media companies to “delete illegal, racist or slanderous comments and posts within 24 hours.” Companies can be fined up to $57 million for content that is not deleted from the platform, such as Nazi symbols, Holocaust denials, or language classified as hate speech. 29
The German legislation’s critics have complained that its definition of “obviously” illegal speech risks censorship and a loss of freedom of speech. As an illustration, the law applies the rules to social media platforms in the country with more than 2 million users. Commentators have noted that is not a reasonable way to define relevant social networks. There could be much smaller networks that inflict greater social damage.
Overly restrictive regulation of internet platforms in open societies sets a dangerous precedent and can encourage authoritarian regimes to continue and/or expand censorship.
In addition, it is not always clear how to identify objectionable content. 30 While it is pretty clear how to define speech advocating violence or harm to other people, it is less apparent when talking about hate speech or “defamation of the state.” What is considered “hateful” to one individual may not be to someone else. There is some ambiguity regarding what constitutes hate speech in a digital context. Does it include mistakes in reporting, opinion piece commentary, political satire, leader misstatements, or outright fabrications? Watchdog organizations complained that “overly broad language could affect a range of platforms and services and put decisions about what is illegal content into the hands of private companies that may be inclined to over-censor in order to avoid potential fines.” 31
Overly restrictive regulation of internet platforms in open societies sets a dangerous precedent and can encourage authoritarian regimes to continue and/or expand censorship. This will restrict global freedom of expression and generate hostility to democratic governance. Democracies that place undue limits on speech risk legitimizing authoritarian leaders and their efforts to crackdown basic human rights. It is crucial that efforts to improve news quality not weaken journalistic content or the investigative landscape facing reporters.
Other approaches
There are several alternatives to deal with falsehoods and disinformation that can be undertaken by various organizations. Many of these ideas represent solutions that combat fake news and disinformation without endangering freedom of expression and investigative journalism.
Government responsibilities
1) One of the most important thing governments around the world can do is to encourage independent, professional journalism . The general public needs reporters who help them make sense of complicated developments and deal with the ever-changing nature of social, economic, and political events. Many areas are going through transformation that I elsewhere have called “megachanges,” and these shifts have created enormous anger, anxiety, and confusion. 32 In a time of considerable turmoil, it is vital to have a healthy Fourth Estate that is independent of public authorities.
2) Governments should avoid crackdowns on the news media’s ability to cover the news. Those activities limit freedom of expression and hamper the ability of journalists to cover political developments. The United States should set a good example with other countries. If American leaders censor or restrict the news media, it encourages other countries to do the same thing.
3) Governments should avoid censoring content and making online platforms liable for misinformation. This could curb free expression, making people hesitant to share their political opinions for fear it could be censored as fake news. Such overly restrictive regulation could set a dangerous precedent and inadvertently encourage authoritarian regimes to weaken freedom of expression.
News industry actions
1) The news industry should continue to focus on high-quality journalism that builds trust and attracts greater audiences. An encouraging development is that many news organizations have experienced major gains in readership and viewership over the last couple of years, and this helps to put major news outlets on a better financial footing. But there have been precipitous drops in public confidence in the news media in recent years, and this has damaged the ability of journalists to report the news and hold leaders accountable. During a time of considerable chaos and disorder, the world needs a strong and viable news media that informs citizens about current events and long-term trends.
2) It is important for news organizations to call out fake news and disinformation without legitimizing them. They can do this by relying upon their in-house professionals and well-respected fact-checkers. In order to educate users about news sites that are created to mislead, nonprofit organizations such as Politifact, Factcheck.org, and Snopes judge the accuracy of leader claims and write stories detailing the truth or lack thereof of particular developments. These sources have become a visible part of election campaigns and candidate assessment in the United States and elsewhere. Research by Dartmouth College Professor Brendan Nyhan has found that labeling a Facebook post as “disputed” reduces the percentage of readers believing the false news by 10 percentage points. 33 In addition, Melissa Zimdars, a communication and media professor at Merrimack College, has created a list of 140 websites that use “distorted headlines and decontextualized or dubious information.” 34 This helps people track promulgators of false news.
It is important for news organizations to call out fake news and disinformation without legitimizing them.
Similar efforts are underway in other countries. In Ukraine, an organization known as StopFake relies upon “peer-to-peer counter propaganda” to dispel false stories. Its researchers assess “news stories for signs of falsified evidence, such as manipulated or misrepresented images and quotes” as well as looking for evidence of systematic misinformation campaigns. Over the past few years, it has found Russian social media posts alleging that Ukrainian military forces were engaging in atrocities against Russian nationalists living in eastern Ukraine or that they had swastikas painted on their vehicles. 35 In a related vein, the French news outlet Le Monde has a “database of more than 600 news sites that have been identified and tagged as ‘satire,’ ‘real,’ [or] ‘fake.’” 36
Crowdsourcing draws on the expertise of large numbers of readers or viewers to discern possible problems in news coverage, and it can be an effective way to deal with fake news. One example is The Guardian’s effort to draw on the wisdom of the crowd to assess 450,000 documents about Parliament member expenses in the United Kingdom. It received the documents but lacked the personnel quickly to analyze their newsworthiness. To deal with this situation, the newspaper created a public website that allowed ordinary people to read each document and designate it into one of four news categories: 1) “not interesting,” 2) “interesting but known,” 3) “interesting,” or 4) “investigate this.” 37 Digital platforms allow news organizations to engage large numbers of readers this way. The Guardian, for example, was able “to attract 20,000 readers to review 170,000 documents in the first 80 hours.” [38] These individuals helped the newspaper to assess which documents were most problematic and therefore worthy of further investigation and ultimately news coverage.
Technology company responsibilities
1) Technology firms should invest in technology to find fake news and identify it for users through algorithms and crowdsourcing . There are innovations in fake news and hoax detection that are useful to media platforms. For example, fake news detection can be automated, and social media companies should invest in their ability to do so. Former FCC Commissioner Tom Wheeler argues that “public interest algorithms” can aid in identifying and publicizing fake news posts and therefore be a valuable tool to protect consumers. 38
In this vein, computer scientist William Yang Wang, relying upon PolitiFact.com, created a public database of 12,836 statements labeled for accuracy and developed an algorithm that compared “surface-level linguistic patterns” from false assertions to wording contained in digital news stories. This allowed him to integrate text and analysis, and identify stories that rely on false information. His conclusion is that “when combining meta-data with text, significant improvements can be achieved for fine-grained fake news detection.” 39 In a similar approach, Eugenio Tacchini and colleagues say it is possible to identify hoaxes with a high degree of accuracy. Testing this proposition with a database of 15,500 Facebook posts and over 909,000 users, they find an accuracy rate of over 99 percent and say outside organizations can use their automatic tool to pinpoint sites engaging in fake news. 40 They use this result to advocate the development of automatic hoax detection systems.
Algorithms are powerful vehicles in the digital era and help shape people’s quest for information and how they find online material. They can also help with automatic hoax detection, and there are ways to identify fake news to educate readers without censoring it. According to Kelly Born of the William and Flora Hewlett Foundation, digital platforms should down rank or flag dubious stories, and find a way to better identify and rank authentic content to improve information-gathering and presentation. 41 As an example, several media platforms have instituted “disputed news” tags that warn readers and viewers about contentious content. This could be anything from information that is outright false to material where major parties disagree about its factualness. It is a way to warn readers about possible inaccuracies in online information. Wikipedia is another platform that does this. Since it publishes crowdsourced material, it is subject to competing claims regarding factual accuracy. It deals with this problem by adding tags to material identifying it as “disputed news.”
Yet this cannot be relied on by itself. A survey of 7,500 individuals undertaken by David Rand and Gordon Pennycook of Yale University argue that alerting readers about inaccurate information doesn’t help much. They explored the impact of independent fact-checkers and claim that “the existence of ‘disputed’ tags made participants just 3.7 percentage points more likely to correctly judge headlines as false.” 42 The authors worry that the outpouring of false news overwhelms fact-checkers and makes it impossible to evaluate disinformation.
Algorithms are powerful vehicles in the digital era, and they can help establish automatic hoax detection systems.
2) These companies shouldn’t make money from fake news manufacturers and should make it hard to monetize hoaxes . It is important to weaken financial incentives for bad content, especially false news and disinformation, as the manufacturing of fake news is often financially motivated. Like all clickbait, false information can be profitable due to ad revenues or general brand-building. Indeed, during the 2016 presidential campaign, trolls in countries such as Macedonia reported making a lot of money through their dissemination of erroneous material. While social media platforms like Facebook have made it harder for users to profit from fake news, 43 ad networks can do much more to stop the monetization of fake news, and publishers can stop carrying the ad networks that refuse to do so.
3) Strengthen online accountability through stronger real-name policies and enforcement against fake accounts. Firms can do this through “real-name registration,” which is the requirement that internet users have to provide the hosting platform with their true identity. This makes it easier to hold individuals accountable for what they post or disseminate online and also stops people from hiding behind fake names when they make offensive comments or engage in prohibited activities. 44 This is relevant to fake news and misinformation because of the likelihood that people will engage in worse behavior if they believe their actions are anonymous and not likely to be made public. As famed Justice Louis Brandeis long ago observed, “sunshine is said to be the best of disinfectants.” 45 It helps to keep people honest and accountable for their public activities.
Educational institutions
1) Funding efforts to enhance news literacy should be a high priority for governments. This is especially the case with people who are going online for the first time. For those individuals, it is hard to distinguish false from real news, and they need to learn how to evaluate news sources, not accept at face value everything they see on social media or digital news sites. Helping people become better consumers of online information is crucial as the world moves towards digital immersion. There should be money to support partnerships between journalists, businesses, educational institutions, and nonprofit organizations to encourage news literacy.
2) Education is especially important for young people . Research by Joseph Kahne and Benjamin Bowyer found that third-party assessments matter to young readers. However, their effects are limited. Those statements judged to be inaccurate reduced reader persuasion, although to a lower extent than alignment with the individual’s prior policy beliefs. 46 If the person already agreed with the statement, it was more difficult for fact-checking to sway them against the information.
How the public can protect itself
1) Individuals can protect themselves from false news and disinformation by following a diversity of people and perspectives . Relying upon a small number of like-minded news sources limits the range of material available to people and increases the odds they may fall victim to hoaxes or false rumors. This method is not entirely fool-proof, but it increases the odds of hearing well-balanced and diverse viewpoints.
2) In the online world, readers and viewers should be skeptical about news sources . In the rush to encourage clicks, many online outlets resort to misleading or sensationalized headlines. They emphasize the provocative or the attention-grabbing, even if that news hook is deceptive. News consumers have to keep their guard up and understand that not everything they read is accurate and many digital sites specialize in false news. Learning how to judge news sites and protect oneself from inaccurate information is a high priority in the digital age.
From this analysis, it is clear there are a number of ways to promote timely, accurate, and civil discourse in the face of false news and disinformation. 47 In today’s world, there is considerable experimentation taking place with online news platforms. News organizations are testing products and services that help them identify hate speech and language that incites violence. There is a major flowering of new models and approaches that bodes well for the future of online journalism and media consumption.
At the same time, everyone has a responsibility to combat the scourge of fake news and disinformation. This ranges from the promotion of strong norms on professional journalism, supporting investigative journalism, reducing financial incentives for fake news, and improving digital literacy among the general public. Taken together, these steps would further quality discourse and weaken the environment that has propelled disinformation around the globe.
Note: I wish to thank Hillary Schaub and Quinn Bornstein for their valuable research assistance. They were very helpful in finding useful materials for this project.
The Brookings Institution is a nonprofit organization devoted to independent research and policy solutions. Its mission is to conduct high-quality, independent research and, based on that research, to provide innovative, practical recommendations for policymakers and the public. The conclusions and recommendations of any Brookings publication are solely those of its author(s), and do not reflect the views of the Institution, its management, or its other scholars.
Support for this publication was generously provided by Facebook. Brookings recognizes that the value it provides is in its absolute commitment to quality, independence, and impact. Activities supported by its donors reflect this commitment.
- Pew Research Center, “Digital News Fact Sheet,” August 7, 2017.
- Pew Research Center, “How Americans Encounter, Recall, and Act Upon Digital News,” February 9, 2017.
- Pew Research Center, “More Than Half of Smartphone Users Get News Alerts, But Few Get Them Often,” September 8, 2016.
- Nic Newman, “Digital News Sources,” Reuters Institute for the Study of Journalism, 2017.
- Jacob Poushter, “Smartphone Ownership and Internet Usage Continues to Climb in Emerging Economies,” Pew Research Center, February 22, 2016.
- Gallup Poll, “Americans’ Trust in Mass Media Sinks to New Low,” September 14, 2016.
- Gallup Poll, “Republicans’, Democrats’ Views of Media Accuracy Diverge,” August 25, 2017.
- Jen Weedon, William Nuland, and Alex Stamos, “Information Operations,” Facebook, April 27, 2017.
- Craig Silverman, “This Analysis Shows How Viral Fake Election News Stories Outperformed Real News on Facebook,” BuzzFeedNews , November 16, 2016.
- Craig Timberg and Elizabeth Dwoskin, “Russian Content on Facebook, Google and Twitter Reached Far More Users Than Companies First Disclosed, Congressional Testimony Says,” Washington Post , October 30, 2017.
- Tim Wu, “Did Twitter Kill the First Amendment?”, New York Times , October 28, 2017, p. !a9.
- Marc Fisher, John Cox, and Peter Hermann, “Pizzagate: From Rumor, to Hashtag, to Gunfire in D.C.,” Washington Post , December 6, 2016.
- Craig Silverman and Jeremy Singer-Vine, “Most Americans Who See Fake News Believe It, New Survey Says,” BuzzFeed News , December 6, 2016.
- Hunt Allcott and Matthew Gentzkow, “Social Media and Fake News in the 2016 Election,” NBER Working Paper, April, 2017, p. 4.
- Vidhi Doshi, “India’s Millions of New Internet Users are Falling for Fake News – Sometimes with Deadly Consequences,” Washington Post , October 1, 2017.
- Emilio Ferrara, Onur Varol, Clayton Davis, Filippo Menczer, and Alessandro Flammini, “The Rise of Social Bots,” Communications of the ACM , July, 2016.
- Michela Del Vicario, Alessandro Bessi, Fabiana Zollo, Fabio Petroni, Antonio Scala, Guido Caldarelli, Eugene Stanley, and Walter Quattrociocchi, “The Spreading of Misinformation Online,” PNAS , January 19, 2016.
- David Lazer, Matthew Baum, Nir Grinberg, Lisa Friedland, Kenneth Joseph, Will Hobbs, and Carolina Mattsson, “Combating Fake News: An Agenda for Research and Action,” Harvard Shorenstein Center on Media, Politics and Public Policy and Harvard Ash Center for Democratic Governance and Innovation, May, 2017, p. 5.
- Office of the United Nations High Commissioner for Human Rights, “UN Expert Urges Governments to End ‘Demonization’ of Critical Media and Protect Journalists,” May 3, 2017.
- Freedom House, “Press Freedom’s Dark Horizon,” 2017.
- Committee to Protect Journalists, “Egypt Arrests Al-Jazeera Producer on Fake News Charge,” December 27, 2016.
- Straits Times , “Indonesia to Set Up Agency to Combat Fake News,” January 6, 2017.
- Mong Palatino, “Philippine Senator Moves to Criminalize ‘Fake News’ – Could This Lead to Censorship?”, Global Voices , July 7, 2017.
- Melissa Eddy and Mark Scott, “Delete Hate Speech or Pay Up, Germany Tells Social Media Companies,” New York Times , June 30, 2017.
- European Digital Rights, “Recommendations on the German Bill ‘Improving Law Enforcement on Social Networks’”, June 20, 2017.
- Courtney Radsch, “Proposed German Legislation Threatens Broad Internet Censorship,” Committee to Protect Journalists, April 20, 2017.
- Darrell M. West, Megachange: Economic Disruption, Political Upheaval, and Social Strife in the 21 st Century , Brookings Institution Press, 2016.
- Brendan Nyhan, “Why the Fact-Checking at Facebook Needs to Be Checked,” New York Times , October 23, 2017.
- Kelly Born, “The Future of Truth: Can Philanthropy Help Mitigate Misinformation?”, William and Flora Hewlett Foundation, June 8, 2017 and Ananya Bhattacharya, “Here’s a Handy Cheat Sheet of False and Misleading ‘News’ Sites,” Quartz , November 17, 2016.
- Maria Haigh, Thomas Haigh, and Nadine Kozak, “Stopping Fake News: The Work Practices of Peer-to-Peer Counter Propaganda,” Journalist Studies , March 31, 2017.
- Kelly Born, “The Future of Truth: Can Philanthropy Help Mitigate Misinformation?”, William and Flora Hewlett Foundation, June 8, 2017.
- Reinhard Handler and Raul Conill, “Open Data, Crowdsouring and Game Mechanics: A Case Study on Civic Participation in the Digital Age,” Computer Supported Cooperative Work , 2016.
- Tom Wheeler, “Using ‘Public Interest Algorithms’ to Tackle the Problems Created by Social Media Algorithms,” Brookings TechTank, November 1, 2017.
- William Yang Wang, “’Liar, Liar Pants on Fire’, A New Benchmark Dataset for Fake News Detection”, Computation and Language , May, 2017.
- Eugenio Tacchini, Gabriele Ballarin, Marco Della Vedova, Stefano Moret, and Luca de Alfaro, “Some Like It Hoax: Automated Fake News Detection in Social Networks, Human-Computer Interaction , April 25, 2017.
- Jason Schwartz, “Study: Tagging Fake News on Facebook Doesn’t Work,” Politico , September 13, 2017, p. 19.
- Mike Isaac, “Facebook Mounts Effort to Limit Tide of Fake News,” New York Times , December 15, 2016.
- Zhixiong Liao, “An Economic Analysis on Internet Regulation in China and Proposals to Policy and Law Makers,” International Journal of Technology Policy and Law , 2016.
- Brainy Quote , “Louis Brandeis,” undated.
- Joseph Kahne and Benjamin Bowyer, “Educating for Democracy in a Partisan Age: Confronting the Challenges of Motivated Reasoning and Misinformation,” American Educational Research Journal , February, 2017.
- Darrell M. West and Beth Stone, “Nudging News Producers and Consumers Toward More Thoughtful, Less Polarized Discourse,” Brookings Institution Center for Effective Public Management, February, 2014.
Internet & Telecommunications Media & Journalism Social Media
Governance Studies
Center for Technology Innovation
Darrell M. West, Roxana Muenster
August 5, 2024
Tom Wheeler
June 24, 2024
Daniel S. Schiff, Kaylyn Jackson Schiff, Natália Bueno
May 30, 2024
Essay on Political Misinformation
Political misinformation refers to the sharing of false information without the intent of causing harm. Since information and technology have become critical in the lives of individuals nowadays, misinformation has a significant impact. People spend substantial amounts of time online chatting and engaging in various forms of communication. The world currently relies on the information circulated online for fact-finding and general information purposes. Therefore, any untrue information shared through the various media with an online presence can be interpreted differently and in ways that bring harm. Political misinformation greatly influences political and public opinions, contributes to polarization and even affects democracy.
Misinformation affects political and public opinions on important issues. Political knowledge is a key element of representative democracy, and it is dangerous when people hold incorrect information confidently. For example, misinformed people will have erroneous beliefs about the welfare policy (Jerit & Zhao, 2020). If a large number of the population shares the misinformation, these beliefs affect their collective opinion. A worrying scenario is where misinformed people base their political choices on incorrect information. During political times, individuals might provide misinformed ideas to researchers that may not reflect the true state of events on the ground. An example of falsehood that had greater implications was the post by Sara Palin that suggested that the Affordable Care Act would bring forth “death panels.” Even though the claim was later discredited, it had caused serious impacts and had been highlighted in over 700 mainstream news articles (Watts et al., 2021). The general political and public opinions reported through the media after research may not reflect the actual standing of the people (Schaffner & Luks 2018). The loyalties of the people might change if the correct information is availed.
Misinformation can also lead to polarization in a country. The Trump administration was driven by the conviction that the U.S. has not been treated as it should over the years on the global platform. The rhetoric was that the nation had experienced a decline in its international standing under former president Barack Obama. The majority of the people, however, thought that the nation was still strong on matters of global politics. The political agenda drafted by Trump to exercise its power and return to its true position almost led to a confrontation with Iran (Islami, 2021). The administration, in this case, was promoting its political agenda by insisting that the U.S. needed to capture its former prestige. With the continued support of the same information through various communications, people who held a different opinion found themselves supporting the government. However, the difference in opinions was still present.
Misinformation can affect the existence of democracies and lead to wars. Watts et al. (2021) argue that when falsehoods are promulgated through the mainstream media, they can cause serious consequences. A good example is the Iraq war in 2003, where many media stations were perpetuating the false claims that the Saddam Hussein regime had weapons of mass destruction (Watts et al., 2021). This example shows the power of misinformation in fueling wars. In 2016, the presidential elections were marred with misinformation, and the mainstream media contributed to magnify its effects. Misinformation can lead to distrust in the public space and incitements that cause war. When the public is continuously misinformed for political reasons, sentiments of hate might develop that can incite the destabilization of democracies.
Misinformation has serious repercussions and the ability to affect the public and political opinions of the people. Also, it can lead to polarization in a country where the political rhetoric differs from the general public opinions. In worst scenarios, misinformation, when highly propagated through the mainstream media, has the power to cause wars. A good example, in this case, is the Iraq war, where the public was convinced that Iraq had weapons of mass destruction. The war led to serious devastations that could have been avoided with the right information.
Islami, M. (2021). Turning the Tide: The Imperatives for Rescuing the Iran Nuclear Deal. Iranian Review of Foreign Affairs, Vol. 12, No. 1, 83-104. Retrieved April 09, 2022, from http://irfajournal.csr.ir/article_145356_2630fa336a40c634aacee11b8ecd4f8c.pdf
Jerit, J., & Zhao, Y. (2020). Political misinformation. Annual Review of Political Science , 23 , 77-94. Retrieved April 09, 2022, from https://www.annualreviews.org/doi/abs/10.1146/annurev-polisci-050718-032814
Schaffner, B. F., & Luks, S. (2018). Misinformation or expressive responding? What an inauguration crowd can tell us about the source of political misinformation in surveys. Public Opinion Quarterly , 82 (1), 135-147. Retrieved April 09, 2022, from https://academic.oup.com/poq/article-abstract/82/1/135/4868126
Watts, D. J., Rothschild, D. M., & Mobius, M. (2021). Measuring the news and its impact on democracy. Proceedings of the National Academy of Sciences , 118 (15). Retrieved April 09, 2022, from https://www.pnas.org/doi/10.1073/pnas.1912443118
Cite This Work
To export a reference to this article please select a referencing style below:
Related Essays
How music impacts imagination, navigating the academic seas: crafting a high-impact track record for future opportunities, feeling bad on facebook: depression disclosures by college students on a social networking site, “why don’t students like school”, balanced curriculum for holistic development, essay on humanistic counseling approach, popular essay topics.
- American Dream
- Artificial Intelligence
- Black Lives Matter
- Bullying Essay
- Career Goals Essay
- Causes of the Civil War
- Child Abusing
- Civil Rights Movement
- Community Service
- Cultural Identity
- Cyber Bullying
- Death Penalty
- Depression Essay
- Domestic Violence
- Freedom of Speech
- Global Warming
- Gun Control
- Human Trafficking
- I Believe Essay
- Immigration
- Importance of Education
- Israel and Palestine Conflict
- Leadership Essay
- Legalizing Marijuanas
- Mental Health
- National Honor Society
- Police Brutality
- Pollution Essay
- Racism Essay
- Romeo and Juliet
- Same Sex Marriages
- Social Media
- The Great Gatsby
- The Yellow Wallpaper
- Time Management
- To Kill a Mockingbird
- Violent Video Games
- What Makes You Unique
- Why I Want to Be a Nurse
- Send us an e-mail
What are you looking for?
The researchers sought to understand how the reward structure of social media sites drives users to develop habits of posting misinformation on social media. (Photo/AdobeStock)
USC study reveals the key reason why fake news spreads on social media
The USC-led study of more than 2,400 Facebook users suggests that platforms — more than individual users — have a larger role to play in stopping the spread of misinformation online.
USC researchers may have found the biggest influencer in the spread of fake news: social platforms’ structure of rewarding users for habitually sharing information.
The team’s findings, published Monday by Proceedings of the National Academy of Sciences , upend popular misconceptions that misinformation spreads because users lack the critical thinking skills necessary for discerning truth from falsehood or because their strong political beliefs skew their judgment.
Just 15% of the most habitual news sharers in the research were responsible for spreading about 30% to 40% of the fake news.
The research team from the USC Marshall School of Business and the USC Dornsife College of Letters, Arts and Sciences wondered: What motivates these users? As it turns out, much like any video game, social media has a rewards system that encourages users to stay on their accounts and keep posting and sharing. Users who post and share frequently, especially sensational, eye-catching information, are likely to attract attention.
“Due to the reward-based learning systems on social media, users form habits of sharing information that gets recognition from others,” the researchers wrote. “Once habits form, information sharing is automatically activated by cues on the platform without users considering critical response outcomes, such as spreading misinformation.”
Posting, sharing and engaging with others on social media can, therefore, become a habit.
“[Misinformation is] really a function of the structure of the social media sites themselves.” — Wendy Wood , USC expert on habits
“Our findings show that misinformation isn’t spread through a deficit of users. It’s really a function of the structure of the social media sites themselves,” said Wendy Wood , an expert on habits and USC emerita Provost Professor of psychology and business.
“The habits of social media users are a bigger driver of misinformation spread than individual attributes. We know from prior research that some people don’t process information critically, and others form opinions based on political biases, which also affects their ability to recognize false stories online,” said Gizem Ceylan, who led the study during her doctorate at USC Marshall and is now a postdoctoral researcher at the Yale School of Management . “However, we show that the reward structure of social media platforms plays a bigger role when it comes to misinformation spread.”
In a novel approach, Ceylan and her co-authors sought to understand how the reward structure of social media sites drives users to develop habits of posting misinformation on social media.
Why fake news spreads: behind the social network
Overall, the study involved 2,476 active Facebook users ranging in age from 18 to 89 who volunteered in response to online advertising to participate. They were compensated to complete a “decision-making” survey approximately seven minutes long.
Surprisingly, the researchers found that users’ social media habits doubled and, in some cases, tripled the amount of fake news they shared. Their habits were more influential in sharing fake news than other factors, including political beliefs and lack of critical reasoning.
Frequent, habitual users forwarded six times more fake news than occasional or new users.
“This type of behavior has been rewarded in the past by algorithms that prioritize engagement when selecting which posts users see in their news feed, and by the structure and design of the sites themselves,” said second author Ian A. Anderson , a behavioral scientist and doctoral candidate at USC Dornsife. “Understanding the dynamics behind misinformation spread is important given its political, health and social consequences.”
Experimenting with different scenarios to see why fake news spreads
In the first experiment, the researchers found that habitual users of social media share both true and fake news.
In another experiment, the researchers found that habitual sharing of misinformation is part of a broader pattern of insensitivity to the information being shared. In fact, habitual users shared politically discordant news — news that challenged their political beliefs — as much as concordant news that they endorsed.
Lastly, the team tested whether social media reward structures could be devised to promote sharing of true over false information. They showed that incentives for accuracy rather than popularity (as is currently the case on social media sites) doubled the amount of accurate news that users share on social platforms.
The study’s conclusions:
- Habitual sharing of misinformation is not inevitable.
- Users could be incentivized to build sharing habits that make them more sensitive to sharing truthful content.
- Effectively reducing misinformation would require restructuring the online environments that promote and support its sharing.
These findings suggest that social media platforms can take a more active step than moderating what information is posted and instead pursue structural changes in their reward structure to limit the spread of misinformation.
About the study: The research was supported and funded by the USC Dornsife College of Letters, Arts and Sciences Department of Psychology, the USC Marshall School of Business and the Yale University School of Management.
Related Articles
Keeping native bees buzzing requires rethinking pest control, physicist’s new diagnostic tools may help make cancer a disease of the past, from lab to sea: inside usc’s carbon capture research.
Thank you for visiting nature.com. You are using a browser version with limited support for CSS. To obtain the best experience, we recommend you use a more up to date browser (or turn off compatibility mode in Internet Explorer). In the meantime, to ensure continued support, we are displaying the site without styles and JavaScript.
- View all journals
- Explore content
- About the journal
- Publish with us
- Sign up for alerts
- Review Article
- Published: 10 March 2022
Misinformation: susceptibility, spread, and interventions to immunize the public
- Sander van der Linden ORCID: orcid.org/0000-0002-0269-1744 1
Nature Medicine volume 28 , pages 460–467 ( 2022 ) Cite this article
66k Accesses
205 Citations
512 Altmetric
Metrics details
- Communication
The spread of misinformation poses a considerable threat to public health and the successful management of a global pandemic. For example, studies find that exposure to misinformation can undermine vaccination uptake and compliance with public-health guidelines. As research on the science of misinformation is rapidly emerging, this conceptual Review summarizes what we know along three key dimensions of the infodemic: susceptibility, spread, and immunization. Extant research is evaluated on the questions of why (some) people are (more) susceptible to misinformation, how misinformation spreads in online social networks, and which interventions can help to boost psychological immunity to misinformation. Implications for managing the infodemic are discussed.
Similar content being viewed by others
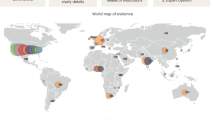
Toolbox of individual-level interventions against online misinformation
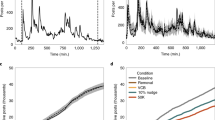
Combining interventions to reduce the spread of viral misinformation
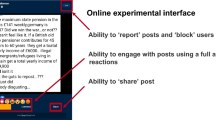
Psychological inoculation protects against the social media infodemic
In early 2020, the World Health Organization (WHO) declared a worldwide ‘infodemic’. An infodemic is characterized by an overabundance of information, particularly false and misleading information 1 . Although researchers have debated the effect of fake news on the outcomes of major societal events, such as political elections 2 , 3 , the spread of misinformation has much clearer potential to cause direct and notable harm to public health, especially during a pandemic. For example, research across different countries has shown that the endorsement of COVID-19 misinformation is robustly associated with people being less likely to follow public-health guidance 4 , 5 , 6 , 7 and having reduced intentions to get vaccinated 4 , 5 and to recommend the vaccine to others 4 . Experimental evidence has found that exposure to misinformation about vaccination resulted in about a 6-percentage-point decrease in the intention to get vaccinated among those who said that they would otherwise “definitely accept a vaccine”, undermining the potential for herd immunity 8 . Analyses of social-network data estimate that, without intervention, anti-vaccination content on social platforms such as Facebook will dominate discourse in the next decade 9 . Other research finds that exposure to misinformation about COVID-19 has been linked to the ingestion of harmful substances 10 and an increased propensity to engage in violent behaviors 11 . Of course, misinformation was a threat to public health long before the pandemic. The debunked link between the MMR vaccine and autism was associated with a significant drop in vaccination coverage in the United Kingdom 12 , Listerine manufacturers falsely claimed that their mouthwash cured the common cold for many decades 13 , misinformation about tobacco products has influenced attitudes toward smoking 14 and, in 2014, Ebola clinics were attacked in Liberia because of the false belief that the virus was part of a government conspiracy 15 .
Given the unprecedented scale and pace at which misinformation can now travel online, research has increasingly relied on models from epidemiology to understand the spread of fake news 16 , 17 , 18 . In these models, the key focus is on the reproduction number ( R 0 )—in other words, the number of individuals who will start posting fake news (that is, secondary cases) following contact with someone who is already posting misinformation (the infectious individual). It is therefore helpful to think of misinformation as a viral pathogen that can infect its host, spreading rapidly from one individual to another within a given network, without the need for physical contact. One benefit of this epidemiological approach lies in the fact that early detection systems could be designed to identify, for example, superspreaders, which would allow for the timely deployment of interventions to curb the spread of viral misinformation 18 .
This Review will provide readers with a conceptual overview of recent literature on misinformation, along with a research agenda (Box 1 ) that covers three major theoretical dimensions aligned with the viral analogy: susceptibility, spread, and immunization. What makes individuals susceptible to misinformation in the first place? Why and how does it spread? And what can we do to boost public immunity?
Before reviewing the extant literature to help answer these questions, it is worth briefly discussing what the term ‘misinformation’ means, because inconsistent definitions affect not only the conceptualization of research designs but also the nature and validity of key outcome measures 19 . Indeed, misinformation has been referred to as an ‘umbrella category of symptoms’ 20 not only because definitions vary, but also because the behavioral consequences for public health might differ depending on the type of misinformation. The term ‘fake news’ is often especially regarded as problematic because it insufficiently describes the full spectrum of misinformation 21 and has become a politicized rhetorical device in itself 22 . Box 2 provides a more detailed discussion of the problems associated with different scholarly definitions of misinformation 23 but for the purpose of this Review, I will simply define misinformation in its broadest possible sense: ‘false or misleading information masquerading as legitimate news,’ regardless of intent 24 . Although disinformation is often differentiated from misinformation insofar as it involves a clear intention to deceive or harm other people, intent can be difficult to establish, so in this Review my treatment of misinformation will cover both intentional and unintentional forms of misinformation.
Box 1 Agenda and recommendations for future research
Research question 1: What factors make people susceptible to misinformation?
Better integrate accuracy-driven with social, political, and cultural motivations to explain people’s susceptibility to misinformation.
Define, develop, and validate standardized instruments for assessing general and domain-specific susceptibility to misinformation.
Research question 2: How does misinformation spread in social networks?
Outline with greater clarity the conditions under which ‘exposure’ is more or less likely to lead to ‘infection,’ including the impact of repeated exposure, the micro-targeting of fake news on social media, contact with superspreaders, the role of echo chambers, and the structure of the social network itself.
Provide more accurate population-level estimates of exposure to misinformation by (1) capturing more diverse types of misinformation and (2) linking exposure to fake news across different kinds of traditional and social-media platforms.
Research question 3: Can we inoculate or immunize people against misinformation?
Focus on evaluating the relative efficacy of different debunking methods in the field, as well as how debunking (therapeutic) and prebunking (prophylactic) interventions could be combined to maximize their protective properties.
Model and evaluate how psychological inoculation methods can spread online and influence real-world sharing behavior on social media.
Box 2 The challenges with defining and operationalizing misinformation
One of the most frequently cited definitions of fake news is “fabricated information that mimics news media content in form but not in organizational process or intent” 119 . This definition implies that what matters in determining whether a story is misinformation or not is the journalistic or editorial process. Other definitions echo similar sentiments insofar the view is taken that misinformation producers do not adhere to editorial norms 120 and that the defining attribution of ‘fake-ness’ happens at the level of the publisher and not at the level of the story 3 . However, others have taken a completely different view by defining misinformation either in terms of the veracity of its content or the presence or absence of common techniques used to produce it 109 .
It could be argued that some definitions are overly narrow because news stories do not need to be completely false in order to be misleading. A highly salient example comes from the Chicago Tribune , a generally credible outlet, which re-published a story in January 2021 with the headline “A healthy doctor died two weeks after getting a COVID-19 vaccine”. This story would not be classified as false on the basis of the source or even the content, as the events were true when considered in isolation. However, it is highly misleading—even considered unethical—to suggest that the doctor died specifically because of the COVID-19 vaccine when there was no evidence to make such a causal connection at the time of publication. This is not an obscure example: it was viewed over 50 million times on Facebook in early 2021 (ref. 121 ).
Another potential challenge with purely content-based definitions is that when expert consensus on a public-health topic is rapidly emerging and subject to uncertainty and change, the definition of what is likely to be true and false can shift over time, making overly simplistic ‘real’ versus ‘fake’ categorizations a potentially unstable property. For example, although news media initially claimed that ibuprofen could worsen coronavirus symptoms, this claim was later retracted as more evidence became available 122 . The problem is that researchers often ask people how accurate or reliable they find a selective series of true and fake headlines that were either created or selected by the researchers on the basis of different definitions of what constitutes misinformation.
There is also variation in outcome measures; sometimes the relevant outcome measure is misinformation susceptibility, and sometimes it is the difference between fake and real news detection, or so-called ‘truth discernment’. The only existing instrument that uses a psychometrically validated set of headlines is the recent Misinformation Susceptibility Test, a standardized measure of news veracity discernment that is normed to the test population 123 . Overall, this means that hundreds of emerging studies on the topic of misinformation have outcome measures that are non-standardized and not always easily comparable.
Susceptibility
Although people use many cognitive heuristics to make judgments about the veracity of a claim (for example, perceived source credibility) 25 , one particularly prominent finding that helps explain why people are susceptible to misinformation is known as the ‘illusory truth’ effect: repeated claims are more likely be judged as true than non-repeated (or novel) claims 26 . Given the fact that many falsehoods are often repeated by the popular media, politicians, and social-media influencers, the relevance of illusory truth has increased substantially. For example, the conspiracy theory that the coronavirus was bio-engineered in a military laboratory in Wuhan, China, and the false claim that “COVID-19 is no worse than the flu” have been repeated many times in the media 27 . The primary cognitive mechanism responsible for the fact that people are more likely to think that repeated claims are true is known as processing fluency: the more a claim is repeated, the more familiar it becomes and the easier it is to process 28 . In other words, the brain uses fluency as a signal for truth. Importantly, research shows that (1) prior exposure to fake news increases its perceived accuracy 29 ; (2) illusory truth can occur for both plausible and implausible claims 30 ; (3) prior knowledge does not necessarily protect people against illusory truth 31 ; and (4) illusory truth does not appear to be moderated by thinking styles such as analytical versus intuitive reasoning 32 .
Although illusory truth can affect everyone, research has noted that some people are still more susceptible to misinformation than others. For example, some common findings include the observation that older individuals are more susceptible to fake news 33 , 34 , potentially owing to factors such as cognitive decline and greater digital illiteracy 35 , although there are exceptions: in the context of COVID-19, older individuals appear less likely to endorse misinformation 4 . Those with a more extreme and right-wing political orientation have also consistently shown to be more susceptible to misinformation 3 , 4 , 33 , 36 , 37 , even when the misinformation in question is non-political 38 , 39 . Yet, the link between ideology and misinformation susceptibility is not always consistent across different cultures 4 , 37 . Other factors such as greater numeracy skills 4 and cognitive and analytic thinking styles 36 , 40 , 41 have consistently been revealed to have a negative correlation with misinformation susceptibility—although other scholars have identified partisanship as a potential moderating factor 42 , 43 , 44 . In fact, these individual differences have given rise to two competing overarching theoretical explanations 45 , 46 for why people are susceptible to misinformation. The first theory is often referred to as the classical ‘inattention’ account; the second is often dubbed the ‘identity-protective’ or ‘motivated cognition’ account. I will discuss emerging evidence for both theories in turn.
The inattention account
The inattention or ‘classical reasoning’ account argues that people are committed to sharing accurate content but the context of social media simply distracts people from making news-sharing decisions that are based on a preference for accuracy 45 . For example, consider that people are often bombarded with news content online, much of which is emotionally charged and political, which, coupled with the fact that people have limited time and resources to think about the veracity of a piece of news, might significantly interfere with their ability to accurately reflect on such content. The inattention account is based on a ‘classical’ reasoning perspective insofar as it draws on dual-process theories of human cognition, which suggest that people rely on two qualitatively different processes of reasoning 47 . These processes are often referred to as System 1, which is predominantly automatic, associative, and intuitive, and System 2, which is more reflective, analytical, and deliberate. A canonical example is the Cognitive Reflection Test (CRT), which administers a series of puzzles in which the intuitive or first answer that comes to mind is often wrong and thus a correct answer requires people to pause and reflect more carefully. The basic point is that activating more analytical System 2-type reasoning can override erroneous System 1-type intuitions. Evidence for the inattention account comes from the fact that people who score higher on the CRT 36 , 41 , who deliberate more 48 , who have greater numeracy skills 4 , and who have higher knowledge and education 37 , 49 are consistently better able to discern between true and false news—regardless of whether the content is politically congruent 36 . In addition, experimental interventions that ‘prime’ people to think more analytically or consider the accuracy of news content 50 , 51 have been shown to improve the quality of people’s news-sharing decisions and decrease acceptance of conspiracy theories 52 .
The motivated reasoning account
In stark contrast to the inattention account stands the theory of (politically) motivated reasoning 53 , 54 , 55 , which posits that information deficits or lack of reflective reasoning are not the primary driver of susceptibility to misinformation. Motivated reasoning occurs when someone starts out their reasoning process with a pre-determined goal (for example, someone might want to believe that vaccines are unsafe because that belief is shared by their family members), so individuals interpret new (mis)information in service of reaching that goal 53 . The motivated account therefore argues that the types of commitments that people have to their affinity groups is what leads them to selectively endorse media content that reinforces deeply held political, religious, or social identities 56 , 57 . There are several variants of the politically motivated reasoning account, but the basic premise is that people pay attention to not just the accuracy of a piece of news content, but also the goals that such information may serve. For example, a fake news story could be viewed as much more plausible when it happens to offer positive information about someone’s political group, or equally when it offers negative information about a political opponent 42 , 57 , 58 . A more extreme and scientifically contentious version of this model, also known as the ‘motivated numeracy’ 59 account, suggests that more reflective and analytical System 2 reasoning abilities do not help people make more accurate assessments but in fact are frequently hijacked in service of identity-based reasoning. Evidence for this claim comes from the fact that partisans with the highest numeracy and education levels tend to be the most polarized on contested scientific issues, such as climate change 60 or stem-cell research 61 . Experimental work has also shown that when people are asked to make causal inferences about a data problem, such as the benefits of a new skin rash treatment, people with greater numeracy skills performed better when the problem was non-political. By contrast, people became more polarized and less accurate when the same data were presented as results from a new study on gun control 59 . These patterns were more pronounced among those with higher numeracy skills. Other research has found that politically conservative individuals are much more likely to (mistakenly) judge misinformation as true when the information is presented as coming from a conservative source than when that same information is presented as coming from a liberal source, and vice versa for politically liberal individuals—highlighting the key role of politics in truth discernment 62 .
Susceptibility: limitations and future research
It is worth mentioning that both accounts face significant critiques and limitations. For example, independent replications of interventions designed to nudge accuracy have revealed mixed findings 63 , and questions have been raised about the conceptualization of partisan bias in these studies 43 , including the possibility that the intervention effects are moderated by people’s political identities 44 . In turn, the motivated numeracy account has faced several failed and mixed replications 64 , 65 , 66 . For example, one large nationally representative study in the United States showed that, although polarization on global warming was indeed greatest among the highest educated partisans at baseline, this effect was neutralized and even reversed by an experimental intervention that induced accuracy motivations by highlighting the scientific consensus on global warming 66 . These findings have led to the discovery of a much larger confound in the motivated-reasoning literature, in that partisan bias could simply be due to selective exposure rather than motivated reasoning 66 , 67 , 68 . This is so because the role of politics is confounded with people’s prior beliefs 66 . Although people are polarized on many issues, this does not mean that they are unwilling to update their (misinformed) beliefs in line with the evidence. Moreover, people might refuse to update their beliefs not because of a motivation to reject the information (because it is incongruent with their political worldview) but simply because they find the information not credible, either because they discount the source or the veracity of the content itself for what appear to be legitimate reasons to those individuals. This ‘equivalence paradox’ 69 makes it difficult to causally disentangle accuracy from motivation-based preferences.
Future research should therefore not only carefully manipulate people’s motivations in the processing of (mis)information that is politically (dis)concordant, but also offer a more integrated theoretical account of susceptibility to misinformation. For example, it is likely that for political fake news, identity-motivations are going to be more salient; however, for misinformation that tackles non-politicized issues (such as falsehoods about cures for the common cold), knowledge deficits, inattention, or confusion might be more likely to play a role. Of course, it is possible for public-health issues—such as COVID-19—to become politicized relatively quickly, in which case the prominence of motivational goals in driving susceptibility to misinformation might increase. Accuracy and motivational goals are also frequently in conflict. For example, people might understand that a news story is unlikely to be true, but if the misinformation promotes the goals of their social group, they might be more inclined to forgo their desire for accuracy in favor of a motivation to conform with the norms of their community 56 , 57 . In other words, in any given context, the importance people assign to accuracy versus social goals is going to determine how and when they are going to update their beliefs in light of misinformation. There is much to be gained by advancing more contextual theories that focus on the interplay between accuracy and socio-political goals in explaining why people are susceptible to misinformation.
Measuring the infodemic
To return to the viral analogy, researchers have adopted models from epidemiology, such as the susceptible–Infected–recovered (SIR) model, to measure and quantify the spread of misinformation in online social networks 17 , 70 . In this context, R 0 often represents individuals who will start posting fake news following contact with someone who is already ‘infected’. Evidence for the potential of an infodemic is taken when R 0 exceeds 1, which signals the potential for exponential growth (when R 0 is lower than 1, the infodemic will eventually sizzle out) and is evidence pointing to a possible infodemic. Analyses of social-media platforms have shown that all have the potential to drive infodemic-like spread, but some are more capable than others 17 . For example, research on Twitter has found that false news is about 70% more likely to be shared than true news, and it takes true news 6 times longer than false stories to reach 1,500 people 71 . Although fake news can thus spread faster and deeper than true news, it is important to emphasize that these findings are based on a relatively narrow definition of fact-checked news (see Box 2 and ref. 70 ), and more recent research has pointed out that these estimates are likely platform-dependent 72 . Importantly, several studies have now shown that fake news typically represents a small part of people’s overall media diet and that the spread of misinformation on social media is highly skewed so that a small number of accounts are responsible for the majority of the content that is shared and consumed, also known as ‘supersharers’ and ‘superconsumers’ 3 , 24 , 73 . Although much of this work has come from the political domain, very similar findings have been found in the context of the COVID-19 pandemic, during which ‘superspreaders’ on Twitter and Facebook were exerting a majority of the influence on the platform 74 . A major issue is the existence of echo chambers, in which the flow of information is often systematically biased toward like-minded others 72 , 75 , 76 . Although the prevalence of echo chambers is debated 77 , the existence of such polarized clusters has shown to aid the virality of misinformation 75 , 78 , 79 and impede the spread of corrections 76 .
Exposure does not equal infection
Importantly, exposure estimates based on social-media data often do not seem to line up with people’s self-reported experiences. Different polls show that over a third of people self-report frequent, if not daily exposure, to misinformation 80 . Of course, the validity of people’s self-reported experiences can be variable, but it raises questions about the accuracy of exposure estimates, which are often based on limited public data and can be sensitive to model assumptions. Moreover, a crucial factor to consider here is that exposure does not equal persuasion (or ‘infection’). For example, research in the context of COVID-19 headlines shows that people’s judgments of headline veracity had little impact on their sharing intentions 45 . People may thus choose to share misinformation for reasons other than accuracy. For example, one recent study 81 found that people often share content that appears ‘interesting if true’. The study indicated that although people rate fake news as less accurate, they also rate it as ‘more interesting if true’ than real news and are thus willing to share it.
Spread: limitations and future research
More generally, the body of research on ‘spreading’ has faced significant limitations, including critical gaps in knowledge. There is skepticism about the rate at which people exposed to misinformation begin to actually believe it because research on media and persuasion effects has shown that it is difficult to persuade people using traditional advertisements 82 . But existing research has often used contrived laboratory designs that may not sufficiently represent the environment in which people make news-sharing decisions. For example, studies often test one-off exposures to a single message rather than persuasion as a function of repeated exposure to misinformation from diverse social and traditional media sources. Accordingly, we need a better understanding of the frequency and intensity with which exposure to misinformation ultimately leads to persuasion. Most studies also rely on publicly available data that people have shared or clicked on, but people may be exposed and influenced by much more information while scrolling on their social-media feed 45 . Moreover, fake news is often conceptualized as a list of URLs that were fact-checked as true or false, but this type of fake news represents only a small segment of misinformation; people may be much more likely to encounter content that is misleading or manipulative without being overtly false (see Box 2 ). Finally, micro-targeting efforts have significantly enhanced the ability for misinformation producers to identify and target subpopulations of individuals who are most susceptible to persuasion 83 . In short, more research is needed before precise and valid conclusions can be made about either population-level exposure or the probability that exposure to misinformation leads to infection (that is, persuasion).
Immunization
A rapidly emerging body of research has started to evaluate the possibility of ‘immunizing’ the public against misinformation at a cognitive level. I will categorize these efforts by whether their application is primarily prophylactic (preventative) or therapeutic (post-exposure), also known as ‘prebunking’ and ‘debunking,’ respectively.
Therapeutic treatments: fact-checking and debunking
The traditional, standard approach to countering misinformation generally involves the correction of a myth or falsehood after people have already been exposed or persuaded by a piece of misinformation. For example, debunking misinformation about autism interventions has shown to be effective in reducing support for non-empirically supported treatments, such as dieting 84 . Exposure to court-ordered corrective advertisements from the tobacco industry on the link between smoking and disease can increase knowledge and reduce misperceptions about smoking 85 . In one randomized controlled trial, a video debunking several myths about vaccination effectively reduced influential misperceptions, such as the false belief that vaccines cause autism or that they reduce the strength of the natural immune system 86 . Meta-analyses have consistently found that fact-checking and debunking interventions can be effective 87 , 88 , including in the context of countering health misinformation on social media 89 . However, not all medical misperceptions are equally amenable to corrections 90 . In fact, these same analyses note that the effectiveness of interventions is significantly attenuated by (1) the quality of the debunk, (2) the passing of time, and (3) prior beliefs and ideologies. For example, the aforementioned studies on autism 84 and corrective smoking advertisements 85 showed no remaining effect after a 1-week and 6-week follow-up, respectively. When designing corrections, simply labeling information as false or incorrect is generally not sufficient because correcting a myth by means of a simple retraction leaves a gap in people’s understanding of why the information is false and what is true instead. Accordingly, the recommendation for practitioners is often to craft much more detailed debunking materials 88 . Reviews of the literature 91 , 92 have indicated that best practice in designing debunking messages involves (1) leading with the truth, (2) appealing to scientific consensus and authoritative expert sources, (3) ensuring that the correction is easily accessible and not more complex than the initial misinformation, (4) a clear explanation of why the misinformation is wrong, and (5) the provision of a coherent alternative causal explanation (Fig. 1 ). Although there is generally a lack of comparative research, some recent studies have shown that optimizing debunking messages according to these guidelines enhances their efficacy when compared with alternative or business-as-usual debunking methods 84 .
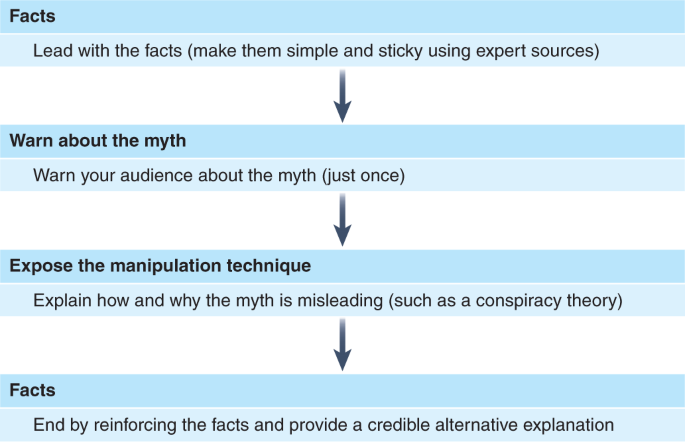
An effective debunking message should open with the facts and present them in a simple and memorable fashion. The audience should then be warned about the myth (do not repeat the myth more than once). The manipulation technique used to mislead people should subsequently be identified and exposed. End by repeating the facts and emphasizing the correct explanation.
Debunking: limitations and future research
Despite these advances, significant concerns have been raised about the application of such post hoc ‘therapeutic’ corrections, mostly notably the risk of a correction backfiring so that people end up believing more in the myth as a result of the correction. This backfire effect can occur along two potential dimensions 92 , 93 , one of which concerns psychological reactance against the correction itself (the ‘worldview’ backfire effect) whereas the other is concerned with the repetition of false information (the ‘familiarity’ backfire effect). Although early research was supportive of the fact that, for example, corrections about myths surrounding the flu and MMR vaccine can cause already concerned individuals to become even more hesitant about vaccination decisions 94 , 95 , more recent studies have failed to find evidence for such worldview backfire effects 93 , 96 . In fact, while evidence of backfire remains widely cited, recent replications have failed to reproduce such effects when correcting misinformation about vaccinations specifically 97 . Thus, although the effect likely exists, its frequency and intensity is less common than previously thought. Worldview backfire concerns can also be minimized by designing debunking messages in a way that coheres rather than conflicts with the recipients’ worldviews 92 . Nonetheless, because debunking forces a rhetorical frame in which the misinformation needs to be repeated in order to correct it (that is, rebutting someone else’s claim), there is a risk that such repetition enhances familiarity with the myth while people subsequently fail to encode the correction in long-term memory. Although research clearly shows that people are more likely to believe repeated (mis)information than non-repeated (mis)information 26 , recent work has found that the risk of ironically strengthening a myth as part of a debunking effort is relatively low 93 , especially when the debunking messages feature the correction prominently relative to the misinformation. The consensus is therefore that, although practitioners should be aware of these backfire concerns, they should not prevent the issuing of corrections given the infrequent nature of these side effects 91 , 93 .
Having said this, there are two other notable problems with therapeutic approaches that limit their efficacy. The first is that retrospective corrections do not reach the same amount of people as the original misinformation. For example, estimates reveal that only about 40% of smokers were exposed to the tobacco industry’s court-ordered corrections 98 . A related concern is that, after being exposed, people continue to make inferences on the basis of falsehoods, even when they acknowledge a correction. This phenomenon is known as the ‘continued influence of misinformation’ 92 , and meta-analyses have found robust evidence of continued influence effects in a wide range of contexts 88 , 99 .
Prophylactic treatments: inoculation theory
Accordingly, researchers have recently begun to explore prophylactic or pre-emptive approaches to countering misinformation, that is, before an individual has been exposed to or has reached ‘infectious’ status. Although prebunking is a more general term used for interventions that pre-emptively remind people to ‘think before they post’ 51 , such reminders in and of themselves do not equip people with any new skills to identify and resist misinformation. The most common framework for preventing unwanted persuasion is psychological inoculation theory 100 , 101 (Fig. 2 ). The theory of psychological inoculation follows the biomedical analogy and posits that, just as vaccines trigger the production of antibodies to help confer immunity against future infection, the same can be achieved with information. By pre-emptively forewarning and exposing people to severely weakened doses of misinformation (coupled with strong refutations), people can cultivate cognitive resistance against future misinformation 102 . Inoculation theory operates via two mechanisms, namely (1) motivational threat (a desire to defend oneself from manipulation attacks) and (2) refutational pre-emption or prebunking (pre-exposure to a weakened example of the attack). For example, research has found that inoculating people against conspiratorial arguments about vaccination before (but not after) exposure to a conspiracy theory effectively raised vaccination intentions 103 . Several recent reviews 102 , 104 and meta-analyses 105 have pointed to the efficacy of psychological inoculation as a robust strategy for conferring immunity to persuasion by misinformation, including many applications in the health domain 106 , such as inoculating people against misinformation about the use of mammography in breast-cancer screening 107 .
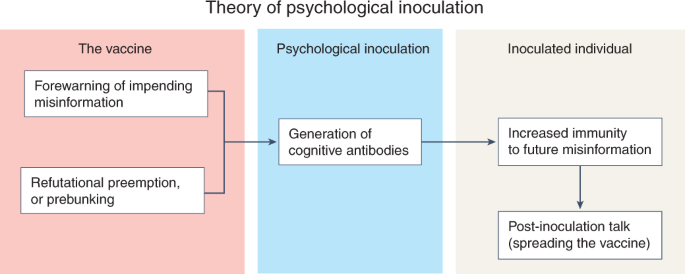
Psychological inoculation consists of two core components: (1) forewarning people that they may be misled by misinformation (to activate the psychological ‘immune system’), and (2) prebunking the misinformation (tactic) by exposing people to a severely weakened dose of it coupled with strong counters and refutations (to generate the cognitive ‘antibodies’). Once people have gained ‘immunity’ they can then vicariously spread the inoculation to others via offline and online interactions.
Several recent advances, in particular, are worth noting. The first is that the field has moved from ‘narrow-spectrum’ or ‘fact-based’ inoculation to ‘broad-spectrum’ or ‘technique-based’ immunization 102 , 108 . The reasoning behind this shift is that, although it is possible to synthesize a severely weakened dose from existing misinformation (and to subsequently refute that weakened example with strong counterarguments), it is difficult to scale the vaccine if this process has to be repeated anew for every piece of misinformation. Instead, scholars have started to identify the common building blocks of misinformation more generally 38 , 109 , including techniques such as impersonating fake experts and doctors, manipulating people’s emotions with fear appeals, and the use of conspiracy theories. Research has found that people can be inoculated against these underlying strategies and, as a result, become relatively more immune to a whole range of misinformation that makes use of these tactics 38 , 102 . This process is sometimes referred to as cross-protection insofar as inoculating people against one strain offers protection against related and different strains of the same misinformation tactic.
A second advance surrounds the application of active versus passive inoculation. Whereas the traditional inoculation process is passive insofar as people pre-emptively receive the specific refutations from the experimenter, the process of active inoculation encourages people to generate their own ‘antibodies’. Perhaps the best-known example of active inoculation are popular gamified inoculation interventions such as Bad News 38 and GoViral! 110 , where players step into the shoes of a misinformation producer and are exposed—in a simulated social-media environment—to weakened doses of common strategies used to spread misinformation. As part of this process, players actively generate their own media content and unveil the techniques of manipulation. Research has found that resistance to deception occurs when people (1) recognize their own vulnerability to being persuaded and (2) perceive undue intent to manipulate their opinion 111 , 112 . These games therefore aim to expose people’s vulnerability, motivating an individuals’ desire to protect themselves against misinformation through pre-exposure to weakened doses. Randomized controlled trials have found that active inoculation games help people identify misinformation 38 , 110 , 113 , 114 , boost confidence in people’s truth-discernment abilities 110 , 113 , and reduce self-reported sharing of misinformation 110 , 115 . Yet, like many biological vaccines, research has found that psychological immunity also wanes over time but can be maintained for several months with regular ‘booster’ shots that re-engage people with the inoculation process 114 . A benefit of this line of research is that these gamified interventions have been evaluated and scaled across millions of people as part of the WHO’s ‘Stop The Spread’ campaign and the United Nations’ ‘Verified’ initiative in collaboration with the UK government 110 , 116 .
Prebunking: limitations and future research
A potential limitation is that, although misinformation tropes are often repeated throughout history (consider similarities in the myths that the cowpox vaccine would turn people into human–cow hybrids and the conspiracy theory that COVID-19 vaccines alter human DNA), inoculation does require at least some advanced knowledge of what misinformation (tactic) people might be exposed to in the future 91 . In addition, as healthcare workers are being trained to combat misinformation 117 , it is important to avoid confusion in terminology when using psychological inoculation to counter vaccine hesitancy. For example, the approach can be implemented without making explicit reference to the vaccination analogy and instead can focus on the value of ‘prebunking’ and helping people unveil the techniques of manipulation.
Several other important open questions remain. For example, analogous to recent advances in experimental medicine on the application of therapeutic vaccines—which can still boost immune responses after infection—research has found that inoculation can still protect people from misinformation even when they have already been exposed to misinformation 108 , 112 , 118 . This makes conceptual sense insofar it may take repeated exposure or a significant amount of time for misinformation to fully persuade people or become integrated with prior attitudes. Yet it remains conceptually unclear at which point therapeutic inoculation transitions into traditional debunking. Moreover, although some comparisons of active versus passive inoculation approaches exist 105 , 110 , the evidence base for active forms of inoculation remains relatively small. Similarly, whereas head-to-head studies that compared prebunking to debunking suggest that prevention is indeed better than cure 103 , more comparative research is needed. Research also finds that it is possible for people to vicariously pass on the inoculation interpersonally or on social media, a process known as ‘post-inoculation talk’ 104 , which alludes to the possibility of herd immunity in online communities 110 , yet no social-network simulations currently exist that evaluate the potential of inoculative approaches. Current studies are also based on self-reported sharing of misinformation. Future research will need to evaluate the extent to which inoculation can scale across the population and influence objective news-sharing behavior on social media.
The spread of misinformation has undermined public-health efforts, from vaccination uptake to public compliance with health-protective behaviors. Research finds that although people are sometimes duped by misinformation because they are distracted on social media and are not paying sufficient attention to accuracy cues, the politicized nature of many public-health challenges suggests that people also believe in and share misinformation because doing so reinforces important socio-political beliefs and identity structures. A more integrated framework is needed that is sensitive to context and can account for varying susceptibility to misinformation on the basis of how people prioritize accuracy and social motives when forming judgments about the veracity of news media. Although ‘exposure’ does not equal ‘infection,’ misinformation can spread fast in online networks, and its virality is often aided by the existence of political echo chambers. Importantly, however, the bulk of misinformation on social media often originates from influential accounts and superspreaders. Therapeutic and prophylactic approaches to countering misinformation have both demonstrated some success, but given the continued influence of misinformation after exposure, there is much value in preventative approaches, and more research is needed on how to best combine debunking and prebunking efforts. Further research is also encouraged to outline the benefits and potential challenges to applying the epidemiological model to understand the psychology behind the spread of misinformation. A major challenge for the field moving forward will be clearly defining how misinformation is measured and conceptualized, as well as the need for standardized psychometric instruments that allow for better comparisons of outcomes across studies.
Zarocostas, J. How to fight an infodemic. Lancet 395 , 676 (2020).
Article CAS PubMed PubMed Central Google Scholar
Allcott, H. & Gentzkow, M. Social media and fake news in the 2016 election. J. Econ. Perspect. 31 , 211–236 (2020).
Article Google Scholar
Grinberg, N. et al. Fake news on Twitter during the 2016 US presidential election. Science 363 , 374–378 (2019).
Article CAS PubMed Google Scholar
Roozenbeek, J. et al. Susceptibility to misinformation about COVID-19 around the world. R. Soc. Open Sci. 7 , 201199 (2020).
Romer, D. & Jamieson, K. H. Conspiracy theories as barriers to controlling the spread of COVID-19 in the US. Soc. Sci. Med. 263 , 113356 (2020).
Article PubMed PubMed Central Google Scholar
Imhoff, R. & Lamberty, P. A bioweapon or a hoax? The link between distinct conspiracy beliefs about the coronavirus disease (COVID-19) outbreak and pandemic behavior. Soc. Psychol. Personal. Sci. 11 , 1110–1118 (2020).
Article PubMed Central Google Scholar
Freeman, D. et al. Coronavirus conspiracy beliefs, mistrust, and compliance with government guidelines in England. Psychol. Med. https://doi.org/10.1017/S0033291720001890 (2020).
Loomba, S. et al. Measuring the impact of COVID-19 vaccine misinformation on vaccination intent in the UK and USA. Nat. Hum. Behav. 5 , 337–348 (2021).
Article PubMed Google Scholar
Johnson, N. et al. The online competition between pro-and anti-vaccination views. Nature 58 , 230–233 (2020).
Aghababaeian, H. et al. Alcohol intake in an attempt to fight COVID-19: a medical myth in Iran. Alcohol 88 , 29–32 (2020).
Jolley, D. & Paterson, J. L. Pylons ablaze: examining the role of 5G COVID‐19 conspiracy beliefs and support for violence. Br. J. Soc. Psychol. 59 , 628–640 (2020).
Dubé, E. et al. Vaccine hesitancy, vaccine refusal and the anti-vaccine movement: influence, impact and implications. Expert Rev. Vaccines 14 , 99–117 (2015).
Armstrong, G. M. et al. A longitudinal evaluation of the Listerine corrective advertising campaign. J. Public Policy Mark. 2 , 16–28 (1983).
Albarracin, D. et al. Misleading claims about tobacco products in YouTube videos: experimental effects of misinformation on unhealthy attitudes. J. Medical Internet Res . 20 , e9959 (2018).
Krishna, A. & Thompson, T. L. Misinformation about health: a review of health communication and misinformation scholarship. Am. Behav. Sci. 65 , 316–332 (2021).
Kucharski, A. Study epidemiology of fake news. Nature 540 , 525–525 (2016).
Cinelli, M. et al. The COVID-19 social media infodemic. Sci. Rep. 10 , 1–10 (2020).
Scales, D. et al. The COVID-19 infodemic—applying the epidemiologic model to counter misinformation. N. Engl. J. Med 385 , 678–681 (2021).
Vraga, E. K. & Bode, L. Defining misinformation and understanding its bounded nature: using expertise and evidence for describing misinformation. Polit. Commun. 37 , 136–144 (2020).
Southwell et al. Misinformation as a misunderstood challenge to public health. Am. J. Prev. Med. 57 , 282–285 (2019).
Wardle, C. & Derakhshan, H. Information Disorder: toward an Interdisciplinary Framework for Research and Policymaking . Council of Europe report DGI (2017)09 (Council of Europe, 2017).
van der Linden, S. et al. You are fake news: political bias in perceptions of fake news. Media Cult. Soc. 42 , 460–470 (2020).
Tandoc, E. C. Jr et al. Defining ‘fake news’ a typology of scholarly definitions. Digit. J. 6 , 137–153 (2018).
Google Scholar
Allen, J. et al. Evaluating the fake news problem at the scale of the information ecosystem. Sci. Adv. 6 , eaay3539 (2020).
Marsh, E. J. & Yang, B. W. in Misinformation and Mass Audiences (eds Southwell, B. G., Thorson, E. A., & Sheble, L) 15–34 (University of Texas Press, 2018).
Dechêne, A. et al. The truth about the truth: a meta-analytic review of the truth effect. Pers. Soc. Psychol. Rev. 14 , 238–257 (2010).
Lewis, T. Eight persistent COVID-19 myths and why people believe them. Scientific American . https://www.scientificamerican.com/article/eight-persistent-covid-19-myths-and-why-people-believe-them/ (2020).
Wang, W. C. et al. On known unknowns: fluency and the neural mechanisms of illusory truth. J. Cogn. Neurosci. 28 , 739–746 (2016).
Pennycook, G. et al. Prior exposure increases perceived accuracy of fake news. J. Exp. Psychol. Gen. 147 , 1865–1880 (2018).
Fazio, L. K. et al. Repetition increases perceived truth equally for plausible and implausible statements. Psychon. Bull. Rev. 26 , 1705–1710 (2019).
Fazio, L. K. et al. Knowledge does not protect against illusory truth. J. Exp. Psychol. Gen. 144 , 993–1002 (2015).
De Keersmaecker, J. et al. Investigating the robustness of the illusory truth effect across individual differences in cognitive ability, need for cognitive closure, and cognitive style. Pers. Soc. Psychol. Bull. 46 , 204–215 (2020).
Guess, A. et al. Less than you think: prevalence and predictors of fake news dissemination on Facebook. Sci. Adv. 5 , eaau4586 (2019).
Saunders, J. & Jess, A. The effects of age on remembering and knowing misinformation. Memory 18 , 1–11 (2010).
Brashier, N. M. & Schacter, D. L. Aging in an era of fake news. Curr. Dir. Psychol. Sci. 29 , 316–323 (2020).
Pennycook, G. & Rand, D. G. Lazy, not biased: susceptibility to partisan fake news is better explained by lack of reasoning than by motivated reasoning. Cognition 188 , 39–50 (2019).
Imhoff, R. et al. Conspiracy mentality and political orientation across 26 countries. Nat. Hum. Behav. https://doi.org/10.1038/s41562-021-01258-7 (2022).
Roozenbeek, J. & van der Linden, S. Fake news game confers psychological resistance against online misinformation. Humanit. Soc. Sci. Commun. 5 , 1–10 (2019).
Van der Linden, S. et al. The paranoid style in American politics revisited: an ideological asymmetry in conspiratorial thinking. Polit. Psychol. 42 , 23–51 (2021).
De keersmaecker, J. & Roets, A. ‘Fake news’: incorrect, but hard to correct. The role of cognitive ability on the impact of false information on social impressions. Intelligence 65 , 107–110 (2017).
Bronstein, M. V. et al. Belief in fake news is associated with delusionality, dogmatism, religious fundamentalism, and reduced analytic thinking. J. Appl. Res. Mem. 8 , 108–117 (2019).
Greene, C. M. et al. Misremembering Brexit: partisan bias and individual predictors of false memories for fake news stories among Brexit voters. Memory 29 , 587–604 (2021).
Gawronski, B. Partisan bias in the identification of fake news. Trends Cogn. Sci. 25 , 723–724 (2021).
Rathje, S et al. Meta-analysis reveals that accuracy nudges have little to no effect for US conservatives: Regarding Pennycook et al. (2020). Psychol. Sci. https://doi.org/10.25384/SAGE.12594110.v2 (2021).
Pennycook, G. & Rand, D. G. The psychology of fake news. Trends Cogn. Sci. 22 , 388–402 (2021).
van der Linden, S. et al. How can psychological science help counter the spread of fake news? Span. J. Psychol. 24 , e25 (2021).
Evans, J. S. B. In two minds: dual-process accounts of reasoning. Trends Cogn. Sci. 7 , 454–459 (2003).
Bago, B. et al. Fake news, fast and slow: deliberation reduces belief in false (but not true) news headlines. J. Exp. Psychol. Gen. 149 , 1608–1613 (2020).
Scherer, L. D. et al. Who is susceptible to online health misinformation? A test of four psychosocial hypotheses. Health Psychol. 40 , 274–284 (2021).
Pennycook, G. et al. Fighting COVID-19 misinformation on social media: experimental evidence for a scalable accuracy-nudge intervention. Psychol. Sci. 31 , 770–780 (2020).
Pennycook, G. et al. Shifting attention to accuracy can reduce misinformation online. Nature 592 , 590–595 (2021).
Swami, V. et al. Analytic thinking reduces belief in conspiracy theories. Cognition 133 , 572–585 (2014).
Kunda, Z. The case for motivated reasoning. Psychol. Bull. 108 , 480–498 (1990).
Kahan, D. M. in Emerging Trends in the Social and Behavioral sciences (eds Scott, R. & Kosslyn, S.) 1–16 (John Wiley & Sons, 2016).
Bolsen, T. et al. The influence of partisan motivated reasoning on public opinion. Polit. Behav. 36 , 235–262 (2014).
Osmundsen, M. et al. Partisan polarization is the primary psychological motivation behind political fake news sharing on Twitter. Am. Polit. Sci. Rev. 115 , 999–1015 (2021).
Van Bavel, J. J. et al. Political psychology in the digital (mis) information age: a model of news belief and sharing. Soc. Issues Policy Rev. 15 , 84–113 (2020).
Rathje, S. et al. Out-group animosity drives engagement on social media. Proc. Natl Acad. Sci. USA 118 , e2024292118 (2021).
Kahan, D. M. et al. Motivated numeracy and enlightened self-government. Behav. Public Policy 1 , 54–86 (2017).
Kahan, D. M. et al. The polarizing impact of science literacy and numeracy on perceived climate change risks. Nat. Clim. Chang. 2 , 732–735 (2012).
Drummond, C. & Fischhoff, B. Individuals with greater science literacy and education have more polarized beliefs on controversial science topics. Proc. Natl Acad. Sci. USA 114 , 9587–9592 (2017).
Traberg, C. S. & van der Linden, S. Birds of a feather are persuaded together: perceived source credibility mediates the effect of political bias on misinformation susceptibility. Pers. Individ. Differ. 185 , 111269 (2022).
Roozenbeek, J. et al. How accurate are accuracy-nudge interventions? A preregistered direct replication of Pennycook et al. (2020). Psychol. Sci. 32 , 1169–1178 (2021).
Persson, E. et al. A preregistered replication of motivated numeracy. Cognition 214 , 104768 (2021).
Connor, P. et al. Motivated numeracy and active reasoning in a Western European sample. Behav. Public Policy 1–23 (2020).
van der Linden, S. et al. Scientific agreement can neutralize politicization of facts. Nat. Hum. Behav. 2 , 2–3 (2018).
Tappin, B. M. et al. Rethinking the link between cognitive sophistication and politically motivated reasoning. J. Exp. Psychol. Gen. 150 , 1095–1114 (2021).
Tappin, B. M. et al. Thinking clearly about causal inferences of politically motivated reasoning: why paradigmatic study designs often undermine causal inference. Curr. Opin. Behav. Sci. 34 , 81–87 (2020).
Druckman, J. N. & McGrath, M. C. The evidence for motivated reasoning in climate change preference formation. Nat. Clim. Chang. 9 , 111–119 (2019).
Juul, J. L. & Ugander, J. Comparing information diffusion mechanisms by matching on cascade size. Proc. Natl. Acad. Sci. USA 118 , e210078611 (2021).
Vosoughi, S. et al. The spread of true and false news online. Science 359 , 1146–1151 (2018).
Cinelli, M. et al. The echo chamber effect on social media. Proc. Natl Acad. Sci. USA 118 , e2023301118 (2021).
Guess, A. et al. Exposure to untrustworthy websites in the 2016 US election. Nat. Hum. Behav. 4 , 472–480 (2020).
Yang, K. C. et al. The COVID-19 infodemic: Twitter versus Facebook. Big Data Soc. 8 , 20539517211013861 (2021).
Del Vicario, M. et al. The spreading of misinformation online. Proc. Natl Acad. Sci. USA 113 , 554–559 (2016).
Zollo, F. et al. Debunking in a world of tribes. PloS ONE 12 , e0181821 (2017).
Guess, A. M. (Almost) everything in moderation: new evidence on Americans’ online media diets. Am. J. Pol. Sci. 65 , 1007–1022 (2021).
Törnberg, P. Echo chambers and viral misinformation: modeling fake news as complex contagion. PLoS ONE 13 , e0203958 (2018).
Choi, D. et al. Rumor propagation is amplified by echo chambers in social media. Sci. Rep. 10 , 1–10 (2020).
Eurobarometer on Fake News and Online Disinformation. European Commission https://ec.europa.eu/digital-single-market/en/news/final-results-eurobarometer-fake-news-and-online-disinformation (2018).
Altay, S. et al. ‘If this account is true, it is most enormously wonderful’: interestingness-if-true and the sharing of true and false news. Digit. Journal. https://doi.org/10.1080/21670811.2021.1941163 (2021).
Kalla, J. L. & Broockman, D. E. The minimal persuasive effects of campaign contact in general elections: evidence from 49 field experiments. Am. Political Sci. Rev. 112 , 148–166 (2018).
Matz, S. C. et al. Psychological targeting as an effective approach to digital mass persuasion. Proc. Natl Acad. Sci. USA 114 , 12714–12719 (2017).
Paynter, J. et al. Evaluation of a template for countering misinformation—real-world autism treatment myth debunking. PloS ONE 14 , e0210746 (2019).
Smith, P. et al. Correcting over 50 years of tobacco industry misinformation. Am. J. Prev. Med 40 , 690–698 (2011).
Yousuf, H. et al. A media intervention applying debunking versus non-debunking content to combat vaccine misinformation in elderly in the Netherlands: a digital randomised trial. EClinicalMedicine 35 , 100881 (2021).
Walter, N. & Murphy, S. T. How to unring the bell: a meta-analytic approach to correction of misinformation. Commun. Monogr. 85 , 423–441 (2018).
Chan, M. P. S. et al. Debunking: a meta-analysis of the psychological efficacy of messages countering misinformation. Psychol. Sci. 28 , 1531–1546 (2017).
Walter, N. et al. Evaluating the impact of attempts to correct health misinformation on social media: a meta-analysis. Health Commun. 36 , 1776–1784 (2021).
Aikin, K. J. et al. Correction of overstatement and omission in direct-to-consumer prescription drug advertising. J. Commun. 65 , 596–618 (2015).
Lewandowsky, S. et al. The Debunking Handbook 2020 https://www.climatechangecommunication.org/wp-content/uploads/2020/10/DebunkingHandbook2020.pdf (2020).
Lewandowsky, S. et al. Misinformation and its correction: continued influence and successful debiasing. Psychol. Sci. Publ. Int 13 , 106–131 (2012).
Swire-Thompson, B. et al. Searching for the backfire effect: measurement and design considerations. J. Appl. Res. Mem. Cogn. 9 , 286–299 (2020).
Nyhan, B. et al. Effective messages in vaccine promotion: a randomized trial. Pediatrics 133 , e835–e842 (2014).
Nyhan, B. & Reifler, J. Does correcting myths about the flu vaccine work? An experimental evaluation of the effects of corrective information. Vaccine 33 , 459–464 (2015).
Wood, T. & Porter, E. The elusive backfire effect: mass attitudes’ steadfast factual adherence. Polit. Behav. 41 , 135–163 (2019).
Haglin, K. The limitations of the backfire effect. Res. Politics https://doi.org/10.1177/2053168017716547 (2017).
Chido-Amajuoyi et al. Exposure to court-ordered tobacco industry antismoking advertisements among US adults. JAMA Netw. Open 2 , e196935 (2019).
Walter, N. & Tukachinsky, R. A meta-analytic examination of the continued influence of misinformation in the face of correction: how powerful is it, why does it happen, and how to stop it? Commun. Res 47 , 155–177 (2020).
Papageorgis, D. & McGuire, W. J. The generality of immunity to persuasion produced by pre-exposure to weakened counterarguments. J. Abnorm. Psychol. 62 , 475–481 (1961).
CAS Google Scholar
McGuire, W. J. in Advances in Experimental Social Psychology (ed Berkowitz, L.) 191–229 (Academic Press, 1964).
Lewandowsky, S. & van der Linden, S. Countering misinformation and fake news through inoculation and prebunking. Eur. Rev. Soc. Psychol. 32 , 348–384 (2021).
Jolley, D. & Douglas, K. M. Prevention is better than cure: addressing anti vaccine conspiracy theories. J. Appl. Soc. Psychol. 47 , 459–469 (2017).
Compton, J. et al. Inoculation theory in the post‐truth era: extant findings and new frontiers for contested science, misinformation, and conspiracy theories. Soc. Personal. Psychol. 15 , e12602 (2021).
Banas, J. A. & Rains, S. A. A meta-analysis of research on inoculation theory. Commun. Monogr. 77 , 281–311 (2010).
Compton, J. et al. Persuading others to avoid persuasion: Inoculation theory and resistant health attitudes. Front. Psychol. 7 , 122 (2016).
Iles, I. A. et al. Investigating the potential of inoculation messages and self-affirmation in reducing the effects of health misinformation. Sci. Commun. 43 , 768–804 (2021).
Cook et al. Neutralizing misinformation through inoculation: Exposing misleading argumentation techniques reduces their influence. PloS ONE 12 , e0175799 (2017).
van der Linden, S., & Roozenbeek, J. in The Psychology of Fake News: Accepting, Sharing, and Correcting Misinformation (eds Greifeneder, R., Jaffe, M., Newman, R., & Schwarz, N.) 147–169 (Psychology Press, 2020).
Basol, M. et al. Towards psychological herd immunity: cross-cultural evidence for two prebunking interventions against COVID-19 misinformation. Big Data Soc. 8 , 20539517211013868 (2021).
Sagarin, B. J. et al. Dispelling the illusion of invulnerability: the motivations and mechanisms of resistance to persuasion. J. Pers. Soc. Psychol. 83 , 526–541 (2002).
van der Linden, S. et al. Inoculating the public against misinformation about climate change. Glob. Chall. 1 , 1600008 (2017).
Basol, M. et al. Good news about bad news: gamified inoculation boosts confidence and cognitive immunity against fake news. J. Cogn. 3 , 2 (2020).
Maertens, R. et al. Long-term effectiveness of inoculation against misinformation: three longitudinal experiments. J. Exp. Psychol. Appl 27 , 1–16 (2021).
Roozenbeek, J., & van der Linden, S. Breaking Harmony Square: a game that ‘inoculates’ against political misinformation. The Harvard Kennedy School Misinformation Review https://doi.org/10.37016/mr-2020-47 (2020).
What is Go Viral? World Health Organization https://www.who.int/news/item/23-09-2021-what-is-go-viral (WHO, 2021).
Abbasi, J. COVID-19 conspiracies and beyond: how physicians can deal with patients’ misinformation. JAMA 325 , 208–210 (2021).
Compton, J. Prophylactic versus therapeutic inoculation treatments for resistance to influence. Commun. Theory 30 , 330–343 (2020).
Lazer, D. M. et al. The science of fake news. Science 359 , 1094–1096 (2018).
Pennycook, G. & Rand, D. G. Who falls for fake news? The roles of bullshit receptivity, overclaiming, familiarity, and analytic thinking. J. Pers. 88 , 185–200 (2020).
Benton, J. Facebook sent a ton of traffic to a Chicago Tribune story. So why is everyone mad at them? NiemanLab https://www.niemanlab.org/2021/08/facebook-sent-a-ton-of-traffic-to-a-chicago-tribune-story-so-why-is-everyone-mad-at-them/ (2021).
Poutoglidou, F. et al. Ibuprofen and COVID-19 disease: separating the myths from facts. Expert Rev. Respir. Med 15 , 979–983 (2021).
Maertens, R. et al. The Misinformation Susceptibility Test (MIST): a psychometrically validated measure of news veracity discernment. Preprint at PsyArXiv https://doi.org/10.31234/osf.io/gk68h (2021).
Download references
Acknowledgements
I am grateful for support from the IRIS Infodemic Coalition (UK Government, no. SCH-00001-3391) and JITSUVAX (EU 2020 Horizon no. 964728). I thank the Cambridge Social Decision-Making Lab and credit R. Maertens in particular for his help with designing Fig. 2 .
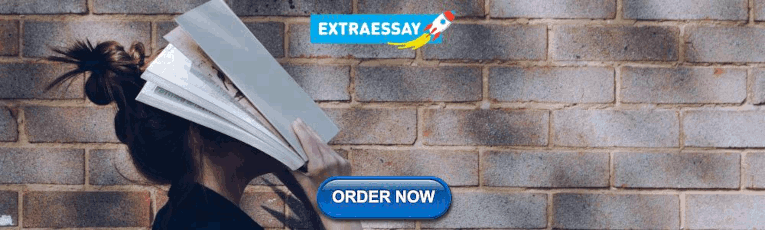
Author information
Authors and affiliations.
Department of Psychology, School of Biology, University of Cambridge, Cambridge, United Kingdom
Sander van der Linden
You can also search for this author in PubMed Google Scholar
Corresponding author
Correspondence to Sander van der Linden .
Ethics declarations
Competing interests.
S.V.D.L. co-designed several interventions in collaboration with the UK Government, DROG, and the WHO, reviewed in this paper, namely GoViral! and Bad News . He does not receive nor hold any financial interests in these interventions. He has received research funding from or consulted for the UK government, the US Government, the European Commission, Facebook, Google, WhatsApp, Edelman, the United Nations, and the WHO on misinformation and infodemic management.
Peer review
Peer review information.
Nature Medicine thanks Brian Southwell and Alison Buttenheim for their contribution to the peer review of this work. Karen O’Leary was the primary editor on this article and managed its editorial process and peer review in collaboration with the rest of the editorial team.
Additional information
Publisher’s note Springer Nature remains neutral with regard to jurisdictional claims in published maps and institutional affiliations.
Rights and permissions
Reprints and permissions
About this article
Cite this article.
van der Linden, S. Misinformation: susceptibility, spread, and interventions to immunize the public. Nat Med 28 , 460–467 (2022). https://doi.org/10.1038/s41591-022-01713-6
Download citation
Received : 21 November 2021
Accepted : 24 January 2022
Published : 10 March 2022
Issue Date : March 2022
DOI : https://doi.org/10.1038/s41591-022-01713-6
Share this article
Anyone you share the following link with will be able to read this content:
Sorry, a shareable link is not currently available for this article.
Provided by the Springer Nature SharedIt content-sharing initiative
This article is cited by
The adaptive community-response (acr) method for collecting misinformation on social media.
- Julian Kauk
- Helene Kreysa
- Stefan R. Schweinberger
Journal of Big Data (2024)
Belief-consistent information is most shared despite being the least surprising
- Jacob T. Goebel
- Mark W. Susmann
- Duane T. Wegener
Scientific Reports (2024)
Conventional and frugal methods of estimating COVID-19-related excess deaths and undercount factors
- Abhishek M. Dedhe
- Aakash A. Chowkase
- Pranav S. Pandit
Diverse misinformation: impacts of human biases on detection of deepfakes on networks
- Juniper Lovato
- Jonathan St-Onge
- Jeremiah Onaolapo
npj Complexity (2024)
Early morning hour and evening usage habits increase misinformation-spread
- Elisabeth Stockinger
- Riccardo Gallotti
- Carina I. Hausladen
Quick links
- Explore articles by subject
- Guide to authors
- Editorial policies
Sign up for the Nature Briefing newsletter — what matters in science, free to your inbox daily.


An official website of the United States government
The .gov means it’s official. Federal government websites often end in .gov or .mil. Before sharing sensitive information, make sure you’re on a federal government site.
The site is secure. The https:// ensures that you are connecting to the official website and that any information you provide is encrypted and transmitted securely.
- Publications
- Account settings
Preview improvements coming to the PMC website in October 2024. Learn More or Try it out now .
- Advanced Search
- Journal List
- Springer Nature - PMC COVID-19 Collection

Fake news, disinformation and misinformation in social media: a review
Esma aïmeur.
Department of Computer Science and Operations Research (DIRO), University of Montreal, Montreal, Canada
Sabrine Amri
Gilles brassard, associated data.
All the data and material are available in the papers cited in the references.
Online social networks (OSNs) are rapidly growing and have become a huge source of all kinds of global and local news for millions of users. However, OSNs are a double-edged sword. Although the great advantages they offer such as unlimited easy communication and instant news and information, they can also have many disadvantages and issues. One of their major challenging issues is the spread of fake news. Fake news identification is still a complex unresolved issue. Furthermore, fake news detection on OSNs presents unique characteristics and challenges that make finding a solution anything but trivial. On the other hand, artificial intelligence (AI) approaches are still incapable of overcoming this challenging problem. To make matters worse, AI techniques such as machine learning and deep learning are leveraged to deceive people by creating and disseminating fake content. Consequently, automatic fake news detection remains a huge challenge, primarily because the content is designed in a way to closely resemble the truth, and it is often hard to determine its veracity by AI alone without additional information from third parties. This work aims to provide a comprehensive and systematic review of fake news research as well as a fundamental review of existing approaches used to detect and prevent fake news from spreading via OSNs. We present the research problem and the existing challenges, discuss the state of the art in existing approaches for fake news detection, and point out the future research directions in tackling the challenges.
Introduction
Context and motivation.
Fake news, disinformation and misinformation have become such a scourge that Marcia McNutt, president of the National Academy of Sciences of the United States, is quoted to have said (making an implicit reference to the COVID-19 pandemic) “Misinformation is worse than an epidemic: It spreads at the speed of light throughout the globe and can prove deadly when it reinforces misplaced personal bias against all trustworthy evidence” in a joint statement of the National Academies 1 posted on July 15, 2021. Indeed, although online social networks (OSNs), also called social media, have improved the ease with which real-time information is broadcast; its popularity and its massive use have expanded the spread of fake news by increasing the speed and scope at which it can spread. Fake news may refer to the manipulation of information that can be carried out through the production of false information, or the distortion of true information. However, that does not mean that this problem is only created with social media. A long time ago, there were rumors in the traditional media that Elvis was not dead, 2 that the Earth was flat, 3 that aliens had invaded us, 4 , etc.
Therefore, social media has become nowadays a powerful source for fake news dissemination (Sharma et al. 2019 ; Shu et al. 2017 ). According to Pew Research Center’s analysis of the news use across social media platforms, in 2020, about half of American adults get news on social media at least sometimes, 5 while in 2018, only one-fifth of them say they often get news via social media. 6
Hence, fake news can have a significant impact on society as manipulated and false content is easier to generate and harder to detect (Kumar and Shah 2018 ) and as disinformation actors change their tactics (Kumar and Shah 2018 ; Micallef et al. 2020 ). In 2017, Snow predicted in the MIT Technology Review (Snow 2017 ) that most individuals in mature economies will consume more false than valid information by 2022.
Recent news on the COVID-19 pandemic, which has flooded the web and created panic in many countries, has been reported as fake. 7 For example, holding your breath for ten seconds to one minute is not a self-test for COVID-19 8 (see Fig. 1 ). Similarly, online posts claiming to reveal various “cures” for COVID-19 such as eating boiled garlic or drinking chlorine dioxide (which is an industrial bleach), were verified 9 as fake and in some cases as dangerous and will never cure the infection.

Fake news example about a self-test for COVID-19 source: https://cdn.factcheck.org/UploadedFiles/Screenshot031120_false.jpg , last access date: 26-12-2022
Social media outperformed television as the major news source for young people of the UK and the USA. 10 Moreover, as it is easier to generate and disseminate news online than with traditional media or face to face, large volumes of fake news are produced online for many reasons (Shu et al. 2017 ). Furthermore, it has been reported in a previous study about the spread of online news on Twitter (Vosoughi et al. 2018 ) that the spread of false news online is six times faster than truthful content and that 70% of the users could not distinguish real from fake news (Vosoughi et al. 2018 ) due to the attraction of the novelty of the latter (Bovet and Makse 2019 ). It was determined that falsehood spreads significantly farther, faster, deeper and more broadly than the truth in all categories of information, and the effects are more pronounced for false political news than for false news about terrorism, natural disasters, science, urban legends, or financial information (Vosoughi et al. 2018 ).
Over 1 million tweets were estimated to be related to fake news by the end of the 2016 US presidential election. 11 In 2017, in Germany, a government spokesman affirmed: “We are dealing with a phenomenon of a dimension that we have not seen before,” referring to an unprecedented spread of fake news on social networks. 12 Given the strength of this new phenomenon, fake news has been chosen as the word of the year by the Macquarie dictionary both in 2016 13 and in 2018 14 as well as by the Collins dictionary in 2017. 15 , 16 Since 2020, the new term “infodemic” was coined, reflecting widespread researchers’ concern (Gupta et al. 2022 ; Apuke and Omar 2021 ; Sharma et al. 2020 ; Hartley and Vu 2020 ; Micallef et al. 2020 ) about the proliferation of misinformation linked to the COVID-19 pandemic.
The Gartner Group’s top strategic predictions for 2018 and beyond included the need for IT leaders to quickly develop Artificial Intelligence (AI) algorithms to address counterfeit reality and fake news. 17 However, fake news identification is a complex issue. (Snow 2017 ) questioned the ability of AI to win the war against fake news. Similarly, other researchers concurred that even the best AI for spotting fake news is still ineffective. 18 Besides, recent studies have shown that the power of AI algorithms for identifying fake news is lower than its ability to create it Paschen ( 2019 ). Consequently, automatic fake news detection remains a huge challenge, primarily because the content is designed to closely resemble the truth in order to deceive users, and as a result, it is often hard to determine its veracity by AI alone. Therefore, it is crucial to consider more effective approaches to solve the problem of fake news in social media.
Contribution
The fake news problem has been addressed by researchers from various perspectives related to different topics. These topics include, but are not restricted to, social science studies , which investigate why and who falls for fake news (Altay et al. 2022 ; Batailler et al. 2022 ; Sterret et al. 2018 ; Badawy et al. 2019 ; Pennycook and Rand 2020 ; Weiss et al. 2020 ; Guadagno and Guttieri 2021 ), whom to trust and how perceptions of misinformation and disinformation relate to media trust and media consumption patterns (Hameleers et al. 2022 ), how fake news differs from personal lies (Chiu and Oh 2021 ; Escolà-Gascón 2021 ), examine how can the law regulate digital disinformation and how governments can regulate the values of social media companies that themselves regulate disinformation spread on their platforms (Marsden et al. 2020 ; Schuyler 2019 ; Vasu et al. 2018 ; Burshtein 2017 ; Waldman 2017 ; Alemanno 2018 ; Verstraete et al. 2017 ), and argue the challenges to democracy (Jungherr and Schroeder 2021 ); Behavioral interventions studies , which examine what literacy ideas mean in the age of dis/mis- and malinformation (Carmi et al. 2020 ), investigate whether media literacy helps identification of fake news (Jones-Jang et al. 2021 ) and attempt to improve people’s news literacy (Apuke et al. 2022 ; Dame Adjin-Tettey 2022 ; Hameleers 2022 ; Nagel 2022 ; Jones-Jang et al. 2021 ; Mihailidis and Viotty 2017 ; García et al. 2020 ) by encouraging people to pause to assess credibility of headlines (Fazio 2020 ), promote civic online reasoning (McGrew 2020 ; McGrew et al. 2018 ) and critical thinking (Lutzke et al. 2019 ), together with evaluations of credibility indicators (Bhuiyan et al. 2020 ; Nygren et al. 2019 ; Shao et al. 2018a ; Pennycook et al. 2020a , b ; Clayton et al. 2020 ; Ozturk et al. 2015 ; Metzger et al. 2020 ; Sherman et al. 2020 ; Nekmat 2020 ; Brashier et al. 2021 ; Chung and Kim 2021 ; Lanius et al. 2021 ); as well as social media-driven studies , which investigate the effect of signals (e.g., sources) to detect and recognize fake news (Vraga and Bode 2017 ; Jakesch et al. 2019 ; Shen et al. 2019 ; Avram et al. 2020 ; Hameleers et al. 2020 ; Dias et al. 2020 ; Nyhan et al. 2020 ; Bode and Vraga 2015 ; Tsang 2020 ; Vishwakarma et al. 2019 ; Yavary et al. 2020 ) and investigate fake and reliable news sources using complex networks analysis based on search engine optimization metric (Mazzeo and Rapisarda 2022 ).
The impacts of fake news have reached various areas and disciplines beyond online social networks and society (García et al. 2020 ) such as economics (Clarke et al. 2020 ; Kogan et al. 2019 ; Goldstein and Yang 2019 ), psychology (Roozenbeek et al. 2020a ; Van der Linden and Roozenbeek 2020 ; Roozenbeek and van der Linden 2019 ), political science (Valenzuela et al. 2022 ; Bringula et al. 2022 ; Ricard and Medeiros 2020 ; Van der Linden et al. 2020 ; Allcott and Gentzkow 2017 ; Grinberg et al. 2019 ; Guess et al. 2019 ; Baptista and Gradim 2020 ), health science (Alonso-Galbán and Alemañy-Castilla 2022 ; Desai et al. 2022 ; Apuke and Omar 2021 ; Escolà-Gascón 2021 ; Wang et al. 2019c ; Hartley and Vu 2020 ; Micallef et al. 2020 ; Pennycook et al. 2020b ; Sharma et al. 2020 ; Roozenbeek et al. 2020b ), environmental science (e.g., climate change) (Treen et al. 2020 ; Lutzke et al. 2019 ; Lewandowsky 2020 ; Maertens et al. 2020 ), etc.
Interesting research has been carried out to review and study the fake news issue in online social networks. Some focus not only on fake news, but also distinguish between fake news and rumor (Bondielli and Marcelloni 2019 ; Meel and Vishwakarma 2020 ), while others tackle the whole problem, from characterization to processing techniques (Shu et al. 2017 ; Guo et al. 2020 ; Zhou and Zafarani 2020 ). However, they mostly focus on studying approaches from a machine learning perspective (Bondielli and Marcelloni 2019 ), data mining perspective (Shu et al. 2017 ), crowd intelligence perspective (Guo et al. 2020 ), or knowledge-based perspective (Zhou and Zafarani 2020 ). Furthermore, most of these studies ignore at least one of the mentioned perspectives, and in many cases, they do not cover other existing detection approaches using methods such as blockchain and fact-checking, as well as analysis on metrics used for Search Engine Optimization (Mazzeo and Rapisarda 2022 ). However, in our work and to the best of our knowledge, we cover all the approaches used for fake news detection. Indeed, we investigate the proposed solutions from broader perspectives (i.e., the detection techniques that are used, as well as the different aspects and types of the information used).
Therefore, in this paper, we are highly motivated by the following facts. First, fake news detection on social media is still in the early age of development, and many challenging issues remain that require deeper investigation. Hence, it is necessary to discuss potential research directions that can improve fake news detection and mitigation tasks. However, the dynamic nature of fake news propagation through social networks further complicates matters (Sharma et al. 2019 ). False information can easily reach and impact a large number of users in a short time (Friggeri et al. 2014 ; Qian et al. 2018 ). Moreover, fact-checking organizations cannot keep up with the dynamics of propagation as they require human verification, which can hold back a timely and cost-effective response (Kim et al. 2018 ; Ruchansky et al. 2017 ; Shu et al. 2018a ).
Our work focuses primarily on understanding the “fake news” problem, its related challenges and root causes, and reviewing automatic fake news detection and mitigation methods in online social networks as addressed by researchers. The main contributions that differentiate us from other works are summarized below:
- We present the general context from which the fake news problem emerged (i.e., online deception)
- We review existing definitions of fake news, identify the terms and features most commonly used to define fake news, and categorize related works accordingly.
- We propose a fake news typology classification based on the various categorizations of fake news reported in the literature.
- We point out the most challenging factors preventing researchers from proposing highly effective solutions for automatic fake news detection in social media.
- We highlight and classify representative studies in the domain of automatic fake news detection and mitigation on online social networks including the key methods and techniques used to generate detection models.
- We discuss the key shortcomings that may inhibit the effectiveness of the proposed fake news detection methods in online social networks.
- We provide recommendations that can help address these shortcomings and improve the quality of research in this domain.
The rest of this article is organized as follows. We explain the methodology with which the studied references are collected and selected in Sect. 2 . We introduce the online deception problem in Sect. 3 . We highlight the modern-day problem of fake news in Sect. 4 , followed by challenges facing fake news detection and mitigation tasks in Sect. 5 . We provide a comprehensive literature review of the most relevant scholarly works on fake news detection in Sect. 6 . We provide a critical discussion and recommendations that may fill some of the gaps we have identified, as well as a classification of the reviewed automatic fake news detection approaches, in Sect. 7 . Finally, we provide a conclusion and propose some future directions in Sect. 8 .
Review methodology
This section introduces the systematic review methodology on which we relied to perform our study. We start with the formulation of the research questions, which allowed us to select the relevant research literature. Then, we provide the different sources of information together with the search and inclusion/exclusion criteria we used to select the final set of papers.
Research questions formulation
The research scope, research questions, and inclusion/exclusion criteria were established following an initial evaluation of the literature and the following research questions were formulated and addressed.
- RQ1: what is fake news in social media, how is it defined in the literature, what are its related concepts, and the different types of it?
- RQ2: What are the existing challenges and issues related to fake news?
- RQ3: What are the available techniques used to perform fake news detection in social media?
Sources of information
We broadly searched for journal and conference research articles, books, and magazines as a source of data to extract relevant articles. We used the main sources of scientific databases and digital libraries in our search, such as Google Scholar, 19 IEEE Xplore, 20 Springer Link, 21 ScienceDirect, 22 Scopus, 23 ACM Digital Library. 24 Also, we screened most of the related high-profile conferences such as WWW, SIGKDD, VLDB, ICDE and so on to find out the recent work.
Search criteria
We focused our research over a period of ten years, but we made sure that about two-thirds of the research papers that we considered were published in or after 2019. Additionally, we defined a set of keywords to search the above-mentioned scientific databases since we concentrated on reviewing the current state of the art in addition to the challenges and the future direction. The set of keywords includes the following terms: fake news, disinformation, misinformation, information disorder, social media, detection techniques, detection methods, survey, literature review.
Study selection, exclusion and inclusion criteria
To retrieve relevant research articles, based on our sources of information and search criteria, a systematic keyword-based search was carried out by posing different search queries, as shown in Table 1 .
List of keywords for searching relevant articles
Fake news + social media |
Fake news + disinformation |
Fake news + misinformation |
Fake news + information disorder |
Fake news + survey |
Fake news + detection methods |
Fake news + literature review |
Fake news + detection techniques |
Fake news + detection + social media |
Disinformation + misinformation + social media |
We discovered a primary list of articles. On the obtained initial list of studies, we applied a set of inclusion/exclusion criteria presented in Table 2 to select the appropriate research papers. The inclusion and exclusion principles are applied to determine whether a study should be included or not.
Inclusion and exclusion criteria
Inclusion criterion | Exclusion criterion |
---|---|
Peer-reviewed and written in the English language | Articles in a different language than English. |
Clearly describes fake news, misinformation and disinformation problems in social networks | Does not focus on fake news, misinformation, or disinformation problem in social networks |
Written by academic or industrial researchers | Short papers, posters or similar |
Have a high number of citations | |
Recent articles only (last ten years) | |
In the case of equivalent studies, the one published in the highest-rated journal or conference is selected to sustain a high-quality set of articles on which the review is conducted | Articles not following these inclusion criteria |
Articles that propose methodologies, methods, or approaches for fake news detection online social networks |
After reading the abstract, we excluded some articles that did not meet our criteria. We chose the most important research to help us understand the field. We reviewed the articles completely and found only 61 research papers that discuss the definition of the term fake news and its related concepts (see Table 4 ). We used the remaining papers to understand the field, reveal the challenges, review the detection techniques, and discuss future directions.
Classification of fake news definitions based on the used term and features
Fake news | Misinformation | Disinformation | False information | Malinformation | Information disorder | |
---|---|---|---|---|---|---|
Intent and authenticity | Shu et al. ( ), Sharma et al. ( ), Mustafaraj and Metaxas ( ), Klein and Wueller ( ), Potthast et al. ( ), Allcott and Gentzkow ( ), Zhou and Zafarani ( ), Zhang and Ghorbani ( ), Conroy et al. ( ), Celliers and Hattingh ( ), Nakov ( ), Shu et al. ( ), Tandoc Jr et al. ( ), Abu Arqoub et al. ( ),Molina et al. ( ), de Cock Buning ( ), Meel and Vishwakarma ( ) | Wu et al. ( ), Shu et al. ( ), Islam et al. ( ), Hameleers et al. ( ) | Kapantai et al. ( ), Shu et al. ( ), Shu et al. ( ),Kumar et al. ( ), Jungherr and Schroeder ( ), Starbird et al. ( ), de Cock Buning ( ), Bastick ( ), Bringula et al. ( ), Tsang ( ), Hameleers et al. ( ), Wu et al. ( ) | – | Shu et al. ( ), Di Domenico et al. ( ), Dame Adjin-Tettey ( ) | Wardle and Derakhshan ( ), Wardle Wardle ( ), Derakhshan and Wardle ( ), Shu et al. ( ) |
Intent or authenticity | Jin et al. ( ), Rubin et al. ( ), Balmas ( ),Brewer et al. ( ), Egelhofer and Lecheler ( ), Lazer et al. ( ), Allen et al. ( ), Guadagno and Guttieri ( ), Van der Linden et al. ( ), ERGA ( ) | Pennycook and Rand ( ), Shao et al. ( ), Shao et al. ( ),Micallef et al. ( ), Ha et al. ( ), Singh et al. ( ), Wu et al. ( ) | Marsden et al. ( ), Ireton and Posetti ( ), ERGA ( ), Baptista and Gradim ( ) | Habib et al. ( ) | Carmi et al. ( ) | – |
Intent and knowledge | Weiss et al. ( ) | – | Bhattacharjee et al. ( ), Khan et al. ( ) | Kumar and Shah ( ), Guo et al. ( ) | – | – |
A brief introduction of online deception
The Cambridge Online Dictionary defines Deception as “ the act of hiding the truth, especially to get an advantage .” Deception relies on peoples’ trust, doubt and strong emotions that may prevent them from thinking and acting clearly (Aïmeur et al. 2018 ). We also define it in previous work (Aïmeur et al. 2018 ) as the process that undermines the ability to consciously make decisions and take convenient actions, following personal values and boundaries. In other words, deception gets people to do things they would not otherwise do. In the context of online deception, several factors need to be considered: the deceiver, the purpose or aim of the deception, the social media service, the deception technique and the potential target (Aïmeur et al. 2018 ; Hage et al. 2021 ).
Researchers are working on developing new ways to protect users and prevent online deception (Aïmeur et al. 2018 ). Due to the sophistication of attacks, this is a complex task. Hence, malicious attackers are using more complex tools and strategies to deceive users. Furthermore, the way information is organized and exchanged in social media may lead to exposing OSN users to many risks (Aïmeur et al. 2013 ).
In fact, this field is one of the recent research areas that need collaborative efforts of multidisciplinary practices such as psychology, sociology, journalism, computer science as well as cyber-security and digital marketing (which are not yet well explored in the field of dis/mis/malinformation but relevant for future research). Moreover, Ismailov et al. ( 2020 ) analyzed the main causes that could be responsible for the efficiency gap between laboratory results and real-world implementations.
In this paper, it is not in our scope of work to review online deception state of the art. However, we think it is crucial to note that fake news, misinformation and disinformation are indeed parts of the larger landscape of online deception (Hage et al. 2021 ).
Fake news, the modern-day problem
Fake news has existed for a very long time, much before their wide circulation became facilitated by the invention of the printing press. 25 For instance, Socrates was condemned to death more than twenty-five hundred years ago under the fake news that he was guilty of impiety against the pantheon of Athens and corruption of the youth. 26 A Google Trends Analysis of the term “fake news” reveals an explosion in popularity around the time of the 2016 US presidential election. 27 Fake news detection is a problem that has recently been addressed by numerous organizations, including the European Union 28 and NATO. 29
In this section, we first overview the fake news definitions as they were provided in the literature. We identify the terms and features used in the definitions, and we classify the latter based on them. Then, we provide a fake news typology based on distinct categorizations that we propose, and we define and compare the most cited forms of one specific fake news category (i.e., the intent-based fake news category).
Definitions of fake news
“Fake news” is defined in the Collins English Dictionary as false and often sensational information disseminated under the guise of news reporting, 30 yet the term has evolved over time and has become synonymous with the spread of false information (Cooke 2017 ).
The first definition of the term fake news was provided by Allcott and Gentzkow ( 2017 ) as news articles that are intentionally and verifiably false and could mislead readers. Then, other definitions were provided in the literature, but they all agree on the authenticity of fake news to be false (i.e., being non-factual). However, they disagree on the inclusion and exclusion of some related concepts such as satire , rumors , conspiracy theories , misinformation and hoaxes from the given definition. More recently, Nakov ( 2020 ) reported that the term fake news started to mean different things to different people, and for some politicians, it even means “news that I do not like.”
Hence, there is still no agreed definition of the term “fake news.” Moreover, we can find many terms and concepts in the literature that refer to fake news (Van der Linden et al. 2020 ; Molina et al. 2021 ) (Abu Arqoub et al. 2022 ; Allen et al. 2020 ; Allcott and Gentzkow 2017 ; Shu et al. 2017 ; Sharma et al. 2019 ; Zhou and Zafarani 2020 ; Zhang and Ghorbani 2020 ; Conroy et al. 2015 ; Celliers and Hattingh 2020 ; Nakov 2020 ; Shu et al. 2020c ; Jin et al. 2016 ; Rubin et al. 2016 ; Balmas 2014 ; Brewer et al. 2013 ; Egelhofer and Lecheler 2019 ; Mustafaraj and Metaxas 2017 ; Klein and Wueller 2017 ; Potthast et al. 2017 ; Lazer et al. 2018 ; Weiss et al. 2020 ; Tandoc Jr et al. 2021 ; Guadagno and Guttieri 2021 ), disinformation (Kapantai et al. 2021 ; Shu et al. 2020a , c ; Kumar et al. 2016 ; Bhattacharjee et al. 2020 ; Marsden et al. 2020 ; Jungherr and Schroeder 2021 ; Starbird et al. 2019 ; Ireton and Posetti 2018 ), misinformation (Wu et al. 2019 ; Shu et al. 2020c ; Shao et al. 2016 , 2018b ; Pennycook and Rand 2019 ; Micallef et al. 2020 ), malinformation (Dame Adjin-Tettey 2022 ) (Carmi et al. 2020 ; Shu et al. 2020c ), false information (Kumar and Shah 2018 ; Guo et al. 2020 ; Habib et al. 2019 ), information disorder (Shu et al. 2020c ; Wardle and Derakhshan 2017 ; Wardle 2018 ; Derakhshan and Wardle 2017 ), information warfare (Guadagno and Guttieri 2021 ) and information pollution (Meel and Vishwakarma 2020 ).
There is also a remarkable amount of disagreement over the classification of the term fake news in the research literature, as well as in policy (de Cock Buning 2018 ; ERGA 2018 , 2021 ). Some consider fake news as a type of misinformation (Allen et al. 2020 ; Singh et al. 2021 ; Ha et al. 2021 ; Pennycook and Rand 2019 ; Shao et al. 2018b ; Di Domenico et al. 2021 ; Sharma et al. 2019 ; Celliers and Hattingh 2020 ; Klein and Wueller 2017 ; Potthast et al. 2017 ; Islam et al. 2020 ), others consider it as a type of disinformation (de Cock Buning 2018 ) (Bringula et al. 2022 ; Baptista and Gradim 2022 ; Tsang 2020 ; Tandoc Jr et al. 2021 ; Bastick 2021 ; Khan et al. 2019 ; Shu et al. 2017 ; Nakov 2020 ; Shu et al. 2020c ; Egelhofer and Lecheler 2019 ), while others associate the term with both disinformation and misinformation (Wu et al. 2022 ; Dame Adjin-Tettey 2022 ; Hameleers et al. 2022 ; Carmi et al. 2020 ; Allcott and Gentzkow 2017 ; Zhang and Ghorbani 2020 ; Potthast et al. 2017 ; Weiss et al. 2020 ; Tandoc Jr et al. 2021 ; Guadagno and Guttieri 2021 ). On the other hand, some prefer to differentiate fake news from both terms (ERGA 2018 ; Molina et al. 2021 ; ERGA 2021 ) (Zhou and Zafarani 2020 ; Jin et al. 2016 ; Rubin et al. 2016 ; Balmas 2014 ; Brewer et al. 2013 ).
The existing terms can be separated into two groups. The first group represents the general terms, which are information disorder , false information and fake news , each of which includes a subset of terms from the second group. The second group represents the elementary terms, which are misinformation , disinformation and malinformation . The literature agrees on the definitions of the latter group, but there is still no agreed-upon definition of the first group. In Fig. 2 , we model the relationship between the most used terms in the literature.

Modeling of the relationship between terms related to fake news
The terms most used in the literature to refer, categorize and classify fake news can be summarized and defined as shown in Table 3 , in which we capture the similarities and show the differences between the different terms based on two common key features, which are the intent and the authenticity of the news content. The intent feature refers to the intention behind the term that is used (i.e., whether or not the purpose is to mislead or cause harm), whereas the authenticity feature refers to its factual aspect. (i.e., whether the content is verifiably false or not, which we label as genuine in the second case). Some of these terms are explicitly used to refer to fake news (i.e., disinformation, misinformation and false information), while others are not (i.e., malinformation). In the comparison table, the empty dash (–) cell denotes that the classification does not apply.
A comparison between used terms based on intent and authenticity
Term | Definition | Intent | Authenticity |
---|---|---|---|
False information | Verifiably false information | – | False |
Misinformation | False information that is shared without the intention to mislead or to cause harm | Not to mislead | False |
Disinformation | False information that is shared to intentionally mislead | To mislead | False |
Malinformation | Genuine information that is shared with an intent to cause harm | To cause harm | Genuine |
In Fig. 3 , we identify the different features used in the literature to define fake news (i.e., intent, authenticity and knowledge). Hence, some definitions are based on two key features, which are authenticity and intent (i.e., news articles that are intentionally and verifiably false and could mislead readers). However, other definitions are based on either authenticity or intent. Other researchers categorize false information on the web and social media based on its intent and knowledge (i.e., when there is a single ground truth). In Table 4 , we classify the existing fake news definitions based on the used term and the used features . In the classification, the references in the cells refer to the research study in which a fake news definition was provided, while the empty dash (–) cells denote that the classification does not apply.

The features used for fake news definition
Fake news typology
Various categorizations of fake news have been provided in the literature. We can distinguish two major categories of fake news based on the studied perspective (i.e., intention or content) as shown in Fig. 4 . However, our proposed fake news typology is not about detection methods, and it is not exclusive. Hence, a given category of fake news can be described based on both perspectives (i.e., intention and content) at the same time. For instance, satire (i.e., intent-based fake news) can contain text and/or multimedia content types of data (e.g., headline, body, image, video) (i.e., content-based fake news) and so on.

Most researchers classify fake news based on the intent (Collins et al. 2020 ; Bondielli and Marcelloni 2019 ; Zannettou et al. 2019 ; Kumar et al. 2016 ; Wardle 2017 ; Shu et al. 2017 ; Kumar and Shah 2018 ) (see Sect. 4.2.2 ). However, other researchers (Parikh and Atrey 2018 ; Fraga-Lamas and Fernández-Caramés 2020 ; Hasan and Salah 2019 ; Masciari et al. 2020 ; Bakdash et al. 2018 ; Elhadad et al. 2019 ; Yang et al. 2019b ) focus on the content to categorize types of fake news through distinguishing the different formats and content types of data in the news (e.g., text and/or multimedia).
Recently, another classification was proposed by Zhang and Ghorbani ( 2020 ). It is based on the combination of content and intent to categorize fake news. They distinguish physical news content and non-physical news content from fake news. Physical content consists of the carriers and format of the news, and non-physical content consists of the opinions, emotions, attitudes and sentiments that the news creators want to express.
Content-based fake news category
According to researchers of this category (Parikh and Atrey 2018 ; Fraga-Lamas and Fernández-Caramés 2020 ; Hasan and Salah 2019 ; Masciari et al. 2020 ; Bakdash et al. 2018 ; Elhadad et al. 2019 ; Yang et al. 2019b ), forms of fake news may include false text such as hyperlinks or embedded content; multimedia such as false videos (Demuyakor and Opata 2022 ), images (Masciari et al. 2020 ; Shen et al. 2019 ), audios (Demuyakor and Opata 2022 ) and so on. Moreover, we can also find multimodal content (Shu et al. 2020a ) that is fake news articles and posts composed of multiple types of data combined together, for example, a fabricated image along with a text related to the image (Shu et al. 2020a ). In this category of fake news forms, we can mention as examples deepfake videos (Yang et al. 2019b ) and GAN-generated fake images (Zhang et al. 2019b ), which are artificial intelligence-based machine-generated fake content that are hard for unsophisticated social network users to identify.
The effects of these forms of fake news content vary on the credibility assessment, as well as sharing intentions which influences the spread of fake news on OSNs. For instance, people with little knowledge about the issue compared to those who are strongly concerned about the key issue of fake news tend to be easier to convince that the misleading or fake news is real, especially when shared via a video modality as compared to the text or the audio modality (Demuyakor and Opata 2022 ).
Intent-based Fake News Category
The most often mentioned and discussed forms of fake news according to researchers in this category include but are not restricted to clickbait , hoax , rumor , satire , propaganda , framing , conspiracy theories and others. In the following subsections, we explain these types of fake news as they were defined in the literature and undertake a brief comparison between them as depicted in Table 5 . The following are the most cited forms of intent-based types of fake news, and their comparison is based on what we suspect are the most common criteria mentioned by researchers.
A comparison between the different types of intent-based fake news
Intent to deceive | Propagation | Negative Impact | Goal | |
---|---|---|---|---|
Clickbait | High | Slow | Low | Popularity, Profit |
Hoax | High | Fast | Low | Other |
Rumor | High | Fast | High | Other |
Satire | Low | Slow | Low | Popularity, Other |
Propaganda | High | Fast | High | Popularity |
Framing | High | Fast | Low | Other |
Conspiracy theory | High | Fast | High | Other |
Clickbait refers to misleading headlines and thumbnails of content on the web (Zannettou et al. 2019 ) that tend to be fake stories with catchy headlines aimed at enticing the reader to click on a link (Collins et al. 2020 ). This type of fake news is considered to be the least severe type of false information because if a user reads/views the whole content, it is possible to distinguish if the headline and/or the thumbnail was misleading (Zannettou et al. 2019 ). However, the goal behind using clickbait is to increase the traffic to a website (Zannettou et al. 2019 ).
A hoax is a false (Zubiaga et al. 2018 ) or inaccurate (Zannettou et al. 2019 ) intentionally fabricated (Collins et al. 2020 ) news story used to masquerade the truth (Zubiaga et al. 2018 ) and is presented as factual (Zannettou et al. 2019 ) to deceive the public or audiences (Collins et al. 2020 ). This category is also known either as half-truth or factoid stories (Zannettou et al. 2019 ). Popular examples of hoaxes are stories that report the false death of celebrities (Zannettou et al. 2019 ) and public figures (Collins et al. 2020 ). Recently, hoaxes about the COVID-19 have been circulating through social media.
The term rumor refers to ambiguous or never confirmed claims (Zannettou et al. 2019 ) that are disseminated with a lack of evidence to support them (Sharma et al. 2019 ). This kind of information is widely propagated on OSNs (Zannettou et al. 2019 ). However, they are not necessarily false and may turn out to be true (Zubiaga et al. 2018 ). Rumors originate from unverified sources but may be true or false or remain unresolved (Zubiaga et al. 2018 ).
Satire refers to stories that contain a lot of irony and humor (Zannettou et al. 2019 ). It presents stories as news that might be factually incorrect, but the intent is not to deceive but rather to call out, ridicule, or to expose behavior that is shameful, corrupt, or otherwise “bad” (Golbeck et al. 2018 ). This is done with a fabricated story or by exaggerating the truth reported in mainstream media in the form of comedy (Collins et al. 2020 ). The intent behind satire seems kind of legitimate and many authors (such as Wardle (Wardle 2017 )) do include satire as a type of fake news as there is no intention to cause harm but it has the potential to mislead or fool people.
Also, Golbeck et al. ( 2018 ) mention that there is a spectrum from fake to satirical news that they found to be exploited by many fake news sites. These sites used disclaimers at the bottom of their webpages to suggest they were “satirical” even when there was nothing satirical about their articles, to protect them from accusations about being fake. The difference with a satirical form of fake news is that the authors or the host present themselves as a comedian or as an entertainer rather than a journalist informing the public (Collins et al. 2020 ). However, most audiences believed the information passed in this satirical form because the comedian usually projects news from mainstream media and frames them to suit their program (Collins et al. 2020 ).
Propaganda refers to news stories created by political entities to mislead people. It is a special instance of fabricated stories that aim to harm the interests of a particular party and, typically, has a political context (Zannettou et al. 2019 ). Propaganda was widely used during both World Wars (Collins et al. 2020 ) and during the Cold War (Zannettou et al. 2019 ). It is a consequential type of false information as it can change the course of human history (e.g., by changing the outcome of an election) (Zannettou et al. 2019 ). States are the main actors of propaganda. Recently, propaganda has been used by politicians and media organizations to support a certain position or view (Collins et al. 2020 ). Online astroturfing can be an example of the tools used for the dissemination of propaganda. It is a covert manipulation of public opinion (Peng et al. 2017 ) that aims to make it seem that many people share the same opinion about something. Astroturfing can affect different domains of interest, based on which online astroturfing can be mainly divided into political astroturfing, corporate astroturfing and astroturfing in e-commerce or online services (Mahbub et al. 2019 ). Propaganda types of fake news can be debunked with manual fact-based detection models such as the use of expert-based fact-checkers (Collins et al. 2020 ).
Framing refers to employing some aspect of reality to make content more visible, while the truth is concealed (Collins et al. 2020 ) to deceive and misguide readers. People will understand certain concepts based on the way they are coined and invented. An example of framing was provided by Collins et al. ( 2020 ): “suppose a leader X says “I will neutralize my opponent” simply meaning he will beat his opponent in a given election. Such a statement will be framed such as “leader X threatens to kill Y” and this framed statement provides a total misrepresentation of the original meaning.
Conspiracy Theories
Conspiracy theories refer to the belief that an event is the result of secret plots generated by powerful conspirators. Conspiracy belief refers to people’s adoption and belief of conspiracy theories, and it is associated with psychological, political and social factors (Douglas et al. 2019 ). Conspiracy theories are widespread in contemporary democracies (Sutton and Douglas 2020 ), and they have major consequences. For instance, lately and during the COVID-19 pandemic, conspiracy theories have been discussed from a public health perspective (Meese et al. 2020 ; Allington et al. 2020 ; Freeman et al. 2020 ).
Comparison Between Most Popular Intent-based Types of Fake News
Following a review of the most popular intent-based types of fake news, we compare them as shown in Table 5 based on the most common criteria mentioned by researchers in their definitions as listed below.
- the intent behind the news, which refers to whether a given news type was mainly created to intentionally deceive people or not (e.g., humor, irony, entertainment, etc.);
- the way that the news propagates through OSN, which determines the nature of the propagation of each type of fake news and this can be either fast or slow propagation;
- the severity of the impact of the news on OSN users, which refers to whether the public has been highly impacted by the given type of fake news; the mentioned impact of each fake news type is mainly the proportion of the negative impact;
- and the goal behind disseminating the news, which can be to gain popularity for a particular entity (e.g., political party), for profit (e.g., lucrative business), or other reasons such as humor and irony in the case of satire, spreading panic or anger, and manipulating the public in the case of hoaxes, made-up stories about a particular person or entity in the case of rumors, and misguiding readers in the case of framing.
However, the comparison provided in Table 5 is deduced from the studied research papers; it is our point of view, which is not based on empirical data.
We suspect that the most dangerous types of fake news are the ones with high intention to deceive the public, fast propagation through social media, high negative impact on OSN users, and complicated hidden goals and agendas. However, while the other types of fake news are less dangerous, they should not be ignored.
Moreover, it is important to highlight that the existence of the overlap in the types of fake news mentioned above has been proven, thus it is possible to observe false information that may fall within multiple categories (Zannettou et al. 2019 ). Here, we provide two examples by Zannettou et al. ( 2019 ) to better understand possible overlaps: (1) a rumor may also use clickbait techniques to increase the audience that will read the story; and (2) propaganda stories, as a special instance of a framing story.
Challenges related to fake news detection and mitigation
To alleviate fake news and its threats, it is crucial to first identify and understand the factors involved that continue to challenge researchers. Thus, the main question is to explore and investigate the factors that make it easier to fall for manipulated information. Despite the tremendous progress made in alleviating some of the challenges in fake news detection (Sharma et al. 2019 ; Zhou and Zafarani 2020 ; Zhang and Ghorbani 2020 ; Shu et al. 2020a ), much more work needs to be accomplished to address the problem effectively.
In this section, we discuss several open issues that have been making fake news detection in social media a challenging problem. These issues can be summarized as follows: content-based issues (i.e., deceptive content that resembles the truth very closely), contextual issues (i.e., lack of user awareness, social bots spreaders of fake content, and OSN’s dynamic natures that leads to the fast propagation), as well as the issue of existing datasets (i.e., there still no one size fits all benchmark dataset for fake news detection). These various aspects have proven (Shu et al. 2017 ) to have a great impact on the accuracy of fake news detection approaches.
Content-based issue, deceptive content
Automatic fake news detection remains a huge challenge, primarily because the content is designed in a way that it closely resembles the truth. Besides, most deceivers choose their words carefully and use their language strategically to avoid being caught. Therefore, it is often hard to determine its veracity by AI without the reliance on additional information from third parties such as fact-checkers.
Abdullah-All-Tanvir et al. ( 2020 ) reported that fake news tends to have more complicated stories and hardly ever make any references. It is more likely to contain a greater number of words that express negative emotions. This makes it so complicated that it becomes impossible for a human to manually detect the credibility of this content. Therefore, detecting fake news on social media is quite challenging. Moreover, fake news appears in multiple types and forms, which makes it hard and challenging to define a single global solution able to capture and deal with the disseminated content. Consequently, detecting false information is not a straightforward task due to its various types and forms Zannettou et al. ( 2019 ).
Contextual issues
Contextual issues are challenges that we suspect may not be related to the content of the news but rather they are inferred from the context of the online news post (i.e., humans are the weakest factor due to lack of user awareness, social bots spreaders, dynamic nature of online social platforms and fast propagation of fake news).
Humans are the weakest factor due to the lack of awareness
Recent statistics 31 show that the percentage of unintentional fake news spreaders (people who share fake news without the intention to mislead) over social media is five times higher than intentional spreaders. Moreover, another recent statistic 32 shows that the percentage of people who were confident about their ability to discern fact from fiction is ten times higher than those who were not confident about the truthfulness of what they are sharing. As a result, we can deduce the lack of human awareness about the ascent of fake news.
Public susceptibility and lack of user awareness (Sharma et al. 2019 ) have always been the most challenging problem when dealing with fake news and misinformation. This is a complex issue because many people believe almost everything on the Internet and the ones who are new to digital technology or have less expertise may be easily fooled (Edgerly et al. 2020 ).
Moreover, it has been widely proven (Metzger et al. 2020 ; Edgerly et al. 2020 ) that people are often motivated to support and accept information that goes with their preexisting viewpoints and beliefs, and reject information that does not fit in as well. Hence, Shu et al. ( 2017 ) illustrate an interesting correlation between fake news spread and psychological and cognitive theories. They further suggest that humans are more likely to believe information that confirms their existing views and ideological beliefs. Consequently, they deduce that humans are naturally not very good at differentiating real information from fake information.
Recent research by Giachanou et al. ( 2020 ) studies the role of personality and linguistic patterns in discriminating between fake news spreaders and fact-checkers. They classify a user as a potential fact-checker or a potential fake news spreader based on features that represent users’ personality traits and linguistic patterns used in their tweets. They show that leveraging personality traits and linguistic patterns can improve the performance in differentiating between checkers and spreaders.
Furthermore, several researchers studied the prevalence of fake news on social networks during (Allcott and Gentzkow 2017 ; Grinberg et al. 2019 ; Guess et al. 2019 ; Baptista and Gradim 2020 ) and after (Garrett and Bond 2021 ) the 2016 US presidential election and found that individuals most likely to engage with fake news sources were generally conservative-leaning, older, and highly engaged with political news.
Metzger et al. ( 2020 ) examine how individuals evaluate the credibility of biased news sources and stories. They investigate the role of both cognitive dissonance and credibility perceptions in selective exposure to attitude-consistent news information. They found that online news consumers tend to perceive attitude-consistent news stories as more accurate and more credible than attitude-inconsistent stories.
Similarly, Edgerly et al. ( 2020 ) explore the impact of news headlines on the audience’s intent to verify whether given news is true or false. They concluded that participants exhibit higher intent to verify the news only when they believe the headline to be true, which is predicted by perceived congruence with preexisting ideological tendencies.
Luo et al. ( 2022 ) evaluate the effects of endorsement cues in social media on message credibility and detection accuracy. Results showed that headlines associated with a high number of likes increased credibility, thereby enhancing detection accuracy for real news but undermining accuracy for fake news. Consequently, they highlight the urgency of empowering individuals to assess both news veracity and endorsement cues appropriately on social media.
Moreover, misinformed people are a greater problem than uninformed people (Kuklinski et al. 2000 ), because the former hold inaccurate opinions (which may concern politics, climate change, medicine) that are harder to correct. Indeed, people find it difficult to update their misinformation-based beliefs even after they have been proved to be false (Flynn et al. 2017 ). Moreover, even if a person has accepted the corrected information, his/her belief may still affect their opinion (Nyhan and Reifler 2015 ).
Falling for disinformation may also be explained by a lack of critical thinking and of the need for evidence that supports information (Vilmer et al. 2018 ; Badawy et al. 2019 ). However, it is also possible that people choose misinformation because they engage in directionally motivated reasoning (Badawy et al. 2019 ; Flynn et al. 2017 ). Online clients are normally vulnerable and will, in general, perceive web-based networking media as reliable, as reported by Abdullah-All-Tanvir et al. ( 2019 ), who propose to mechanize fake news recognition.
It is worth noting that in addition to bots causing the outpouring of the majority of the misrepresentations, specific individuals are also contributing a large share of this issue (Abdullah-All-Tanvir et al. 2019 ). Furthermore, Vosoughi et al. (Vosoughi et al. 2018 ) found that contrary to conventional wisdom, robots have accelerated the spread of real and fake news at the same rate, implying that fake news spreads more than the truth because humans, not robots, are more likely to spread it.
In this case, verified users and those with numerous followers were not necessarily responsible for spreading misinformation of the corrupted posts (Abdullah-All-Tanvir et al. 2019 ).
Viral fake news can cause much havoc to our society. Therefore, to mitigate the negative impact of fake news, it is important to analyze the factors that lead people to fall for misinformation and to further understand why people spread fake news (Cheng et al. 2020 ). Measuring the accuracy, credibility, veracity and validity of news contents can also be a key countermeasure to consider.
Social bots spreaders
Several authors (Shu et al. 2018b , 2017 ; Shi et al. 2019 ; Bessi and Ferrara 2016 ; Shao et al. 2018a ) have also shown that fake news is likely to be created and spread by non-human accounts with similar attributes and structure in the network, such as social bots (Ferrara et al. 2016 ). Bots (short for software robots) exist since the early days of computers. A social bot is a computer algorithm that automatically produces content and interacts with humans on social media, trying to emulate and possibly alter their behavior (Ferrara et al. 2016 ). Although they are designed to provide a useful service, they can be harmful, for example when they contribute to the spread of unverified information or rumors (Ferrara et al. 2016 ). However, it is important to note that bots are simply tools created and maintained by humans for some specific hidden agendas.
Social bots tend to connect with legitimate users instead of other bots. They try to act like a human with fewer words and fewer followers on social media. This contributes to the forwarding of fake news (Jiang et al. 2019 ). Moreover, there is a difference between bot-generated and human-written clickbait (Le et al. 2019 ).
Many researchers have addressed ways of identifying and analyzing possible sources of fake news spread in social media. Recent research by Shu et al. ( 2020a ) describes social bots use of two strategies to spread low-credibility content. First, they amplify interactions with content as soon as it is created to make it look legitimate and to facilitate its spread across social networks. Next, they try to increase public exposure to the created content and thus boost its perceived credibility by targeting influential users that are more likely to believe disinformation in the hope of getting them to “repost” the fabricated content. They further discuss the social bot detection systems taxonomy proposed by Ferrara et al. ( 2016 ) which divides bot detection methods into three classes: (1) graph-based, (2) crowdsourcing and (3) feature-based social bot detection methods.
Similarly, Shao et al. ( 2018a ) examine social bots and how they promote the spread of misinformation through millions of Twitter posts during and following the 2016 US presidential campaign. They found that social bots played a disproportionate role in spreading articles from low-credibility sources by amplifying such content in the early spreading moments and targeting users with many followers through replies and mentions to expose them to this content and induce them to share it.
Ismailov et al. ( 2020 ) assert that the techniques used to detect bots depend on the social platform and the objective. They note that a malicious bot designed to make friends with as many accounts as possible will require a different detection approach than a bot designed to repeatedly post links to malicious websites. Therefore, they identify two models for detecting malicious accounts, each using a different set of features. Social context models achieve detection by examining features related to an account’s social presence including features such as relationships to other accounts, similarities to other users’ behaviors, and a variety of graph-based features. User behavior models primarily focus on features related to an individual user’s behavior, such as frequency of activities (e.g., number of tweets or posts per time interval), patterns of activity and clickstream sequences.
Therefore, it is crucial to consider bot detection techniques to distinguish bots from normal users to better leverage user profile features to detect fake news.
However, there is also another “bot-like” strategy that aims to massively promote disinformation and fake content in social platforms, which is called bot farms or also troll farms. It is not social bots, but it is a group of organized individuals engaging in trolling or bot-like promotion of narratives in a coordinated fashion (Wardle 2018 ) hired to massively spread fake news or any other harmful content. A prominent troll farm example is the Russia-based Internet Research Agency (IRA), which disseminated inflammatory content online to influence the outcome of the 2016 U.S. presidential election. 33 As a result, Twitter suspended accounts connected to the IRA and deleted 200,000 tweets from Russian trolls (Jamieson 2020 ). Another example to mention in this category is review bombing (Moro and Birt 2022 ). Review bombing refers to coordinated groups of people massively performing the same negative actions online (e.g., dislike, negative review/comment) on an online video, game, post, product, etc., in order to reduce its aggregate review score. The review bombers can be both humans and bots coordinated in order to cause harm and mislead people by falsifying facts.
Dynamic nature of online social platforms and fast propagation of fake news
Sharma et al. ( 2019 ) affirm that the fast proliferation of fake news through social networks makes it hard and challenging to assess the information’s credibility on social media. Similarly, Qian et al. ( 2018 ) assert that fake news and fabricated content propagate exponentially at the early stage of its creation and can cause a significant loss in a short amount of time (Friggeri et al. 2014 ) including manipulating the outcome of political events (Liu and Wu 2018 ; Bessi and Ferrara 2016 ).
Moreover, while analyzing the way source and promoters of fake news operate over the web through multiple online platforms, Zannettou et al. ( 2019 ) discovered that false information is more likely to spread across platforms (18% appearing on multiple platforms) compared to real information (11%).
Furthermore, recently, Shu et al. ( 2020c ) attempted to understand the propagation of disinformation and fake news in social media and found that such content is produced and disseminated faster and easier through social media because of the low barriers that prevent doing so. Similarly, Shu et al. ( 2020b ) studied hierarchical propagation networks for fake news detection. They performed a comparative analysis between fake and real news from structural, temporal and linguistic perspectives. They demonstrated the potential of using these features to detect fake news and they showed their effectiveness for fake news detection as well.
Lastly, Abdullah-All-Tanvir et al. ( 2020 ) note that it is almost impossible to manually detect the sources and authenticity of fake news effectively and efficiently, due to its fast circulation in such a small amount of time. Therefore, it is crucial to note that the dynamic nature of the various online social platforms, which results in the continued rapid and exponential propagation of such fake content, remains a major challenge that requires further investigation while defining innovative solutions for fake news detection.
Datasets issue
The existing approaches lack an inclusive dataset with derived multidimensional information to detect fake news characteristics to achieve higher accuracy of machine learning classification model performance (Nyow and Chua 2019 ). These datasets are primarily dedicated to validating the machine learning model and are the ultimate frame of reference to train the model and analyze its performance. Therefore, if a researcher evaluates their model based on an unrepresentative dataset, the validity and the efficiency of the model become questionable when it comes to applying the fake news detection approach in a real-world scenario.
Moreover, several researchers (Shu et al. 2020d ; Wang et al. 2020 ; Pathak and Srihari 2019 ; Przybyla 2020 ) believe that fake news is diverse and dynamic in terms of content, topics, publishing methods and media platforms, and sophisticated linguistic styles geared to emulate true news. Consequently, training machine learning models on such sophisticated content requires large-scale annotated fake news data that are difficult to obtain (Shu et al. 2020d ).
Therefore, datasets are also a great topic to work on to enhance data quality and have better results while defining our solutions. Adversarial learning techniques (e.g., GAN, SeqGAN) can be used to provide machine-generated data that can be used to train deeper models and build robust systems to detect fake examples from the real ones. This approach can be used to counter the lack of datasets and the scarcity of data available to train models.
Fake news detection literature review
Fake news detection in social networks is still in the early stage of development and there are still challenging issues that need further investigation. This has become an emerging research area that is attracting huge attention.
There are various research studies on fake news detection in online social networks. Few of them have focused on the automatic detection of fake news using artificial intelligence techniques. In this section, we review the existing approaches used in automatic fake news detection, as well as the techniques that have been adopted. Then, a critical discussion built on a primary classification scheme based on a specific set of criteria is also emphasized.
Categories of fake news detection
In this section, we give an overview of most of the existing automatic fake news detection solutions adopted in the literature. A recent classification by Sharma et al. ( 2019 ) uses three categories of fake news identification methods. Each category is further divided based on the type of existing methods (i.e., content-based, feedback-based and intervention-based methods). However, a review of the literature for fake news detection in online social networks shows that the existing studies can be classified into broader categories based on two major aspects that most authors inspect and make use of to define an adequate solution. These aspects can be considered as major sources of extracted information used for fake news detection and can be summarized as follows: the content-based (i.e., related to the content of the news post) and the contextual aspect (i.e., related to the context of the news post).
Consequently, the studies we reviewed can be classified into three different categories based on the two aspects mentioned above (the third category is hybrid). As depicted in Fig. 5 , fake news detection solutions can be categorized as news content-based approaches, the social context-based approaches that can be divided into network and user-based approaches, and hybrid approaches. The latter combines both content-based and contextual approaches to define the solution.

Classification of fake news detection approaches
News Content-based Category
News content-based approaches are fake news detection approaches that use content information (i.e., information extracted from the content of the news post) and that focus on studying and exploiting the news content in their proposed solutions. Content refers to the body of the news, including source, headline, text and image-video, which can reflect subtle differences.
Researchers of this category rely on content-based detection cues (i.e., text and multimedia-based cues), which are features extracted from the content of the news post. Text-based cues are features extracted from the text of the news, whereas multimedia-based cues are features extracted from the images and videos attached to the news. Figure 6 summarizes the most widely used news content representation (i.e., text and multimedia/images) and detection techniques (i.e., machine learning (ML), deep Learning (DL), natural language processing (NLP), fact-checking, crowdsourcing (CDS) and blockchain (BKC)) in news content-based category of fake news detection approaches. Most of the reviewed research works based on news content for fake news detection rely on the text-based cues (Kapusta et al. 2019 ; Kaur et al. 2020 ; Vereshchaka et al. 2020 ; Ozbay and Alatas 2020 ; Wang 2017 ; Nyow and Chua 2019 ; Hosseinimotlagh and Papalexakis 2018 ; Abdullah-All-Tanvir et al. 2019 , 2020 ; Mahabub 2020 ; Bahad et al. 2019 ; Hiriyannaiah et al. 2020 ) extracted from the text of the news content including the body of the news and its headline. However, a few researchers such as Vishwakarma et al. ( 2019 ) and Amri et al. ( 2022 ) try to recognize text from the associated image.

News content-based category: news content representation and detection techniques
Most researchers of this category rely on artificial intelligence (AI) techniques (such as ML, DL and NLP models) to improve performance in terms of prediction accuracy. Others use different techniques such as fact-checking, crowdsourcing and blockchain. Specifically, the AI- and ML-based approaches in this category are trying to extract features from the news content, which they use later for content analysis and training tasks. In this particular case, the extracted features are the different types of information considered to be relevant for the analysis. Feature extraction is considered as one of the best techniques to reduce data size in automatic fake news detection. This technique aims to choose a subset of features from the original set to improve classification performance (Yazdi et al. 2020 ).
Table 6 lists the distinct features and metadata, as well as the used datasets in the news content-based category of fake news detection approaches.
The features and datasets used in the news content-based approaches
Feature and metadata | Datasets | Reference |
---|---|---|
The average number of words in sentences, number of stop words, the sentiment rate of the news measured through the difference between the number of positive and negative words in the article | Getting real about fake news , Gathering mediabiasfactcheck , KaiDMML FakeNewsNet , Real news for Oct-Dec 2016 | Kapusta et al. ( ) |
The length distribution of the title, body and label of the article | News trends, Kaggle, Reuters | Kaur et al. ( ) |
Sociolinguistic, historical, cultural, ideological and syntactical features attached to particular words, phrases and syntactical constructions | FakeNewsNet | Vereshchaka et al. ( ) |
Term frequency | BuzzFeed political news, Random political news, ISOT fake news | Ozbay and Alatas ( ) |
The statement, speaker, context, label, justification | POLITIFACT, LIAR | Wang ( ) |
Spatial vicinity of each word, spatial/contextual relations between terms, and latent relations between terms and articles | Kaggle fake news dataset | Hosseinimotlagh and Papalexakis ( ) |
Word length, the count of words in a tweeted statement | Twitter dataset, Chile earthquake 2010 datasets | Abdullah-All-Tanvir et al. ( ) |
The number of words that express negative emotions | Twitter dataset | Abdullah-All-Tanvir et al. ( ) |
Labeled data | BuzzFeed , PolitiFact | Mahabub ( ) |
The relationship between the news article headline and article body. The biases of a written news article | Kaggle: real_or_fake , Fake news detection | Bahad et al. ( ) |
Historical data. The topic and sentiment associated with content textual. The subject and context of the text, semantic knowledge of the content | Facebook dataset | Del Vicario et al. ( ) |
The veracity of image text. The credibility of the top 15 Google search results related to the image text | Google images, the Onion, Kaggle | Vishwakarma et al. ( ) |
Topic modeling of text and the associated image of the online news | Twitter dataset , Weibo | Amri et al. ( ) |
a https://www.kaggle.com/anthonyc1/gathering-real-news-for-oct-dec-2016 , last access date: 26-12-2022
b https://mediabiasfactcheck.com/ , last access date: 26-12-2022
c https://github.com/KaiDMML/FakeNewsNet , last access date: 26-12-2022
d https://www.kaggle.com/anthonyc1/gathering-real-news-for-oct-dec-2016 , last access date: 26-12-2022
e https://www.cs.ucsb.edu/~william/data/liar_dataset.zip , last access date: 26-12-2022
f https://www.kaggle.com/mrisdal/fake-news , last access date: 26-12-2022
g https://github.com/BuzzFeedNews/2016-10-facebook-fact-check , last access date: 26-12-2022
h https://www.politifact.com/subjects/fake-news/ , last access date: 26-12-2022
i https://www.kaggle.com/rchitic17/real-or-fake , last access date: 26-12-2022
j https://www.kaggle.com/jruvika/fake-news-detection , last access date: 26-12-2022
k https://github.com/MKLab-ITI/image-verification-corpus , last access date: 26-12-2022
l https://drive.google.com/file/d/14VQ7EWPiFeGzxp3XC2DeEHi-BEisDINn/view , last access date: 26-12-2022
Social Context-based Category
Unlike news content-based solutions, the social context-based approaches capture the skeptical social context of the online news (Zhang and Ghorbani 2020 ) rather than focusing on the news content. The social context-based category contains fake news detection approaches that use the contextual aspects (i.e., information related to the context of the news post). These aspects are based on social context and they offer additional information to help detect fake news. They are the surrounding data outside of the fake news article itself, where they can be an essential part of automatic fake news detection. Some useful examples of contextual information may include checking if the news itself and the source that published it are credible, checking the date of the news or the supporting resources, and checking if any other online news platforms are reporting the same or similar stories (Zhang and Ghorbani 2020 ).
Social context-based aspects can be classified into two subcategories, user-based and network-based, and they can be used for context analysis and training tasks in the case of AI- and ML-based approaches. User-based aspects refer to information captured from OSN users such as user profile information (Shu et al. 2019b ; Wang et al. 2019c ; Hamdi et al. 2020 ; Nyow and Chua 2019 ; Jiang et al. 2019 ) and user behavior (Cardaioli et al. 2020 ) such as user engagement (Uppada et al. 2022 ; Jiang et al. 2019 ; Shu et al. 2018b ; Nyow and Chua 2019 ) and response (Zhang et al. 2019a ; Qian et al. 2018 ). Meanwhile, network-based aspects refer to information captured from the properties of the social network where the fake content is shared and disseminated such as news propagation path (Liu and Wu 2018 ; Wu and Liu 2018 ) (e.g., propagation times and temporal characteristics of propagation), diffusion patterns (Shu et al. 2019a ) (e.g., number of retweets, shares), as well as user relationships (Mishra 2020 ; Hamdi et al. 2020 ; Jiang et al. 2019 ) (e.g., friendship status among users).
Figure 7 summarizes some of the most widely adopted social context representations, as well as the most used detection techniques (i.e., AI, ML, DL, fact-checking and blockchain), in the social context-based category of approaches.

Social context-based category: social context representation and detection techniques
Table 7 lists the distinct features and metadata, the adopted detection cues, as well as the used datasets, in the context-based category of fake news detection approaches.
The features, detection cues and datasets used int the social context-based approaches
Feature and metadata | Detection cues | Datasets | Reference |
---|---|---|---|
Users’ sharing behaviors, explicit and implicit profile features | User-based: user profile information | FakeNewsNet | Shu et al. ( ) |
Users’ trust level, explicit and implicit profile features of “experienced” users who can recognize fake news items as false and “naive” users who are more likely to believe fake news | User-based: user engagement | FakeNewsNet, BuzzFeed, PolitiFact | Shu et al. ( ) |
Users’ replies on fake content, the reply stances | User-based: user response | RumourEval, PHEME | Zhang et al. ( ) |
Historical user responses to previous articles | User-based: user response | Weibo, Twitter dataset | Qian et al. ( ) |
Speaker name, job title, political party affiliation, etc. | User-based: user profile information | LIAR | Wang et al. ( ) |
Latent relationships among users, the influence of the users with high prestige on the other users | Networks-based: user relationships | Twitter15 and Twitter16 | Mishra ( ) |
The inherent tri-relationships among publishers, news items and users (i.e., publisher-news relations and user-news interactions) | Networks-based: diffusion patterns | FakeNewsNet | Shu et al. ( ) |
Propagation paths of news stories constructed from the retweets of source tweets | Networks-based: news propagation path | Weibo, Twitter15, Twitter16 | Liu and Wu ( ) |
The propagation of messages in a social network | Networks-based: news propagation path | Twitter dataset | Wu and Liu ( ) |
Spatiotemporal information (i.e., location, timestamps of user engagements), user’s Twitter profile, the user engagement to both fake and real news | User-based: user engagement | FakeNewsNet, PolitiFact, GossipCop, Twitter | Nyow and Chua ( ) |
The credibility of information sources, characteristics of the user, and their social graph | User and network-based: user profile information and user relationships | Ego-Twitter | Hamdi et al. ( ) |
Number of follows and followers on social media (user followee/follower, The friendship network), users’ similarities | User and network-based: user profile information, user engagement and user relationships | FakeNewsNet | Jiang et al. ( ) |
a https://www.dropbox.com/s/7ewzdrbelpmrnxu/rumdetect2017.zip , last access date: 26-12-2022 b https://snap.stanford.edu/data/ego-Twitter.html , last access date: 26-12-2022
Hybrid approaches
Most researchers are focusing on employing a specific method rather than a combination of both content- and context-based methods. This is because some of them (Wu and Rao 2020 ) believe that there still some challenging limitations in the traditional fusion strategies due to existing feature correlations and semantic conflicts. For this reason, some researchers focus on extracting content-based information, while others are capturing some social context-based information for their proposed approaches.
However, it has proven challenging to successfully automate fake news detection based on just a single type of feature (Ruchansky et al. 2017 ). Therefore, recent directions tend to do a mixture by using both news content-based and social context-based approaches for fake news detection.
Table 8 lists the distinct features and metadata, as well as the used datasets, in the hybrid category of fake news detection approaches.
The features and datasets used in the hybrid approaches
Feature and metadata | Datasets | Reference |
---|---|---|
Features and textual metadata of the news content: title, content, date, source, location | SOT fake news dataset, LIAR dataset and FA-KES dataset | Elhadad et al. ( ) |
Spatiotemporal information (i.e., location, timestamps of user engagements), user’s Twitter profile, the user engagement to both fake and real news | FakeNewsNet, PolitiFact, GossipCop, Twitter | Nyow and Chua ( ) |
The domains and reputations of the news publishers. The important terms of each news and their word embeddings and topics. Shares, reactions and comments | BuzzFeed | Xu et al. ( ) |
Shares and propagation path of the tweeted content. A set of metrics comprising of created discussions such as the increase in authors, attention level, burstiness level, contribution sparseness, author interaction, author count and the average length of discussions | Twitter dataset | Aswani et al. ( ) |
Features extracted from the evolution of news and features from the users involved in the news spreading: The news veracity, the credibility of news spreaders, and the frequency of exposure to the same piece of news | Twitter dataset | Previti et al. ( ) |
Similar semantics and conflicting semantics between posts and comments | RumourEval, PHEME | Wu and Rao ( ) |
Information from the publisher, including semantic and emotional information in news content. Semantic and emotional information from users. The resultant latent representations from news content and user comments | Guo et al. ( ) | |
Relationships between news articles, creators and subjects | PolitiFact | Zhang et al. ( ) |
Source domains of the news article, author names | George McIntire fake news dataset | Deepak and Chitturi ( ) |
The news content, social context and spatiotemporal information. Synthetic user engagements generated from historical temporal user engagement patterns | FakeNewsNet | Shu et al. ( ) |
The news content, social reactions, statements, the content and language of posts, the sharing and dissemination among users, content similarity, stance, sentiment score, headline, named entity, news sharing, credibility history, tweet comments | SHPT, PolitiFact | Wang et al. ( ) |
The source of the news, its headline, its author, its publication time, the adherence of a news source to a particular party, likes, shares, replies, followers-followees and their activities | NELA-GT-2019, Fakeddit | Raza and Ding ( ) |
Fake news detection techniques
Another vision for classifying automatic fake news detection is to look at techniques used in the literature. Hence, we classify the detection methods based on the techniques into three groups:
- Human-based techniques: This category mainly includes the use of crowdsourcing and fact-checking techniques, which rely on human knowledge to check and validate the veracity of news content.
- Artificial Intelligence-based techniques: This category includes the most used AI approaches for fake news detection in the literature. Specifically, these are the approaches in which researchers use classical ML, deep learning techniques such as convolutional neural network (CNN), recurrent neural network (RNN), as well as natural language processing (NLP).
- Blockchain-based techniques: This category includes solutions using blockchain technology to detect and mitigate fake news in social media by checking source reliability and establishing the traceability of the news content.
Human-based Techniques
One specific research direction for fake news detection consists of using human-based techniques such as crowdsourcing (Pennycook and Rand 2019 ; Micallef et al. 2020 ) and fact-checking (Vlachos and Riedel 2014 ; Chung and Kim 2021 ; Nyhan et al. 2020 ) techniques.
These approaches can be considered as low computational requirement techniques since both rely on human knowledge and expertise for fake news detection. However, fake news identification cannot be addressed solely through human force since it demands a lot of effort in terms of time and cost, and it is ineffective in terms of preventing the fast spread of fake content.
Crowdsourcing. Crowdsourcing approaches (Kim et al. 2018 ) are based on the “wisdom of the crowds” (Collins et al. 2020 ) for fake content detection. These approaches rely on the collective contributions and crowd signals (Tschiatschek et al. 2018 ) of a group of people for the aggregation of crowd intelligence to detect fake news (Tchakounté et al. 2020 ) and to reduce the spread of misinformation on social media (Pennycook and Rand 2019 ; Micallef et al. 2020 ).
Micallef et al. ( 2020 ) highlight the role of the crowd in countering misinformation. They suspect that concerned citizens (i.e., the crowd), who use platforms where disinformation appears, can play a crucial role in spreading fact-checking information and in combating the spread of misinformation.
Recently Tchakounté et al. ( 2020 ) proposed a voting system as a new method of binary aggregation of opinions of the crowd and the knowledge of a third-party expert. The aggregator is based on majority voting on the crowd side and weighted averaging on the third-party site.
Similarly, Huffaker et al. ( 2020 ) propose a crowdsourced detection of emotionally manipulative language. They introduce an approach that transforms classification problems into a comparison task to mitigate conflation content by allowing the crowd to detect text that uses manipulative emotional language to sway users toward positions or actions. The proposed system leverages anchor comparison to distinguish between intrinsically emotional content and emotionally manipulative language.
La Barbera et al. ( 2020 ) try to understand how people perceive the truthfulness of information presented to them. They collect data from US-based crowd workers, build a dataset of crowdsourced truthfulness judgments for political statements, and compare it with expert annotation data generated by fact-checkers such as PolitiFact.
Coscia and Rossi ( 2020 ) introduce a crowdsourced flagging system that consists of online news flagging. The bipolar model of news flagging attempts to capture the main ingredients that they observe in empirical research on fake news and disinformation.
Unlike the previously mentioned researchers who focus on news content in their approaches, Pennycook and Rand ( 2019 ) focus on using crowdsourced judgments of the quality of news sources to combat social media disinformation.
Fact-Checking. The fact-checking task is commonly manually performed by journalists to verify the truthfulness of a given claim. Indeed, fact-checking features are being adopted by multiple online social network platforms. For instance, Facebook 34 started addressing false information through independent fact-checkers in 2017, followed by Google 35 the same year. Two years later, Instagram 36 followed suit. However, the usefulness of fact-checking initiatives is questioned by journalists 37 , as well as by researchers such as Andersen and Søe ( 2020 ). On the other hand, work is being conducted to boost the effectiveness of these initiatives to reduce misinformation (Chung and Kim 2021 ; Clayton et al. 2020 ; Nyhan et al. 2020 ).
Most researchers use fact-checking websites (e.g., politifact.com, 38 snopes.com, 39 Reuters, 40 , etc.) as data sources to build their datasets and train their models. Therefore, in the following, we specifically review examples of solutions that use fact-checking (Vlachos and Riedel 2014 ) to help build datasets that can be further used in the automatic detection of fake content.
Yang et al. ( 2019a ) use PolitiFact fact-checking website as a data source to train, tune, and evaluate their model named XFake, on political data. The XFake system is an explainable fake news detector that assists end users to identify news credibility. The fakeness of news items is detected and interpreted considering both content and contextual (e.g., statements) information (e.g., speaker).
Based on the idea that fact-checkers cannot clean all data, and it must be a selection of what “matters the most” to clean while checking a claim, Sintos et al. ( 2019 ) propose a solution to help fact-checkers combat problems related to data quality (where inaccurate data lead to incorrect conclusions) and data phishing. The proposed solution is a combination of data cleaning and perturbation analysis to avoid uncertainties and errors in data and the possibility that data can be phished.
Tchechmedjiev et al. ( 2019 ) propose a system named “ClaimsKG” as a knowledge graph of fact-checked claims aiming to facilitate structured queries about their truth values, authors, dates, journalistic reviews and other kinds of metadata. “ClaimsKG” designs the relationship between vocabularies. To gather vocabularies, a semi-automated pipeline periodically gathers data from popular fact-checking websites regularly.
AI-based Techniques
Previous work by Yaqub et al. ( 2020 ) has shown that people lack trust in automated solutions for fake news detection However, work is already being undertaken to increase this trust, for instance by von der Weth et al. ( 2020 ).
Most researchers consider fake news detection as a classification problem and use artificial intelligence techniques, as shown in Fig. 8 . The adopted AI techniques may include machine learning ML (e.g., Naïve Bayes, logistic regression, support vector machine SVM), deep learning DL (e.g., convolutional neural networks CNN, recurrent neural networks RNN, long short-term memory LSTM) and natural language processing NLP (e.g., Count vectorizer, TF-IDF Vectorizer). Most of them combine many AI techniques in their solutions rather than relying on one specific approach.

Examples of the most widely used AI techniques for fake news detection
Many researchers are developing machine learning models in their solutions for fake news detection. Recently, deep neural network techniques are also being employed as they are generating promising results (Islam et al. 2020 ). A neural network is a massively parallel distributed processor with simple units that can store important information and make it available for use (Hiriyannaiah et al. 2020 ). Moreover, it has been proven (Cardoso Durier da Silva et al. 2019 ) that the most widely used method for automatic detection of fake news is not simply a classical machine learning technique, but rather a fusion of classical techniques coordinated by a neural network.
Some researchers define purely machine learning models (Del Vicario et al. 2019 ; Elhadad et al. 2019 ; Aswani et al. 2017 ; Hakak et al. 2021 ; Singh et al. 2021 ) in their fake news detection approaches. The more commonly used machine learning algorithms (Abdullah-All-Tanvir et al. 2019 ) for classification problems are Naïve Bayes, logistic regression and SVM.
Other researchers (Wang et al. 2019c ; Wang 2017 ; Liu and Wu 2018 ; Mishra 2020 ; Qian et al. 2018 ; Zhang et al. 2020 ; Goldani et al. 2021 ) prefer to do a mixture of different deep learning models, without combining them with classical machine learning techniques. Some even prove that deep learning techniques outperform traditional machine learning techniques (Mishra et al. 2022 ). Deep learning is one of the most widely popular research topics in machine learning. Unlike traditional machine learning approaches, which are based on manually crafted features, deep learning approaches can learn hidden representations from simpler inputs both in context and content variations (Bondielli and Marcelloni 2019 ). Moreover, traditional machine learning algorithms almost always require structured data and are designed to “learn” to act by understanding labeled data and then use it to produce new results with more datasets, which requires human intervention to “teach them” when the result is incorrect (Parrish 2018 ), while deep learning networks rely on layers of artificial neural networks (ANN) and do not require human intervention, as multilevel layers in neural networks place data in a hierarchy of different concepts, which ultimately learn from their own mistakes (Parrish 2018 ). The two most widely implemented paradigms in deep neural networks are recurrent neural networks (RNN) and convolutional neural networks (CNN).
Still other researchers (Abdullah-All-Tanvir et al. 2019 ; Kaliyar et al. 2020 ; Zhang et al. 2019a ; Deepak and Chitturi 2020 ; Shu et al. 2018a ; Wang et al. 2019c ) prefer to combine traditional machine learning and deep learning classification, models. Others combine machine learning and natural language processing techniques. A few combine deep learning models with natural language processing (Vereshchaka et al. 2020 ). Some other researchers (Kapusta et al. 2019 ; Ozbay and Alatas 2020 ; Ahmed et al. 2020 ) combine natural language processing with machine learning models. Furthermore, others (Abdullah-All-Tanvir et al. 2019 ; Kaur et al. 2020 ; Kaliyar 2018 ; Abdullah-All-Tanvir et al. 2020 ; Bahad et al. 2019 ) prefer to combine all the previously mentioned techniques (i.e., ML, DL and NLP) in their approaches.
Table 11 , which is relegated to the Appendix (after the bibliography) because of its size, shows a comparison of the fake news detection solutions that we have reviewed based on their main approaches, the methodology that was used and the models.
Comparison of AI-based fake news detection techniques
Reference | Approach | Method | Model |
---|---|---|---|
Del Vicario et al. ( ) | An approach to analyze the sentiment associated with data textual content and add semantic knowledge to it | ML | Linear Regression (LIN), Logistic Regression (LOG), Support Vector Machine (SVM) with Linear Kernel, K-Nearest Neighbors (KNN), Neural Network Models (NN), Decision Trees (DT) |
Elhadad et al. ( ) | An approach to select hybrid features from the textual content of the news, which they consider as blocks, without segmenting text into parts (title, content, date, source, etc.) | ML | Decision Tree, KNN, Logistic Regression, SVM, Naïve Bayes with n-gram, LSVM, Perceptron |
Aswani et al. ( ) | A hybrid artificial bee colony approach to identify and segregate buzz in Twitter and analyze user-generated content (UGC) to mine useful information (content buzz/popularity) | ML | KNN with artificial bee colony optimization |
Hakak et al. ( ) | An ensemble of machine learning approaches for effective feature extraction to classify fake news | ML | Decision Tree, Random Forest and Extra Tree Classifier |
Singh et al. ( ) | A multimodal approach, combining text and visual analysis of online news stories to automatically detect fake news through predictive analysis to detect features most strongly associated with fake news | ML | Logistic Regression, Linear Discrimination Analysis, Quadratic Discriminant Analysis, K-Nearest Neighbors, Naïve Bayes, Support Vector Machine, Classification and Regression Tree, and Random Forest Analysis |
Amri et al. ( ) | An explainable multimodal content-based fake news detection system | ML | Vision-and-Language BERT (VilBERT), Local Interpretable Model-Agnostic Explanations (LIME), Latent Dirichlet Allocation (LDA) topic modeling |
Wang et al. ( ) | A hybrid deep neural network model to learn the useful features from contextual information and to capture the dependencies between sequences of contextual information | DL | Recurrent and Convolutional Neural Networks (RNN and CNN) |
Wang ( ) | A hybrid convolutional neural network approach for automatic fake news detection | DL | Recurrent and Convolutional Neural Networks (RNN and CNN) |
Liu and Wu ( ) | An early detection approach of fake news to classify the propagation path to mine the global and local changes of user characteristics in the diffusion path | DL | Recurrent and Convolutional Neural Networks (RNN and CNN) |
Mishra ( ) | Unsupervised network representation learning methods to learn user (node) embeddings from both the follower network and the retweet network and to encode the propagation path sequence | DL | RNN: (long short-term memory unit (LSTM)) |
Qian et al. ( ) | A Two-Level Convolutional Neural Network with User Response Generator (TCNN-URG) where TCNN captures semantic information from the article text by representing it at the sentence and word level. The URG learns a generative model of user responses to article text from historical user responses that it can use to generate responses to new articles to assist fake news detection | DL | Convolutional Neural Network (CNN) |
Zhang et al. ( ) | Based on a set of explicit features extracted from the textual information, a deep diffusive network model is built to infer the credibility of news articles, creators and subjects simultaneously | DL | Deep Diffusive Network Model Learning |
Goldani et al. ( ) | A capsule networks (CapsNet) approach for fake news detection using two architectures for different lengths of news statements and claims that capsule neural networks have been successful in computer vision and are receiving attention for use in Natural Language Processing (NLP) | DL | Capsule Networks (CapsNet) |
Wang et al. ( ) | An automated approach to distinguish different cases of fake news (i.e., hoaxes, irony and propaganda) while assessing and classifying news articles and claims including linguistic cues as well as user credibility and news dissemination in social media | DL, ML | Convolutional Neural Network (CNN), long Short-Term Memory (LSTM), logistic regression |
Abdullah-All-Tanvir et al. ( ) | A model to recognize forged news messages from twitter posts, by figuring out how to anticipate precision appraisals, in view of computerizing forged news identification in Twitter dataset. A combination of traditional machine learning, as well as deep learning classification models, is tested to enhance the accuracy of prediction | DL, ML | Naïve Bayes, Logistic Regression, Support Vector Machine, Recurrent Neural Network (RNN), Long Short-Term Memory (LSTM) |
Kaliyar et al. ( ) | An approach named (FNDNet) based on the combination between unsupervised learning algorithm GloVe and deep convolutional neural network for fake news detection | DL, ML | Deep Convolutional Neural Network (CNN), Global Vectors (GloVe) |
Zhang et al. ( ) | A hybrid approach to encode auxiliary information coming from people’s replies alone in temporal order. Such auxiliary information is then used to update a priori belief generating a posteriori belief | DL, ML | Deep Learning Model, Long Short-Term Memory Neural Network (LSTM) |
Deepak and Chitturi ( ) | A system that consists of live data mining in addition to the deep learning model | DL, ML | Feedforward Neural Network (FNN) and LSTM Word Vector Model |
Shu et al. ( ) | A multidimensional fake news data repository “FakeNewsNet” and conduct an exploratory analysis of the datasets to evaluate them | DL, ML | Convolutional Neural Network (CNN), Support Vector Machines (SVMs), Logistic Regression (LR), Naïve Bayes (NB) |
Vereshchaka et al. ( ) | A sociocultural textual analysis, computational linguistics analysis, and textual classification using NLP, as well as deep learning models to distinguish fake from real news to mitigate the problem of disinformation | DL, NLP | Short-Term Memory (LSTM), Recurrent Neural Network (RNN) and Gated Recurrent Unit (GRU) |
Kapusta et al. ( ) | A sentiment and frequency analysis using both machine learning and NLP in what is called text mining to processing news content sentiment analysis and frequency analysis to compare basic text characteristics of fake and real news articles | ML, NLP | The Natural Language Toolkit (NLTK), TF-IDF |
Ozbay and Alatas ( ) | A hybrid approach based on text analysis and supervised artificial intelligence for fake news detection | ML, NLP | Supervised algorithms: BayesNet, JRip, OneR, Decision Stump, ZeroR, Stochastic Gradient Descent (SGD), CV Parameter Selection (CVPS), Randomizable Filtered Classifier (RFC), Logistic Model Tree (LMT). NLP: TF weighting |
Ahmed et al. ( ) | A machine learning and NLP text-based processing to identify fake news. Various features of the text are extracted through text processing and after that those features are incorporated into classification | ML, NLP | Machine learning classifiers (i.e., Passive-aggressive, Naïve Bayes and Support Vector Machine) |
Abdullah-All-Tanvir et al. ( ) | A hybrid neural network approach to identify authentic news on popular Twitter threads which would outperform the traditional neural network architecture’s performance. Three traditional supervised algorithms and two Deep Neural are combined to train the defined model. Some NLP concepts were also used to implement some of the traditional supervised machine learning algorithms over their dataset | ML, DL, NLP | Traditional supervised algorithm (i.e., Logistic Regression, Bayesian Classifier and Support Vector Machine). Deep Neural Networks (i.e., Recurrent Neural Network, Long Short-Term Memory LSTM). NLP concepts such as Count vectorizer and TF-IDF Vectorizer |
Kaur et al. ( ) | A hybrid method to identify news articles as fake or real through finding out which classification model identifies false features accurately | ML, DL, NLP | Neural Networks (NN) and Ensemble Models. Supervised Machine Learning Classifiers such as Naïve Bayes (NB), Decision Tree (DT), Support Vector Machine (SVM), Linear Models. Term Frequency-Inverse Document Frequency (TF-IDF), Count-Vectorizer (CV), Hashing-Vectorizer (HV) |
Kaliyar ( ) | A fake news detection approach to classify the news article or other documents into certain or not. Natural language processing, machine learning and deep learning techniques are used to implement the defined models and to predict the accuracy of different models and classifiers | ML, DL, NLP | Machine Learning Models: Naïve Bayes, K-nearest Neighbors, Decision Tree, Random Forest. Deep Learning Networks: Shallow Convolutional Neural Networks (CNN), Very Deep Convolutional Neural Network (VDCNN), Long Short-Term Memory Network (LSTM), Gated Recurrent Unit Network (GRU). A combination of Convolutional Neural Network with Long Short-Term Memory (CNN-LSTM) and Convolutional Neural Network with Gated Recurrent Unit (CNN-LSTM) |
Mahabub ( ) | An intelligent detection system to manage the classification of news as being either real or fake | ML, DL, NLP | Machine Learning: Naïve Bayes, KNN, SVM, Random Forest, Artificial Neural Network, Logistic Regression, Gradient Boosting, AdaBoost |
Bahad et al. ( ) | A method based on Bi-directional LSTM-recurrent neural network to analyze the relationship between the news article headline and article body | ML, DL, NLP | Unsupervised Learning algorithm: Global Vectors (GloVe). Bi-directional LSTM-recurrent Neural Network |
Blockchain-based Techniques for Source Reliability and Traceability
Another research direction for detecting and mitigating fake news in social media focuses on using blockchain solutions. Blockchain technology is recently attracting researchers’ attention due to the interesting features it offers. Immutability, decentralization, tamperproof, consensus, record keeping and non-repudiation of transactions are some of the key features that make blockchain technology exploitable, not just for cryptocurrencies, but also to prove the authenticity and integrity of digital assets.
However, the proposed blockchain approaches are few in number and they are fundamental and theoretical approaches. Specifically, the solutions that are currently available are still in research, prototype, and beta testing stages (DiCicco and Agarwal 2020 ; Tchechmedjiev et al. 2019 ). Furthermore, most researchers (Ochoa et al. 2019 ; Song et al. 2019 ; Shang et al. 2018 ; Qayyum et al. 2019 ; Jing and Murugesan 2018 ; Buccafurri et al. 2017 ; Chen et al. 2018 ) do not specify which fake news type they are mitigating in their studies. They mention news content in general, which is not adequate for innovative solutions. For that, serious implementations should be provided to prove the usefulness and feasibility of this newly developing research vision.
Table 9 shows a classification of the reviewed blockchain-based approaches. In the classification, we listed the following:
- The type of fake news that authors are trying to mitigate, which can be multimedia-based or text-based fake news.
- The techniques used for fake news mitigation, which can be either blockchain only, or blockchain combined with other techniques such as AI, Data mining, Truth-discovery, Preservation metadata, Semantic similarity, Crowdsourcing, Graph theory and SIR model (Susceptible, Infected, Recovered).
- The feature that is offered as an advantage of the given solution (e.g., Reliability, Authenticity and Traceability). Reliability is the credibility and truthfulness of the news content, which consists of proving the trustworthiness of the content. Traceability aims to trace and archive the contents. Authenticity consists of checking whether the content is real and authentic.
A checkmark ( ✓ ) in Table 9 denotes that the mentioned criterion is explicitly mentioned in the proposed solution, while the empty dash (–) cell for fake news type denotes that it depends on the case: The criterion was either not explicitly mentioned (e.g., fake news type) in the work or the classification does not apply (e.g., techniques/other).
A classification of popular blockchain-based approaches for fake news detection in social media
Reference | Fake News Type | Techniques | Feature | |
---|---|---|---|---|
Multimedia | Text | |||
Shae and Tsai ( ) | AI | Reliability | ||
Ochoa et al. ( ) | – | Data Mining, Truth-Discovery | Reliability | |
Huckle and White ( ) | – | Preservation Metadata | Reliability | |
Song et al. ( ) | – | – | – | Traceability |
Shang et al. ( ) | – | – | – | Traceability |
Qayyum et al. ( ) | – | – | Semantic Similarity | Reliability |
Jing and Murugesan ( ) | – | – | AI | Reliability |
Buccafurri et al. ( ) | – | – | Crowd-Sourcing | Reliability |
Chen et al. ( ) | – | – | SIR Model | Reliability |
Hasan and Salah ( ) | – | – | Authenticity | |
Tchechmedjiev et al. ( ) | – | – | Graph theory | Reliability |
After reviewing the most relevant state of the art for automatic fake news detection, we classify them as shown in Table 10 based on the detection aspects (i.e., content-based, contextual, or hybrid aspects) and the techniques used (i.e., AI, crowdsourcing, fact-checking, blockchain or hybrid techniques). Hybrid techniques refer to solutions that simultaneously combine different techniques from previously mentioned categories (i.e., inter-hybrid methods), as well as techniques within the same class of methods (i.e., intra-hybrid methods), in order to define innovative solutions for fake news detection. A hybrid method should bring the best of both worlds. Then, we provide a discussion based on different axes.
Fake news detection approaches classification
Artificial Intelligence | Crowdsourcing (CDS) | Blockchain (BKC) | Fact-checking | Hybrid | |||
---|---|---|---|---|---|---|---|
ML | DL | NLP | |||||
Content | Del Vicario et al. ( ), Hosseinimotlagh and Papalexakis ( ), Hakak et al. ( ), Singh et al. ( ), Amri et al. ( ) | Wang ( ), Hiriyannaiah et al. ( ) | Zellers et al. ( ) | Kim et al. ( ), Tschiatschek et al. ( ), Tchakounté et al. ( ), Huffaker et al. ( ), La Barbera et al. ( ), Coscia and Rossi ( ), Micallef et al. ( ) | Song et al. ( ) | Sintos et al. ( ) | ML, DL, NLP: Abdullah-All-Tanvir et al. ( ), Kaur et al. ( ), Mahabub ( ), Bahad et al. ( ) Kaliyar ( ) |
ML, DL: | |||||||
Abdullah-All-Tanvir et al. ( ), Kaliyar et al. ( ), Deepak and Chitturi ( ) | |||||||
DL, NLP: Vereshchaka et al. ( ) | |||||||
ML, NLP: Kapusta et al. ( ), Ozbay and Alatas Ozbay and Alatas ( ), Ahmed et al. ( ) | |||||||
BKC, CDS: Buccafurri et al. ( ) | |||||||
Context | – | Qian et al. ( ), Liu and Wu ( ), Hamdi et al. ( ), Wang et al. ( ), Mishra ( ) | – | Pennycook and Rand ( ) | Huckle and White ( ), Shang et al. ( ) | Tchechmedjiev et al. ( ) | ML, DL: Zhang et al. ( ), Shu et al. ( ), Shu et al. ( ), Wu and Liu ( ) |
BKC, AI: Ochoa et al. ( ) | |||||||
BKC, SIR: Chen et al. ( ) | |||||||
Hybrid | Aswani et al. ( ), Previti et al. ( ), Elhadad et al. ( ), Nyow and Chua ( ) | Ruchansky et al. ( ), Wu and Rao ( ), Guo et al. ( ), Zhang et al. ( ) | Xu et al. ( ) | – | Qayyum et al. ( ), Hasan and Salah ( ), Tchechmedjiev et al. ( ) | Yang et al. ( ) | ML, DL: Shu et al. ( ), Wang et al. ( ) |
BKC, AI: Shae and Tsai ( ), Jing and Murugesan ( ) |
News content-based methods
Most of the news content-based approaches consider fake news detection as a classification problem and they use AI techniques such as classical machine learning (e.g., regression, Bayesian) as well as deep learning (i.e., neural methods such as CNN and RNN). More specifically, classification of social media content is a fundamental task for social media mining, so that most existing methods regard it as a text categorization problem and mainly focus on using content features, such as words and hashtags (Wu and Liu 2018 ). The main challenge facing these approaches is how to extract features in a way to reduce the data used to train their models and what features are the most suitable for accurate results.
Researchers using such approaches are motivated by the fact that the news content is the main entity in the deception process, and it is a straightforward factor to analyze and use while looking for predictive clues of deception. However, detecting fake news only from the content of the news is not enough because the news is created in a strategic intentional way to mimic the truth (i.e., the content can be intentionally manipulated by the spreader to make it look like real news). Therefore, it is considered to be challenging, if not impossible, to identify useful features (Wu and Liu 2018 ) and consequently tell the nature of such news solely from the content.
Moreover, works that utilize only the news content for fake news detection ignore the rich information and latent user intelligence (Qian et al. 2018 ) stored in user responses toward previously disseminated articles. Therefore, the auxiliary information is deemed crucial for an effective fake news detection approach.
Social context-based methods
The context-based approaches explore the surrounding data outside of the news content, which can be an effective direction and has some advantages in areas where the content approaches based on text classification can run into issues. However, most existing studies implementing contextual methods mainly focus on additional information coming from users and network diffusion patterns. Moreover, from a technical perspective, they are limited to the use of sophisticated machine learning techniques for feature extraction, and they ignore the usefulness of results coming from techniques such as web search and crowdsourcing which may save much time and help in the early detection and identification of fake content.
Hybrid approaches can simultaneously model different aspects of fake news such as the content-based aspects, as well as the contextual aspect based on both the OSN user and the OSN network patterns. However, these approaches are deemed more complex in terms of models (Bondielli and Marcelloni 2019 ), data availability, and the number of features. Furthermore, it remains difficult to decide which information among each category (i.e., content-based and context-based information) is most suitable and appropriate to be used to achieve accurate and precise results. Therefore, there are still very few studies belonging to this category of hybrid approaches.
Early detection
As fake news usually evolves and spreads very fast on social media, it is critical and urgent to consider early detection directions. Yet, this is a challenging task to do especially in highly dynamic platforms such as social networks. Both news content- and social context-based approaches suffer from this challenging early detection of fake news.
Although approaches that detect fake news based on content analysis face this issue less, they are still limited by the lack of information required for verification when the news is in its early stage of spread. However, approaches that detect fake news based on contextual analysis are most likely to suffer from the lack of early detection since most of them rely on information that is mostly available after the spread of fake content such as social engagement, user response, and propagation patterns. Therefore, it is crucial to consider both trusted human verification and historical data as an attempt to detect fake content during its early stage of propagation.
Conclusion and future directions
In this paper, we introduced the general context of the fake news problem as one of the major issues of the online deception problem in online social networks. Based on reviewing the most relevant state of the art, we summarized and classified existing definitions of fake news, as well as its related terms. We also listed various typologies and existing categorizations of fake news such as intent-based fake news including clickbait, hoax, rumor, satire, propaganda, conspiracy theories, framing as well as content-based fake news including text and multimedia-based fake news, and in the latter, we can tackle deepfake videos and GAN-generated fake images. We discussed the major challenges related to fake news detection and mitigation in social media including the deceptiveness nature of the fabricated content, the lack of human awareness in the field of fake news, the non-human spreaders issue (e.g., social bots), the dynamicity of such online platforms, which results in a fast propagation of fake content and the quality of existing datasets, which still limits the efficiency of the proposed solutions. We reviewed existing researchers’ visions regarding the automatic detection of fake news based on the adopted approaches (i.e., news content-based approaches, social context-based approaches, or hybrid approaches) and the techniques that are used (i.e., artificial intelligence-based methods; crowdsourcing, fact-checking, and blockchain-based methods; and hybrid methods), then we showed a comparative study between the reviewed works. We also provided a critical discussion of the reviewed approaches based on different axes such as the adopted aspect for fake news detection (i.e., content-based, contextual, and hybrid aspects) and the early detection perspective.
To conclude, we present the main issues for combating the fake news problem that needs to be further investigated while proposing new detection approaches. We believe that to define an efficient fake news detection approach, we need to consider the following:
- Our choice of sources of information and search criteria may have introduced biases in our research. If so, it would be desirable to identify those biases and mitigate them.
- News content is the fundamental source to find clues to distinguish fake from real content. However, contextual information derived from social media users and from the network can provide useful auxiliary information to increase detection accuracy. Specifically, capturing users’ characteristics and users’ behavior toward shared content can be a key task for fake news detection.
- Moreover, capturing users’ historical behavior, including their emotions and/or opinions toward news content, can help in the early detection and mitigation of fake news.
- Furthermore, adversarial learning techniques (e.g., GAN, SeqGAN) can be considered as a promising direction for mitigating the lack and scarcity of available datasets by providing machine-generated data that can be used to train and build robust systems to detect the fake examples from the real ones.
- Lastly, analyzing how sources and promoters of fake news operate over the web through multiple online platforms is crucial; Zannettou et al. ( 2019 ) discovered that false information is more likely to spread across platforms (18% appearing on multiple platforms) compared to valid information (11%).
Appendix: A Comparison of AI-based fake news detection techniques
This Appendix consists only in the rather long Table 11 . It shows a comparison of the fake news detection solutions based on artificial intelligence that we have reviewed according to their main approaches, the methodology that was used, and the models, as explained in Sect. 6.2.2 .
Author Contributions
The order of authors is alphabetic as is customary in the third author’s field. The lead author was Sabrine Amri, who collected and analyzed the data and wrote a first draft of the paper, all along under the supervision and tight guidance of Esma Aïmeur. Gilles Brassard reviewed, criticized and polished the work into its final form.
This work is supported in part by Canada’s Natural Sciences and Engineering Research Council.
Availability of data and material
Declarations.
On behalf of all authors, the corresponding author states that there is no conflict of interest.
1 https://www.nationalacademies.org/news/2021/07/as-surgeon-general-urges-whole-of-society-effort-to-fight-health-misinformation-the-work-of-the-national-academies-helps-foster-an-evidence-based-information-environment , last access date: 26-12-2022.
2 https://time.com/4897819/elvis-presley-alive-conspiracy-theories/ , last access date: 26-12-2022.
3 https://www.therichest.com/shocking/the-evidence-15-reasons-people-think-the-earth-is-flat/ , last access date: 26-12-2022.
4 https://www.grunge.com/657584/the-truth-about-1952s-alien-invasion-of-washington-dc/ , last access date: 26-12-2022.
5 https://www.journalism.org/2021/01/12/news-use-across-social-media-platforms-in-2020/ , last access date: 26-12-2022.
6 https://www.pewresearch.org/fact-tank/2018/12/10/social-media-outpaces-print-newspapers-in-the-u-s-as-a-news-source/ , last access date: 26-12-2022.
7 https://www.buzzfeednews.com/article/janelytvynenko/coronavirus-fake-news-disinformation-rumors-hoaxes , last access date: 26-12-2022.
8 https://www.factcheck.org/2020/03/viral-social-media-posts-offer-false-coronavirus-tips/ , last access date: 26-12-2022.
9 https://www.factcheck.org/2020/02/fake-coronavirus-cures-part-2-garlic-isnt-a-cure/ , last access date: 26-12-2022.
10 https://www.bbc.com/news/uk-36528256 , last access date: 26-12-2022.
11 https://en.wikipedia.org/wiki/Pizzagate_conspiracy_theory , last access date: 26-12-2022.
12 https://www.theguardian.com/world/2017/jan/09/germany-investigating-spread-fake-news-online-russia-election , last access date: 26-12-2022.
13 https://www.macquariedictionary.com.au/resources/view/word/of/the/year/2016 , last access date: 26-12-2022.
14 https://www.macquariedictionary.com.au/resources/view/word/of/the/year/2018 , last access date: 26-12-2022.
15 https://apnews.com/article/47466c5e260149b1a23641b9e319fda6 , last access date: 26-12-2022.
16 https://blog.collinsdictionary.com/language-lovers/collins-2017-word-of-the-year-shortlist/ , last access date: 26-12-2022.
17 https://www.gartner.com/smarterwithgartner/gartner-top-strategic-predictions-for-2018-and-beyond/ , last access date: 26-12-2022.
18 https://www.technologyreview.com/s/612236/even-the-best-ai-for-spotting-fake-news-is-still-terrible/ , last access date: 26-12-2022.
19 https://scholar.google.ca/ , last access date: 26-12-2022.
20 https://ieeexplore.ieee.org/ , last access date: 26-12-2022.
21 https://link.springer.com/ , last access date: 26-12-2022.
22 https://www.sciencedirect.com/ , last access date: 26-12-2022.
23 https://www.scopus.com/ , last access date: 26-12-2022.
24 https://www.acm.org/digital-library , last access date: 26-12-2022.
25 https://www.politico.com/magazine/story/2016/12/fake-news-history-long-violent-214535 , last access date: 26-12-2022.
26 https://en.wikipedia.org/wiki/Trial_of_Socrates , last access date: 26-12-2022.
27 https://trends.google.com/trends/explore?hl=en-US &tz=-180 &date=2013-12-06+2018-01-06 &geo=US &q=fake+news &sni=3 , last access date: 26-12-2022.
28 https://ec.europa.eu/digital-single-market/en/tackling-online-disinformation , last access date: 26-12-2022.
29 https://www.nato.int/cps/en/natohq/177273.htm , last access date: 26-12-2022.
30 https://www.collinsdictionary.com/dictionary/english/fake-news , last access date: 26-12-2022.
31 https://www.statista.com/statistics/657111/fake-news-sharing-online/ , last access date: 26-12-2022.
32 https://www.statista.com/statistics/657090/fake-news-recogition-confidence/ , last access date: 26-12-2022.
33 https://www.nbcnews.com/tech/social-media/now-available-more-200-000-deleted-russian-troll-tweets-n844731 , last access date: 26-12-2022.
34 https://www.theguardian.com/technology/2017/mar/22/facebook-fact-checking-tool-fake-news , last access date: 26-12-2022.
35 https://www.theguardian.com/technology/2017/apr/07/google-to-display-fact-checking-labels-to-show-if-news-is-true-or-false , last access date: 26-12-2022.
36 https://about.instagram.com/blog/announcements/combatting-misinformation-on-instagram , last access date: 26-12-2022.
37 https://www.wired.com/story/instagram-fact-checks-who-will-do-checking/ , last access date: 26-12-2022.
38 https://www.politifact.com/ , last access date: 26-12-2022.
39 https://www.snopes.com/ , last access date: 26-12-2022.
40 https://www.reutersagency.com/en/ , last access date: 26-12-2022.
Publisher's Note
Springer Nature remains neutral with regard to jurisdictional claims in published maps and institutional affiliations.
Contributor Information
Esma Aïmeur, Email: ac.laertnomu.ori@ruemia .
Sabrine Amri, Email: [email protected] .
Gilles Brassard, Email: ac.laertnomu.ori@drassarb .
- Abdullah-All-Tanvir, Mahir EM, Akhter S, Huq MR (2019) Detecting fake news using machine learning and deep learning algorithms. In: 7th international conference on smart computing and communications (ICSCC), IEEE, pp 1–5 10.1109/ICSCC.2019.8843612
- Abdullah-All-Tanvir, Mahir EM, Huda SMA, Barua S (2020) A hybrid approach for identifying authentic news using deep learning methods on popular Twitter threads. In: International conference on artificial intelligence and signal processing (AISP), IEEE, pp 1–6 10.1109/AISP48273.2020.9073583
- Abu Arqoub O, Abdulateef Elega A, Efe Özad B, Dwikat H, Adedamola Oloyede F. Mapping the scholarship of fake news research: a systematic review. J Pract. 2022; 16 (1):56–86. doi: 10.1080/17512786.2020.1805791. [ CrossRef ] [ Google Scholar ]
- Ahmed S, Hinkelmann K, Corradini F. Development of fake news model using machine learning through natural language processing. Int J Comput Inf Eng. 2020; 14 (12):454–460. [ Google Scholar ]
- Aïmeur E, Brassard G, Rioux J. Data privacy: an end-user perspective. Int J Comput Netw Commun Secur. 2013; 1 (6):237–250. [ Google Scholar ]
- Aïmeur E, Hage H, Amri S (2018) The scourge of online deception in social networks. In: 2018 international conference on computational science and computational intelligence (CSCI), IEEE, pp 1266–1271 10.1109/CSCI46756.2018.00244
- Alemanno A. How to counter fake news? A taxonomy of anti-fake news approaches. Eur J Risk Regul. 2018; 9 (1):1–5. doi: 10.1017/err.2018.12. [ CrossRef ] [ Google Scholar ]
- Allcott H, Gentzkow M. Social media and fake news in the 2016 election. J Econ Perspect. 2017; 31 (2):211–36. doi: 10.1257/jep.31.2.211. [ CrossRef ] [ Google Scholar ]
- Allen J, Howland B, Mobius M, Rothschild D, Watts DJ. Evaluating the fake news problem at the scale of the information ecosystem. Sci Adv. 2020 doi: 10.1126/sciadv.aay3539. [ PMC free article ] [ PubMed ] [ CrossRef ] [ Google Scholar ]
- Allington D, Duffy B, Wessely S, Dhavan N, Rubin J. Health-protective behaviour, social media usage and conspiracy belief during the Covid-19 public health emergency. Psychol Med. 2020 doi: 10.1017/S003329172000224X. [ PMC free article ] [ PubMed ] [ CrossRef ] [ Google Scholar ]
- Alonso-Galbán P, Alemañy-Castilla C (2022) Curbing misinformation and disinformation in the Covid-19 era: a view from cuba. MEDICC Rev 22:45–46 10.37757/MR2020.V22.N2.12 [ PubMed ] [ CrossRef ]
- Altay S, Hacquin AS, Mercier H. Why do so few people share fake news? It hurts their reputation. New Media Soc. 2022; 24 (6):1303–1324. doi: 10.1177/1461444820969893. [ CrossRef ] [ Google Scholar ]
- Amri S, Sallami D, Aïmeur E (2022) Exmulf: an explainable multimodal content-based fake news detection system. In: International symposium on foundations and practice of security. Springer, Berlin, pp 177–187. 10.1109/IJCNN48605.2020.9206973
- Andersen J, Søe SO. Communicative actions we live by: the problem with fact-checking, tagging or flagging fake news-the case of Facebook. Eur J Commun. 2020; 35 (2):126–139. doi: 10.1177/0267323119894489. [ CrossRef ] [ Google Scholar ]
- Apuke OD, Omar B. Fake news and Covid-19: modelling the predictors of fake news sharing among social media users. Telematics Inform. 2021; 56 :101475. doi: 10.1016/j.tele.2020.101475. [ PMC free article ] [ PubMed ] [ CrossRef ] [ Google Scholar ]
- Apuke OD, Omar B, Tunca EA, Gever CV. The effect of visual multimedia instructions against fake news spread: a quasi-experimental study with Nigerian students. J Librariansh Inf Sci. 2022 doi: 10.1177/09610006221096477. [ CrossRef ] [ Google Scholar ]
- Aswani R, Ghrera S, Kar AK, Chandra S. Identifying buzz in social media: a hybrid approach using artificial bee colony and k-nearest neighbors for outlier detection. Soc Netw Anal Min. 2017; 7 (1):1–10. doi: 10.1007/s13278-017-0461-2. [ CrossRef ] [ Google Scholar ]
- Avram M, Micallef N, Patil S, Menczer F (2020) Exposure to social engagement metrics increases vulnerability to misinformation. arXiv preprint arxiv:2005.04682 , 10.37016/mr-2020-033
- Badawy A, Lerman K, Ferrara E (2019) Who falls for online political manipulation? In: Companion proceedings of the 2019 world wide web conference, pp 162–168 10.1145/3308560.3316494
- Bahad P, Saxena P, Kamal R. Fake news detection using bi-directional LSTM-recurrent neural network. Procedia Comput Sci. 2019; 165 :74–82. doi: 10.1016/j.procs.2020.01.072. [ CrossRef ] [ Google Scholar ]
- Bakdash J, Sample C, Rankin M, Kantarcioglu M, Holmes J, Kase S, Zaroukian E, Szymanski B (2018) The future of deception: machine-generated and manipulated images, video, and audio? In: 2018 international workshop on social sensing (SocialSens), IEEE, pp 2–2 10.1109/SocialSens.2018.00009
- Balmas M. When fake news becomes real: combined exposure to multiple news sources and political attitudes of inefficacy, alienation, and cynicism. Commun Res. 2014; 41 (3):430–454. doi: 10.1177/0093650212453600. [ CrossRef ] [ Google Scholar ]
- Baptista JP, Gradim A. Understanding fake news consumption: a review. Soc Sci. 2020 doi: 10.3390/socsci9100185. [ CrossRef ] [ Google Scholar ]
- Baptista JP, Gradim A. A working definition of fake news. Encyclopedia. 2022; 2 (1):632–645. doi: 10.3390/encyclopedia2010043. [ CrossRef ] [ Google Scholar ]
- Bastick Z. Would you notice if fake news changed your behavior? An experiment on the unconscious effects of disinformation. Comput Hum Behav. 2021; 116 :106633. doi: 10.1016/j.chb.2020.106633. [ CrossRef ] [ Google Scholar ]
- Batailler C, Brannon SM, Teas PE, Gawronski B. A signal detection approach to understanding the identification of fake news. Perspect Psychol Sci. 2022; 17 (1):78–98. doi: 10.1177/1745691620986135. [ PubMed ] [ CrossRef ] [ Google Scholar ]
- Bessi A, Ferrara E (2016) Social bots distort the 2016 US presidential election online discussion. First Monday 21(11-7). 10.5210/fm.v21i11.7090
- Bhattacharjee A, Shu K, Gao M, Liu H (2020) Disinformation in the online information ecosystem: detection, mitigation and challenges. arXiv preprint arXiv:2010.09113
- Bhuiyan MM, Zhang AX, Sehat CM, Mitra T. Investigating differences in crowdsourced news credibility assessment: raters, tasks, and expert criteria. Proc ACM Hum Comput Interact. 2020; 4 (CSCW2):1–26. doi: 10.1145/3415164. [ CrossRef ] [ Google Scholar ]
- Bode L, Vraga EK. In related news, that was wrong: the correction of misinformation through related stories functionality in social media. J Commun. 2015; 65 (4):619–638. doi: 10.1111/jcom.12166. [ CrossRef ] [ Google Scholar ]
- Bondielli A, Marcelloni F. A survey on fake news and rumour detection techniques. Inf Sci. 2019; 497 :38–55. doi: 10.1016/j.ins.2019.05.035. [ CrossRef ] [ Google Scholar ]
- Bovet A, Makse HA. Influence of fake news in Twitter during the 2016 US presidential election. Nat Commun. 2019; 10 (1):1–14. doi: 10.1038/s41467-018-07761-2. [ PMC free article ] [ PubMed ] [ CrossRef ] [ Google Scholar ]
- Brashier NM, Pennycook G, Berinsky AJ, Rand DG. Timing matters when correcting fake news. Proc Natl Acad Sci. 2021 doi: 10.1073/pnas.2020043118. [ PMC free article ] [ PubMed ] [ CrossRef ] [ Google Scholar ]
- Brewer PR, Young DG, Morreale M. The impact of real news about “fake news”: intertextual processes and political satire. Int J Public Opin Res. 2013; 25 (3):323–343. doi: 10.1093/ijpor/edt015. [ CrossRef ] [ Google Scholar ]
- Bringula RP, Catacutan-Bangit AE, Garcia MB, Gonzales JPS, Valderama AMC. “Who is gullible to political disinformation?” Predicting susceptibility of university students to fake news. J Inf Technol Polit. 2022; 19 (2):165–179. doi: 10.1080/19331681.2021.1945988. [ CrossRef ] [ Google Scholar ]
- Buccafurri F, Lax G, Nicolazzo S, Nocera A (2017) Tweetchain: an alternative to blockchain for crowd-based applications. In: International conference on web engineering, Springer, Berlin, pp 386–393. 10.1007/978-3-319-60131-1_24
- Burshtein S. The true story on fake news. Intell Prop J. 2017; 29 (3):397–446. [ Google Scholar ]
- Cardaioli M, Cecconello S, Conti M, Pajola L, Turrin F (2020) Fake news spreaders profiling through behavioural analysis. In: CLEF (working notes)
- Cardoso Durier da Silva F, Vieira R, Garcia AC (2019) Can machines learn to detect fake news? A survey focused on social media. In: Proceedings of the 52nd Hawaii international conference on system sciences. 10.24251/HICSS.2019.332
- Carmi E, Yates SJ, Lockley E, Pawluczuk A (2020) Data citizenship: rethinking data literacy in the age of disinformation, misinformation, and malinformation. Intern Policy Rev 9(2):1–22 10.14763/2020.2.1481
- Celliers M, Hattingh M (2020) A systematic review on fake news themes reported in literature. In: Conference on e-Business, e-Services and e-Society. Springer, Berlin, pp 223–234. 10.1007/978-3-030-45002-1_19
- Chen Y, Li Q, Wang H (2018) Towards trusted social networks with blockchain technology. arXiv preprint arXiv:1801.02796
- Cheng L, Guo R, Shu K, Liu H (2020) Towards causal understanding of fake news dissemination. arXiv preprint arXiv:2010.10580
- Chiu MM, Oh YW. How fake news differs from personal lies. Am Behav Sci. 2021; 65 (2):243–258. doi: 10.1177/0002764220910243. [ CrossRef ] [ Google Scholar ]
- Chung M, Kim N. When I learn the news is false: how fact-checking information stems the spread of fake news via third-person perception. Hum Commun Res. 2021; 47 (1):1–24. doi: 10.1093/hcr/hqaa010. [ CrossRef ] [ Google Scholar ]
- Clarke J, Chen H, Du D, Hu YJ. Fake news, investor attention, and market reaction. Inf Syst Res. 2020 doi: 10.1287/isre.2019.0910. [ CrossRef ] [ Google Scholar ]
- Clayton K, Blair S, Busam JA, Forstner S, Glance J, Green G, Kawata A, Kovvuri A, Martin J, Morgan E, et al. Real solutions for fake news? Measuring the effectiveness of general warnings and fact-check tags in reducing belief in false stories on social media. Polit Behav. 2020; 42 (4):1073–1095. doi: 10.1007/s11109-019-09533-0. [ CrossRef ] [ Google Scholar ]
- Collins B, Hoang DT, Nguyen NT, Hwang D (2020) Fake news types and detection models on social media a state-of-the-art survey. In: Asian conference on intelligent information and database systems. Springer, Berlin, pp 562–573 10.1007/978-981-15-3380-8_49
- Conroy NK, Rubin VL, Chen Y. Automatic deception detection: methods for finding fake news. Proc Assoc Inf Sci Technol. 2015; 52 (1):1–4. doi: 10.1002/pra2.2015.145052010082. [ CrossRef ] [ Google Scholar ]
- Cooke NA. Posttruth, truthiness, and alternative facts: Information behavior and critical information consumption for a new age. Libr Q. 2017; 87 (3):211–221. doi: 10.1086/692298. [ CrossRef ] [ Google Scholar ]
- Coscia M, Rossi L. Distortions of political bias in crowdsourced misinformation flagging. J R Soc Interface. 2020; 17 (167):20200020. doi: 10.1098/rsif.2020.0020. [ PMC free article ] [ PubMed ] [ CrossRef ] [ Google Scholar ]
- Dame Adjin-Tettey T. Combating fake news, disinformation, and misinformation: experimental evidence for media literacy education. Cogent Arts Human. 2022; 9 (1):2037229. doi: 10.1080/23311983.2022.2037229. [ CrossRef ] [ Google Scholar ]
- Deepak S, Chitturi B. Deep neural approach to fake-news identification. Procedia Comput Sci. 2020; 167 :2236–2243. doi: 10.1016/j.procs.2020.03.276. [ CrossRef ] [ Google Scholar ]
- de Cock Buning M (2018) A multi-dimensional approach to disinformation: report of the independent high level group on fake news and online disinformation. Publications Office of the European Union
- Del Vicario M, Quattrociocchi W, Scala A, Zollo F. Polarization and fake news: early warning of potential misinformation targets. ACM Trans Web (TWEB) 2019; 13 (2):1–22. doi: 10.1145/3316809. [ CrossRef ] [ Google Scholar ]
- Demuyakor J, Opata EM. Fake news on social media: predicting which media format influences fake news most on facebook. J Intell Commun. 2022 doi: 10.54963/jic.v2i1.56. [ CrossRef ] [ Google Scholar ]
- Derakhshan H, Wardle C (2017) Information disorder: definitions. In: Understanding and addressing the disinformation ecosystem, pp 5–12
- Desai AN, Ruidera D, Steinbrink JM, Granwehr B, Lee DH. Misinformation and disinformation: the potential disadvantages of social media in infectious disease and how to combat them. Clin Infect Dis. 2022; 74 (Supplement–3):e34–e39. doi: 10.1093/cid/ciac109. [ PMC free article ] [ PubMed ] [ CrossRef ] [ Google Scholar ]
- Di Domenico G, Sit J, Ishizaka A, Nunan D. Fake news, social media and marketing: a systematic review. J Bus Res. 2021; 124 :329–341. doi: 10.1016/j.jbusres.2020.11.037. [ CrossRef ] [ Google Scholar ]
- Dias N, Pennycook G, Rand DG. Emphasizing publishers does not effectively reduce susceptibility to misinformation on social media. Harv Kennedy School Misinform Rev. 2020 doi: 10.37016/mr-2020-001. [ CrossRef ] [ Google Scholar ]
- DiCicco KW, Agarwal N (2020) Blockchain technology-based solutions to fight misinformation: a survey. In: Disinformation, misinformation, and fake news in social media. Springer, Berlin, pp 267–281, 10.1007/978-3-030-42699-6_14
- Douglas KM, Uscinski JE, Sutton RM, Cichocka A, Nefes T, Ang CS, Deravi F. Understanding conspiracy theories. Polit Psychol. 2019; 40 :3–35. doi: 10.1111/pops.12568. [ CrossRef ] [ Google Scholar ]
- Edgerly S, Mourão RR, Thorson E, Tham SM. When do audiences verify? How perceptions about message and source influence audience verification of news headlines. J Mass Commun Q. 2020; 97 (1):52–71. doi: 10.1177/1077699019864680. [ CrossRef ] [ Google Scholar ]
- Egelhofer JL, Lecheler S. Fake news as a two-dimensional phenomenon: a framework and research agenda. Ann Int Commun Assoc. 2019; 43 (2):97–116. doi: 10.1080/23808985.2019.1602782. [ CrossRef ] [ Google Scholar ]
- Elhadad MK, Li KF, Gebali F (2019) A novel approach for selecting hybrid features from online news textual metadata for fake news detection. In: International conference on p2p, parallel, grid, cloud and internet computing. Springer, Berlin, pp 914–925, 10.1007/978-3-030-33509-0_86
- ERGA (2018) Fake news, and the information disorder. European Broadcasting Union (EBU)
- ERGA (2021) Notions of disinformation and related concepts. European Regulators Group for Audiovisual Media Services (ERGA)
- Escolà-Gascón Á. New techniques to measure lie detection using Covid-19 fake news and the Multivariable Multiaxial Suggestibility Inventory-2 (MMSI-2) Comput Hum Behav Rep. 2021; 3 :100049. doi: 10.1016/j.chbr.2020.100049. [ CrossRef ] [ Google Scholar ]
- Fazio L. Pausing to consider why a headline is true or false can help reduce the sharing of false news. Harv Kennedy School Misinformation Rev. 2020 doi: 10.37016/mr-2020-009. [ CrossRef ] [ Google Scholar ]
- Ferrara E, Varol O, Davis C, Menczer F, Flammini A. The rise of social bots. Commun ACM. 2016; 59 (7):96–104. doi: 10.1145/2818717. [ CrossRef ] [ Google Scholar ]
- Flynn D, Nyhan B, Reifler J. The nature and origins of misperceptions: understanding false and unsupported beliefs about politics. Polit Psychol. 2017; 38 :127–150. doi: 10.1111/pops.12394. [ CrossRef ] [ Google Scholar ]
- Fraga-Lamas P, Fernández-Caramés TM. Fake news, disinformation, and deepfakes: leveraging distributed ledger technologies and blockchain to combat digital deception and counterfeit reality. IT Prof. 2020; 22 (2):53–59. doi: 10.1109/MITP.2020.2977589. [ CrossRef ] [ Google Scholar ]
- Freeman D, Waite F, Rosebrock L, Petit A, Causier C, East A, Jenner L, Teale AL, Carr L, Mulhall S, et al. Coronavirus conspiracy beliefs, mistrust, and compliance with government guidelines in England. Psychol Med. 2020 doi: 10.1017/S0033291720001890. [ PMC free article ] [ PubMed ] [ CrossRef ] [ Google Scholar ]
- Friggeri A, Adamic L, Eckles D, Cheng J (2014) Rumor cascades. In: Proceedings of the international AAAI conference on web and social media
- García SA, García GG, Prieto MS, Moreno Guerrero AJ, Rodríguez Jiménez C. The impact of term fake news on the scientific community. Scientific performance and mapping in web of science. Soc Sci. 2020 doi: 10.3390/socsci9050073. [ CrossRef ] [ Google Scholar ]
- Garrett RK, Bond RM. Conservatives’ susceptibility to political misperceptions. Sci Adv. 2021 doi: 10.1126/sciadv.abf1234. [ PMC free article ] [ PubMed ] [ CrossRef ] [ Google Scholar ]
- Giachanou A, Ríssola EA, Ghanem B, Crestani F, Rosso P (2020) The role of personality and linguistic patterns in discriminating between fake news spreaders and fact checkers. In: International conference on applications of natural language to information systems. Springer, Berlin, pp 181–192 10.1007/978-3-030-51310-8_17
- Golbeck J, Mauriello M, Auxier B, Bhanushali KH, Bonk C, Bouzaghrane MA, Buntain C, Chanduka R, Cheakalos P, Everett JB et al (2018) Fake news vs satire: a dataset and analysis. In: Proceedings of the 10th ACM conference on web science, pp 17–21, 10.1145/3201064.3201100
- Goldani MH, Momtazi S, Safabakhsh R. Detecting fake news with capsule neural networks. Appl Soft Comput. 2021; 101 :106991. doi: 10.1016/j.asoc.2020.106991. [ CrossRef ] [ Google Scholar ]
- Goldstein I, Yang L. Good disclosure, bad disclosure. J Financ Econ. 2019; 131 (1):118–138. doi: 10.1016/j.jfineco.2018.08.004. [ CrossRef ] [ Google Scholar ]
- Grinberg N, Joseph K, Friedland L, Swire-Thompson B, Lazer D. Fake news on Twitter during the 2016 US presidential election. Science. 2019; 363 (6425):374–378. doi: 10.1126/science.aau2706. [ PubMed ] [ CrossRef ] [ Google Scholar ]
- Guadagno RE, Guttieri K (2021) Fake news and information warfare: an examination of the political and psychological processes from the digital sphere to the real world. In: Research anthology on fake news, political warfare, and combatting the spread of misinformation. IGI Global, pp 218–242 10.4018/978-1-7998-7291-7.ch013
- Guess A, Nagler J, Tucker J. Less than you think: prevalence and predictors of fake news dissemination on Facebook. Sci Adv. 2019 doi: 10.1126/sciadv.aau4586. [ PMC free article ] [ PubMed ] [ CrossRef ] [ Google Scholar ]
- Guo C, Cao J, Zhang X, Shu K, Yu M (2019) Exploiting emotions for fake news detection on social media. arXiv preprint arXiv:1903.01728
- Guo B, Ding Y, Yao L, Liang Y, Yu Z. The future of false information detection on social media: new perspectives and trends. ACM Comput Surv (CSUR) 2020; 53 (4):1–36. doi: 10.1145/3393880. [ CrossRef ] [ Google Scholar ]
- Gupta A, Li H, Farnoush A, Jiang W. Understanding patterns of covid infodemic: a systematic and pragmatic approach to curb fake news. J Bus Res. 2022; 140 :670–683. doi: 10.1016/j.jbusres.2021.11.032. [ PMC free article ] [ PubMed ] [ CrossRef ] [ Google Scholar ]
- Ha L, Andreu Perez L, Ray R. Mapping recent development in scholarship on fake news and misinformation, 2008 to 2017: disciplinary contribution, topics, and impact. Am Behav Sci. 2021; 65 (2):290–315. doi: 10.1177/0002764219869402. [ CrossRef ] [ Google Scholar ]
- Habib A, Asghar MZ, Khan A, Habib A, Khan A. False information detection in online content and its role in decision making: a systematic literature review. Soc Netw Anal Min. 2019; 9 (1):1–20. doi: 10.1007/s13278-019-0595-5. [ CrossRef ] [ Google Scholar ]
- Hage H, Aïmeur E, Guedidi A (2021) Understanding the landscape of online deception. In: Research anthology on fake news, political warfare, and combatting the spread of misinformation. IGI Global, pp 39–66. 10.4018/978-1-7998-2543-2.ch014
- Hakak S, Alazab M, Khan S, Gadekallu TR, Maddikunta PKR, Khan WZ. An ensemble machine learning approach through effective feature extraction to classify fake news. Futur Gener Comput Syst. 2021; 117 :47–58. doi: 10.1016/j.future.2020.11.022. [ CrossRef ] [ Google Scholar ]
- Hamdi T, Slimi H, Bounhas I, Slimani Y (2020) A hybrid approach for fake news detection in Twitter based on user features and graph embedding. In: International conference on distributed computing and internet technology. Springer, Berlin, pp 266–280. 10.1007/978-3-030-36987-3_17
- Hameleers M. Separating truth from lies: comparing the effects of news media literacy interventions and fact-checkers in response to political misinformation in the us and netherlands. Inf Commun Soc. 2022; 25 (1):110–126. doi: 10.1080/1369118X.2020.1764603. [ CrossRef ] [ Google Scholar ]
- Hameleers M, Powell TE, Van Der Meer TG, Bos L. A picture paints a thousand lies? The effects and mechanisms of multimodal disinformation and rebuttals disseminated via social media. Polit Commun. 2020; 37 (2):281–301. doi: 10.1080/10584609.2019.1674979. [ CrossRef ] [ Google Scholar ]
- Hameleers M, Brosius A, de Vreese CH. Whom to trust? media exposure patterns of citizens with perceptions of misinformation and disinformation related to the news media. Eur J Commun. 2022 doi: 10.1177/02673231211072667. [ CrossRef ] [ Google Scholar ]
- Hartley K, Vu MK. Fighting fake news in the Covid-19 era: policy insights from an equilibrium model. Policy Sci. 2020; 53 (4):735–758. doi: 10.1007/s11077-020-09405-z. [ PMC free article ] [ PubMed ] [ CrossRef ] [ Google Scholar ]
- Hasan HR, Salah K. Combating deepfake videos using blockchain and smart contracts. IEEE Access. 2019; 7 :41596–41606. doi: 10.1109/ACCESS.2019.2905689. [ CrossRef ] [ Google Scholar ]
- Hiriyannaiah S, Srinivas A, Shetty GK, Siddesh G, Srinivasa K (2020) A computationally intelligent agent for detecting fake news using generative adversarial networks. Hybrid computational intelligence: challenges and applications. pp 69–96 10.1016/B978-0-12-818699-2.00004-4
- Hosseinimotlagh S, Papalexakis EE (2018) Unsupervised content-based identification of fake news articles with tensor decomposition ensembles. In: Proceedings of the workshop on misinformation and misbehavior mining on the web (MIS2)
- Huckle S, White M. Fake news: a technological approach to proving the origins of content, using blockchains. Big Data. 2017; 5 (4):356–371. doi: 10.1089/big.2017.0071. [ PubMed ] [ CrossRef ] [ Google Scholar ]
- Huffaker JS, Kummerfeld JK, Lasecki WS, Ackerman MS (2020) Crowdsourced detection of emotionally manipulative language. In: Proceedings of the 2020 CHI conference on human factors in computing systems. pp 1–14 10.1145/3313831.3376375
- Ireton C, Posetti J. Journalism, fake news & disinformation: handbook for journalism education and training. Paris: UNESCO Publishing; 2018. [ Google Scholar ]
- Islam MR, Liu S, Wang X, Xu G. Deep learning for misinformation detection on online social networks: a survey and new perspectives. Soc Netw Anal Min. 2020; 10 (1):1–20. doi: 10.1007/s13278-020-00696-x. [ PMC free article ] [ PubMed ] [ CrossRef ] [ Google Scholar ]
- Ismailov M, Tsikerdekis M, Zeadally S. Vulnerabilities to online social network identity deception detection research and recommendations for mitigation. Fut Internet. 2020; 12 (9):148. doi: 10.3390/fi12090148. [ CrossRef ] [ Google Scholar ]
- Jakesch M, Koren M, Evtushenko A, Naaman M (2019) The role of source and expressive responding in political news evaluation. In: Computation and journalism symposium
- Jamieson KH. Cyberwar: how Russian hackers and trolls helped elect a president: what we don’t, can’t, and do know. Oxford: Oxford University Press; 2020. [ Google Scholar ]
- Jiang S, Chen X, Zhang L, Chen S, Liu H (2019) User-characteristic enhanced model for fake news detection in social media. In: CCF International conference on natural language processing and Chinese computing, Springer, Berlin, pp 634–646. 10.1007/978-3-030-32233-5_49
- Jin Z, Cao J, Zhang Y, Luo J (2016) News verification by exploiting conflicting social viewpoints in microblogs. In: Proceedings of the AAAI conference on artificial intelligence
- Jing TW, Murugesan RK (2018) A theoretical framework to build trust and prevent fake news in social media using blockchain. In: International conference of reliable information and communication technology. Springer, Berlin, pp 955–962, 10.1007/978-3-319-99007-1_88
- Jones-Jang SM, Mortensen T, Liu J. Does media literacy help identification of fake news? Information literacy helps, but other literacies don’t. Am Behav Sci. 2021; 65 (2):371–388. doi: 10.1177/0002764219869406. [ CrossRef ] [ Google Scholar ]
- Jungherr A, Schroeder R. Disinformation and the structural transformations of the public arena: addressing the actual challenges to democracy. Soc Media Soc. 2021 doi: 10.1177/2056305121988928. [ CrossRef ] [ Google Scholar ]
- Kaliyar RK (2018) Fake news detection using a deep neural network. In: 2018 4th international conference on computing communication and automation (ICCCA), IEEE, pp 1–7 10.1109/CCAA.2018.8777343
- Kaliyar RK, Goswami A, Narang P, Sinha S. Fndnet—a deep convolutional neural network for fake news detection. Cogn Syst Res. 2020; 61 :32–44. doi: 10.1016/j.cogsys.2019.12.005. [ CrossRef ] [ Google Scholar ]
- Kapantai E, Christopoulou A, Berberidis C, Peristeras V. A systematic literature review on disinformation: toward a unified taxonomical framework. New Media Soc. 2021; 23 (5):1301–1326. doi: 10.1177/1461444820959296. [ CrossRef ] [ Google Scholar ]
- Kapusta J, Benko L, Munk M (2019) Fake news identification based on sentiment and frequency analysis. In: International conference Europe middle east and North Africa information systems and technologies to support learning. Springer, Berlin, pp 400–409, 10.1007/978-3-030-36778-7_44
- Kaur S, Kumar P, Kumaraguru P. Automating fake news detection system using multi-level voting model. Soft Comput. 2020; 24 (12):9049–9069. doi: 10.1007/s00500-019-04436-y. [ CrossRef ] [ Google Scholar ]
- Khan SA, Alkawaz MH, Zangana HM (2019) The use and abuse of social media for spreading fake news. In: 2019 IEEE international conference on automatic control and intelligent systems (I2CACIS), IEEE, pp 145–148. 10.1109/I2CACIS.2019.8825029
- Kim J, Tabibian B, Oh A, Schölkopf B, Gomez-Rodriguez M (2018) Leveraging the crowd to detect and reduce the spread of fake news and misinformation. In: Proceedings of the eleventh ACM international conference on web search and data mining, pp 324–332. 10.1145/3159652.3159734
- Klein D, Wueller J. Fake news: a legal perspective. J Internet Law. 2017; 20 (10):5–13. [ Google Scholar ]
- Kogan S, Moskowitz TJ, Niessner M (2019) Fake news: evidence from financial markets. Available at SSRN 3237763
- Kuklinski JH, Quirk PJ, Jerit J, Schwieder D, Rich RF. Misinformation and the currency of democratic citizenship. J Polit. 2000; 62 (3):790–816. doi: 10.1111/0022-3816.00033. [ CrossRef ] [ Google Scholar ]
- Kumar S, Shah N (2018) False information on web and social media: a survey. arXiv preprint arXiv:1804.08559
- Kumar S, West R, Leskovec J (2016) Disinformation on the web: impact, characteristics, and detection of Wikipedia hoaxes. In: Proceedings of the 25th international conference on world wide web, pp 591–602. 10.1145/2872427.2883085
- La Barbera D, Roitero K, Demartini G, Mizzaro S, Spina D (2020) Crowdsourcing truthfulness: the impact of judgment scale and assessor bias. In: European conference on information retrieval. Springer, Berlin, pp 207–214. 10.1007/978-3-030-45442-5_26
- Lanius C, Weber R, MacKenzie WI. Use of bot and content flags to limit the spread of misinformation among social networks: a behavior and attitude survey. Soc Netw Anal Min. 2021; 11 (1):1–15. doi: 10.1007/s13278-021-00739-x. [ PMC free article ] [ PubMed ] [ CrossRef ] [ Google Scholar ]
- Lazer DM, Baum MA, Benkler Y, Berinsky AJ, Greenhill KM, Menczer F, Metzger MJ, Nyhan B, Pennycook G, Rothschild D, et al. The science of fake news. Science. 2018; 359 (6380):1094–1096. doi: 10.1126/science.aao2998. [ PubMed ] [ CrossRef ] [ Google Scholar ]
- Le T, Shu K, Molina MD, Lee D, Sundar SS, Liu H (2019) 5 sources of clickbaits you should know! Using synthetic clickbaits to improve prediction and distinguish between bot-generated and human-written headlines. In: 2019 IEEE/ACM international conference on advances in social networks analysis and mining (ASONAM). IEEE, pp 33–40. 10.1145/3341161.3342875
- Lewandowsky S (2020) Climate change, disinformation, and how to combat it. In: Annual Review of Public Health 42. 10.1146/annurev-publhealth-090419-102409 [ PubMed ]
- Liu Y, Wu YF (2018) Early detection of fake news on social media through propagation path classification with recurrent and convolutional networks. In: Proceedings of the AAAI conference on artificial intelligence, pp 354–361
- Luo M, Hancock JT, Markowitz DM. Credibility perceptions and detection accuracy of fake news headlines on social media: effects of truth-bias and endorsement cues. Commun Res. 2022; 49 (2):171–195. doi: 10.1177/0093650220921321. [ CrossRef ] [ Google Scholar ]
- Lutzke L, Drummond C, Slovic P, Árvai J. Priming critical thinking: simple interventions limit the influence of fake news about climate change on Facebook. Glob Environ Chang. 2019; 58 :101964. doi: 10.1016/j.gloenvcha.2019.101964. [ CrossRef ] [ Google Scholar ]
- Maertens R, Anseel F, van der Linden S. Combatting climate change misinformation: evidence for longevity of inoculation and consensus messaging effects. J Environ Psychol. 2020; 70 :101455. doi: 10.1016/j.jenvp.2020.101455. [ CrossRef ] [ Google Scholar ]
- Mahabub A. A robust technique of fake news detection using ensemble voting classifier and comparison with other classifiers. SN Applied Sciences. 2020; 2 (4):1–9. doi: 10.1007/s42452-020-2326-y. [ CrossRef ] [ Google Scholar ]
- Mahbub S, Pardede E, Kayes A, Rahayu W. Controlling astroturfing on the internet: a survey on detection techniques and research challenges. Int J Web Grid Serv. 2019; 15 (2):139–158. doi: 10.1504/IJWGS.2019.099561. [ CrossRef ] [ Google Scholar ]
- Marsden C, Meyer T, Brown I. Platform values and democratic elections: how can the law regulate digital disinformation? Comput Law Secur Rev. 2020; 36 :105373. doi: 10.1016/j.clsr.2019.105373. [ CrossRef ] [ Google Scholar ]
- Masciari E, Moscato V, Picariello A, Sperlí G (2020) Detecting fake news by image analysis. In: Proceedings of the 24th symposium on international database engineering and applications, pp 1–5. 10.1145/3410566.3410599
- Mazzeo V, Rapisarda A. Investigating fake and reliable news sources using complex networks analysis. Front Phys. 2022; 10 :886544. doi: 10.3389/fphy.2022.886544. [ CrossRef ] [ Google Scholar ]
- McGrew S. Learning to evaluate: an intervention in civic online reasoning. Comput Educ. 2020; 145 :103711. doi: 10.1016/j.compedu.2019.103711. [ CrossRef ] [ Google Scholar ]
- McGrew S, Breakstone J, Ortega T, Smith M, Wineburg S. Can students evaluate online sources? Learning from assessments of civic online reasoning. Theory Res Soc Educ. 2018; 46 (2):165–193. doi: 10.1080/00933104.2017.1416320. [ CrossRef ] [ Google Scholar ]
- Meel P, Vishwakarma DK. Fake news, rumor, information pollution in social media and web: a contemporary survey of state-of-the-arts, challenges and opportunities. Expert Syst Appl. 2020; 153 :112986. doi: 10.1016/j.eswa.2019.112986. [ CrossRef ] [ Google Scholar ]
- Meese J, Frith J, Wilken R. Covid-19, 5G conspiracies and infrastructural futures. Media Int Aust. 2020; 177 (1):30–46. doi: 10.1177/1329878X20952165. [ CrossRef ] [ Google Scholar ]
- Metzger MJ, Hartsell EH, Flanagin AJ. Cognitive dissonance or credibility? A comparison of two theoretical explanations for selective exposure to partisan news. Commun Res. 2020; 47 (1):3–28. doi: 10.1177/0093650215613136. [ CrossRef ] [ Google Scholar ]
- Micallef N, He B, Kumar S, Ahamad M, Memon N (2020) The role of the crowd in countering misinformation: a case study of the Covid-19 infodemic. arXiv preprint arXiv:2011.05773
- Mihailidis P, Viotty S. Spreadable spectacle in digital culture: civic expression, fake news, and the role of media literacies in “post-fact society. Am Behav Sci. 2017; 61 (4):441–454. doi: 10.1177/0002764217701217. [ CrossRef ] [ Google Scholar ]
- Mishra R (2020) Fake news detection using higher-order user to user mutual-attention progression in propagation paths. In: Proceedings of the IEEE/CVF conference on computer vision and pattern recognition workshops, pp 652–653
- Mishra S, Shukla P, Agarwal R. Analyzing machine learning enabled fake news detection techniques for diversified datasets. Wirel Commun Mobile Comput. 2022 doi: 10.1155/2022/1575365. [ CrossRef ] [ Google Scholar ]
- Molina MD, Sundar SS, Le T, Lee D. “Fake news” is not simply false information: a concept explication and taxonomy of online content. Am Behav Sci. 2021; 65 (2):180–212. doi: 10.1177/0002764219878224. [ CrossRef ] [ Google Scholar ]
- Moro C, Birt JR (2022) Review bombing is a dirty practice, but research shows games do benefit from online feedback. Conversation. https://research.bond.edu.au/en/publications/review-bombing-is-a-dirty-practice-but-research-shows-games-do-be
- Mustafaraj E, Metaxas PT (2017) The fake news spreading plague: was it preventable? In: Proceedings of the 2017 ACM on web science conference, pp 235–239. 10.1145/3091478.3091523
- Nagel TW. Measuring fake news acumen using a news media literacy instrument. J Media Liter Educ. 2022; 14 (1):29–42. doi: 10.23860/JMLE-2022-14-1-3. [ CrossRef ] [ Google Scholar ]
- Nakov P (2020) Can we spot the “fake news” before it was even written? arXiv preprint arXiv:2008.04374
- Nekmat E. Nudge effect of fact-check alerts: source influence and media skepticism on sharing of news misinformation in social media. Soc Media Soc. 2020 doi: 10.1177/2056305119897322. [ CrossRef ] [ Google Scholar ]
- Nygren T, Brounéus F, Svensson G. Diversity and credibility in young people’s news feeds: a foundation for teaching and learning citizenship in a digital era. J Soc Sci Educ. 2019; 18 (2):87–109. doi: 10.4119/jsse-917. [ CrossRef ] [ Google Scholar ]
- Nyhan B, Reifler J. Displacing misinformation about events: an experimental test of causal corrections. J Exp Polit Sci. 2015; 2 (1):81–93. doi: 10.1017/XPS.2014.22. [ CrossRef ] [ Google Scholar ]
- Nyhan B, Porter E, Reifler J, Wood TJ. Taking fact-checks literally but not seriously? The effects of journalistic fact-checking on factual beliefs and candidate favorability. Polit Behav. 2020; 42 (3):939–960. doi: 10.1007/s11109-019-09528-x. [ CrossRef ] [ Google Scholar ]
- Nyow NX, Chua HN (2019) Detecting fake news with tweets’ properties. In: 2019 IEEE conference on application, information and network security (AINS), IEEE, pp 24–29. 10.1109/AINS47559.2019.8968706
- Ochoa IS, de Mello G, Silva LA, Gomes AJ, Fernandes AM, Leithardt VRQ (2019) Fakechain: a blockchain architecture to ensure trust in social media networks. In: International conference on the quality of information and communications technology. Springer, Berlin, pp 105–118. 10.1007/978-3-030-29238-6_8
- Ozbay FA, Alatas B. Fake news detection within online social media using supervised artificial intelligence algorithms. Physica A. 2020; 540 :123174. doi: 10.1016/j.physa.2019.123174. [ CrossRef ] [ Google Scholar ]
- Ozturk P, Li H, Sakamoto Y (2015) Combating rumor spread on social media: the effectiveness of refutation and warning. In: 2015 48th Hawaii international conference on system sciences, IEEE, pp 2406–2414. 10.1109/HICSS.2015.288
- Parikh SB, Atrey PK (2018) Media-rich fake news detection: a survey. In: 2018 IEEE conference on multimedia information processing and retrieval (MIPR), IEEE, pp 436–441.10.1109/MIPR.2018.00093
- Parrish K (2018) Deep learning & machine learning: what’s the difference? Online: https://parsers.me/deep-learning-machine-learning-whats-the-difference/ . Accessed 20 May 2020
- Paschen J. Investigating the emotional appeal of fake news using artificial intelligence and human contributions. J Prod Brand Manag. 2019; 29 (2):223–233. doi: 10.1108/JPBM-12-2018-2179. [ CrossRef ] [ Google Scholar ]
- Pathak A, Srihari RK (2019) Breaking! Presenting fake news corpus for automated fact checking. In: Proceedings of the 57th annual meeting of the association for computational linguistics: student research workshop, pp 357–362
- Peng J, Detchon S, Choo KKR, Ashman H. Astroturfing detection in social media: a binary n-gram-based approach. Concurr Comput: Pract Exp. 2017; 29 (17):e4013. doi: 10.1002/cpe.4013. [ CrossRef ] [ Google Scholar ]
- Pennycook G, Rand DG. Fighting misinformation on social media using crowdsourced judgments of news source quality. Proc Natl Acad Sci. 2019; 116 (7):2521–2526. doi: 10.1073/pnas.1806781116. [ PMC free article ] [ PubMed ] [ CrossRef ] [ Google Scholar ]
- Pennycook G, Rand DG. Who falls for fake news? The roles of bullshit receptivity, overclaiming, familiarity, and analytic thinking. J Pers. 2020; 88 (2):185–200. doi: 10.1111/jopy.12476. [ PubMed ] [ CrossRef ] [ Google Scholar ]
- Pennycook G, Bear A, Collins ET, Rand DG. The implied truth effect: attaching warnings to a subset of fake news headlines increases perceived accuracy of headlines without warnings. Manag Sci. 2020; 66 (11):4944–4957. doi: 10.1287/mnsc.2019.3478. [ CrossRef ] [ Google Scholar ]
- Pennycook G, McPhetres J, Zhang Y, Lu JG, Rand DG. Fighting Covid-19 misinformation on social media: experimental evidence for a scalable accuracy-nudge intervention. Psychol Sci. 2020; 31 (7):770–780. doi: 10.1177/0956797620939054. [ PMC free article ] [ PubMed ] [ CrossRef ] [ Google Scholar ]
- Potthast M, Kiesel J, Reinartz K, Bevendorff J, Stein B (2017) A stylometric inquiry into hyperpartisan and fake news. arXiv preprint arXiv:1702.05638
- Previti M, Rodriguez-Fernandez V, Camacho D, Carchiolo V, Malgeri M (2020) Fake news detection using time series and user features classification. In: International conference on the applications of evolutionary computation (Part of EvoStar), Springer, Berlin, pp 339–353. 10.1007/978-3-030-43722-0_22
- Przybyla P (2020) Capturing the style of fake news. In: Proceedings of the AAAI conference on artificial intelligence, pp 490–497. 10.1609/aaai.v34i01.5386
- Qayyum A, Qadir J, Janjua MU, Sher F. Using blockchain to rein in the new post-truth world and check the spread of fake news. IT Prof. 2019; 21 (4):16–24. doi: 10.1109/MITP.2019.2910503. [ CrossRef ] [ Google Scholar ]
- Qian F, Gong C, Sharma K, Liu Y (2018) Neural user response generator: fake news detection with collective user intelligence. In: IJCAI, vol 18, pp 3834–3840. 10.24963/ijcai.2018/533
- Raza S, Ding C. Fake news detection based on news content and social contexts: a transformer-based approach. Int J Data Sci Anal. 2022; 13 (4):335–362. doi: 10.1007/s41060-021-00302-z. [ PMC free article ] [ PubMed ] [ CrossRef ] [ Google Scholar ]
- Ricard J, Medeiros J (2020) Using misinformation as a political weapon: Covid-19 and Bolsonaro in Brazil. Harv Kennedy School misinformation Rev 1(3). https://misinforeview.hks.harvard.edu/article/using-misinformation-as-a-political-weapon-covid-19-and-bolsonaro-in-brazil/
- Roozenbeek J, van der Linden S. Fake news game confers psychological resistance against online misinformation. Palgrave Commun. 2019; 5 (1):1–10. doi: 10.1057/s41599-019-0279-9. [ CrossRef ] [ Google Scholar ]
- Roozenbeek J, van der Linden S, Nygren T. Prebunking interventions based on the psychological theory of “inoculation” can reduce susceptibility to misinformation across cultures. Harv Kennedy School Misinformation Rev. 2020 doi: 10.37016//mr-2020-008. [ CrossRef ] [ Google Scholar ]
- Roozenbeek J, Schneider CR, Dryhurst S, Kerr J, Freeman AL, Recchia G, Van Der Bles AM, Van Der Linden S. Susceptibility to misinformation about Covid-19 around the world. R Soc Open Sci. 2020; 7 (10):201199. doi: 10.1098/rsos.201199. [ PMC free article ] [ PubMed ] [ CrossRef ] [ Google Scholar ]
- Rubin VL, Conroy N, Chen Y, Cornwell S (2016) Fake news or truth? Using satirical cues to detect potentially misleading news. In: Proceedings of the second workshop on computational approaches to deception detection, pp 7–17
- Ruchansky N, Seo S, Liu Y (2017) Csi: a hybrid deep model for fake news detection. In: Proceedings of the 2017 ACM on conference on information and knowledge management, pp 797–806. 10.1145/3132847.3132877
- Schuyler AJ (2019) Regulating facts: a procedural framework for identifying, excluding, and deterring the intentional or knowing proliferation of fake news online. Univ Ill JL Technol Pol’y, vol 2019, pp 211–240
- Shae Z, Tsai J (2019) AI blockchain platform for trusting news. In: 2019 IEEE 39th international conference on distributed computing systems (ICDCS), IEEE, pp 1610–1619. 10.1109/ICDCS.2019.00160
- Shang W, Liu M, Lin W, Jia M (2018) Tracing the source of news based on blockchain. In: 2018 IEEE/ACIS 17th international conference on computer and information science (ICIS), IEEE, pp 377–381. 10.1109/ICIS.2018.8466516
- Shao C, Ciampaglia GL, Flammini A, Menczer F (2016) Hoaxy: A platform for tracking online misinformation. In: Proceedings of the 25th international conference companion on world wide web, pp 745–750. 10.1145/2872518.2890098
- Shao C, Ciampaglia GL, Varol O, Yang KC, Flammini A, Menczer F. The spread of low-credibility content by social bots. Nat Commun. 2018; 9 (1):1–9. doi: 10.1038/s41467-018-06930-7. [ PMC free article ] [ PubMed ] [ CrossRef ] [ Google Scholar ]
- Shao C, Hui PM, Wang L, Jiang X, Flammini A, Menczer F, Ciampaglia GL. Anatomy of an online misinformation network. PLoS ONE. 2018; 13 (4):e0196087. doi: 10.1371/journal.pone.0196087. [ PMC free article ] [ PubMed ] [ CrossRef ] [ Google Scholar ]
- Sharma K, Qian F, Jiang H, Ruchansky N, Zhang M, Liu Y. Combating fake news: a survey on identification and mitigation techniques. ACM Trans Intell Syst Technol (TIST) 2019; 10 (3):1–42. doi: 10.1145/3305260. [ CrossRef ] [ Google Scholar ]
- Sharma K, Seo S, Meng C, Rambhatla S, Liu Y (2020) Covid-19 on social media: analyzing misinformation in Twitter conversations. arXiv preprint arXiv:2003.12309
- Shen C, Kasra M, Pan W, Bassett GA, Malloch Y, O’Brien JF. Fake images: the effects of source, intermediary, and digital media literacy on contextual assessment of image credibility online. New Media Soc. 2019; 21 (2):438–463. doi: 10.1177/1461444818799526. [ CrossRef ] [ Google Scholar ]
- Sherman IN, Redmiles EM, Stokes JW (2020) Designing indicators to combat fake media. arXiv preprint arXiv:2010.00544
- Shi P, Zhang Z, Choo KKR. Detecting malicious social bots based on clickstream sequences. IEEE Access. 2019; 7 :28855–28862. doi: 10.1109/ACCESS.2019.2901864. [ CrossRef ] [ Google Scholar ]
- Shu K, Sliva A, Wang S, Tang J, Liu H. Fake news detection on social media: a data mining perspective. ACM SIGKDD Explor Newsl. 2017; 19 (1):22–36. doi: 10.1145/3137597.3137600. [ CrossRef ] [ Google Scholar ]
- Shu K, Mahudeswaran D, Wang S, Lee D, Liu H (2018a) Fakenewsnet: a data repository with news content, social context and spatialtemporal information for studying fake news on social media. arXiv preprint arXiv:1809.01286 , 10.1089/big.2020.0062 [ PubMed ]
- Shu K, Wang S, Liu H (2018b) Understanding user profiles on social media for fake news detection. In: 2018 IEEE conference on multimedia information processing and retrieval (MIPR), IEEE, pp 430–435. 10.1109/MIPR.2018.00092
- Shu K, Wang S, Liu H (2019a) Beyond news contents: the role of social context for fake news detection. In: Proceedings of the twelfth ACM international conference on web search and data mining, pp 312–320. 10.1145/3289600.3290994
- Shu K, Zhou X, Wang S, Zafarani R, Liu H (2019b) The role of user profiles for fake news detection. In: Proceedings of the 2019 IEEE/ACM international conference on advances in social networks analysis and mining, pp 436–439. 10.1145/3341161.3342927
- Shu K, Bhattacharjee A, Alatawi F, Nazer TH, Ding K, Karami M, Liu H. Combating disinformation in a social media age. Wiley Interdiscip Rev: Data Min Knowl Discov. 2020; 10 (6):e1385. doi: 10.1002/widm.1385. [ CrossRef ] [ Google Scholar ]
- Shu K, Mahudeswaran D, Wang S, Liu H. Hierarchical propagation networks for fake news detection: investigation and exploitation. Proc Int AAAI Conf Web Soc Media AAAI Press. 2020; 14 :626–637. [ Google Scholar ]
- Shu K, Wang S, Lee D, Liu H (2020c) Mining disinformation and fake news: concepts, methods, and recent advancements. In: Disinformation, misinformation, and fake news in social media. Springer, Berlin, pp 1–19 10.1007/978-3-030-42699-6_1
- Shu K, Zheng G, Li Y, Mukherjee S, Awadallah AH, Ruston S, Liu H (2020d) Early detection of fake news with multi-source weak social supervision. In: ECML/PKDD (3), pp 650–666
- Singh VK, Ghosh I, Sonagara D. Detecting fake news stories via multimodal analysis. J Am Soc Inf Sci. 2021; 72 (1):3–17. doi: 10.1002/asi.24359. [ CrossRef ] [ Google Scholar ]
- Sintos S, Agarwal PK, Yang J (2019) Selecting data to clean for fact checking: minimizing uncertainty vs. maximizing surprise. Proc VLDB Endowm 12(13), 2408–2421. 10.14778/3358701.3358708 [ CrossRef ]
- Snow J (2017) Can AI win the war against fake news? MIT Technology Review Online: https://www.technologyreview.com/s/609717/can-ai-win-the-war-against-fake-news/ . Accessed 3 Oct. 2020
- Song G, Kim S, Hwang H, Lee K (2019) Blockchain-based notarization for social media. In: 2019 IEEE international conference on consumer clectronics (ICCE), IEEE, pp 1–2 10.1109/ICCE.2019.8661978
- Starbird K, Arif A, Wilson T (2019) Disinformation as collaborative work: Surfacing the participatory nature of strategic information operations. In: Proceedings of the ACM on human–computer interaction, vol 3(CSCW), pp 1–26 10.1145/3359229
- Sterret D, Malato D, Benz J, Kantor L, Tompson T, Rosenstiel T, Sonderman J, Loker K, Swanson E (2018) Who shared it? How Americans decide what news to trust on social media. Technical report, Norc Working Paper Series, WP-2018-001, pp 1–24
- Sutton RM, Douglas KM. Conspiracy theories and the conspiracy mindset: implications for political ideology. Curr Opin Behav Sci. 2020; 34 :118–122. doi: 10.1016/j.cobeha.2020.02.015. [ CrossRef ] [ Google Scholar ]
- Tandoc EC, Jr, Thomas RJ, Bishop L. What is (fake) news? Analyzing news values (and more) in fake stories. Media Commun. 2021; 9 (1):110–119. doi: 10.17645/mac.v9i1.3331. [ CrossRef ] [ Google Scholar ]
- Tchakounté F, Faissal A, Atemkeng M, Ntyam A. A reliable weighting scheme for the aggregation of crowd intelligence to detect fake news. Information. 2020; 11 (6):319. doi: 10.3390/info11060319. [ CrossRef ] [ Google Scholar ]
- Tchechmedjiev A, Fafalios P, Boland K, Gasquet M, Zloch M, Zapilko B, Dietze S, Todorov K (2019) Claimskg: a knowledge graph of fact-checked claims. In: International semantic web conference. Springer, Berlin, pp 309–324 10.1007/978-3-030-30796-7_20
- Treen KMd, Williams HT, O’Neill SJ. Online misinformation about climate change. Wiley Interdiscip Rev Clim Change. 2020; 11 (5):e665. doi: 10.1002/wcc.665. [ CrossRef ] [ Google Scholar ]
- Tsang SJ. Motivated fake news perception: the impact of news sources and policy support on audiences’ assessment of news fakeness. J Mass Commun Q. 2020 doi: 10.1177/1077699020952129. [ CrossRef ] [ Google Scholar ]
- Tschiatschek S, Singla A, Gomez Rodriguez M, Merchant A, Krause A (2018) Fake news detection in social networks via crowd signals. In: Companion proceedings of the the web conference 2018, pp 517–524. 10.1145/3184558.3188722
- Uppada SK, Manasa K, Vidhathri B, Harini R, Sivaselvan B. Novel approaches to fake news and fake account detection in OSNS: user social engagement and visual content centric model. Soc Netw Anal Min. 2022; 12 (1):1–19. doi: 10.1007/s13278-022-00878-9. [ PMC free article ] [ PubMed ] [ CrossRef ] [ Google Scholar ]
- Van der Linden S, Roozenbeek J (2020) Psychological inoculation against fake news. In: Accepting, sharing, and correcting misinformation, the psychology of fake news. 10.4324/9780429295379-11
- Van der Linden S, Panagopoulos C, Roozenbeek J. You are fake news: political bias in perceptions of fake news. Media Cult Soc. 2020; 42 (3):460–470. doi: 10.1177/0163443720906992. [ CrossRef ] [ Google Scholar ]
- Valenzuela S, Muñiz C, Santos M. Social media and belief in misinformation in mexico: a case of maximal panic, minimal effects? Int J Press Polit. 2022 doi: 10.1177/19401612221088988. [ CrossRef ] [ Google Scholar ]
- Vasu N, Ang B, Teo TA, Jayakumar S, Raizal M, Ahuja J (2018) Fake news: national security in the post-truth era. RSIS
- Vereshchaka A, Cosimini S, Dong W (2020) Analyzing and distinguishing fake and real news to mitigate the problem of disinformation. In: Computational and mathematical organization theory, pp 1–15. 10.1007/s10588-020-09307-8
- Verstraete M, Bambauer DE, Bambauer JR (2017) Identifying and countering fake news. Arizona legal studies discussion paper 73(17-15). 10.2139/ssrn.3007971
- Vilmer J, Escorcia A, Guillaume M, Herrera J (2018) Information manipulation: a challenge for our democracies. In: Report by the Policy Planning Staff (CAPS) of the ministry for europe and foreign affairs, and the institute for strategic research (RSEM) of the Ministry for the Armed Forces
- Vishwakarma DK, Varshney D, Yadav A. Detection and veracity analysis of fake news via scrapping and authenticating the web search. Cogn Syst Res. 2019; 58 :217–229. doi: 10.1016/j.cogsys.2019.07.004. [ CrossRef ] [ Google Scholar ]
- Vlachos A, Riedel S (2014) Fact checking: task definition and dataset construction. In: Proceedings of the ACL 2014 workshop on language technologies and computational social science, pp 18–22. 10.3115/v1/W14-2508
- von der Weth C, Abdul A, Fan S, Kankanhalli M (2020) Helping users tackle algorithmic threats on social media: a multimedia research agenda. In: Proceedings of the 28th ACM international conference on multimedia, pp 4425–4434. 10.1145/3394171.3414692
- Vosoughi S, Roy D, Aral S. The spread of true and false news online. Science. 2018; 359 (6380):1146–1151. doi: 10.1126/science.aap9559. [ PubMed ] [ CrossRef ] [ Google Scholar ]
- Vraga EK, Bode L. Using expert sources to correct health misinformation in social media. Sci Commun. 2017; 39 (5):621–645. doi: 10.1177/1075547017731776. [ CrossRef ] [ Google Scholar ]
- Waldman AE. The marketplace of fake news. Univ Pa J Const Law. 2017; 20 :845. [ Google Scholar ]
- Wang WY (2017) “Liar, liar pants on fire”: a new benchmark dataset for fake news detection. arXiv preprint arXiv:1705.00648
- Wang L, Wang Y, de Melo G, Weikum G. Understanding archetypes of fake news via fine-grained classification. Soc Netw Anal Min. 2019; 9 (1):1–17. doi: 10.1007/s13278-019-0580-z. [ CrossRef ] [ Google Scholar ]
- Wang Y, Han H, Ding Y, Wang X, Liao Q (2019b) Learning contextual features with multi-head self-attention for fake news detection. In: International conference on cognitive computing. Springer, Berlin, pp 132–142. 10.1007/978-3-030-23407-2_11
- Wang Y, McKee M, Torbica A, Stuckler D. Systematic literature review on the spread of health-related misinformation on social media. Soc Sci Med. 2019; 240 :112552. doi: 10.1016/j.socscimed.2019.112552. [ PMC free article ] [ PubMed ] [ CrossRef ] [ Google Scholar ]
- Wang Y, Yang W, Ma F, Xu J, Zhong B, Deng Q, Gao J (2020) Weak supervision for fake news detection via reinforcement learning. In: Proceedings of the AAAI conference on artificial intelligence, pp 516–523. 10.1609/aaai.v34i01.5389
- Wardle C (2017) Fake news. It’s complicated. Online: https://medium.com/1st-draft/fake-news-its-complicated-d0f773766c79 . Accessed 3 Oct 2020
- Wardle C. The need for smarter definitions and practical, timely empirical research on information disorder. Digit J. 2018; 6 (8):951–963. doi: 10.1080/21670811.2018.1502047. [ CrossRef ] [ Google Scholar ]
- Wardle C, Derakhshan H. Information disorder: toward an interdisciplinary framework for research and policy making. Council Eur Rep. 2017; 27 :1–107. [ Google Scholar ]
- Weiss AP, Alwan A, Garcia EP, Garcia J. Surveying fake news: assessing university faculty’s fragmented definition of fake news and its impact on teaching critical thinking. Int J Educ Integr. 2020; 16 (1):1–30. doi: 10.1007/s40979-019-0049-x. [ CrossRef ] [ Google Scholar ]
- Wu L, Liu H (2018) Tracing fake-news footprints: characterizing social media messages by how they propagate. In: Proceedings of the eleventh ACM international conference on web search and data mining, pp 637–645. 10.1145/3159652.3159677
- Wu L, Rao Y (2020) Adaptive interaction fusion networks for fake news detection. arXiv preprint arXiv:2004.10009
- Wu L, Morstatter F, Carley KM, Liu H. Misinformation in social media: definition, manipulation, and detection. ACM SIGKDD Explor Newsl. 2019; 21 (2):80–90. doi: 10.1145/3373464.3373475. [ CrossRef ] [ Google Scholar ]
- Wu Y, Ngai EW, Wu P, Wu C. Fake news on the internet: a literature review, synthesis and directions for future research. Intern Res. 2022 doi: 10.1108/INTR-05-2021-0294. [ CrossRef ] [ Google Scholar ]
- Xu K, Wang F, Wang H, Yang B. Detecting fake news over online social media via domain reputations and content understanding. Tsinghua Sci Technol. 2019; 25 (1):20–27. doi: 10.26599/TST.2018.9010139. [ CrossRef ] [ Google Scholar ]
- Yang F, Pentyala SK, Mohseni S, Du M, Yuan H, Linder R, Ragan ED, Ji S, Hu X (2019a) Xfake: explainable fake news detector with visualizations. In: The world wide web conference, pp 3600–3604. 10.1145/3308558.3314119
- Yang X, Li Y, Lyu S (2019b) Exposing deep fakes using inconsistent head poses. In: ICASSP 2019-2019 IEEE international conference on acoustics, speech and signal processing (ICASSP), IEEE, pp 8261–8265. 10.1109/ICASSP.2019.8683164
- Yaqub W, Kakhidze O, Brockman ML, Memon N, Patil S (2020) Effects of credibility indicators on social media news sharing intent. In: Proceedings of the 2020 CHI conference on human factors in computing systems, pp 1–14. 10.1145/3313831.3376213
- Yavary A, Sajedi H, Abadeh MS. Information verification in social networks based on user feedback and news agencies. Soc Netw Anal Min. 2020; 10 (1):1–8. doi: 10.1007/s13278-019-0616-4. [ CrossRef ] [ Google Scholar ]
- Yazdi KM, Yazdi AM, Khodayi S, Hou J, Zhou W, Saedy S. Improving fake news detection using k-means and support vector machine approaches. Int J Electron Commun Eng. 2020; 14 (2):38–42. doi: 10.5281/zenodo.3669287. [ CrossRef ] [ Google Scholar ]
- Zannettou S, Sirivianos M, Blackburn J, Kourtellis N. The web of false information: rumors, fake news, hoaxes, clickbait, and various other shenanigans. J Data Inf Qual (JDIQ) 2019; 11 (3):1–37. doi: 10.1145/3309699. [ CrossRef ] [ Google Scholar ]
- Zellers R, Holtzman A, Rashkin H, Bisk Y, Farhadi A, Roesner F, Choi Y (2019) Defending against neural fake news. arXiv preprint arXiv:1905.12616
- Zhang X, Ghorbani AA. An overview of online fake news: characterization, detection, and discussion. Inf Process Manag. 2020; 57 (2):102025. doi: 10.1016/j.ipm.2019.03.004. [ CrossRef ] [ Google Scholar ]
- Zhang J, Dong B, Philip SY (2020) Fakedetector: effective fake news detection with deep diffusive neural network. In: 2020 IEEE 36th international conference on data engineering (ICDE), IEEE, pp 1826–1829. 10.1109/ICDE48307.2020.00180
- Zhang Q, Lipani A, Liang S, Yilmaz E (2019a) Reply-aided detection of misinformation via Bayesian deep learning. In: The world wide web conference, pp 2333–2343. 10.1145/3308558.3313718
- Zhang X, Karaman S, Chang SF (2019b) Detecting and simulating artifacts in GAN fake images. In: 2019 IEEE international workshop on information forensics and security (WIFS), IEEE, pp 1–6 10.1109/WIFS47025.2019.9035107
- Zhou X, Zafarani R. A survey of fake news: fundamental theories, detection methods, and opportunities. ACM Comput Surv (CSUR) 2020; 53 (5):1–40. doi: 10.1145/3395046. [ CrossRef ] [ Google Scholar ]
- Zubiaga A, Aker A, Bontcheva K, Liakata M, Procter R. Detection and resolution of rumours in social media: a survey. ACM Comput Surv (CSUR) 2018; 51 (2):1–36. doi: 10.1145/3161603. [ CrossRef ] [ Google Scholar ]
Free to Speak - Safe to Learn Democratic Schools for All
Dealing with propaganda, misinformation and fake news.

It is vital for schools to provide students with a solid education on media and information literacy as part of the curriculum.
Teachers must be well-trained in the subject to empower students with the necessary competences to critically understand and assess information reported by all forms of media.
Projects in partnership with national and local authorities and media organisations are encouraged.
Facts & figures
Two thirds of EU citizens report coming across fake news at least once a week.[ 1 ]
Over 80% of EU citizens say they see fake news both as an issue for their country and for democracy in general.[ 2 ]
Half of EU citizens aged 15-30 say they need critical thinking and information skills to help them combat fake news and extremism in society.[ 3 ]
What is propaganda, misinformation and fake news?
The terms ‘propaganda’, ‘misinformation’ and ‘fake news’ often overlap in meaning. They are used to refer to a range of ways in which sharing information causes harm, intentionally or unintentionally – usually in relation to the promotion of a particular moral or political cause or point of view .
It is possible to separate out three clearly different uses of information which fall into this category:
- Mis-information - false information shared with no intention of causing harm
- Dis-information - false information shared intentionally to cause harm
- Mal-information - true information shared intentionally to cause harm.[ 4 ]
Although none of these phenomena are new, they have taken on new significance recently with the widespread availability of sophisticated forms of information and communication technology. The sharing of text, images, videos, or links online, for example, allows information to go viral within hours.
Why is propaganda, misinformation and fake news important at school?
Since information and communication technology is so central to their lives nowadays, young people are particularly vulnerable to propaganda, misinformation and fake news . Young people spend a significant amount of their time watching television, playing online games, chatting, blogging, listening to music, posting photos of themselves and searching for other people with whom to communicate online. They rely heavily on information circulated online for their knowledge of the world and how they perceive reality. Many parents do not have sufficient technical competence to keep up with their children’s online activity, or educate them about the risks they might be facing. Schools, therefore, have a duty to provide young people with the critical and information skills which they cannot access at home .
“The significant rise of fake news as propaganda in recent years makes it critical that students have the skills they need to identify truth and discern bias.”[ 5 ]
The ability to respond critically to online propaganda, misinformation and fake news is more than a safe-guarding tool, however, it is also an important democratic competence in its own right. Analytical and critical thinking, and knowledge and critical understanding of the world, including the role of language and communication lie at the heart of the Council of Europe Reference Framework of Competences for Democratic Culture . They are central to Digital Citizenship Education and Media and Information Literacy .[ 6 ]
“School is the one place where it is absolutely crucial to train future citizens to understand, to criticise and to create information. It is in schools that the digital citizen must begin and maintain constant critical thinking in order to attain meaningful participation in his or her community.”[ 7 ]
The ability to handle off-line as well as online propaganda, misinformation and fake news is also a key skill in a number of other school subjects , e.g., History, Social Studies, Science, Religious Studies and Art. Young people may study the use of nationalistic and patriotic slogans, or so-called ‘atrocity propaganda’ in WW1 in History, for example; or art forms designed to support particular ideologies in Art lessons.
Another area in which information and communication technology is becoming an issue for schools is through adverse comments made about teachers and schools on social media . Schools are finding that parents and others increasingly turn to social media when they have a dispute or disagreement with their school, e.g., over school rules, school policies, or staff behaviour. How to handle online critical or defamatory comments or campaigns of this sort has become a matter of concern for leaders and managers in some schools.[ 8 ]
What are the challenges?
There are a number of challenges facing schools wishing to take propaganda, misinformation and fake news seriously as an educational or social issue:
- Teachers’ own online activity and area of experience is often quite limited and frequently lags behind that of their students . This can make them reticent to take on this area of teaching and learning without a significant commitment to professional development.
- The speed with which technology and young peoples’ online activity changes makes it difficult for teachers to keep up-to-date with recent developments. Even professional development programmes can go rapidly out-of-date.
- It can be difficult finding a discrete slot in the school timetable where issues relating to the creation and sharing of information can be taught. While aspects may be raised in a number of subjects, it can be a problem finding a space in an over-full curriculum where the phenomenon can be dealt with head-on as an issue in its own right.
- The description ‘fake news’ does not mean there is such a category as ‘true’ news. All news is a selection and written to suit a particular audience for a particular purpose. Providing the depth of analysis and sophisticated skills that do justice to this topic can be a challenge for some schools, especially in terms of teacher competence and training.
“States should take measures to promote media and digital literacy, including by covering these topics as part of the regular school curriculum and by engaging with civil society and other stakeholders to raise awareness about these issues.”[ 9 ]
How can schools get active?
Providing training for teachers on media and information literacy is the key to raising the profile of the issue in schools. Even though it may have a tendency to date, training can at least alert teaching staff to the importance of this area of learning for their students. The more important teachers see the area, the more they will feel the need to continuously up-date their skills themselves.
While it is important to recruit as many teachers as possible to this work, it can be more effective in the long run to start by appointing an individual teacher, or a small team , to lead on media and information literacy in the school. This element of specialist expertise can be charged with:
- Keeping staff up to date with new developments in information and communication technology
- Training them in strategies for handling propaganda, misinformation and fake news
- Helping them integrate these issues into the curriculum of different subjects
- Leading on school-policy development and action planning in this area.
In addition to these sorts of developments, there are a number of other initiatives a school can take to meet the challenges of the rapidly changing world of online propaganda, misinformation and fake news. These include:
- Special days or events in school on the subject of propaganda, misinformation or fake news as a way of overcoming the problems of an over-crowded formal curriculum
- Peer education initiatives in which older students instruct and counsel younger students in the safe handling of information they access in the media
- Partnerships with outside professionals or companies with expertise in this area, e.g., journalists, IT companies, universities
- Virtual links with schools in other regions or countries enabling students to get a different perspective on news and current affairs
- Recruiting parents with expertise in information and communication technology to help with school policy development or work alongside teaching staff to enrich student learning.
[1] Flash Eurobarometer 464 , 2018
[3] Flash Eurobarometer 455, 2018
[4] Wardle & Derakhshan, H., 2017. Information Disorder: Toward an interdisciplinary framework for research and policy making. Strasbourg, France: Council of Europe.
[5] When is fake news propaganda?, Facing History and Ourselves , 2018
[6] Digital Citizenship Education Handbook , 2019
[8] Council of Europe: Managing Controversy: a whole school training tool , 2017
[9] OSCE: Joint declaration on freedom of expression and “fake news”, disinformation and propaganda
Resources on Dealing with Propaganda, misinformation and fake news
Crossroads of European Histories - Multiple Outlooks on Five Key Moments in the History of Europe (CD + Book) (2009)
Education for Democracy and Human Rights in 10 Steps (2017)
Shared histories for a Europe without dividing lines (2014)
Through the Wild Web Woods – An online Internet safety game for children - Teachers guide (2013)
What is the Charter on citizenship and human rights education?
Official texts
Comparative study on blocking, filtering and take-down of illegal internet content (2017)
Guidelines for educators on combating intolerance and discrimination against Muslims – Addressing Islamophobia through Education (2011)
Policy documents
Compasito - manual on human rights education for children (2000)
Compass - manual for human rights education with young people online resource (2002)
Digital citizenship education - Volume 1: Overview and new perspective. Supporting children and young people to participate safely, effectively, critically and responsibly in a world filled with social media and digital technologies. (2017)
Digital Citizenship Education (DCE) - 10 Domains leaflet
Gender Matters. A manual on addressing gender-based violence affecting young people 2007 (reprint 2013)
Intercultural dialogue on Campus (Council of Europe higher education series No. 11) (2009)
Language support for adult refugees: a Council of Europe toolkit (2017)
Living with Controversy - Teaching Controversial Issues Through Education for Democratic Citizenship and Human Rights (EDC/HRE) (2016)
Managing controversy (2017). A self-reflection tool for school leaders and senior managers
Open minds, free minds - No easy prey for counterfeit medicines and similarly dangerous medicines - Psycho-pedagogical concept guide for teachers (2015)
Quality history education in the 21st century. Principles and guidelines (2018)
White paper on Intercultural dialogue “Living together as equals in dignity” (2008)
Young people building Europe (2016)
“Developing competences for Democratic Culture in the digital era” strategy paper (2017)
All different – all Equal (2016). Education pack
Developing a culture of co-operation when teaching and learning history. Training units for teachers (2016)
Internet literacy handbook (2017)
Mirrors: Manual on combating antigypsyism through human rights education (2015)
Starting points for combating hate speech online (2015)
The 20th century: an interplay of views (2002)
The changing face of Europe - population flows in the 20th century (2002)
The shoah on screen - Representing crimes against humanity (2006)
The Use of Sources in Teaching and Learning History (2009) (Vol.1) (Vol. 2)
We can! Taking action against Hate Speech through Counter and Alternative Narratives (2017)
Anti-rumours handbook (2018)
European Pack for visiting Auschwitz-Birkenau Memorial and Museum: Guidelines for Teachers and Educators (2010)
Le témoignage du survivant en classe.
Living democracy – manuals for teachers (2009-2011)
Teaching about the Holocaust in the 21st century (2001)
Victims of Nazism - A mosaic of Fates. Pedagogical Factsheets for teachers (2015)
Related schools projects
French-Finnish School Lycée franco-finlandais d’Helsinki
Collège Charles Péguy de Palaiseau
Balda Public School
Khoni Public School N 3
LEPL Borjomi Municipality Akhaldaba Public School
LEPL Public School of village Khevasheni of Adigeni Municipality
Sachkhere Public School #3
Nelson Mandela Realschule plus Trier
Epal Korydallou
Makrygialos High School of Pieria
Bremore Educate Together Secondary School
Liceo Scientifico Statale “Nicolò Copernico”
Vilnius Kachialov Gymnasium
Gimnazija “Tanasije Pejatović”
Huseby ungdomsskole
Kuben upper secondary school
Adam Mickiewicz High School in Gdynia
Agrupamento de Escolas de Caneças
Agrupamento de Escolas do Cerco do Porto, Porto
Agrupamento de Escolas João da Rosa
Eça de Queirós School Cluster
Liceul Teoretic de Informatica Grigore Moisil Iasi
”Petefi Šandor” elementary school
United Kingdom
Batley Girls' High School
Making children’s and students’ voices heard
Addressing controversial issues, preventing violence and bullying, tackling discrimination, improving well-being at school.
The great debate: Does artificial intelligence have any place in American politics?
Surprisingly, several people interviewed said they’d support an ai candidate — but that doesn’t mitigate the danger of deepfakes.
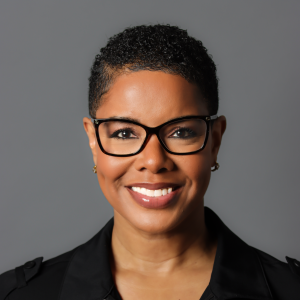
This is the third in a series of essays produced by Love Now Media and Technical.ly exploring the impact of artificial intelligence on various aspects of life in Philadelphia. Read the first essay on AI and personal safety and the second on AI and higher education . The series is produced with the support of the Philadelphia Journalism Collaborative , a coalition of more than 25 local newsrooms doing solutions reporting on things that affect daily life where the problem and symptoms are obvious, but what’s driving them isn’t. Follow at @PHLJournoCollab.
Back in May of this year, I read an article in Wired about India’s seeming embrace of using artificial intelligence in their political and election processes.
AI-generated deepfakes of politicians were actually sanctioned by national parties , even though officials and the technologists creating the images and audio admitted a large majority of constituents probably didn’t know they were interacting with fake posts.
The good: Gathering info and sparking interest
As it relates to US elections, there does seem to be a general consensus to allow artificial intelligence to do what AI does best, which is analyzing large amounts of data.
Numerous articles, including those from the Brookings Institute and the Ash Center for Democratic Governance and Innovation at Harvard University, extol the benefits of AI in performing functions like identifying anomalies in voter lists, efficiently scanning paper ballots and interpreting and enhancing poll results.
Asked by LoveNowMedia if he thought AI had any place in government, Isaiah from Cheltenham agreed, saying, “I think that maybe we can use AI as a tool when it comes to information gathering.”
Megan from Center City also believes there could be a place for artificial intelligence in elections. “I think there’s a place for AI…it could be used to bring up information that has been forgotten or help process some data.”
“[AI candidates] would be a cool addition, and everyone would be more interested.” Darian, Southwest Philadelphia
Darian from Southwest Philadelphia was a big proponent of using AI in elections. He believes one way to make use of artificial intelligence is in ensuring everyone’s voice is heard.
“AI could be used in surveys. You could have surveys and use the AI to get the answers to [reach] more people. I feel like people’s word isn’t being heard nowadays, and with AI it could be better.” He even went so far as to say that “maybe if AI is introduced [in elections], it would be a cool addition, and everyone would be more interested [in voting].”
The use of artificial intelligence in elections has also been touted as technology that could level the political playing field by lowering the cost of running campaigns.
By analyzing voter demographics data, campaigns can better target potential supporters to maximize advertising spending or monitor social media and other platforms to get real-time feedback on campaign performance. And even with all the potential for its misuse, AI can still be used to provide some level of election security by analyzing patterns to detect irregularities in voter registrations and electronic voting machines.
Treasure, an interviewee from Northeast Philadelphia, doesn’t think AI has much of a place in government, but still believes artificial intelligence could “maybe correct data on the counts about who voted.”
The bad: Spreading misinformation and suppressing votes
While even some skeptics can see a use case for how AI could streamline our electoral process, there are still lots of other things to consider.
There are no shortage of ways in which artificial intelligence could be used to deceive voters by spreading misinformation and disinformation, with examples already popping up in this year’s election cycle. Misleading AI-generated robocalls targeting then-candidate Joe Biden in the New Hampshire primary made national headlines, while the Trump campaign came under fire when Donald Trump reposted fake images implying he had Taylor Swift’s endorsement for president.
Voters are hearing rumors through various platforms and sharing the information, like Massara from Southwest Philadelphia who shared that she has “seen some pictures…of past presidents…meddling with kids and stuff like that. It wasn’t real, but it fed into the media like it was real.”
The ability of misinformation to spread quickly among voters of all demographics is a legitimate concern that this country doesn’t yet seem to have an answer for.
Using AI as a tool in voter suppression is one of the biggest threats this country will face in the upcoming presidential race.
In addition to the New Hampshire robocalls that attempted to trick voters into not voting in the primary election, groups like the Conservative Partnership Institute have been deploying AI systems to perform what have been called ‘faulty and error-prone’ analysis on demographic data in an attempt to file mass voter challenges, potentially removing thousands of people from registration rolls. Tactics like these typically disproportionately affect voters of color, voters from lower-income communities, and voters with disabilities as well.
Tips to identify misinformation online
- Consider the source — Is the information coming from a reputable source
- Check the date — Just because this may be the first time you’re seeing an article doesn’t necessarily mean it’s new or current.
- Check other sources — Always confirm information with multiple legitimate sources online.
- Check the location — Is the photo you’re looking at a picture that accurately reflects what you’re reading?
- Check your emotions — Misinformation is usually deliberately inflammatory and relies on elevated feelings of anger or outrage to spread. When it comes to anything you see on the internet, a good rule of thumb is “Verify, THEN share.”
There are also examples of how artificial intelligence negatively affects individual candidates and drives election outcomes in local races. Consider the case of Adrian Perkins, who in 2022 was running for reelection as mayor of Shreveport, Louisiana. A TV commercial funded by a rival political action committee used AI to superimpose Perkins’ face onto an actor’s body. He believes the effect of that negative ad had a significant impact on his losing the election. And earlier this year in Philadelphia, Sheriff Rochelle Bilal’s campaign was exposed for fake news stories posted to her campaign website that had been generated by ChatGPT.
As to the question of whether AI has a place in government, Abby from South Philadelphia was clear. “No,” Abby said. “It’s a people’s government.”
Here are some tips from MIT’s Media Lab to help you spot deepfake photos and videos, and you can take a quiz to test your AI-detection skills here .
And the unexpected: Support for AI candidates?
In a surprising twist, when Love Now Media asked interviewees if they would trust an AI-generated elected official, 2 of 7 respondents actually said yes.
“Seeing as though AI is computer technology,” Faheemah from Germantown told the Love Now Media interviewers. “I feel like they are smarter than most people so maybe so.”
Darian, who had suggested using surveys to gather voter sentiment, was also supportive. “I think pretty much, most of the time I would [trust an AI elected official],” they said. “It depends on how good it is and how it’s used.”
That reality may be coming sooner than you might have thought. In the UK, an AI chatbot recently ran for parliament.
This summer, AI Steve was listed on the ballot under the independent SmarterUK party. Entrepreneur Steve Endacott created the chatbot as a listening agent, with the plan for AI Steve to conduct conversations with voters to understand the policies they care about.
The human Steve said he planned only to only serve as proxy, someone who would physically cast votes, answering based on the uncovered during AI Steve’s interactions with constituents. The advantage of an AI politician, Endacott said, is “its ability to increase efficiency and transparency in politics by having conversations with voters 24/7, then analyzing and summarizing these conversations so the party can form policies voters actually care about.”
Spoiler: AI Steve didn’t win, only garnering 179 votes overall .
But do we really need artificial intelligence and all the technology that goes with it to effectively represent ourselves in government?
The final question in the Love Now Media interviews asked respondents what they thought elected officials could do to spread more love in the community. We’ll let the voices of the people be the final word:
“Just being authentic and trying to understand people, reach out to people and being more in touch than they are currently.” — Isaiah
“Come down from the offices. Connect more with people of color, people in general. I feel like in today’s society we lack trust within people in politics. They aren’t even listening to people’s voices anymore it’s just like ‘vote for me, vote for me’… but they aren’t actually taking the time to consider how people are trying to change the city and change where they’re living…they need to come out and engage with actual living people…” — Massara
“I think they [politicians] can show people what they’re passionate about. Not just tell people what they want to hear. But our officials should genuinely care about their citizens. And, you know, just make people feel comfortable and accepted for who they truly are.” — Megan
Before you go...
Please consider supporting Technical.ly to keep our independent journalism strong. Unlike most business-focused media outlets, we don’t have a paywall. Instead, we count on your personal and organizational support.
Join our growing Slack community
Join 5,000 tech professionals and entrepreneurs in our community Slack today!
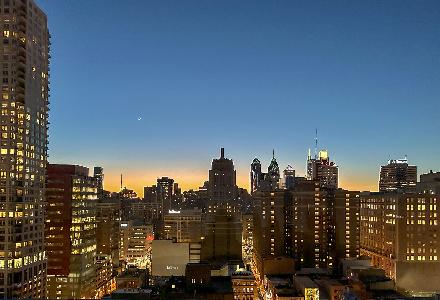
A look at Philadelphia's labor force: The data behind the demographics
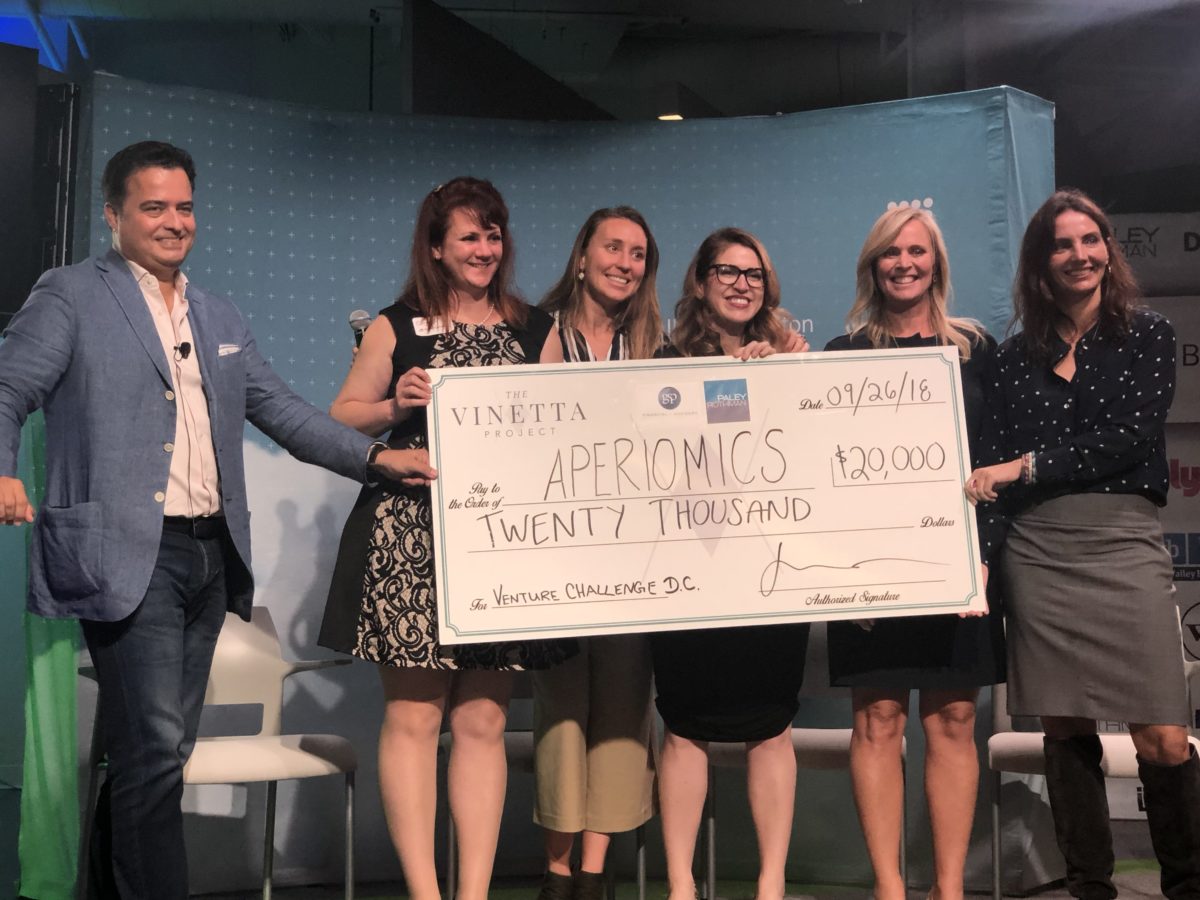
What female founders need to know about investment and fundraising trends
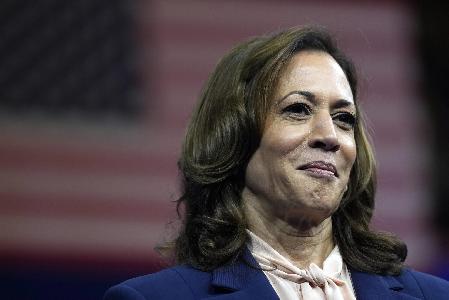
No, Kamala Harris isn’t the official candidate of the Philadelphia Eagles – and the city’s ads didn’t get hacked
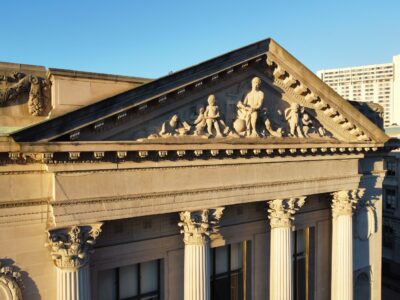
An overlooked part of entrepreneurial ecosystems? The lawyers
China-linked 'Spamouflage' network mimics Americans online to sway US political debate
As voters prepare to cast their ballots in the November election, U.S. adversaries like China are making their own plans
WASHINGTON -- When he first emerged on social media, the user known as Harlan claimed to be a New Yorker and an Army veteran who supported Donald Trump for president. Harlan said he was 29, and his profile picture showed a smiling, handsome young man.
A few months later, Harlan underwent a transformation. Now, he claimed to be 31 and from Florida.
New research into Chinese disinformation networks targeting American voters shows Harlan's claims were as fictitious as his profile picture, which analysts think was created using artificial intelligence.
As voters prepare to cast their ballots this fall, China has been making its own plans, cultivating networks of fake social media users designed to mimic Americans. Whoever or wherever he really is, Harlan is a small part of a larger effort by U.S. adversaries to use social media to influence and upend America’s political debate.
The account was traced back to Spamouflage, a Chinese disinformation group, by analysts at Graphika, a New York-based firm that tracks online networks. Known to online researchers for several years, Spamouflage earned its moniker through its habit of spreading large amounts of seemingly unrelated content alongside disinformation.
“One of the world's largest covert online influence operations — an operation run by Chinese state actors — has become more aggressive in its efforts to infiltrate and to sway U.S. political conversations ahead of the election,” Jack Stubbs, Graphika's chief intelligence officer, told The Associated Press.
Intelligence and national security officials have said that Russia , China and Iran have all mounted online influence operations targeting U.S. voters ahead of the November election. Russia remains the top threat, intelligence officials say, even as Iran has become more aggressive in recent months, covertly supporting U.S. protests against the war in Gaza and attempting to hack into the email systems of the two presidential candidates.
China, however, has taken a more cautious, nuanced approach . Beijing sees little advantage in supporting one presidential candidate over the other, intelligence analysts say. Instead, China's disinformation efforts focus on campaign issues particularly important to Beijing — such as American policy toward Taiwan — while seeking to undermine confidence in elections, voting and the U.S. in general .
Officials have said it's a longer-term effort that will continue well past Election Day as China and other authoritarian nations try to use the internet to erode support for democracy .
Chinese Embassy spokesperson Liu Pengyu rejected Graphika's findings as full of “prejudice and malicious speculation" and said that "China has no intention and will not interfere” in the election.
X, the platform formerly known as Twitter, suspended several of the accounts linked to the Spamouflage network after questions were raised about their authenticity. The company did not respond to questions about the reasons for the suspensions, or whether they were connected to Graphika's report.
TikTok also removed accounts linked to Spamouflage, including Harlan's.
“We will continue to remove deceptive accounts and harmful misinformation as we protect the integrity of our platform during the US elections,” a TikTok spokesperson wrote in a statement emailed on Tuesday.
Compared with armed conflict or economic sanctions, online influence operations can be a low-cost, low-risk means of flexing geopolitical power. Given the increasing reliance on digital communications, the use of online disinformation and fake information networks is only likely to increase, said Max Lesser, senior analyst for emerging threats at the Foundation for Defense of Democracies, a national security think tank in Washington.
“We’re going to see a widening of the playing field when it comes to influence operations, where it’s not just Russia, China and Iran but you also see smaller actors getting involved,” Lesser said.
That list could include not only nations but also criminal organizations, domestic extremist groups and terrorist organizations, Lesser said.
When analysts first noticed Spamouflage five years ago, the network tended to post generically pro-China, anti-American content. In recent years, the tone sharpened as Spamouflage expanded and began focusing on divisive political topics like gun control, crime, race relations and support for Israel during its war in Gaza. The network also began creating large numbers of fake accounts designed to mimic American users.
Spamouflage accounts don't post much original content, instead using platforms like X or TikTok to recycle and repost content from far-right and far-left users. Some of the accounts seemed designed to appeal to Republicans, while others cater to Democrats.
While Harlan's accounts succeeded in getting traction — one video mocking President Joe Biden was seen 1.5 million times — many of the accounts created by the Spamouflage campaign did not. It's a reminder that online influence operations are often a numbers game: the more accounts, the more content, the better the chance that one specific post goes viral.
Many of the accounts newly linked to Spamouflage took pains to pose as Americans, sometimes in obvious ways. “I am an American,” one of the accounts proclaimed. Some of the accounts gave themselves away by using stilted English or strange word choices. Some were clumsier than others: “Broken English, brilliant brain, I love Trump,” read the biographical section of one account.
Harlan's profile picture, which Graphika researchers believe was created using AI , was identical to one used in an earlier account linked to Spamouflage. Messages sent to the person operating Harlan’s accounts were not returned.
Popular Reads

Moore says he made an 'honest mistake' failing to correct application claiming Bronze Star
- Aug 29, 7:07 PM

FACT FOCUS: Posts falsely claim video shows Harris promising to censor X and owner Elon Musk
- Sep 3, 4:19 PM
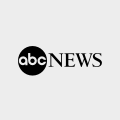
Former aide to New York's governor has been charged with being an agent of the Chinese government
- Sep 3, 11:43 AM

Former aide to 2 New York governors is charged with being an agent of the Chinese government
- Sep 3, 10:51 AM
Mongolia holds welcome ceremony for Russian President Vladimir Putin despite international warrant for his arrest
- Sep 3, 1:27 AM
ABC News Live
24/7 coverage of breaking news and live events
Peer Reviewed
Who knowingly shares false political information online?
Article metrics.
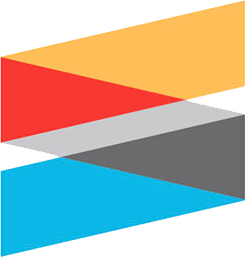
CrossRef Citations
Altmetric Score
PDF Downloads
Some people share misinformation accidentally, but others do so knowingly. To fully understand the spread of misinformation online, it is important to analyze those who purposely share it. Using a 2022 U.S. survey, we found that 14 percent of respondents reported knowingly sharing misinformation, and that these respondents were more likely to also report support for political violence, a desire to run for office, and warm feelings toward extremists. These respondents were also more likely to have elevated levels of a psychological need for chaos, dark tetrad traits, and paranoia. Our findings illuminate one vector through which misinformation is spread.
Department of Political Science, University of Miami, USA
Department of Psychological and Brain Sciences, Indiana University, USA
Department of English, University of Miami, USA
Department of Electrical and Computer Engineering, University of Miami, USA
Department of Interactive Media, University of Miami, USA
Department of Computer Science, University of Miami, USA
Department of Biology, University of Miami, USA
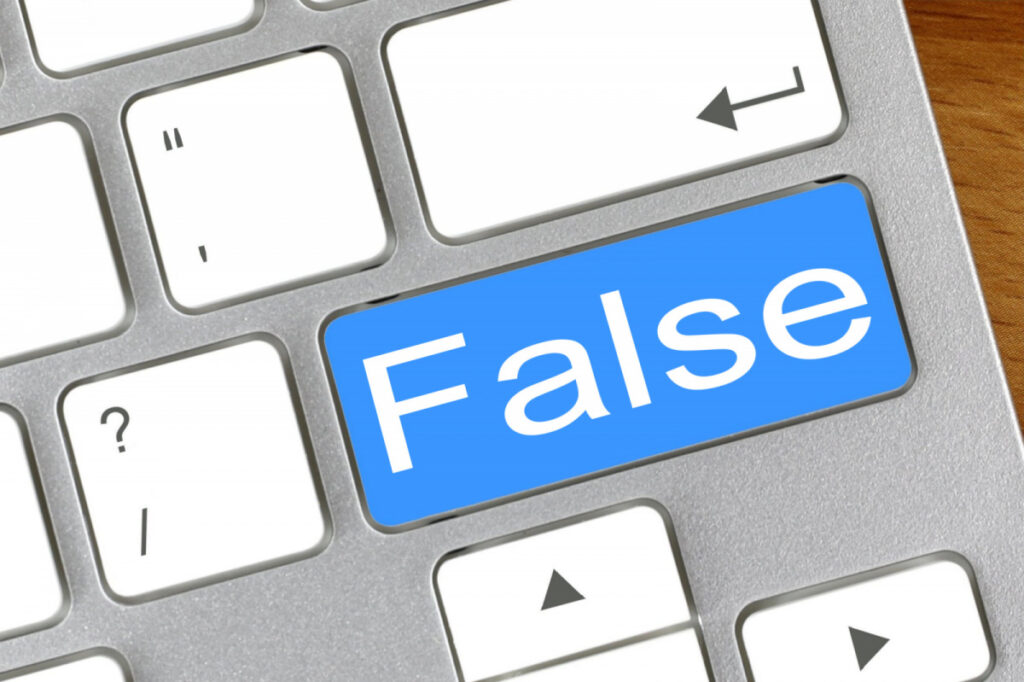
Research Questions
- What percentage of Americans admit to knowingly sharing political information on social media they believe may be false?
- How politically engaged, and in what ways, are people who report knowingly sharing false political information online?
- Are people who report knowingly sharing false political information online more likely to report extremist views and support for extremist groups?
- What are the psychological, political, and social characteristics of those who report knowingly sharing false political information online?
Essay Summary
- While most people are exposed to small amounts of misinformation online (in comparison to their overall news diet), previous studies have shown that only a small number of people are responsible for sharing most of it. Gaining a better understanding of the motivations of social media users who share information they believe to be false could lead to interventions aimed at limiting the spread of online misinformation.
- Using a national survey from the United States ( n = 2,001; May–June 2022), we asked respondents if they share political information on social media that they believe is false; 14% indicated that they do.
- Respondents who reported purposefully sharing false political information online were more likely to harbor (i) a desire to run for political office, (ii) support for political violence, and (iii) positive feelings toward QAnon, Proud Boys, White Nationalists, and Vladimir Putin. Furthermore, these respondents displayed elevated levels of anti-social characteristics, including a psychological need for chaos, “dark” personality traits (narcissism, psychopathy, Machiavellianism, and sadism), paranoia, dogmatism, and argumentativeness.
- People who reported sharing political information they believe is false on social media were more likely to use social media platforms known for promoting extremist views and conspiracy theories (e.g., 8Kun, Telegram, Truth Social).
Implications
A growing body of research shows that online misinformation is both easily accessible (Allcott & Gentzkow, 2017; Del Vicario et al., 2016) and can spread quickly through online social networks (Vosoughi et al., 2018). Though misinformation —information that is false or misleading according to the best currently established knowledge (Ecker et al., 2021; Vraga & Bode, 2020)—is often spread unintentionally, disinformation , a subcategory of misinformation, is spread with the deliberate intent to deceive (Starbird, 2019). Critically, the pervasiveness of online mis- and disinformation has made attempts by online news and social media companies to prevent, curtail, or remove it from various platforms difficult (Courchesne et al., 2021; Ha et al., 2022; Sanderson et al., 2021; Vincent et al., 2022). While the causal impact of online misinformation is often difficult to determine (Enders et al., 2022; Uscinski et al., 2022), numerous studies have shown that exposure is (at least) correlated with false beliefs (Bryanov & Vziatysheva, 2021), beliefs in conspiracy theories (Xiao et al., 2021), and nonnormative behaviors, including vaccine refusal (Romer & Jamieson, 2021).
Numerous studies have investigated the spread of political mis- and disinformation as a “top-down” phenomenon (Garrett, 2017; Lasser et al., 2022; Mosleh & Rand, 2022) emanating from domestic political actors (Berlinski et al., 2021), untrustworthy websites (Guess et al., 2020), and hostile foreign governments (Bail et al., 2019), and flowing through social media and other networks (Johnson et al., 2022). Indeed, studies have found that most online political content, as well as most online misinformation, is produced by a relatively small number of accounts (Grinberg et al., 2019; Hughes, 2019). Other research has focused on how the public interacts with and evaluates misinformation to identify the individual differences related not only to falling for misinformation but also to unintentionally spreading it (Littrell et al., 2021a; Pennycook & Rand, 2021).
However, rather than being unknowingly duped into sharing misinformation, many people (who are not political elites, paid activists, or foreign political actors) knowingly share false information in a deliberate attempt to deceive or mislead others, often in the service of a specific goal (Buchanan & Benson, 2019; Littrell et al., 2021b; MacKenzie & Bhatt, 2020; Metzger et al., 2021). For instance, people who create and spread fake news content and highly partisan disinformation online are often motivated by the desire that such posts will “go viral,” attracting attention that will hopefully provide a reliable stream of advertising revenue (Guess & Lyons, 2020; Pennycook & Rand, 2020; Tucker et al., 2018). Others may do so to discredit political or ideological outgroups, advance their own ideological agenda or that of their partisan ingroup, or simply because they enjoy instigating discord and chaos online (Garrett et al., 2019; Marwick & Lewis, 2017; Petersen et al., 2023).
Though the art of deception is likely as old as communication itself, in the past, a person’s ability to meaningfully communicate with (and perhaps deceive) large groups was arguably limited. In contrast, social media now gives every person the power to rapidly broadcast (false) information to potentially global, mass audiences (DePaulo et al., 1996; Guess & Lyons, 2020). This implicates social media as a critical vector in the spread of misinformation. Whatever the motivations for sharing false information online, a better understanding of the human sources who create it by identifying the psychological, political, and ideological factors common to those who do so intentionally can provide crucial insights to aid in developing interventions that decrease its spread.
In a national survey of the United States, we asked participants to rate their agreement (“strongly agree” to “strongly disagree”) with the statement, “I share information on social media about politics even though I believe it may be false.” In total, 14% of respondents agreed or strongly agreed with this statement; these findings coincide with those of other studies on similar topics (Buchanan & Kempley, 2021; Halevy et al., 2014; Serota & Levine, 2015). Normatively, it is encouraging that only a small minority of our respondents indicated that they share false information about politics on social media. However, the fact that 14% of the U.S. adult population claims to purposely spread political misinformation online is nonetheless troubling. Rather than being exclusively a top-down phenomenon, the purposeful sharing of false information by members of the public appears to be an important vector of misinformation that deserves more attention from researchers and practitioners.
Of further concern, our findings show that people who claimed to knowingly share information on social media about politics were more politically active in meaningful ways. First and perhaps foremost, such respondents were not only more likely to state a desire to run for political office but were also more likely to feel qualified for office, compared to people that do not claim to knowingly share false information. This finding is troubling from a normative perspective since such people might not be honest with constituents if elected (consider, for example, Representative George Santos of New York), and this could further erode our information environment (e.g., Celse & Chang, 2019). However, this finding may also offer crucial insights to better understand the tendency and motivations of at least some politicians to share misinformation or outright lie to the public (Arendt, 1972; Sunstein, 2021). Beyond aspirations for political office, spreading political misinformation online is positively associated with support for political violence, civil disobedience, and protests. Moreover, though spreading misinformation is also associated with participating in political campaigns, it is only weakly related to attending political meetings, contacting elected representatives, or staying informed about politics. Taken together, these findings paint a somewhat nuanced picture: People who were more likely to self-report having intentionally shared false political information on social media were more likely to be politically active and efficacious in certain aggressive ways, while simultaneously being less likely to participate in more benign or arguably positive ways.
Our findings also revealed that respondents who reported sharing false political information on social media were more likely to express support for extremist groups such as QAnon, Proud Boys, and White Nationalists. These observations coincide with previous studies linking extremist groups to the spread of misinformation, disinformation, and conspiracy theories (Moran et al., 2021; Nguyen & Gokhale, 2022; Stern, 2019). One possible explanation for this association is that supporters of extremist groups recognize their outsider status in comparison to mainstream political groups, leveraging false information to manage public impressions, attract new members, and further their group’s cause. Alternatively, it could be that the beliefs promoted by extremist groups are so disconnected from our shared political reality that these groups may need to rely on falsehoods to manipulate their own followers and prevent attrition of group membership. While the exact nature of these associations remains unclear, future research should further interrogate the connection between sharing false information and support for extremism and extremist groups. In line with previous studies (Lawson & Kakkar, 2021), our findings show that people who reported sharing false political information on social media were more likely to report higher levels of antisocial psychological characteristics. Specifically, they reported higher levels of a “need for chaos,” “dark tetrad” personality traits (a combined measure of narcissism, Machiavellianism, psychopathy, and sadism), paranoia, dogmatism, and argumentativeness when compared to respondents who did not report knowingly sharing false information on social media. Much like the Joker in the movie The Dark Knight , people who intentionally spread false information online may, at least on some level, simply want to “watch the world burn” (Arceneaux et al., 2021). Indeed, previous studies suggest that much of the toxicity of social media is not due to a “mismatch” between human psychology and online platforms (i.e., that online platforms bring out the worst in otherwise nice people); instead, such toxicity results from a relatively smaller fraction of people with status-seeking antisocial tendencies, who act overtly antisocial online, and are drawn to interactions in which they express elevated levels of aggressiveness toward others with toxic language (Bor & Petersen, 2022; Kim et al., 2021). Such observations are echoed in our own results, which showed that people who knowingly share false information online were also more likely to indicate that posting on social media gives them a greater feeling of power and control and allows them to express themselves more freely.
While research on the associations between religiosity and lying/dishonesty has shown mixed results (e.g., Desmond & Kraus, 2012; Grant et al., 2019), we found that religiosity positively predicts knowingly sharing false information online. Additionally, despite numerous studies of online activity suggesting that people on the political right are more likely to share misinformation (e.g., DeVerna et al., 2022; Garrett & Bond, 2021), our findings show no significant association between self-reported sharing of false information online and political identity or the strength of one’s partisan or ideological views.
Our findings offer a broad psychological and ideological blueprint of individuals who reported intentionally spreading false information online, implicating specific personality and attitudinal characteristics as potential motivators of such behavior. Overall, these individuals are more antagonistic and argumentative, have a higher need for chaos, and tend to be more dogmatic and religious. Additionally, they are more politically engaged and active, often in counterproductive and destructive ways, and show higher support for extremist groups. They are also more likely to get their news from fringe social media sources and feel a heightened sense of power and self-expression from their online interactions. Taken together, these findings suggest that interventions which focus on eliminating the perceived social incentives gained from intentionally spreading misinformation online (e.g., heightened feelings of satisfaction, power, and enjoyment associated with discrediting ideological outgroups, instigating chaos, and “trolling”) may be effective at attenuating this type of online behavior.
Though some research has shown promising results using general interventions such as “accuracy nudges” (Pennycook et al., 2021) and educational video games to inoculate people against misinformation (Roozenbeek et al., 2022), more direct measures may also need to be implemented by social media companies. For example, companies might consider restructuring the online environment to remove overt social incentives that may inadvertently reward pernicious behavior (e.g., reconsidering how “likes” and sharing are implemented) and instead create online ecosystems that reward more positive social media interactions. For practitioners, at the very least, our finding that some people claim to share online misinformation for reasons other than simply being duped, suggests that future interventions aimed at limiting the spread of misinformation should attempt to address users who both unknowingly and knowingly share misinformation, as these two groups of users may require different interventions. More specifically, if one does not care about accuracy, then accuracy nudges will do little to prevent them from sharing misinformation. Taken together, our findings further implicate personality and attitudinal characteristics as potentially significant motivators for the spread of misinformation. As such, we join others who have called for greater integration of personality research into the study of online misinformation and the ways in which it spreads (e.g., Lawson & Kakkar, 2021; van der Linden et al., 2021).
Finding 1: Most people do not report intentionally spreading false political information online.
We asked participants to rate their agreement (“strongly agree” to “strongly disagree”) with the statement, “I share information on social media about politics even though I believe it may be false.” At best, agreement with this statement reflects a carefree disregard for the truth, a key characteristic of certain types of “bullshitting” (Frankfurt, 2009; Littrell et al., 2021a). However, at worst, strong agreement with this statement is admitting to intentional deception (i.e., lying). Though most participants disagreed with this statement, a non-trivial percentage of respondents (14%) indicated that they do intentionally share false political information on social media (Figure 1). These findings are consistent with empirical studies of similar constructs, such as lying (Buchanan & Kempley, 2021; Halevy et al., 2014; Serota & Levine, 2015) and “bullshitting” (Littrell et al., 2021a), which have shown that a small but consistent percentage of people admit to intentionally misleading others.
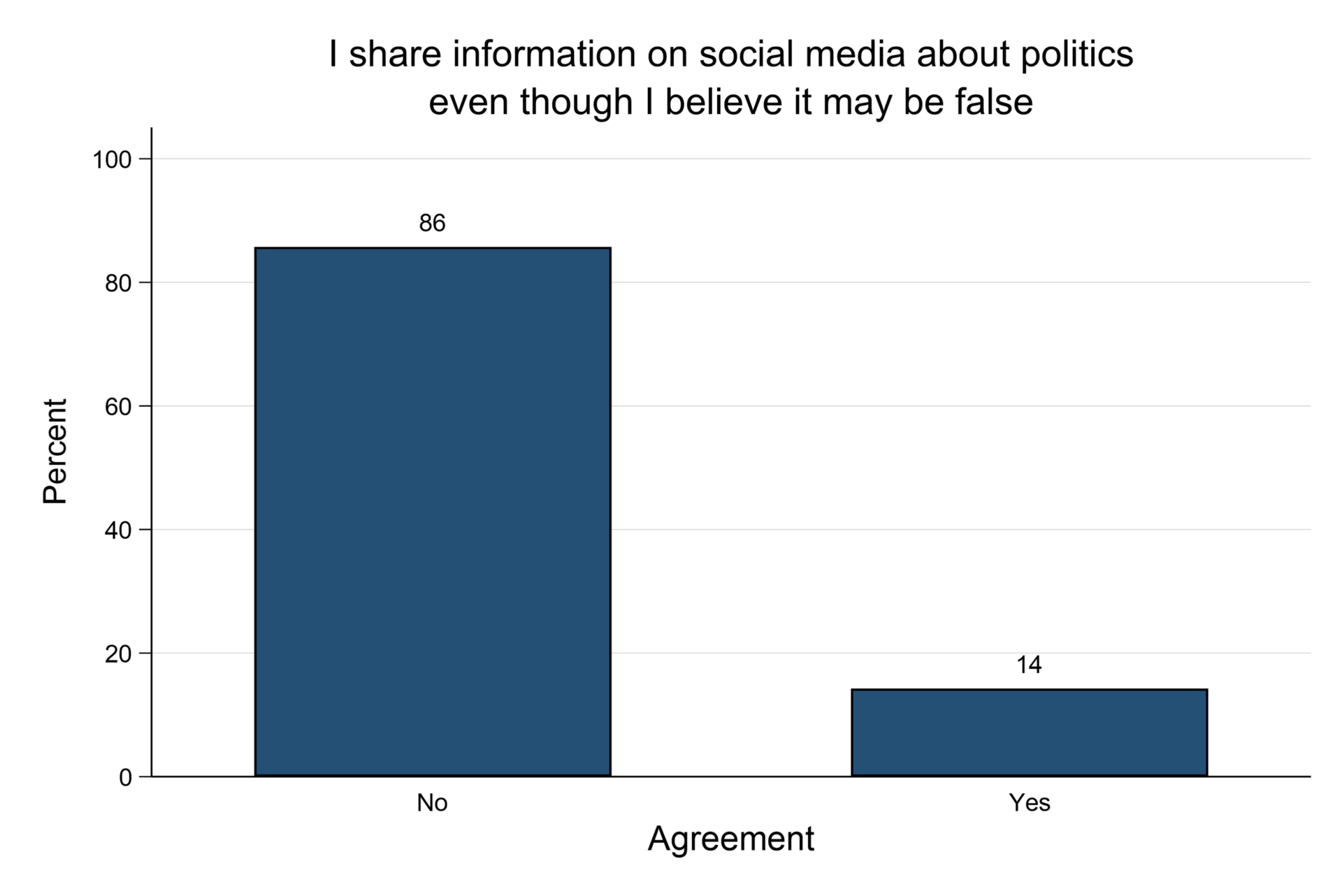
Notably, it is possible that the prevalence of knowingly sharing political misinformation online is somewhat underreported in our data, given that some of the spreaders of it in our sample could have denied it when responding to that item (which, ironically, would be another instance of them spreading misinformation). Indeed, some research has found that survey respondents may sometimes hide their true beliefs or express agreement or support for a specific idea they actually oppose either as a joke or to signal their group identity (i.e., Lopez & Hillygus, 2018; Schaffner & Luks, 2018; Smallpage et al., 2022). Further, self-reported measures of behavior are sometimes only weakly correlated with actual behavior (Dang et al., 2020). However, there are good reasons to have confidence in this self-reported measure. First, self-report surveys have high reliability for measuring complex psychological constructs (e.g., beliefs, attitudes, preferences) and are sometimes better at predicting real-world outcomes than behavioral measures of those same constructs (Kaiser & Oswald, 2022). Second, the percentage of respondents in our sample who admitted to spreading false political information online aligns with findings from previous research. For example, Serota and Levine (2015) found that 14.1% of their sample admitted to telling at least one “big lie” per day, while Littrell and colleagues (2021a) found 17.3% of their sample admitted to engaging in “persuasive bullshitting” on a regular basis. These numbers are similar to the 14% of our sample who self-reported knowingly sharing false information. Third, previous studies have found that self-report measures of lying and bullshitting positively correlate with behavioral measures of those same constructs (Halevy et al., 2014; Littrell et al., 2021a; Zettler et al., 2015). Given that our dependent variable captures a conceptually similar construct to those other measures, we are confident that our self-report data reflects real-world behavior, at least to a reasonable degree.
Crucially, we also found that the correlational patterns we reported across multiple variables are highly consistent and make sense with respect to what prior theory would predict of people who share information they believe to be false. Indeed, “need for chaos” has recently been shown to be a strong motivator of sharing hostile, misleading political rumors online (Petersen et al., 2023) and, as Figure 4 illustrates, “need for chaos” was also the strongest positive predictor in our study of sharing false political information online (β = .18, p < .001). Moreover, as an added test of the reliability and validity of our dependent variable, we examined correlations between responses to our measure of sharing false political information online and single-scale items that reflect similar behavioral tendencies. For instance, our dependent variable is significantly and positively correlated (r = .53, p < .001) with the statement, “Just for kicks, I’ve said mean things to people on social media,” from the Sadism subscale of our “Dark Tetrad” measure. Additionally, our dependent variable also correlated well with two conceptually similar items from the Machiavellianism subscale, “I tend to manipulate others to get my way” (r = .43, p < .001) and “I have used deceit or lied to get my way” (r = .35, p < .001). Although the sizes of these effects do not suggest that these constructs are isomorphic, it is helpful to note that our dependent variable item specifically measures sharing false information about politics on social media, which arises from a diversity of motivations, and not lying about anything and everything across all domains.
Finding 2: Reporting sharing false political information is associated with politically motivated behaviors and attitudes.
Self-reported sharing of false political information on social media was significantly and positively correlated with having contacted an elected official within the previous year ( r = .24, p < .001) and with the belief that, “People like me can influence government” ( r = .23, p < .001). Additionally, people who self-report sharing false political information online also reported more frequent attendance at political meetings ( r = .36, p < .001) and volunteering during elections ( r = .41, p < .001) compared to participants who do not report sharing false political information online.
Although these findings may give the impression that respondents reporting spreading online political misinformation are somewhat civically virtuous, these respondents also report engaging in aggressive and disruptive political behaviors. Specifically, reporting spreading false information was significantly associated with greater reported involvement in political protests ( r = .40, p < .001), acts of civil disobedience ( r = .44, p < .001), and political violence ( r = .46, p < .001). Spreading false information online was also significantly and positively related to believing that one is qualified for public office ( r = .40, p < .001) and the desire to possibly run for office one day ( r = .52, p < .001), but was only weakly related to staying informed about government and current affairs (“follows politics”; r = .05, p = .024).
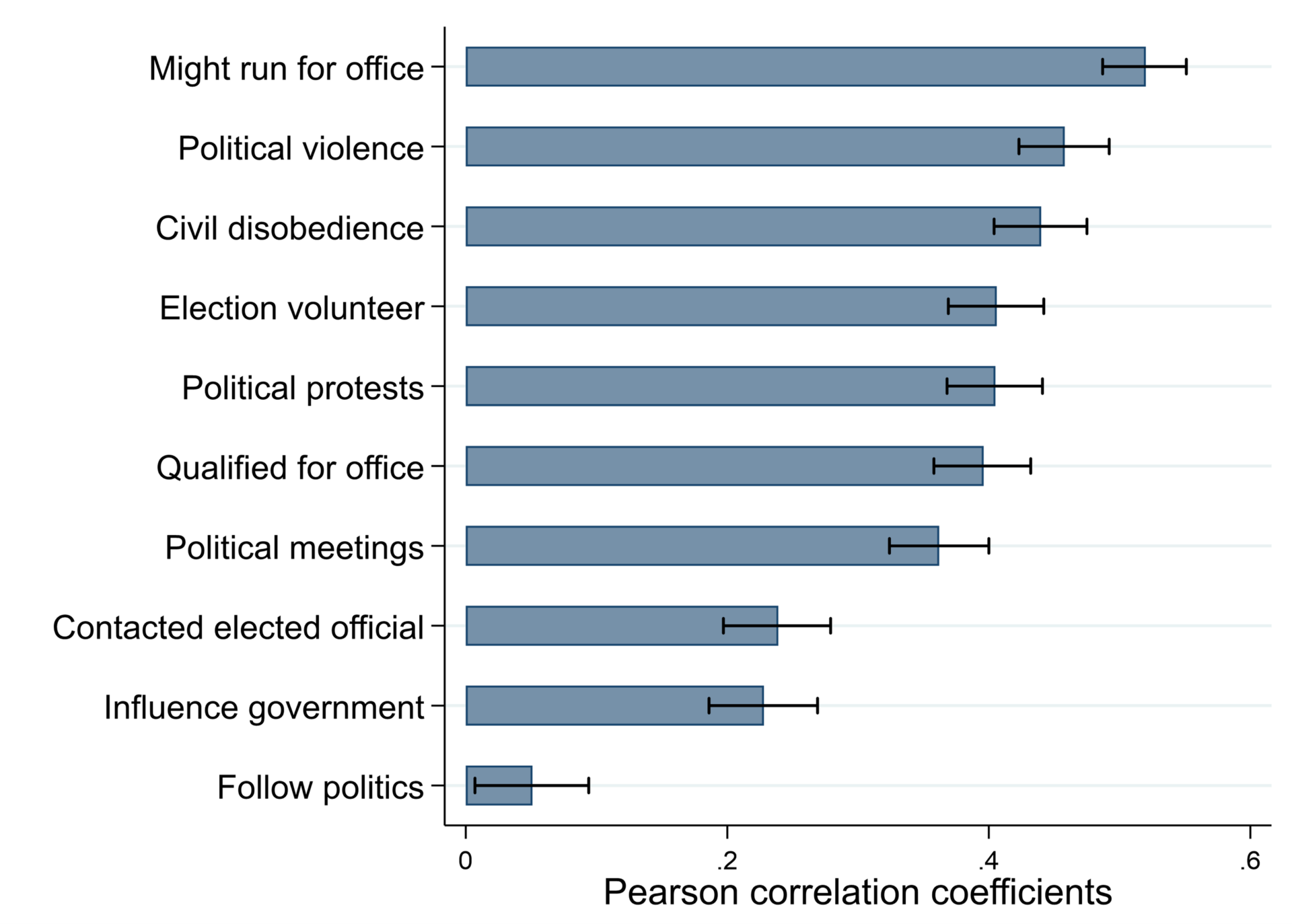
Finding 3: Reporting sharing false political information online is associated with support for extremist groups.
Using a sliding scale from 0 to 100, participants rated their feelings about various public figures and groups (Figure 3). While the self-reported tendency to knowingly share false political information online was weakly, but positively, associated with support for more mainstream public figures such as Donald Trump ( r = .14, p < .001), Joe Biden ( r = .13, p < .001), and Bernie Sanders ( r = .09, p < .001), it was more strongly associated with support for Vladimir Putin ( r = .40, p < .001). Likewise, self-reported sharing of false political information online was weakly but positively associated with support for the Democrat Party ( r = .13, p < .001) and the Republican Party ( r = .13, p < .001), but was most strongly associated with support for extremist groups such as the QAnon movement ( r = .45, p < .001), Proud Boys ( r = .42, p < .001), and White Nationalists ( r = .42, p < .001).
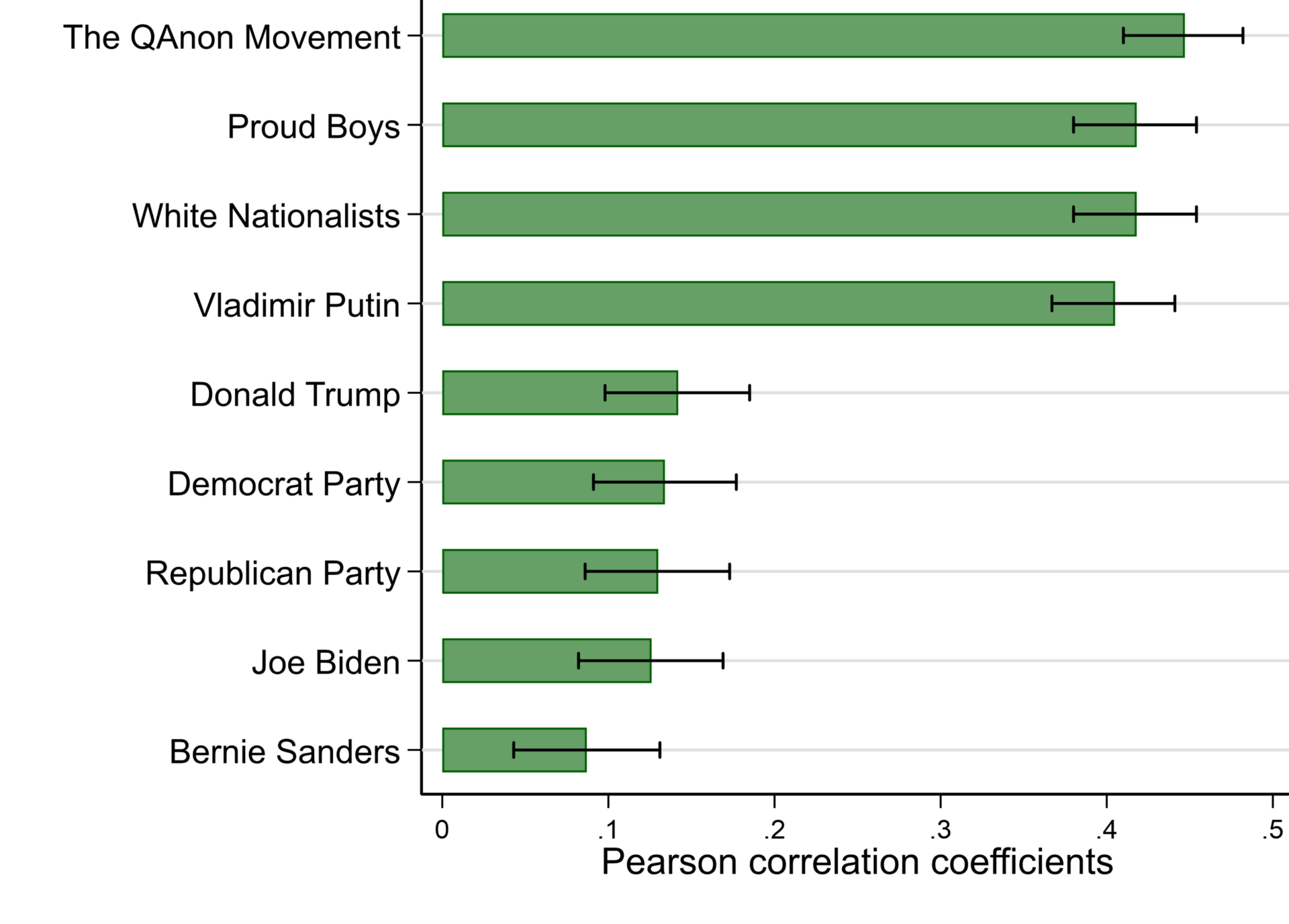
Finding 4: Reporting sharing false political information online is associated with dark psychological traits.
We constructed a multiple linear regression model to better understand the extent to which various psychological, political, and demographic characteristics might underlie the proclivity to knowingly share political misinformation on social media. Holding all other variables constant, a greater “need for chaos” ( β = .18, p < .001) as well as higher levels of antagonistic, “dark tetrad” personality traits (a single factor measure of narcissism, Machiavellianism, psychopathy, and sadism; β = .18, p < .001) were the strongest positive predictors of self-reported sharing of political misinformation online. Self-reported sharing of false information was also predicted by higher levels of paranoia ( β = .11, p < .001), dogmatism ( β = .09, p = .001), and argumentativeness ( β = .06, p = .035). People who feel that posting on social media gives them greater feelings of power and control ( β = .14, p < .001) and allows them to more freely express opinions and attitudes they are reluctant to express in person ( β = .06, p = .038) are also more likely to report knowingly sharing false political information online. Importantly, though sharing political misinformation online is positively predicted by religiosity ( β = .07, p = .003), it is not significantly associated with political identity or the strength of one’s partisan or ideological views.
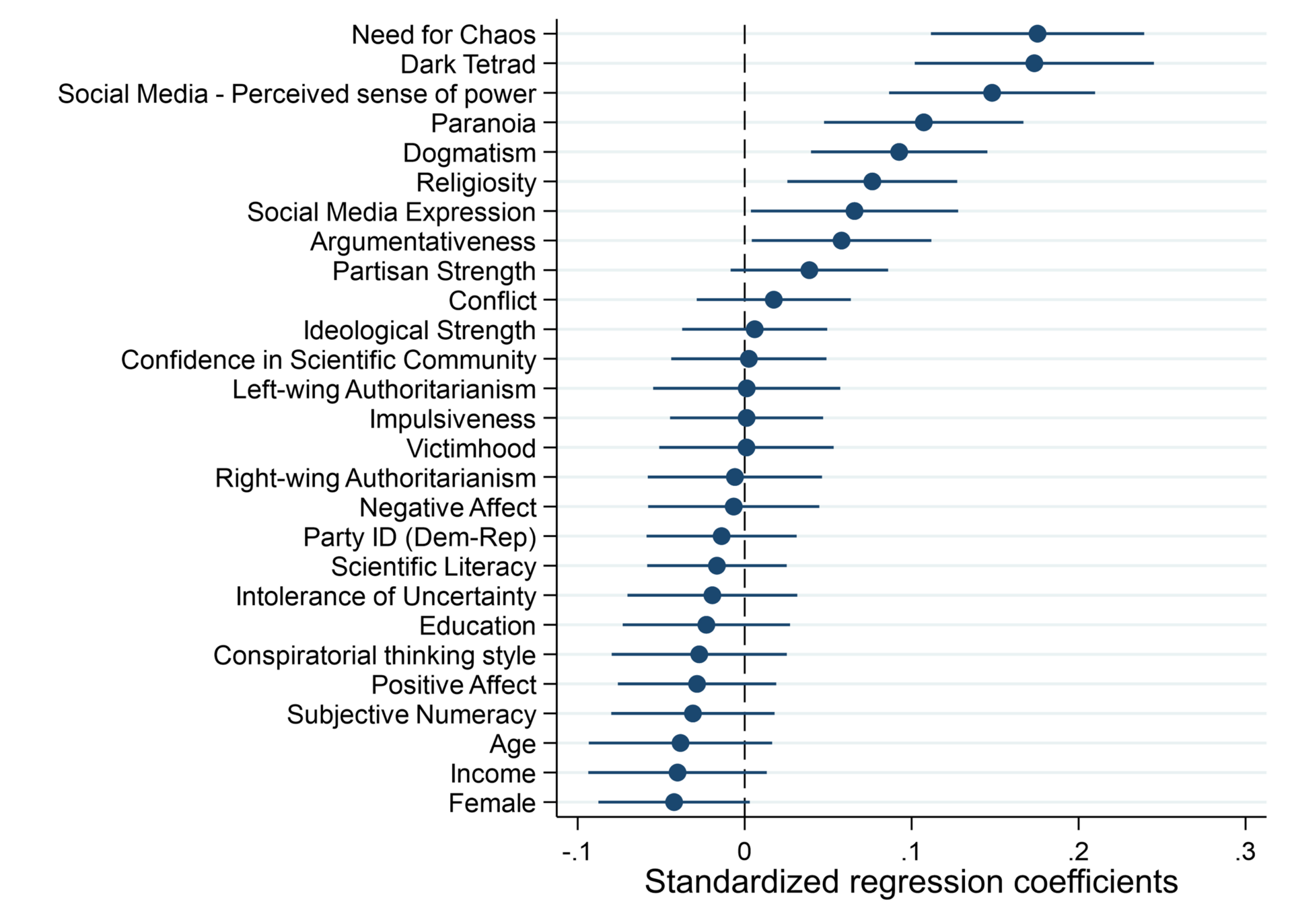
Finding 5: People who report intentionally sharing false political information online are more likely to get their news from social media sites, particularly from outlets that are known for perpetuating fringe views.
On a scale from “everyday” to “never,” participants reported how often they get “information about current events, public issues, or politics” from various media sources, including offline legacy media sources (e.g., network television, cable news, local television, print newspapers, radio) and online media sources (e.g., online newspapers, blogs, YouTube, and various social media platforms). A principal components analysis of the online media sources revealed three distinct categories: 1) online mainstream news media , made up of TV news websites, online news magazines, online newspapers; 2) mainstream social media sites , such as YouTube, Facebook, Twitter, Instagram; and 3) alternative social media sites , which comprised blogs, Reddit, Truth Social, Telegram, and 8Kun (factor loadings are listed in Table A8 of the appendix). After reverse-coding the scale for analysis, we examined bivariate correlations to determine whether the proclivity to share false political information online is meaningfully associated with the types of media sources participants get their information from.
As shown in Figure 5A, reporting sharing false political information online is strongly associated with more frequent use of alternative ( r = .46, p < .001) and mainstream ( r = .42, p < .001) social media sites and weakly-to-moderately correlated with getting information from online ( r = .20, p < .001) or offline/legacy ( r = .17, p < .001) mainstream news sources. On an individual level (Figure 5B), reporting sharing false political information online was most strongly associated with getting information on current events, public issues, and politics from Truth Social ( r = .41, p < .001), Telegram ( r = .41, p < .001), and 8Kun ( r = .41, p < .001), of which the latter two are popular among fringe groups known for promoting extremist views and conspiracy theories (Urman & Katz, 2022; Zeng & Schäfer, 2021).
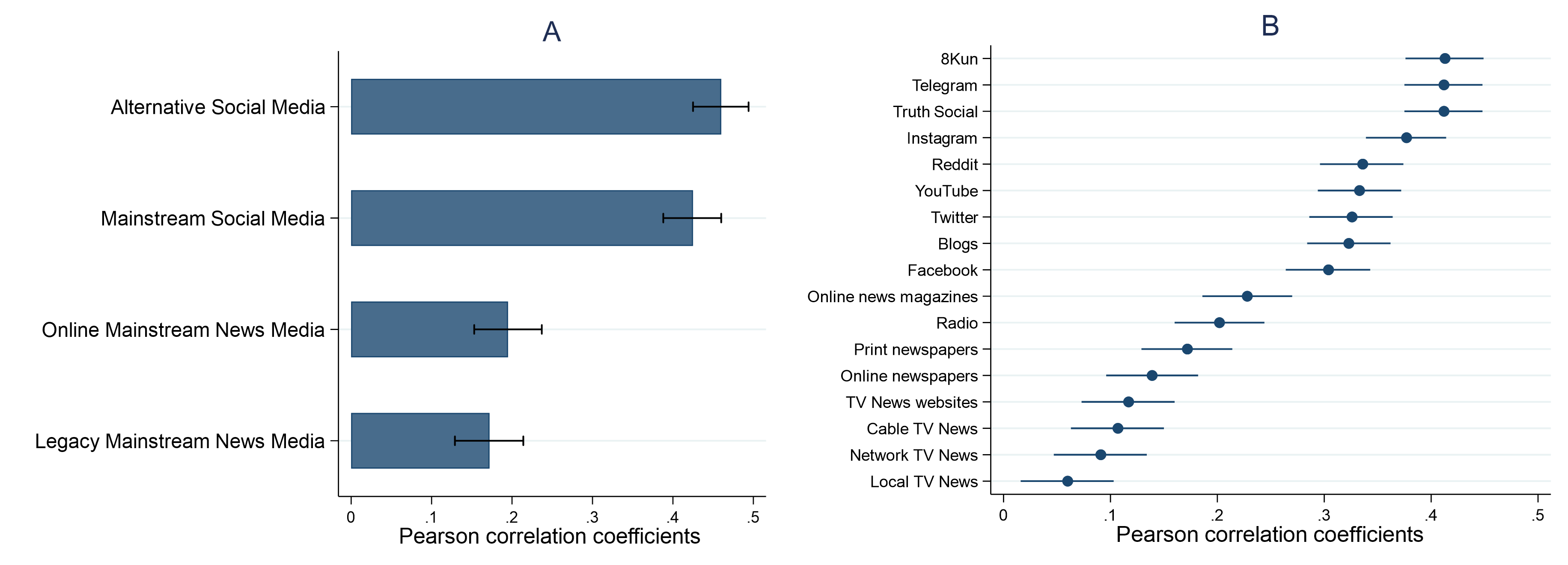
We surveyed 2,001 American adults (900 male, 1,101 female, M age = 48.54, SD age = 18.51, Bachelor’s degree or higher = 43.58%) from May 26 through June 30, 2022, using Qualtrics ( qualtrics.com ). For this survey, Qualtrics partnered with Cint and Dynata to recruit a demographically representative sample (self-reported sex, age, race, education, and income) based on U.S. Census records. Cint and Dynata maintain panels of subjects that are only used for research, and both comply fully with European Society for Opinion and Marketing Research (ESOMAR) standards for protecting research participants’ privacy and information security. Additionally, and in keeping with Qualtrics data quality standards, responses were excluded from the data set from participants who failed six attention check items or completed the survey in less than one-half of the estimated median completion time of 18.6 minutes (calculated from a soft-launch test of the questionnaire, n = 50). In exchange for their participation, respondents received incentives redeemable from the sample provider. These data were collected as part of a larger survey.
Our dependent variable asked respondents to rate their agreement with the following statement using a 5-point Likert-type scale (Figure 1):
“I share information on social media about politics even though I believe it may be false.”
In addition to this question, participants were also asked to rate the strength of certain political beliefs (i.e., whether they feel qualified to run for office, whether they think they might run for office one day, and whether they believe someone like them can influence government) and the frequency that they engaged in specific political behaviors in the previous 12 months (contacting elected officials, volunteering during an election, staying informed about government, and participating in political meetings, protests, civil disobedience, or violence). We calculated bivariate Pearson’s correlation coefficients for each of these variables with the item measuring whether one shares false political information on social media, which we have displayed in Table A4 of the Appendix.
Participants also used “feelings thermometers” to rate their attitudes toward a number of public political figures and groups. Each public figure or group was rated on a scale from 0 to 100, with scores of 0 to 50 reflecting negative feelings and scores from above 50 to 100 reflecting positive feelings. Although all correlations between the sharing false political information variable the public figures and groups were statistically significant, the strongest associations were with more adversarial figures (e.g., Putin) and groups (e.g., The QAnon Movement, Proud Boys, White Nationalists). We have plotted these associations in Figure 3.
To provide a more complete description of individuals who are more likely to report intentionally sharing false political information online, we examine the predictive utility of a number of psychological attributes, political attitudes, and demographics variables in an ordinary least squares (OLS) multiple linear regression model (Figure 4). We provide precise estimates in tabular form for all predictors as well as the overall model in the Appendix.
Finally, participants were asked to rate 17 media sources according to the frequency (“everyday” to “never”) with which they use each for staying informed on current events, public issues, and politics. A principal components analysis revealed that the media sources represented four categories: legacy mainstream news media (network TV, cable TV, local TV, radio, and print newspapers), online mainstream news media (TV news websites, online news magazines, online newspapers), mainstream social media sites (YouTube, Facebook, Twitter, Instagram), and alternative social media sites (blogs, Reddit, Truth Social, Telegram, 8Kun). We calculated bivariate correlations between reporting sharing false political information online with the four categories of media sources as well as the 17 individual sources. We have plotted these associations in Figure 5 and provide a full list of intercorrelations for all variables as well as factor loadings from the PCA in the Appendix.
- Conspiracy Theories
- / Platforms
- / Psychology
- / Social Media
Cite this Essay
Littrell, S., Klofstad, C., Diekman, A., Funchion, J., Murthi, M., Premaratne, K., Seelig, M., Verdear, D., Wuchty, S., & Uscinski, J. E. (2023). Who knowingly shares false political information online?. Harvard Kennedy School (HKS) Misinformation Review . https://doi.org/10.37016/mr-2020-121
Bibliography
Allcott, H., & Gentzkow, M. (2017). Social media and fake news in the 2016 election. Journal of Economic Perspectives , 31 (2), 211–236. https://doi.org/10.1257/jep.31.2.211
Arceneaux, K., Gravelle, T. B., Osmundsen, M., Petersen, M. B., Reifler, J., & Scotto, T. J. (2021). Some people just want to watch the world burn: The prevalence, psychology and politics of the ‘need for chaos’. Philosophical Transactions of the Royal Society B: Biological Sciences , 376 (1822), 20200147. https://doi.org/10.1098/rstb.2020.0147
Arendt, H. (1972). Crises of the Republic: Lying in politics, civil disobedience on violence, thoughts on politics, and revolution . Houghton Mifflin Harcourt.
Armaly, M. T., & Enders, A. M. (2022). ‘Why me?’ The role of perceived victimhood in American politics. Political Behavior , 44 (4), 1583–1609. https://doi.org/10.1007/s11109-020-09662-x
Bail, C., Guay, B., Maloney, E., Combs, A., Hillygus, D. S., Merhout, F., Freelon, D., & Volfovsky, A. (2019). Assessing the Russian Internet Research Agency’s impact on the political attitudes and behaviors of American Twitter users in late 2017. Proceedings of the National Academy of Sciences , 117 (1), 243–250. https://doi.org/10.1073/pnas.1906420116
Berlinski, N., Doyle, M., Guess, A. M., Levy, G., Lyons, B., Montgomery, J. M., Nyhan, B., & Reifler, J. (2021). The effects of unsubstantiated claims of voter fraud on confidence in elections. Journal of Experimental Political Science , 10 (1), 34–49. https://doi.org/10.1017/XPS.2021.18
Bizumic, B., & Duckitt, J. (2018). Investigating right wing authoritarianism with a very short authoritarianism scale. Journal of Social and Political Psychology , 6 (1), 129–150. https://doi.org/10.5964/jspp.v6i1.835
Bor, A., & Petersen, M. B. (2022). The psychology of online political hostility: A comprehensive, cross-national test of the mismatch hypothesis. American Political Science Review , 116 (1), 1–18. https://doi.org/10.1017/S0003055421000885
Bryanov, K., & Vziatysheva, V. (2021). Determinants of individuals’ belief in fake news: A scoping review determinants of belief in fake news. PLOS ONE , 16 (6), e0253717. https://doi.org/10.1371/journal.pone.0253717
Buchanan, T., & Benson, V. (2019). Spreading disinformation on Facebook: Do trust in message source, risk propensity, or personality affect the organic reach of “fake news”? Social Media + Society , 5 (4), 2056305119888654. https://doi.org/10.1177/2056305119888654
Buchanan, T., & Kempley, J. (2021). Individual differences in sharing false political information on social media: Direct and indirect effects of cognitive-perceptual schizotypy and psychopathy. Personality and Individual Differences , 182 , 111071. https://doi.org/10.1016/j.paid.2021.111071
Buhr, K., & Dugas, M. J. (2002). The intolerance of uncertainty scale: Psychometric properties of the English version. Behaviour Research and Therapy , 40 (8), 931–945. https://doi.org/10.1016/S0005-7967(01)00092-4
Celse, J., & Chang, K. (2019). Politicians lie, so do I. Psychological Research , 83 (6), 1311–1325. https://doi.org/10.1007/s00426-017-0954-7
Choi, T. R., & Sung, Y. (2018). Instagram versus Snapchat: Self-expression and privacy concern on social media. Telematics and Informatics , 35 (8), 2289–2298. https://doi.org/10.1016/j.tele.2018.09.009
Chun, J. W., & Lee, M. J. (2017). When does individuals’ willingness to speak out increase on social media? Perceived social support and perceived power/control. Computers in Human Behavior , 74 , 120–129. https://doi.org/10.1016/j.chb.2017.04.010
Conrad, K. J., Riley, B. B., Conrad, K. M., Chan, Y.-F., & Dennis, M. L. (2010). Validation of the Crime and Violence Scale (CVS) against the Rasch measurement model including differences by gender, race, and age. Evaluation Review , 34 (2), 83–115. https://doi.org/10.1177/0193841×10362162
Costello, T. H., Bowes, S. M., Stevens, S. T., Waldman, I. D., Tasimi, A., & Lilienfeld, S. O. (2022). Clarifying the structure and nature of left-wing authoritarianism. Journal of Personality and Social Psychology , 122 (1), 135–170. https://doi.org/10.1037/pspp0000341
Courchesne, L., Ilhardt, J., & Shapiro, J. N. (2021). Review of social science research on the impact of countermeasures against influence operations. Harvard Kennedy School (HKS) Misinformation Review, 2 (5). https://doi.org/10.37016/mr-2020-79
Crawford, J. R., & Henry, J. D. (2004). The positive and negative affect schedule (PANAS): Construct validity, measurement properties and normative data in a large non-clinical sample. British Journal of Clinical Psychology , 43 (3), 245–265. https://doi.org/10.1348/0144665031752934
Dang, J., King, K. M., & Inzlicht, M. (2020). Why are self-report and behavioral measures weakly correlated? Trends in Cognitive Sciences , 24 (4), 267–269. https://doi.org/10.1016/j.tics.2020.01.007
Del Vicario, M., Bessi, A., Zollo, F., Petroni, F., Scala, A., Caldarelli, G., Stanley, H. E., & Quattrociocchi, W. (2016). The spreading of misinformation online. Proceedings of the National Academy of Sciences , 113 (3), 554–559. https://doi.org/10.1073/pnas.1517441113
DePaulo, B. M., Kashy, D. A., Kirkendol, S. E., Wyer, M. M., & Epstein, J. A. (1996). Lying in everyday life. Journal of Personality and Social Psychology , 70 (5), 979–995. https://doi.org/10.1037/0022-3514.70.5.979
Desmond, S. A., & Kraus, R. (2012). Liar, liar: Adolescent religiosity and lying to parents. Interdisciplinary Journal of Research on Religion , 8, 1–26. https://www.religjournal.com/pdf/ijrr08005.pdf
DeVerna, M. R., Guess, A. M., Berinsky, A. J., Tucker, J. A., & Jost, J. T. (2022). Rumors in retweet: Ideological asymmetry in the failure to correct misinformation. Personality and Social Psychology Bulletin , 01461672221114222. https://doi.org/10.1177/01461672221114222
Durand, M.-A., Yen, R. W., O’Malley, J., Elwyn, G., & Mancini, J. (2020). Graph literacy matters: Examining the association between graph literacy, health literacy, and numeracy in a Medicaid eligible population. PLOS ONE , 15 (11), e0241844. https://doi.org/10.1371/journal.pone.0241844
Ecker, U. K. H., Sze, B. K. N., & Andreotta, M. (2021). Corrections of political misinformation: No evidence for an effect of partisan worldview in a US convenience sample. Philosophical Transactions of the Royal Society B: Biological Sciences , 376 (1822), 20200145. https://doi.org/10.1098/rstb.2020.0145
Edelson, J., Alduncin, A., Krewson, C., Sieja, J. A., & Uscinski, J. E. (2017). The effect of conspiratorial thinking and motivated reasoning on belief in election fraud. Political Research Quarterly , 70 (4), 933–946. https://doi.org/10.1177/1065912917721061
Enders, A. M., Uscinski, J., Klofstad, C., & Stoler, J. (2022). On the relationship between conspiracy theory beliefs, misinformation, and vaccine hesitancy. PLOS ONE , 17 (10), e0276082. https://doi.org/10.1371/journal.pone.0276082
Frankfurt, H. G. (2009). On bullshit . Princeton University Press.
Garrett, R. K. (2017). The “echo chamber” distraction: Disinformation campaigns are the problem, not audience fragmentation. Journal of Applied Research in Memory and Cognition , 6 (4), 370–376. https://doi.org/10.1016/j.jarmac.2017.09.011
Garrett, R. K., & Bond, R. M. (2021). Conservatives’ susceptibility to political misperceptions. Science Advances , 7 (23), eabf1234. https://doi.org/10.1126/sciadv.abf1234
Garrett, R. K., Long, J. A., & Jeong, M. S. (2019). From partisan media to misperception: Affective polarization as mediator. Journal of Communication , 69 (5), 490–512. https://doi.org/10.1093/joc/jqz028
Grant, J. E., Paglia, H. A., & Chamberlain, S. R. (2019). The phenomenology of lying in young adults and relationships with personality and cognition. Psychiatric Quarterly , 90 (2), 361–369. https://doi.org/10.1007/s11126-018-9623-2
Green, C. E. L., Freeman, D., Kuipers, E., Bebbington, P., Fowler, D., Dunn, G., & Garety, P. A. (2008). Measuring ideas of persecution and social reference: The Green et al. Paranoid Thought Scales (GPTS). Psychological Medicine , 38 (1), 101–111. https://doi.org/10.1017/S0033291707001638
Grinberg, N., Joseph, K., Friedland, L., Swire-Thompson, B., & Lazer, D. (2019). Fake news on Twitter during the 2016 U.S. presidential election. Science , 363 (6425), 374–378. https://doi.org/10.1126/science.aau2706
Guess, A., Nyhan, B., & Reifler, J. (2020). Exposure to untrustworthy websites in the 2016 U.S. election. Nature Human Behaviour , 4 (5), 472–480. https://doi.org/10.1038/s41562-020-0833-x
Guess, A. M., & Lyons, B. A. (2020). Misinformation, disinformation, and online propaganda. In N. Persily & J. A. Tucker (Eds.), Social media and democracy: The state of the field and prospects for reform (pp. 10–33), Cambridge University Press. https://doi.org/10.1017/9781108890960
Ha, L., Graham, T., & Gray, J. (2022). Where conspiracy theories flourish: A study of YouTube comments and Bill Gates conspiracy theories. Harvard Kennedy School (HKS) Misinformation Review, 3 (5). https://doi.org/10.37016/mr-2020-107
Halevy, R., Shalvi, S., & Verschuere, B. (2014). Being honest about dishonesty: Correlating self-reports and actual lying. Human Communication Research , 40 (1), 54–72. https://doi.org/10.1111/hcre.12019
Hughes, A. (2019). A small group of prolific users account for a majority of political tweets sent by U.S. adults. Pew Research Center. https://pewrsr.ch/35YXMrM
Johnson, T. J., Wallace, R., & Lee, T. (2022). How social media serve as a super-spreader of misinformation, disinformation, and conspiracy theories regarding health crises. In J. H. Lipschultz, K. Freberg, & R. Luttrell (Eds.), The Emerald handbook of computer-mediated communication and social media (pp. 67–84). Emerald Publishing Limited. https://doi.org/10.1108/978-1-80071-597-420221005
Jonason, P. K., & Webster, G. D. (2010). The dirty dozen: A concise measure of the dark triad. Psychological Assessment , 22 (2), 420–432. https://doi.org/https://doi.org/10.1037/a0019265
Kaiser, C., & Oswald, A. J. (2022). The scientific value of numerical measures of human feelings. Proceedings of the National Academy of Sciences , 119 (42), e2210412119. https://doi.org/doi:10.1073/pnas.2210412119
Kim, J. W., Guess, A., Nyhan, B., & Reifler, J. (2021). The distorting prism of social media: How self-selection and exposure to incivility fuel online comment toxicity. Journal of Communication , 71 (6), 922–946. https://doi.org/10.1093/joc/jqab034
Lasser, J., Aroyehun, S. T., Simchon, A., Carrella, F., Garcia, D., & Lewandowsky, S. (2022). Social media sharing of low-quality news sources by political elites. PNAS Nexus , 1 (4). https://doi.org/10.1093/pnasnexus/pgac186
Lawson, M. A., & Kakkar, H. (2021). Of pandemics, politics, and personality: The role of conscientiousness and political ideology in the sharing of fake news. Journal of Experimental Psychology: General , 151 (5), 1154–1177. https://doi.org/10.1037/xge0001120
Littrell, S., Risko, E. F., & Fugelsang, J. A. (2021a). The bullshitting frequency scale: Development and psychometric properties. British Journal of Social Psychology , 60 (1), e12379. https://doi.org/10.1111/bjso.12379
Littrell, S., Risko, E. F., & Fugelsang, J. A. (2021b). ‘You can’t bullshit a bullshitter’ (or can you?): Bullshitting frequency predicts receptivity to various types of misleading information. British Journal of Social Psychology , 60 (4), 1484–1505. https://doi.org/10.1111/bjso.12447
Lopez, J., & Hillygus, D. S. (March 14, 2018). Why so serious?: Survey trolls and misinformation . SSRN. http://dx.doi.org/10.2139/ssrn.3131087
MacKenzie, A., & Bhatt, I. (2020). Lies, bullshit and fake news: Some epistemological concerns. Postdigital Science and Education , 2 (1), 9–13. https://doi.org/10.1007/s42438-018-0025-4
Marwick, A., & Lewis, R. (2017). Media manipulation and disinformation online . Data & Society Research Institute. https://datasociety.net/library/media-manipulation-and-disinfo-online/
McClosky, H., & Chong, D. (1985). Similarities and differences between left-wing and right-wing radicals. British Journal of Political Science , 15 (3), 329–363. https://doi.org/10.1017/S0007123400004221
Metzger, M. J., Flanagin, A. J., Mena, P., Jiang, S., & Wilson, C. (2021). From dark to light: The many shades of sharing misinformation online. Media and Communication , 9 (1), 134–143. https://doi.org/10.17645/mac.v9i1.3409
Moran, R. E., Prochaska, S., Schlegel, I., Hughes, E. M., & Prout, O. (2021). Misinformation or activism: Mapping networked moral panic through an analysis of #savethechildren. AoIR Selected Papers of Internet Research , 2021 . https://doi.org/10.5210/spir.v2021i0.12212
Mosleh, M., & Rand, D. G. (2022). Measuring exposure to misinformation from political elites on Twitter. Nature Communications , 13 , 7144. https://doi.org/10.1038/s41467-022-34769-6
Nguyen, H., & Gokhale, S. S. (2022). Analyzing extremist social media content: A case study of Proud Boys. Social Network Analysis and Mining , 12 (1), 115. https://doi.org/10.1007/s13278-022-00940-6
Okamoto, S., Niwa, F., Shimizu, K., & Sugiman, T. (2001). The 2001 survey for public attitudes towards and understanding of science and technology in Japan. National Institute of Science and Technology Policy Ministry of Education, Culture, Sports, Science and Technology. https://nistep.repo.nii.ac.jp/record/4385/files/NISTEP-NR072-SummaryE.pdf
Paulhus, D. L., Buckels, E. E., Trapnell, P. D., & Jones, D. N. (2020). Screening for dark personalities. European Journal of Psychological Assessment , 37 (3), 208–222. https://doi.org/10.1027/1015-5759/a000602
Pennycook, G., Epstein, Z., Mosleh, M., Arechar, A. A., Eckles, D., & Rand, D. G. (2021). Shifting attention to accuracy can reduce misinformation online. Nature , 592 (7855), 590–595. https://doi.org/10.1038/s41586-021-03344-2
Pennycook, G., & Rand, D. G. (2020). Who falls for fake news? The roles of bullshit receptivity, overclaiming, familiarity, and analytic thinking. Journal of Personality , 88 (2), 185–200. https://doi.org/10.1111/jopy.12476
Pennycook, G., & Rand, D. G. (2021). The psychology of fake news. Trends in Cognitive Sciences , 25 (5), 388–402. https://doi.org/10.1016/j.tics.2021.02.007
Petersen, M. B., Osmundsen, M., & Arceneaux, K. (2023). The “need for chaos” and motivations to share hostile political rumors. American Political Science Review , 1–20. https://doi.org/10.1017/S0003055422001447
Romer, D., & Jamieson, K. H. (2021). Patterns of media use, strength of belief in Covid-19 conspiracy theories, and the prevention of Covid-19 from March to July 2020 in the United States: Survey study. Journal of Medical Internet Research , 23 (4), e25215. https://doi.org/10.2196/25215
Roozenbeek, J., van der Linden, S., Goldberg, B., Rathje, S., & Lewandowsky, S. (2022). Psychological inoculation improves resilience against misinformation on social media. Science Advances , 8 (34), eabo6254. https://doi.org/doi:10.1126/sciadv.abo6254
Sanderson, Z., Brown, M. A., Bonneau, R., Nagler, J., & Tucker, J. A. (2021). Twitter flagged Donald Trump’s tweets with election misinformation: They continued to spread both on and off the platform. Harvard Kennedy School (HKS) Misinformation Review , 2 (4). https://doi.org/10.37016/mr-2020-77
Schaffner, B. F., & Luks, S. (2018). Misinformation or expressive responding? What an inauguration crowd can tell us about the source of political misinformation in surveys. Public Opinion Quarterly , 82 (1), 135–147. https://doi.org/10.1093/poq/nfx042
Serota, K. B., & Levine, T. R. (2015). A few prolific liars: Variation in the prevalence of lying. Journal of Language and Social Psychology , 34 (2), 138–157. https://doi.org/10.1177/0261927X14528804
Smallpage, S. M., Enders, A. M., Drochon, H., & Uscinski, J. E. (2022). The impact of social desirability bias on conspiracy belief measurement across cultures. Political Science Research and Methods , 11 (3), 555–569. https://doi.org/10.1017/psrm.2022.1
Starbird, K. (2019). Disinformation’s spread: Bots, trolls and all of us. Nature , 571 (7766), 449–450. https://doi.org/10.1038/d41586-019-02235-x
Stern, A. M. (2019). Proud Boys and the white ethnostate: How the alt-right is warping the American imagination . Beacon Press.
Sunstein, C. R. (2021). Liars: Falsehoods and free speech in an age of deception . Oxford University Press.
Tucker, J. A., Guess, A., Barberá, P., Vaccari, C., Siegel, A., Sanovich, S., Stukal, D., & Nyhan, B. (2018). Social media, political polarization, and political disinformation: A review of the scientific literature. SSRN. https://dx.doi.org/10.2139/ssrn.3144139
Urman, A., & Katz, S. (2022). What they do in the shadows: Examining the far-right networks on Telegram. Information, Communication & Society , 25 (7), 904–923. https://doi.org/10.1080/1369118X.2020.1803946
Uscinski, J., Enders, A., Seelig, M. I., Klofstad, C. A., Funchion, J. R., Everett, C., Wuchty, S., Premaratne, K., & Murthi, M. N. (2021). American politics in two dimensions: Partisan and ideological identities versus anti-establishment orientations. American Journal of Political Science , 65 (4), 773–1022. https://doi.org/10.1111/ajps.12616
Uscinski, J., Enders, A. M., Klofstad, C., & Stoler, J. (2022). Cause and effect: On the antecedents and consequences of conspiracy theory beliefs. Current Opinion in Psychology , 47, 101364. https://doi.org/10.1016/j.copsyc.2022.101364
van der Linden, S., Roozenbeek, J., Maertens, R., Basol, M., Kácha, O., Rathje, S., & Traberg, C. S. (2021). How can psychological science help counter the spread of fake news? The Spanish Journal of Psychology , 24 , e25. https://doi.org/10.1017/SJP.2021.23
Vincent, E. M., Théro, H., & Shabayek, S. (2022). Measuring the effect of Facebook’s downranking interventions against groups and websites that repeatedly share misinformation. Harvard Kennedy School (HKS) Misinformation Review, 3 (3). https://doi.org/10.37016/mr-2020-100
Vosoughi, S., Roy, D., & Aral, S. (2018). The spread of true and false news online. Science , 359 (6380), 1146–1151. https://doi.org/10.1126/science.aap9559
Vraga, E. K., & Bode, L. (2020). Defining misinformation and understanding its bounded nature: Using expertise and evidence for describing misinformation. Political Communication , 37 (1), 136–144. https://doi.org/10.1080/10584609.2020.1716500
Xiao, X., Borah, P., & Su, Y. (2021). The dangers of blind trust: Examining the interplay among social media news use, misinformation identification, and news trust on conspiracy beliefs. Public Understanding of Science , 30 (8), 977–992. https://doi.org/10.1177/0963662521998025
Zeng, J., & Schäfer, M. S. (2021). Conceptualizing “dark platforms.” Covid-19-related conspiracy theories on 8kun and Gab. Digital Journalism , 9 (9), 1321–1343. https://doi.org/10.1080/21670811.2021.1938165
Zettler, I., Hilbig, B. E., Moshagen, M., & de Vries, R. E. (2015). Dishonest responding or true virtue? A behavioral test of impression management. Personality and Individual Differences , 81 , 107–111. https://doi.org/10.1016/j.paid.2014.10.007
This research was funded by grants from the National Science Foundation #2123635 and #2123618.
Competing Interests
All authors declare no competing interests.
Approval for this study was granted by the University of Miami Human Subject Research Office on May 13, 2022 (Protocol #20220472).
This is an open access article distributed under the terms of the Creative Commons Attribution License , which permits unrestricted use, distribution, and reproduction in any medium, provided that the original author and source are properly credited.
Data Availability
All materials needed to replicate this study are available via the Harvard Dataverse: https://doi.org/10.7910/DVN/AWNAKN

Vance Championed 2017 Report on Families From Architects of Project 2025
JD Vance, as he was dipping his toe into politics, praised the Heritage Foundation report — 29 essays opposing abortion and seeking to instruct Americans on how to raise children — as “admirable.”
In his introduction to the 2017 Heritage Foundation report, JD Vance argued that economic struggles were inextricable from what he saw as cultural decay. Credit... Jamie Kelter Davis for The New York Times
Supported by
- Share full article

By Lisa Lerer
- Sept. 3, 2024
Years before he became the Republican vice-presidential nominee, JD Vance endorsed a little-noticed 2017 report by the Heritage Foundation that proposed a sweeping conservative agenda to restrict sexual and reproductive freedoms and remake American families.
In a series of 29 separate essays, conservative commentators, policy experts, community leaders and Christian clergy members opposed the spread of in vitro fertilization and other fertility treatments, describing those treatments as harmful to women. They praised the rapidly expanding number of state laws restricting abortion rights and access, saying that the procedure should become “unthinkable” in America. And they cited hunger as a “great motivation” for Americans to find work.
Mr. Vance, then known as the author of a best-selling memoir, became a champion of the project. He wrote the introduction and praised the volume as “admirable,” and was the keynote speaker at the public release of the report at Heritage’s offices in Washington.
The report was released just months after Donald J. Trump became president, as social conservatives were laying the foundation for an aggressive agenda restricting sexual freedom and reproductive rights. Those policies became a hallmark of the Trump administration and Mr. Vance’s political career.
Taken together, the pieces in the report amount to an effort to instruct Americans on what their families should be, when to grow them and the best way to raise their children. Authors argued in the 2017 report that women should become pregnant at younger ages and that a two-parent, heterosexual household was the “ideal” environment for children.
“The ideal situation for any child is growing up with the mother and father who brought that child into the world,” wrote Katrina Trinko, a conservative journalist, in an essay detailing the “tragedy” of babies born to single mothers.
We are having trouble retrieving the article content.
Please enable JavaScript in your browser settings.
Thank you for your patience while we verify access. If you are in Reader mode please exit and log into your Times account, or subscribe for all of The Times.
Thank you for your patience while we verify access.
Already a subscriber? Log in .
Want all of The Times? Subscribe .
Advertisement
It could again take Pa. days to count mail ballots in November, despite lawmakers having since 2020 to address the issue
“They should be ashamed of themselves,” Forrest Lehman, director of elections in Lycoming County, said of Pa. lawmakers who have failed to address the issue.
/cloudfront-us-east-1.images.arcpublishing.com/pmn/BOZ6ILNQJBH2FK76BDD6QXPVAU.jpg)
Election officials in Pennsylvania are warning that because of inaction in Harrisburg, the swing state’s votes may again take days to count, creating a window for bad actors to sow distrust in the results.
County election officials have been petitioning state lawmakers to update the state’s election code for years. They’ve also persistently asked the General Assembly to clarify questions surrounding which mail ballots can and cannot be counted to address voting rights litigation that has played out in Pennsylvania since no-excuse mail voting was authorized in 2019.
But as November approaches, little has changed since 2020 — when former President Donald Trump used the slow counting process to promote unfounded claims of voter fraud in the wake of his defeat.
After four years, state lawmakers are unlikely to make changes before Election Day, which adds additional stress for county officials and sets up a scenario to again put the state at the center of conspiracy theories and controversies in a close election between Trump and Vice President Kamala Harris .
“They should be ashamed of themselves,” said Forrest Lehman, director of elections in Lycoming County. “Counties have been asking repeatedly for some of this relief for years and we still can’t get it and then everyone seems fine with letting us take the blame for it.”
Meanwhile, litigation over what mail ballots to count and reject are working their way in court leaving officials unclear exactly which ballots they’ll have to count or reject in November while efforts to reform state law to allow more time to process mail ballots have failed to progress in the Capitol.
“They’re not doing their jobs. So then [counties] have to be the adults in the room and figure out how to interpret these ambiguities and come up with policies we think make sense.”
Window for misinformation
Under current law, county election offices cannot begin opening mail ballots and preparing them to be counted, a process called pre-canvassing, until 7 a.m. on Election Day.
The short window can create a strain on resources, requiring more Election Day staff to ensure mail ballots are ready to be counted by the time polls close at 8 p.m., and it also makes it more difficult for election officials to finish counting mail ballots that day.
The Department of State said in a statement providing more time should be nonpartisan. Pennsylvania Secretary of State Al Schmidt called the legislative inaction “frustrating” in an interview with the Washington Post last month.
In 2020, this strain was one of the reasons county election offices took several days to finish tallying mail ballots. And it also fueled unfounded claims of voter fraud.
When voters went to bed on election night, Trump was leading in Pennsylvania among ballots that had been counted. Biden ultimately won Pennsylvania because of his overwhelming lead in mail ballots, counted in the following days. Trump baselessly insisted this was evidence of cheating. Schmidt, then a Republican member of the Philadelphia City Commissioners, faced death threats after he pushed back against Trump’s false claims and the former president called him out by name on Twitter.
The former president has indicated he’s likely to claim fraud again in November if Harris wins the state. The Trump campaign launched a website last week c alled Swamp the Vote to encourage Republicans to vote early and by mail. The messaging on the website continues to argue, without evidence, that Democrats may cheat using mail ballots — even as Trump encourages his supporters to vote by mail.
“We never want what happened in 2020 to happen again,” Trump says in a video on the website. “But until then Republicans must win and we must use every appropriate tool available to beat the Democrats.”
Advocates worry that the legislature’s inaction on pre-canvassing will set the stage for misinformation to run wild again and create a potentially dangerous environment.
“The legislature’s failure to act is an absolute abdication of their responsibility to all Pennsylvanians, not just Pennsylvania voters, if not also the nation,” said Lauren Cristella, president of the Committee of Seventy, a Philly-based government watchdog.
City Commissioner Seth Bluestein, a Republican, said the window of time between a race being unofficially called by the Associated Press and TV networks and polls closing is an opportunity for misinformation to spread. The votes are not formally certified until counties hold their canvasses in the days and weeks following the election.
“If we had pre-canvassing we would be able to release the results from the mail ballots on election night alongside the in-person results and it would close and narrow that window of time when misinformation spreads.”
If turnout is high enough, and enough of those votes are cast by mail, election officials will be dealing with many of the same logistical hurdles they faced in 2020 that caused mail ballots to be tallied after election night. But the counting process is expected to be faster this year than 2020.
Fewer mail ballots are expected than 2020 when the pandemic spurred high rates of mail voting nationwide. The number of voters casting ballots by mail has dramatically reduced in recent elections.
Bluestein predicted the mail ballots would be counted within one or two days in Philadelphia this November.
In 2022 lawmakers passed a law offering more state funding to election offices , but in exchange outside funding was banned and election offices are now required to work around the clock until all ballots are counted. That means offices will have workers counting all night rather than taking breaks.
Additionally, election offices have had more time to adjust to Pennsylvania’s no-excuse mail voting law. Offices have purchased equipment that speeds up the ballot counting process and have found ways to count the high number of ballots more efficiently.
“I’m pretty confident we’re not going to see that long lag that we had in 2020,” City Commissioner Lisa Deeley, a Democrat, said. But she noted that when networks feel comfortable calling the race will depend in part on how many mail ballots counties receive and how tight the election is.
“It will take as long as it takes and how long that is and what that pressure point becomes is all reliant on the state of the race.”
State Rep. Seth Grove, a York County Republican, said Pennsylvania counties have had relatively few struggles counting ballots quickly since counties began working around the clock.
“The 7 a.m. [start time] mixed with the continuous count has really, I think, neutered the need for more additional days pre-canvassing these ballots,” Grove said, arguing addressing pre-canvassing alone could cause issues for county voting security measures.
Pennsylvania House Democrats in April advanced a bill that would have allowed for an additional week of pre-canvassing ahead of Election Day. But the bill stalled in the GOP-controlled Senate.
State Sen. Cris Dush (R., Jefferson), who leads election policy in the state Senate, did not respond to questions from the Inquirer about why he hasn’t taken up the bill, but Senate GOP leaders told VoteBeat in May that they wouldn’t discuss election changes without inclusion of a voter ID requirement.
Republicans passed a bill in 2021 that tied voter ID to pre-canvassing and other measures. It was vetoed by then-Gov. Tom Wolf, a Democrat.
“There are massive trust issues trying to find a compromise between Republicans and Democrats,” Grove said. “We don’t want to see a … where we negotiate in good faith and then have the Democrats come back and knock off those compromises in the legal processes.”
State Rep. Scott Conklin, a State College Democrat, blamed political gamesmanship.
“They’re setting it up so that if their man wins, they won in spite of the system. If their person loses, oh, it has to be ‘Look, they stole the election,’” Conklin said of GOP lawmakers.
Rejected mail ballots
Ahead of November’s election, Pennsylvania’s election code, and, specifically, statutes established when lawmakers approved no-excuse mail voting in 2019, remain under a flurry of litigation.
Pennsylvania law requires voters to sign and date their ballot and place it in a secrecy envelope in order for it to be counted.
The Pennsylvania Commonwealth Court issued a ruling Friday instructing election officials not to enforce the requirement that ballots be dated, finding it infringed on voting rights and did not serve a compelling government interest. The ruling could be appealed.
In addition to the ballot dating case, the American Civil Liberties Union has challenged the lack of notification in Washington County for when ballots are rejected. The litigation has led to often shifting guidelines and patchwork of responses across the state as counties interpret the case law in differing ways.
“In some respects, the legislature is essentially delegating to the courts,” said Thad Hall, elections director in Mercer County. “Now you get this huge difference based on where you live.”
The resolution of those lawsuits could bring more clarity on some of those items by Election Day, depending on rulings.
“Our goal is to have it clarified one way or the other before November,” said Marian Schneider, senior voting rights policy council at the American Civil Liberties Union of Pennsylvania.
But continued changes based on court rulings and county to county inconsistencies could cause confusion ahead of the election. Cristella, president of the Committee of Seventy, said it will be important that rulings come in a timely manner, so that voters can learn the new rules.
“It just adds a level of chaos that nobody needs,” she said.
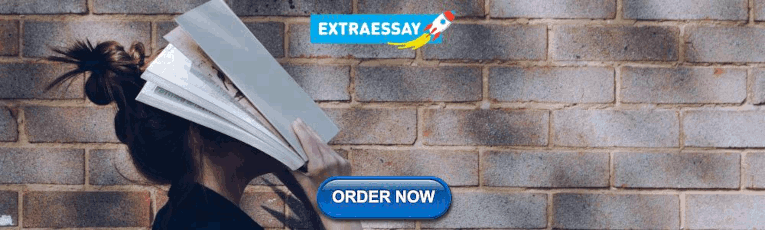
IMAGES
COMMENTS
Howard (Citation 2020, pp. 153-167) identifies the political economy of data gathering and retention as the centrepiece of the problem with disinformation, misinformation and fake news. As such, he proposes that restrictions need to be placed on the commercial trading of information, and that there should be new requirements on both the ...
What is the difference between misinformation and disinformation? That was among the key questions considered at the recent Strategies for Combating Political Misinformation panel hosted by the Columbia University School of Professional Studies (SPS) Strategic Communication and Political Analytics graduate programs. The discussion centered on the varying factors that determine the influence of ...
Misinformation and competing views of reality abounded throughout 2020. By Amy Mitchell, Mark Jurkowitz, J. Baxter Oliphant and Elisa Shearer. Unprecedented national news events, a sharp and sometimes hostile political divide, and polarized news streams created a ripe environment for misinformation and made-up news in 2020.
Misinformation poses a bigger threat to democracy than you might think. In today's polarized political climate, researchers who combat mistruths have come under attack and been labelled as ...
Misinformation occurs when people hold incorrect factual beliefs and do so confidently. The problem, first conceptualized by Kuklinski and colleagues in 2000, plagues political systems and is exceedingly difficult to correct. In this review, we assess the empirical literature on political misinformation in the United States and consider what scholars have learned since the publication of that ...
Pervasive political misinformation threatens the integrity of American electoral democracy but not in the manner most commonly examined. We argue the presumed influence of misinformation (PIM) may be just as pernicious, and widespread, as any direct influence that political misinformation may have on voters. Our online survey of 2,474 respondents in the United States shows
Fact-checking and warnings of misinformation are increasingly salient and prevalent components of modern news media and political communications. While many warnings about political misinformation are valid and enable people to reject misleading information, the quality and validity of misinformation warnings can vary widely. Replicating and extending research from the fields of social ...
This essay advocates a critical approach to disinformation research that is grounded in history, culture, and politics, and centers questions of power and inequality. In the United States, identity, particularly race, plays a key role in the messages and strategies of disinformation producers and who disinformation and misinformation resonates with. Expanding what "counts" as disinformation
Table 1. Misinformation mentions and proportions 8 The proportions in parentheses in Table 1 indicate the ratio of misinformation to information—that is, the number of mentions of misinformation divided by the number of units of information (articles, segments, tweets, or surveys about the candidates). Mentions are aggregated by misinformation-related topic rather than by article, so a given ...
Challenges of misinformation, disinformation, and fake news. Although there are different normative perspectives on how informed citizens in democracies should be, there is broad scholarly consensus that democratic efficiency and representation greatly benefit the more informed citizens are about politics and society (Delli Carpini & Keeter, Citation 1996; Hochschild & Einstein, Citation 2015).
Misinformation occurs when people hold incorrect factual beliefs and do so confidently. The problem plagues political systems and is exceedingly difficult to correct. In this review, we assess the empirical literature on political misinformation in the United States and consider what scholars have learned since the publication of that early study. We conclude that research on this topic has ...
In conjunction with the circulation of claims of election fraud and misinformation throughout the country, the public's trust in our democratic system subsequently declined as well. An ABC NEWS ...
This introductory essay is organized into four sections following this one. We begin by situating our understanding of disinformation within the wider crisis of democracy and political communication. ... Still, this early work in political misinformation drew at least two substantial conclusions from which present-day research still draws: 1 ...
Journal of Experimental Political Science, 2015. doi: 10.1017/XPS.2014.22. Abstract: "Misinformation can be very difficult to correct and may have lasting effects even after it is discredited. One reason for this persistence is the manner in which people make causal inferences based on available information about a given event or outcome.
Organised social media manipulation campaigns were found in each of the 81 surveyed countries, up 15% in one year, from 70 countries in 2019. Governments, public relations firms and political parties are producing misinformation on an industrial scale, according to the report.
With the current political situation in a state of great flux in the U.S. and around the world, there are questions concerning the quality of the information available to the general public and ...
As Lewandowsky et al. (2012, p. 120) argue, "skepticism can reduce susceptibility to misinformation effects if it prompts people to question the origins of information that may later turn out to be false," while at the same time ensuring that accurate information is recognized and valued.
Political misinformation refers to the sharing of false information without the intent of causing harm. Since information and technology have become critical in the lives of individuals nowadays, misinformation has a significant impact. People spend substantial amounts of time online chatting and engaging in various forms of communication. The world currently relies on the information […]
USC study reveals the key reason why fake news spreads on social media. The USC-led study of more than 2,400 Facebook users suggests that platforms — more than individual users — have a larger role to play in stopping the spread of misinformation online. January 17, 2023 By Pamela Madrid.
America's right flank remains the chief purveyor of misinformation, but this summer's political tumult created ideal conditions for falsehoods to spread among progressives. By Stuart A ...
Those with a more extreme and right-wing political orientation have also consistently shown to be more susceptible to misinformation 3,4,33,36,37, even when the misinformation in question is non ...
We surveyed 150 academic experts on misinformation and identified areas of expert consensus. Experts defined misinformation as false and misleading information, though views diverged on the importance of intentionality and what exactly constitutes misinformation. The most popular reason why people believe and share misinformation was partisanship, while lack of education was one of the least
We used the remaining papers to understand the field, reveal the challenges, review the detection techniques, and discuss future directions. ... comparing the effects of news media literacy interventions and fact-checkers in response to political misinformation in the us and netherlands. Inf Commun Soc. 2022; 25 (1):110-126. doi: 10.1080 ...
Propaganda, misinformation and fake news have the potential to polarise public opinion, to promote violent extremism and hate speech and, ultimately, to undermine democracies and reduce trust in the democratic processes. It is vital for schools to provide students with a solid education on media and information literacy as part of the curriculum.
Tips to identify misinformation online. Consider the source — Is the information coming from a reputable source; Check the date — Just because this may be the first time you're seeing an article doesn't necessarily mean it's new or current.; Check other sources — Always confirm information with multiple legitimate sources online.; Check the location — Is the photo you're ...
In recent years, the tone sharpened as Spamouflage expanded and began focusing on divisive political topics like gun control, crime, race relations and support for Israel during its war in Gaza.
Mr. Roth is the president of Wesleyan University. Last year was a tough one on college campuses, so over the summer a lot of people asked me if I was hoping things would be less political this ...
Normatively, it is encouraging that only a small minority of our respondents indicated that they share false information about politics on social media. However, the fact that 14% of the U.S. adult population claims to purposely spread political misinformation online is nonetheless troubling.
JD Vance, as he was dipping his toe into politics, praised the Heritage Foundation report — 29 essays opposing abortion and seeking to instruct Americans on how to raise children — as ...
City Commissioner Seth Bluestein, a Republican, said the window of time between a race being unofficially called by the Associated Press and TV networks and polls closing is an opportunity for misinformation to spread. The votes are not formally certified until counties hold their canvasses in the days and weeks following the election.