If you're seeing this message, it means we're having trouble loading external resources on our website.
If you're behind a web filter, please make sure that the domains *.kastatic.org and *.kasandbox.org are unblocked.
To log in and use all the features of Khan Academy, please enable JavaScript in your browser.
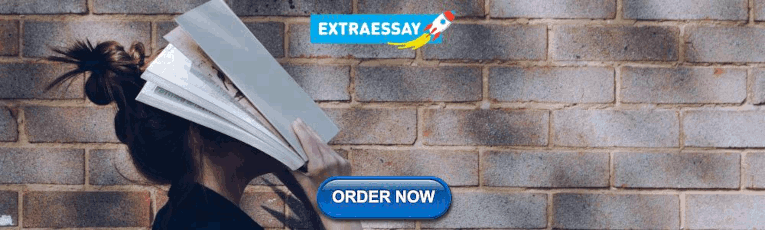
Biology archive
Course: biology archive > unit 1.
- The scientific method
Controlled experiments
- The scientific method and experimental design

Introduction
How are hypotheses tested.
- One pot of seeds gets watered every afternoon.
- The other pot of seeds doesn't get any water at all.
Control and experimental groups
Independent and dependent variables, independent variables, dependent variables, variability and repetition, controlled experiment case study: co 2 and coral bleaching.
- What your control and experimental groups would be
- What your independent and dependent variables would be
- What results you would predict in each group
Experimental setup
- Some corals were grown in tanks of normal seawater, which is not very acidic ( pH around 8.2 ). The corals in these tanks served as the control group .
- Other corals were grown in tanks of seawater that were more acidic than usual due to addition of CO 2 . One set of tanks was medium-acidity ( pH about 7.9 ), while another set was high-acidity ( pH about 7.65 ). Both the medium-acidity and high-acidity groups were experimental groups .
- In this experiment, the independent variable was the acidity ( pH ) of the seawater. The dependent variable was the degree of bleaching of the corals.
- The researchers used a large sample size and repeated their experiment. Each tank held 5 fragments of coral, and there were 5 identical tanks for each group (control, medium-acidity, and high-acidity). Note: None of these tanks was "acidic" on an absolute scale. That is, the pH values were all above the neutral pH of 7.0 . However, the two groups of experimental tanks were moderately and highly acidic to the corals , that is, relative to their natural habitat of plain seawater.
Analyzing the results
Non-experimental hypothesis tests, case study: coral bleaching and temperature, attribution:, works cited:.
- Hoegh-Guldberg, O. (1999). Climate change, coral bleaching, and the future of the world's coral reefs. Mar. Freshwater Res. , 50 , 839-866. Retrieved from www.reef.edu.au/climate/Hoegh-Guldberg%201999.pdf.
- Anthony, K. R. N., Kline, D. I., Diaz-Pulido, G., Dove, S., and Hoegh-Guldberg, O. (2008). Ocean acidification causes bleaching and productivity loss in coral reef builders. PNAS , 105 (45), 17442-17446. http://dx.doi.org/10.1073/pnas.0804478105 .
- University of California Museum of Paleontology. (2016). Misconceptions about science. In Understanding science . Retrieved from http://undsci.berkeley.edu/teaching/misconceptions.php .
- Hoegh-Guldberg, O. and Smith, G. J. (1989). The effect of sudden changes in temperature, light and salinity on the density and export of zooxanthellae from the reef corals Stylophora pistillata (Esper, 1797) and Seriatopora hystrix (Dana, 1846). J. Exp. Mar. Biol. Ecol. , 129 , 279-303. Retrieved from http://www.reef.edu.au/ohg/res-pic/HG%20papers/HG%20and%20Smith%201989%20BLEACH.pdf .
Additional references:
Want to join the conversation.
- Upvote Button navigates to signup page
- Downvote Button navigates to signup page
- Flag Button navigates to signup page


- Science Notes Posts
- Contact Science Notes
- Todd Helmenstine Biography
- Anne Helmenstine Biography
- Free Printable Periodic Tables (PDF and PNG)
- Periodic Table Wallpapers
- Interactive Periodic Table
- Periodic Table Posters
- Science Experiments for Kids
- How to Grow Crystals
- Chemistry Projects
- Fire and Flames Projects
- Holiday Science
- Chemistry Problems With Answers
- Physics Problems
- Unit Conversion Example Problems
- Chemistry Worksheets
- Biology Worksheets
- Periodic Table Worksheets
- Physical Science Worksheets
- Science Lab Worksheets
- My Amazon Books
Control Group Definition and Examples

The control group is the set of subjects that does not receive the treatment in a study. In other words, it is the group where the independent variable is held constant. This is important because the control group is a baseline for measuring the effects of a treatment in an experiment or study. A controlled experiment is one which includes one or more control groups.
- The experimental group experiences a treatment or change in the independent variable. In contrast, the independent variable is constant in the control group.
- A control group is important because it allows meaningful comparison. The researcher compares the experimental group to it to assess whether or not there is a relationship between the independent and dependent variable and the magnitude of the effect.
- There are different types of control groups. A controlled experiment has one more control group.
Control Group vs Experimental Group
The only difference between the control group and experimental group is that subjects in the experimental group receive the treatment being studied, while participants in the control group do not. Otherwise, all other variables between the two groups are the same.
Control Group vs Control Variable
A control group is not the same thing as a control variable. A control variable or controlled variable is any factor that is held constant during an experiment. Examples of common control variables include temperature, duration, and sample size. The control variables are the same for both the control and experimental groups.
Types of Control Groups
There are different types of control groups:
- Placebo group : A placebo group receives a placebo , which is a fake treatment that resembles the treatment in every respect except for the active ingredient. Both the placebo and treatment may contain inactive ingredients that produce side effects. Without a placebo group, these effects might be attributed to the treatment.
- Positive control group : A positive control group has conditions that guarantee a positive test result. The positive control group demonstrates an experiment is capable of producing a positive result. Positive controls help researchers identify problems with an experiment.
- Negative control group : A negative control group consists of subjects that are not exposed to a treatment. For example, in an experiment looking at the effect of fertilizer on plant growth, the negative control group receives no fertilizer.
- Natural control group : A natural control group usually is a set of subjects who naturally differ from the experimental group. For example, if you compare the effects of a treatment on women who have had children, the natural control group includes women who have not had children. Non-smokers are a natural control group in comparison to smokers.
- Randomized control group : The subjects in a randomized control group are randomly selected from a larger pool of subjects. Often, subjects are randomly assigned to either the control or experimental group. Randomization reduces bias in an experiment. There are different methods of randomly assigning test subjects.
Control Group Examples
Here are some examples of different control groups in action:
Negative Control and Placebo Group
For example, consider a study of a new cancer drug. The experimental group receives the drug. The placebo group receives a placebo, which contains the same ingredients as the drug formulation, minus the active ingredient. The negative control group receives no treatment. The reason for including the negative group is because the placebo group experiences some level of placebo effect, which is a response to experiencing some form of false treatment.
Positive and Negative Controls
For example, consider an experiment looking at whether a new drug kills bacteria. The experimental group exposes bacterial cultures to the drug. If the group survives, the drug is ineffective. If the group dies, the drug is effective.
The positive control group has a culture of bacteria that carry a drug resistance gene. If the bacteria survive drug exposure (as intended), then it shows the growth medium and conditions allow bacterial growth. If the positive control group dies, it indicates a problem with the experimental conditions. A negative control group of bacteria lacking drug resistance should die. If the negative control group survives, something is wrong with the experimental conditions.
- Bailey, R. A. (2008). Design of Comparative Experiments . Cambridge University Press. ISBN 978-0-521-68357-9.
- Chaplin, S. (2006). “The placebo response: an important part of treatment”. Prescriber . 17 (5): 16–22. doi: 10.1002/psb.344
- Hinkelmann, Klaus; Kempthorne, Oscar (2008). Design and Analysis of Experiments, Volume I: Introduction to Experimental Design (2nd ed.). Wiley. ISBN 978-0-471-72756-9.
- Pithon, M.M. (2013). “Importance of the control group in scientific research.” Dental Press J Orthod . 18 (6):13-14. doi: 10.1590/s2176-94512013000600003
- Stigler, Stephen M. (1992). “A Historical View of Statistical Concepts in Psychology and Educational Research”. American Journal of Education . 101 (1): 60–70. doi: 10.1086/444032
Related Posts
Controlled Experiment
Saul Mcleod, PhD
Editor-in-Chief for Simply Psychology
BSc (Hons) Psychology, MRes, PhD, University of Manchester
Saul Mcleod, PhD., is a qualified psychology teacher with over 18 years of experience in further and higher education. He has been published in peer-reviewed journals, including the Journal of Clinical Psychology.
Learn about our Editorial Process
Olivia Guy-Evans, MSc
Associate Editor for Simply Psychology
BSc (Hons) Psychology, MSc Psychology of Education
Olivia Guy-Evans is a writer and associate editor for Simply Psychology. She has previously worked in healthcare and educational sectors.
This is when a hypothesis is scientifically tested.
In a controlled experiment, an independent variable (the cause) is systematically manipulated, and the dependent variable (the effect) is measured; any extraneous variables are controlled.
The researcher can operationalize (i.e., define) the studied variables so they can be objectively measured. The quantitative data can be analyzed to see if there is a difference between the experimental and control groups.
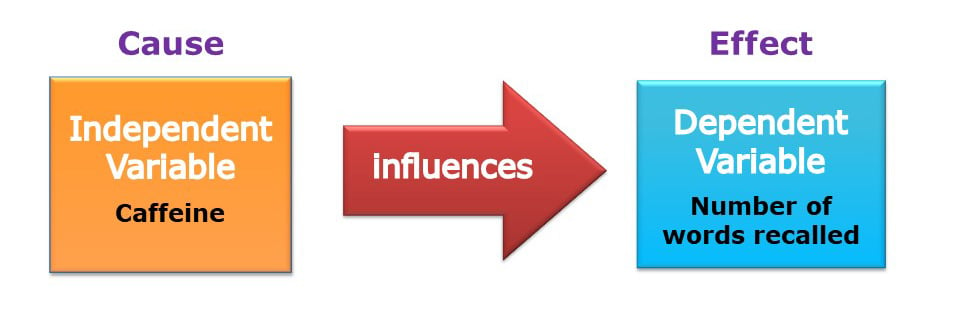
What is the control group?
In experiments scientists compare a control group and an experimental group that are identical in all respects, except for one difference – experimental manipulation.
Unlike the experimental group, the control group is not exposed to the independent variable under investigation and so provides a baseline against which any changes in the experimental group can be compared.
Since experimental manipulation is the only difference between the experimental and control groups, we can be sure that any differences between the two are due to experimental manipulation rather than chance.
Randomly allocating participants to independent variable groups means that all participants should have an equal chance of participating in each condition.
The principle of random allocation is to avoid bias in how the experiment is carried out and limit the effects of participant variables.
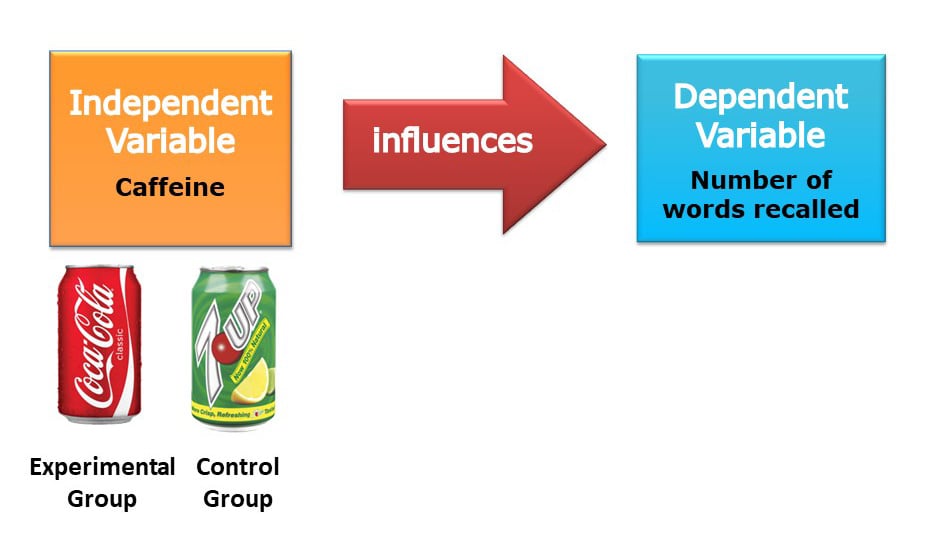
What are extraneous variables?
The researcher wants to ensure that the manipulation of the independent variable has changed the changes in the dependent variable.
Hence, all the other variables that could affect the dependent variable to change must be controlled. These other variables are called extraneous or confounding variables.
Extraneous variables should be controlled were possible, as they might be important enough to provide alternative explanations for the effects.
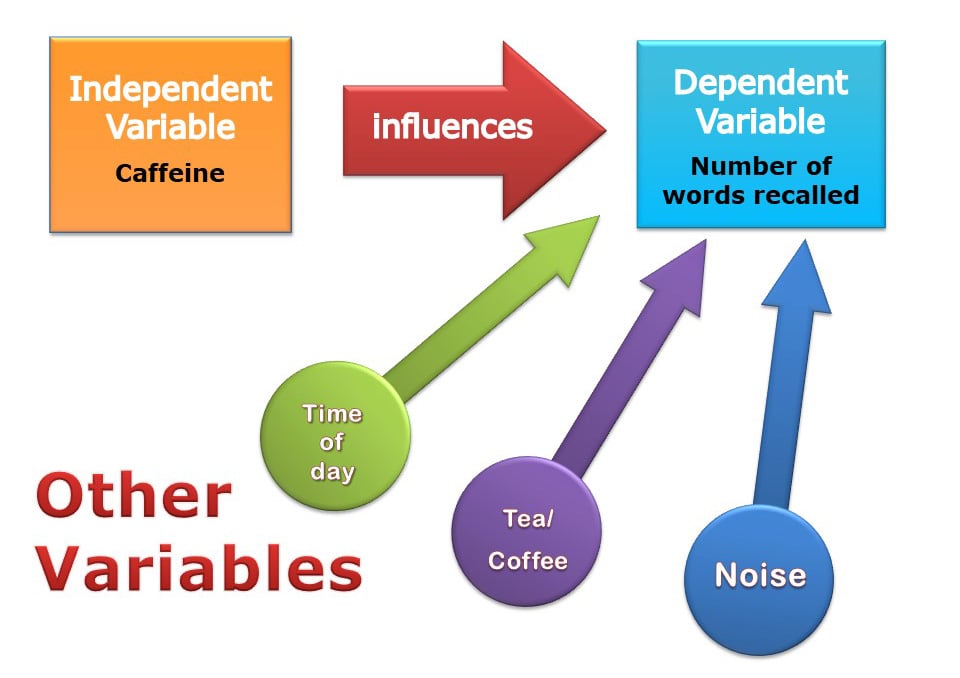
In practice, it would be difficult to control all the variables in a child’s educational achievement. For example, it would be difficult to control variables that have happened in the past.
A researcher can only control the current environment of participants, such as time of day and noise levels.
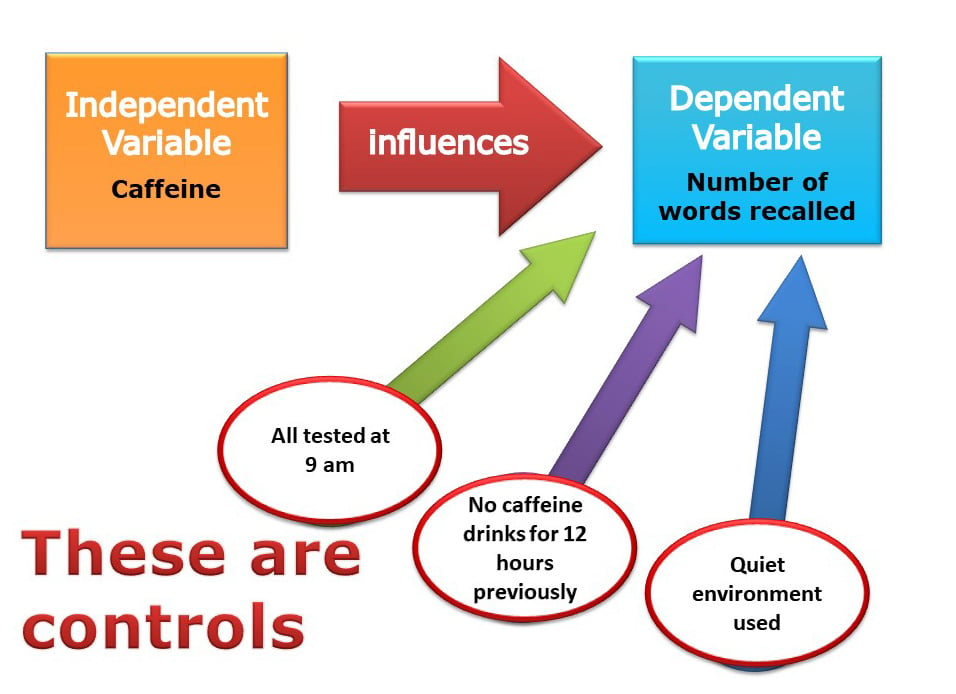
Why conduct controlled experiments?
Scientists use controlled experiments because they allow for precise control of extraneous and independent variables. This allows a cause-and-effect relationship to be established.
Controlled experiments also follow a standardized step-by-step procedure. This makes it easy for another researcher to replicate the study.
Key Terminology
Experimental group.
The group being treated or otherwise manipulated for the sake of the experiment.
Control Group
They receive no treatment and are used as a comparison group.
Ecological validity
The degree to which an investigation represents real-life experiences.
Experimenter effects
These are the ways that the experimenter can accidentally influence the participant through their appearance or behavior.
Demand characteristics
The clues in an experiment lead the participants to think they know what the researcher is looking for (e.g., the experimenter’s body language).
Independent variable (IV)
The variable the experimenter manipulates (i.e., changes) – is assumed to have a direct effect on the dependent variable.
Dependent variable (DV)
Variable the experimenter measures. This is the outcome (i.e., the result) of a study.
Extraneous variables (EV)
All variables that are not independent variables but could affect the results (DV) of the experiment. Extraneous variables should be controlled where possible.
Confounding variables
Variable(s) that have affected the results (DV), apart from the IV. A confounding variable could be an extraneous variable that has not been controlled.
Random Allocation
Randomly allocating participants to independent variable conditions means that all participants should have an equal chance of participating in each condition.
Order effects
Changes in participants’ performance due to their repeating the same or similar test more than once. Examples of order effects include:
(i) practice effect: an improvement in performance on a task due to repetition, for example, because of familiarity with the task;
(ii) fatigue effect: a decrease in performance of a task due to repetition, for example, because of boredom or tiredness.
What is the control in an experiment?
In an experiment , the control is a standard or baseline group not exposed to the experimental treatment or manipulation. It serves as a comparison group to the experimental group, which does receive the treatment or manipulation.
The control group helps to account for other variables that might influence the outcome, allowing researchers to attribute differences in results more confidently to the experimental treatment.
Establishing a cause-and-effect relationship between the manipulated variable (independent variable) and the outcome (dependent variable) is critical in establishing a cause-and-effect relationship between the manipulated variable.
What is the purpose of controlling the environment when testing a hypothesis?
Controlling the environment when testing a hypothesis aims to eliminate or minimize the influence of extraneous variables. These variables other than the independent variable might affect the dependent variable, potentially confounding the results.
By controlling the environment, researchers can ensure that any observed changes in the dependent variable are likely due to the manipulation of the independent variable, not other factors.
This enhances the experiment’s validity, allowing for more accurate conclusions about cause-and-effect relationships.
It also improves the experiment’s replicability, meaning other researchers can repeat the experiment under the same conditions to verify the results.
Why are hypotheses important to controlled experiments?
Hypotheses are crucial to controlled experiments because they provide a clear focus and direction for the research. A hypothesis is a testable prediction about the relationship between variables.
It guides the design of the experiment, including what variables to manipulate (independent variables) and what outcomes to measure (dependent variables).
The experiment is then conducted to test the validity of the hypothesis. If the results align with the hypothesis, they provide evidence supporting it.
The hypothesis may be revised or rejected if the results do not align. Thus, hypotheses are central to the scientific method, driving the iterative inquiry, experimentation, and knowledge advancement process.
What is the experimental method?
The experimental method is a systematic approach in scientific research where an independent variable is manipulated to observe its effect on a dependent variable, under controlled conditions.

Related Articles
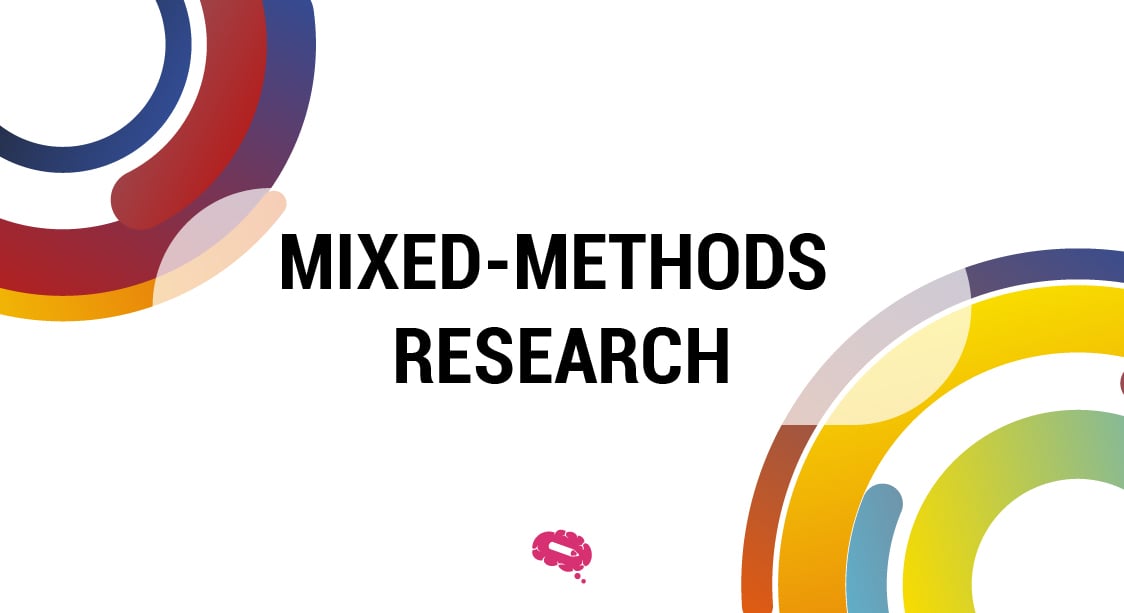
Research Methodology
Mixed Methods Research
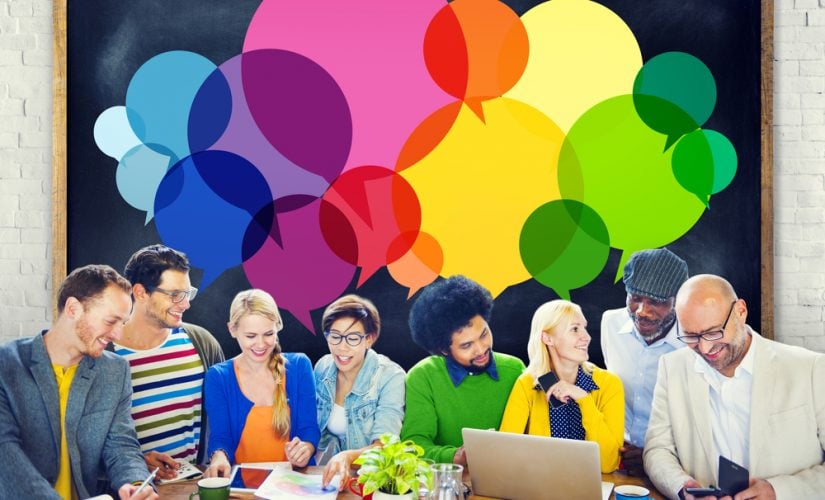
Conversation Analysis
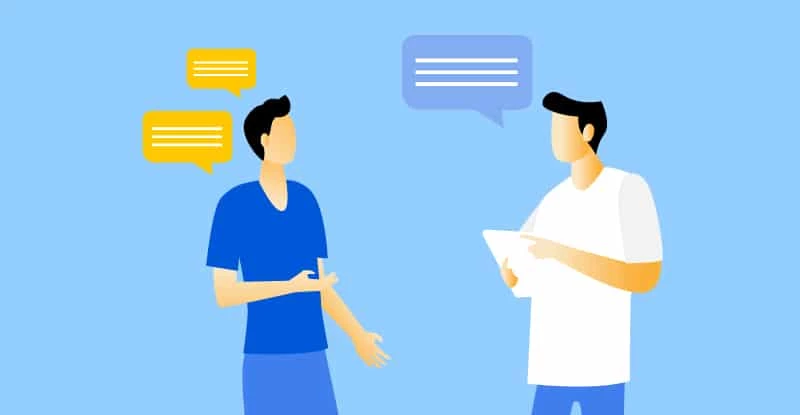
Discourse Analysis
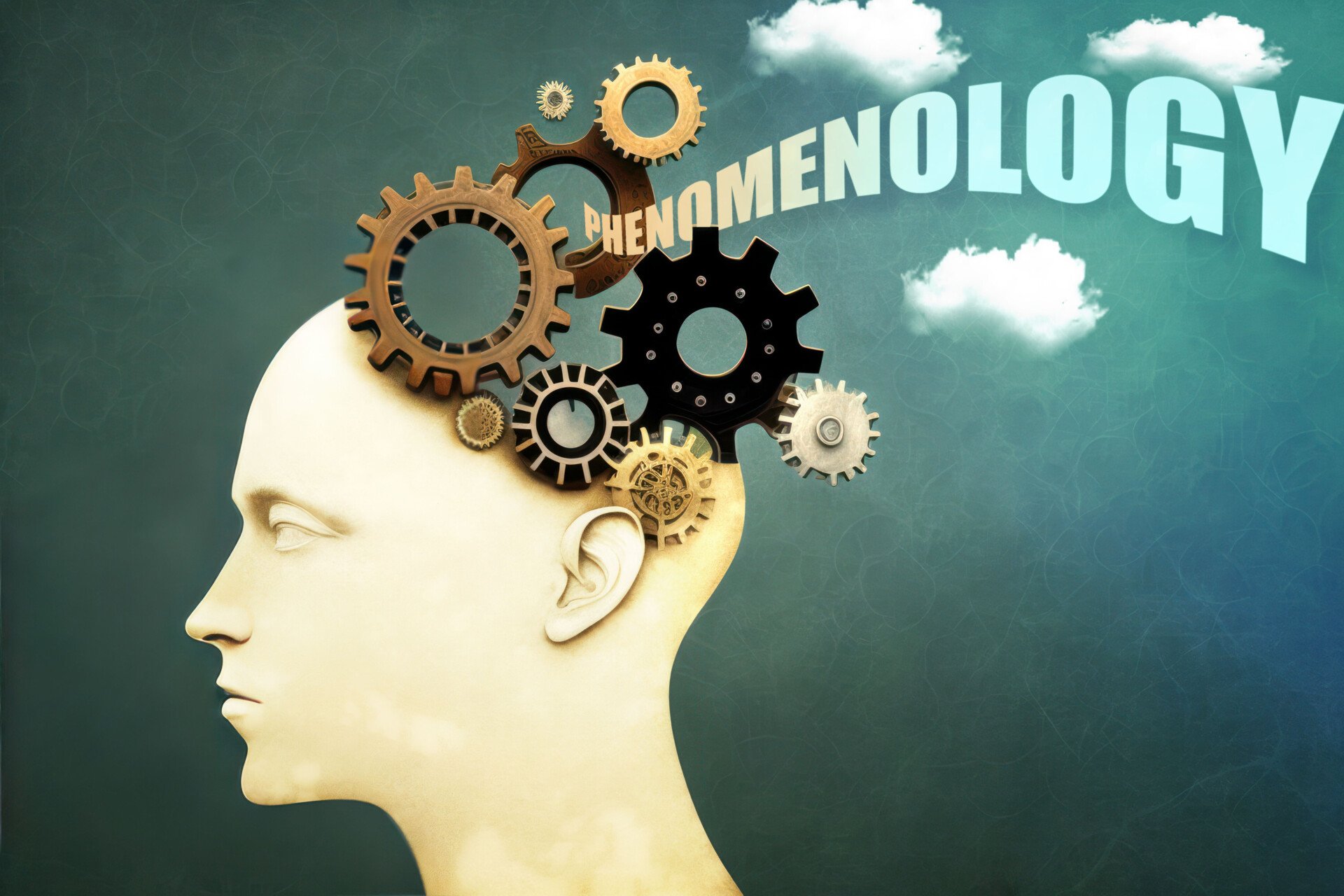
Phenomenology In Qualitative Research
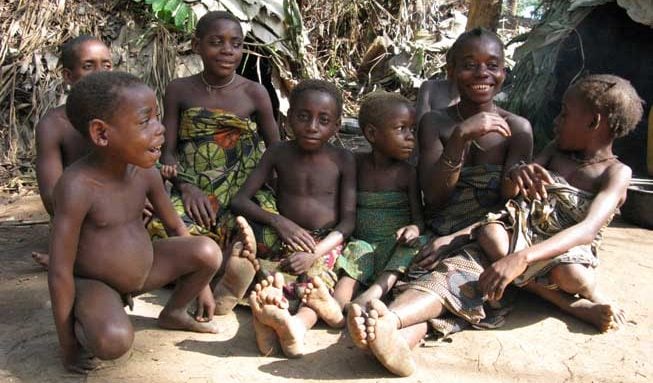
Ethnography In Qualitative Research
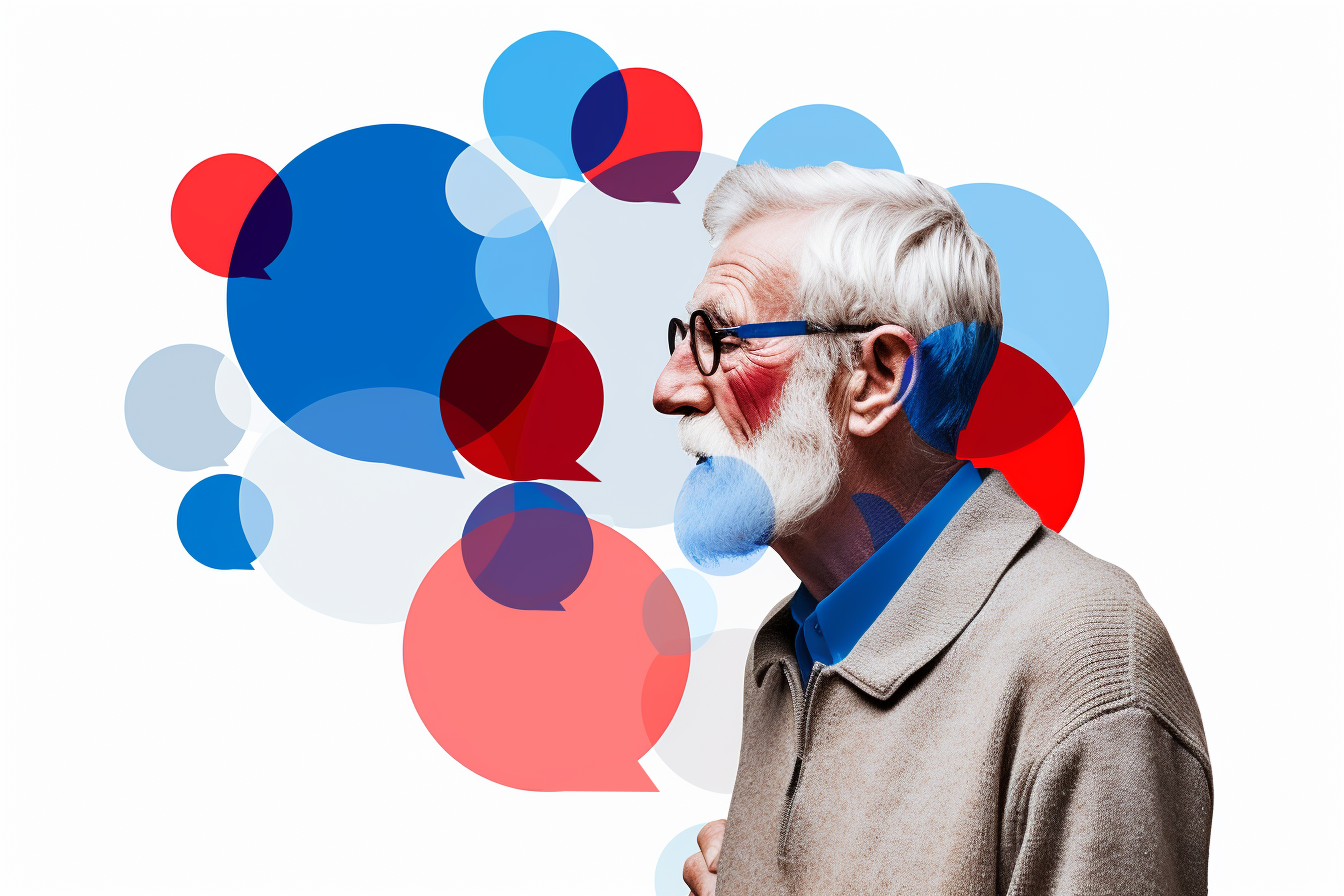
Narrative Analysis In Qualitative Research
- Skip to secondary menu
- Skip to main content
- Skip to primary sidebar
Statistics By Jim
Making statistics intuitive
Control Group in an Experiment
By Jim Frost 3 Comments
A control group in an experiment does not receive the treatment. Instead, it serves as a comparison group for the treatments. Researchers compare the results of a treatment group to the control group to determine the effect size, also known as the treatment effect.

Imagine that a treatment group receives a vaccine and it has an infection rate of 10%. By itself, you don’t know if that’s an improvement. However, if you also have an unvaccinated control group with an infection rate of 20%, you know the vaccine improved the outcome by 10 percentage points.
By serving as a basis for comparison, the control group reveals the treatment’s effect.
Related post : Effect Sizes in Statistics
Using Control Groups in Experiments
Most experiments include a control group and at least one treatment group. In an ideal experiment, the subjects in all groups start with the same overall characteristics except that those in the treatment groups receive a treatment. When the groups are otherwise equivalent before treatment begins, you can attribute differences after the experiment to the treatments.
Randomized controlled trials (RCTs) assign subjects to the treatment and control groups randomly. This process helps ensure the groups are comparable when treatment begins. Consequently, treatment effects are the most likely cause for differences between groups at the end of the study. Statisticians consider RCTs to be the gold standard. To learn more about this process, read my post, Random Assignment in Experiments .
Observational studies either can’t use randomized groups or don’t use them because they’re too costly or problematic. In these studies, the characteristics of the control group might be different from the treatment groups at the start of the study, making it difficult to estimate the treatment effect accurately at the end. Case-Control studies are a specific type of observational study that uses a control group.
For these types of studies, analytical methods and design choices, such as regression analysis and matching, can help statistically mitigate confounding variables. Matching involves selecting participants with similar characteristics. For each participant in the treatment group, the researchers find a subject with comparable traits to include in the control group. To learn more about this type of study and matching, read my post, Observational Studies Explained .
Control groups are key way to increase the internal validity of an experiment. To learn more, read my post about internal and external validity .
Randomized versus non-randomized control groups are just several of the different types you can have. We’ll look at more kinds later!
Related posts : When to Use Regression Analysis
Example of a Control Group
Suppose we want to determine whether regular vitamin consumption affects the risk of dying. Our experiment has the following two experimental groups:
- Control group : Does not consume vitamin supplements
- Treatment group : Regularly consumes vitamin supplements.
In this experiment, we randomly assign subjects to the two groups. Because we use random assignment, the two groups start with similar characteristics, including healthy habits, physical attributes, medical conditions, and other factors affecting the outcome. The intentional introduction of vitamin supplements in the treatment group is the only systematic difference between the groups.
After the experiment is complete, we compare the death risk between the treatment and control groups. Because the groups started roughly equal, we can reasonably attribute differences in death risk at the end of the study to vitamin consumption. By having the control group as the basis of comparison, the effect of vitamin consumption becomes clear!
Types of Control Groups
Researchers can use different types of control groups in their experiments. Earlier, you learned about the random versus non-random kinds, but there are other variations. You can use various types depending on your research goals, constraints, and ethical issues, among other things.
Negative Control Group
The group introduces a condition that the researchers expect won’t have an effect. This group typically receives no treatment. These experiments compare the effectiveness of the experimental treatment to no treatment. For example, in a vaccine study, a negative control group does not get the vaccine.
Positive Control Group
Positive control groups typically receive a standard treatment that science has already proven effective. These groups serve as a benchmark for the performance of a conventional treatment. In this vein, experiments with positive control groups compare the effectiveness of a new treatment to a standard one.
For example, an old blood pressure medicine can be the treatment in a positive control group, while the treatment group receives the new, experimental blood pressure medicine. The researchers want to determine whether the new treatment is better than the previous treatment.
In these studies, subjects can still take the standard medication for their condition, a potentially critical ethics issue.
Placebo Control Group
Placebo control groups introduce a treatment lookalike that will not affect the outcome. Standard examples of placebos are sugar pills and saline solution injections instead of genuine medicine. The key is that the placebo looks like the actual treatment. Researchers use this approach when the recipients’ belief that they’re receiving the treatment might influence their outcomes. By using placebos, the experiment controls for these psychological benefits. The researchers want to determine whether the treatment performs better than the placebo effect.
Learn more about the Placebo Effect .
Blinded Control Groups
If the subject’s awareness of their group assignment might affect their outcomes, the researchers can use a blinded experimental design that does not tell participants their group membership. Typically, blinded control groups will receive placebos, as described above. In a double-blinded control group, both subjects and researchers don’t know group assignments.
Waitlist Control Group
When there is a waitlist to receive a new treatment, those on the waitlist can serve as a control group until they receive treatment. This type of design avoids ethical concerns about withholding a better treatment until the study finishes. This design can be a variation of a positive control group because the subjects might be using conventional medicines while on the waitlist.
Historical Control Group
When historical data for a comparison group exists, it can serve as a control group for an experiment. The group doesn’t exist in the study, but the researchers compare the treatment group to the existing data. For example, the researchers might have infection rate data for unvaccinated individuals to compare to the infection rate among the vaccinated participants in their study. This approach allows everyone in the experiment to receive the new treatment. However, differences in place, time, and other circumstances can reduce the value of these comparisons. In other words, other factors might account for the apparent effects.
Share this:

Reader Interactions
December 19, 2021 at 9:17 am
Thank you very much Jim for your quick and comprehensive feedback. Extremely helpful!! Regards, Arthur
December 17, 2021 at 4:46 pm
Thank you very much Jim, very interesting article.
Can I select a control group at the end of intervention/experiment? Currently I am managing a project in rural Cambodia in five villages, however I did not select any comparison/control site at the beginning. Since I know there are other villages which have not been exposed to any type of intervention, can i select them as a control site during my end-line data collection or it will not be a legitimate control? Thank you very much, Arthur
December 18, 2021 at 1:51 am
You might be able to use that approach, but it’s not ideal. The ideal is to have control groups defined at the beginning of the study. You can use the untreated villages as a type of historical control groups that I talk about in this article. Or, if they’re awaiting to receive the intervention, it might be akin to a waitlist control group.
If you go that route, you’ll need to consider whether there was some systematic reason why these villages have not received any intervention. For example, are the villages in question more remote? And, if there is a systematic reason, would that affect your outcome variable? More generally, are they systematically different? How well do the untreated villages represent your target population?
If you had selected control villages at the beginning, you’d have been better able to ensure there weren’t any systematic differences between the villages receiving interventions and those that didn’t.
If the villages that didn’t receive any interventions are systematically different, you’ll need to incorporate that into your interpretation of the results. Are they different in ways that affect the outcomes you’re measuring? Can those differences account for the difference in outcomes between the treated and untreated villages? Hopefully, you’d be able to measure those differences between untreated/treated villages.
So, yes, you can use that approach. It’s not perfect and there will potentially be more things for you to consider and factor into your conclusions. Despite these drawbacks, it’s possible that using a pseudo control group like that is better than not doing that because at least you can make comparisons to something. Otherwise, you won’t know whether the outcomes in the intervention villages represent an improvement! Just be aware of the extra considerations!
Best of luck with your research!
Comments and Questions Cancel reply
Have a language expert improve your writing
Run a free plagiarism check in 10 minutes, automatically generate references for free.
- Knowledge Base
- Methodology
- Control Groups and Treatment Groups | Uses & Examples
Control Groups & Treatment Groups | Uses & Examples
Published on 6 May 2022 by Lauren Thomas . Revised on 13 April 2023.
In a scientific study, a control group is used to establish a cause-and-effect relationship by isolating the effect of an independent variable .
Researchers change the independent variable in the treatment group and keep it constant in the control group. Then they compare the results of these groups.
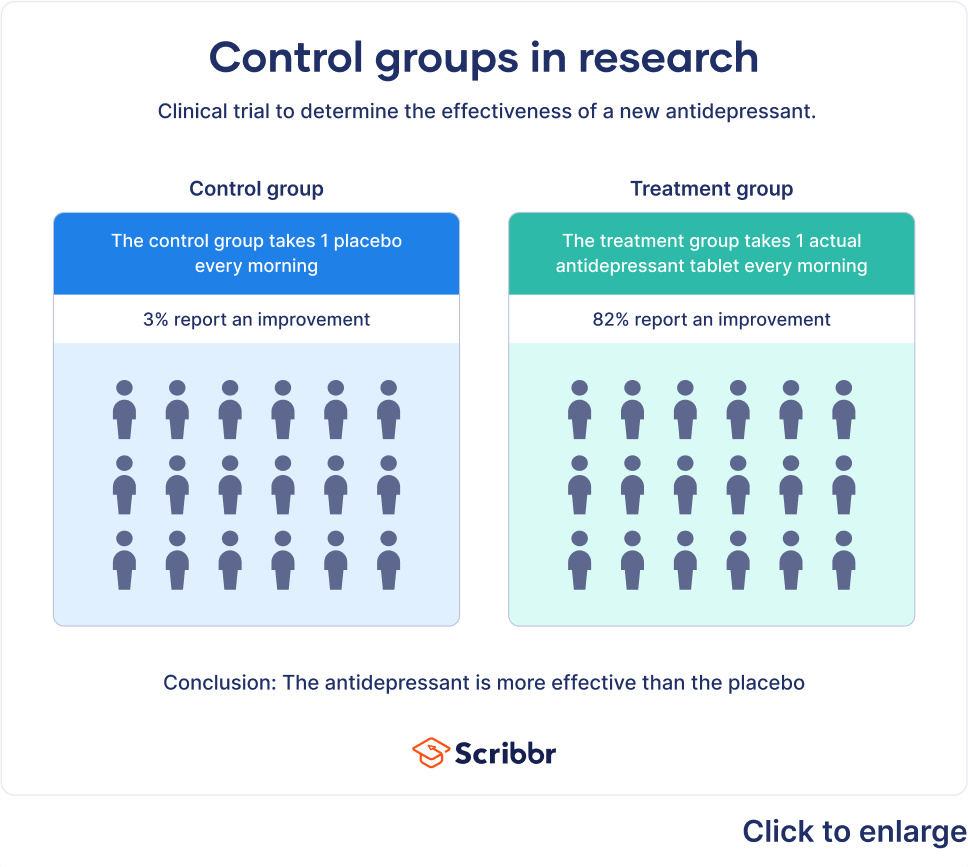
Using a control group means that any change in the dependent variable can be attributed to the independent variable.
Table of contents
Control groups in experiments, control groups in non-experimental research, importance of control groups, frequently asked questions about control groups.
Control groups are essential to experimental design . When researchers are interested in the impact of a new treatment, they randomly divide their study participants into at least two groups:
- The treatment group (also called the experimental group ) receives the treatment whose effect the researcher is interested in.
- The control group receives either no treatment, a standard treatment whose effect is already known, or a placebo (a fake treatment).
The treatment is any independent variable manipulated by the experimenters, and its exact form depends on the type of research being performed. In a medical trial, it might be a new drug or therapy. In public policy studies, it could be a new social policy that some receive and not others.
In a well-designed experiment, all variables apart from the treatment should be kept constant between the two groups. This means researchers can correctly measure the entire effect of the treatment without interference from confounding variables .
- You pay the students in the treatment group for achieving high grades.
- Students in the control group do not receive any money.
Studies can also include more than one treatment or control group. Researchers might want to examine the impact of multiple treatments at once, or compare a new treatment to several alternatives currently available.
- The treatment group gets the new pill.
- Control group 1 gets an identical-looking sugar pill (a placebo).
- Control group 2 gets a pill already approved to treat high blood pressure.
Since the only variable that differs between the three groups is the type of pill, any differences in average blood pressure between the three groups can be credited to the type of pill they received.
- The difference between the treatment group and control group 1 demonstrates the effectiveness of the pill as compared to no treatment.
- The difference between the treatment group and control group 2 shows whether the new pill improves on treatments already available on the market.
Prevent plagiarism, run a free check.
Although control groups are more common in experimental research, they can be used in other types of research too. Researchers generally rely on non-experimental control groups in two cases: quasi-experimental or matching design.
Control groups in quasi-experimental design
While true experiments rely on random assignment to the treatment or control groups, quasi-experimental design uses some criterion other than randomisation to assign people.
Often, these assignments are not controlled by researchers, but are pre-existing groups that have received different treatments. For example, researchers could study the effects of a new teaching method that was applied in some classes in a school but not others, or study the impact of a new policy that is implemented in one region but not in the neighbouring region.
In these cases, the classes that did not use the new teaching method, or the region that did not implement the new policy, is the control group.
Control groups in matching design
In correlational research , matching represents a potential alternate option when you cannot use either true or quasi-experimental designs.
In matching designs, the researcher matches individuals who received the ‘treatment’, or independent variable under study, to others who did not – the control group.
Each member of the treatment group thus has a counterpart in the control group identical in every way possible outside of the treatment. This ensures that the treatment is the only source of potential differences in outcomes between the two groups.
Control groups help ensure the internal validity of your research. You might see a difference over time in your dependent variable in your treatment group. However, without a control group, it is difficult to know whether the change has arisen from the treatment. It is possible that the change is due to some other variables.
If you use a control group that is identical in every other way to the treatment group, you know that the treatment – the only difference between the two groups – must be what has caused the change.
For example, people often recover from illnesses or injuries over time regardless of whether they’ve received effective treatment or not. Thus, without a control group, it’s difficult to determine whether improvements in medical conditions come from a treatment or just the natural progression of time.
Risks from invalid control groups
If your control group differs from the treatment group in ways that you haven’t accounted for, your results may reflect the interference of confounding variables instead of your independent variable.
Minimising this risk
A few methods can aid you in minimising the risk from invalid control groups.
- Ensure that all potential confounding variables are accounted for , preferably through an experimental design if possible, since it is difficult to control for all the possible confounders outside of an experimental environment.
- Use double-blinding . This will prevent the members of each group from modifying their behavior based on whether they were placed in the treatment or control group, which could then lead to biased outcomes.
- Randomly assign your subjects into control and treatment groups. This method will allow you to not only minimise the differences between the two groups on confounding variables that you can directly observe, but also those you cannot.
An experimental group, also known as a treatment group, receives the treatment whose effect researchers wish to study, whereas a control group does not. They should be identical in all other ways.
A true experiment (aka a controlled experiment) always includes at least one control group that doesn’t receive the experimental treatment.
However, some experiments use a within-subjects design to test treatments without a control group. In these designs, you usually compare one group’s outcomes before and after a treatment (instead of comparing outcomes between different groups).
For strong internal validity , it’s usually best to include a control group if possible. Without a control group, it’s harder to be certain that the outcome was caused by the experimental treatment and not by other variables.
In a controlled experiment , all extraneous variables are held constant so that they can’t influence the results. Controlled experiments require:
- A control group that receives a standard treatment, a fake treatment, or no treatment
- Random assignment of participants to ensure the groups are equivalent
Depending on your study topic, there are various other methods of controlling variables .
A confounding variable , also called a confounder or confounding factor, is a third variable in a study examining a potential cause-and-effect relationship.
A confounding variable is related to both the supposed cause and the supposed effect of the study. It can be difficult to separate the true effect of the independent variable from the effect of the confounding variable.
In your research design , it’s important to identify potential confounding variables and plan how you will reduce their impact.
Cite this Scribbr article
If you want to cite this source, you can copy and paste the citation or click the ‘Cite this Scribbr article’ button to automatically add the citation to our free Reference Generator.
Thomas, L. (2023, April 13). Control Groups & Treatment Groups | Uses & Examples. Scribbr. Retrieved 24 June 2024, from https://www.scribbr.co.uk/research-methods/control-groups/
Is this article helpful?
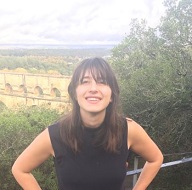
Lauren Thomas
Other students also liked, controlled experiments | methods & examples of control, a quick guide to experimental design | 5 steps & examples, correlation vs causation | differences, designs & examples.
Frequently asked questions
Do experiments always need a control group.
A true experiment (a.k.a. a controlled experiment) always includes at least one control group that doesn’t receive the experimental treatment.
However, some experiments use a within-subjects design to test treatments without a control group. In these designs, you usually compare one group’s outcomes before and after a treatment (instead of comparing outcomes between different groups).
For strong internal validity , it’s usually best to include a control group if possible. Without a control group, it’s harder to be certain that the outcome was caused by the experimental treatment and not by other variables.
Frequently asked questions: Methodology
Attrition refers to participants leaving a study. It always happens to some extent—for example, in randomized controlled trials for medical research.
Differential attrition occurs when attrition or dropout rates differ systematically between the intervention and the control group . As a result, the characteristics of the participants who drop out differ from the characteristics of those who stay in the study. Because of this, study results may be biased .
Action research is conducted in order to solve a particular issue immediately, while case studies are often conducted over a longer period of time and focus more on observing and analyzing a particular ongoing phenomenon.
Action research is focused on solving a problem or informing individual and community-based knowledge in a way that impacts teaching, learning, and other related processes. It is less focused on contributing theoretical input, instead producing actionable input.
Action research is particularly popular with educators as a form of systematic inquiry because it prioritizes reflection and bridges the gap between theory and practice. Educators are able to simultaneously investigate an issue as they solve it, and the method is very iterative and flexible.
A cycle of inquiry is another name for action research . It is usually visualized in a spiral shape following a series of steps, such as “planning → acting → observing → reflecting.”
To make quantitative observations , you need to use instruments that are capable of measuring the quantity you want to observe. For example, you might use a ruler to measure the length of an object or a thermometer to measure its temperature.
Criterion validity and construct validity are both types of measurement validity . In other words, they both show you how accurately a method measures something.
While construct validity is the degree to which a test or other measurement method measures what it claims to measure, criterion validity is the degree to which a test can predictively (in the future) or concurrently (in the present) measure something.
Construct validity is often considered the overarching type of measurement validity . You need to have face validity , content validity , and criterion validity in order to achieve construct validity.
Convergent validity and discriminant validity are both subtypes of construct validity . Together, they help you evaluate whether a test measures the concept it was designed to measure.
- Convergent validity indicates whether a test that is designed to measure a particular construct correlates with other tests that assess the same or similar construct.
- Discriminant validity indicates whether two tests that should not be highly related to each other are indeed not related. This type of validity is also called divergent validity .
You need to assess both in order to demonstrate construct validity. Neither one alone is sufficient for establishing construct validity.
- Discriminant validity indicates whether two tests that should not be highly related to each other are indeed not related
Content validity shows you how accurately a test or other measurement method taps into the various aspects of the specific construct you are researching.
In other words, it helps you answer the question: “does the test measure all aspects of the construct I want to measure?” If it does, then the test has high content validity.
The higher the content validity, the more accurate the measurement of the construct.
If the test fails to include parts of the construct, or irrelevant parts are included, the validity of the instrument is threatened, which brings your results into question.
Face validity and content validity are similar in that they both evaluate how suitable the content of a test is. The difference is that face validity is subjective, and assesses content at surface level.
When a test has strong face validity, anyone would agree that the test’s questions appear to measure what they are intended to measure.
For example, looking at a 4th grade math test consisting of problems in which students have to add and multiply, most people would agree that it has strong face validity (i.e., it looks like a math test).
On the other hand, content validity evaluates how well a test represents all the aspects of a topic. Assessing content validity is more systematic and relies on expert evaluation. of each question, analyzing whether each one covers the aspects that the test was designed to cover.
A 4th grade math test would have high content validity if it covered all the skills taught in that grade. Experts(in this case, math teachers), would have to evaluate the content validity by comparing the test to the learning objectives.
Snowball sampling is a non-probability sampling method . Unlike probability sampling (which involves some form of random selection ), the initial individuals selected to be studied are the ones who recruit new participants.
Because not every member of the target population has an equal chance of being recruited into the sample, selection in snowball sampling is non-random.
Snowball sampling is a non-probability sampling method , where there is not an equal chance for every member of the population to be included in the sample .
This means that you cannot use inferential statistics and make generalizations —often the goal of quantitative research . As such, a snowball sample is not representative of the target population and is usually a better fit for qualitative research .
Snowball sampling relies on the use of referrals. Here, the researcher recruits one or more initial participants, who then recruit the next ones.
Participants share similar characteristics and/or know each other. Because of this, not every member of the population has an equal chance of being included in the sample, giving rise to sampling bias .
Snowball sampling is best used in the following cases:
- If there is no sampling frame available (e.g., people with a rare disease)
- If the population of interest is hard to access or locate (e.g., people experiencing homelessness)
- If the research focuses on a sensitive topic (e.g., extramarital affairs)
The reproducibility and replicability of a study can be ensured by writing a transparent, detailed method section and using clear, unambiguous language.
Reproducibility and replicability are related terms.
- Reproducing research entails reanalyzing the existing data in the same manner.
- Replicating (or repeating ) the research entails reconducting the entire analysis, including the collection of new data .
- A successful reproduction shows that the data analyses were conducted in a fair and honest manner.
- A successful replication shows that the reliability of the results is high.
Stratified sampling and quota sampling both involve dividing the population into subgroups and selecting units from each subgroup. The purpose in both cases is to select a representative sample and/or to allow comparisons between subgroups.
The main difference is that in stratified sampling, you draw a random sample from each subgroup ( probability sampling ). In quota sampling you select a predetermined number or proportion of units, in a non-random manner ( non-probability sampling ).
Purposive and convenience sampling are both sampling methods that are typically used in qualitative data collection.
A convenience sample is drawn from a source that is conveniently accessible to the researcher. Convenience sampling does not distinguish characteristics among the participants. On the other hand, purposive sampling focuses on selecting participants possessing characteristics associated with the research study.
The findings of studies based on either convenience or purposive sampling can only be generalized to the (sub)population from which the sample is drawn, and not to the entire population.
Random sampling or probability sampling is based on random selection. This means that each unit has an equal chance (i.e., equal probability) of being included in the sample.
On the other hand, convenience sampling involves stopping people at random, which means that not everyone has an equal chance of being selected depending on the place, time, or day you are collecting your data.
Convenience sampling and quota sampling are both non-probability sampling methods. They both use non-random criteria like availability, geographical proximity, or expert knowledge to recruit study participants.
However, in convenience sampling, you continue to sample units or cases until you reach the required sample size.
In quota sampling, you first need to divide your population of interest into subgroups (strata) and estimate their proportions (quota) in the population. Then you can start your data collection, using convenience sampling to recruit participants, until the proportions in each subgroup coincide with the estimated proportions in the population.
A sampling frame is a list of every member in the entire population . It is important that the sampling frame is as complete as possible, so that your sample accurately reflects your population.
Stratified and cluster sampling may look similar, but bear in mind that groups created in cluster sampling are heterogeneous , so the individual characteristics in the cluster vary. In contrast, groups created in stratified sampling are homogeneous , as units share characteristics.
Relatedly, in cluster sampling you randomly select entire groups and include all units of each group in your sample. However, in stratified sampling, you select some units of all groups and include them in your sample. In this way, both methods can ensure that your sample is representative of the target population .
A systematic review is secondary research because it uses existing research. You don’t collect new data yourself.
The key difference between observational studies and experimental designs is that a well-done observational study does not influence the responses of participants, while experiments do have some sort of treatment condition applied to at least some participants by random assignment .
An observational study is a great choice for you if your research question is based purely on observations. If there are ethical, logistical, or practical concerns that prevent you from conducting a traditional experiment , an observational study may be a good choice. In an observational study, there is no interference or manipulation of the research subjects, as well as no control or treatment groups .
It’s often best to ask a variety of people to review your measurements. You can ask experts, such as other researchers, or laypeople, such as potential participants, to judge the face validity of tests.
While experts have a deep understanding of research methods , the people you’re studying can provide you with valuable insights you may have missed otherwise.
Face validity is important because it’s a simple first step to measuring the overall validity of a test or technique. It’s a relatively intuitive, quick, and easy way to start checking whether a new measure seems useful at first glance.
Good face validity means that anyone who reviews your measure says that it seems to be measuring what it’s supposed to. With poor face validity, someone reviewing your measure may be left confused about what you’re measuring and why you’re using this method.
Face validity is about whether a test appears to measure what it’s supposed to measure. This type of validity is concerned with whether a measure seems relevant and appropriate for what it’s assessing only on the surface.
Statistical analyses are often applied to test validity with data from your measures. You test convergent validity and discriminant validity with correlations to see if results from your test are positively or negatively related to those of other established tests.
You can also use regression analyses to assess whether your measure is actually predictive of outcomes that you expect it to predict theoretically. A regression analysis that supports your expectations strengthens your claim of construct validity .
When designing or evaluating a measure, construct validity helps you ensure you’re actually measuring the construct you’re interested in. If you don’t have construct validity, you may inadvertently measure unrelated or distinct constructs and lose precision in your research.
Construct validity is often considered the overarching type of measurement validity , because it covers all of the other types. You need to have face validity , content validity , and criterion validity to achieve construct validity.
Construct validity is about how well a test measures the concept it was designed to evaluate. It’s one of four types of measurement validity , which includes construct validity, face validity , and criterion validity.
There are two subtypes of construct validity.
- Convergent validity : The extent to which your measure corresponds to measures of related constructs
- Discriminant validity : The extent to which your measure is unrelated or negatively related to measures of distinct constructs
Naturalistic observation is a valuable tool because of its flexibility, external validity , and suitability for topics that can’t be studied in a lab setting.
The downsides of naturalistic observation include its lack of scientific control , ethical considerations , and potential for bias from observers and subjects.
Naturalistic observation is a qualitative research method where you record the behaviors of your research subjects in real world settings. You avoid interfering or influencing anything in a naturalistic observation.
You can think of naturalistic observation as “people watching” with a purpose.
A dependent variable is what changes as a result of the independent variable manipulation in experiments . It’s what you’re interested in measuring, and it “depends” on your independent variable.
In statistics, dependent variables are also called:
- Response variables (they respond to a change in another variable)
- Outcome variables (they represent the outcome you want to measure)
- Left-hand-side variables (they appear on the left-hand side of a regression equation)
An independent variable is the variable you manipulate, control, or vary in an experimental study to explore its effects. It’s called “independent” because it’s not influenced by any other variables in the study.
Independent variables are also called:
- Explanatory variables (they explain an event or outcome)
- Predictor variables (they can be used to predict the value of a dependent variable)
- Right-hand-side variables (they appear on the right-hand side of a regression equation).
As a rule of thumb, questions related to thoughts, beliefs, and feelings work well in focus groups. Take your time formulating strong questions, paying special attention to phrasing. Be careful to avoid leading questions , which can bias your responses.
Overall, your focus group questions should be:
- Open-ended and flexible
- Impossible to answer with “yes” or “no” (questions that start with “why” or “how” are often best)
- Unambiguous, getting straight to the point while still stimulating discussion
- Unbiased and neutral
A structured interview is a data collection method that relies on asking questions in a set order to collect data on a topic. They are often quantitative in nature. Structured interviews are best used when:
- You already have a very clear understanding of your topic. Perhaps significant research has already been conducted, or you have done some prior research yourself, but you already possess a baseline for designing strong structured questions.
- You are constrained in terms of time or resources and need to analyze your data quickly and efficiently.
- Your research question depends on strong parity between participants, with environmental conditions held constant.
More flexible interview options include semi-structured interviews , unstructured interviews , and focus groups .
Social desirability bias is the tendency for interview participants to give responses that will be viewed favorably by the interviewer or other participants. It occurs in all types of interviews and surveys , but is most common in semi-structured interviews , unstructured interviews , and focus groups .
Social desirability bias can be mitigated by ensuring participants feel at ease and comfortable sharing their views. Make sure to pay attention to your own body language and any physical or verbal cues, such as nodding or widening your eyes.
This type of bias can also occur in observations if the participants know they’re being observed. They might alter their behavior accordingly.
The interviewer effect is a type of bias that emerges when a characteristic of an interviewer (race, age, gender identity, etc.) influences the responses given by the interviewee.
There is a risk of an interviewer effect in all types of interviews , but it can be mitigated by writing really high-quality interview questions.
A semi-structured interview is a blend of structured and unstructured types of interviews. Semi-structured interviews are best used when:
- You have prior interview experience. Spontaneous questions are deceptively challenging, and it’s easy to accidentally ask a leading question or make a participant uncomfortable.
- Your research question is exploratory in nature. Participant answers can guide future research questions and help you develop a more robust knowledge base for future research.
An unstructured interview is the most flexible type of interview, but it is not always the best fit for your research topic.
Unstructured interviews are best used when:
- You are an experienced interviewer and have a very strong background in your research topic, since it is challenging to ask spontaneous, colloquial questions.
- Your research question is exploratory in nature. While you may have developed hypotheses, you are open to discovering new or shifting viewpoints through the interview process.
- You are seeking descriptive data, and are ready to ask questions that will deepen and contextualize your initial thoughts and hypotheses.
- Your research depends on forming connections with your participants and making them feel comfortable revealing deeper emotions, lived experiences, or thoughts.
The four most common types of interviews are:
- Structured interviews : The questions are predetermined in both topic and order.
- Semi-structured interviews : A few questions are predetermined, but other questions aren’t planned.
- Unstructured interviews : None of the questions are predetermined.
- Focus group interviews : The questions are presented to a group instead of one individual.
Deductive reasoning is commonly used in scientific research, and it’s especially associated with quantitative research .
In research, you might have come across something called the hypothetico-deductive method . It’s the scientific method of testing hypotheses to check whether your predictions are substantiated by real-world data.
Deductive reasoning is a logical approach where you progress from general ideas to specific conclusions. It’s often contrasted with inductive reasoning , where you start with specific observations and form general conclusions.
Deductive reasoning is also called deductive logic.
There are many different types of inductive reasoning that people use formally or informally.
Here are a few common types:
- Inductive generalization : You use observations about a sample to come to a conclusion about the population it came from.
- Statistical generalization: You use specific numbers about samples to make statements about populations.
- Causal reasoning: You make cause-and-effect links between different things.
- Sign reasoning: You make a conclusion about a correlational relationship between different things.
- Analogical reasoning: You make a conclusion about something based on its similarities to something else.
Inductive reasoning is a bottom-up approach, while deductive reasoning is top-down.
Inductive reasoning takes you from the specific to the general, while in deductive reasoning, you make inferences by going from general premises to specific conclusions.
In inductive research , you start by making observations or gathering data. Then, you take a broad scan of your data and search for patterns. Finally, you make general conclusions that you might incorporate into theories.
Inductive reasoning is a method of drawing conclusions by going from the specific to the general. It’s usually contrasted with deductive reasoning, where you proceed from general information to specific conclusions.
Inductive reasoning is also called inductive logic or bottom-up reasoning.
A hypothesis states your predictions about what your research will find. It is a tentative answer to your research question that has not yet been tested. For some research projects, you might have to write several hypotheses that address different aspects of your research question.
A hypothesis is not just a guess — it should be based on existing theories and knowledge. It also has to be testable, which means you can support or refute it through scientific research methods (such as experiments, observations and statistical analysis of data).
Triangulation can help:
- Reduce research bias that comes from using a single method, theory, or investigator
- Enhance validity by approaching the same topic with different tools
- Establish credibility by giving you a complete picture of the research problem
But triangulation can also pose problems:
- It’s time-consuming and labor-intensive, often involving an interdisciplinary team.
- Your results may be inconsistent or even contradictory.
There are four main types of triangulation :
- Data triangulation : Using data from different times, spaces, and people
- Investigator triangulation : Involving multiple researchers in collecting or analyzing data
- Theory triangulation : Using varying theoretical perspectives in your research
- Methodological triangulation : Using different methodologies to approach the same topic
Many academic fields use peer review , largely to determine whether a manuscript is suitable for publication. Peer review enhances the credibility of the published manuscript.
However, peer review is also common in non-academic settings. The United Nations, the European Union, and many individual nations use peer review to evaluate grant applications. It is also widely used in medical and health-related fields as a teaching or quality-of-care measure.
Peer assessment is often used in the classroom as a pedagogical tool. Both receiving feedback and providing it are thought to enhance the learning process, helping students think critically and collaboratively.
Peer review can stop obviously problematic, falsified, or otherwise untrustworthy research from being published. It also represents an excellent opportunity to get feedback from renowned experts in your field. It acts as a first defense, helping you ensure your argument is clear and that there are no gaps, vague terms, or unanswered questions for readers who weren’t involved in the research process.
Peer-reviewed articles are considered a highly credible source due to this stringent process they go through before publication.
In general, the peer review process follows the following steps:
- First, the author submits the manuscript to the editor.
- Reject the manuscript and send it back to author, or
- Send it onward to the selected peer reviewer(s)
- Next, the peer review process occurs. The reviewer provides feedback, addressing any major or minor issues with the manuscript, and gives their advice regarding what edits should be made.
- Lastly, the edited manuscript is sent back to the author. They input the edits, and resubmit it to the editor for publication.
Exploratory research is often used when the issue you’re studying is new or when the data collection process is challenging for some reason.
You can use exploratory research if you have a general idea or a specific question that you want to study but there is no preexisting knowledge or paradigm with which to study it.
Exploratory research is a methodology approach that explores research questions that have not previously been studied in depth. It is often used when the issue you’re studying is new, or the data collection process is challenging in some way.
Explanatory research is used to investigate how or why a phenomenon occurs. Therefore, this type of research is often one of the first stages in the research process , serving as a jumping-off point for future research.
Exploratory research aims to explore the main aspects of an under-researched problem, while explanatory research aims to explain the causes and consequences of a well-defined problem.
Explanatory research is a research method used to investigate how or why something occurs when only a small amount of information is available pertaining to that topic. It can help you increase your understanding of a given topic.
Clean data are valid, accurate, complete, consistent, unique, and uniform. Dirty data include inconsistencies and errors.
Dirty data can come from any part of the research process, including poor research design , inappropriate measurement materials, or flawed data entry.
Data cleaning takes place between data collection and data analyses. But you can use some methods even before collecting data.
For clean data, you should start by designing measures that collect valid data. Data validation at the time of data entry or collection helps you minimize the amount of data cleaning you’ll need to do.
After data collection, you can use data standardization and data transformation to clean your data. You’ll also deal with any missing values, outliers, and duplicate values.
Every dataset requires different techniques to clean dirty data , but you need to address these issues in a systematic way. You focus on finding and resolving data points that don’t agree or fit with the rest of your dataset.
These data might be missing values, outliers, duplicate values, incorrectly formatted, or irrelevant. You’ll start with screening and diagnosing your data. Then, you’ll often standardize and accept or remove data to make your dataset consistent and valid.
Data cleaning is necessary for valid and appropriate analyses. Dirty data contain inconsistencies or errors , but cleaning your data helps you minimize or resolve these.
Without data cleaning, you could end up with a Type I or II error in your conclusion. These types of erroneous conclusions can be practically significant with important consequences, because they lead to misplaced investments or missed opportunities.
Data cleaning involves spotting and resolving potential data inconsistencies or errors to improve your data quality. An error is any value (e.g., recorded weight) that doesn’t reflect the true value (e.g., actual weight) of something that’s being measured.
In this process, you review, analyze, detect, modify, or remove “dirty” data to make your dataset “clean.” Data cleaning is also called data cleansing or data scrubbing.
Research misconduct means making up or falsifying data, manipulating data analyses, or misrepresenting results in research reports. It’s a form of academic fraud.
These actions are committed intentionally and can have serious consequences; research misconduct is not a simple mistake or a point of disagreement but a serious ethical failure.
Anonymity means you don’t know who the participants are, while confidentiality means you know who they are but remove identifying information from your research report. Both are important ethical considerations .
You can only guarantee anonymity by not collecting any personally identifying information—for example, names, phone numbers, email addresses, IP addresses, physical characteristics, photos, or videos.
You can keep data confidential by using aggregate information in your research report, so that you only refer to groups of participants rather than individuals.
Research ethics matter for scientific integrity, human rights and dignity, and collaboration between science and society. These principles make sure that participation in studies is voluntary, informed, and safe.
Ethical considerations in research are a set of principles that guide your research designs and practices. These principles include voluntary participation, informed consent, anonymity, confidentiality, potential for harm, and results communication.
Scientists and researchers must always adhere to a certain code of conduct when collecting data from others .
These considerations protect the rights of research participants, enhance research validity , and maintain scientific integrity.
In multistage sampling , you can use probability or non-probability sampling methods .
For a probability sample, you have to conduct probability sampling at every stage.
You can mix it up by using simple random sampling , systematic sampling , or stratified sampling to select units at different stages, depending on what is applicable and relevant to your study.
Multistage sampling can simplify data collection when you have large, geographically spread samples, and you can obtain a probability sample without a complete sampling frame.
But multistage sampling may not lead to a representative sample, and larger samples are needed for multistage samples to achieve the statistical properties of simple random samples .
These are four of the most common mixed methods designs :
- Convergent parallel: Quantitative and qualitative data are collected at the same time and analyzed separately. After both analyses are complete, compare your results to draw overall conclusions.
- Embedded: Quantitative and qualitative data are collected at the same time, but within a larger quantitative or qualitative design. One type of data is secondary to the other.
- Explanatory sequential: Quantitative data is collected and analyzed first, followed by qualitative data. You can use this design if you think your qualitative data will explain and contextualize your quantitative findings.
- Exploratory sequential: Qualitative data is collected and analyzed first, followed by quantitative data. You can use this design if you think the quantitative data will confirm or validate your qualitative findings.
Triangulation in research means using multiple datasets, methods, theories and/or investigators to address a research question. It’s a research strategy that can help you enhance the validity and credibility of your findings.
Triangulation is mainly used in qualitative research , but it’s also commonly applied in quantitative research . Mixed methods research always uses triangulation.
In multistage sampling , or multistage cluster sampling, you draw a sample from a population using smaller and smaller groups at each stage.
This method is often used to collect data from a large, geographically spread group of people in national surveys, for example. You take advantage of hierarchical groupings (e.g., from state to city to neighborhood) to create a sample that’s less expensive and time-consuming to collect data from.
No, the steepness or slope of the line isn’t related to the correlation coefficient value. The correlation coefficient only tells you how closely your data fit on a line, so two datasets with the same correlation coefficient can have very different slopes.
To find the slope of the line, you’ll need to perform a regression analysis .
Correlation coefficients always range between -1 and 1.
The sign of the coefficient tells you the direction of the relationship: a positive value means the variables change together in the same direction, while a negative value means they change together in opposite directions.
The absolute value of a number is equal to the number without its sign. The absolute value of a correlation coefficient tells you the magnitude of the correlation: the greater the absolute value, the stronger the correlation.
These are the assumptions your data must meet if you want to use Pearson’s r :
- Both variables are on an interval or ratio level of measurement
- Data from both variables follow normal distributions
- Your data have no outliers
- Your data is from a random or representative sample
- You expect a linear relationship between the two variables
Quantitative research designs can be divided into two main categories:
- Correlational and descriptive designs are used to investigate characteristics, averages, trends, and associations between variables.
- Experimental and quasi-experimental designs are used to test causal relationships .
Qualitative research designs tend to be more flexible. Common types of qualitative design include case study , ethnography , and grounded theory designs.
A well-planned research design helps ensure that your methods match your research aims, that you collect high-quality data, and that you use the right kind of analysis to answer your questions, utilizing credible sources . This allows you to draw valid , trustworthy conclusions.
The priorities of a research design can vary depending on the field, but you usually have to specify:
- Your research questions and/or hypotheses
- Your overall approach (e.g., qualitative or quantitative )
- The type of design you’re using (e.g., a survey , experiment , or case study )
- Your sampling methods or criteria for selecting subjects
- Your data collection methods (e.g., questionnaires , observations)
- Your data collection procedures (e.g., operationalization , timing and data management)
- Your data analysis methods (e.g., statistical tests or thematic analysis )
A research design is a strategy for answering your research question . It defines your overall approach and determines how you will collect and analyze data.
Questionnaires can be self-administered or researcher-administered.
Self-administered questionnaires can be delivered online or in paper-and-pen formats, in person or through mail. All questions are standardized so that all respondents receive the same questions with identical wording.
Researcher-administered questionnaires are interviews that take place by phone, in-person, or online between researchers and respondents. You can gain deeper insights by clarifying questions for respondents or asking follow-up questions.
You can organize the questions logically, with a clear progression from simple to complex, or randomly between respondents. A logical flow helps respondents process the questionnaire easier and quicker, but it may lead to bias. Randomization can minimize the bias from order effects.
Closed-ended, or restricted-choice, questions offer respondents a fixed set of choices to select from. These questions are easier to answer quickly.
Open-ended or long-form questions allow respondents to answer in their own words. Because there are no restrictions on their choices, respondents can answer in ways that researchers may not have otherwise considered.
A questionnaire is a data collection tool or instrument, while a survey is an overarching research method that involves collecting and analyzing data from people using questionnaires.
The third variable and directionality problems are two main reasons why correlation isn’t causation .
The third variable problem means that a confounding variable affects both variables to make them seem causally related when they are not.
The directionality problem is when two variables correlate and might actually have a causal relationship, but it’s impossible to conclude which variable causes changes in the other.
Correlation describes an association between variables : when one variable changes, so does the other. A correlation is a statistical indicator of the relationship between variables.
Causation means that changes in one variable brings about changes in the other (i.e., there is a cause-and-effect relationship between variables). The two variables are correlated with each other, and there’s also a causal link between them.
While causation and correlation can exist simultaneously, correlation does not imply causation. In other words, correlation is simply a relationship where A relates to B—but A doesn’t necessarily cause B to happen (or vice versa). Mistaking correlation for causation is a common error and can lead to false cause fallacy .
Controlled experiments establish causality, whereas correlational studies only show associations between variables.
- In an experimental design , you manipulate an independent variable and measure its effect on a dependent variable. Other variables are controlled so they can’t impact the results.
- In a correlational design , you measure variables without manipulating any of them. You can test whether your variables change together, but you can’t be sure that one variable caused a change in another.
In general, correlational research is high in external validity while experimental research is high in internal validity .
A correlation is usually tested for two variables at a time, but you can test correlations between three or more variables.
A correlation coefficient is a single number that describes the strength and direction of the relationship between your variables.
Different types of correlation coefficients might be appropriate for your data based on their levels of measurement and distributions . The Pearson product-moment correlation coefficient (Pearson’s r ) is commonly used to assess a linear relationship between two quantitative variables.
A correlational research design investigates relationships between two variables (or more) without the researcher controlling or manipulating any of them. It’s a non-experimental type of quantitative research .
A correlation reflects the strength and/or direction of the association between two or more variables.
- A positive correlation means that both variables change in the same direction.
- A negative correlation means that the variables change in opposite directions.
- A zero correlation means there’s no relationship between the variables.
Random error is almost always present in scientific studies, even in highly controlled settings. While you can’t eradicate it completely, you can reduce random error by taking repeated measurements, using a large sample, and controlling extraneous variables .
You can avoid systematic error through careful design of your sampling , data collection , and analysis procedures. For example, use triangulation to measure your variables using multiple methods; regularly calibrate instruments or procedures; use random sampling and random assignment ; and apply masking (blinding) where possible.
Systematic error is generally a bigger problem in research.
With random error, multiple measurements will tend to cluster around the true value. When you’re collecting data from a large sample , the errors in different directions will cancel each other out.
Systematic errors are much more problematic because they can skew your data away from the true value. This can lead you to false conclusions ( Type I and II errors ) about the relationship between the variables you’re studying.
Random and systematic error are two types of measurement error.
Random error is a chance difference between the observed and true values of something (e.g., a researcher misreading a weighing scale records an incorrect measurement).
Systematic error is a consistent or proportional difference between the observed and true values of something (e.g., a miscalibrated scale consistently records weights as higher than they actually are).
On graphs, the explanatory variable is conventionally placed on the x-axis, while the response variable is placed on the y-axis.
- If you have quantitative variables , use a scatterplot or a line graph.
- If your response variable is categorical, use a scatterplot or a line graph.
- If your explanatory variable is categorical, use a bar graph.
The term “ explanatory variable ” is sometimes preferred over “ independent variable ” because, in real world contexts, independent variables are often influenced by other variables. This means they aren’t totally independent.
Multiple independent variables may also be correlated with each other, so “explanatory variables” is a more appropriate term.
The difference between explanatory and response variables is simple:
- An explanatory variable is the expected cause, and it explains the results.
- A response variable is the expected effect, and it responds to other variables.
In a controlled experiment , all extraneous variables are held constant so that they can’t influence the results. Controlled experiments require:
- A control group that receives a standard treatment, a fake treatment, or no treatment.
- Random assignment of participants to ensure the groups are equivalent.
Depending on your study topic, there are various other methods of controlling variables .
There are 4 main types of extraneous variables :
- Demand characteristics : environmental cues that encourage participants to conform to researchers’ expectations.
- Experimenter effects : unintentional actions by researchers that influence study outcomes.
- Situational variables : environmental variables that alter participants’ behaviors.
- Participant variables : any characteristic or aspect of a participant’s background that could affect study results.
An extraneous variable is any variable that you’re not investigating that can potentially affect the dependent variable of your research study.
A confounding variable is a type of extraneous variable that not only affects the dependent variable, but is also related to the independent variable.
In a factorial design, multiple independent variables are tested.
If you test two variables, each level of one independent variable is combined with each level of the other independent variable to create different conditions.
Within-subjects designs have many potential threats to internal validity , but they are also very statistically powerful .
Advantages:
- Only requires small samples
- Statistically powerful
- Removes the effects of individual differences on the outcomes
Disadvantages:
- Internal validity threats reduce the likelihood of establishing a direct relationship between variables
- Time-related effects, such as growth, can influence the outcomes
- Carryover effects mean that the specific order of different treatments affect the outcomes
While a between-subjects design has fewer threats to internal validity , it also requires more participants for high statistical power than a within-subjects design .
- Prevents carryover effects of learning and fatigue.
- Shorter study duration.
- Needs larger samples for high power.
- Uses more resources to recruit participants, administer sessions, cover costs, etc.
- Individual differences may be an alternative explanation for results.
Yes. Between-subjects and within-subjects designs can be combined in a single study when you have two or more independent variables (a factorial design). In a mixed factorial design, one variable is altered between subjects and another is altered within subjects.
In a between-subjects design , every participant experiences only one condition, and researchers assess group differences between participants in various conditions.
In a within-subjects design , each participant experiences all conditions, and researchers test the same participants repeatedly for differences between conditions.
The word “between” means that you’re comparing different conditions between groups, while the word “within” means you’re comparing different conditions within the same group.
Random assignment is used in experiments with a between-groups or independent measures design. In this research design, there’s usually a control group and one or more experimental groups. Random assignment helps ensure that the groups are comparable.
In general, you should always use random assignment in this type of experimental design when it is ethically possible and makes sense for your study topic.
To implement random assignment , assign a unique number to every member of your study’s sample .
Then, you can use a random number generator or a lottery method to randomly assign each number to a control or experimental group. You can also do so manually, by flipping a coin or rolling a dice to randomly assign participants to groups.
Random selection, or random sampling , is a way of selecting members of a population for your study’s sample.
In contrast, random assignment is a way of sorting the sample into control and experimental groups.
Random sampling enhances the external validity or generalizability of your results, while random assignment improves the internal validity of your study.
In experimental research, random assignment is a way of placing participants from your sample into different groups using randomization. With this method, every member of the sample has a known or equal chance of being placed in a control group or an experimental group.
“Controlling for a variable” means measuring extraneous variables and accounting for them statistically to remove their effects on other variables.
Researchers often model control variable data along with independent and dependent variable data in regression analyses and ANCOVAs . That way, you can isolate the control variable’s effects from the relationship between the variables of interest.
Control variables help you establish a correlational or causal relationship between variables by enhancing internal validity .
If you don’t control relevant extraneous variables , they may influence the outcomes of your study, and you may not be able to demonstrate that your results are really an effect of your independent variable .
A control variable is any variable that’s held constant in a research study. It’s not a variable of interest in the study, but it’s controlled because it could influence the outcomes.
Including mediators and moderators in your research helps you go beyond studying a simple relationship between two variables for a fuller picture of the real world. They are important to consider when studying complex correlational or causal relationships.
Mediators are part of the causal pathway of an effect, and they tell you how or why an effect takes place. Moderators usually help you judge the external validity of your study by identifying the limitations of when the relationship between variables holds.
If something is a mediating variable :
- It’s caused by the independent variable .
- It influences the dependent variable
- When it’s taken into account, the statistical correlation between the independent and dependent variables is higher than when it isn’t considered.
A confounder is a third variable that affects variables of interest and makes them seem related when they are not. In contrast, a mediator is the mechanism of a relationship between two variables: it explains the process by which they are related.
A mediator variable explains the process through which two variables are related, while a moderator variable affects the strength and direction of that relationship.
There are three key steps in systematic sampling :
- Define and list your population , ensuring that it is not ordered in a cyclical or periodic order.
- Decide on your sample size and calculate your interval, k , by dividing your population by your target sample size.
- Choose every k th member of the population as your sample.
Systematic sampling is a probability sampling method where researchers select members of the population at a regular interval – for example, by selecting every 15th person on a list of the population. If the population is in a random order, this can imitate the benefits of simple random sampling .
Yes, you can create a stratified sample using multiple characteristics, but you must ensure that every participant in your study belongs to one and only one subgroup. In this case, you multiply the numbers of subgroups for each characteristic to get the total number of groups.
For example, if you were stratifying by location with three subgroups (urban, rural, or suburban) and marital status with five subgroups (single, divorced, widowed, married, or partnered), you would have 3 x 5 = 15 subgroups.
You should use stratified sampling when your sample can be divided into mutually exclusive and exhaustive subgroups that you believe will take on different mean values for the variable that you’re studying.
Using stratified sampling will allow you to obtain more precise (with lower variance ) statistical estimates of whatever you are trying to measure.
For example, say you want to investigate how income differs based on educational attainment, but you know that this relationship can vary based on race. Using stratified sampling, you can ensure you obtain a large enough sample from each racial group, allowing you to draw more precise conclusions.
In stratified sampling , researchers divide subjects into subgroups called strata based on characteristics that they share (e.g., race, gender, educational attainment).
Once divided, each subgroup is randomly sampled using another probability sampling method.
Cluster sampling is more time- and cost-efficient than other probability sampling methods , particularly when it comes to large samples spread across a wide geographical area.
However, it provides less statistical certainty than other methods, such as simple random sampling , because it is difficult to ensure that your clusters properly represent the population as a whole.
There are three types of cluster sampling : single-stage, double-stage and multi-stage clustering. In all three types, you first divide the population into clusters, then randomly select clusters for use in your sample.
- In single-stage sampling , you collect data from every unit within the selected clusters.
- In double-stage sampling , you select a random sample of units from within the clusters.
- In multi-stage sampling , you repeat the procedure of randomly sampling elements from within the clusters until you have reached a manageable sample.
Cluster sampling is a probability sampling method in which you divide a population into clusters, such as districts or schools, and then randomly select some of these clusters as your sample.
The clusters should ideally each be mini-representations of the population as a whole.
If properly implemented, simple random sampling is usually the best sampling method for ensuring both internal and external validity . However, it can sometimes be impractical and expensive to implement, depending on the size of the population to be studied,
If you have a list of every member of the population and the ability to reach whichever members are selected, you can use simple random sampling.
The American Community Survey is an example of simple random sampling . In order to collect detailed data on the population of the US, the Census Bureau officials randomly select 3.5 million households per year and use a variety of methods to convince them to fill out the survey.
Simple random sampling is a type of probability sampling in which the researcher randomly selects a subset of participants from a population . Each member of the population has an equal chance of being selected. Data is then collected from as large a percentage as possible of this random subset.
Quasi-experimental design is most useful in situations where it would be unethical or impractical to run a true experiment .
Quasi-experiments have lower internal validity than true experiments, but they often have higher external validity as they can use real-world interventions instead of artificial laboratory settings.
A quasi-experiment is a type of research design that attempts to establish a cause-and-effect relationship. The main difference with a true experiment is that the groups are not randomly assigned.
Blinding is important to reduce research bias (e.g., observer bias , demand characteristics ) and ensure a study’s internal validity .
If participants know whether they are in a control or treatment group , they may adjust their behavior in ways that affect the outcome that researchers are trying to measure. If the people administering the treatment are aware of group assignment, they may treat participants differently and thus directly or indirectly influence the final results.
- In a single-blind study , only the participants are blinded.
- In a double-blind study , both participants and experimenters are blinded.
- In a triple-blind study , the assignment is hidden not only from participants and experimenters, but also from the researchers analyzing the data.
Blinding means hiding who is assigned to the treatment group and who is assigned to the control group in an experiment .
An experimental group, also known as a treatment group, receives the treatment whose effect researchers wish to study, whereas a control group does not. They should be identical in all other ways.
Individual Likert-type questions are generally considered ordinal data , because the items have clear rank order, but don’t have an even distribution.
Overall Likert scale scores are sometimes treated as interval data. These scores are considered to have directionality and even spacing between them.
The type of data determines what statistical tests you should use to analyze your data.
A Likert scale is a rating scale that quantitatively assesses opinions, attitudes, or behaviors. It is made up of 4 or more questions that measure a single attitude or trait when response scores are combined.
To use a Likert scale in a survey , you present participants with Likert-type questions or statements, and a continuum of items, usually with 5 or 7 possible responses, to capture their degree of agreement.
In scientific research, concepts are the abstract ideas or phenomena that are being studied (e.g., educational achievement). Variables are properties or characteristics of the concept (e.g., performance at school), while indicators are ways of measuring or quantifying variables (e.g., yearly grade reports).
The process of turning abstract concepts into measurable variables and indicators is called operationalization .
There are various approaches to qualitative data analysis , but they all share five steps in common:
- Prepare and organize your data.
- Review and explore your data.
- Develop a data coding system.
- Assign codes to the data.
- Identify recurring themes.
The specifics of each step depend on the focus of the analysis. Some common approaches include textual analysis , thematic analysis , and discourse analysis .
There are five common approaches to qualitative research :
- Grounded theory involves collecting data in order to develop new theories.
- Ethnography involves immersing yourself in a group or organization to understand its culture.
- Narrative research involves interpreting stories to understand how people make sense of their experiences and perceptions.
- Phenomenological research involves investigating phenomena through people’s lived experiences.
- Action research links theory and practice in several cycles to drive innovative changes.
Hypothesis testing is a formal procedure for investigating our ideas about the world using statistics. It is used by scientists to test specific predictions, called hypotheses , by calculating how likely it is that a pattern or relationship between variables could have arisen by chance.
Operationalization means turning abstract conceptual ideas into measurable observations.
For example, the concept of social anxiety isn’t directly observable, but it can be operationally defined in terms of self-rating scores, behavioral avoidance of crowded places, or physical anxiety symptoms in social situations.
Before collecting data , it’s important to consider how you will operationalize the variables that you want to measure.
When conducting research, collecting original data has significant advantages:
- You can tailor data collection to your specific research aims (e.g. understanding the needs of your consumers or user testing your website)
- You can control and standardize the process for high reliability and validity (e.g. choosing appropriate measurements and sampling methods )
However, there are also some drawbacks: data collection can be time-consuming, labor-intensive and expensive. In some cases, it’s more efficient to use secondary data that has already been collected by someone else, but the data might be less reliable.
Data collection is the systematic process by which observations or measurements are gathered in research. It is used in many different contexts by academics, governments, businesses, and other organizations.
There are several methods you can use to decrease the impact of confounding variables on your research: restriction, matching, statistical control and randomization.
In restriction , you restrict your sample by only including certain subjects that have the same values of potential confounding variables.
In matching , you match each of the subjects in your treatment group with a counterpart in the comparison group. The matched subjects have the same values on any potential confounding variables, and only differ in the independent variable .
In statistical control , you include potential confounders as variables in your regression .
In randomization , you randomly assign the treatment (or independent variable) in your study to a sufficiently large number of subjects, which allows you to control for all potential confounding variables.
A confounding variable is closely related to both the independent and dependent variables in a study. An independent variable represents the supposed cause , while the dependent variable is the supposed effect . A confounding variable is a third variable that influences both the independent and dependent variables.
Failing to account for confounding variables can cause you to wrongly estimate the relationship between your independent and dependent variables.
To ensure the internal validity of your research, you must consider the impact of confounding variables. If you fail to account for them, you might over- or underestimate the causal relationship between your independent and dependent variables , or even find a causal relationship where none exists.
Yes, but including more than one of either type requires multiple research questions .
For example, if you are interested in the effect of a diet on health, you can use multiple measures of health: blood sugar, blood pressure, weight, pulse, and many more. Each of these is its own dependent variable with its own research question.
You could also choose to look at the effect of exercise levels as well as diet, or even the additional effect of the two combined. Each of these is a separate independent variable .
To ensure the internal validity of an experiment , you should only change one independent variable at a time.
No. The value of a dependent variable depends on an independent variable, so a variable cannot be both independent and dependent at the same time. It must be either the cause or the effect, not both!
You want to find out how blood sugar levels are affected by drinking diet soda and regular soda, so you conduct an experiment .
- The type of soda – diet or regular – is the independent variable .
- The level of blood sugar that you measure is the dependent variable – it changes depending on the type of soda.
Determining cause and effect is one of the most important parts of scientific research. It’s essential to know which is the cause – the independent variable – and which is the effect – the dependent variable.
In non-probability sampling , the sample is selected based on non-random criteria, and not every member of the population has a chance of being included.
Common non-probability sampling methods include convenience sampling , voluntary response sampling, purposive sampling , snowball sampling, and quota sampling .
Probability sampling means that every member of the target population has a known chance of being included in the sample.
Probability sampling methods include simple random sampling , systematic sampling , stratified sampling , and cluster sampling .
Using careful research design and sampling procedures can help you avoid sampling bias . Oversampling can be used to correct undercoverage bias .
Some common types of sampling bias include self-selection bias , nonresponse bias , undercoverage bias , survivorship bias , pre-screening or advertising bias, and healthy user bias.
Sampling bias is a threat to external validity – it limits the generalizability of your findings to a broader group of people.
A sampling error is the difference between a population parameter and a sample statistic .
A statistic refers to measures about the sample , while a parameter refers to measures about the population .
Populations are used when a research question requires data from every member of the population. This is usually only feasible when the population is small and easily accessible.
Samples are used to make inferences about populations . Samples are easier to collect data from because they are practical, cost-effective, convenient, and manageable.
There are seven threats to external validity : selection bias , history, experimenter effect, Hawthorne effect , testing effect, aptitude-treatment and situation effect.
The two types of external validity are population validity (whether you can generalize to other groups of people) and ecological validity (whether you can generalize to other situations and settings).
The external validity of a study is the extent to which you can generalize your findings to different groups of people, situations, and measures.
Cross-sectional studies cannot establish a cause-and-effect relationship or analyze behavior over a period of time. To investigate cause and effect, you need to do a longitudinal study or an experimental study .
Cross-sectional studies are less expensive and time-consuming than many other types of study. They can provide useful insights into a population’s characteristics and identify correlations for further research.
Sometimes only cross-sectional data is available for analysis; other times your research question may only require a cross-sectional study to answer it.
Longitudinal studies can last anywhere from weeks to decades, although they tend to be at least a year long.
The 1970 British Cohort Study , which has collected data on the lives of 17,000 Brits since their births in 1970, is one well-known example of a longitudinal study .
Longitudinal studies are better to establish the correct sequence of events, identify changes over time, and provide insight into cause-and-effect relationships, but they also tend to be more expensive and time-consuming than other types of studies.
Longitudinal studies and cross-sectional studies are two different types of research design . In a cross-sectional study you collect data from a population at a specific point in time; in a longitudinal study you repeatedly collect data from the same sample over an extended period of time.
Longitudinal study | Cross-sectional study |
---|---|
observations | Observations at a in time |
Observes the multiple times | Observes (a “cross-section”) in the population |
Follows in participants over time | Provides of society at a given point |
There are eight threats to internal validity : history, maturation, instrumentation, testing, selection bias , regression to the mean, social interaction and attrition .
Internal validity is the extent to which you can be confident that a cause-and-effect relationship established in a study cannot be explained by other factors.
In mixed methods research , you use both qualitative and quantitative data collection and analysis methods to answer your research question .
The research methods you use depend on the type of data you need to answer your research question .
- If you want to measure something or test a hypothesis , use quantitative methods . If you want to explore ideas, thoughts and meanings, use qualitative methods .
- If you want to analyze a large amount of readily-available data, use secondary data. If you want data specific to your purposes with control over how it is generated, collect primary data.
- If you want to establish cause-and-effect relationships between variables , use experimental methods. If you want to understand the characteristics of a research subject, use descriptive methods.
A confounding variable , also called a confounder or confounding factor, is a third variable in a study examining a potential cause-and-effect relationship.
A confounding variable is related to both the supposed cause and the supposed effect of the study. It can be difficult to separate the true effect of the independent variable from the effect of the confounding variable.
In your research design , it’s important to identify potential confounding variables and plan how you will reduce their impact.
Discrete and continuous variables are two types of quantitative variables :
- Discrete variables represent counts (e.g. the number of objects in a collection).
- Continuous variables represent measurable amounts (e.g. water volume or weight).
Quantitative variables are any variables where the data represent amounts (e.g. height, weight, or age).
Categorical variables are any variables where the data represent groups. This includes rankings (e.g. finishing places in a race), classifications (e.g. brands of cereal), and binary outcomes (e.g. coin flips).
You need to know what type of variables you are working with to choose the right statistical test for your data and interpret your results .
You can think of independent and dependent variables in terms of cause and effect: an independent variable is the variable you think is the cause , while a dependent variable is the effect .
In an experiment, you manipulate the independent variable and measure the outcome in the dependent variable. For example, in an experiment about the effect of nutrients on crop growth:
- The independent variable is the amount of nutrients added to the crop field.
- The dependent variable is the biomass of the crops at harvest time.
Defining your variables, and deciding how you will manipulate and measure them, is an important part of experimental design .
Experimental design means planning a set of procedures to investigate a relationship between variables . To design a controlled experiment, you need:
- A testable hypothesis
- At least one independent variable that can be precisely manipulated
- At least one dependent variable that can be precisely measured
When designing the experiment, you decide:
- How you will manipulate the variable(s)
- How you will control for any potential confounding variables
- How many subjects or samples will be included in the study
- How subjects will be assigned to treatment levels
Experimental design is essential to the internal and external validity of your experiment.
I nternal validity is the degree of confidence that the causal relationship you are testing is not influenced by other factors or variables .
External validity is the extent to which your results can be generalized to other contexts.
The validity of your experiment depends on your experimental design .
Reliability and validity are both about how well a method measures something:
- Reliability refers to the consistency of a measure (whether the results can be reproduced under the same conditions).
- Validity refers to the accuracy of a measure (whether the results really do represent what they are supposed to measure).
If you are doing experimental research, you also have to consider the internal and external validity of your experiment.
A sample is a subset of individuals from a larger population . Sampling means selecting the group that you will actually collect data from in your research. For example, if you are researching the opinions of students in your university, you could survey a sample of 100 students.
In statistics, sampling allows you to test a hypothesis about the characteristics of a population.
Quantitative research deals with numbers and statistics, while qualitative research deals with words and meanings.
Quantitative methods allow you to systematically measure variables and test hypotheses . Qualitative methods allow you to explore concepts and experiences in more detail.
Methodology refers to the overarching strategy and rationale of your research project . It involves studying the methods used in your field and the theories or principles behind them, in order to develop an approach that matches your objectives.
Methods are the specific tools and procedures you use to collect and analyze data (for example, experiments, surveys , and statistical tests ).
In shorter scientific papers, where the aim is to report the findings of a specific study, you might simply describe what you did in a methods section .
In a longer or more complex research project, such as a thesis or dissertation , you will probably include a methodology section , where you explain your approach to answering the research questions and cite relevant sources to support your choice of methods.
Ask our team
Want to contact us directly? No problem. We are always here for you.
- Email [email protected]
- Start live chat
- Call +1 (510) 822-8066
- WhatsApp +31 20 261 6040
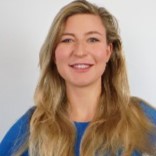
Our team helps students graduate by offering:
- A world-class citation generator
- Plagiarism Checker software powered by Turnitin
- Innovative Citation Checker software
- Professional proofreading services
- Over 300 helpful articles about academic writing, citing sources, plagiarism, and more
Scribbr specializes in editing study-related documents . We proofread:
- PhD dissertations
- Research proposals
- Personal statements
- Admission essays
- Motivation letters
- Reflection papers
- Journal articles
- Capstone projects
Scribbr’s Plagiarism Checker is powered by elements of Turnitin’s Similarity Checker , namely the plagiarism detection software and the Internet Archive and Premium Scholarly Publications content databases .
The add-on AI detector is powered by Scribbr’s proprietary software.
The Scribbr Citation Generator is developed using the open-source Citation Style Language (CSL) project and Frank Bennett’s citeproc-js . It’s the same technology used by dozens of other popular citation tools, including Mendeley and Zotero.
You can find all the citation styles and locales used in the Scribbr Citation Generator in our publicly accessible repository on Github .
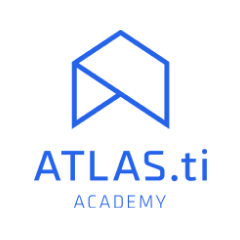
Understanding Control Groups for Research
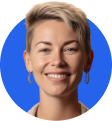
Introduction
What are control groups in research, examples of control groups in research, control group vs. experimental group, types of control groups, control groups in non-experimental research.
A control group is typically thought of as the baseline in an experiment. In an experiment, clinical trial, or other sort of controlled study, there are at least two groups whose results are compared against each other.
The experimental group receives some sort of treatment, and their results are compared against those of the control group, which is not given the treatment. This is important to determine whether there is an identifiable causal relationship between the treatment and the resulting effects.
As intuitive as this may sound, there is an entire methodology that is useful to understanding the role of the control group in experimental research and as part of a broader concept in research. This article will examine the particulars of that methodology so you can design your research more rigorously .
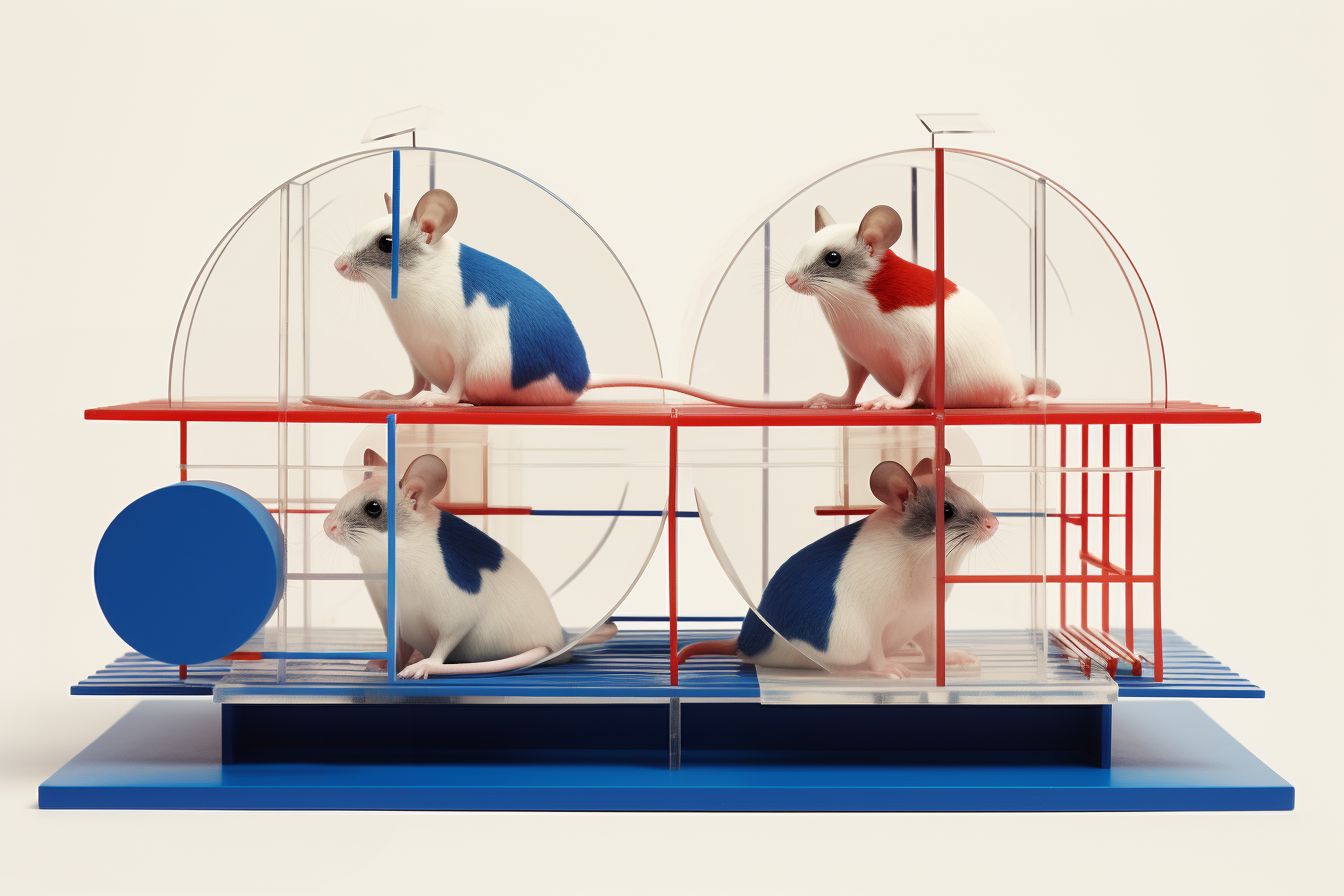
Suppose that a friend or colleague of yours has a headache. You give them some over-the-counter medicine to relieve some of the pain. Shortly after they take the medicine, the pain is gone and they feel better. In casual settings, we can assume that it must be the medicine that was the cause of their headache going away.
In scientific research, however, we don't really know if the medicine made a difference or if the headache would have gone away on its own. Maybe in the time it took for the headache to go away, they ate or drank something that might have had an effect. Perhaps they had a quick nap that helped relieve the tension from the headache. Without rigorously exploring this phenomenon , any number of confounding factors exist that can make us question the actual efficacy of any particular treatment.
Experimental research relies on observing differences between the two groups by "controlling" the independent variable , or in the case of our example above, the medicine that is given or not given depending on the group. The dependent variable in this case is the change in how the person suffering the headache feels, and the difference between taking and not taking the medicine is evidence (or lack thereof) that the treatment is effective.
The catch is that, between the control group and other groups (typically called experimental groups), it's important to ensure that all other factors are the same or at least as similar as possible. Things such as age, fitness level, and even occupation can affect the likelihood someone has a headache and whether a certain medication is effective.
Faced with this dynamic, researchers try to make sure that participants in their control group and experimental group are as similar as possible to each other, with the only difference being the treatment they receive.
Experimental research is often associated with scientists in lab coats holding beakers containing liquids with funny colors. Clinical trials that deal with medical treatments rely primarily, if not exclusively, on experimental research designs involving comparisons between control and experimental groups.
However, many studies in the social sciences also employ some sort of experimental design which calls for the use of control groups. This type of research is useful when researchers are trying to confirm or challenge an existing notion or measure the difference in effects.
Workplace efficiency research
How might a company know if an employee training program is effective? They may decide to pilot the program to a small group of their employees before they implement the training to their entire workforce.
If they adopt an experimental design, they could compare results between an experimental group of workers who participate in the training program against a control group who continues as per usual without any additional training.
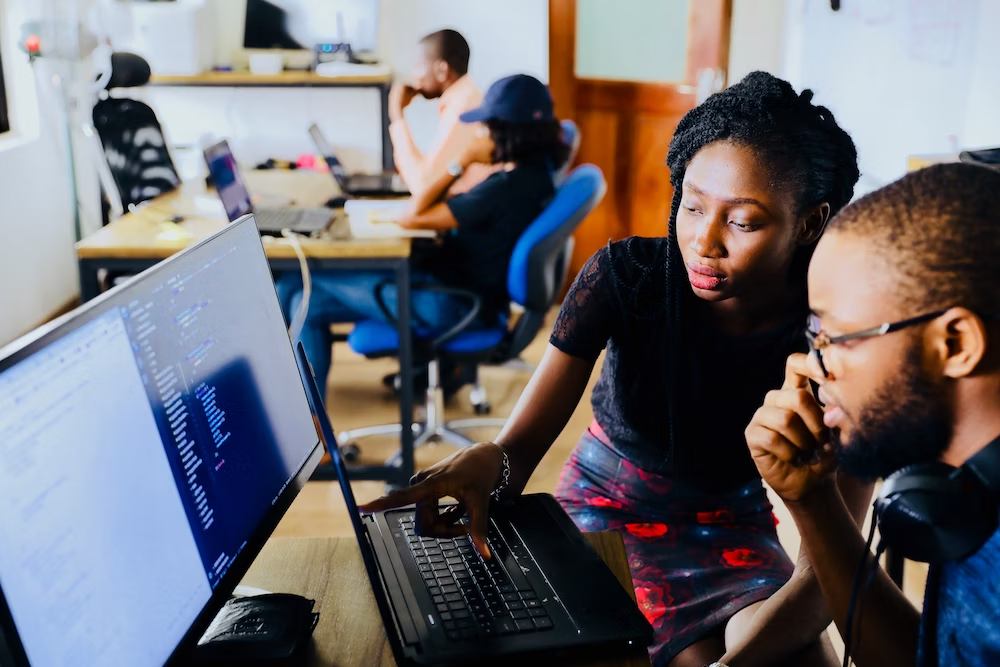
Qualitative data analysis starts with ATLAS.ti
Turn data into rich insights with our powerful data analysis software. Get started with a free trial.
Mental health research
Music certainly has profound effects on psychology, but what kind of music would be most effective for concentration? Here, a researcher might be interested in having participants in a control group perform a series of tasks in an environment with no background music, and participants in multiple experimental groups perform those same tasks with background music of different genres. The subsequent analysis could determine how well people perform with classical music, jazz music, or no music at all in the background.
Educational research
Suppose that you want to improve reading ability among elementary school students, and there is research on a particular teaching method that is associated with facilitating reading comprehension. How do you measure the effects of that teaching method?
A study could be conducted on two groups of otherwise equally proficient students to measure the difference in test scores. The teacher delivers the same instruction to the control group as they have to previous students, but they teach the experimental group using the new technique. A reading test after a certain amount of instruction could determine the extent of effectiveness of the new teaching method.
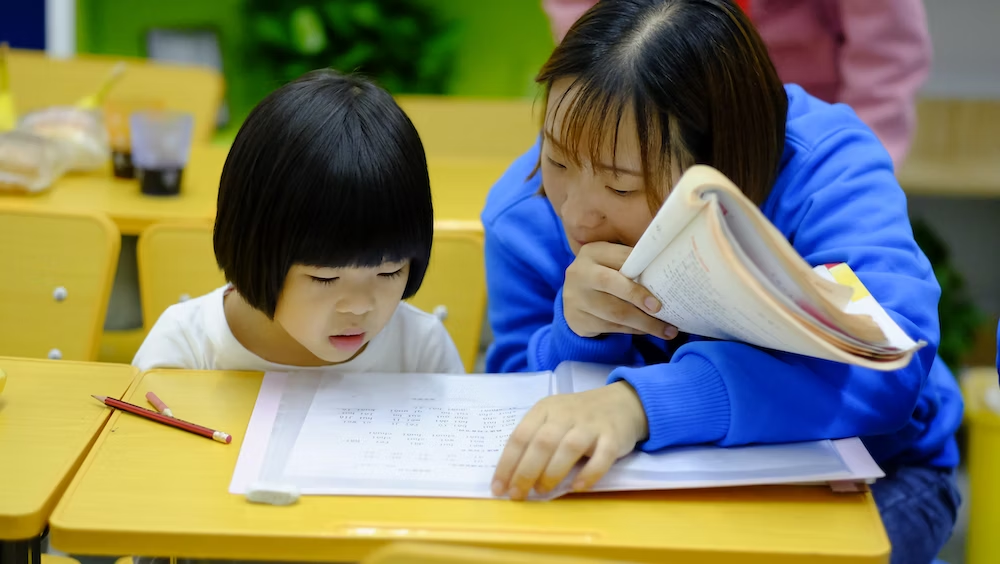
As you can see from the three examples above, experimental groups are the counterbalance to control groups. A control group offers an essential point of comparison. For an experimental study to be considered credible, it must establish a baseline against which novel research is conducted.
Researchers can determine the makeup of their experimental and control groups from their literature review . Remember that the objective of a review is to establish what is known about the object of inquiry and what is not known. Where experimental groups explore the unknown aspects of scientific knowledge, a control group is a sort of simulation of what would happen if the treatment or intervention was not administered. As a result, it will benefit researchers to have a foundational knowledge of the existing research to create a credible control group against which experimental results are compared, especially in terms of remaining sensitive to relevant participant characteristics that could confound the effects of your treatment or intervention so that you can appropriately distribute participants between the experimental and control groups.
There are multiple control groups to consider depending on the study you are looking to conduct. All of them are variations of the basic control group used to establish a baseline for experimental conditions.
No-treatment control group
This kind of control group is common when trying to establish the effects of an experimental treatment against the absence of treatment. This is arguably the most straightforward approach to an experimental design as it aims to directly demonstrate how a certain change in conditions produces an effect.
Placebo control group
In this case, the control group receives some sort of treatment under the exact same procedures as those in the experimental group. The only difference in this case is that the treatment in the placebo control group has already been judged to be ineffective, except that the research participants don't know that it is ineffective.
Placebo control groups (or negative control groups) are useful for allowing researchers to account for any psychological or affective factors that might impact the outcomes. The negative control group exists to explicitly eliminate factors other than changes in the independent variable conditions as causes of the effects experienced in the experimental group.
Positive control group
Contrasted with a no-treatment control group, a positive control group employs a treatment against which the treatment in the experimental group is compared. However, unlike in a placebo group, participants in a positive control group receive treatment that is known to have an effect.
If we were to use our first example of headache medicine, a researcher could compare results between medication that is commonly known as effective against the newer medication that the researcher thinks is more effective. Positive control groups are useful for validating experimental results when compared against familiar results.
Historical control group
Rather than study participants in control group conditions, researchers may employ existing data to create historical control groups. This form of control group is useful for examining changing conditions over time, particularly when incorporating past conditions that can't be replicated in the analysis.
Qualitative research more often relies on non-experimental research such as observations and interviews to examine phenomena in their natural environments. This sort of research is more suited for inductive and exploratory inquiries, not confirmatory studies meant to test or measure a phenomenon.
That said, the broader concept of a control group is still present in observational and interview research in the form of a comparison group. Comparison groups are used in qualitative research designs to show differences between phenomena, with the exception being that there is no baseline against which data is analyzed.
Comparison groups are useful when an experimental environment cannot produce results that would be applicable to real-world conditions. Research inquiries examining the social world face challenges of having too many variables to control, making observations and interviews across comparable groups more appropriate for data collection than clinical or sterile environments.
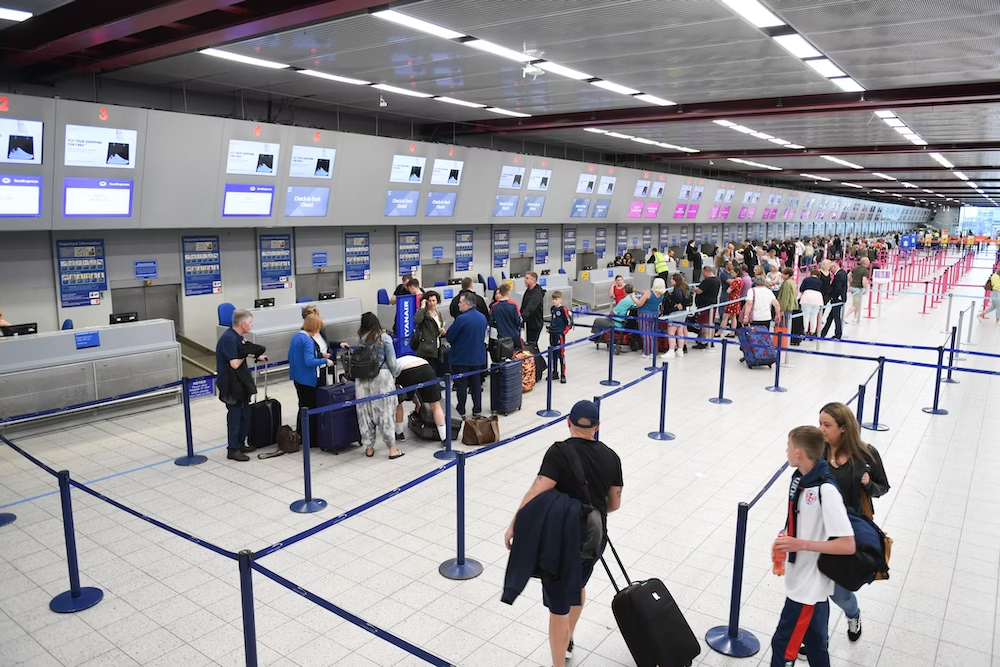
Analyze data and generate rich results with ATLAS.ti
Try out a free trial of ATLAS.ti to see how you can make the most of your qualitative data.
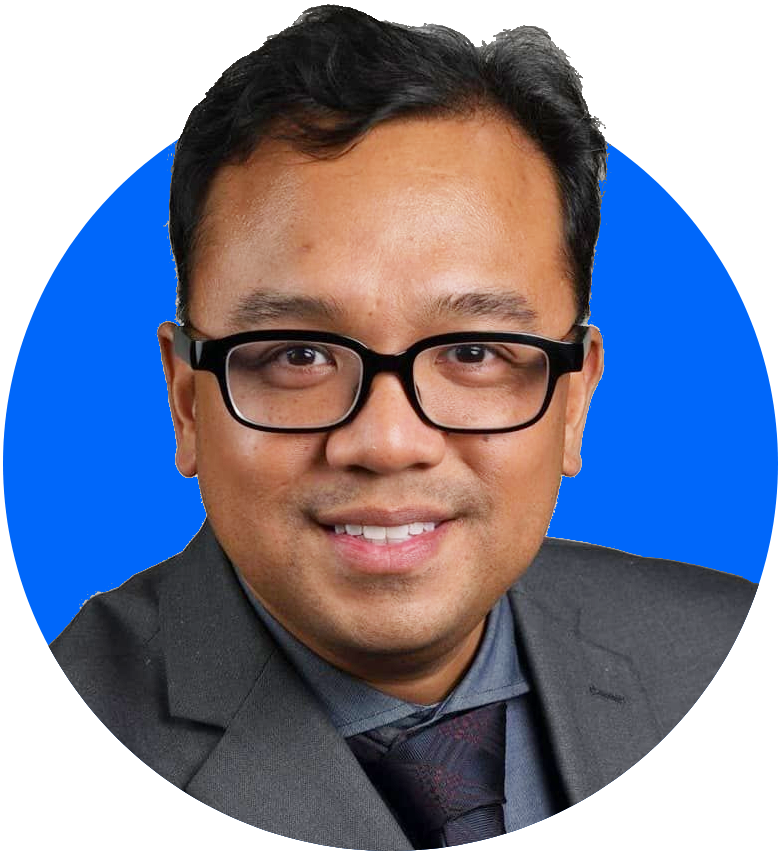

Control Group Design: The Cornerstone of True Experimental Research

Table of Contents
Have you ever wondered how scientists determine the effectiveness of a new medication or therapeutic technique? The answer lies within a cornerstone of psychological research: the control group design . This powerful tool allows researchers to uncover the true effects of an intervention by comparing outcomes between treated and untreated groups. So, let’s dive into the intricacies of this design and uncover why it’s so pivotal in the scientific quest for knowledge.
What is control group design?
Control group design is a methodological approach where one group receives the experimental treatment, while a separate ‘control’ group does not. The control group serves as a benchmark to measure the effect of the variable being tested. This comparison can reveal whether changes in the experimental group are indeed due to the treatment or if they could be attributed to other factors.
The different forms of control group design
Though the concept might seem straightforward, control group design is nuanced and can be executed in various forms, each tailored to address specific research questions and concerns.
Post\-test only control group design
This form involves two groups: one receiving the treatment and the other not. Both groups are measured after the treatment period, providing data on the effect of the treatment. This design is particularly useful when pretesting might influence the participants’ responses to the treatment.
Pretest\-posttest control group design
In this approach, both the experimental and control groups are measured before and after the treatment. The pretest ensures that any changes observed in the post-test can be attributed to the treatment rather than to pre-existing differences between the groups.
Addressing validity concerns with control group design
Control group design doesn’t just sort groups and compare outcomes; it’s a sophisticated strategy to bolster the study’s validity. Let’s delve into how it safeguards against threats to experimental validity.
Internal validity
Internal validity refers to the degree to which we can be confident that the change in the dependent variable was indeed caused by the independent variable, and not by other factors. Control groups help to rule out alternative explanations by ensuring that the only difference between groups is the treatment variable.
External validity
External validity is about the generalizability of the findings. By using control groups that closely resemble the target population, researchers can make stronger claims about how their findings might apply in real-world settings.
The Solomon Four Group Design
What if you’re concerned about both pretesting effects and external validity? Enter the Solomon Four Group Design. This robust method combines both post-test only and pretest-posttest configurations across four different groups, providing a comprehensive safeguard against validity threats.
How it works
The Solomon Four Group Design involves four groups, where two receive the treatment and two serve as controls. One treated and one control group are pretested, while the others are not. This design helps identify any pretesting effects and further isolates the treatment’s impact, offering a fuller picture of the treatment’s effectiveness.
Ensuring research integrity with control group design
Control group design is more than just a way to compare outcomes. It’s a fundamental approach to ensuring that research findings are accurate, reliable, and applicable. By methodically controlling for extraneous variables and threats to validity, researchers can draw more definitive conclusions about the effects of their treatments.
Minimizing biases
By randomly assigning participants to the experimental or control groups, control group design minimizes selection biases, ensuring that the groups are comparable at the start of the experiment.
Enhancing replicability
Control group design also enhances the replicability of research. By providing a clear structure for the experiment, other researchers can replicate the study to confirm its findings, which is a fundamental aspect of the scientific method.
The control group design is a testament to the meticulous nature of scientific inquiry. By thoughtfully comparing treated and untreated groups, researchers can illuminate the true effects of a variable, paving the way for discoveries that can enhance our understanding of human behavior and improve psychological treatments. It’s a method that epitomizes the rigor and integrity of experimental research in psychology.
What do you think? How might the control group design be applied to current issues in psychology? Can you think of a situation where a control group design might not be the best approach for a study?
How useful was this post?
Click on a star to rate it!
Average rating 0 / 5. Vote count: 0
No votes so far! Be the first to rate this post.
We are sorry that this post was not useful for you!
Let us improve this post!
Tell us how we can improve this post?
Submit a Comment Cancel reply
Your email address will not be published. Required fields are marked *
Save my name, email, and website in this browser for the next time I comment.
Submit Comment
Research Methods in Psychology
1 Introduction to Psychological Research – Objectives and Goals, Problems, Hypothesis and Variables
- Nature of Psychological Research
- The Context of Discovery
- Context of Justification
- Characteristics of Psychological Research
- Goals and Objectives of Psychological Research
2 Introduction to Psychological Experiments and Tests
- Independent and Dependent Variables
- Extraneous Variables
- Experimental and Control Groups
- Introduction of Test
- Types of Psychological Test
- Uses of Psychological Tests
3 Steps in Research
- Research Process
- Identification of the Problem
- Review of Literature
- Formulating a Hypothesis
- Identifying Manipulating and Controlling Variables
- Formulating a Research Design
- Constructing Devices for Observation and Measurement
- Sample Selection and Data Collection
- Data Analysis and Interpretation
- Hypothesis Testing
- Drawing Conclusion
4 Types of Research and Methods of Research
- Historical Research
- Descriptive Research
- Correlational Research
- Qualitative Research
- Ex-Post Facto Research
- True Experimental Research
- Quasi-Experimental Research
5 Definition and Description Research Design, Quality of Research Design
- Research Design
- Purpose of Research Design
- Design Selection
- Criteria of Research Design
- Qualities of Research Design
6 Experimental Design (Control Group Design and Two Factor Design)
- Experimental Design
- Control Group Design
- Two Factor Design
7 Survey Design
- Survey Research Designs
- Steps in Survey Design
- Structuring and Designing the Questionnaire
- Interviewing Methodology
- Data Analysis
- Final Report
8 Single Subject Design
- Single Subject Design: Definition and Meaning
- Phases Within Single Subject Design
- Requirements of Single Subject Design
- Characteristics of Single Subject Design
- Types of Single Subject Design
- Advantages of Single Subject Design
- Disadvantages of Single Subject Design
9 Observation Method
- Definition and Meaning of Observation
- Characteristics of Observation
- Types of Observation
- Advantages and Disadvantages of Observation
- Guides for Observation Method
10 Interview and Interviewing
- Definition of Interview
- Types of Interview
- Aspects of Qualitative Research Interviews
- Interview Questions
- Convergent Interviewing as Action Research
- Research Team
11 Questionnaire Method
- Definition and Description of Questionnaires
- Types of Questionnaires
- Purpose of Questionnaire Studies
- Designing Research Questionnaires
- The Methods to Make a Questionnaire Efficient
- The Types of Questionnaire to be Included in the Questionnaire
- Advantages and Disadvantages of Questionnaire
- When to Use a Questionnaire?
12 Case Study
- Definition and Description of Case Study Method
- Historical Account of Case Study Method
- Designing Case Study
- Requirements for Case Studies
- Guideline to Follow in Case Study Method
- Other Important Measures in Case Study Method
- Case Reports
13 Report Writing
- Purpose of a Report
- Writing Style of the Report
- Report Writing – the Do’s and the Don’ts
- Format for Report in Psychology Area
- Major Sections in a Report
14 Review of Literature
- Purposes of Review of Literature
- Sources of Review of Literature
- Types of Literature
- Writing Process of the Review of Literature
- Preparation of Index Card for Reviewing and Abstracting
15 Methodology
- Definition and Purpose of Methodology
- Participants (Sample)
- Apparatus and Materials
16 Result, Analysis and Discussion of the Data
- Definition and Description of Results
- Statistical Presentation
- Tables and Figures
17 Summary and Conclusion
- Summary Definition and Description
- Guidelines for Writing a Summary
- Writing the Summary and Choosing Words
- A Process for Paraphrasing and Summarising
- Summary of a Report
- Writing Conclusions
18 References in Research Report
- Reference List (the Format)
- References (Process of Writing)
- Reference List and Print Sources
- Electronic Sources
- Book on CD Tape and Movie
- Reference Specifications
- General Guidelines to Write References
Share on Mastodon
- Bipolar Disorder
- Therapy Center
- When To See a Therapist
- Types of Therapy
- Best Online Therapy
- Best Couples Therapy
- Best Family Therapy
- Managing Stress
- Sleep and Dreaming
- Understanding Emotions
- Self-Improvement
- Healthy Relationships
- Student Resources
- Personality Types
- Guided Meditations
- Verywell Mind Insights
- 2024 Verywell Mind 25
- Mental Health in the Classroom
- Editorial Process
- Meet Our Review Board
- Crisis Support
Experimental Group in Psychology Experiments
Kendra Cherry, MS, is a psychosocial rehabilitation specialist, psychology educator, and author of the "Everything Psychology Book."
:max_bytes(150000):strip_icc():format(webp)/IMG_9791-89504ab694d54b66bbd72cb84ffb860e.jpg)
Emily is a board-certified science editor who has worked with top digital publishing brands like Voices for Biodiversity, Study.com, GoodTherapy, Vox, and Verywell.
:max_bytes(150000):strip_icc():format(webp)/Emily-Swaim-1000-0f3197de18f74329aeffb690a177160c.jpg)
In a randomized and controlled psychology experiment , the researchers are examining the impact of an experimental condition on a group of participants (does the independent variable 'X' cause a change in the dependent variable 'Y'?). To determine cause and effect, there must be at least two groups to compare, the experimental group and the control group.
The participants who are in the experimental condition are those who receive the treatment or intervention of interest. The data from their outcomes are collected and compared to the data from a group that did not receive the experimental treatment. The control group may have received no treatment at all, or they may have received a placebo treatment or the standard treatment in current practice.
Comparing the experimental group to the control group allows researchers to see how much of an impact the intervention had on the participants.
A Closer Look at Experimental Groups
Imagine that you want to do an experiment to determine if listening to music while working out can lead to greater weight loss. After getting together a group of participants, you randomly assign them to one of three groups. One group listens to upbeat music while working out, one group listens to relaxing music, and the third group listens to no music at all. All of the participants work out for the same amount of time and the same number of days each week.
In this experiment, the group of participants listening to no music while working out is the control group. They serve as a baseline with which to compare the performance of the other two groups. The other two groups in the experiment are the experimental groups. They each receive some level of the independent variable, which in this case is listening to music while working out.
In this experiment, you find that the participants who listened to upbeat music experienced the greatest weight loss result, largely because those who listened to this type of music exercised with greater intensity than those in the other two groups. By comparing the results from your experimental groups with the results of the control group, you can more clearly see the impact of the independent variable.
Some Things to Know
When it comes to using experimental groups in a psychology experiment, there are a few important things to know:
- In order to determine the impact of an independent variable, it is important to have at least two different treatment conditions. This usually involves using a control group that receives no treatment against an experimental group that receives the treatment. However, there can also be a number of different experimental groups in the same experiment.
- Care must be taken when assigning participants to groups. So how do researchers determine who is in the control group and who is in the experimental group? In an ideal situation, the researchers would use random assignment to place participants in groups. In random assignment, each individual stands an equal shot at being assigned to either group. Participants might be randomly assigned using methods such as a coin flip or a number draw. By using random assignment, researchers can help ensure that the groups are not unfairly stacked with people who share characteristics that might unfairly skew the results.
- Variables must be well-defined. Before you begin manipulating things in an experiment, you need to have very clear operational definitions in place. These definitions clearly explain what your variables are, including exactly how you are manipulating the independent variable and exactly how you are measuring the outcomes.
A Word From Verywell
Experiments play an important role in the research process and allow psychologists to investigate cause-and-effect relationships between different variables. Having one or more experimental groups allows researchers to vary different levels or types of the experimental variable and then compare the effects of these changes against a control group. The goal of this experimental manipulation is to gain a better understanding of the different factors that may have an impact on how people think, feel, and act.
Byrd-Bredbenner C, Wu F, Spaccarotella K, Quick V, Martin-Biggers J, Zhang Y. Systematic review of control groups in nutrition education intervention research . Int J Behav Nutr Phys Act. 2017;14(1):91. doi:10.1186/s12966-017-0546-3
Steingrimsdottir HS, Arntzen E. On the utility of within-participant research design when working with patients with neurocognitive disorders . Clin Interv Aging. 2015;10:1189-1200. doi:10.2147/CIA.S81868
Oberste M, Hartig P, Bloch W, et al. Control group paradigms in studies investigating acute effects of exercise on cognitive performance—An experiment on expectation-driven placebo effects . Front Hum Neurosci. 2017;11:600. doi:10.3389/fnhum.2017.00600
Kim H. Statistical notes for clinical researchers: Analysis of covariance (ANCOVA) . Restor Dent Endod . 2018;43(4):e43. doi:10.5395/rde.2018.43.e43
Bate S, Karp NA. A common control group — Optimising the experiment design to maximise sensitivity . PLoS ONE. 2014;9(12):e114872. doi:10.1371/journal.pone.0114872
Myers A, Hansen C. Experimental Psychology . 7th Ed. Cengage Learning; 2012.
By Kendra Cherry, MSEd Kendra Cherry, MS, is a psychosocial rehabilitation specialist, psychology educator, and author of the "Everything Psychology Book."
- COVID-19 Tracker
- Biochemistry
- Anatomy & Physiology
- Microbiology
- Neuroscience
- Animal Kingdom
- NGSS High School
- Latest News
- Editors’ Picks
- Weekly Digest
- Quotes about Biology
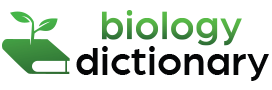
Control Group
Reviewed by: BD Editors
Control Group Definition
In scientific experiments, the control group is the group of subject that receive no treatment or a standardized treatment. Without the control group, there would be nothing to compare the treatment group to. When statistics refer to something being “X times more likely to happen” they are referring to the difference in the measurement between the treatment and control group. The control group provides a baseline in the experiment. The variable that is being studied in the experiment is not changed or is limited to zero in the control group. This insures that the effects of the variable are being studied. Most experiments try to add the variable back in increments to different treatment groups, to really begin to discern the effects of the variable in the system.
Ideally, the control group is subject to the same exact conditions as the treatment groups. This insures that only the effects produced by the variable are being measured. In a study of plants, for instance, all the plants would ideally be in the same room, with the same light and air conditions. In biological studies, it is also important that the organisms in the treatment and control groups come from the same population. Ideally, the organisms would all be clones of each other, to reduce genetic differences. This is the case in many artificially selected lab species, which have been selected to be very similar to each other. This ensures that the results obtained are valid.
Examples of Control Group
Testing enzyme strength.
In a simple biological lab experiment, students can test the effects of different concentrations of enzyme. The student can prepare a stock solution of enzyme by spitting into a beaker. Human spit contains the enzyme amylase, which breaks down starches. The concentration of enzyme can be varied by dividing the stock solution and adding in various amounts of water. Once various solutions of different strength enzyme have been produced, the experiment can begin.
In several treatment beakers are placed the following ingredients: starch, iodine, and the different solutions of enzyme. In the control group, a beaker is filled with starch and iodine, but no enzyme. When iodine is in the presence of starch, it turns black. As the enzyme depletes the starch in each beaker, the solution clears up and is a lighter yellow or brown color. In this way, the student can tell how long the enzymes in each beaker take to completely process the same amount of substrate. The control group is important because it will tell the student if the starch breaks down without the enzyme, which it will, given enough time.
Testing Drugs and the Placebo Effect
When drugs are tested on humans, control groups are also used. Although control groups were just considered good science, they have found an interesting phenomena in drug trials. Oftentimes, control groups in drug trials consist of people who also have the disease or ailment, but who don’t receive the medicine being tested. Instead, to keep the control group the same as the treatment groups, the patients in the control group are also given a pill. This is a sugar pill usually and contains no medicine. This practice of having a control group is important for drug trial, because it validates the results obtained. However, the control groups have also demonstrated an interesting effect, known as the placebo effect
In some drug trials, where the control group is given a fake medicine, patients start to see results. Scientists call this the placebo effect, and as of yet it is mostly unexplained. Some scientists have suggested that people get better simply because they believed they were going to get better, but this theory remains untested. Other scientists claim that unknown variables in the experiment caused the patients to get better. This theory remains unproven, as well.
Related Biology Terms
- Treatment Group – The group that receives the variable, or altered amounts of the variable.
- Variable – The part of the experiment being studied which is changed, or altered, throughout the experiment.
- Scientific Method – The steps scientist follow to ensure their results are valid and reproducible.
- Placebo Effect – A phenomenon when patients in the control group experience the same effects as those in the treatment group, though no treatment was given.
Cite This Article
Subscribe to our newsletter, privacy policy, terms of service, scholarship, latest posts, white blood cell, t cell immunity, satellite cells, embryonic stem cells, popular topics, homeostasis, hydrochloric acid, scientific method, translation.
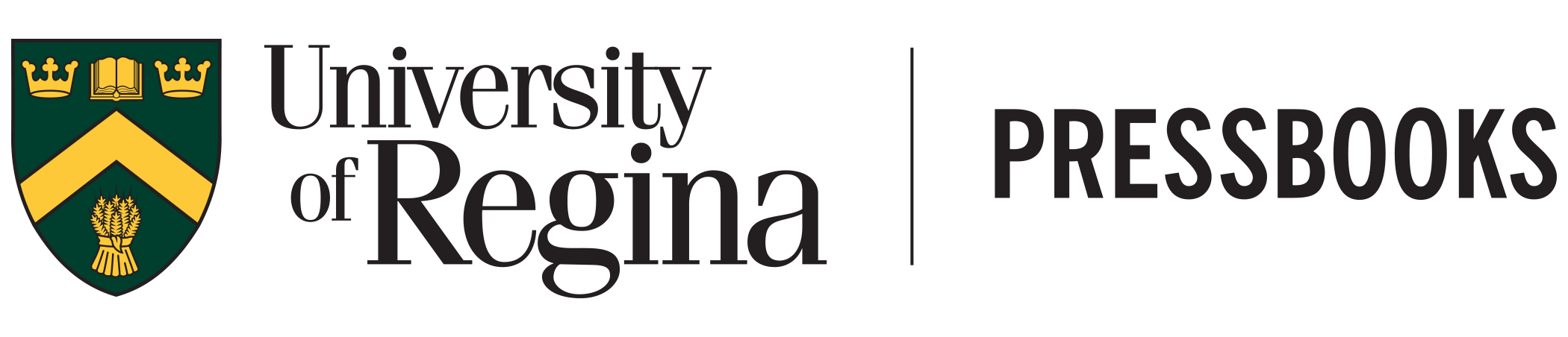
Want to create or adapt books like this? Learn more about how Pressbooks supports open publishing practices.
30 8.1 Experimental design: What is it and when should it be used?
Learning objectives.
- Define experiment
- Identify the core features of true experimental designs
- Describe the difference between an experimental group and a control group
- Identify and describe the various types of true experimental designs
Experiments are an excellent data collection strategy for social workers wishing to observe the effects of a clinical intervention or social welfare program. Understanding what experiments are and how they are conducted is useful for all social scientists, whether they actually plan to use this methodology or simply aim to understand findings from experimental studies. An experiment is a method of data collection designed to test hypotheses under controlled conditions. In social scientific research, the term experiment has a precise meaning and should not be used to describe all research methodologies.
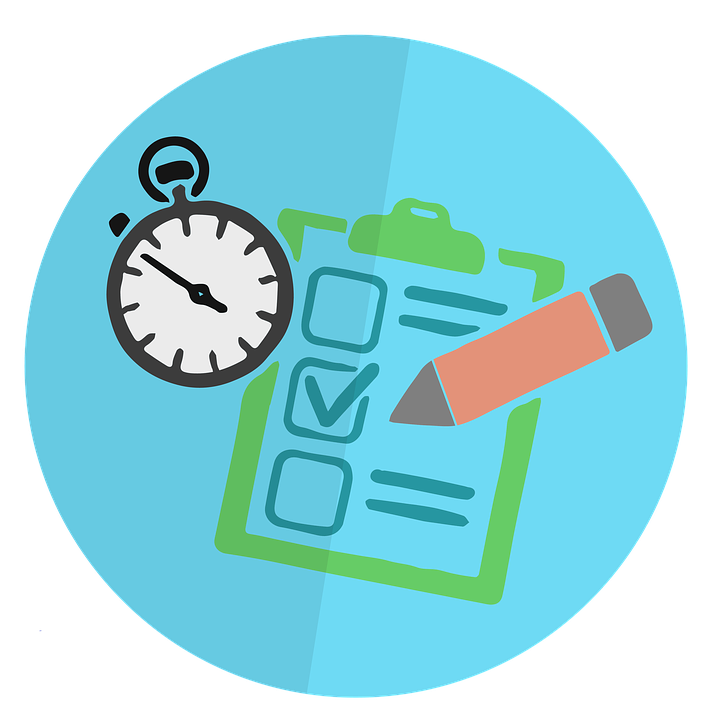
Experiments have a long and important history in social science. Behaviorists such as John Watson, B. F. Skinner, Ivan Pavlov, and Albert Bandura used experimental design to demonstrate the various types of conditioning. Using strictly controlled environments, behaviorists were able to isolate a single stimulus as the cause of measurable differences in behavior or physiological responses. The foundations of social learning theory and behavior modification are found in experimental research projects. Moreover, behaviorist experiments brought psychology and social science away from the abstract world of Freudian analysis and towards empirical inquiry, grounded in real-world observations and objectively-defined variables. Experiments are used at all levels of social work inquiry, including agency-based experiments that test therapeutic interventions and policy experiments that test new programs.
Several kinds of experimental designs exist. In general, designs considered to be true experiments contain three basic key features:
- random assignment of participants into experimental and control groups
- a “treatment” (or intervention) provided to the experimental group
- measurement of the effects of the treatment in a post-test administered to both groups
Some true experiments are more complex. Their designs can also include a pre-test and can have more than two groups, but these are the minimum requirements for a design to be a true experiment.
Experimental and control groups
In a true experiment, the effect of an intervention is tested by comparing two groups: one that is exposed to the intervention (the experimental group , also known as the treatment group) and another that does not receive the intervention (the control group ). Importantly, participants in a true experiment need to be randomly assigned to either the control or experimental groups. Random assignment uses a random number generator or some other random process to assign people into experimental and control groups. Random assignment is important in experimental research because it helps to ensure that the experimental group and control group are comparable and that any differences between the experimental and control groups are due to random chance. We will address more of the logic behind random assignment in the next section.
Treatment or intervention
In an experiment, the independent variable is receiving the intervention being tested—for example, a therapeutic technique, prevention program, or access to some service or support. It is less common in of social work research, but social science research may also have a stimulus, rather than an intervention as the independent variable. For example, an electric shock or a reading about death might be used as a stimulus to provoke a response.
In some cases, it may be immoral to withhold treatment completely from a control group within an experiment. If you recruited two groups of people with severe addiction and only provided treatment to one group, the other group would likely suffer. For these cases, researchers use a control group that receives “treatment as usual.” Experimenters must clearly define what treatment as usual means. For example, a standard treatment in substance abuse recovery is attending Alcoholics Anonymous or Narcotics Anonymous meetings. A substance abuse researcher conducting an experiment may use twelve-step programs in their control group and use their experimental intervention in the experimental group. The results would show whether the experimental intervention worked better than normal treatment, which is useful information.
The dependent variable is usually the intended effect the researcher wants the intervention to have. If the researcher is testing a new therapy for individuals with binge eating disorder, their dependent variable may be the number of binge eating episodes a participant reports. The researcher likely expects her intervention to decrease the number of binge eating episodes reported by participants. Thus, she must, at a minimum, measure the number of episodes that occur after the intervention, which is the post-test . In a classic experimental design, participants are also given a pretest to measure the dependent variable before the experimental treatment begins.
Types of experimental design
Let’s put these concepts in chronological order so we can better understand how an experiment runs from start to finish. Once you’ve collected your sample, you’ll need to randomly assign your participants to the experimental group and control group. In a common type of experimental design, you will then give both groups your pretest, which measures your dependent variable, to see what your participants are like before you start your intervention. Next, you will provide your intervention, or independent variable, to your experimental group, but not to your control group. Many interventions last a few weeks or months to complete, particularly therapeutic treatments. Finally, you will administer your post-test to both groups to observe any changes in your dependent variable. What we’ve just described is known as the classical experimental design and is the simplest type of true experimental design. All of the designs we review in this section are variations on this approach. Figure 8.1 visually represents these steps.

An interesting example of experimental research can be found in Shannon K. McCoy and Brenda Major’s (2003) study of people’s perceptions of prejudice. In one portion of this multifaceted study, all participants were given a pretest to assess their levels of depression. No significant differences in depression were found between the experimental and control groups during the pretest. Participants in the experimental group were then asked to read an article suggesting that prejudice against their own racial group is severe and pervasive, while participants in the control group were asked to read an article suggesting that prejudice against a racial group other than their own is severe and pervasive. Clearly, these were not meant to be interventions or treatments to help depression, but were stimuli designed to elicit changes in people’s depression levels. Upon measuring depression scores during the post-test period, the researchers discovered that those who had received the experimental stimulus (the article citing prejudice against their same racial group) reported greater depression than those in the control group. This is just one of many examples of social scientific experimental research.
In addition to classic experimental design, there are two other ways of designing experiments that are considered to fall within the purview of “true” experiments (Babbie, 2010; Campbell & Stanley, 1963). The posttest-only control group design is almost the same as classic experimental design, except it does not use a pretest. Researchers who use posttest-only designs want to eliminate testing effects , in which participants’ scores on a measure change because they have already been exposed to it. If you took multiple SAT or ACT practice exams before you took the real one you sent to colleges, you’ve taken advantage of testing effects to get a better score. Considering the previous example on racism and depression, participants who are given a pretest about depression before being exposed to the stimulus would likely assume that the intervention is designed to address depression. That knowledge could cause them to answer differently on the post-test than they otherwise would. In theory, as long as the control and experimental groups have been determined randomly and are therefore comparable, no pretest is needed. However, most researchers prefer to use pretests in case randomization did not result in equivalent groups and to help assess change over time within both the experimental and control groups.
Researchers wishing to account for testing effects but also gather pretest data can use a Solomon four-group design. In the Solomon four-group design , the researcher uses four groups. Two groups are treated as they would be in a classic experiment—pretest, experimental group intervention, and post-test. The other two groups do not receive the pretest, though one receives the intervention. All groups are given the post-test. Table 8.1 illustrates the features of each of the four groups in the Solomon four-group design. By having one set of experimental and control groups that complete the pretest (Groups 1 and 2) and another set that does not complete the pretest (Groups 3 and 4), researchers using the Solomon four-group design can account for testing effects in their analysis.
Group 1 | X | X | X |
Group 2 | X | X | |
Group 3 | X | X | |
Group 4 | X |
Solomon four-group designs are challenging to implement in the real world because they are time- and resource-intensive. Researchers must recruit enough participants to create four groups and implement interventions in two of them.
Overall, true experimental designs are sometimes difficult to implement in a real-world practice environment. It may be impossible to withhold treatment from a control group or randomly assign participants in a study. In these cases, pre-experimental and quasi-experimental designs–which we will discuss in the next section–can be used. However, the differences in rigor from true experimental designs leave their conclusions more open to critique.
Experimental design in macro-level research
You can imagine that social work researchers may be limited in their ability to use random assignment when examining the effects of governmental policy on individuals. For example, it is unlikely that a researcher could randomly assign some states to implement decriminalization of recreational marijuana and some states not to in order to assess the effects of the policy change. There are, however, important examples of policy experiments that use random assignment, including the Oregon Medicaid experiment. In the Oregon Medicaid experiment, the wait list for Oregon was so long, state officials conducted a lottery to see who from the wait list would receive Medicaid (Baicker et al., 2013). Researchers used the lottery as a natural experiment that included random assignment. People selected to be a part of Medicaid were the experimental group and those on the wait list were in the control group. There are some practical complications macro-level experiments, just as with other experiments. For example, the ethical concern with using people on a wait list as a control group exists in macro-level research just as it does in micro-level research.
Key Takeaways
- True experimental designs require random assignment.
- Control groups do not receive an intervention, and experimental groups receive an intervention.
- The basic components of a true experiment include a pretest, posttest, control group, and experimental group.
- Testing effects may cause researchers to use variations on the classic experimental design.
- Classic experimental design- uses random assignment, an experimental and control group, as well as pre- and posttesting
- Control group- the group in an experiment that does not receive the intervention
- Experiment- a method of data collection designed to test hypotheses under controlled conditions
- Experimental group- the group in an experiment that receives the intervention
- Posttest- a measurement taken after the intervention
- Posttest-only control group design- a type of experimental design that uses random assignment, and an experimental and control group, but does not use a pretest
- Pretest- a measurement taken prior to the intervention
- Random assignment-using a random process to assign people into experimental and control groups
- Solomon four-group design- uses random assignment, two experimental and two control groups, pretests for half of the groups, and posttests for all
- Testing effects- when a participant’s scores on a measure change because they have already been exposed to it
- True experiments- a group of experimental designs that contain independent and dependent variables, pretesting and post testing, and experimental and control groups
Image attributions
exam scientific experiment by mohamed_hassan CC-0
Foundations of Social Work Research Copyright © 2020 by Rebecca L. Mauldin is licensed under a Creative Commons Attribution-NonCommercial-ShareAlike 4.0 International License , except where otherwise noted.
Share This Book
✂️ The Future of Marketing Is Personal: Personalize Experiences at Scale with Ninetailed AI Platform Ninetailed AI →
Control Group
What is a control group in an experiment.
A control group is a set of subjects in an experiment who are not exposed to the independent variable. The purpose of a control group is to serve as a baseline for comparison. By having a group that is not exposed to the treatment, researchers can compare the results of the experimental group and determine whether the independent variable had an impact.
In some cases, there may be more than one control group. This is often done when there are multiple treatments or when researchers want to compare different groups of subjects. Having multiple control groups allows researchers to isolate the effect of each treatment and better understand how each one works.
Control groups are an important part of any experiment, as they help ensure that the results are accurate and reliable. Without a control group, it would be difficult to determine whether the results of an experiment are due to the independent variable or other factors.
When designing an experiment, it is important to carefully consider what kind of control group you will need. There are many different ways to set up a control group, and the best approach will depend on the specific goals of your research.
Control Group vs. Experimental Group
A control group is a group in an experiment that does not receive the experimental treatment. The purpose of a control group is to provide a baseline against which to compare the experimental group results.
An experimental group is a group in an experiment that receives the experimental treatment. The purpose of an experimental group is to test whether or not the experimental treatment has an effect.
The differences between control and experimental groups are important to consider when designing an experiment. The most important difference is that the control group provides a comparison for the results of the experimental group. This comparison is essential in order to determine whether or not the experimental treatment had an effect. Without a control group, it would be impossible to know if the results of the experiment are due to the treatment or not.
Another important difference between a control group and an experimental group is that the experimental group is the only group that receives the experimental treatment. This is necessary in order to ensure that any results seen in the experimental group can be attributed to the treatment and not to other factors.
Control groups and experimental groups are both essential parts of experiments. Without a control group, it would be impossible to know if the results of an experiment are due to the treatment or not. Without an experimental group, it would be impossible to test whether or not a treatment has an effect.
What Is the Purpose of a Control Group
The purpose of a control group is to serve as a baseline for comparison. By having a group that is not exposed to the treatment, researchers can compare the results of the experimental group and determine whether the independent variable had an impact.
Why Is a Control Group Important in an Experiment
A control group is an essential part of any experiment. It is a group of subjects who are not exposed to the independent variable being tested. The purpose of a control group is to provide a baseline against which the results from the treatment group can be compared.
Without a control group, it would be impossible to determine whether the results of an experiment are due to the treatment or some other factor. For example, imagine you are testing the effects of a new drug on patients with high blood pressure. If you did not have a control group, you would not know if the decrease in blood pressure was due to the drug or something else, such as the placebo effect.
A control group must be carefully designed to match the treatment group in all important respects, except for the one factor that is being tested. This ensures that any differences in the results can be attributed to the independent variable and not to other factors.
Get a weekly roundup of Ninetailed updates, curated posts, and helpful insights about the digital experience, MACH, composable, and more right into your inbox
Keep Reading on This Topic
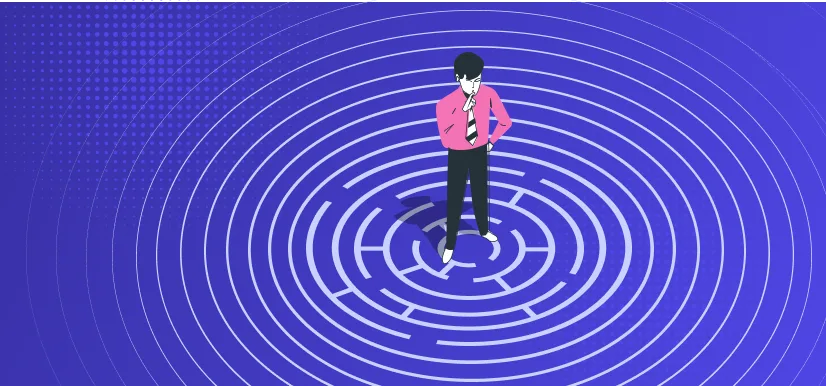
In this blog post, we will explore nine of the most common personalization challenges and discuss how to overcome them.
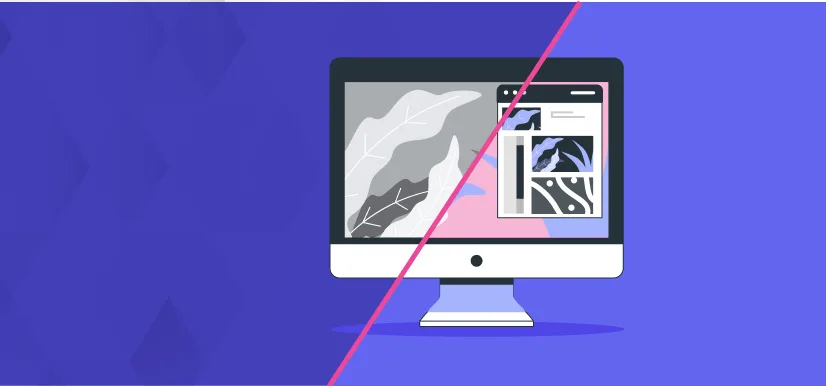
In this post, we will discuss some of the best practices and tips for using website content personalization to delight your customers and enhance user experiences.
Information
- Author Services
Initiatives
You are accessing a machine-readable page. In order to be human-readable, please install an RSS reader.
All articles published by MDPI are made immediately available worldwide under an open access license. No special permission is required to reuse all or part of the article published by MDPI, including figures and tables. For articles published under an open access Creative Common CC BY license, any part of the article may be reused without permission provided that the original article is clearly cited. For more information, please refer to https://www.mdpi.com/openaccess .
Feature papers represent the most advanced research with significant potential for high impact in the field. A Feature Paper should be a substantial original Article that involves several techniques or approaches, provides an outlook for future research directions and describes possible research applications.
Feature papers are submitted upon individual invitation or recommendation by the scientific editors and must receive positive feedback from the reviewers.
Editor’s Choice articles are based on recommendations by the scientific editors of MDPI journals from around the world. Editors select a small number of articles recently published in the journal that they believe will be particularly interesting to readers, or important in the respective research area. The aim is to provide a snapshot of some of the most exciting work published in the various research areas of the journal.
Original Submission Date Received: .
- Active Journals
- Find a Journal
- Proceedings Series
- For Authors
- For Reviewers
- For Editors
- For Librarians
- For Publishers
- For Societies
- For Conference Organizers
- Open Access Policy
- Institutional Open Access Program
- Special Issues Guidelines
- Editorial Process
- Research and Publication Ethics
- Article Processing Charges
- Testimonials
- Preprints.org
- SciProfiles
- Encyclopedia
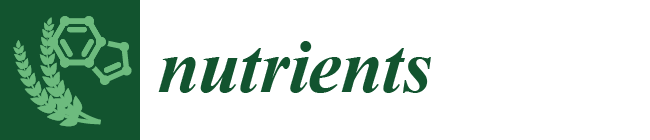
Article Menu
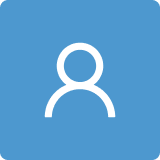
- Subscribe SciFeed
- Recommended Articles
- Google Scholar
- on Google Scholar
- Table of Contents
Find support for a specific problem in the support section of our website.
Please let us know what you think of our products and services.
Visit our dedicated information section to learn more about MDPI.
JSmol Viewer
Effectiveness of psychobiotic bifidobacterium breve bb05 in managing psychosomatic diarrhea in college students by regulating gut microbiota: a randomized, double-blind, placebo-controlled trial.
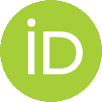
Graphical Abstract
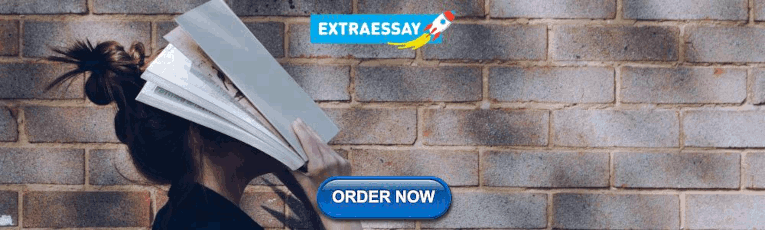
1. Introduction
2. materials and methods, 2.1. study design and ethical approval, 2.2. randomization and masking, 2.3. intervention procedure and management, 2.4. questionnaires, 2.5. enzyme-linked immunosorbent assay (elisa), 2.6. dna extraction and 16s rrna sequencing, 2.7. sample size, 2.8. statistical analysis, 3.1. diarrhea affects mental health and gut microbiota on college students (observational experiment), 3.1.1. baseline characteristics and scales results of participants in the observational experiment, 3.1.2. diarrhea and perturbance in gut microbial diversity and composition in college students, 3.2. b. breve bb05 intervention improves gut dysbiosis and mental health in diarrheal college students (intervention experiment), 3.2.1. baseline characteristics and the impact of b. breve bb05 on diarrhea symptoms and associated anxiety and depression, 3.2.2. b. breve bb05 supplement enriches and improves the compromised gut microbiota in diarrheal students, 3.3. correlation analysis among phenotypes, gut microbiota, and related fecal neurotransmitters, 4. discussion, 5. conclusions, author contributions, institutional review board statement, informed consent statement, data availability statement, conflicts of interest.
- Li, Y.; Xia, S.; Jiang, X.; Feng, C.; Gong, S.; Ma, J.; Fang, Z.; Yin, J.; Yin, Y. Gut microbiota and diarrhea: An updated review. Front. Cell. Infect. Microbiol. 2021 , 11 , 625210. [ Google Scholar ] [ CrossRef ] [ PubMed ]
- Barr, W.; Smith, A. Acute diarrhea. Am. Fam. Physician 2014 , 89 , 180–189. [ Google Scholar ] [ PubMed ]
- Grindrod, K.A.; Houle, S.K.D.; Fernandes, H. Traveler’s diarrhea. Can. Fam. Physician Med. Fam. Can. 2019 , 65 , 483–486. [ Google Scholar ]
- Yates, J. Traveler’s diarrhea. Am. Fam. Physician 2005 , 71 , 2095–2100. [ Google Scholar ] [ PubMed ]
- Riddle, M.S.; DuPont, H.L.; Connor, B.A. ACG Clinical Guideline: Diagnosis, treatment, and prevention of acute diarrheal infections in adults. Am. J. Gastroenterol. 2016 , 111 , 602–622. [ Google Scholar ] [ CrossRef ] [ PubMed ]
- Rogers, G.B.; Keating, D.J.; Young, R.L.; Wong, M.L.; Licinio, J.; Wesselingh, S. From gut dysbiosis to altered brain function and mental illness: Mechanisms and pathways. Mol. Psychiatry 2016 , 21 , 738–748. [ Google Scholar ] [ CrossRef ] [ PubMed ]
- Liu, L.; Wang, H.; Chen, X.; Zhang, Y.; Zhang, H.; Xie, P. Gut microbiota and its metabolites in depression: From pathogenesis to treatment. EBioMedicine 2023 , 90 , 104527. [ Google Scholar ] [ CrossRef ]
- Fujita, K.; Kaku, M.; Yanagase, Y.; Ezaki, T.; Furuse, K.; Ozawa, A.; Saidi, S.M.; Sang, W.K.; Waiyaki, P.G. Physicochemical characteristics and flora of diarrhoeal and recovery faeces in children with acute gastro-enteritis in Kenya. Ann. Trop. Paediatr. 1990 , 10 , 339–345. [ Google Scholar ] [ CrossRef ]
- Monira, S.; Nakamura, S.; Gotoh, K.; Izutsu, K.; Watanabe, H.; Alam, N.H.; Nakaya, T.; Horii, T.; Ali, S.I.; Iida, T.; et al. Metagenomic profile of gut microbiota in children during cholera and recovery. Gut Pathog. 2013 , 5 , 1. [ Google Scholar ] [ CrossRef ]
- Goralczyk-Binkowska, A.; Szmajda-Krygier, D.; Kozlowska, E. The Microbiota-Gut-Brain Axis in Psychiatric Disorders. Int. J. Mol. Sci. 2022 , 23 , 11245. [ Google Scholar ] [ CrossRef ]
- Huang, F.; Wu, X. Brain Neurotransmitter Modulation by Gut Microbiota in Anxiety and Depression. Front. Cell Dev. Biol. 2021 , 9 , 649103. [ Google Scholar ] [ CrossRef ] [ PubMed ]
- Margolis, K.G.; Cryan, J.F.; Mayer, E.A. The Microbiota-Gut-Brain Axis: From Motility to Mood. Gastroenterology 2021 , 160 , 1486–1501. [ Google Scholar ] [ CrossRef ] [ PubMed ]
- Kim, S.K.; Guevarra, R.B.; Kim, Y.T.; Kwon, J.; Kim, H.; Cho, J.H.; Kim, H.B.; Lee, J.H. Role of probiotics in human gut microbiome-associated diseases. J. Microbiol. Biotechnol. 2019 , 29 , 1335–1340. [ Google Scholar ] [ CrossRef ]
- Guarino, A.; Ashkenazi, S.; Gendrel, D.; Lo Vecchio, A.; Shamir, R.; Szajewska, H. European society for pediatric gastroenterology, hepatology, and nutrition/European society for pediatric infectious diseases evidence-based guidelines for the management of acute gastroenteritis in children in Europe: Update 2014. J. Pediatr. Gastroenterol. Nutr. 2014 , 59 , 132–152. [ Google Scholar ] [ CrossRef ] [ PubMed ]
- Chen, K.; Jin, S.; Ma, Y.; Cai, L.; Xu, P.; Nie, Y.; Luo, L.; Yu, Q.; Shen, Y.; Ma, W.; et al. Adjunctive efficacy of Lactis XLTG11 for Acute diarrhea in children: A randomized, blinded, placebo-controlled study. Nutrition 2023 , 111 , 112052. [ Google Scholar ] [ CrossRef ] [ PubMed ]
- Masuda, H.; Tanabe, Y.; Sakai, H.; Matsumoto, K.; Shimomura, A.; Doi, M.; Miyoshi, Y.; Takahashi, M.; Sagara, Y.; Tokunaga, S.; et al. Efficacy of probiotics and trimebutine maleate for abemaciclib-induced diarrhea: A randomized, open-label phase II trial (MERMAID, WJOG11318B). Breast 2023 , 71 , 22–28. [ Google Scholar ] [ CrossRef ] [ PubMed ]
- Rau, S.; Gregg, A.; Yaceczko, S.; Limketkai, B. Prebiotics and Probiotics for Gastrointestinal Disorders. Nutrients 2024 , 16 , 778. [ Google Scholar ] [ CrossRef ]
- Li, J.; Wang, J.; Wang, M.; Zheng, L.; Cen, Q.; Wang, F.; Zhu, L.; Pang, R.; Zhang, A. Bifidobacterium : A probiotic for the prevention and treatment of depression. Front. Microbiol. 2023 , 14 , 1174800. [ Google Scholar ] [ CrossRef ]
- Desbonnet, L.; Garrett, L.; Clarke, G.; Kiely, B.; Cryan, J.F.; Dinan, T.G. Effects of the probiotic Bifidobacterium infantis in the maternal separation model of depression. Neuroscience 2010 , 170 , 1179–1188. [ Google Scholar ] [ CrossRef ]
- Vork, L.; Wilms, E.; Penders, J.; Jonkers, D. Stool consistency: Looking beyond the bristol stool form scale. J. Neurogastroenterol. Motil. 2019 , 25 , 625. [ Google Scholar ] [ CrossRef ]
- Ren, W.; Qiu, H.; Yang, Y.; Zhu, X.; Zhu, C.; Mao, G.; Mao, S.; Lin, Y.; Shen, S.; Li, C.; et al. Randomized controlled trial of cognitive behavioural therapy for depressive and anxiety symptoms in Chinese women with breast cancer. Psychiatry Res. 2019 , 271 , 52–59. [ Google Scholar ] [ CrossRef ] [ PubMed ]
- Cooke, A.S.; Watt, K.A.; Albery, G.F.; Morgan, E.R.; Dungait, J.A.J. Lactoferrin quantification in cattle faeces by ELISA. PeerJ 2020 , 8 , e8631. [ Google Scholar ] [ CrossRef ] [ PubMed ]
- Lai, H.H.; Chiu, C.H.; Kong, M.S.; Chang, C.J.; Chen, C.C. Probiotic Lactobacillus casei: Effective for Managing Childhood Diarrhea by Altering Gut Microbiota and Attenuating Fecal Inflammatory Markers. Nutrients 2019 , 11 , 1150. [ Google Scholar ] [ CrossRef ] [ PubMed ]
- Kim, J.Y.; Lim, M.H. Psychological factors to predict chronic diarrhea and constipation in Korean high school students. Medicine 2021 , 100 , e26442. [ Google Scholar ] [ CrossRef ] [ PubMed ]
- Singh, P.; Nee, J. Role of diet in diarrhea-predominant irritable bowel syndrome. J. Clin. Gastroenterol. 2021 , 55 , 25–29. [ Google Scholar ] [ CrossRef ] [ PubMed ]
- Werner-Seidler, A.; Perry, Y.; Calear, A.L.; Newby, J.M.; Christensen, H. School-based depression and anxiety prevention programs for young people: A systematic review and meta-analysis. Clin. Psychol. Rev. 2017 , 51 , 30–47. [ Google Scholar ] [ CrossRef ] [ PubMed ]
- Socała, K.; Doboszewska, U.; Szopa, A.; Serefko, A.; Włodarczyk, M.; Zielińska, A.; Poleszak, E.; Fichna, J.; Wlaź, P. The role of microbiota-gut-brain axis in neuropsychiatric and neurological disorders. Pharmacol. Res. 2021 , 172 , 105840. [ Google Scholar ] [ CrossRef ] [ PubMed ]
- Mayer, E.A.; Nance, K.; Chen, S. The gut-brain axis. Annu. Rev. Med. 2022 , 73 , 439–453. [ Google Scholar ] [ CrossRef ] [ PubMed ]
- Hou, K.; Wu, Z.X.; Chen, X.Y.; Wang, J.Q.; Zhang, D.; Xiao, C.; Zhu, D.; Koya, J.B.; Wei, L.; Li, J.; et al. Microbiota in health and diseases. Signal Transduct. Target. Ther. 2022 , 7 , 135. [ Google Scholar ] [ CrossRef ]
- Sharp, R. The Hamilton rating scale for depression. Occup. Med. 2015 , 65 , 340. [ Google Scholar ] [ CrossRef ]
- Zhang, W.; Yan, Y.; Wu, Y.; Yang, H.; Zhu, P.; Yan, F.; Zhao, R.; Tian, P.; Wang, T.; Fan, Q.; et al. Medicinal herbs for the treatment of anxiety: A systematic review and network meta-analysis. Pharmacol. Res. 2022 , 179 , 106204. [ Google Scholar ] [ CrossRef ] [ PubMed ]
- Longstreth, G.F.; Thompson, W.G.; Chey, W.D.; Houghton, L.A.; Mearin, F.; Spiller, R.C. Functional bowel disorders. Gastroenterology 2006 , 130 , 1480–1491. [ Google Scholar ] [ CrossRef ] [ PubMed ]
- Gallo, A.; Passaro, G.; Gasbarrini, A.; Landolfi, R.; Montalto, M. Modulation of microbiota as treatment for intestinal inflammatory disorders: An uptodate. World J. Gastroenterol. 2016 , 22 , 7186–7202. [ Google Scholar ] [ CrossRef ] [ PubMed ]
- Choi, Y.J.; Shin, S.H.; Shin, H.S. Immunomodulatory effects of Bifidobacterium spp. and Use of Bifidobacterium breve and Bifidobacterium longum on acute diarrhea in children. J. Microbiol. Biotechnol. 2022 , 32 , 1186–1194. [ Google Scholar ] [ CrossRef ] [ PubMed ]
- Tian, P.; Chen, Y.; Zhu, H.; Wang, L.; Qian, X.; Zou, R.; Zhao, J.; Zhang, H.; Qian, L.; Wang, Q.; et al. Bifidobacterium breve CCFM1025 attenuates major depression disorder via regulating gut microbiome and tryptophan metabolism: A randomized clinical trial. Brain Behav. Immun. 2022 , 100 , 233–241. [ Google Scholar ] [ CrossRef ] [ PubMed ]
- Wu, F.; Guo, X.; Zhang, J.; Zhang, M.; Ou, Z.; Peng, Y. Phascolarctobacterium faecium abundant colonization in human gastrointestinal tract. Exp. Ther. Med. 2017 , 14 , 3122–3126. [ Google Scholar ] [ CrossRef ] [ PubMed ]
- Zhou, J.; Zhang, Q.; Zhao, Y.; Zou, Y.; Chen, M.; Zhou, S.; Wang, Z. The relationship of Megamonas species with nonalcoholic fatty liver disease in children and adolescents revealed by metagenomics of gut microbiota. Sci. Rep. 2022 , 12 , 22001. [ Google Scholar ] [ CrossRef ] [ PubMed ]
- Cryan, J.F.; O’Riordan, K.J.; Cowan, C.S.M.; Sandhu, K.V.; Bastiaanssen, T.F.S.; Boehme, M.; Codagnone, M.G.; Cussotto, S.; Fulling, C.; Golubeva, A.V.; et al. The microbiota-gut-brain axis. Physiol. Rev. 2019 , 99 , 1877–2013. [ Google Scholar ] [ CrossRef ] [ PubMed ]
- Osadchiy, V.; Martin, C.R.; Mayer, E.A. Gut microbiome and modulation of CNS function. Compr. Physiol. 2019 , 10 , 57–72. [ Google Scholar ] [ CrossRef ]
- Zheng, L.; Kelly, C.J.; Battista, K.D.; Schaefer, R.; Lanis, J.M.; Alexeev, E.E.; Wang, R.X.; Onyiah, J.C.; Kominsky, D.J.; Colgan, S.P. Microbial-Derived Butyrate Promotes Epithelial Barrier Function through IL-10 Receptor-Dependent Repression of Claudin-2. J. Immunol. 2017 , 199 , 2976–2984. [ Google Scholar ] [ CrossRef ]
- Chen, G.; Ran, X.; Li, B.; Li, Y.; He, D.; Huang, B.; Fu, S.; Liu, J.; Wang, W. Sodium Butyrate Inhibits Inflammation and Maintains Epithelium Barrier Integrity in a TNBS-induced Inflammatory Bowel Disease Mice Model. EBioMedicine 2018 , 30 , 317–325. [ Google Scholar ] [ CrossRef ] [ PubMed ]
- Sanmarco, L.M.; Wheeler, M.A.; Gutiérrez-Vázquez, C.; Polonio, C.M.; Linnerbauer, M.; Pinho-Ribeiro, F.A.; Li, Z.; Giovannoni, F.; Batterman, K.V.; Scalisi, G.; et al. Gut-licensed IFNγ(+) NK cells drive LAMP1(+)TRAIL(+) anti-inflammatory astrocytes. Nature 2021 , 590 , 473–479. [ Google Scholar ] [ CrossRef ] [ PubMed ]
- Fitzpatrick, Z.; Frazer, G.; Ferro, A.; Clare, S.; Bouladoux, N.; Ferdinand, J.; Tuong, Z.K.; Negro-Demontel, M.L.; Kumar, N.; Suchanek, O.; et al. Gut-educated IgA plasma cells defend the meningeal venous sinuses. Nature 2020 , 587 , 472–476. [ Google Scholar ] [ CrossRef ] [ PubMed ]
- Morris, G.; Berk, M.; Carvalho, A.; Caso, J.R.; Sanz, Y.; Walder, K.; Maes, M. The Role of the Microbial Metabolites Including Tryptophan Catabolites and Short Chain Fatty Acids in the Pathophysiology of Immune-Inflammatory and Neuroimmune Disease. Mol. Neurobiol. 2017 , 54 , 4432–4451. [ Google Scholar ] [ CrossRef ] [ PubMed ]
- Sharon, G.; Cruz, N.J.; Kang, D.W.; Gandal, M.J.; Wang, B.; Kim, Y.M.; Zink, E.M.; Casey, C.P.; Taylor, B.C.; Lane, C.J.; et al. Human Gut Microbiota from Autism Spectrum Disorder Promote Behavioral Symptoms in Mice. Cell 2019 , 177 , 1600–1618.e1617. [ Google Scholar ] [ CrossRef ] [ PubMed ]
- Buffington, S.A.; Dooling, S.W.; Sgritta, M.; Noecker, C.; Murillo, O.D.; Felice, D.F.; Turnbaugh, P.J.; Costa-Mattioli, M. Dissecting the contribution of host genetics and the microbiome in complex behaviors. Cell 2021 , 184 , 1740–1756.e1716. [ Google Scholar ] [ CrossRef ] [ PubMed ]
- Wang, Q.; Yang, Q.; Liu, X. The microbiota-gut-brain axis and neurodevelopmental disorders. Protein Cell 2023 , 14 , 762–775. [ Google Scholar ] [ CrossRef ] [ PubMed ]
- Bruckner, J.J.; Stednitz, S.J.; Grice, M.Z.; Zaidan, D.; Massaquoi, M.S.; Larsch, J.; Tallafuss, A.; Guillemin, K.; Washbourne, P.; Eisen, J.S. The microbiota promotes social behavior by modulating microglial remodeling of forebrain neurons. PLoS Biol. 2022 , 20 , e3001838. [ Google Scholar ] [ CrossRef ] [ PubMed ]
- Guida, F.; Turco, F.; Iannotta, M.; De Gregorio, D.; Palumbo, I.; Sarnelli, G.; Furiano, A.; Napolitano, F.; Boccella, S.; Luongo, L.; et al. Antibiotic-induced microbiota perturbation causes gut endocannabinoidome changes, hippocampal neuroglial reorganization and depression in mice. Brain Behav. Immun. 2018 , 67 , 230–245. [ Google Scholar ] [ CrossRef ]
- Sushma, G.; Vaidya, B.; Sharma, S.; Devabattula, G.; Bishnoi, M.; Kondepudi, K.K.; Sharma, S.S. Bifidobacterium breve Bif11 supplementation improves depression-related neurobehavioural and neuroinflammatory changes in the mouse. Neuropharmacology 2023 , 229 , 109480. [ Google Scholar ] [ CrossRef ]
- Baldwin, D.; Rudge, S. The role of serotonin in depression and anxiety. Int. Clin. Psychopharmacol. 1995 , 9 (Suppl. S4), 41–45. [ Google Scholar ] [ CrossRef ] [ PubMed ]
- Yano, J.M.; Yu, K.; Donaldson, G.P.; Shastri, G.G.; Ann, P.; Ma, L.; Nagler, C.R.; Ismagilov, R.F.; Mazmanian, S.K.; Hsiao, E.Y. Indigenous bacteria from the gut microbiota regulate host serotonin biosynthesis. Cell 2015 , 161 , 264–276. [ Google Scholar ] [ CrossRef ] [ PubMed ]
- Mineur, Y.S.; Picciotto, M.R. The role of acetylcholine in negative encoding bias: Too much of a good thing? Eur. J. Neurosci. 2021 , 53 , 114–125. [ Google Scholar ] [ CrossRef ] [ PubMed ]
- Zheng, F.; Zhou, Q.; Cao, Y.; Shi, H.; Wu, H.; Zhang, B.; Huang, F.; Wu, X. P2Y(12) deficiency in mouse impairs noradrenergic system in brain, and alters anxiety-like neurobehavior and memory. Genes. Brain Behav. 2019 , 18 , e12458. [ Google Scholar ] [ CrossRef ] [ PubMed ]
- Sobko, T.; Liang, S.; Cheng, W.H.G.; Tun, H.M. Impact of outdoor nature-related activities on gut microbiota, fecal serotonin, and perceived stress in preschool children: The Play&Grow randomized controlled trial. Sci. Rep. 2020 , 10 , 21993. [ Google Scholar ] [ CrossRef ] [ PubMed ]
- Rauf, A.; Khalil, A.A.; Rahman, U.U.; Khalid, A.; Naz, S.; Shariati, M.A.; Rebezov, M.; Urtecho, E.Z.; de Albuquerque, R.; Anwar, S.; et al. Recent advances in the therapeutic application of short-chain fatty acids (SCFAs): An updated review. Crit. Rev. Food Sci. Nutr. 2022 , 62 , 6034–6054. [ Google Scholar ] [ CrossRef ]
- Xu, B.; Wang, Z.; Wang, Y.; Zhang, K.; Li, J.; Zhou, L.; Li, B. Milk-derived Lactobacillus with high production of short-chain fatty acids relieves antibiotic-induced diarrhea in mice. Food Funct. 2024 , 15 , 5329–5342. [ Google Scholar ] [ CrossRef ]
Click here to enlarge figure
Characteristics | C Group (n = 50) | M Group (n = 50) | p Value |
---|---|---|---|
Age | 19.70 ± 0.92 | 19.4 ± 0.86 | / |
BMI | 22.34 ± 1.97 | 22.58 ± 1.19 | / |
Female:Male (n:n) | 1:1 (25.00:25.00) | 1:1 (25.00:25.00) | / |
HAMA-14 | 1.00 ± 0.91 | 4.60 ± 3.03 ** | <0.01 |
HDRS-17 | 0.86 ± 0.93 | 3.33 ± 1.88 ** | <0.01 |
BSS | 3.70 ± 0.76 | 5.90 ± 1.03 ** | <0.01 |
Characteristics | MP Group (n = 50) | p Value | MB Group (n = 50) | p Value | ||
---|---|---|---|---|---|---|
Week 0 | Week 2 | Week 0 | Week 2 | |||
Age | 19.43 ± 1.04 | / | 19.63 ± 0.81 | / | ||
BMI | 22.40 ± 1.72 | / | 21.77 ± 1.64 | / | ||
Female:Male (n:n) | 1:1 (25.00:25.00) | / | 1:1 (25.00:25.00) | / | ||
HAMA-14 | 5.42 ± 2.41 | 4.74 ± 1.99 | 0.1314 | 5.86 ± 2.65 | 0.38 ± 0.75 ** | <0.01 |
HDRS-17 | 5.50 ± 2.32 | 4.70 ± 1.81 | 0.0865 | 6.12 ± 2.98 | 0.58 ± 1.37 ** | <0.01 |
BSS | 5.78 ± 0.91 | 4.62 ± 0.83 *** | <0.001 | 6.10 ± 0.76 | 3.66 ± 0.59 ** | <0.01 |
The statements, opinions and data contained in all publications are solely those of the individual author(s) and contributor(s) and not of MDPI and/or the editor(s). MDPI and/or the editor(s) disclaim responsibility for any injury to people or property resulting from any ideas, methods, instructions or products referred to in the content. |
Share and Cite
Wang, Y.; Wang, Y.; Ding, K.; Liu, Y.; Liu, D.; Chen, W.; Zhang, X.; Luo, C.; Zhang, H.; Xu, T.; et al. Effectiveness of Psychobiotic Bifidobacterium breve BB05 in Managing Psychosomatic Diarrhea in College Students by Regulating Gut Microbiota: A Randomized, Double-Blind, Placebo-Controlled Trial. Nutrients 2024 , 16 , 1989. https://doi.org/10.3390/nu16131989
Wang Y, Wang Y, Ding K, Liu Y, Liu D, Chen W, Zhang X, Luo C, Zhang H, Xu T, et al. Effectiveness of Psychobiotic Bifidobacterium breve BB05 in Managing Psychosomatic Diarrhea in College Students by Regulating Gut Microbiota: A Randomized, Double-Blind, Placebo-Controlled Trial. Nutrients . 2024; 16(13):1989. https://doi.org/10.3390/nu16131989
Wang, Yufan, Yufei Wang, Kunpeng Ding, Yuhan Liu, Dingming Liu, Weijun Chen, Xinyi Zhang, Chuanlin Luo, Hongyan Zhang, Tangchang Xu, and et al. 2024. "Effectiveness of Psychobiotic Bifidobacterium breve BB05 in Managing Psychosomatic Diarrhea in College Students by Regulating Gut Microbiota: A Randomized, Double-Blind, Placebo-Controlled Trial" Nutrients 16, no. 13: 1989. https://doi.org/10.3390/nu16131989
Article Metrics
Article access statistics, further information, mdpi initiatives, follow mdpi.
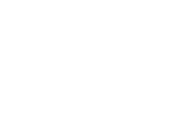
Subscribe to receive issue release notifications and newsletters from MDPI journals
- Open access
- Published: 19 June 2024
Effects of life-story review on quality of life, depression, and life satisfaction in older adults in Oman: a randomized controlled study
- Bushra Rashid Al-Ghafri 1 ,
- Hamed Al-Sinawi 2 ,
- Ahmed Mohammed Al-Harrasi 2 ,
- Yaqoub Al-Saidi 1 ,
- Abdulaziz Al-Mahrezi ORCID: orcid.org/0000-0003-2359-7498 3 ,
- Zahir Badar Al-Ghusaini 4 ,
- Khalfan Bakhit Rashid Al-Zeedy 5 &
- Moon Fai Chan ORCID: orcid.org/0000-0002-2899-3118 1
BMC Geriatrics volume 24 , Article number: 530 ( 2024 ) Cite this article
160 Accesses
1 Altmetric
Metrics details
There is a need for healthcare providers to develop life-story review interventions to enhance the mental well-being and quality of life of older adults. The primary aim of this study is to examine the effects of telling their life stories and creating a life-story book intervention on QoL, depressive symptoms, and life satisfaction in a group of older adults in Oman.
A repeated-measures randomized controlled design was conducted in Oman. A total of 75 older adults (response rate = 40.1%) were randomly assigned to the intervention ( n = 38) or control ( n = 37) groups. Demographic data were collected as the baseline. Depression, life satisfaction, and quality of life scores were collected from each participant at weeks 1, 2, 3, 4, and 8.
Their average age is 67.3 ± 5.5 years (range 60–82 years). There are more women ( n = 50, 66.7%) than men. Over the 8 weeks, the intervention group exhibited a notable decrease in depression (intervention: 2.5 ± 1.2 vs. control: 5.3 ± 2.1, p < .001) but an increase in life satisfaction (24.6 ± 3.1 vs. 21.9 ± 6.1, p < .001) and quality of life (physical: 76.2 ± 12.7 vs. 53.6 ± 15.5, p < .001; psychological: 76.4 ± 12.1 vs. 59.9 ± 21.5, p < .001; Social relation: 78.3 ± 11.7 vs. 61.8 ± 16.6, p < .001; environment: 70.8 ± 10.2 vs. 58.6 ± 16.1, p < .001) compared to the control group.
The life-story review intervention proved effective in diminishing depression and boosting life satisfaction and quality of life among the older sample within the 8-week study. Healthcare providers can apply such interventions to improve older adults’ mental health and well-being.
Peer Review reports
The World Health Organization has determined 4 domains for QoL: physical health, psychological, social relationships, and environment [ 1 ]. QoL in older adults is a critical aspect of senior care and is influenced by these domains. Each of these domains plays a crucial role in the overall QoL of older adults. It expands the definition of health to include a personal sense of physical and mental health, social functioning, and emotional well-being. Physical activity, living environment, and diet are key self-care behaviors contributing to health and QoL [ 2 ]. Jelicic & Kempen [ 3 ] examined 5,279 community-dwelling older adults and found that the lower the life quality is, the more chronic conditions older adults have. Physical health includes mobility, daily activities, functional capacity, energy, pain, and sleep [ 4 ]. Psychological well-being includes self-image, negative thoughts, positive attitudes, self-esteem, and mental status [ 5 ]. As depression levels rise, QoL levels in older adults will reduce [ 6 ], and when their life satisfaction levels rise, their QoL levels will improve [ 7 ]. A study in China found that higher life satisfaction of older adults can enhance the effects of social support on their social relationships, which reduces depressive symptoms [ 8 ]. A systematic review revealed 286 empirical studies showing that social networks and a sense of well-being are positively associated with life satisfaction in older adults [ 9 ]. QoL measures permit researchers to compare the status of different groups over time and assess the effectiveness of public health interventions [ 7 ]. Environmental factors also play a significant role in the QoL of older adults [ 5 ]. These four main factors influence the QoL of older adults, and understanding these can help develop strategies, care, and support to improve their QoL.
Previous researchers [ 10 , 11 , 12 , 13 ] have identified various factors influencing older adults’ life satisfaction. It has been found that participation in exercise or physical activities among older adults is a complementary factor that can boost life satisfaction levels [ 10 ]. A study in Poland found that seniors who participated were satisfied with their lives [ 11 ]. Another study in Oman, a 4-week follow-up study, surveyed a group of older adults in a community setting, and it reported no significant change in their life satisfaction levels [ 12 ]. However, a study reports that when depression levels rose, life satisfaction correspondingly decreased [ 11 ]. Another study of older adults in Korea reports that the living environment positively impacts life satisfaction [ 13 ]. These insights can be useful in enhancing life satisfaction levels by implementing suitable programs that promote healthy lifestyles among older adults [ 10 ].
Depression is a mental health condition that can affect how individuals feel, act, and think [ 14 ]. Depressive symptoms are a common problem among older adults, and they often go along with chronic illnesses that have significant impacts on their QoL [ 6 , 15 ]. Previous studies have shown that many older adults reported lower QoL and life satisfaction but higher depressive symptoms [ 16 ]. A study in China of older adults found that depression reduces an older person’s physical activities and social network because they may lose interest in the things they normally enjoy [ 17 ]. A study in Oman reported a 16.9% prevalence rate of depression among Omani older adults, which is expected to be much higher in the upcoming decade due to an aging problem [ 18 ]. A qualitative study found that Omani older adults who are depressed or experiencing a crisis may suffer from emotional and bodily disturbances and find it difficult to express themselves verbally [ 19 ].
There is a need for healthcare providers to develop interventions to improve the physical health, mental well-being, and overall QoL of older adults. Sharing one’s life story has been recommended to alleviate depressive symptoms and enhance QoL and life satisfaction among older adults [ 20 , 21 ]. A life-story work is a “ term given to biographical approaches in health and social care settings that give people time to share their memories and talk about their life experiences ” [ 22 ]. Wills & Day [ 23 ] asserted that people long to narrate their life stories, whether past or present, which correspond with their life experiences. These stories are influenced by social, political, and economic factors and culture, religion, and relationships, which confers a unique personality and identity [ 24 , 25 ]. Some researchers suggested that reminiscence might provide a mechanism to facilitate adaptation and produce continuity in inner psychological characteristics, social behavior, and social circumstances [ 26 ]. Reminiscence is a psychosocial intervention focusing on remembering and sharing past stories that reinforce a sense of identity and self-worth. While reminiscence focuses on sharing stories and improving social interaction, life review aims to create a meaningful life story that integrates positive and negative experiences. A meta-analysis examining the effects of reminiscence interventions reveals a broad range of outcomes. Specifically, it shows moderate improvements in ego integrity and depression. However, the effects on purpose in life satisfaction and positive well-being were smaller than those in the control group [ 9 ]. Reminiscence and life review could empower individuals to find solace, meaning, and coherence while reflecting on their life journey. The foundational theoretical model for life review integrates the psychosocial development theory [ 27 ] and Butler’s life review theory [ 28 ]. This combined approach has been used in previous studies [ 16 , 20 ] and forms the basis for the current study. By examining various life events, individuals can discover a sense of unity, purpose, and significance in their lives, reinforcing their identity and self-promotion [ 29 ]. The study findings revealed that older adults in the life story review group exhibited fewer depressive symptoms, reduced feelings of hopelessness, and improved life satisfaction compared to those in the control group [ 29 ]. This concept is encapsulated in the Theory of Narrative Identity [ 24 ]. Life-story books, typically filled with photos, keepsakes, and personal histories, are therapeutic by facilitating better communication among healthcare providers, clients, and relatives [ 30 ]. The study findings indicated that older adults who created life-story books experienced an increase in ego integrity, and their mental health showed signs of improvement after participating in the intervention [ 30 ]. Creating a life storybook offers a safe way to articulate deep-seated emotions and achieve emotional release [ 21 ]. Furthermore, no local research has been conducted on using life story reviews and creating life-story books as a healthcare intervention among older adults in Oman.
The primary aim of this study is to examine the effects of telling their life stories and creating a life-story book intervention on QoL, depressive symptoms, and life satisfaction in a group of older adults in Oman.
There is a statistically significant difference in each outcome measure between groups during the 8-week study;
There is a statistically significant difference in each outcome measure on the 5 time points for each group.
This is a repeated-measures experimental randomized control design with two groups of older Oman adults living in the community. The flow chart of this study is shown in Fig. 1 .
Participants and ethical consideration
Inclusion criteria are Omani adults aged 60 or above who can communicate in Arabic or English and provide signed consent. In Oman, those aged 60 years and above were considered older adults [ 18 , 19 ]. Those elderly who are diagnosed with Alzheimer’s disease, other neurocognitive disorders, Parkinson’s disease, or a major mental disorder, those using sleep medications, and those unwilling to be audio-recorded in the intervention group were excluded. This study was approved by the Sultan Qaboos University Medical Research Ethics Committee (MREC #2028), and all participants are required to provide written or verbal informed consent.
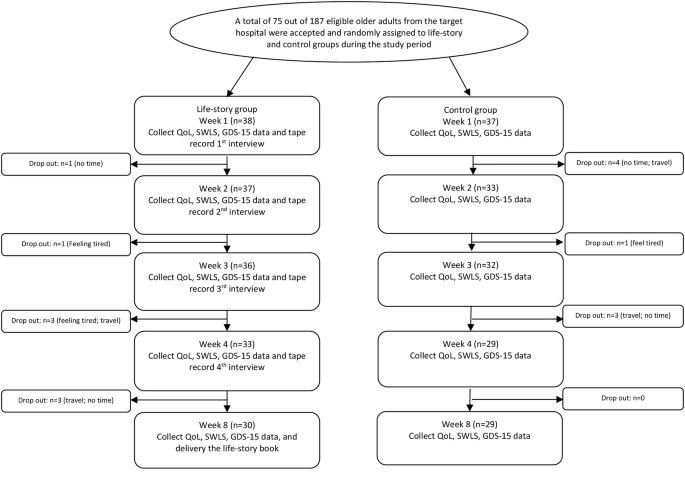
Flow chart of the study
Power analysis
The power analysis for this study is grounded on depression scores, one of the primary outcomes. A design involving repeated measures with two groups was employed, and the PASS statistical software was utilized to determine the necessary sample sizes [ 31 ]. Based on a prior meta-analysis conducted by our research team [ 32 ], this study anticipates effect sizes of 0.32, 0.47, and 0.47 for the between-group (2 groups), within-time (5 times) and interaction of the between-group and within-time factors, respectively. The study requires 38 subjects in each group, totaling 76 subjects. This will result in power levels of 79%, 92%, and 92% for the between-group, within-time, and interaction factors, respectively, at a 5% significance level.
Outcome measures
The study instrument was divided into two parts:
Demographic characteristics: Age, gender, marital status, educational level, and medical history were collected as baseline information for all participants [ 21 , 22 ].
Quality of life (QoL): The participants’ QoL levels were evaluated using the Arabic version of the World Health Organization quality of life questionnaire (WHOQoL-BREF) [ 33 , 34 ]. It is a 26-item, and each item has a 5-point scale that is divided into 4 aspects (social relationships, physical, environment, and psychological) and two items on perceived “Satisfaction with health” and “General rating of QoL”. The Arabic version has an acceptable Cronbach’s alpha = 0.7 [ 34 ], and percentage scores range from 0 to 100, with higher scores indicating better quality of life in each domain and item.
Life satisfaction: The Arabic version of the Satisfaction with Life Scale (SWLS) [ 35 ] collected the participants’ life satisfaction levels. This tool has good reliability ( a = 0.86) and is composed of 5 questions, each with a response option on a scale from 1 (strongly disagree) to 7 (strongly agree). The overall score can vary between 5 and 35, with a higher score indicating higher life satisfaction.
Depressive symptoms: The participants’ depression levels were measured by the Arabic version of the Geriatric Depression Scale (GDS-15) [ 36 ]. This tool has good reliability (a = 0.88) and includes 15 fixed-response questions that gauge the emotional state of older adults over the past week. The cumulative score is calculated, with higher totals reflecting more intense symptoms of depression.
Data collection procedure
After ethical approval from the University, the recruitment will take place at the inpatient clinics of the Sultan Qaboos University Hospital. A researcher, the first author, was present in the clinics and extended invitations to potential subjects while they awaited their appointments. Subjects willing to join the study were randomly assigned to either an intervention or a control group. The first author was trained by an experienced healthcare professional, the co-author. She also asked to conduct a pilot study by recruiting 1 subject per group to ensure the data collection and logistic process follows the protocol. The researcher explained the roles to each participant in the study, and written consent was obtained before the interviews. Participants’ identities were protected because all data were identified only by case numbers. Participants were told that they could withdraw from the study at any time. For both groups, outcomes were collected at five time points: baseline (week 1), week 2, week 3, week 4, and week 8.
Intervention group
The intervention group had five meetings, as detailed in Fig. 1 . The first four meetings were home interviews, where the older participants reviewed one life stage each: childhood, adolescence, adulthood, and current life. The guiding questions for each stage were based on Chan et al. [ 20 ], Erikson’s [ 27 ], and Butler’s [ 28 ] theories of psychosocial development. The researchers drafted the story from the verbatim transcripts after each meeting. During the interviews, participants are prompted with guiding questions to encourage them to express their emotions and share their narratives. After the interviews were completed, transcripts were created and consolidated. The participants proofread the story in the next meeting, which enhanced their memory and agency [ 23 ]. At the fourth meeting, the participants saw and revised their life-story book with their chosen photos. At the fifth meeting, the participants received their life storybooks.
Control group
The control and intervention groups had the same encounter times. However, the life-story interview was exclusive to the intervention group; the control group did not participate in this activity. Participants were required to complete assessments for three outcome measures in these meetings. To avoid studying contamination, the researcher minimized other conversations or discussions with subjects about life issues.
Randomization
Participants who fulfilled the eligibility criteria and expressed interest in participating were chosen from the hospital’s outpatient clinics. Each participant was given a unique identification number. Based on factors such as age and gender, they were randomly allocated to either the intervention or control group. An online software, Research Randomizer [ 37 ], generated a list of 38 unique numbers ranging from 1 to 76. Participants whose identification numbers matched those on the list were assigned to the intervention group, while the remaining participants were placed in the control group. This is not a double-blind or single-blind study because the participants and the researcher know who receives a life story or control group.
Statistical analysis
The analysis was divided into three parts. Part one uses descriptive statistics (e.g., mean, percentage, standard deviation) to explore the profile of the participants. Part two employs univariate analysis (e.g., t-test, c² test) to compare demographic characteristics between the two groups to ensure the homogeneity of their demographic characteristics. Part three is to address the two main hypotheses of this study. Considering that the outcomes we collected were time-dependent and demographic characteristics could potentially influence these outcomes, we employed a Generalized Estimating Equation (GEE) model. The GEE is a well-known method for analyzing longitudinal data, and it does not necessitate complete data at all time points. No imputation is required to replace missing values for further analysis [ 38 ]. In addition, the GEE model permitted the inclusion of demographic factors (e.g., gender, age) that could be used to adjust the results of each outcome [ 21 ]. The Wald χ 2 statistics were used to examine any significant differences between groups at each time point and within times for each group for each outcome. All analyses were conducted using IBM SPSS v23, and a 5% significance level was set for this study.
Participant characteristics
Out of 187 eligible older adults, 75 participated in the study, resulting in a response rate of 40.1% between October 2021 and November 2023. The primary reasons for refusal included lack of interest, time constraints, and family members’ restrictions. Thirty-eight and thirty-seven were randomly allocated to the intervention and control groups. However, 16 (8 from the intervention group and 8 from the control group) dropped out due to personal reasons, including no time or going for long travel. Table 1 shows the median age of participants is 67.0, and the range is 60–82 years, with an average age of 67.3 ± 5.5 years. There are more women ( n = 50, 66.7%) than men. More than 78% ( n = 59) of them had to take medication due to chronic diseases. For those with chronic illness, 71.2% ( n = 42) had diabetes mellitus, 69.5% ( n = 41) suffered from hypertension, 52.5% ( n = 31) had cholesterol, and 18.6% ( n = 11) suffered from cardiovascular diseases. Participants met their relatives ( n = 54, 72.0%) at least once a week, but 53.4% ( n = 40) met friends less than once a week. In addition, more than half of them ( n = 30) do not do any physical activity every week. The demographic characteristics of the two groups exhibited no statistically significant differences (Table 1 ).
The effect of the life-story Intervention on depression and life satisfaction compared with the control over time
In Table 2 , the baseline measures of depression levels between the two groups were compared. The intervention group (6.4 ± 0.7) had a significantly higher depression level than the control group (5.2 ± 1.7, t = 4.107, p < .001). Still, the GEE results show that there is a greater reduction in depression levels in older adults in the intervention group than in the control group on week 4 (Intervention: 3.5 ± 0.9 vs. Control: 5.3 ± 1.7, χ 2 = 83.583, p < .001) and week 8 (2.5 ± 1.2 vs. 5.3 ± 2.1, χ 2 = 108.726, p < .001). Within weeks, the depression score in the life-story group reduced dramatically (baseline vs. week 4, p < .001; baseline vs. week 8, p < .001), while the depression score in the control group remained with no significant change (baseline vs. week 4, p = .550; baseline vs. week 8, p = .850) (Fig. 2 a).
On the life satisfaction scores, in the beginning, participants in the intervention group had a significantly lower life satisfaction level (15.3 ± 3.1) than the control group (24.2 ± 4.5, t = 9.967, p < .001). In the GEE analysis, the life-story group obtained a significantly higher score than the control groups in week 4 (Intervention: 21.9 ± 2.8 vs. Control: 22.6 ± 5.5, χ 2 = 101.870, p < .001) and week 8 (24.6 ± 3.1 vs. 21.9 ± 6.1, χ 2 = 167.846, p < .001). Over 8-week period, the life satisfaction score in the life-story group improved from week 1 (15.3 ± 3.1) to week 4 (21.9 ± 2.8, p < .001) and week 8 (24.6 ± 3.1, p < .001), while the life satisfaction score in the control group remained unchanged statistically from baseline (24.2 ± 4.5) to week 4 (22.6 ± 5.5, p = .253) until in week 8 (21.9 ± 6.1, p = .023). However, these changes were lower than the intervention group (Fig. 2 b).
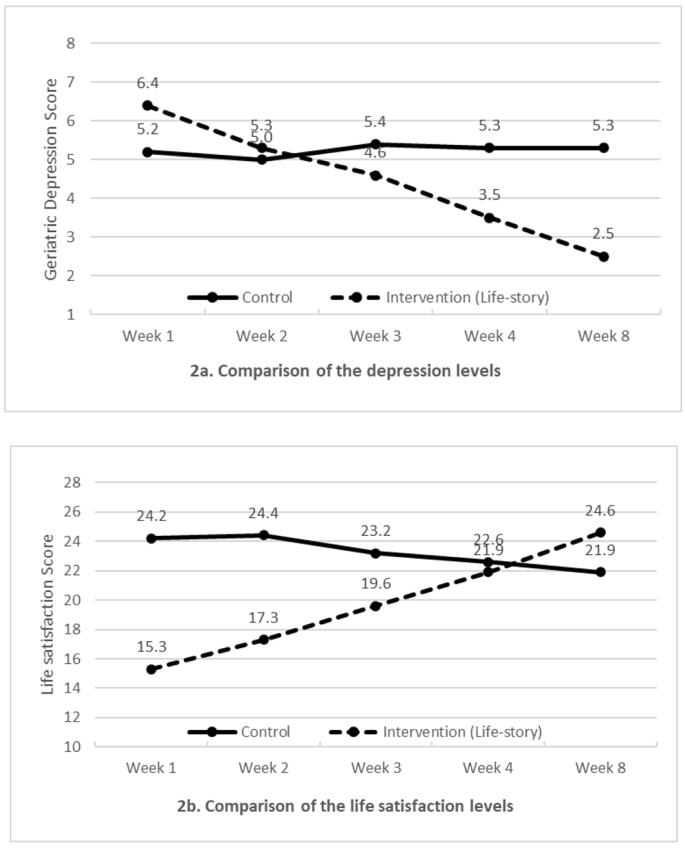
Comparison of the average depression and life satisfaction levels between older adults in the life-story and control groups
The effect of the life-story intervention on quality of life compared with the control over time
Quality of life (WHOQOL-BREF) scores were compared between the intervention and control groups (Table 3 ). There are 4 sub-domains (physical, psychological, social relationship, and environment). The general quality of life and satisfaction with health score were used to compare the two groups over an 8-week study.
In the physical domain, the life-story group obtained a significantly higher score than the control groups during the 8-week study, especially in week 4 (71.4 ± 10.7 vs. 54.9 ± 15.2, χ 2 = 104.589, p < .001) and week 8 (76.2 ± 12.7 vs. 53.6 ± 15.5, χ 2 = 127.000, p < .001). Within 8 weeks, older adults’ physical domain score in the life-story group improved dramatically from baseline (46.9 ± 11.7) to week 8 (76.2 ± 12.7, p < .001), while no significant change (baseline: 55.9 ± 15.9 vs. week 8: 53.6 ± 15.5, p = .494) on the control group (Fig. 3 a).
In the psychological domain, a significantly higher score was found in the control (65.2 ± 17.3) than in the life-story group (44.8 ± 8.6, t = 6.421, p < .001) in the baseline. However, the psychological scores were significantly improved in the life-story group (week 4: 67.9 ± 10.2; week 8: 76.4 ± 12.1) than the control group (week 4: 62.2 ± 18.2, χ 2 = 42.467, p < .001; week 8: 59.9 ± 21.5, χ 2 = 114.337, p < .001) during the 8-week study. Over the 8 weeks, older adults’ psychological domain scores in the life-story group improved dramatically (baseline vs. week 8, p < .001). At the same time, there was no significant change (baseline vs. week 8, p = .188) in the control group (Fig. 3 b).
In the social relationship domain, there was significantly more improvement in the intervention group (week 4: 74.0 ± 11.0; week 8: 78.3 ± 11.7) than the control group in week 4 (64.4 ± 13.3, χ 2 = 21.945, p < .001) and week 8 (61.8 ± 16.6, χ 2 = 33.745, p < .001) during the 8-week study. The social relationship scores in the life-story group improved from baseline (63.6 ± 8.1) to week 8 (78.3 ± 11.7, p < .001), while no significant change (baseline: 66.7 ± 15.2 vs. week 8: 61.8 ± 16.6, p = .133) on the control group (Fig. 3 c).
In the environment domain, a significant improvement was found in the life-story group (week 4: 66.4 ± 8.5; week 8: 70.8 ± 10.2) compared to the control group in week 4 (61.0 ± 14.5, χ 2 = 40.086, p < .001) and week 8 (58.6 ± 16.1, χ 2 = 70.498, p < .001). Over the 8 weeks, the environment score in the life-story group improved from baseline (51.5 ± 9.5) to week 8 (70.8 ± 10.2, p < .001), while no significant change (baseline: 60.7 ± 12.6 vs. week 8: 58.6 ± 16.1, p = .735) in the control group (Fig. 3 d).
In the general quality of life score, a significantly higher score was found in the control (62.8 ± 21.7) than in the life-story group (53.3 ± 18.5, t = 2.044, p = .045) in the baseline. However, a significant improvement was found in the older adults in the intervention group (80.8 ± 12.6) than in the control group (66.4 ± 21.4, χ 2 = 9.917, p = .002) in week 8. Within time, older adults’ general quality of life score in the life-story group improved dramatically (baseline vs. week 8, p < .001). At the same time, there was no significant change (baseline vs. week 8, p = .615) in the control group (Fig. 3 e).
In the satisfaction with health score, no significant difference was found between the control (55.4 ± 22.9) and the life-story group (50.7 ± 23.6, t = 0.883, p = .380) in the baseline. In the 8-week study, a significantly higher score was found for the older adults in the intervention group (75.0 ± 13.1) than the control group (55.2 ± 22.5, χ 2 = 9.758, p = .002) in week 8. Over 8 weeks, the satisfaction with health score in the life-story group improved from baseline to week 8 ( p < .001). In contrast, no significant reduction (baseline vs. week 8, p = .997) was found in the control group (Fig. 3 f).
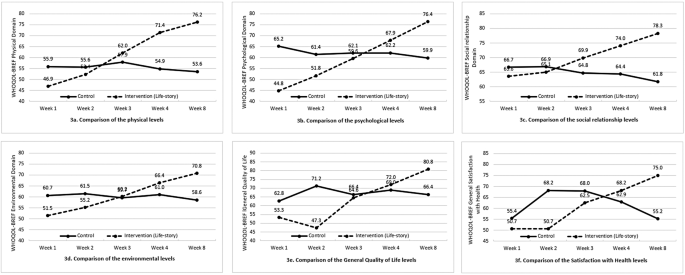
Comparison of the average quality of life (WHOQOL-BREF) between older adults in the life-story and control groups
The conclusive report highlights that creating life-story books effectively mitigates depression and enhances life satisfaction and overall quality of life for older adults in the Omani community. Specifically, participants in the life-story group experienced significant improvements in life satisfaction compared to their counterparts in the control group after initiating the life-story book creation process. The use of narrative intervention, including reminiscence and life review, has demonstrated its efficacy as a powerful therapeutic approach for addressing depressive symptoms in older adults [ 39 ]. However, a prior study reported no significant effect of life review therapy on life satisfaction [ 40 ]. However, that study differed from ours in that it involved severely depressed older adults who were on psychiatric medication. Our research aligns with the studies conducted by Ligon et al. [ 41 ] and Chan et al. [ 20 ], which investigated verbal reminiscence therapy’s effects on older adults’ well-being using a design that included initial, subsequent, and follow-up evaluations. The findings revealed that the differences between the control and experimental groups became statistically significant after 10 weeks. In our study, the older adults were not on any psychiatric medication, and they engaged in a life-story book-making process that involved recalling both positive and negative memories. Erickson [ 27 ] proposed that looking back on one’s life is the last and final developmental task. Through this process of retrospection, the older adults in our study may have achieved a sense of fulfillment and wholeness [ 19 ]. Therefore, they may have developed a sense of purpose and happiness over time [ 13 ]. Our study posits that the narrative intervention was advantageous for the participants. Additionally, the life-story review was carried out through individual and one-to-one sessions at the participants’ residences, offering a secure space conducive to emotional expression and release.
Cully et al. [ 42 ] suggested that a life story review helps older adults process and release pent-up emotions, which can lessen depression. Our research indicates a notable decrease in depression among the older adults of the life-story intervention over 8 weeks, aligning with similar findings in the Chinese [ 16 ] and Malaysian [ 21 ] populations. Westerhof and Slatman [ 43 ], in their meta-analysis on life-story review, found that reducing depressive symptoms can consequently improve life satisfaction because this can improve someone’s mental well-being and can contribute to an increase in life satisfaction. Previous studies explain this kind of improvement because participants shared their past experiences and emotions with the researcher during the process, which can help alleviate feelings of sadness [ 15 , 28 ]. This study found that those participants in the life-story group experienced appreciation and understanding when engaging with compassionate listeners. This interaction appears to substantially improve their life satisfaction and instill a deeper sense of value in their lives [ 44 ]. We understand that the baseline outcome on life satisfaction and depression levels are different between groups. In the study, the intervention group demonstrated better outcomes than the control group in terms of depression levels. The control group’s average depression scores remained consistent throughout the assessment sessions, while those of the intervention group decreased. If the baseline depression levels were similar between the two groups, the results would be more significant and robust. Similarly, for life satisfaction, the control group’s scores declined across sessions, whereas the intervention group’s scores reached the baseline levels of the control group. Again, if baseline differences were negligible, the results would be more significant and unaffected by these variations.
When conducting life story interventions in Oman, it is crucial to consider the religious and socio-cultural factors that may influence the study results. Oman is deeply rooted in Islamic traditions, which profoundly shape social norms, values, and behaviors. For instance, religious practices like praying 5 times daily can impact how people look at their life satisfaction and QoL [ 45 ]. Additionally, the concept of fate in Islam may affect older adults’ attitudes towards life satisfaction [ 46 ]. Oman typically has extended and closely-knit family structures, emphasizing collective well-being over individualism [ 47 , 48 ]. Community cohesion and support networks are also vital aspects of Omani culture. Social gatherings, mutual aid, and community-based health initiatives are common, so they may not feel lonely and have fewer depressive symptoms [ 46 ]. Understanding these issues can help in designing the content of the life-story review interventions that ensure better acceptance and engagement [ 47 , 49 ]. Including these religious and socio-cultural factors could help to improve the content of the life-story review intervention, which should be more likely to be more effective in Oman.
Conducting a life-story review and creating a life-story book can significantly improve the QoL in older adults. Our study results are consistent with previous research focusing on a life review or a life-story book-making process, improving older adults’ mental health and well-being [ 30 ]. This process may enhance the participant’s general well-being, which may help reduce their loneliness [ 20 ]. We found that making a life-story book influenced depression and life satisfaction, which enhanced the QoL of the intervention group participants, perhaps because of the cumulative benefits of this activity [ 16 , 41 ]. Life-story work promotes attributing meaning to one’s life cycle, enhancing a sense of purpose and fulfillment [ 27 ]. It encourages social interaction and engagement, leading to a greater sense of belonging and improved social relationships [ 7 ]. It also improves psychosocial well-being, which can lead to higher QoL [ 17 ]. During the sessions, they may have resolved negative emotions related to different life stages, which may have led to gradual improvements in their mental health and well-being scores [ 24 ]. In conclusion, life-story work can positively impact older adults’ QoL by enhancing their cognitive and psychosocial well-being, increasing life satisfaction, and reducing depressive symptoms.
Implications for clinical practice
The study emphasized early and effective intervention to enhance older adults’ well-being. This intervention could also prove valuable for older adults in community hospitals or day-care centers. By reviewing life and creating personal life-story books, healthcare professionals can gain deeper insights into each patient’s unique experiences, enabling tailored care to improve their QoL significantly [ 20 ]. It also adds to the current understanding of how producing life-story books as part of a life review can positively influence life satisfaction and depression among older adults. However, this result was based on a relatively small sample size study, so they should be interpreted cautiously. More studies need to focus on the progressive effects of similar interventions on older people.
Limitations of this study
There are a few limitations that may affect the results of this study. First, these findings are based on a small sample size, and local studies are scarce to support them, indicating the need for further research. Second, this study had many female participants, which may not accurately represent the male population. It could be beneficial to conduct separate analyses for each gender on every outcome measure. However, more studies focusing on the male population are recommended due to the small sample size, particularly among male participants. Third, this study’s participants were recruited from the capital, which may not represent the entire Omani population. Some tribes still reside in the desert, and their traditional cultures and beliefs may differ from those living in the capital, suggesting the need for more studies in rural areas. Fourth, due to the inability to blind both participants and researchers, the Hawthorne effect could potentially influence the study results. Fifth, the current intervention’s benefits lasted 8 weeks; future studies should investigate its long-term effects on older adults over extended periods.
Conclusions
This study showed statistically significant reductions in depression and improved life satisfaction and quality of life in older Omani adults in the life-story group compared to the control group. Conducting a life-story review and creating a life-story book is an effective intervention to alleviate the quality of life of older adults. Primary healthcare providers can guide older adults in adopting this method as a form of self-care, facilitating emotional release and fostering a therapeutic process in their everyday lives.
Data availability
The dataset used in this research can be made available with a reasonable request from the corresponding author.
Abbreviations
Quality of life (WHOWOL-BREF)
Geriatric Depression Scale – Short form 15 items
Satisfaction with life scale
Generalized Estimating Equation
WHOQOL Group. Development of the World Health Organization WHOQOL-BREF Quality of Life Assessment. Psychol Med. 1998;28:551–8.
Article Google Scholar
Chia F, Huang W-Y, Huang H, Wu C-E. Promoting healthy behaviors in older adults to optimize Health-promoting lifestyle: an intervention study. Int J Environ Res Public Health. 2023;20(2):1628. https://doi.org/10.3390/ijerph20021628
Article PubMed PubMed Central Google Scholar
Jelicic M, Kempen GIJM. Effect of self-rated health on cognitive performance in community dwelling elderly. Educ Gerontol. 1999;25:13–7.
Solis-Navarro L, Masot O, Torres-Castro R, Otto-Yáñez M, Fernández-Jané C, Solà-Madurell M, Coda A, Cyrus-Barker E, Sitjà-Rabert M, Pérez LM. Effects on Sleep Quality of Physical Exercise Programs in older adults: a systematic review and Meta-analysis. Clocks Sleep. 2023;5(2):152–66. https://doi.org/10.3390/clockssleep5020014
Kang H, Kim H. Ageism and Psychological Well-being among older adults: a systematic review. Gerontol Geriatr Med. 2022;8:23337214221087023. https://doi.org/10.1177/23337214221087023
Ahadi B, Hassani B. Loneliness and quality of life in older adults: the mediating role of Depression. Ageing Int. 2021;46:337–50. https://doi.org/10.1007/s12126-021-09408-y
Zhong Q, Chen C, Chen S. Effectiveness on quality of life and life satisfaction for older adults: a systematic review and Meta-analysis of Life Review and Reminiscence Therapy across settings. Behav Sci (Basel). 2023;13(10):830. https://doi.org/10.3390/bs13100830
Article PubMed Google Scholar
Tian HM, Wang P. The role of perceived social support and depressive symptoms in the relationship between forgiveness and life satisfaction among older people. Aging Ment Health. 2021;25(6):1042–8. https://doi.org/10.1080/13607863.2020.1746738
Pinquart M, Forstmeier S. Effect of reminiscence interventions on psychosocial outcomes: a meta-analysis. Aging Ment Health. 2012;16(5):541–58. https://doi.org/10.1080/13607863.2011.651434
Cho D, Cheon WO. Adults’ advance aging and life satisfaction levels: effects of lifestyles and Health capabilities. Behav Sci. 2023;13(4):293. https://doi.org/10.3390/bs13040293
van Damme-Ostapowicz K, Cybulski M, Galczyk M, Krajewska-Kulak E, Sobolewski M, Zalewska A. Life satisfaction and depressive symptoms of mentally active older adults in Poland: a cross-sectional study. BMC Geriatr. 2021;21:466. https://doi.org/10.1186/s12877-021-02405-5
Al-Ghafri BR, Al Nabhani MQ, Al-Sinawi H, Al-Mahrezi A, Ghusaini A, Al-Harrasi ZB, et al. Coping with the post-COVID-19 pandemic: perceived changes of older adults in their life satisfaction, depression, and quality of life. Qual Ageing Older Adults. 2023;24(3):83–96. https://doi.org/10.1108/QAOA-02-2023-0007
Park J-H, Kang S-W. Factors related to life satisfaction of older adults at home: a focus on residential conditions. Healthcare. 2022;10:1279. https://doi.org/10.3390/healthcare10071279
Chand SP, Arif H. Depression. In: StatPearls [Internet]. Treasure Island (FL): StatPearls Publishing , 2024;Jan-. https://www.ncbi.nlm.nih.gov/books/NBK430847/
Do TTH, Nguyen DTM, Nguyen LT. Depressive symptoms and their correlates among older people in Rural Viet Nam: a study highlighting the role of family factors. Health Serv Insights. 2022;15:11786329221125410. https://doi.org/10.1177/11786329221125410
Ng SE, Tien A, Thayala J, Ho RCM, Chan MF. The effect of life-story review on depression of older community-dwelling Chinese adults in Singapore: a preliminary results. Int J Geriatr Psychiatry. 2013;28(3):328–30.
Article CAS PubMed Google Scholar
Rong J, Wang X, Ge Y, Ge Y, Chen G, Ding H. Association between functional disability and depressive symptoms among older adults in rural China: a cross-sectional study. BMJ Open. 2021;11:e047939. https://doi.org/10.1136/bmjopen-2020-047939
Article PubMed Central Google Scholar
Al-Sinawi H. Situation of elderly in Sultanate of Oman. Middle East J Age Ageing. 2016;13(2):26–7.
Al-Ghafri BR, Eltayib RAA, Al-Ghusaini ZB, Al-Nabhani MQ, Al-Mahrezi A, Al-Saidi Y, et al. A qualitative study to explore the life experiences of older adults in Oman. Eur J Invest Health Psychol Educ. 2023;13(10):2135–49. https://doi.org/10.3390/ejihpe13100150
Chan MF, Leong KS, Heng BL, Mathew BK, Khan SB, Lourdusamy SS, et al. Reducing depression among community-dwelling older adults using life-story review: a pilot study. Geriatr Nurs. 2014;35(2):105–10. https://doi.org/10.1016/j.gerinurse.2013.10.011
Chan MF. Life-Story Book Creation to Enhance Life Satisfaction for Older Adults. In: Zangeneh, M, editors Essentials in Health and Mental Health. Advances in Mental Health and Addiction. 2024; Chap. 2: 27–38. Springer, Cham. https://doi.org/10.1007/978-3-031-56192-4_2
Roesler C. Narratological methodology for identifying archetypal story patterns in autobiographical narratives. J Anal Psychol. 2006;51:574–86.
Wills T, Day MR. Valuing the person’s story: use of life-story books in a continuous setting. Clin Interv Aging. 2018;3(3):547–52.
Google Scholar
Stuart S. Ageing well? Older adults’ stories of life transitions and serious leisure. Int J Sociol Leisure. 2022;5:93–117.
Bosch O. Telling stories, creating (and saving) her life. An analysis of the autobiography of Ayaan Hirsi Ali. Women’s Stud Int Forum. 2008;31:138–47.
Atchley R. A continuity theory of normal aging. Gerontooncologist. 1989;29(2):183–90.
Article CAS Google Scholar
Erikson E. Identity and the life cycle. New York, NY: Norton & Company; 1980.
Butler R. The life review: an interpretation of reminiscence in the aged. Psychiatry. 1963;26:65–76.
Serrano JP, Latorre JM, Gatz M, Montanes J. Life Review Therapy using Autobiographical Retrieval Practice for older adults with depressive symptomatology. Psychol Aging. 2014;19(2):272–7.
Yamazaki S, Ono M, Shimada C, Hayashida CT, Tomioka M, Osada H, Ikeuchi T. Feasibility of a simplified version of guided autobiography community-dwelling older adults: a pilot study. Int J Reminisc Life Rev. 2024;10(1):1–5.
Hintze J, NCSS, PASS and GESS., Kaysville. Utah, USA. https://www.ncss.com
Al-Ghafri BR, Al-Mahrezi A, Chan MF. Effectiveness of life review on depression among elderly: a systematic review and meta-analysis. Pan Afr Med J. 2021;40:168. https://doi.org/10.11604/pamj.2021.40.168.30040
Bani-Issa W. Evaluation of the health-related quality of life of Emirati people with diabetes: integration of sociodemographic and disease-related variables. East Mediterr Health J. 2011;17(11):825–30.
Ohaeri JU, Awadalla AW. The reliability and validity of the short version of the WHO Quality of Life Instrument in an arab general population. Ann Saudi Med. 2009;29(2):98–104.
Abdallah T. The satisfaction with Life Scale (SWLS): psychometric properties an arabic-speaking Sample. Int J Adolescence Youth. 1998;7:113–9.
Chaaya M, Sibai AM, Roueiheb ZE, Chemaitelly H, Chahine LM, Al-Amin H, Mahfoud Z. Validation of the arabic version of the short geriatric depression scale (GDS-15). Int Psychogeriatr. 2008;20(3):571–81. https://doi.org/10.1017/S1041610208006741
Urbaniak GC, Plous S. Research Randomizer (Version 4.0) [Computer software]. Accessed on Jan 22, 2021, from http://www.randomizer.org/
Hanley JA, Negassa A, Edwardes M, Forrester JF. Statistical analysis of correlated data using generalized estimating equations: an orientation. Am J Epidmiol. 2003;157(4):364–75. https://doi.org/10.1093/aje/kwf215
Pinquart M, Duberstein PR, Lyness JM. Effects of psychotherapy and other behavioral interventions on clinically depressed older adults: a meta-analysis. Aging Ment Health. 2007;11(6):645–57.
Serrano Selva JP, Latorre Postigo JM, Ros Segura L, Navarro Bravo B, Aguilar Córcoles MJ, Nieto López M, Ricarte Trives JJ, Gatz M. Life review therapy using autobiographical retrieval practice for older adults with clinical depression. Psicothema. 2012;24(2):224–9.
PubMed Google Scholar
Ligon M, Welleford EA, Cotter J, Lam M. Oral history: a pragmatic approach to improving life satisfaction of elders. J Intergenerational Relationships. 2012;10(2):147–59.
Cully J, La Voie D, Gfeller J. (2001) Reminiscence, personality, and psychological functioning in older adults. Gerontologist . 2001;41(1):89–95.
Westerhof GJ, Slatman S. In search of the best evidence for Life Review Therapy to reduce depressive symptoms in older adults: a Meta-analysis of Randomized controlled trials. Clin Psychol Sci Pract. 2019;26(4):11. https://doi.org/10.1111/cpsp.12301
Gaydos HL. Understanding personal narratives: an approach to practice. J Adv Nurs. 2005;49(3):254–9.
Al-Kandari YY, Al-Saleh NM, Al-Bahrani MS. Fasting during Ramadan: knowledge, attitude, and practice among omanis. J Relig Health. 2019;58(2):536–50.
El-Islam MF. Arabic cultural psychiatry. Arab J Psychiatry. 2008;19(2):65–72.
Saxena D, El Bcheraoui C, Zhao Y. Health disparities in Oman: a nationwide survey. BMC Public Health. 2020;2020(201):1238.
Al-Bar MA, Chamsi-Pasha H. Contemporary bioethics: islamic perspective. Springer; 2015.
Al-Muniri A, Al-Sinawi H, Al-Sumri M. Community participation in health: Oman’s experience. Sultan Qaboos Univ Med J. 2017;17(4):e424–30.
Download references
Acknowledgements
The authors would like to thank you for the participation of the older adults.
This study was supported by the Ministry of Higher Education, Research and Innovation Grant, Oman (RC/RG-MED/FMCO/21/01).
Author information
Authors and affiliations.
Department of Family Medicine and Public Health, College of Medicine and Health Sciences, Sultan Qaboos University, Muscat, Oman
Bushra Rashid Al-Ghafri, Yaqoub Al-Saidi & Moon Fai Chan
Department of Behavioral Medicine, Sultan Qaboos University Hospital, Muscat, Oman
Hamed Al-Sinawi & Ahmed Mohammed Al-Harrasi
Director General, Sultan Qaboos University Hospital, Muscat, Oman
Abdulaziz Al-Mahrezi
Department of Arabic and Literature, College of Arts, Sultan Qaboos University, Muscat, Oman
Zahir Badar Al-Ghusaini
Department of Internal Medicine, Sultan Qaboos University Hospital, Muscat, Oman
Khalfan Bakhit Rashid Al-Zeedy
You can also search for this author in PubMed Google Scholar
Contributions
MFC, HAS, AMAH, and YAS designed the study. MFC and BRAG collected and analyzed the data. MFC and BRAG wrote the first draft of the manuscript. HAS, AMAH, YAS, AAM, ZBAG, and KBRAZ reviewed the manuscript. All authors read and agreed to the published version of the manuscript.
Corresponding author
Correspondence to Moon Fai Chan .
Ethics declarations
Ethical approval.
Ethical approval was obtained by the Sultan Qaboos University Medical Research Ethics Committee (MREC #2028). All study participants provided their informed consent in writing. For those unable to read or write, verbal informed consent was duly obtained.
Consent for publication
Not applicable.
Competing interests
All authors declared no potential conflicts of interest in this study, its authorship, and its publication.
Additional information
Publisher’s note.
Springer Nature remains neutral with regard to jurisdictional claims in published maps and institutional affiliations.
Rights and permissions
Open Access This article is licensed under a Creative Commons Attribution 4.0 International License, which permits use, sharing, adaptation, distribution and reproduction in any medium or format, as long as you give appropriate credit to the original author(s) and the source, provide a link to the Creative Commons licence, and indicate if changes were made. The images or other third party material in this article are included in the article’s Creative Commons licence, unless indicated otherwise in a credit line to the material. If material is not included in the article’s Creative Commons licence and your intended use is not permitted by statutory regulation or exceeds the permitted use, you will need to obtain permission directly from the copyright holder. To view a copy of this licence, visit http://creativecommons.org/licenses/by/4.0/ . The Creative Commons Public Domain Dedication waiver ( http://creativecommons.org/publicdomain/zero/1.0/ ) applies to the data made available in this article, unless otherwise stated in a credit line to the data.
Reprints and permissions
About this article
Cite this article.
Al-Ghafri, B.R., Al-Sinawi, H., Al-Harrasi, A.M. et al. Effects of life-story review on quality of life, depression, and life satisfaction in older adults in Oman: a randomized controlled study. BMC Geriatr 24 , 530 (2024). https://doi.org/10.1186/s12877-024-05133-8
Download citation
Received : 10 April 2024
Accepted : 10 June 2024
Published : 19 June 2024
DOI : https://doi.org/10.1186/s12877-024-05133-8
Share this article
Anyone you share the following link with will be able to read this content:
Sorry, a shareable link is not currently available for this article.
Provided by the Springer Nature SharedIt content-sharing initiative
- Life-story review
- Older adults
- Life satisfaction
- Quality of life
BMC Geriatrics
ISSN: 1471-2318
- Submission enquiries: [email protected]
- General enquiries: [email protected]
What Is a Controlled Experiment?
Definition and Example
- Scientific Method
- Chemical Laws
- Periodic Table
- Projects & Experiments
- Biochemistry
- Physical Chemistry
- Medical Chemistry
- Chemistry In Everyday Life
- Famous Chemists
- Activities for Kids
- Abbreviations & Acronyms
- Weather & Climate
- Ph.D., Biomedical Sciences, University of Tennessee at Knoxville
- B.A., Physics and Mathematics, Hastings College
A controlled experiment is one in which everything is held constant except for one variable . Usually, a set of data is taken to be a control group , which is commonly the normal or usual state, and one or more other groups are examined where all conditions are identical to the control group and to each other except for one variable.
Sometimes it's necessary to change more than one variable, but all of the other experimental conditions will be controlled so that only the variables being examined change. And what is measured is the variables' amount or the way in which they change.
Controlled Experiment
- A controlled experiment is simply an experiment in which all factors are held constant except for one: the independent variable.
- A common type of controlled experiment compares a control group against an experimental group. All variables are identical between the two groups except for the factor being tested.
- The advantage of a controlled experiment is that it is easier to eliminate uncertainty about the significance of the results.
Example of a Controlled Experiment
Let's say you want to know if the type of soil affects how long it takes a seed to germinate, and you decide to set up a controlled experiment to answer the question. You might take five identical pots, fill each with a different type of soil, plant identical bean seeds in each pot, place the pots in a sunny window, water them equally, and measure how long it takes for the seeds in each pot to sprout.
This is a controlled experiment because your goal is to keep every variable constant except the type of soil you use. You control these features.
Why Controlled Experiments Are Important
The big advantage of a controlled experiment is that you can eliminate much of the uncertainty about your results. If you couldn't control each variable, you might end up with a confusing outcome.
For example, if you planted different types of seeds in each of the pots, trying to determine if soil type affected germination, you might find some types of seeds germinate faster than others. You wouldn't be able to say, with any degree of certainty, that the rate of germination was due to the type of soil. It might as well have been due to the type of seeds.
Or, if you had placed some pots in a sunny window and some in the shade or watered some pots more than others, you could get mixed results. The value of a controlled experiment is that it yields a high degree of confidence in the outcome. You know which variable caused or did not cause a change.
Are All Experiments Controlled?
No, they are not. It's still possible to obtain useful data from uncontrolled experiments, but it's harder to draw conclusions based on the data.
An example of an area where controlled experiments are difficult is human testing. Say you want to know if a new diet pill helps with weight loss. You can collect a sample of people, give each of them the pill, and measure their weight. You can try to control as many variables as possible, such as how much exercise they get or how many calories they eat.
However, you will have several uncontrolled variables, which may include age, gender, genetic predisposition toward a high or low metabolism, how overweight they were before starting the test, whether they inadvertently eat something that interacts with the drug, etc.
Scientists try to record as much data as possible when conducting uncontrolled experiments, so they can see additional factors that may be affecting their results. Although it is harder to draw conclusions from uncontrolled experiments, new patterns often emerge that would not have been observable in a controlled experiment.
For example, you may notice the diet drug seems to work for female subjects, but not for male subjects, and this may lead to further experimentation and a possible breakthrough. If you had only been able to perform a controlled experiment, perhaps on male clones alone, you would have missed this connection.
- Box, George E. P., et al. Statistics for Experimenters: Design, Innovation, and Discovery . Wiley-Interscience, a John Wiley & Soncs, Inc., Publication, 2005.
- Creswell, John W. Educational Research: Planning, Conducting, and Evaluating Quantitative and Qualitative Research . Pearson/Merrill Prentice Hall, 2008.
- Pronzato, L. "Optimal experimental design and some related control problems". Automatica . 2008.
- Robbins, H. "Some Aspects of the Sequential Design of Experiments". Bulletin of the American Mathematical Society . 1952.
- Examples of Independent and Dependent Variables
- Difference Between Independent and Dependent Variables
- Random Error vs. Systematic Error
- Null Hypothesis Examples
- Chemistry Glassware Types, Names and Uses
- What Is the Difference Between a Control Variable and Control Group?
- Understanding Simple vs Controlled Experiments
- What are Controlled Experiments?
- Understanding Experimental Groups
- The Difference Between Control Group and Experimental Group
- The Role of a Controlled Variable in an Experiment
- What Is an Experiment? Definition and Design
- What Is a Control Group?
- Scientific Method Vocabulary Terms
- 8th Grade Science Fair Project Ideas
- Scientific Variable
Effectiveness of artificial intelligence integration in design-based learning on design thinking mindset, creative and reflective thinking skills: An experimental study
- Open access
- Published: 22 June 2024
Cite this article
You have full access to this open access article
- Mustafa Saritepeci 1 &
- Hatice Yildiz Durak ORCID: orcid.org/0000-0002-5689-1805 1
116 Accesses
Explore all metrics
Integrating Artificial Intelligence (AI) into learning activities is an essential opportunity to develop students' varied thinking skills. On the other hand, design-based learning (DBL) can more effectively foster creative design processes with AI technologies to overcome real-world challenges. In this context, AI-supported DBL activities have a significant potential for teaching and developing thinking skills. However, there is a lack of experimental interventions in the literature examining the effects of integrating AI into learner-centered methods on active engagement and thinking skills. The current study aims to explore the effectiveness of AI integration as a guidance and collaboration tool in a DBL process. In this context, the effect of the experimental application on the participants’ design thinking mindset, creative self-efficacy (CSE), and reflective thinking (RT) self-efficacy levels and the relationship between them were examined. The participants used ChatGPT and Midjourney in the digital story development process as part of the experimental treatment. The only difference between the control and experimental groups in the digital storytelling process is the AI applications used in the experimental treatment (ChatGPT and Midjourney). In this quasi-experimental method study, participants were randomly assigned to treatment, an AI integration intervention, at the departmental level. 87 participants (undergraduate students) in the experimental group and 99 (undergraduate students) in the control group. The implementation process lasted five weeks. Partial Least Squares (PLS), Structural Equation Modeling (SEM), and Multi-Group Analysis (MGA) were made according to the measurements made at the T0 point before the experiment and at the T1 point after the experiment. According to the research result, the intervention in both groups contributed to the creative self-efficacy, critical reflection, and reflection development of the participants. On the other hand, the design thinking mindset levels of both groups did not show a significant difference in the comparison of the T0 point and the T1 point.
Similar content being viewed by others
Fostering Integrated STEM and Entrepreneurial Mindsets Through Design Thinking
TPACK and Toondoo Digital Storytelling Tool Transform Teaching and Learning
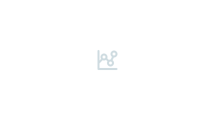
Designing purposeful digital learning
Avoid common mistakes on your manuscript.
1 Introduction
Developments such as artificial intelligence are followed by theoretical and applied studies on integrating these new technologies into learning processes (Aksu Dünya & Yıldız Durak, 2023 ; Durak & Onan, 2023 ). Technological developments change how businesses do (Kandlhofer et al., 2016 ) and the ways of learning and teaching. Chatbot platforms have components that will profoundly affect learning-teaching processes, including various threats and opportunities (Yildiz Durak, 2023a ). Although preparing student homework through such environments is a threat, these environments have essential advantages, such as accessing information in the learning-teaching process and providing integration of favorable aspects with methods that support various student activities and creativity. There is a lack of experimental intervention in the literature examining the effects of the integration of artificial intelligence into learner-centered methods on learner active participation and creativity (Lund & Wang, 2023 ). In this context, this study includes an experimental intervention to address the shortcomings mentioned in the literature.
Design thinking is a skill teachers should have for the effective use of technology in education (Beckwith, 1988 ; Tsai & Chai, 2012 ). Teachers’ lack of design thinking skills is defined as one obstacle in technology integration. These barriers are classified in the literature as primary, secondary, and tertiary (Ertmer, 1999 ; Ertmer et al., 2012 ; Tsai & Chai, 2012 ). Primary barriers are related to a lack of infrastructure, training, and support (Snoeyink & Ertmer, 2001 ). Secondary barriers generally include teachers’ affective perceptions (e.g., belief, openness to change, self-confidence, and attitude) toward technology integration (Ertmer et al., 2012 ; Keengwe et al., 2008 ). Removing primary and secondary barriers does not guarantee that technology integration will provide meaningful learning (Saritepeci, 2021 ; Yildiz Durak, 2021 ). Tsai and Chai ( 2012 ) explained this situation with tertiary barriers. The learning process is not static; it is dynamic and constantly changing. Therefore, teachers need to have design thinking skills to transform this variable nature of the learning process (Tsai & Chai, 2012 ; Yildiz Durak et al., 2023 ). Overcoming tertiary barriers significantly facilitates the effective use of technology in education. Beckwith’s ( 1988 ) Educational Technology III perspective, which expresses the most effective form of technology use in education, is a flexible structure to provide learners with more meaningful experiences instead of following a systematic process strictly dependent on instructional design, methods, and techniques in educational environments. The Educational Technology III perspective refers to design-based learning practices.
The dizzying developments that occur with technological innovations in today’s business, social, and economic life make our predictions about what kind of job a K12 student will do in the future (Darling-Hammond, 2000 ; Saritepeci, 2021 ). In this case, removing the educational technology III perspective and the tertiary barriers to technology integration is essential. Teachers and pre-service teachers should have the skills to be successful in the coming years, which are uncertain in many ways, and to create opportunities to support these learners. The design-based learning approach has remarkable importance in developing the design-oriented thinking skills of the pre-service teacher. In this context, a structure in which artificial intelligence applications are integrated into the digital storytelling method application processes, one of the most effective applications of the design-based learning approach in learning processes, will support the design-oriented thinking skills of pre-service teachers.
2 Related works
Studies on the use of artificial intelligence in education focus on various areas such as intelligent tutoring system (ITS), (Chen, 2008 ; Rastegarmoghadam & Ziarati, 2017 ), personalized learning (Chen & Hsu, 2008 ; Narciss et al., 2014 ; Zhou et al., 2018 ), assessment-feedback (Cope et al., 2021 ; Muñoz-Merino et al., 2018 ; Ramnarain-Seetohul et al., 2022 ; Ramesh & Sanampudi, 2022 ; Samarakou et al., 2016 ; Wang et al., 2018 ), educational data mining (Chen & Chen, 2009 ; Munir et al., 2022 ) and adaptive learning (Arroyo et al., 2014 ; Wauters et al., 2010 ; Kardan et al., 2015 ). These studies aim to improve the quality of the learning-teaching process by providing individualized learning experiences and increasing the effectiveness of teaching methods.
The intelligent tutoring system is the most prominent study subject in studies on the use of AI in education (Tang et al., 2021 ). ITS focuses on using AI to provide learners with personalized and automated feedback and guide them through the learning process. Indeed, there is evidence in the literature that using ITS in various teaching areas can improve learning outcomes. Huang et al. ( 2016 ) reported that using ITS in mathematics teaching reduces the gaps between advantaged and disadvantaged learners.
Personalized learning environments, another prominent use of AI in education, aim to provide an experience where the learning process is shaped within the framework of learner characteristics. In addition, supporting the learning of individuals who are disadvantaged in subjects such as learning disabilities is a promising field of study. Indeed, Walkington ( 2013 ) noted that personalized learning experience provides more positive and robust learning outcomes. Similarly, Ku et al. ( 2007 ) investigated the effect of a personalized learning environment on solving math problems. The study results show that the experimental group learners, especially those with lower-level mathematics knowledge, performed better than the control group.
Assessment and feedback, one of the forms of AI in education, is another area where the number of studies on the COVID-19 epidemic has increased (Ahmad et al., 2022 ; Hooda et al., 2022 ). Ahmad et al. ( 2022 ) compared artificial intelligence and machine learning techniques for assessment, grading, and feedback and found that accuracy rates ranged from 71 to 84%. Shermis and Burstein ( 2016 ) stated that the automatic essay evaluation system gave similar scores to student work with human evaluators, but the system had difficulties in studies that were different in terms of creativity and structure organization. Accordingly, more development and research should be done to help AI systems produce more effective results in assessment and grading. In another study, AI-supported constructive and personalized feedback on the texts created by learners effectively improved reflective thinking skills (Liu et al., 2023 ). In the same study, this intervention reduced the cognitive load of the learners in the experimental group and improved self-efficacy and self-regulated learning levels.
The use of AI in educational data mining and machine learning has been increasing in recent years to discover patterns in students’ data, such as navigation and interaction in online learning environments, to predict their future performance or to provide a personalized learning experience (Baker et al., 2016 ; Munir et al., 2022 ; Rienties et al., 2020 ). Sandra et al. ( 2021 ) conducted a literature review of machine learning algorithms used to predict learner performance and they examined 285 studies published in the IEEE Access and Science Direct databases between 2019–2021. The study results show that the most frequently used machine learning algorithm to predict learner performance is the classification machine learning algorithm, followed by NN, Naïve Bayes, Logistic Regression, SVM, and Decision Tree algorithms.
The main purpose of artificial intelligence studies in the field of AI is to create an independent learning environment by reducing the supervision and control of any pedagogical entity by providing learners with a personalized learning process within the framework of the learner and subject area characteristics (Cui, 2022 ; Zhe, 2021 ). To achieve this, system designs for predicting learner behaviors with intelligent systems, providing automatic assessment, feedback, and personalized learning experiences, and intervention studies examining their effectiveness come first. This study develops a different perspective and experiences of the learner’s create-to-learn process in collaboration with AI. There are predictions in various studies that AI and collaborative learning processes can support the creativity of learners (Kafai & Burke, 2014 ; Kandlhofer et al., 2016 ; Lim & Leinonen, 2021 ; Marrone et al., 2022 ). In this regard, Lund and Wang ( 2023 ) emphasized that the focus should be on developing creativity and critical thinking skills by enabling learners to use AI applications in any learning task (Fig. 1 ).
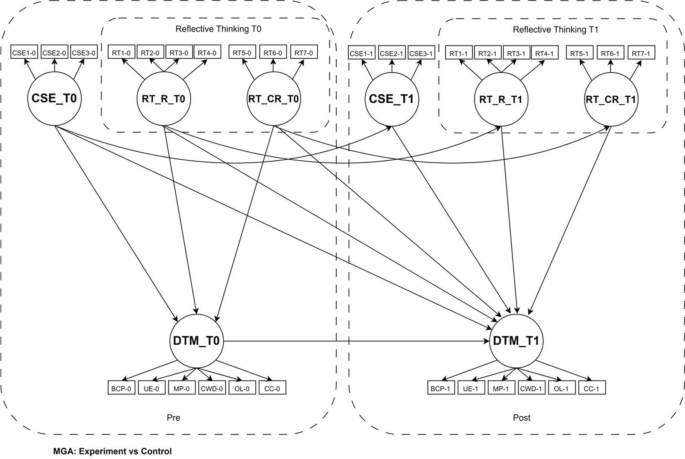
Proposed structural model. * T0: Time 0 (pretest), T1: Time 1 (posttest). * CSE: Creative self-efficacy, RT_R: Reflective thinking- Reflection, RT_CR: Reflective thinking- Critical reflection, DTM: Design thinking mindset
3 Focus of study
This study investigates the effectiveness of artificial intelligence integration (Chat GPT and Midjourney application) as a guidance and collaboration tool in the design-based process integrated into educational environments in a design-based learning process. In this context, whether the experimental application was effective in the design thinking mindset levels of the participants and their relationship with creative, reflective thinking self-efficacy was examined.
Participants were tasked with developing a digital story in a design-based process. In the context of experimental treatment, participants were systematically encouraged to use Chat GPT and Midjourney as guidance tools in the digital story development process. Apart from this treatment, the design-based learning process of the control group is very similar to the experimental group.
Therefore, all participants were exposed to the same environment at the university where the application was made, and they did not enroll in any additional technology education courses. This pretest–posttest experimental method study with a control group continued for four weeks, during which the student-produced an active product in design-based learning. In the current research context, the following research questions were addressed:
RQ1: Is the integration of artificial intelligence in a design-based learning process effective on the levels of design thinking mindset, and creative and reflective thinking self-efficacy?
RQ2: Do the relationships between design thinking mindset and creative and reflective thinking self-efficacy levels differ in the context of the experimental process?
In line with these research questions, the following hypotheses were tested:
H1a. Creative self-efficacy for 5 weeks is greater for the experimental group.
H1b. Influence of creative self-efficacy on the design thinking mindset is similar for two groups.
H1c. Influence of creative self-efficacy after 5 weeks on the design thinking mindset is similar for two groups.
H1d. Influence of creative self-efficacy after 5 weeks on the design thinking mindset is greater for the experimental group.
H2a . Influence of critical reflection on the design thinking mindset is similar for two groups.
H2b Influence of critical reflection on the design thinking mindset after 5 weeks is greater for the experimental group.
H2c. Critical reflection for 5 weeks is greater for the experimental group.
H2d. Influence of critical reflection after 5 weeks on the design thinking mindset is greater for the experimental group.
H3a . Influence of reflection on the design thinking mindset is similar for two groups.
H3b. Influence of reflection on the design thinking mindset after 5 weeks is greater for the experimental group.
H3c. Reflection for 5 weeks is greater for the experimental group.
H3d. Influence of reflection after 5 weeks on the design thinking mindset is greater for the experimental group.
H4. Design thinking mindset for 5 weeks is greater for the experimental group.
4.1 Research design
This study is a quasi-experimental method study with the pretest–posttest control group (Fig. 2 ). In this experimental methodology study, participants were randomly assigned to treatment, an AI integration intervention, at the departmental level. There were 87 (46.8%) participants in the experimental group and 99 (53.2%) participants in the control group. The participants were pre-service teachers studying in the undergraduate program of the faculty of education.
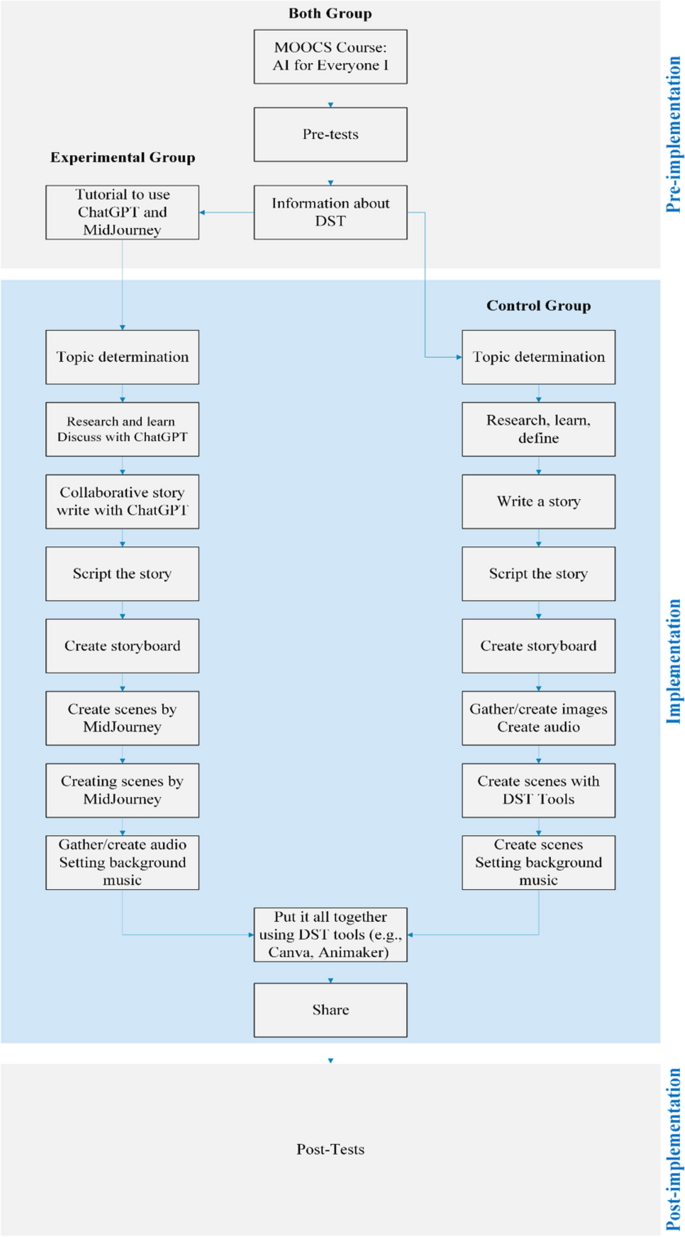
Implementation Process
The treatment in this study also served the purposes of the educational technology course as the application of design-based learning activity as an important tool in educational technology that participants (pre-service teachers) might consider using in their future teaching careers.
In addition, all participants have been exposed to the same opportunities regarding the use of digital technologies in education and none of them attended an additional course. Therefore, the prior knowledge of both groups was similar. Participation in the surveys is completely voluntary. For this reason, although 232 and 260 participants participated in the pretest and posttest, respectively, 186 students who filled in both questionnaires and participated in the application were included in the study. However, both groups were given the same input on design-based learning activities and tasks. Therefore, there is no learning loss for the control group.
4.2 Participants
The participants were 186 pre-service teachers studying at a state university in Turkey. All participants are enrolled in an undergraduate instructional technology course and study in five different departments. The ages of the participants vary between 17–28 years, with an average age of 19.12. 74.2% of the participants were female and 25.8% were male. The high rate of women is because the education faculties in Turkey have a similar demographic structure. The majority of the participants are first-year and second-year students.
The daily use of social technology (social media, etc.) is 3.89 (in hours). Technology usage time for entertainment (watching movies and series, listening to music, etc.) is 2.7 h. While the daily use of technology for gaming (mobile, computer, console games, etc.) is 0.81, the period of use of technology for educational purposes is 1.74. The participants use technology primarily for social and entertainment purposes.
4.3 Procedure
4.3.1 experimental group.
In this group, students performed the DST task as a DBL activity using ChatGPT and MidJourney artificial intelligence applications. These tasks include selecting topics, collaborative story writing with ChatGPT, scripting, creating scripted scenes with MidJourney, and voice acting, as well as integrating them. Examples of multimedia items prepared by the students in this group are shown in Fig. 3 .
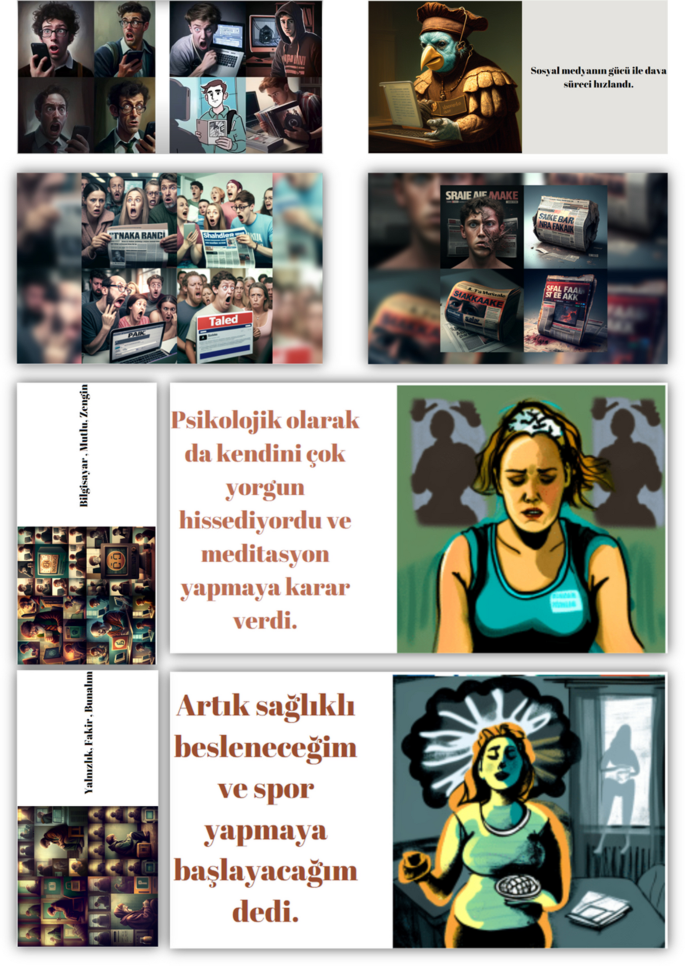
Experimental group student products-screenshot
The artificial intelligence applications they will use in this task were introduced one week before the application. Students did various free activities with these applications. In the first week of the application, students were asked to choose a topic within a specific context. The students researched their chosen topic and chatted with ChatGPT to deepen their knowledge. The students created the stories within the steps of the instruction presented by the instructor in collaboration with ChatGPT. (1) ChatGPT should be asked three questions while creating the story setup. Each question should contribute to the formation of the story. (2) A story should be created by organizing ChatGPT's answers. (3) At least 20% and a maximum of 50% of the story must belong to the student. To assess whether the students executed these three steps accurately and to offer feedback when needed, they shared the link to the page containing their conversations with the questions and answers they used to create their stories with the course instructor. The instructor compared the text accessed from this page with the final text of the student's story. He scanned the final versions of the student stories on Turnitin to check if the student's contribution to the story creation was no more than 50%.
In the next stage (weeks 2 and 3), students created each scene using MidJourney artificial intelligence bots in line with the storyboards they created by scripting their stories. The most important challenge for the students was to ensure continuity in interrelated and successive scenes using MidJourney bots, and they created the audio files by voicing the texts related to each scene. In the fourth week, students combined elements such as scenarios, scenes, and sound recordings using digital story development tools (Canva, Animaker, etc.). The final version of the digital stories was shared on the Google Classroom platform.
Learners sent the product they created for each application step and information about the process from the activity links on the Google Classroom course page. The course instructor reviewed these posts and provided corrective feedback to the students.
4.3.2 Control group
In this group, students were tasked with preparing a digital story on a topic as DBL activities. This task includes choosing a subject, writing a story, scripting, preparing multimedia elements, and integrating them. Products such as storyboards and videos produced by students in DBL activities carried out in this group are shown in Fig. 4 .
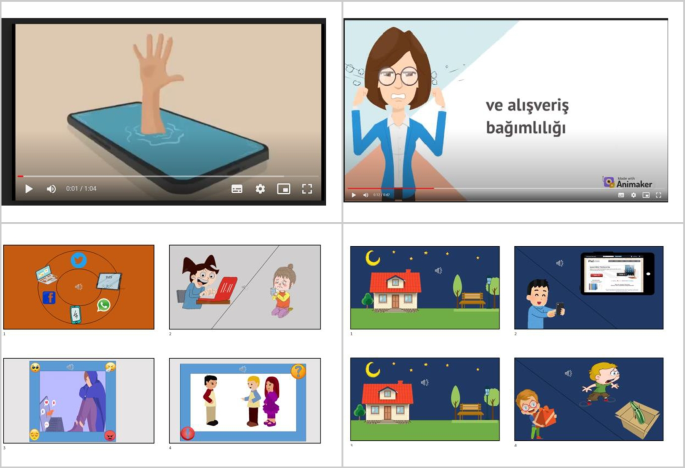
Control group student products-screenshot
In the first week of the application, the participants were asked to choose a topic within a context, as in the experimental group. The students researched the determined topic, created a story related to the subject, then scripted the story and prepared the storyboards. In the second and third weeks of the application, the students created the audio files by vocalizing the texts related to each scene (according to the scenario) in line with the storyboard. Furthermore, pictures, backgrounds, and characters were created in line with the scenario (usually compiled from ready-made pictures and characters). In the fourth week, digital story development tools combined scenarios, pictures, backgrounds, sound recordings, and characters. The final version of the stories was shared on the Google Classroom platform.
4.4 Data collection and analysis
Data were collected at two-time points via the online form. Personal Information Form and three different data collection tools were used in this study.
4.4.1 Instrumentation
Self-description form.
There are 8 questions in the personal information form. These were created to collect information about gender, age, department, class, and total hours spent using digital technologies for different purposes.
Design Thinking Mindset Scale
The scale was developed by Ladachart et al. ( 2021 ) and consists of six sub-dimensions: being comfortable with problems, user empathy, mindfulness of the process, collaborative working with diversity, orientation to learning, and creative confidence. The rating is in a 5-point Likert type. The validity and reliability values of the scale are presented in Sect. 5.
Reflective Thinking Scale
Kember et al. ( 2000 ) developed this scale to measure students’ belief in their ability to be creative; the Turkish adaptation of this scale was created by Başol and Evin Gencel ( 2013 ). Although the scale consists of four sub-dimensions, two were included in the study because they were suitable for the study, and the rating is in a 5-point Likert type. The validity and reliability values of the scale are presented in Sect. 5.
Creative Self-Efficacy Scale
The original scale, developed by Tierney and Farmer ( 2011 ) to measure their belief in their ability to be creative, was adapted into Turkish by Atabek ( 2020 ). The scale consists of three items, and the rating is a 7-point Likert type. In the context of this study, the data before the analysis was converted into a 5-point Likert structure, and the validity and reliability values of the scale are presented in Sect. 5.
4.4.2 Analysis
The effect of design-based learning activities integrated with artificial intelligence as a teaching intervention was tried to be measured by repeated measurement. Data collection tools were applied in the first week (T0) and the fifth week (T1) in the experimental and control groups. For analysis, only the responses (survey data) provided by students who fully participated in the application and answered the data collection tools at both T0 and T1 points were included. Partial Least Squares-Structural Equation Modeling (PLS-SEM) was used to analyze the data and test the hypotheses. SmartPLS 4 was used in the analysis (Ringle et al., 2022 ). The PLS-SEM method allowed the parameters of complex models to be estimated without making any distribution assumptions on the data. In addition, the differences between the experimental and control groups were examined using the Multiple Group Analysis (MGA) features in PLS-SEM, and it was tested whether there was a significant difference between MGA and group-specific external loads and path coefficients.
In the first stage, the measurement model was tested. In the second stage, the structural model was evaluated in the context of MGA.
5.1 Measurement model
When the measurement and structural models were evaluated, the indicator loads were higher than the recommended value of 0.7 (See Appendix Table 7 ).
Internal consistency reliability is represented by Cronbach’s alpha, composite reliability (CR), and rho_a (See Table 1 ). All values are above the threshold value of 0.70 by default. For convergent validity, the average variance extracted (AVE) value is used and this value is expected to be above 0.5. The values in the model were found to be higher than this threshold value.
Heterotrait-monotrait ratio (HTMT) and the Fornell-Larcker criterion were used for discriminant validity. The values found indicate that discriminant validity has been achieved, as seen in Tables 2 and 3 .
Considering all the data obtained, the measurement model of the proposed model is suitable for testing hypotheses.
The structural model of the PLS-SEM was examined as it provides the measurement model assumptions. PLS-SEM was run using 1000 bootstrapping. The significant differences in the path coefficients of the assumed relationships between design thinking mindset levels and creative and reflective thinking self-efficacy between the experimental and control groups were examined, and the findings are presented in Table 4 .
According to Table 4 , the structural model was examined in terms of significant differences in the path coefficients of the assumed relationships to test the research hypotheses, and the creative self-efficacy and reflective thinking dimensions for the students in the experimental and control groups differed after the treatment process.
R2 values indicate the explanatory power of the structural model and these values show moderate to significant power (See Table 5 ).
To examine whether there is a significant difference between the path coefficients for the experimental and control groups, the PLS-MGA Parametric test values were examined and the results are presented in Table 6 .
According to Table 6 , the findings show that there is no significant difference in the effect of creative self-efficacy, and reflective thinking on design thinking mindset between the two groups. After the treatment process, there is no significant difference in the relationships between creative self-efficacy, reflective thinking, and design thinking mindset. The significance levels of the path coefficients showed that the hypotheses were not supported.
6 Discussion and conclusion
This study examined the effect of AI integration, which is integrated into the digital storytelling process, a design-based learning method, on design thinking mindset and whether it is effective in its relations with creative, reflective thinking self-efficacy. The participants used ChatGPT and Midjourney applications in the digital story development process as part of the experimental treatment. The only difference in the digital storytelling process between the control and experimental groups is the AI applications used in the experimental treatment. The experimental intervention covers four weeks. Data were collected from the participants before (T0) and after the application (T1) with data collection tools. There is a significant difference at the T1 point compared to the T0 point in both groups' creative self-efficacy, critical reflection, and reflection levels. Accordingly, the intervention in both groups contributed to the participants' creative self-efficacy, critical reflection, and reflection development. On the other hand, the design thinking mindset levels of both groups did not show a significant difference in the comparison of the T0 point and the T1 point.
According to the multigroup comparison of the creative self-efficacy level at T0 and T1 points, there was no significant difference between the groups. When compared to T0 at the T1 point, creative self-efficacy improvement was achieved in both groups. This is valuable as it shows that the creative self-efficacy contribution of intensive use of AI support in a design-based learning environment is similar. Indeed, creativity, recognized as one of the core competencies in education, is part of CSE, which includes the belief that an individual is capable of producing creative results (Yildiz Durak, 2023b ). There are predictions in various studies that AI and collaborative learning processes can support the creativity of learners (Kafai & Burke, 2014 ; Kandlhofer et al., 2016 ; Lim & Leinonen, 2021 ; Marrone et al., 2022 ). Marrone et al. ( 2022 ) provided eight-week training sessions on creativity and AI to middle school students. In their subsequent interviews with the students, the most dominant opinion was that AI support had a crucial role in supporting their creativity. In support of this, the experimental treatment in our study requires various creative interventions from the students: (1) Students asked at least three questions to ChatGPT while creating a story. (2) Each question contained abstracting from the previous AI answer and directions on how to continue. (3) they also created their constructs by creating connecting sentences and paragraphs to gather the answers given by ChatGPT. In addition, the second part where creativity came into play was creating scenes related to the story in the Midjourney environment. (4) While creating these scenes, the student had to plan scenes by abstracting the story he had created in collaboration with AI, create those scenes, and provide detailed parameters to the Midjourney bot to ensure continuity between the scenes. It may be that, relatively, in the expectation control group, the realization of this whole process by the students through various creative practices will further support creativity and self-efficacy. Regarding this situation, Riedl and O’Neill ( 2009 ) highlighted that although these tools (Canva, Animaker, etc.) make it possible to develop creative content, the user may not get significant results. In this context, they pose an essential question: “Can an intelligent system augment non-expert creative ability?”. Lim and Leinonen ( 2021 ) argued that AI-powered structures can effectively support creativity and that humans and machines can learn from each other to produce original works. Taking this one step further, AI will contribute to students’ creativity in learning and teaching processes (Kafai & Burke, 2014 ). Indeed, Wang et al. ( 2023 ) found a significant relationship between students' AI capability levels and their creativity, explaining 28.4% of the variance in creativity.
According to the research findings, all ways between reflective thinking scale sub-dimensions critical reflection and reflection and design thinking mindset are insignificant (H2a, H2b, H2d, H3a, H3b, H3d). In addition, there is no significant difference between the groups according to the multi-group comparison at T0—T1 points for reflection and critical reflection. On the other hand, there is a significant improvement in the critical reflection and reflection levels at the T1 point of both groups compared to the T0 point. Accordingly, AI collaboration has a similar effect to the process in the control group on the learners’ reflective thinking levels in the design-based learning process. In support of this, we have evidence that incorporating AI in various forms in educational processes has essential outcomes for reflective thinking. Indeed, Liu et al. ( 2023 ) reported that an intervention involving incorporating AI into the learning process as a feedback tool to support reflective thinking in foreign language teaching resulted in remarkable improvements in learning outcomes and student self-efficacy.
DBL involves learners assimilating new learning content to overcome authentic problems and creating innovative products and designs to showcase this learning in the simplest way possible. In this study, DST processes, which allow the application of DBL to different learning areas, are included in both interventions. In the literature, DST helps learners reflect on what they have learned (Ivala et al., 2014 ; Jenkins & Lonsdale, 2007 ; Nam, 2017 ; Robin, 2016 ; Sandars and Murray, 2011 ) and develop reflective thinking skills (Durak, 2018 ; Durak, 2020 ; Malita & Martin, 2010 ; Sadik, 2008 ; Sarıtepeci, 2017 ) is a method with critical elements. The critical implication here is that all processes of AI collaboration on reflection and critical reflection have a similar effect as the DST process planned by the learners. The similar effect of AI collaboration allowed learners to understand the benefits of AI in the DST process and to develop in-depth learning by combining their thought processes with AI and finding creative ways to reflect on their learning. Indeed, Shum and Lucas ( 2020 ) claims that AI can help individuals think more deeply about challenging experiences. The DST process includes stages (story writing, scenario creation, planning scenes, etc.) that allow learners to embody their reflections on their learning (Ohler, 2006 ; Sarıtepeci, 2017 ).
The multi-group analysis results of the road between the design thinking mindset T0 – T1 points are insignificant (H4). In addition, there was no significant improvement in design thinking mindset scores in both groups compared to T0 at the T1 point. Accordingly, the effect of the design-based learning process carried out in the experimental and control groups on the learners’ design thinking mindset scores was limited. The study’s expectation was the development of the design thinking levels of the learners and, as a result, meaningful improvements in the design thinking mindset levels. This result may be because the application process is not long enough to develop versatile skills such as design thinking. Razzouk and Shute ( 2012 ) emphasized that design thinking is challenging to acquire in a limited context. However, they argue that students can learn to design thinking skills together with scaffolding, feedback, and sufficient practice opportunities. The DST process included scaffolding and feedback processes in both groups. Although there are different stages for acquiring and developing design thinking skills during the application process, the similar characteristics of the design thinking mindset level may indicate the need for more extended practice. However, the fact that the design thinking mindset is a self-reporting tool limits our predictions about individuals' design thinking skill acquisition and development in the process.
7 Conclusion
In conclusion, the intensive use of AI support in a design-based learning environment similarly impacts the development of participants' creative self-efficacy, reflective thinking, and design thinking mindset levels. The AI collaboration process showed a similar effect to the planned design-based learning process by allowing learners to understand the benefits of AI in the design thinking mindset and to develop in-depth learning by combining their thought processes with AI. However, it is essential to note that the study's expectation of meaningful improvements in the design thinking mindset levels was unmet. This suggests that more extended practice periods and more support and feedback processes may be necessary to effectively develop versatile skills such as design thinking.
The research contributes to our understanding of the impact of AI collaboration on learners' levels of creative self-efficacy, reflective thinking, and design thinking mindset. Further studies with extended practice periods and additional scaffolding and feedback processes could provide valuable insights into the effective development of design thinking skills in AI-supported design-based learning environments.
Data availability
The datasets generated during and/or analysed during the current study are available from the corresponding author on reasonable request.
Ahmad, S. F., Alam, M. M., Rahmat, M., Mubarik, M. S., & Hyder, S. I. (2022). Academic and administrative role of artificial intelligence in education. Sustainability, 14 (3), 1101.
Article Google Scholar
Aksu Dünya, B., & Yıldız Durak, H. (2023). Hi! Tell me how to do it: Examination of undergraduate students’ chatbot-integrated course experiences. Quality & Quantity , 1–16. https://doi.org/10.1007/s11135-023-01800-x
Atabek, O. (2020). Adaptation of creative self-efficacy scale into Turkish language. WOrld Journal on Educational Technology: Current Issues., 12 (2), 084–097.
Google Scholar
Arroyo, I., Woolf, B. P., Burelson, W., Muldner, K., Rai, D., & Tai, M. (2014). A multimedia adaptive tutoring system for mathematics that addresses cognition, metacognition and affect. International Journal of Artificial Intelligence in Education, 24 , 387–426. https://doi.org/10.1007/s40593-014-0023-y#Sec17
Baker, R. S., Martin, T., & Rossi, L. M. (2016). Educational data mining and learning analytics. The Wiley handbook of cognition and assessment: Frameworks, methodologies, and applications , 379–396. https://doi.org/10.1002/9781118956588.ch16
Başol, G., & Evin Gencel, İ. (2013). Yansıtıcı düşünme düzeyini belirleme ölçeği: Geçerlik ve güvenirlik çalışması. Kuram Ve Uygulamada Eğitim Bilimleri, 13 (2), 929–946.
Beckwith, D. (1988). The future of educational technology. Canadian Journal of Educational Comunication 17 (1), 3–20.
Chen, C. M. (2008). Intelligent web-based learning system with personalized learning path guidance. Computers & Education, 51 (2), 787–814. https://doi.org/10.1016/j.compedu.2007.08.004
Chen, C. M., & Hsu, S. H. (2008). Personalized intelligent mobile learning system for supportive effective English learning. Educational Technology and Society Journal, 11 (3), 153–180.
Chen, C. M., & Chen, M. C. (2009). Mobile formative assessment tool based on data mining techniques for supporting web-based learning. Computers & Education, 52 (1), 256–273. https://doi.org/10.1016/j.compedu.2008.08.005
Cope, B., Kalantzis, M., & Searsmith, D. (2021). Artificial intelligence for education: Knowledge and its assessment in AI-enabled learning ecologies. Educational Philosophy and Theory, 53 (12), 1229–1245. https://doi.org/10.1080/00131857.2020.1728732
Cui, K. (2022). Artificial intelligence and creativity: Piano teaching with augmented reality applications. Interactive Learning Environments, 31 (10), 7017–7028. https://doi.org/10.1080/10494820.2022.2059520
Darling-Hammond, L. (2000). Teacher quality and student achievement. Education Policy Analysis Archives, 8 , 1.
Durak, H. Y. (2018). Digital story design activities used for teaching programming effect on learning of programming concepts, programming self-efficacy, and participation and analysis of student experiences. Journal of Computer Assisted Learning, 34 (6), 740–752.
Durak, H. Y. (2020). The effects of using different tools in programming teaching of secondary school students on engagement, computational thinking and reflective thinking skills for problem solving. Technology, Knowledge and Learning, 25 (1), 179–195.
Durak, H.Y. & Onan, A. (2023). Adaptation of behavioral intention to use and learn chatbot in education scale into Turkish. Ahmet Keleşoğlu Eğitim Fakültesi Dergisi (AKEF) Dergisi , 5(2), 1162-1172.
Ertmer, P. A. (1999). Addressing first-and second-order barriers to change: Strategies for technology integration. Educational Technology Research and Development, 47 (4), 47–61.
Ertmer, P. A., Ottenbreit-Leftwich, A. T., Sadik, O., Sendurur, E., & Sendurur, P. (2012). Teacher beliefs and technology integration practices: A critical relationship . Computers & Education, 59 (2), 423–435.
Hooda, M., Rana, C., Dahiya, O., Rizwan, A., & Hossain, M. S. (2022). Artificial intelligence for assessment and feedback to enhance student success in higher education. Mathematical Problems in Engineering, 1–19. https://doi.org/10.1155/2022/5215722
Huang, X., Craig, S. D., Xie, J., Graesser, A., & Hu, X. (2016). Intelligent tutoring systems work as a math gap reducer in 6th grade after-school program. Learning and Individual Differences, 47 , 258–265. https://doi.org/10.1016/j.lindif.2016.01.012
Ivala, E., Gachago, D., Condy, J., & Chigona, A. (2014). Digital Storytelling and Reflection in Higher Education: A Case of Pre-Service Student Teachers and Their Lecturers at a University of Technology. Journal of Education and Training Studies, 2 (1), 217–2273. https://doi.org/10.11114/jets.v2i1.286
Jenkins, M., & Lonsdale, J. (2007). Evaluating the effectiveness of digital storytelling for student reflection. ASCILITE conference (pp. 440–444). Singapore.
Kardan, A. A., Aziz, M., & Shahpasand, M. (2015). Adaptive systems: A content analysis on technical side for e-learning environments. Artificial Intelligence Review, 44 (3), 365–391. https://doi.org/10.1007/s10462-015-9430-1
Kafai, Y. B., & Burke, Q. (2014). Connected Code: Why Children Need to Learn Programming . MIT Press.
Book Google Scholar
Kandlhofer, M., Steinbauer, G., Hirschmugl-Gaisch, S., & Huber, P. (2016). Artificial intelligence and computer science in education: From kinder-garten to university. In IEEE Frontiers in Education Conference (pp. 1–9). https://doi.org/10.1109/FIE.2016.7757570
Keengwe, J., Onchwari, G., & Wachira, P. (2008). Computer technology integration and student learning: Barriers and promise. JOurnal of Science Education and Technology, 17 (6), 560–565.
Kember, D., Leung, D. Y., Jones, A., Loke, A. Y., McKay, J., Sinclair, K., ... & Yeung, E. (2000). Development of a questionnaire to measure the level of reflective thinking. Assessment & Evaluation in Higher Education, 25 (4), 381–395.
Ku, H. Y., Harter, C. A., Liu, P. L., Thompson, L., & Cheng, Y. C. (2007). The effects of individually personalized computer-based instructional program on solving mathematics problems. Computers in Human Behavior, 23 (3), 1195–1210.
Ladachart, L., Ladachart, L., Phothong, W., & Suaklay, N. (2021, March). Validation of a design thinking mindset questionnaire with Thai elementary teachers. In Journal of physics: conference series (vol. 1835, No. 1, p. 012088). IOP Publishing. https://doi.org/10.1088/1742-6596/1835/1/012088
Lim, J., & Leinonen, T. (2021). Creative peer system an experimental design for fostering creativity with artificial intelligence in multimodal and sociocultural learning environments. In CEUR workshop proceedings (vol. 2902, pp. 41–48). RWTH Aachen University. https://research.aalto.fi/en/publications/creative-peer-system-an-experimental-design-for-fostering-creativ
Liu, C., Hou, J., Tu, Y. F., Wang, Y., & Hwang, G. J. (2023). Incorporating a reflective thinking promoting mechanism into artificial intelligence-supported English writing environments. Interactive Learning Environments, 31 (9), 5614–5632. https://doi.org/10.1080/10494820.2021.2012812
Lund, B. D., & Wang, T. (2023). Chatting about ChatGPT: How may AI and GPT impact academia and libraries? Library Hi Tech News, 40 (3), 26–29.
Malita, L., & Martin, C. (2010). Digital storytelling as web passport to success in the 21st century. Procedia-Social and Behavioral Sciences, 2 (2), 3060–3064.
Marrone, R., Taddeo, V., & Hill, G. (2022). Creativity and Artificial Intelligence—A Student Perspective. Journal of Intelligence, 10 (3), 65. https://doi.org/10.3390/jintelligence10030065
Munir, H., Vogel, B., & Jacobsson, A. (2022). Artificial intelligence and machine learning approaches in digital education: A systematic revision. Information, 13 (4), 203. https://doi.org/10.3390/info13040203
Muñoz-Merino, P. J., Novillo, R. G., & Kloos, C. D. (2018). Assessment of skills and adaptive learning for parametric exercises combining knowledge spaces and item response theory. Applied Soft Computing, 68 , 110–124. https://doi.org/10.1016/j.asoc.2018.03.045
Nam, C. W. (2017). The effects of digital storytelling on student achievement, social presence, and attitude in online collaborative learning environments. Interactive Learning Environments, 25 (3), 412–427. https://doi.org/10.1080/10494820.2015.1135173
Narciss, S., Sosnovsky, S., Schnaubert, L., Andrès, E., Eichelmann, A., Goguadze, G., & Melis, E. (2014). Exploring feedback and student characteristics relevant for personalizing feedback strategies. Computers & Education, 71 , 56–76. https://doi.org/10.1016/j.compedu.2013.09.011
Ohler, J. (2006). The world of digital storytelling. Educational Leadership, 63 (4), 44–47.
Ramesh, D., & Sanampudi, S. K. (2022). An automated essay scoring systems: A systematic literature review. Artificial Intelligence Review, 55 (3), 2495–2527. https://doi.org/10.1007/s10462-021-10068-2
Ramnarain-Seetohul, V., Bassoo, V., & Rosunally, Y. (2022). Similarity measures in automated essay scoring systems: A ten-year review. Education and Information Technologies, 27 (4), 5573–5604. https://doi.org/10.1007/s10639-021-10838-z
Rastegarmoghadam, M., & Ziarati, K. (2017). Improved modeling of intelligent tutoring systems using ant colony optimization. Education and Information Technologies, 22 (10), 67–1087. https://doi.org/10.1007/s10639-016-9472-2
Razzouk, R., & Shute, V. (2012). What is design thinking and why is it important? Review of Educational Research, 82 (3), 330–348.
Riedl, M. O., & O’Neill, B. (2009). Computer as audience: A strategy for artificial intelligence support of human creativity. In Proc. CHI workshop of computational creativity support . https://www.academia.edu/download/35796332/riedl.pdf
Rienties, B., Køhler Simonsen, H., & Herodotou, C. (2020). July). Defining the boundaries between artificial intelligence in education, computer-supported collaborative learning, educational data mining, and learning analytics: A need for coherence. Frontiers in Education, 5 , 1–5. https://doi.org/10.3389/feduc.2020.00128
Ringle, C. M., Wende, S., & Becker, J.-M. (2022). SmartPLS 4. Oststeinbek: SmartPLS GmbH, http://www.smartpls.com .
Robin, B. R. (2016). The power of digital storytelling to support teaching and learning. Digital Education Review, 30 , 17–29.
Sadik, A. (2008). Digital storytelling: A meaningful technology-integrated approach for engaged student learning. Educational Technology Research and Development, 56 , 487–506. https://doi.org/10.1007/s11423-008-9091-8
Samarakou, M., Fylladitakis, E. D., Karolidis, D., Früh, W. G., Hatziapostolou, A., Athinaios, S. S., & Grigoriadou, M. (2016). Evaluation of an intelligent open learning system for engineering education. Knowledge Management & E-Learning, 8 (3), 496.
Sandars, J., & Murray, C. (2011). Digital storytelling to facilitate reflective learning in medical students. Medical Education, 45 (6), 649–649. https://doi.org/10.1111/j.1365-2923.2011.03991.x
Sandra, L., Lumbangaol, F., & Matsuo, T. (2021). Machine learning algorithm to predict student’s performance: a systematic literature review. TEM Journal, 10 (4), 1919–1927. https://doi.org/10.18421/TEM104-56
Sarıtepeci, M. (2017). An experimental study on the investigation of the effect of digital storytelling on reflective thinking ability at middle school level. Bartın University Journal of Faculty of Education, 6 (3), 1367–1384. https://doi.org/10.14686/buefad.337772
Saritepeci, M. (2021). Students’ and parents’ opinions on the use of digital storytelling in science education. Technology, Knowledge and Learning, 26 (1), 193–213. https://doi.org/10.1007/s10758-020-09440-y
Shermis, M. D., & Burstein, J. (2016). Handbook of Automated Essay Evaluation: Current applications and new directions . Routledge.
Shum, S. B., & Lucas, C. (2020). Learning to reflect on challenging experiences: An AI mirroring approach. In Proceedings of the CHI 2020 workshop on detection and design for cognitive biases in people and computing systems .
Snoeyink, R., & Ertmer, P. A. (2001). Thrust into technology: How veteran teachers respond. Journal of Educational Technology Systems, 30 (1), 85–111. https://doi.org/10.2190/YDL7-XH09-RLJ6-MTP1
Tang, K. Y., Chang, C. Y., & Hwang, G. J. (2021). Trends in artificial intelligence-supported e-learning: A systematic review and co-citation network analysis (1998–2019). Interactive Learning Environments , 1–19. https://doi.org/10.1080/10494820.2021.1875001 .
Tierney, P., & Farmer, S. M. (2011). Creative self-efficacy development and creative performance over time. Journal of Applied Psychology, 96 (2), 277–293. https://doi.org/10.1037/a0020952
Tsai, C.-C., & Chai, C. S. (2012). The” third”-order barrier for technology-integration instruction: Implications for teacher education. Australasian Journal of Educational Technology , 28 (6). https://doi.org/10.14742/ajet.810 .
Walkington, C. A. (2013). Using adaptive learning technologies to personalize instruction to student interests: The impact of relevant contexts on performance and learning outcomes. Journal of Educational Psychology, 105 (4), 932–945. https://doi.org/10.1037/a0031882
Wang, Z., Liu, J., & Dong, R. (2018). Intelligent auto-grading system. In 2018 5th IEEE international conference on cloud computing and intelligence systems (CCIS) (pp. 430–435). IEEE. https://doi.org/10.1109/CCIS.2018.8691244
Wang, S., Sun, Z., & Chen, Y. (2023). Effects of higher education institutes’ artificial intelligence capability on students’ self-efficacy, creativity and learning performance. EDucation and Information Technologies, 28 (5), 4919–4939. https://doi.org/10.1007/s10639-022-11338-4#Sec2
Wauters, K., Desmet, P., & Van Den Noortgate, W. (2010). Adaptive item-based learning environments based on the item response theory: Possibilities and challenges. Journal of Computer Assisted Learning, 26 (6), 549–562. https://doi.org/10.1111/j.1365-2729.2010.00368.x
Yildiz Durak, H. (2021). Preparing pre-service teachers to integrate teaching technologies into their classrooms: Examining the effects of teaching environments based on open-ended, hands-on and authentic tasks. Education and Information Technologies, 26 (5), 5365–5387.
Yildiz Durak, H. (2023a). Conversational agent-based guidance: Examining the effect of chatbot usage frequency and satisfaction on visual design self-efficacy, engagement, satisfaction, and learner autonomy. Education and Information Technologies, 28 , 471–488. https://doi.org/10.1007/s10639-022-11149-7
Yildiz Durak, H. (2023b). Examining various variables related to authentic learning self-efficacy of university students in educational online social networks: Creative self-efficacy, rational experiential thinking, and cognitive flexibility. Current Psychology, 42 (25), 22093–22102.
Yildiz Durak, H., Atman Uslu, N., Canbazoğlu Bilici, S., & Güler, B. (2023). Examining the predictors of TPACK for integrated STEM: Science teaching self-efficacy, computational thinking, and design thinking. Education and Information Technologies, 28 (7), 7927–7954.
Zhe, T. (2021). Research on the model of music sight-singing guidance system based on artificial intelligence. Complexity, 2021 , 1–11. https://doi.org/10.1155/2021/3332216
Zhou, Y., Huang, C., Hu, Q., Zhu, J., & Tang, Y. (2018). Personalized learning full-path recommendation model based on LSTM neural networks. Information Sciences, 444 , 135–152. https://doi.org/10.1016/j.ins.2018.02.053
Download references
Open access funding provided by the Scientific and Technological Research Council of Türkiye (TÜBİTAK).
Author information
Authors and affiliations.
Eregli Faculty of Education, Department of Educational Sciences, Necmettin Erbakan University, Konya, Turkey
Mustafa Saritepeci & Hatice Yildiz Durak
You can also search for this author in PubMed Google Scholar
Corresponding author
Correspondence to Hatice Yildiz Durak .
Ethics declarations
Access of data.
Our data are not yet available online in any institutional database. However, we will send the whole data package by request. The request should be sent to Assoc. Professor [email protected].
Ethical statement
The research was conducted in a school in Turkey and approved by the school administration. Participation was voluntary and anonymous. Informed consent was obtained from all participants.
Conflict of interests
We have not received any funding or other support to present the views expressed in this paper. The authors declare no conflicts of interest with respect to the authorship or the publication of this paper.
Additional information
Publisher's note.
Springer Nature remains neutral with regard to jurisdictional claims in published maps and institutional affiliations.
Rights and permissions
Open Access This article is licensed under a Creative Commons Attribution 4.0 International License, which permits use, sharing, adaptation, distribution and reproduction in any medium or format, as long as you give appropriate credit to the original author(s) and the source, provide a link to the Creative Commons licence, and indicate if changes were made. The images or other third party material in this article are included in the article's Creative Commons licence, unless indicated otherwise in a credit line to the material. If material is not included in the article's Creative Commons licence and your intended use is not permitted by statutory regulation or exceeds the permitted use, you will need to obtain permission directly from the copyright holder. To view a copy of this licence, visit http://creativecommons.org/licenses/by/4.0/ .
Reprints and permissions
About this article
Saritepeci, M., Yildiz Durak, H. Effectiveness of artificial intelligence integration in design-based learning on design thinking mindset, creative and reflective thinking skills: An experimental study. Educ Inf Technol (2024). https://doi.org/10.1007/s10639-024-12829-2
Download citation
Received : 21 November 2023
Accepted : 28 May 2024
Published : 22 June 2024
DOI : https://doi.org/10.1007/s10639-024-12829-2
Share this article
Anyone you share the following link with will be able to read this content:
Sorry, a shareable link is not currently available for this article.
Provided by the Springer Nature SharedIt content-sharing initiative
- Artificial intelligence
- Design-based learning activities
- ChatGPT, Design thinking mindset
- Thinking skills
- Storytelling
- Find a journal
- Publish with us
- Track your research
Developer Console
- Developer Discord
- Get started
Intro to Claude
Learn about claude.
- Security and compliance
Build with Claude
- Define sucess criteria
- Develop test cases
Prompt engineering
- Text generation
- Google Sheets add-on
- Tool use (function calling)
Test and evaluate
Strengthen guardrails
- Using the Evaluation Tool
- System status
- Claude 3 model card
Anthropic Cookbook
- Anthropic Courses
Welcome to Claude
Claude is a highly performant, trustworthy, and intelligent AI platform built by Anthropic. Claude excels at tasks involving language, reasoning, analysis, coding, and more.
Get started
If you’re new to Claude, start here to learn the essentials and make your first API call.
Explore Claude’s capabilities and development flow.
Learn how to make your first API call in minutes.
Prompt Library
Explore example prompts for inspiration.
Claude consists of a family of large language models that enable you to balance intelligence, speed, and cost.
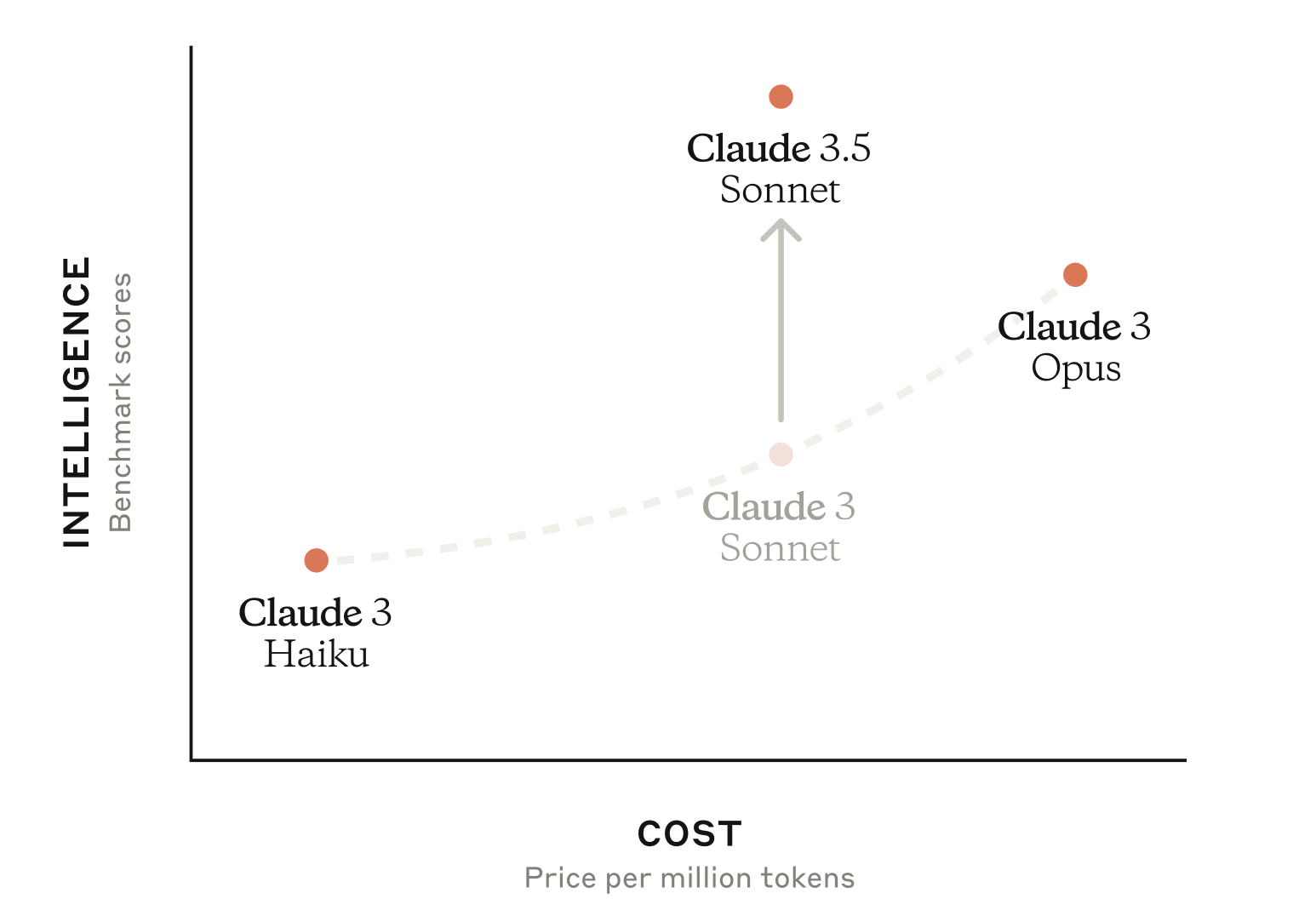
Compare our state-of-the-art models.
Develop with Claude
Anthropic has best-in-class developer tools to build scalable applications with Claude.
Enjoy easier, more powerful prompting in your browser with the Workbench and prompt generator tool.
API Reference
Explore, implement, and scale with the Anthropic API and SDKs.
Learn with interactive Jupyter notebooks that demonstrate uploading PDFs, embeddings, and more.
Key capabilities
Claude can assist with many tasks that involve text, code, and images.
Text and code generation
Summarize text, answer questions, extract data, translate text, and explain and generate code.
Process and analyze visual input and generate text and code from images.
Help Center
Find answers to frequently asked account and billing questions.
Service Status
Check the status of Anthropic services.
- Develop with Claude
- Key capabilities
This paper is in the following e-collection/theme issue:
Published on 25.6.2024 in Vol 26 (2024)
Effectiveness of Web-Based Mindfulness-Based Interventions for Patients With Cancer: Systematic Review and Meta-Analyses
Authors of this article:

- Ting Wang * , MNS ;
- Chulei Tang * , PhD ;
- Xiaoman Jiang, MSc ;
- Yinning Guo, MNS ;
- Shuqin Zhu, PhD ;
- Qin Xu, MM
School of Nursing, Nanjing Medical University, Nanjing, China
*these authors contributed equally
Corresponding Author:
School of Nursing, Nanjing Medical University
101 Longmian Avenue, Jiangning District
Nanjing, 211166
Phone: 86 13601587208
Email: [email protected]
Background: Cancer has emerged as a considerable global health concern, contributing substantially to both morbidity and mortality. Recognizing the urgent need to enhance the overall well-being and quality of life (QOL) of cancer patients, a growing number of researchers have started using online mindfulness-based interventions (MBIs) in oncology. However, the effectiveness and optimal implementation methods of these interventions remain unknown.
Objective: This study evaluates the effectiveness of online MBIs, encompassing both app- and website-based MBIs, for patients with cancer and provides insights into the potential implementation and sustainability of these interventions in real-world settings.
Methods: Searches were conducted across 8 electronic databases, including the Cochrane Library, Web of Science, PubMed, Embase, SinoMed, CINAHL Complete, Scopus, and PsycINFO, until December 30, 2022. Randomized controlled trials involving cancer patients aged ≥18 years and using app- and website-based MBIs compared to standard care were included. Nonrandomized studies, interventions targeting health professionals or caregivers, and studies lacking sufficient data were excluded. Two independent authors screened articles, extracted data using standardized forms, and assessed the risk of bias in the studies using the Cochrane Bias Risk Assessment Tool. Meta-analyses were performed using Review Manager (version 5.4; The Cochrane Collaboration) and the meta package in R (R Foundation for Statistical Computing). Standardized mean differences (SMDs) were used to determine the effects of interventions. The Reach, Effectiveness, Adoption, Implementation, and Maintenance framework was used to assess the potential implementation and sustainability of these interventions in real-world settings.
Results: Among 4349 articles screened, 15 (0.34%) were included. The total population comprised 1613 participants, of which 870 (53.9%) were in the experimental conditions and 743 (46.1%) were in the control conditions. The results of the meta-analysis showed that compared with the control group, the QOL (SMD 0.37, 95% CI 0.18-0.57; P <.001), sleep (SMD −0.36, 95% CI −0.71 to −0.01; P =.04), anxiety (SMD −0.48, 95% CI −0.75 to −0.20; P <.001), depression (SMD −0.36, 95% CI −0.61 to −0.11; P =.005), distress (SMD −0.50, 95% CI −0.75 to −0.26; P <.001), and perceived stress (SMD −0.89, 95% CI −1.33 to −0.45; P =.003) of the app- and website-based MBIs group in patients with cancer was significantly alleviated after the intervention. However, no significant differences were found in the fear of cancer recurrence (SMD −0.30, 95% CI −1.04 to 0.44; P =.39) and posttraumatic growth (SMD 0.08, 95% CI −0.26 to 0.42; P =.66). Most interventions were multicomponent, website-based health self-management programs, widely used by international and multilingual patients with cancer.
Conclusions: App- and website-based MBIs show promise for improving mental health and QOL outcomes in patients with cancer, and further research is needed to optimize and customize these interventions for individual physical and mental symptoms.
Trial Registration: PROSPERO CRD42022382219; https://www.crd.york.ac.uk/prospero/display_record.php?RecordID=382219
Introduction
The 2020 Global Cancer Statistics Report estimates that there are 19.3 million new cases of cancer worldwide and approximately 10 million cancer-related deaths [ 1 ]. The leading cause of disease and mortality among humans today is cancer [ 2 , 3 ]. The physical symptoms of patients with cancer have been alleviated because of the continuous advancement of medical technology, but the psychological problems of patients with cancer have not been adequately treated. The process of treating cancer is typically complex, with many patients experiencing negative side effects of cancer treatments, such as chemotherapy and radiation therapy, that may impact their mental health, quality of life (QOL), and sleep quality. Targeted interventions to address these cancer-related symptoms can reduce the psychological burden of cancer treatment and diagnosis, which is critical to improving patients’ QOL and promoting their health [ 4 ]. With an increasing number of patients with cancer and a desire for physical and mental health, cancer care research is focusing on identifying the psychological problems of patients with cancer and developing and implementing patient-centered psychological care plans [ 5 , 6 ]. Rehabilitation for patients with cancer increasingly uses mental health as a therapeutic strategy; however, effective psychological intervention strategies are still urgently needed to satisfy the demands of patients with cancer [ 7 ].
Mindfulness-based interventions (MBIs) have emerged as promising intervention techniques for patients with cancer. Mindfulness can be defined as the ability to observe thoughts, bodily sensations, or feelings in the present moment with an open and accepting orientation toward one’s experiences [ 8 ]. MBIs, which incorporate mindfulness practices into various therapies in mental health care, have been found to increase psychological flexibility and alleviate intense emotional states. MBIs can include additional mental training, such as mindfulness-based stress reduction (MBSR) [ 9 ], and acceptance and commitment therapy [ 10 ], which addresses psychological issues by increasing psychological flexibility [ 11 ]. Cognitive-behavioral therapy has been combined with MBSR, resulting in mindfulness-based cognitive therapy (MBCT) for preventing depression relapses [ 12 ]. Mindfulness-based cancer recovery (MBCR), an adaptation of MBSR, comprises contents tailored for patients with cancer [ 13 ]. Through facilitating awareness and nonjudgmental acceptance of moment-to-moment experiences, these MBIs are presumed to alleviate intense emotional states. Mindfulness interventions have been shown to improve the psychological status of patients with cancer [ 14 , 15 ].
The rapid development of information technologies has led to the delivery of MBIs via the internet, which is more practical than face-to-face interaction and can overcome time and geographic barriers, and it has been established that online MBIs are more suitable for people with psychological and physical symptoms [ 16 ]. Implementing psychological interventions through online or remote health can be a potential cost benefit for current referral pathways and treatment models [ 17 ]. online MBIs can be used as the adjunctive therapy in patients with cancer to manage cancer-related symptoms [ 18 ].
Despite the increasing popularity of online mindfulness-based therapies for patients with cancer and the growing number of randomized controlled trials (RCTs) examining such programs, there has not been a systematic review of these studies and their descriptions of the interventions regarding their characteristics (eg, delivery mode and approach). To date, only 2 systematic reviews addressing the impact of online interventions on health outcomes in patients with cancer have been published. However, these reviews have notable limitations. The first review [ 19 ] only searched 4 databases, potentially leading to publication bias and compromising the reliability of the findings. Furthermore, this systematic review did not conduct sensitivity, subgroup, or meta-analyses. The second review [ 20 ] evaluated the validity of online MBIs on only 4 health outcomes: anxiety, depression, QOL, and mindfulness. However, the restricted quantity of RCTs and papers within each subgroup analysis poses a challenge in reaching definitive conclusions. In addition, the external validity (eg, generalizability or applicability) based on the RE-AIM (Reach, Effectiveness, Adoption, Implementation, and Maintenance) framework has not been examined in online MBIs for patients with cancer. Thus, attempts to synthesize the literature on the impact of online MBIs on the health of patients with cancer are limited, and there is a lack of analysis of the barriers and facilitators to the development of current online MBIs.
This systematic review aims to synthesize the effectiveness of online MBIs, encompassing both app- and website-based MBIs, for patients with cancer, comprehensively assessing a wide range of outcomes, including psychological, physiological, and QOL aspects. We conducted a comprehensive search to evaluate the validity of app- and website-based MBIs on psychological outcomes in patients with cancer, using high-quality RCTs to assess many health outcomes before and after treatment. Moreover, this study aims to provide an overview of the outcomes related to the interventions, including their effectiveness and potential for implementation and sustainability in real-world settings. We used the RE-AIM framework [ 21 ] to evaluate the potential for implementation and sustainability of these interventions in real-world settings. Using this framework, we can provide a comprehensive evaluation of an intervention’s potential impact and identify common traits of effective interventions. Overall, this study fills gaps in the literature by comprehensively evaluating the effectiveness and potential for implementation and sustainability of app- and website-based MBIs for patients with cancer.
Search Strategy
The protocol of this review was registered in PROSPERO (CRD42022382219) and written following the PRISMA (Preferred Reporting Items for Systematic Reviews and Meta-Analyses) reporting guideline. The methods outlined in the protocol were strictly adhered to throughout the experimental procedures. The databases were searched until December 30, 2022. To identify relevant studies for inclusion in our systematic reviews, we developed comprehensive search strategies and used 8 databases: Cochrane Library, Web of Science, PubMed, Embase, SinoMed, CINAHL Complete, Scopus, and PsycINFO. The literature search language was limited to Chinese and English. The search strategies used a combination of subject headings (eg, Medical Subject Headings in PubMed) and keywords for the following 5 concepts: mindfulness, carcinoma, intervention, telemedicine, and randomly. Multimedia Appendix 1 shows detailed database search strategies. Reference lists of included studies and relevant systematic reviews were also manually searched for additional relevant studies. Search results were captured using citation management software, and duplicates were removed.
Inclusion and Exclusion Criteria
Because of the explorative nature of this meta-analysis, we opted for rather broad inclusion criteria. The inclusion criteria were as follows: (1) studies that included patients with cancer (aged ≥18 years) with any cancer type and stage, including those receiving anticancer treatment, those in remission, those considered cured, and those in the terminal phases of the disease; (2) studies that used MBIs (including MBSR, MBCT, and MBCR) and administered the MBI via the internet (including websites, web conferencing, web-based games, and web-based video) or a smartphone app; (3) studies in which eligible controls were required to receive standard care or usual care; (4) studies were eligible if a mental health outcome (eg, fear of cancer recurrence [FCR] as measured with the Fear of Cancer Recurrence Inventory [FCRI] and posttraumatic growth [PTG] as measured with the Posttraumatic Growth Inventory), anxiety, depression, distress, stress, and sleep) or QOL was assessed; and (5) the RCT was published in English or Chinese.
Exclusion criteria were (1) other types of studies (eg, observational, review, protocol, and case report); (2) studies of health professionals, caregivers, or mixed populations in which outcomes for survivors of cancer could not be extracted; and (3) insufficient information to calculate an effect size or determine eligibility.
Screening and Data Extraction
Two reviewers independently screened all titles and abstracts; then, they independently screened full-text articles, and conflicts were resolved by consensus. Data were independently extracted by 2 reviewers using a data extraction form adapted from the Cochrane Handbook [ 22 ] and reported using PRISMA guidelines [ 23 ]. We extracted data from included trials using standardized data extraction forms. Study-level variables included the year of publication, country of study, age of participants, cancer diagnosis, delivery mode, reminder, cancer-adapted MBIs, primary and secondary outcomes, intervention and follow-up durations, intervention and control group details, outcome measurement metrics, and outcomes scores up to postintervention. Any discrepancy or uncertainties were resolved through regular meetings and discussion among the research team.
Risk-of-Bias Assessment
The risk of bias was independently assessed by 2 reviewers using the Cochrane Risk-of-Bias tool, with differences reconciled through discussion [ 24 ]. A total of 6 domains encompassed random sequence generation, allocation concealment, blinding of participants and personnel, blinding of outcome assessment, incomplete outcome data, and selective outcome reporting. Each domain was judged as low, high, or unclear risk. Discrepancies in assessments between the 2 reviewers were resolved by consensus or by a third reviewer as required.
Meta-Analytic Method
This study conducted a meta-analysis using Review Manager (version 5.4; The Cochrane Collaboration) and the meta package in R (R Foundation for Statistical Computing). The primary and secondary outcome mean and SD values at postintervention follow-up for intervention and control groups were converted to standardized mean difference (SMD), using Hedges g . The value of SMD <0.5 would be interpreted as small, SMD ≥0.5 as medium, and SMD ≥0.8 as large effect size [ 25 ]. Authors of studies with missing data were contacted through email. However, if no data were provided, a narrative synthesis would be conducted. The I 2 statistic was used to estimate the percentage of heterogeneity across the primary studies not attributable to random sample error alone. A value of 0% indicated no heterogeneity, and values of 25%, 50%, and 75% reflected low, moderate, and high degrees of heterogeneity, respectively [ 26 ]. Acknowledging differences across studies because of the varied population, length of intervention, and length of follow-up, meta-analyses were performed fitting random effects models [ 27 ]. In addition, subgroup analyses were conducted to examine effect sizes across different subgroups; the specific moderating variables included technology, sex, intervention type, intervention duration, study quality, and scale.
RE-AIM Framework
The RE-AIM framework is a valuable tool for evaluating interventions in health care [ 28 ]. Its 5 dimensions assess an intervention’s potential for large-scale adoption, implementation, and sustainability, providing a comprehensive evaluation of its real-world efficacy and viability [ 29 ]. Reach refers to the extent of successfully targeting and engaging the intended audience, evaluated using the percentage of eligible patients enrolled in the study (n enrolled/n eligible). Efficacy measures the effect on outcomes such as mental health and QOL. Effect sizes (95% CIs) for the primary outcome were used to assess efficacy. Adoption measures the extent to which organizations or health care providers are willing and able to offer the intervention to their patients or clients, and barriers to adoption are evaluated by who recruited participants and where the intervention was offered. Implementation evaluates how effectively the intervention is delivered and received by patients, including factors such as adherence and fidelity, and is evaluated by measures such as adherence to the intervention, percentage of dropouts of the most complex intervention (n postintervention follow-up/n baseline×100), intervention cost, and author-reported plans to upscale or implement. Maintenance measures the extent to which the intervention can be sustained over time and integrated into routine care, and it is evaluated by the duration of results and the author-reported availability of the intervention [ 30 ].
Description of Studies
The systematic search revealed 4349 original articles, of which 54 (0.12%) were assessed at full-text level, and 15 (0.03%) studies were included in the final synthesis. Figure 1 displays the study flowchart of the search results and Table 1 presents the characteristics extracted from the included literature in the study. The total population comprised 1613 participants, of which 870 (53.94%) and 743 (46.06%) were in the experimental and control conditions, respectively. In most (13/15, 87%) studies, the majority of participants were women. Participants were aged from 41.84 to 66.45 years. Four studies were based on MBCR, 3 on MBCT, 2 on MBSR, and 6 on mindfulness-based programs. The 6 studies included interventions that were indeed rooted in mindfulness practices; however, they did not strictly adhere to the conventional frameworks of MBCT, MBCR, or MBSR. Instead, they used a variety of mindfulness-based approaches tailored to their respective study populations.
Furthermore, these studies did not specify the exact intervention methods used but were categorized as mindfulness-based programs . Because of the unique nature of these interventions, we cannot determine whether they belong to MBCT, MBCR, or MBSR interventions; we have categorized them as mindfulness-based programs , encompassing diverse methodologies beyond the traditional MBCT, MBCR, or MBSR frameworks. Trials used usual care (8 trials) and waitlists (7 trials) equally as comparators. Six studies had participants with breast cancer, 7 with mixed cancer types, and 2 with other cancer types. Five studies were conducted in China; 5 in the United States; and 1 each in the Netherlands, Denmark, Iran, Australia, and Canada.

Study; country | Cancer type; age (y), mean (SD); gender (female; %) | Intervention (n); delivery mode | Reminders | Intervention duration, number of sessions; intervention dose | Cancer adapted | Technology | Control group (n) | Measurements | Outcomes: measure instrument |
Chang et al [ ], 2022; China | Breast; 49.6 (12.0); 100 | MBSR (41); web-based software and digital interactive whiteboard | — | 6 wk, 6 sessions; 2 h/wk | — | Website | Waitlist (26) | Pre and post | Depression, anxiety, and stress: DASS-21 |
Compen et al [ ], 2018; Netherlands | Mixed; 51.7 (10.7); 85 | MBCT (90); email, meditation audio file, and written feedback | — | 8 wk; 40-45 min/d twice daily | — | Website | Usual care (78) | Pre and post | Distress: HADS , FCR : FCRI , and QOL : SF-12 |
Kubo et al [ ], 2019; US | Mixed; 58.2 (14.4); 68 | MBP (54); audio instruction, lecture videos, and foundation course | Study staff made phone calls if an intervention participant completed <3 | 8 wk, 2 h/wk | Specifically for individuals affected by cancer | App | Usual care (43) | Pre and post | Distress: NCCN , anxiety and depression: HADS, PTG : PTGI , sleep: PROMIS , and QOL:FACT-G |
Kubo et al [ ], 2018; US | Mixed; 66.5 (9.7); 69 | MBP (52); online classroom and manual | The app can send reminders using push notifications; study staff made phone calls if an intervention participant completed <3 | 6 wk; 2 h/wk | Cancer pack, which was designed specifically for individuals affected by cancer | App | Usual care (51) | Pre and post | QOL: FACIT−Pal , distress: NCCN, and anxiety and depression: HADS |
Liu et al [ ], 2022; China | HCC ; 55.7 (8); 22 | MBCT (61); WeChat audio and online platforms | Every day (texting) | 6 wk, 20 min/d for 5 d/wk | Adoption of the main issues and needs of patients with HCC | App | Waitlist (61) | Pre, post, 1 mo FU , 3 mo FU | Distress: HADS, sleep: PSQI , QOL: FACT-hep , and stress: PSS |
Messer et al [ ], 2020; US | Mixed; 51 (10.6); 76 | MBSR (11); guided meditation audio clips and brief textual lessons | — | 6 wk; mean duration of 12 min/session | — | Website | Usual care (10) | Pre and post | Distress: HADS, QOL: POMS-SF , and sleep: PSQI |
Nissen et al [ ], 2018; Denmark | Breast and prostate; 55.9 (12.1); 91 | MBCT (104); website written material, audio exercises, writing tasks, and videos | — | 10 wk, 10 sessions; 2 h/wk for 45 min/d | Program adjustments to meet the needs of survivors of cancer | Website | Waitlist (46) | Pre, post, and 6 mo FU | Anxiety: STAI‐Y , depression: BDI‐II , stress: PSS‐10, and sleep: ISI |
Peng et al [ ], 2022; China | Breast; 41.8 (2.9); 100 | MBP (30); website meeting 5P medicine approach | — | 6 wk, 6 sessions; 1.5 h/wk | On the basis of specific considerations for survivors of cancer | App | Usual care (30) | Pre, post, and 1 mo FU | FCR: FCRI-SF and QOL: Eortc-Qlq-C30 |
Rosen [ ], 2017; US | Breast; 53 (10.3); 100 | MBP (48); app-based courses include audio and video | General weekly check-in emails | 9 wk | — | App | Waitlist (47) | Pre, wk 5, wk 9, and wk 4 FU | QOL: FACT-B |
Rosen et al [ ], 2018; US | Breast; 51.6 (10.3); 100 | MBP (57); app-based audio and animated video | Weekly check-in email | 9 wk | — | App | Waitlist (55) | Pre, post, and 1 mo FU | QOL: FACT‐B |
Russell et al [ ], 2019; Australia | Melanoma; 53.4 (13.1); 54 | MBP (46); embedded short videos, PDF transcript of the videos, and MP3 audio email | Automatically generated email reminders twice daily | 6 wk | Survey to understand the knowledge of meditation among people with melanoma | Website | Waitlist (23) | Pre and post | FCR: FCRI and stress: PSS-10 |
Shen et al [ ], 2021; China | Breast; 47.4 (7.5); 100 | MBCR (37); online course, WeChat group, audio-video materials, and pictures | Every day (texting) | 8 wk, 8 sessions; 15 min/d for 6 d/wk | Combine rich experience in rehabilitation psychotherapy of breast cancer | App | Usual care (40) | Pre and post | Stress: CPSS and anxiety: SAS |
Wang [ ], 2022; China | Breast; 46.8 (7.9); 100 | MBCR (51); web-based courses and intervention materials | — | 4 wk, 4 sessions; 1.5 h/wk and 30 min daily | On the basis of the problems in the pilot study and participant feedback, adjusted internet-delivered MBCR program | Website | Usual care (52) | Pre and post | QOL: FACT-B |
Yousefi et al [ ], 2022; Iran | Colorectal and stomach; 54.9 (6.6); 42 | MBCR (25); web-based session | An alert reminder message was sent 2 h before each session | 9 wk, 9 sessions; 90 min/wk | Cancer-specific MBSR program was used in the study | Website | Usual care (25) | Pre, post, and 2 mo FU | Stress: DASS‐21 and sleep: ISI |
Zernicke et al [ ], 2014; Canada | Mixed; 58 (10.7); 72 | MBCR (30); web-based classroom, guided meditation recordings, and videos | — | 8 wk, 8 sessions; 45 min/d | Cancer-adapted MBSR | Website | Waitlist (32) | Pre and post | Depression and anxiety: POMS , stress: CSOSI , PTG: PTGI |
a MBSR: mindfulness-based stress reduction.
b Not applicable.
c DASS-21: Depression, Anxiety, and Stress Scale-21.
d MBCT: mindfulness-based cognitive therapy.
e HADS: Hospital Anxiety and Depression Scale.
f FCR: fear of cancer recurrence.
g FCRI: Fear of Cancer Recurrence Inventory.
h QOL: quality of life.
i SF-12: 12-item Short-Form health survey.
j MBP: mindfulness-based program.
k NCCN: National Comprehensive Cancer Network Distress Thermometer.
l PTG: posttraumatic growth.
m PTGI: 21-item Posttraumatic Growth Inventory.
n PROMIS: 8-item PROMIS Sleep Disturbance scale.
o FACT-G: 27-item Functional Assessment of Cancer Therapy General Scale.
p FACIT‐Pal: 46-item Functional Assessment of Chronic Illness Therapy—Palliative Care.
q HCC: hepatocellular carcinoma.
r FU: follow-up.
s PSQI: Pittsburgh Sleep Quality Index.
t FACT-Hep: Functional Assessment of Cancer Therapy-Hepatobiliary Carcinoma.
u PSS: Perceived Stress Scale.
v POMS-SF: Profile of Mood States-Short Form.
w STAI‐Y: State-Trait Anxiety Inventory Y-Form.
x BDI‐II: Beck Depression Inventory.
y ISI: Insomnia Severity Index.
z 5P: The specific name of an application designed to promote mind and brain health and cultivate happiness.
aa FCRI-SF: Fear of Cancer Recurrence Inventory-Short Form.
ab Eortc-Qlq-C30: European Organization for Research and Treatment of Cancer questionnaire.
ac FACT-B: Functional Assessment of Cancer Therapy-Breast version 4.
ad MBCR: Mindfulness-based cancer recovery.
ae CPSS: Chinese version of the Perceived Stress Scale.
af SAS: Self-Rating Anxiety Scale.
ag POMS: Profile of Mood States.
ah CSOSI: Calgary Symptoms of Stress Inventory.
Risk of Bias
The risk-of-bias assessment is presented in Multimedia Appendix 2 [ 31 - 45 ]. Most studies (9/15, 60%) adequately generated and concealed allocation ( Figure 2 ). In most studies (14/15, 93%), patient blinding was not possible because of the nature of online MBIs and was not considered to increase the risk of bias. However, of the 15 studies, 8 (53%) [ 31 , 33 , 37 - 40 , 42 , 44 ] presented insufficient information regarding researcher and outcome assessor blinding, whereas 7 (47%) reported blinding researchers [ 32 , 34 - 36 , 41 , 43 , 45 ] (low risk). A total of 14 studies reported complete outcome data (low risk), and 1 study had insufficient detail [ 44 ] (unclear risk). In 1 study [ 40 ], attrition was high and comparisons or reasons for attrition were not provided. Finally, 66% (10/15) of the studies did not reference a protocol or trial registration (unclear risk).

Meta-Analysis
Effects on qol.
A total of 8 studies reported the effects of app- and website-based MBIs on QOL among patients with cancer. To measure QOL in patients with cancer, 4 health-related QOL measures were used, including the Functional Assessment of Chronic Illness Therapy [ 34 ], the Functional Assessment of Cancer Therapy [ 33 , 35 , 39 , 40 , 43 ], the Short-Form 12 [ 32 ], and the European Organization for Research and Treatment of Cancer questionnaire [ 38 ], all of which have been validated in this patient population. Higher scores reflected a higher QOL. Because the physical and psychological components of the scale were measured separately and it was not possible to determine the overall change in the QOL, the data from 1 study [ 32 ] were not summarized. A total of 7 studies including 569 participants were evaluated in the meta-analysis. No significant heterogeneity was found between studies ( I 2 =26%; P =.23; Figure 3 [ 33 - 41 , 43 - 45 ]). The intervention group had a significant QOL improvement compared to the control group (SMD 0.37, 95% CI 0.18-0.57; P <.001). In addition, the exclusion of any single study at one time did not change the pooled results markedly.

Effects on Sleep
Five studies investigated the impact of app- and website-based MBIs on sleep quality using 3 assessment tools: the 8-item PROMIS Sleep Disturbance scale [ 33 ], the Insomnia Severity Index [ 37 , 44 ], and the Pittsburgh Sleep Quality Index [ 35 , 36 ]. A higher score indicated a worse sleep quality. Moderate heterogeneity of effect sizes was observed ( I ²=58%; P =.05; Figure 3 ). Grouping the studies by type of technology, scale, and intervention type did not resolve heterogeneity, so a random effects model was chosen to pool the results. The result revealed that app- and website-based MBIs could alleviate patients’ sleep issues, with a statistical difference (SMD −0.36, 95% CI −0.72 to −0.01; P =.04). Only 1 outlier was detected [ 36 ]. After omitting the studies from the analysis, the effect size dropped to an SMD of −0.25 (95% CI −0.54 to 0.04; P =.09), and heterogeneity reduced substantially ( I 2 =38%). The possible reason for this change may be attributed to the fact that small sample sizes tend to yield more pronounced effects.
Effects on FCR
A total of 3 studies measured FCR; the pooled data included 224 participants. Two FCR measures were used: FCRI [ 32 , 41 ] and the Short-Form FCRI [ 38 ]. A higher score indicated a higher level of FCR. There is great heterogeneity among the studies ( I 2 =86%; P =.009; Figure 3 ). After the data of the study by Russell et al [ 41 ] are eliminated by the method of eliminating one by one, there is significantly lower heterogeneity ( I 2 =0%; P =.70). This may be due to Russell et al [ 41 ] presurveying patients with cancer so that the intervention on FCR was more effective. The results showed that the difference between the network-based MBIs and the control group was not statistically significant (SMD −0.30, 95% CI −1.04 to 0.44; P =.39).
Effects on PTG
Two studies examined the effect of app- and website-based MBIs on PTG, with a total of 134 participants. The measurement tool exclusively used across 2 studies to assess PTG was the Posttraumatic Growth Inventory [ 33 , 45 ]. Higher scores indicated greater PTG. No significant heterogeneity was found between studies ( I 2 =0%; P =.38; Figure 3 ). We found that app- and website-based MBIs did not lead to a significant increase in PTG score (SMD 0.08, 95% CI −0.26 to 0.42; P =.66).
Effects on Anxiety
Anxiety levels were assessed in 6 studies using 5 validated scales. These scales include the Hospital Anxiety and Depression Scale (HADS) [ 33 , 34 ], the Depression Anxiety Stress Scale Depression Inventory [ 31 ], the State-Trait Anxiety Inventory Y-Form [ 37 ], the Self-Rating Anxiety Scale [ 42 ], and the Profile of Mood States [ 45 ]. Higher scores on these scales indicated elevated levels of anxiety. Meta-analysis showed that app- and website-based MBIs lead to a significant reduction in anxiety (SMD −0.48, 95% CI −0.75 to −0.20; P <.001; Figure 4 [ 31 - 37 , 41 , 42 , 44 , 45 ]). Moderate heterogeneity was found between studies ( I 2 =52%; P =.07). Grouping the studies by type of technology and intervention duration did not resolve heterogeneity ( Table 2 ). Furthermore, when we examined subgroups based on sex, we found that studies including female participants had a significantly larger pooled effect size (SMD −0.67, 95% CI −1.01 to −0.33; P <.001) than the studies including both male and female participants (referred to as the mixed-gender subgroup; SMD −0.39, 95% CI −0.76 to −0.02; P =.04; Figure 4 ). The differences across these 2 subgroups were statistically nonsignificant (χ 2 1 =1.2; P =.28).

Subgroup and stratification | Studies, n (%) | SMD (95% CI) | value for heterogeneity | value for pooled results | value for interaction | ||||||||||
.54 | |||||||||||||||
Website | 3 (50) | −0.57 (−0.82 to −0.31) | .47 | 0 | .0001 | ||||||||||
App | 3 (50) | −0.38 (−0.93 to 0.18) | .02 | 74 | .18 | ||||||||||
.43 | |||||||||||||||
<8 wk | 2 (33) | −0.62 (−0.97 to−0.27) | .57 | 0 | <.001 | ||||||||||
≥8 wk | 4 (67) | −0.41 (−0.81 to −0.01) | .03 | 67 | .04 | ||||||||||
.28 | |||||||||||||||
Female | 2 (33) | −0.67 (−1.01 to −0.33) | .41 | 0 | <.001 | ||||||||||
Mixed | 4 (67) | −0.39 (−0.76 to −0.02) | .05 | 62 | .04 | ||||||||||
.67 | |||||||||||||||
MBCR | 2 (33) | −0.70 (−0.05 to −0.36) | .55 | 0 | <.001 | ||||||||||
MBCT | 1 (17) | −0.43 (−0.82 to 0.03) | — | — | .04 | ||||||||||
MBIs | 2 (33) | −0.28 (−1.14 to 0.59) | .01 | 85 | .53 | ||||||||||
MBSR | 1 (17) | −0.52(−1.02 to −0.02) | — | — | .04 | ||||||||||
.07 | |||||||||||||||
Unclear risk | 5 (83) | −0.43 (−0.74 to −0.12) | .06 | 56 | .007 | ||||||||||
High risk | 1 (17) | −0.72 (−1.20 to −0.24) | — | — | .004 | ||||||||||
.68 | |||||||||||||||
Website | 4 (80) | −0.87 (−1.44 to −0.29) | .002 | 80 | .003 | ||||||||||
App | 1 (20) | −1.02 (−1.50 to −0.55) | — | — | <.001 | ||||||||||
<.001 | |||||||||||||||
MBCR | 3 (60) | −0.96 (−1.27 to−0.66) | .39 | 0 | <.001 | ||||||||||
MBCT | 1 (20) | −0.21 (-0.61 to 0.18) | — | — | .29 | ||||||||||
MBIs | 1 (20) | −1.41 (−1.97 to −0.86) | — | — | <.001 | ||||||||||
.33 | |||||||||||||||
PROMs | 1 (20) | −0.09 (−0.55 to 0.38) | — | — | .72 | ||||||||||
PSQI | 2 (40) | −0.78 (−1.58 to 0.02) | .11 | 60 | .05 | ||||||||||
ISI | 2 (40) | −0.23 (−0.83 to 0.36) | .09 | 65 | .44 | ||||||||||
.20 | |||||||||||||||
Website | 3 (60) | −0.52 (−1.22 to 0.19) | .02 | 75 | .15 | ||||||||||
App | 2 (40) | −0.02 (−0.32 to 0.28) | .70 | 0 | .91 | ||||||||||
.16 | |||||||||||||||
<8 wk | 2 (40) | −0.78 (−1.58 to −0.02) | .11 | 60 | .05 | ||||||||||
≥8 wk | 3 (60) | −0.16 (−0.49 to 0.17) | .23 | 32 | .35 | ||||||||||
.11 | |||||||||||||||
Unclear risk | 4 (80) | −2.03 (−2.93 to −1.13) | .12 | 49 | <.001 | ||||||||||
High risk | 1 (20) | 0.20 (−2.93 to 2.79) | — | — | .88 |
a SMD: standardized mean difference.
b MBCR: mindfulness-based cancer recovery.
c MBCT: mindfulness-based cognitive therapy.
d Not applicable.
e MBI: mindfulness-based intervention.
f MBSR: mindfulness-based stress reduction.
g Unclear risk: unclear risk of bias for one or more key domains.
h High risk: high risk of bias for one or more key domains.
i PROM: patient‐reported outcome measure.
j PSQI: Pittsburgh Sleep Quality Index.
k ISI: Insomnia Severity Index.
Effects on Depression
Depression was assessed across 5 studies using various standardized instruments. These included the Depression Anxiety Stress Scale-21 [ 31 ], HADS [ 33 , 34 ], Beck Depression Inventory [ 37 ], and Profile of Mood States [ 45 ]. Elevated levels of depression were indicated by higher scores on these scales. The pooled data included 384 participants and showed a significant difference in improvement between the intervention and control groups (SMD −0.36, 95% CI −0.61 to −0.11; P =.005; Figure 4 ). Moderate heterogeneity of effect sizes was observed ( I 2 =31%; P =.21). In the sensitivity analysis using the one-study-out method, we found that the pooled estimates were not significantly altered when any 1 study was omitted in turn. The range of P values obtained varied from .0001 to .03, indicating that the summary effect size is robust.
Effects on Perceived Stress
A total of studies investigated the effects of app- and website-based MBIs on stress. Four distress measures were used: the Perceived Stress Scale [ 35 , 37 , 41 ], the Chinese version of the Perceived Stress Scale [ 42 ], the Depression and Stress Scale [ 44 ], and the Calgary Symptoms of Stress Inventory [ 45 ]. A total of 5 studies including 366 participants were evaluated in the meta-analysis. The data from 1 study was not pooled because the mean values and SD of outcomes were not reported [ 35 ], and between-study heterogeneity was found ( I 2 =75%; P =.003; Figure 4 ). This meta-analysis revealed a reduction in stress of −0.89 (95% CI −1.33 to −0.45) when comparing the intervention group to the control group at the postintervention stage.
To further explore the potential sources of heterogeneity, we conducted subgroup analyses by type of technology and intervention type ( Table 2 ). The 2 studies using apps (SMD −1.02, 95% CI −1.50 to −0.55; I 2 =0) were found to have low heterogeneity, whereas the 3 studies based on website-based technologies (SMD −0.87, 95% CI −1.44 to −0.29; P =.002) exhibited higher heterogeneity. After conducting sensitivity analysis and eliminating 1 study at a time, the exclusion of the study by Nissen et al [ 37 ] resulted in significantly lower heterogeneity (I 2 =21%; P =.28). One possible reason is that the study by Nissen et al [ 37 ], which offered internet-delivered MBCT as a routine based on a screening procedure, may have included less motivated participants compared to studies with self-referral. In addition, Nissen et al [ 37 ] used a lower cutoff value for screening the study population, which could have resulted in a floor effect.
Effects on Distress
In the analysis of distress (involving 5 studies), HADS [ 32 , 35 , 36 ] and the National Comprehensive Cancer Network Distress Thermometer [ 33 , 34 ] were used to assess the current distress level. Low heterogeneity was found between studies ( I 2 =30%; P =.22; Figure 4 ), and the random effects model indicated that app- and website-based MBIs were associated with reduced distress levels in patients with cancer (SMD −0.50, 95% CI −0.75 to −0.26; P <.001).
Subgroup Analysis
Table 2 displays the results of subgroup analyses that were conducted to investigate the heterogeneity in the association between anxiety, perceived stress, and sleep in the context of MBIs. To explain the variability in the effects of mindfulness, we examined various moderating variables, such as technology, sex, intervention type, intervention duration, study quality, and scale. No statistically significant variables were found in the subgroup analysis of anxiety and sleep, whereas the type of intervention ( P <.001) was a significant moderating variable for perceived stress
Publication Bias
Funnel plots and statistical tests were not performed as any of the outcomes had at least 10 studies to ensure sufficient power in detecting asymmetry [ 46 ]. However, we reduced the possibility of publication bias by conducting a thorough search across multiple databases to identify published studies [ 47 ].
Details of the RE-AIM Framework assessment are presented in Multimedia Appendix 3 [ 31 - 45 ]. Of the 15 studies, 14 (93%) reported 13% to 92% of eligible patients. Efficacy (effect size and 95% CI of primary outcome) was reported in 33% (5/15) of the studies [ 33 , 35 , 37 , 39 , 40 ] (Cohen d or η 2 ). For adoption barriers, health professionals or researchers conducted recruitment for all studies, and 53% (8/15) of the studies [ 35 - 38 , 41 - 44 ] recruited participants in person (hospital and cancer center). For implementation, intervention adherence ranged from 59% to 100% of participants completing all scheduled components. Dropouts of most complex interventions ranged from 0% to 48%, with 40% (6/15) of the studies [ 31 , 38 - 40 , 42 , 45 ] having <10% dropouts. The cost was reported in 4 studies [ 33 , 34 , 39 , 40 ], including the paid app (priced at US $77 for 6 months and US $69.99 for 12 months) and the app already publicly available. In total, 46% (7/15) of the studies [ 35 , 37 - 40 , 43 , 44 ] reported maintenance of results, and 46% (7/15) of the studies [ 35 , 37 - 40 , 43 , 44 ] sustained results for 1 to 9 months. Four studies [ 33 , 34 , 39 , 40 ] explicitly reported on the potential for the interventions to remain accessible or whether there were plans for their continued implementation.
Principal Findings
The objective of this study is to assess the effectiveness of MBIs in improving the mental health and QOL of patients with cancer. We discovered that patients’ QOL can be greatly enhanced by app- and website-based MBIs, which also significantly lowers psychological distress, sleep problems, anxiety, depression, and perceived stress. This systematic review of meta-analyses and the RE-AIM framework demonstrate that app- and website-based interventions have a wide range of effects and are highly used by different (international and multilingual) patients with cancer. However, the use and accessibility of app- and website-based MBIs for patients with cancer have been constrained because of service fees and patient mobility limitations [ 48 ]; app- and website-based MBIs are mainly conducted in high-income countries. The possible explanation is the distinction between communication and economy; some high-income countries may have national health services in place to promote app- and website-based MBIs, whereas developing nations may not. Study shows that in many low- and middle-income countries, the accessibility of evidence-based mental health treatments remains limited [ 49 ]. The time commitment, teacher shortage, and high cost of classic mindfulness interventions may have hindered efforts to spread the associated benefits to individuals in developing countries [ 50 ]. For instance, Indonesia has yet to implement evidence-based internet-based mindfulness therapy, emphasizing the need for expanding evidence-based mental health interventions in resource-constrained settings.
The results of this study suggest that app- and website-based MBIs are effective in improving QOL and reducing anxiety and depressive symptoms in patients with cancer, which is consistent with previous meta-analyses [ 18 , 20 ]. A possible explanation for this is that app- and website-based MBIs can alleviate negative emotions, enhance positive emotions, and increase mindfulness skills among patients with cancer, as elaborated by previous research [ 51 ]. Moreover, the sleep quality of patients with cancer also improved after MBIs. This outcome may be attributed to the inclusion of techniques in the program that target sleep difficulties [ 7 ] and the nonjudging aspect of mindfulness, which can enhance sleep quality by mitigating stress and everyday tensions. Previous studies [ 52 ] have confirmed the moderate effect of mindfulness interventions on sleep quality, which suggests that the use of app- and website-based MBIs to manage QOL and sleep in patients with cancer should be further supported.
App- and website-based MBIs have shown potential in helping patients with cancer develop emotional regulation skills and cope with the distress associated with diagnosis and treatment [ 53 ]. It makes patients feel better emotionally and physically and helps patients with cancer reduce their psychological distress [ 54 ]. Incorporating MBIs into oncological treatment can promote emotional and physical well-being and alleviate psychological distress [ 55 ]. MBIs have been found to regulate biological variables associated with stress [ 56 ], such as immune function, hypothalamic-pituitary-adrenal regulation, and autonomic nervous system activity, thereby reducing pressure on patients. The data from this review showed that MBCR appeared to be particularly effective in reducing perceived stress, whereas MBCT was not effective in reducing stress after the intervention [ 51 ]. This finding was unexpected, given that many previous studies have suggested the effectiveness of MBCT in reducing stress [ 57 ]. However, because of the limited number of included studies, it is difficult to draw definitive conclusions regarding the comparative effectiveness of different MBIs.
However, although not statistically significant, app- and website-based MBIs can improve the level of PTG and FCR in patients with cancer. FCR is one of the most common problems of survivors of cancer, and it has been known that FCR can persist throughout the treatment and survival trajectory [ 58 ]; thus, specific intervention is needed for survivors of cancer who have clinically significant FCR. Previous meta-analysis showed that cognitive therapy and mindfulness exercises are very suitable for combating FCR [ 59 ]. Numerous psychological and behavioral mechanisms of change within mindfulness interventions have been suggested, encompassing acceptance, emotion regulation skills, and the reduction of ruminative thoughts [ 60 ]. The meta-analysis by Gu et al [ 61 ] provided empirical confirmation that rumination significantly mediates the impact of MBIs on mental health outcomes. In addition, the study by Butow et al [ 62 ] identified rumination as a crucial psychological mechanism associated with FCR. Therefore, the study suggests that the effectiveness of mindfulness interventions in addressing the FCR may be attributed to their potential to improve patients’ levels of rumination. The improved PTG observed in this study may be explained by the systematic training in moment-by-moment awareness, and MBIs focus on viewing thoughts and feelings as mental events [ 63 ]. Such a decentered relationship enables a perception of mental events as aspects of experience moving through awareness, showing that mindfulness practice supports personal growth and transformation.
In this study, it was observed that short-term MBIs with a duration of <8 weeks exhibited a larger effect size concerning the outcomes of anxiety and sleep. In the study by Wang et al [ 43 ], short-term MBIs were found to be more effective in improving physical health compared to long-term MBIs, and interventions lasting <8 weeks demonstrated a greater effect size, possibly attributed to the increased participant engagement resulting from the shorter intervention duration and simplified intervention complexity. Shorter interventions may be more feasible and acceptable for patients with cancer who are dealing with a range of physical and emotional challenges [ 64 ]. Future research should aim to replicate and expand on these results, including investigating the optimal duration and timing of app- and website-based MBIs for patients with cancer.
Recommendations for Future Research
To the best of our knowledge, this study represents the first meta-analysis using the RE-AIM framework, systematically reviewing and synthesizing the effectiveness of MBIs for patients with cancer across various types of interventions. By accurately reporting the RE-AIM dimensions, this study seeks to enhance the replicability and universality of mindfulness interventions in oncology settings. Our assessment of app- and website-based MBIs for patients with cancer, conducted within the framework of RE-AIM, reveals that the participation rates of eligible patients range from 13% to 92%. The calculated median participation rate, at 67% (IQR 47.5%-82%), emphasizes the effectiveness of the interventions in reaching a substantial portion of the target population. However, only a minority of studies reported on efficacy, which limited our ability to draw conclusions on overall effectiveness. Recruitment was primarily conducted by health professionals or researchers, and more than half of the studies (8/15, 53%) recruited participants in person, potentially limiting generalizability. Intervention adherence was generally high, but dropout rates varied widely, indicating that certain interventions may be more challenging for some patients. Cost was reported in only a few studies (4/15, 27%), with implications for accessibility. Long-term effects were reported in more than half of the studies (7/15, 47%), highlighting the need for further research. This study underscores the importance of considering the RE-AIM framework in the implementation and evaluation of these interventions. Further research is needed to fully understand their potential benefits and limitations in real-world settings.
Internet-based interventions have previously been shown to be effective for anxiety disorders and fear-related disorders and have achieved the same effect as face-to-face treatment [ 65 ]. Consistent with the results of this study, delivery via the internet, group, or app is feasible and effective. Our results suggest that among forms of online MBIs for patients with cancer, the most widely studied type was website-based interventions. This observation is in line with an analysis conducted in recent years [ 66 ], which indicated that the most widely studied type of telehealth for patients with breast cancer was website-based interventions. Website-based MBIs may offer more content, functionality, and instruction than app-based interventions, which may enhance user engagement, learning, and practice of positive thinking skills [ 67 , 68 ]. Website-based MBIs had higher completion rates and lower attrition rates compared to app-based interventions, which may be due to factors such as convenience, accessibility, engagement, and personalization [ 69 ]. Finally, in our review, a website-based study [ 41 ] that greatly improved FCR and stress highlighted the sustainability and self-management of the intervention and enabled flexible navigation by accessing website content according to user preferences. Therefore, website-based MBIs may offer more opportunities for personalization and tailoring interventions to individual needs.
In our analysis, 53% (8/15) of the studies implemented a weekly or daily reminder system through various channels, such as email, text messages, apps, or smartphone notifications, to facilitate app- and website-based MBIs. However, the prevalence of reminder systems in the studies under review is relatively limited (7/15, 47%), a finding consistent with the investigation by Matis et al [ 19 ]. Matis et al conducted a systematic evaluation in this field, discussing the limited prevalence of reminder systems in reviewed studies and highlighting the current lack of direct comparisons between interventions with and without reminders. In addition, the study found that the frequency of reminders was positively associated with the magnitude of the intervention effect [ 70 ]. Consequently, to promote patient involvement in app- and website-based MBIs, it is vital to set reminders [ 67 ]. Some studies have also set up expert feedback, answers, and a variety of supervision methods to avoid reduced patient compliance. Therefore, app- and website-based MBIs can enhance engagement using features such as reminders, feedback, personalization, and facilitator-led components. However, it is important to note that the specific frequency, timing, and content of the reminders may vary depending on the individual and the context of the intervention. Our study results reveal heterogeneity in the types, frequencies, and content of reminder systems, preventing the establishment of specific standards for their effectiveness. Despite the evident practicality of reminder systems, a more comprehensive investigation into their types, frequencies, and effectiveness is imperative within the context of app- and website-based MBIs.
This systematic review found that most app- and website-based interventions have adopted online classrooms; application-based measures to implement mindfulness interventions; and multicomponent interventions that include audio, video, and documents. However, the study did not clarify which factors affect behavioral changes. Despite these differences, 67% (10/15) of the interventions are designed specifically for the cancer population and provide customizable interventions. For example, as demonstrated by Wang et al [ 43 ], a pilot website-based MBIs was conducted for patients with cancer; this is an adapted version of MBSR specifically tailored for individuals dealing with cancer-related stressors. The MBCR program retains the core principles and practices of MBSR while integrating specific intervention materials to address challenges associated with cancer, such as common experiences related to cancer, sleep issues, pain, and FCR, which is greatly beneficial for improving the physical and mental symptoms of patients with cancer. MBCR will provide a platform for patients with cancer to engage in discussions and address challenges related to cancer. Future app- and website-based MBIs should take into account the characteristics of patients and determine which intervention plan is most suitable for patients with cancer, emphasizing feedback sessions and communication with therapists to enable patients to learn self-management and make intervention plans sustainable.
Limitations
Although this review summarizes international RCTs for various outcomes, there are limitations. First, because the research results are measured by various tools, it may hinder the comparability of research outcomes. Second, in the 15 trials, there are differences in the personnel, duration, and methods of app- and website-based MBIs in various studies. Patients included in these studies have different characteristics. Third, The inability to access or adequately translate studies in languages other than English and Chinese may introduce bias into the selection process, potentially limiting the comprehensiveness of the findings. Finally, in the subgroup analysis, the study of each subgroup is limited, which may reduce the ability to draw conclusions on the differences in the consistency of intervention effects between subgroups. The abovementioned factors may lead to heterogeneity between studies, which is closely related to the summary results, so these results need to be interpreted carefully. Nevertheless, the meta-analysis included RCTs only and used a random effect model to pool results to give the most conservative estimates. In addition, subgroup analysis and sensitivity analysis were conducted and showed that the pooled estimations were relatively robust.
Conclusions
This meta-analysis provides definite evidence regarding the efficacy of app- and website-based MBIs for patients with cancer. Our findings suggest that app- and website-based MBIs can be effective in improving OL, sleep, and mental health and can be integrated into stepped care in clinical practice. Future experiments should pay more attention to the development of intervention programs based on the wishes and characteristics of patients with cancer and study how to optimize interventions further and customize interventions based on individual physical and mental symptoms.
Acknowledgments
This study was supported by the Exploration of Trajectories and Intervention Program of Frailty for Gastric Cancer Survivors Based on the Health Ecology Theory project supported by the National Natural Science Foundation of China (number 82073407), the Studies on Construction of Core Competency Model and Development of Assessment Tool for Nurses of Hospice Care project supported by the National Natural Science Foundation of China (number 72004099), and the Basic Science (Natural Science) Foundation of Higher Education Institutions of Jiangsu Province (project number 22KJB320013).
Data Availability
All data generated or analyzed during this study are included in this published article and its supplementary information files.
Authors' Contributions
TW and XJ developed the key ideas for the manuscript and the hypotheses. TW developed the search strategy and conducted the literature searches. YG, XJ, and CT conducted screening and coding. QX, CT, and SZ contributed to manuscript review and editing. TW conducted the statistical analyses, summarized the findings, and prepared the initial draft of the manuscript. All authors contributed to and approved the final manuscript.
Conflicts of Interest
None declared.
Search strategy.
Summary of the risk of bias of studies included in the systematic review.
RE-AIM (Reach, Effectiveness, Adoption, Implementation, and Maintenance) framework.
Preferred Reporting Items for Systematic Reviews and Meta-Analyses (PRISMA) checklist.
- Sung H, Ferlay J, Siegel RL, Laversanne M, Soerjomataram I, Jemal A, et al. Global cancer statistics 2020: GLOBOCAN estimates of incidence and mortality worldwide for 36 cancers in 185 countries. CA Cancer J Clin. May 2021;71(3):209-249. [ FREE Full text ] [ CrossRef ] [ Medline ]
- Choi HC, Lam KO, Pang HH, Tsang SK, Ngan RK, Lee AW. Global comparison of cancer outcomes: standardization and correlation with healthcare expenditures. BMC Public Health. Aug 07, 2019;19(1):1065. [ FREE Full text ] [ CrossRef ] [ Medline ]
- Siegel RL, Miller KD, Wagle NS, Jemal A. Cancer statistics, 2023. CA Cancer J Clin. Jan 2023;73(1):17-48. [ FREE Full text ] [ CrossRef ] [ Medline ]
- Wei X, Yuan R, Yang J, Zheng W, Jin Y, Wang M, et al. Effects of Baduanjin exercise on cognitive function and cancer-related symptoms in women with breast cancer receiving chemotherapy: a randomized controlled trial. Support Care Cancer. Jul 2022;30(7):6079-6091. [ CrossRef ] [ Medline ]
- Mead KH, Raskin S, Willis A, Arem H, Murtaza S, Charney L, et al. Identifying patients' priorities for quality survivorship: conceptualizing a patient-centered approach to survivorship care. J Cancer Surviv. Dec 2020;14(6):939-958. [ CrossRef ] [ Medline ]
- Nekhlyudov L, Mollica MA, Jacobsen PB, Mayer DK, Shulman LN, Geiger AM. Developing a quality of cancer survivorship care framework: implications for clinical care, research, and policy. J Natl Cancer Inst. Nov 01, 2019;111(11):1120-1130. [ FREE Full text ] [ CrossRef ] [ Medline ]
- Schell LK, Monsef I, Wöckel A, Skoetz N. Mindfulness-based stress reduction for women diagnosed with breast cancer. Cochrane Database Syst Rev. Mar 27, 2019;3(3):CD011518. [ FREE Full text ] [ CrossRef ] [ Medline ]
- Hadash Y, Bernstein A. Behavioral assessment of mindfulness: defining features, organizing framework, and review of emerging methods. Curr Opin Psychol. Aug 2019;28:229-237. [ CrossRef ] [ Medline ]
- Forte P, Abate V, Bolognini I, Mazzoni O, Quagliariello V, Maurea N, et al. Mindfulness-based stress reduction in cancer patients: impact on overall survival, quality of life and risk factor. Eur Rev Med Pharmacol Sci. Sep 2023;27(17):8190-8197. [ FREE Full text ] [ CrossRef ] [ Medline ]
- Mathew A, Doorenbos AZ, Jang MK, Hershberger PE. Acceptance and commitment therapy in adult cancer survivors: a systematic review and conceptual model. J Cancer Surviv. Jun 2021;15(3):427-451. [ FREE Full text ] [ CrossRef ] [ Medline ]
- Zhang D, Lee EK, Mak EC, Ho CY, Wong SY. Mindfulness-based interventions: an overall review. Br Med Bull. Jun 10, 2021;138(1):41-57. [ FREE Full text ] [ CrossRef ] [ Medline ]
- Segal Z, Dimidjian S, Vanderkruik R, Levy J. A maturing mindfulness-based cognitive therapy reflects on two critical issues. Curr Opin Psychol. Aug 2019;28:218-222. [ FREE Full text ] [ CrossRef ] [ Medline ]
- Schellekens MP, Tamagawa R, Labelle LE, Speca M, Stephen J, Drysdale E, et al. Mindfulness-Based Cancer Recovery (MBCR) versus Supportive Expressive Group Therapy (SET) for distressed breast cancer survivors: evaluating mindfulness and social support as mediators. J Behav Med. Jun 2017;40(3):414-422. [ FREE Full text ] [ CrossRef ] [ Medline ]
- Johns SA, Tarver WL, Secinti E, Mosher CE, Stutz PV, Carnahan JL, et al. Effects of mindfulness-based interventions on fatigue in cancer survivors: a systematic review and meta-analysis of randomized controlled trials. Crit Rev Oncol Hematol. Apr 2021;160:103290. [ FREE Full text ] [ CrossRef ] [ Medline ]
- Park S, Sato Y, Takita Y, Tamura N, Ninomiya A, Kosugi T, et al. Mindfulness-based cognitive therapy for psychological distress, fear of cancer recurrence, fatigue, spiritual well-being, and quality of life in patients with breast cancer-a randomized controlled trial. J Pain Symptom Manage. Aug 2020;60(2):381-389. [ FREE Full text ] [ CrossRef ] [ Medline ]
- Toivonen KI, Zernicke K, Carlson LE. Web-based mindfulness interventions for people with physical health conditions: systematic review. J Med Internet Res. Aug 31, 2017;19(8):e303. [ FREE Full text ] [ CrossRef ] [ Medline ]
- van Agteren J, Iasiello M, Lo L, Bartholomaeus J, Kopsaftis Z, Carey M, et al. A systematic review and meta-analysis of psychological interventions to improve mental wellbeing. Nat Hum Behav. May 2021;5(5):631-652. [ CrossRef ] [ Medline ]
- Xunlin NG, Lau Y, Klainin-Yobas P. The effectiveness of mindfulness-based interventions among cancer patients and survivors: a systematic review and meta-analysis. Support Care Cancer. Apr 2020;28(4):1563-1578. [ CrossRef ] [ Medline ]
- Matis J, Svetlak M, Slezackova A, Svoboda M, Šumec R. Mindfulness-based programs for patients with cancer via ehealth and mobile health: systematic review and synthesis of quantitative research. J Med Internet Res. Nov 16, 2020;22(11):e20709. [ FREE Full text ] [ CrossRef ] [ Medline ]
- Fung JY, Lim H, Vongsirimas N, Klainin-Yobas P. Effectiveness of eHealth mindfulness-based interventions on cancer-related symptoms among cancer patients and survivors: a systematic review and meta-analysis. J Telemed Telecare. Apr 2024;30(3):451-465. [ CrossRef ] [ Medline ]
- Bu S, Smith A, Janssen A, Donnelly C, Dadich A, Mackenzie LJ, et al. Optimising implementation of telehealth in oncology: a systematic review examining barriers and enablers using the RE-AIM planning and evaluation framework. Crit Rev Oncol Hematol. Dec 2022;180:103869. [ CrossRef ] [ Medline ]
- Cumpston M, Li T, Page MJ, Chandler J, Welch VA, Higgins JP, et al. Updated guidance for trusted systematic reviews: a new edition of the Cochrane Handbook for Systematic Reviews of Interventions. Cochrane Database Syst Rev. Oct 03, 2019;10(10):ED000142. [ FREE Full text ] [ CrossRef ] [ Medline ]
- Page MJ, McKenzie JE, Bossuyt PM, Boutron I, Hoffmann TC, Mulrow CD, et al. The PRISMA 2020 statement: an updated guideline for reporting systematic reviews. BMJ. Mar 29, 2021;372:n71. [ FREE Full text ] [ CrossRef ] [ Medline ]
- Page MJ, Li T, Cumpston M, Welch VA, Higgins JP, Thomas J, et al. Cochrane Handbook for Systematic Reviews of Interventions, Second Edition. London, UK. The Cochrane Collaboration; 2019.
- Andrade C. Mean difference, standardized mean difference (SMD), and their use in meta-analysis: as simple as it gets. J Clin Psychiatry. Sep 22, 2020;81(5):20f13681. [ FREE Full text ] [ CrossRef ] [ Medline ]
- Higgins JP, Thompson SG. Quantifying heterogeneity in a meta-analysis. Stat Med. Jun 15, 2002;21(11):1539-1558. [ CrossRef ] [ Medline ]
- Borenstein M, Hedges LV, Higgins JP, Rothstein HR. A basic introduction to fixed-effect and random-effects models for meta-analysis. Res Synth Methods. Apr 2010;1(2):97-111. [ CrossRef ] [ Medline ]
- Hodgson W, Kirk A, Lennon M, Janssen X, Russell E, Wani C, et al. RE-AIM (reach, effectiveness, adoption, implementation, and maintenance) evaluation of the use of activity trackers in the clinical care of adults diagnosed with a chronic disease: integrative systematic review. J Med Internet Res. Nov 13, 2023;25:e44919. [ FREE Full text ] [ CrossRef ] [ Medline ]
- Glasgow RE, Harden SM, Gaglio B, Rabin B, Smith ML, Porter GC, et al. RE-AIM planning and evaluation framework: adapting to new science and practice with a 20-year review. Front Public Health. Mar 29, 2019;7:64. [ FREE Full text ] [ CrossRef ] [ Medline ]
- Singleton AC, Raeside R, Hyun KK, Partridge SR, Di Tanna GL, Hafiz N, et al. Electronic health interventions for patients with breast cancer: systematic review and meta-analyses. J Clin Oncol. Jul 10, 2022;40(20):2257-2270. [ FREE Full text ] [ CrossRef ] [ Medline ]
- Chang YC, Chiu CF, Wang CK, Wu CT, Liu LC, Wu YC. Short-term effect of internet-delivered mindfulness-based stress reduction on mental health, self-efficacy, and body image among women with breast cancer during the COVID-19 pandemic. Front Psychol. Oct 25, 2022;13:949446. [ FREE Full text ] [ CrossRef ] [ Medline ]
- Compen F, Bisseling E, Schellekens M, Donders R, Carlson L, van der Lee M, et al. Face-to-face and internet-based mindfulness-based cognitive therapy compared with treatment as usual in reducing psychological distress in patients with cancer: a multicenter randomized controlled trial. J Clin Oncol. Aug 10, 2018;36(23):2413-2421. [ CrossRef ]
- Kubo A, Kurtovich E, McGinnis M, Aghaee S, Altschuler A, Quesenberry CJ, et al. A randomized controlled trial of mHealth mindfulness intervention for cancer patients and informal cancer caregivers: a feasibility study within an integrated health care delivery system. Integr Cancer Ther. May 16, 2019;18:1534735419850634. [ FREE Full text ] [ CrossRef ] [ Medline ]
- Kubo A, Kurtovich E, McGinnis M, Aghaee S, Altschuler A, Quesenberry CJ, et al. Pilot pragmatic randomized trial of mHealth mindfulness-based intervention for advanced cancer patients and their informal caregivers. Psychooncology. Feb 2024;33(2):e5557. [ CrossRef ] [ Medline ]
- Liu Z, Li M, Jia Y, Wang S, Zheng L, Wang C, et al. A randomized clinical trial of guided self-help intervention based on mindfulness for patients with hepatocellular carcinoma: effects and mechanisms. Jpn J Clin Oncol. Mar 03, 2022;52(3):227-236. [ CrossRef ] [ Medline ]
- Messer D, Horan JJ, Larkey LK, Shanholtz CE. Effects of internet training in mindfulness meditation on variables related to cancer recovery. Mindfulness. Jun 4, 2019;10(10):2143-2151. [ CrossRef ]
- Nissen ER, O'Connor M, Kaldo V, Højris I, Borre M, Zachariae R, et al. Internet-delivered mindfulness-based cognitive therapy for anxiety and depression in cancer survivors: a randomized controlled trial. Psychooncology. Jan 18, 2020;29(1):68-75. [ FREE Full text ] [ CrossRef ] [ Medline ]
- Peng L, Yang Y, Chen M, Xu C, Chen Y, Liu R, et al. Effects of an online mindfulness-based intervention on Fear of Cancer Recurrence and quality of life among Chinese breast cancer survivors. Complement Ther Clin Pract. Nov 2022;49:101686. [ CrossRef ] [ Medline ]
- Rosen KD. Is there an app for that? An exploratory randomized controlled trial of app-based mindfulness training for women with breast cancer. The University of Texas at San Antonio. 2016. URL: https://www.proquest.com/openview/025a3306918540d35302bcee3e4ac77b/1?pq-origsite=gscholar&cbl=18750 [accessed 2024-06-04]
- Rosen KD, Paniagua SM, Kazanis W, Jones S, Potter JS. Quality of life among women diagnosed with breast cancer: a randomized waitlist controlled trial of commercially available mobile app-delivered mindfulness training. Psychooncology. Aug 01, 2018;27(8):2023-2030. [ CrossRef ] [ Medline ]
- Russell L, Ugalde A, Orellana L, Milne D, Krishnasamy M, Chambers R, et al. A pilot randomised controlled trial of an online mindfulness-based program for people diagnosed with melanoma. Support Care Cancer. Jul 30, 2019;27(7):2735-2746. [ CrossRef ] [ Medline ]
- Shen A, Qiang W, Wang Q, Zhao Z, Wang S. Effects of online mindfulness-based cancer rehabilitation on perceived stress, fatigue, and anxiety in transitional breast cancer survivors. J Nurs Train. 2021;36(23):2123. [ CrossRef ]
- Wang L, Chen X, Peng Y, Zhang K, Ma J, Xu L, et al. Effect of a 4-week internet-delivered mindfulness-based cancer recovery intervention on the symptom burden and quality of life of patients with breast cancer: randomized controlled trial. J Med Internet Res. Nov 22, 2022;24(11):e40059. [ FREE Full text ] [ CrossRef ] [ Medline ]
- Yousefi A, Arani AM, Ghadiany M, Jafari M, Lighvan MA, Asanjarani B, et al. The effectiveness of online mindfulness-based cancer recovery program on psychological variables of colorectal and stomach cancer patients: a randomized control trial. Med Sci. Feb 2022;26(120). [ CrossRef ]
- Zernicke KA, Campbell TS, Speca M, McCabe-Ruff K, Flowers S, Carlson LE. A randomized wait-list controlled trial of feasibility and efficacy of an online mindfulness-based cancer recovery program: the eTherapy for cancer applying mindfulness trial. Psychosom Med. May 2014;76(4):257-267. [ CrossRef ] [ Medline ]
- Drucker AM, Fleming P, Chan AW. Research techniques made simple: assessing risk of bias in systematic reviews. J Invest Dermatol. Nov 2016;136(11):e109-e114. [ FREE Full text ] [ CrossRef ] [ Medline ]
- Oberoi S, Yang J, Woodgate RL, Niraula S, Banerji S, Israels SJ, et al. Association of mindfulness-based interventions with anxiety severity in adults with cancer: a systematic review and meta-analysis. JAMA Netw Open. Aug 03, 2020;3(8):e2012598. [ FREE Full text ] [ CrossRef ] [ Medline ]
- Vrontaras N, Koulierakis G, Ntourou I, Karakatsoulis G, Sergentanis TN, Kyrou D, et al. Psychosocial interventions on the posttraumatic growth of adults with cancer: a systematic review and meta-analysis of clinical trials. Psychooncology. Dec 2023;32(12):1798-1826. [ CrossRef ] [ Medline ]
- Listiyandini RA, Andriani A, Kusristanti C, Moulds M, Mahoney A, Newby JM. Culturally adapting an internet-delivered mindfulness intervention for Indonesian university students experiencing psychological distress: mixed methods study. JMIR Form Res. Aug 31, 2023;7:e47126. [ FREE Full text ] [ CrossRef ] [ Medline ]
- Wu R, Liu LL, Zhu H, Su WJ, Cao ZY, Zhong SY, et al. Brief mindfulness meditation improves emotion processing. Front Neurosci. 2019;13:1074. [ FREE Full text ] [ CrossRef ] [ Medline ]
- Cillessen L, van de Ven MO, Compen FR, Bisseling EM, van der Lee ML, Speckens AE. Predictors and effects of usage of an online mindfulness intervention for distressed cancer patients: usability study. J Med Internet Res. Oct 02, 2020;22(10):e17526. [ FREE Full text ] [ CrossRef ] [ Medline ]
- Burrowes SA, Goloubeva O, Stafford K, McArdle PF, Goyal M, Peterlin BL, et al. Enhanced mindfulness-based stress reduction in episodic migraine-effects on sleep quality, anxiety, stress, and depression: a secondary analysis of a randomized clinical trial. Pain. Mar 01, 2022;163(3):436-444. [ FREE Full text ] [ CrossRef ] [ Medline ]
- Glynn BA, Khoo EL, MacLeay HM, Duong A, Cantave R, Poulin PA. Exploring cancer patients' experiences of an online mindfulness-based program: a qualitative investigation. Mindfulness (N Y). 2020;11(7):1666-1677. [ FREE Full text ] [ CrossRef ] [ Medline ]
- King K. How ‘mindfulness’ can improve mental focus and help you feel better emotionally and physically. WTOP News. Jul 28, 2020. URL: https://wtop.com/health-fitness/2020/07/how-mindfulness-can-improve-mental-focus-and-help-you-feel-better-emotionally-and-physically/ [accessed 2024-06-04]
- Cillessen L, Johannsen M, Speckens AE, Zachariae R. Mindfulness-based interventions for psychological and physical health outcomes in cancer patients and survivors: a systematic review and meta-analysis of randomized controlled trials. Psychooncology. Dec 2019;28(12):2257-2269. [ FREE Full text ] [ CrossRef ] [ Medline ]
- Witek Janusek L, Tell D, Mathews HL. Mindfulness based stress reduction provides psychological benefit and restores immune function of women newly diagnosed with breast cancer: a randomized trial with active control. Brain Behav Immun. Aug 2019;80:358-373. [ CrossRef ] [ Medline ]
- Pedro J, Monteiro-Reis S, Carvalho-Maia C, Henrique R, Jerónimo C, Silva ER. Evidence of psychological and biological effects of structured Mindfulness-Based Interventions for cancer patients and survivors: a meta-review. Psychooncology. Nov 2021;30(11):1836-1848. [ FREE Full text ] [ CrossRef ] [ Medline ]
- Tauber NM, O'Toole MS, Dinkel A, Galica J, Humphris G, Lebel S, et al. Effect of psychological intervention on fear of cancer recurrence: a systematic review and meta-analysis. J Clin Oncol. Nov 01, 2019;37(31):2899-2915. [ FREE Full text ] [ CrossRef ] [ Medline ]
- Hall DL, Luberto CM, Philpotts LL, Song R, Park ER, Yeh GY. Mind-body interventions for fear of cancer recurrence: a systematic review and meta-analysis. Psychooncology. Nov 2018;27(11):2546-2558. [ FREE Full text ] [ CrossRef ] [ Medline ]
- Jain S, Shapiro SL, Swanick S, Roesch SC, Mills PJ, Bell I, et al. A randomized controlled trial of mindfulness meditation versus relaxation training: effects on distress, positive states of mind, rumination, and distraction. Ann Behav Med. Feb 2007;33(1):11-21. [ CrossRef ] [ Medline ]
- Gu J, Strauss C, Bond R, Cavanagh K. How do mindfulness-based cognitive therapy and mindfulness-based stress reduction improve mental health and wellbeing? A systematic review and meta-analysis of mediation studies. Clin Psychol Rev. Apr 2015;37:1-12. [ CrossRef ] [ Medline ]
- Butow PN, Turner J, Gilchrist J, Sharpe L, Smith AB, Fardell JE, et al. Randomized trial of ConquerFear: a novel, theoretically based psychosocial intervention for fear of cancer recurrence. J Clin Oncol. Dec 20, 2017;35(36):4066-4077. [ CrossRef ]
- Britton WB. Can mindfulness be too much of a good thing? The value of a middle way. Curr Opin Psychol. Aug 2019;28:159-165. [ FREE Full text ] [ CrossRef ] [ Medline ]
- Li J, Li C, Puts M, Wu YC, Lyu MM, Yuan B, et al. Effectiveness of mindfulness-based interventions on anxiety, depression, and fatigue in people with lung cancer: a systematic review and meta-analysis. Int J Nurs Stud. Apr 2023;140:104447. [ CrossRef ] [ Medline ]
- Domhardt M, Geßlein H, von Rezori RE, Baumeister H. Internet- and mobile-based interventions for anxiety disorders: a meta-analytic review of intervention components. Depress Anxiety. Mar 2019;36(3):213-224. [ CrossRef ] [ Medline ]
- Chen YY, Guan BS, Li ZK, Li XY. Effect of telehealth intervention on breast cancer patients' quality of life and psychological outcomes: a meta-analysis. J Telemed Telecare. Apr 2018;24(3):157-167. [ CrossRef ] [ Medline ]
- Winter N, Russell L, Ugalde A, White V, Livingston P. Engagement strategies to improve adherence and retention in web-based mindfulness programs: systematic review. J Med Internet Res. Jan 12, 2022;24(1):e30026. [ FREE Full text ] [ CrossRef ] [ Medline ]
- Goldberg SB, Lam SU, Simonsson O, Torous J, Sun S. Mobile phone-based interventions for mental health: a systematic meta-review of 14 meta-analyses of randomized controlled trials. PLOS Digit Health. 2022;1(1):e0000002. [ FREE Full text ] [ CrossRef ] [ Medline ]
- Sylvia LG, Lunn MR, Obedin-Maliver J, McBurney RN, Nowell WB, Nosheny RL, et al. Web-based mindfulness-based interventions for well-being: randomized comparative effectiveness trial. J Med Internet Res. Sep 12, 2022;24(9):e35620. [ FREE Full text ] [ CrossRef ] [ Medline ]
- Mrazek AJ, Mrazek MD, Cherolini CM, Cloughesy JN, Cynman DJ, Gougis LJ, et al. The future of mindfulness training is digital, and the future is now. Curr Opin Psychol. Aug 2019;28:81-86. [ CrossRef ] [ Medline ]
Abbreviations
fear of cancer recurrence |
Fear of Cancer Recurrence Inventory |
Hospital Anxiety and Depression Scale |
mindfulness-based cognitive therapy |
mindfulness-based intervention |
mindfulness-based stress reduction |
Preferred Reporting Items for Systematic Reviews and Meta-Analyses |
posttraumatic growth |
quality of life |
randomized controlled trial |
Reach, Effectiveness, Adoption, Implementation, and Maintenance |
standardized mean difference |
Edited by T de Azevedo Cardoso; submitted 30.03.23; peer-reviewed by J Luo, K Kershner, F Chio; comments to author 31.12.23; revised version received 26.03.24; accepted 23.04.24; published 25.06.24.
©Ting Wang, Chulei Tang, Xiaoman Jiang, Yinning Guo, Shuqin Zhu, Qin Xu. Originally published in the Journal of Medical Internet Research (https://www.jmir.org), 25.06.2024.
This is an open-access article distributed under the terms of the Creative Commons Attribution License (https://creativecommons.org/licenses/by/4.0/), which permits unrestricted use, distribution, and reproduction in any medium, provided the original work, first published in the Journal of Medical Internet Research (ISSN 1438-8871), is properly cited. The complete bibliographic information, a link to the original publication on https://www.jmir.org/, as well as this copyright and license information must be included.
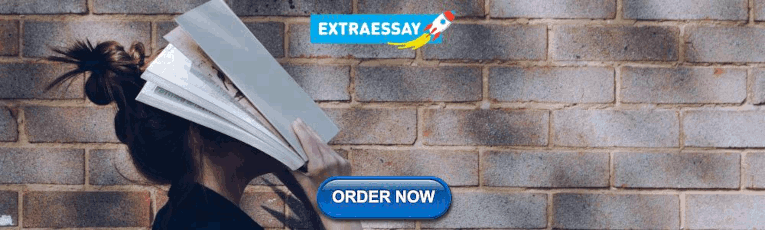
IMAGES
VIDEO
COMMENTS
A true experiment (a.k.a. a controlled experiment) always includes at least one control group that doesn't receive the experimental treatment.. However, some experiments use a within-subjects design to test treatments without a control group. In these designs, you usually compare one group's outcomes before and after a treatment (instead of comparing outcomes between different groups).
Put simply; an experimental group is a group that receives the variable, or treatment, that the researchers are testing, whereas the control group does not. These two groups should be identical in all other aspects. 2. What is the purpose of a control group in an experiment.
There are two groups in the experiment, and they are identical except that one receives a treatment (water) while the other does not. The group that receives the treatment in an experiment (here, the watered pot) is called the experimental group, while the group that does not receive the treatment (here, the dry pot) is called the control group.The control group provides a baseline that lets ...
A control group is not the same thing as a control variable. A control variable or controlled variable is any factor that is held constant during an experiment. Examples of common control variables include temperature, duration, and sample size. The control variables are the same for both the control and experimental groups.
Published on April 19, 2021 by Pritha Bhandari . Revised on June 22, 2023. In experiments, researchers manipulate independent variables to test their effects on dependent variables. In a controlled experiment, all variables other than the independent variable are controlled or held constant so they don't influence the dependent variable.
A control group in a scientific experiment is a group separated from the rest of the experiment, where the independent variable being tested cannot influence the results. This isolates the independent variable's effects on the experiment and can help rule out alternative explanations of the experimental results. Control groups can also be separated into two other types: positive or negative.
Table of Contents control group, the standard to which comparisons are made in an experiment.Many experiments are designed to include a control group and one or more experimental groups; in fact, some scholars reserve the term experiment for study designs that include a control group. Ideally, the control group and the experimental groups are identical in every way except that the experimental ...
In an experiment, the control is a standard or baseline group not exposed to the experimental treatment or manipulation.It serves as a comparison group to the experimental group, which does receive the treatment or manipulation. The control group helps to account for other variables that might influence the outcome, allowing researchers to attribute differences in results more confidently to ...
A control group in an experiment does not receive the treatment. Instead, it serves as a comparison group for the treatments. Researchers compare the results of a treatment group to the control group to determine the effect size, also known as the treatment effect.. A control group is important because it is a benchmark that allows scientists to draw conclusions about the treatment's ...
To test its effectiveness, you run an experiment with a treatment and two control groups. The treatment group gets the new pill. Control group 1 gets an identical-looking sugar pill (a placebo). Control group 2 gets a pill already approved to treat high blood pressure. Since the only variable that differs between the three groups is the type of ...
Treatment and control groups. In the design of experiments, hypotheses are applied to experimental units in a treatment group. [1] In comparative experiments, members of a control group receive a standard treatment, a placebo, or no treatment at all. [2] There may be more than one treatment group, more than one control group, or both.
Positive control groups: In this case, researchers already know that a treatment is effective but want to learn more about the impact of variations of the treatment.In this case, the control group receives the treatment that is known to work, while the experimental group receives the variation so that researchers can learn more about how it performs and compares to the control.
A true experiment (a.k.a. a controlled experiment) always includes at least one control group that doesn't receive the experimental treatment. However, some experiments use a within-subjects design to test treatments without a control group. In these designs, you usually compare one group's outcomes before and after a treatment (instead of ...
In many studies, control groups are crucial for the conclusion that can be drawn from the investigation. In the case of an experimental treatment study, a well-created control group makes the group type the independent variable of the experiment. Ideally, all conditions in the control groups (including the sample characteristics) should be ...
A control group is typically thought of as the baseline in an experiment. In an experiment, clinical trial, or other sort of controlled study, there are at least two groups whose results are compared against each other. The experimental group receives some sort of treatment, and their results are compared against those of the control group ...
Control group design is fundamental to psychological research, offering a means to measure the effect of a variable by comparing outcomes between treated and untreated groups. This design can take several forms, including post-test only and pretest-posttest configurations, each with its own advantages in minimizing experimental validity threats. The Solomon Four Group Design further enhances ...
The control group and experimental group are compared against each other in an experiment. The only difference between the two groups is that the independent variable is changed in the experimental group. ... A simple example of a controlled experiment may be used to determine whether or not plants need to be watered to live. The control group ...
Experiments play an important role in the research process and allow psychologists to investigate cause-and-effect relationships between different variables. Having one or more experimental groups allows researchers to vary different levels or types of the experimental variable and then compare the effects of these changes against a control group.
In controlled experiments, researchers use random assignment (i.e. participants are randomly assigned to be in the experimental group or the control group) in order to minimize potential confounding variables in the study. For example, imagine a study of a new drug in which all of the female participants were assigned to the experimental group and all of the male participants were assigned to ...
The control group provides a baseline in the experiment. The variable that is being studied in the experiment is not changed or is limited to zero in the control group. This insures that the effects of the variable are being studied. Most experiments try to add the variable back in increments to different treatment groups, to really begin to ...
In a true experiment, the effect of an intervention is tested by comparing two groups: one that is exposed to the intervention (the experimental group, also known as the treatment group) and another that does not receive the intervention (the control group). Importantly, participants in a true experiment need to be randomly assigned to either ...
A control group is an essential part of any experiment. It is a group of subjects who are not exposed to the independent variable being tested. The purpose of a control group is to provide a baseline against which the results from the treatment group can be compared. Without a control group, it would be impossible to determine whether the ...
In the recent study of proton therapy, the expectation of the normal tissue-sparing effect of the proton minibeam radiation therapy (pMBRT) using a multi-slit collimator (MSC) is increasing. We designed and conducted animal experiments to verify the sparing effect on normal tissues. Proton beam irradiation was carried out on two groups of mice except a control group (0 Gy). One group was ...
Diarrhea of college students (DCS) is a prevalent issue among college students, affecting their daily lives and academic performance. This study aims to explore the potential effect of Bifidobacterium breve BB05 supplements on the DCS. Initially, fifty healthy and fifty diarrheal students were recruited in the observational experiment and allocated into control and diarrhea groups, respectively.
Background There is a need for healthcare providers to develop life-story review interventions to enhance the mental well-being and quality of life of older adults. The primary aim of this study is to examine the effects of telling their life stories and creating a life-story book intervention on QoL, depressive symptoms, and life satisfaction in a group of older adults in Oman. Methods A ...
Controlled Experiment. A controlled experiment is simply an experiment in which all factors are held constant except for one: the independent variable. A common type of controlled experiment compares a control group against an experimental group. All variables are identical between the two groups except for the factor being tested.
The study results show that the experimental group learners, especially those with lower-level mathematics knowledge, performed better than the control group. Assessment and feedback, one of the forms of AI in education, is another area where the number of studies on the COVID-19 epidemic has increased (Ahmad et al., 2022 ; Hooda et al., 2022 ).
Welcome to Claude. Claude is a highly performant, trustworthy, and intelligent AI platform built by Anthropic. Claude excels at tasks involving language, reasoning, analysis, coding, and more. Introducing Claude 3.5 Sonnet, our most intelligent model yet. Read more in our blog post.
Background: Cancer has emerged as a considerable global health concern, contributing substantially to both morbidity and mortality. Recognizing the urgent need to enhance the overall well-being and quality of life (QOL) of cancer patients, a growing number of researchers have started using online mindfulness-based interventions (MBIs) in oncology.