Learn about our leading AI models
Discover the AI models behind our most impactful innovations, understand their capabilities, and find the right one when you're ready to build your own AI project.
- Gemini models
- Open models
- Industry-specific
- Ready for developers
- Text generation
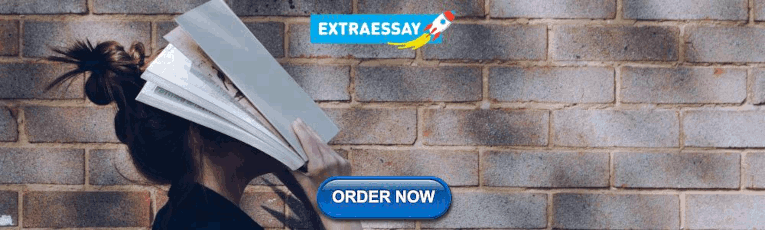
Code generation
- Image generation
Video generation
Gemini models Ready for developers Multimodal Text generation Code generation
Gemini 1.0 Ultra
Our largest model for highly complex tasks.
Performance excellence
From natural image, audio, and video understanding to mathematical reasoning, performance exceeds current state-of-the-art results on 30 of the 32 widely-used academic and multimodal benchmarks used in large language model research and development.
Advanced reasoning
The first model to outperform human experts on massive multitask language understanding, which uses 57 subjects such as math, physics, history, law, medicine, ethics, and more for testing both world knowledge and problem solving abilities.
Gemini 1.5 Pro
Our best model for general performance across a wide range of tasks.
Complex reasoning about vast amounts of information
Can seamlessly analyze, classify and summarize large amounts of content within a given prompt.
Better reasoning across modalities
Can perform highly sophisticated understanding and reasoning tasks for different modalities.
Problem-solving with longer blocks of code
When given a prompt with more than 100,000 lines of code, it can better reason across examples, suggest helpful modifications and give explanations about how different parts of the code works.
Gemini 1.0 Pro
Our best model for scaling across a wide range of tasks.
Complex reasoning systems
Fine-tuned both to be a coding model to generate proposal solution candidates, and to be a reward model that is leveraged to recognize and extract the most promising code candidates.
Advanced audio understanding
Significantly outperforms the USM and Whisper models across all ASR and AST tasks, both for English and multilingual test sets.
Gemini 1.0 Nano
Our most efficient model for on-device tasks.
Reasoning, functionality & language understanding
Excels at on-device tasks, such as summarization, reading comprehension, text completion tasks, and exhibits impressive capabilities in reasoning, STEM, coding, multimodal, and multilingual tasks relative to their sizes.
Broad accessibility
With capabilities accessible to a larger set of platforms and devices, the Gemini models expand accessibility to everyone.
Gemini 1.5 Flash
Our lightweight model, optimized for speed and efficiency.
Built for speed
Sub-second average first-token latency for the vast majority of developer and enterprise use cases.
Quality at lower cost
On most common tasks, 1.5 Flash achieves comparable quality to larger models, at a fraction of the cost.
Long-context understanding
Process hours of video and audio, and hundreds of thousands of words or lines of code.
Ready for developers Text generation Code generation
A state-of-the-art language model with improved multilingual, reasoning and coding capabilities.
Demonstrates improved capabilities in logic, common sense reasoning, and mathematics.
Multilingual translation
Improved its ability to understand, generate and translate nuanced text — including idioms, poems and riddles. PaLM 2 also passes advanced language proficiency exams at the “mastery” level.
Improved coding
Excels at popular programming languages like Python and JavaScript, but is also capable of generating specialized code in languages like Prolog, Fortran, and Verilog.
Ready for developers Image generation
A family of text-to-image models with an unprecedented degree of photorealism and a deep level of language understanding.
High quality Images
Achieves accurate, high-quality photorealistic outputs with improved image+text understanding and a variety of novel training and modeling techniques.
Text rendering support
Text-to-image models often struggle to include text accurately. Imagen 3 improves this process, ensuring the correct words or phrases appear in the generated images.
Prompt understanding
Imagen 3 understands prompts written in natural, everyday language, making it easier to get the output you want without complex prompt engineering.
Includes built-in safety precautions to help ensure that generated images align with Google’s Responsible AI principles.
Ready for developers Code generation
A family of models that generate code based on a natural language description. It can be used to create functions, web pages, unit tests, and other types of code.
Code completion
Suggests the next few lines based on the existing context of code.
Generates code based on natural language prompts from a developer.
Lets developers converse with a bot to get help with debugging, documentation, learning new concepts, and other code-related questions.
Ready for developers Text generation
A family of universal Speech Models trained on 12 million hours of speech to enable automatic speech recognition (ASR) for 100+ languages.
Broad language support
Can transcribe in over 100 languages with excellent speech recognition.
High accuracy
Achieves state-of-the-art Word Error Rate (WER) on a variety of public test sets and languages. It delivers 98% speech recognition accuracy in English and over 300% relative improvement in several languages with less than 10M speakers.
Large model size
Chirp's 2-billion-parameter model outpaces previous speech models to deliver superior performance.
Our most capable generative video model. A tool to explore new applications and creative possibilities with video generation.
Advanced Cinematic effects
With just text prompts, it creates high-quality, 1080P videos that can go beyond 60 seconds. Lets you control the camera, and prompt for things like time lapse or aerial shots of a landscape.
Detail and tone understanding
Interprets and visualizes the tone of prompts. Subtle cues in body language, lighting, and even color choices could dramatically shift the look of a generated video.
Improved consistency and quality of video
Able to retain visual consistency in appearance, locations and style across multiple scenes in a longer video.
More control
Veo allows users to edit videos through prompts, including modifying, adding or replacing visual elements and it can generate a video from an image input, using the image to fit within any frame of the output and the prompt as guidance for how the video should proceed.
Industry-specific Ready for developers Text generation
A family of models fine-tuned for the healthcare industry.
Transform your healthcare workflow
Revolutionizes the way medical information is accessed, analyzed, and applied. Reduces administrative burdens and helps synthesize information seamlessly.
Build customized solutions
MedLM is a customizable solution that can embed into your workflow and integrate with your data to augment your healthcare capabilities.
Innovate safely and responsibly
Born from a belief that together, technology and medical experts can innovate safely, MedLM helps you stay on the cutting edge.
Industry-specific Text generation
A family of models fine-tuned for learning, infused with teacher-advised education capabilities and pedagogical evaluations.
Inspire active learning
Allow for practice and healthy struggle with timely feedback.
Manage cognitive load
Present relevant, well-structured information in multiple modalities.
Adapt to learner
Dynamically adjust to goals and needs, grounding in relevant materials.
Stimulate curiosity
Inspire engagement to provide motivation through the learning journey.
Deepen metacognition
Plan, monitor and help the learner reflect on progress.
A family of models fine-tuned for cybersecurity.
Industry-leading threat data
Tuned, trained and grounded in threat intelligence from Google, VirusTotal, and Mandiant to bring up-to-date security information and context to users.
Infused in Google Cloud Security products
Gemini in Security agents use SecLM to help defenders protect their organizations.
Supercharging security use cases
Cybersecurity professionals can easily make sense of complex information and perform specialized tasks and workflows.
Open models Ready for developers Text generation
A family of lightweight, state-of-the-art open models from Google, built from the same research and technology used to create the Gemini models.
Responsible by design
Incorporating comprehensive safety measures, these models help ensure responsible and trustworthy AI solutions through curated datasets and rigorous tuning.
Unmatched performance at size
Gemma models achieve exceptional benchmark results at its 2B and 7B sizes, even outperforming some larger open models.
Framework flexible
With Keras 3.0, enjoy seamless compatibility with JAX, TensorFlow, and PyTorch, empowering you to effortlessly choose and switch frameworks depending on your task.
Open models Ready for developers Code generation
A collection of lightweight open code models built on top of Gemma. CodeGemma models perform a variety of tasks like code completion, code generation, code chat, and instruction following.
Intelligent code completion and generation
Complete lines, functions, and even generate entire blocks of code, whether you're working locally or using Google Cloud resources.
Enhanced accuracy
Trained on 500 billion tokens data from web documents, mathematics, and code. Generates code that's not only more syntactically correct but also semantically meaningful, reducing errors and debugging time.
Multi-language proficiency
Supports Python, JavaScript, Java, Kotlin, C++, C#, Rust, Go, and other languages.
RecurrentGemma
A technically distinct model that leverages recurrent neural networks and local attention to improve memory efficiency.
Reduced memory usage
Lower memory requirements allow for the generation of longer samples on devices with limited memory, such as single GPUs or CPUs.
Higher throughput
Can perform inference at significantly higher batch sizes, thus generating substantially more tokens per second (especially when generating long sequences).
Research innovation
Showcases a non-transformer model that achieves high performance, highlighting advancements in deep learning research.
Open models Ready for developers Multimodal Text generation
Our first multimodal Gemma model, designed for class-leading fine-tune performance across diverse vision-language tasks.
Powerful fine tuning
Designed for class-leading fine-tune performance on a wide range of vision-language tasks like:
- image and short video captioning
- visual question answering
- understanding text in images
- object detection
- and object segmentation
Extensive language support
Supports a wide range of languages.
Ready to build?
See how ai can help you…, be more creative.
Create high-quality, photorealistic images with ImageFX.
Mix and generate custom beats with MusicFX.
Generate engaging videos in minutes with VideoFX.
Supercharge your creativity and productivity with Gemini.
Bring your best ideas to life with Gemini for Google Workspace.
Do your best thinking with a research and writing tool, grounded in the information you give it.
*SynthID helps identify AI-generated content by embedding an imperceptible watermark on text, images, audio, and video content generated by our models.
Responsibility is the bedrock of all of our models.
AI is helping us deliver on our mission in exciting new ways, yet it's still an emerging technology that surfaces new challenges and questions as it evolves.
To us, building AI responsibly means both addressing these challenges and questions while maximizing the benefits for people and society. In navigating this complexity, we’re guided by our AI Principles and cutting-edge research, along with feedback from experts, users, and partners.
These efforts are helping us continually improve our models with new advances like AI-assisted redteaming and prevent their misuse with technologies like SynthID. They are also unlocking exciting, real-world progress towards some of society’s most pressing challenges like predicting floods and accelerating research on neglected diseases.
Introduced in 2016, WaveNet was one of the first AI models to generate natural-sounding speech. Since then, it has inspired research, products, and applications in Google — and beyond.
- Copy link ×
The challenge
Learning from human speech, rapid advances, the power of voice, widespread legacy.
For decades, computer scientists tried reproducing nuances of the human voice to make computer-generated voices more natural.
Most text-to-speech systems relied on “concatenative synthesis” — a pain-staking process of cutting voice recordings into phonetic sounds and recombining them to form new words and sentences - or DSP (digital signal processing) algorithms known as "vocoders".
The resulting voices often sounded mechanical and contained artifacts such as glitches, buzzes and whistles. Making changes required entirely new recordings — an expensive and time-consuming process.
WaveNet took a different approach to audio generation by using a neural network to model predict individual audio samples. This approach allowed WaveNet to produce high-fidelity, synthetic audio, allowing people to interact more naturally with their digital products
“ WaveNet rapidly went from a research prototype to an advanced product used by millions around the world.
Koray Kavukcuoglu Vice President of Research
WaveNet is a generative model trained on human speech samples. It creates waveforms of speech patterns by predicting which sounds are most likely to follow each other, each built one sample at a time, with up to 24,000 samples per second of sound.
The model incorporates natural-sounding elements, such as lip-smacking and breathing patterns. And includes vital layers of communication like intonation, accents, emotion — delivering a richness and depth to computer-generated voices.
For example, when we first introduced WaveNet, we created American English and Mandarin Chinese voices that narrowed the gap between human and computer-generated voices by 50%.
“ WaveNet is a general purpose technology that has allowed us to unlock a range of new applications, from improving video calls on even the weakest connections to helping people regain their original voice after losing the ability to speak.
Zachary Gleicher Product Manager
Early versions of WaveNet were time consuming to interact with, taking hours to generate just one second of audio.
Using a technique called distillation — transferring knowledge from a larger to smaller model — we reengineered WaveNet to run 1,000 times faster than our research prototype, creating one second of speech in just 50 milliseconds.
In parallel, we also developed WaveRNN — a simpler, faster, and more computationally efficient model that could run on devices, like mobile phones, rather than in a data center.
Both WaveNet and WaveRNN became crucial components of many of Google’s best known services such as the Google Assistant, Maps Navigation, Voice Search and Cloud Text-To-Speech.
They also helped inspire entirely new product experiences. For example, an extension known as WaveNetEQ helped improve the quality of calls for Duo, Google’s video-calling app.
But perhaps one of its most profound impacts was helping people living with progressive neurological diseases like ALS (amyotrophic lateral sclerosis) regain their voice.
In 2014, former NFL linebacker Tim Shaw’s voice deteriorated due to his ALS. To help, Google’s Project Euphonia (developed a service to better understand Shaw’s impaired speech.
WaveRNN was combined with other speech technologies and a dataset of archive media interviews to create a natural-sounding version of Shaw’s voice, helping him speak again.
WaveNet demonstrated an entirely new approach to voice synthesis that helped people regain their voices, translate content across multiple languages, create custom audio content, and much more.
Its emergence also unlocked new research approaches and technologies for generating natural sounding voices.
Today, thanks to WaveNet, there is a new generation of voice synthesis products that continue its legacy and help billions of people around the world overcome barriers in communication, culture, and commerce.
- Help Center
- Google Assistant
- Privacy Policy
- Terms of Service
- Submit feedback
Learn how Google improves speech models
Many Google products involve speech recognition. For example, Google Assistant allows you to ask for help by voice, Gboard lets you dictate messages to your friends, and Google Meet provides auto captioning for your meetings.
Speech technologies increasingly rely on deep neural networks, a type of machine learning that helps us build more accurate and faster speech recognition models. Generally deep neural networks need larger amounts of data to work well and improve over time. This process of improvement is called model training.
What technologies we use to train speech models
Google’s speech team uses 3 broad classes of technologies to train speech models: conventional learning, federated learning, and ephemeral learning. Depending on the task and situation, some of these are more effective than others, and in some cases, we use a combination of them. This allows us to achieve the best quality possible, while providing privacy by design.
Conventional learning is how most of our speech models are trained.
How conventional learning works to train speech models
- With your explicit consent, audio samples are collected and stored on Google’s servers.
- A portion of these audio samples are annotated by human reviewers.
- In supervised training: Models are trained to mimic annotations from human reviewers for the same audio.
- In unsupervised training: Machine annotations are used instead of human annotations.
When training on equal amounts of data, supervised training typically results in better speech recognition models than unsupervised training because the annotations are higher quality. On the other hand, unsupervised training can learn from more audio samples since it learns from machine annotations, which are easier to produce.
How your data stays private
Learn more about how Google keeps your data private .
Federated learning is a privacy preserving technique developed at Google to train AI models directly on your phone or other device. We use federated learning to train a speech model when the model runs on your device and data is available for the model to learn from.
How federated learning works to train speech models
With federated learning, we train speech models without sending your audio data to Google’s servers.
- To enable federated learning, we save your audio data on your device.
- A training algorithm learns from this data on your device.
- A new speech model is formed by combining the aggregated learnings from your device along with learnings from all other participating devices.
How ephemeral learning works to train speech models
- As our systems convert incoming audio samples into text, those samples are sent to short-term memory (RAM).
- While the data is in RAM, a training algorithm learns from those audio data samples in real time.
- These audio data samples are deleted from short-term memory within minutes.
With ephemeral learning, your audio data samples are:
- Only held in short-term memory (RAM) and for no more than a few minutes.
- Never accessible by a human.
- Never stored on a server.
- Used to train models without any additional data that can identify you.
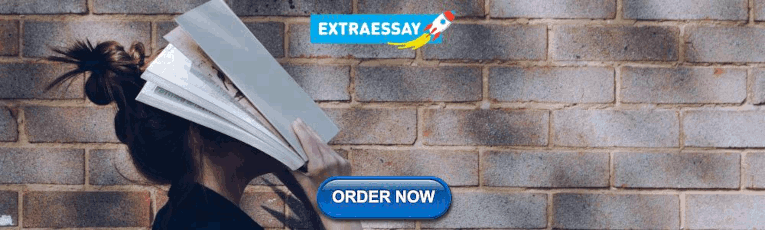
How Google will use & invest in these technologies
We’ll continue to use all 3 technologies, often in combination for higher quality. We’re also actively working to improve both federated and ephemeral learning for speech technologies. Our goal is to make them more effective and useful, and in ways that preserve privacy by default.
Need more help?
Try these next steps:.
It Speaks! Create Synthetic Speech Using Text-to-Speech
Checkpoints.
Enable the Text-to-Speech API
Create a service account
- Setup and requirements
- Task 1. Enable the Text-to-Speech API
- Task 2. Create a virtual environment
- Task 3. Create a service account
- Task 4. Get a list of available voices
- Task 5. Create synthetic speech from text
- Task 6. Create synthetic speech from SSML
- Task 7. Configure audio output and device profiles
- Congratulations!
The Text-to-Speech API lets you create audio files of machine-generated, or synthetic , human speech. You provide the content as text or Speech Synthesis Markup Language (SSML) , specify a voice (a unique 'speaker' of a language with a distinctive tone and accent), and configure the output; the Text-to-Speech API returns to you the content that you sent as spoken word, audio data, delivered by the voice that you specified.
In this lab you will create a series of audio files using the Text-to-Speech API, then listen to them to compare the differences.
What you'll learn
In this lab you use the Text-to-Speech API to do the following:
- Create a series of audio files
- Listen and compare audio files
- Configure audio output
Before you click the Start Lab button
Read these instructions. Labs are timed and you cannot pause them. The timer, which starts when you click Start Lab , shows how long Google Cloud resources will be made available to you.
This hands-on lab lets you do the lab activities yourself in a real cloud environment, not in a simulation or demo environment. It does so by giving you new, temporary credentials that you use to sign in and access Google Cloud for the duration of the lab.
To complete this lab, you need:
- Access to a standard internet browser (Chrome browser recommended).
- Time to complete the lab---remember, once you start, you cannot pause a lab.
How to start your lab and sign in to the Google Cloud console
Click the Start Lab button. If you need to pay for the lab, a pop-up opens for you to select your payment method. On the left is the Lab Details panel with the following:
- The Open Google Cloud console button
- Time remaining
- The temporary credentials that you must use for this lab
- Other information, if needed, to step through this lab
Click Open Google Cloud console (or right-click and select Open Link in Incognito Window if you are running the Chrome browser).
The lab spins up resources, and then opens another tab that shows the Sign in page.
Tip: Arrange the tabs in separate windows, side-by-side.
If necessary, copy the Username below and paste it into the Sign in dialog.
You can also find the Username in the Lab Details panel.
Click Next .
Copy the Password below and paste it into the Welcome dialog.
You can also find the Password in the Lab Details panel.
Click through the subsequent pages:
- Accept the terms and conditions.
- Do not add recovery options or two-factor authentication (because this is a temporary account).
- Do not sign up for free trials.
After a few moments, the Google Cloud console opens in this tab.
Activate Cloud Shell
Cloud Shell is a virtual machine that is loaded with development tools. It offers a persistent 5GB home directory and runs on the Google Cloud. Cloud Shell provides command-line access to your Google Cloud resources.
When you are connected, you are already authenticated, and the project is set to your Project_ID , . The output contains a line that declares the Project_ID for this session:
gcloud is the command-line tool for Google Cloud. It comes pre-installed on Cloud Shell and supports tab-completion.
- (Optional) You can list the active account name with this command:
- Click Authorize .
- (Optional) You can list the project ID with this command:
Set the region for your project
In Cloud Shell, enter the following command to set the region to run your project in this lab:
On the top of the Dashboard, click +Enable APIs and Services .
Enter "text-to-speech" in the search box.
Click Cloud Text-to-Speech API .
Click Enable to enable the Cloud Text-to-Speech API.
Wait for a few seconds for the API to be enabled for the project. Once enabled, the Cloud Text-to-Speech API page shows details, metrics and more.
Click Check my progress to verify the objective. Enable the Text-to-Speech API
Python virtual environments are used to isolate package installation from the system.
- Install the virtualenv environment:
- Build the virtual environment:
- Activate the virtual environment.
You should use a service account to authenticate your calls to the Text-to-Speech API.
- To create a service account, run the following command in Cloud Shell:
- Now generate a key to use that service account:
- Finally, set the GOOGLE_APPLICATION_CREDENTIALS environment variable to the location of your key file:
Click Check my progress to verify the objective. Create a service account
As mentioned previously, the Text-to-Speech API provides many different voices and languages that you can use to create audio files. You can use any of the available voices as the speaker for your content.
- The following curl command gets the list of all the voices you can select from when creating synthetic speech using the Text-to-Speech API:
The Text-to-Speech API returns a JSON-formatted result that looks similar to the following:
Looking at the results from the curl command, notice that each voice has four fields:
- name : The ID of the voice that you provide when you request that voice.
- ssmlGender : The gender of the voice to speak the text, as defined in the SSML W3 Recommendation .
- naturalSampleRateHertz : The sampling rate of the voice.
- languageCodes : The list of language codes associated with that voice.
Also notice that some languages have several voices to choose from.
- To scope the results returned from the API to just a single language code, run:
Now that you've seen how to get the names of voices to speak your text, it's time to create some synthetic speech!
For this, you build your request to the Text-to-Speech API in a text file titled synthesize-text.json .
- Create this file in Cloud Shell by running the following command:
- Using a line editor (for example nano , vim , or emacs ) or the Cloud Shell code editor, add the following code to synthesize-text.json :
- Save the file and exit the line editor.
The JSON-formatted request body provides three objects:
- The input object provides the text to translate into synthetic speech.
- The voice object specifies the voice to use for the synthetic speech.
- The audioConfig object tells the Text-to-Speech API what kind of audio encoding to send back.
- Use the following code to call the Text-to-Speech API using the curl command:
The output of this call is saved to a file called synthesize-text.txt .
- Open the synthesize-text.txt file. Notice that the Text-to-Speech API provides the audio output in base64-encoded text assigned to the audioContent field, similar to what's shown below:
To translate the response into audio, you need to select the audio data it contains and decode it into an audio file - for this lab, MP3. Although there are many ways that you can do this, in this lab you'll use some simple Python code. Don't worry if you're not a Python expert; you need only create the file and invoke it from the command line.
- Create a file named tts_decode.py :
- Using a line editor (for example nano , vim , or emacs ) or the Cloud Shell code editor, add the following code into tts_decode.py :
Save tts_decode.py and exit the line editor.
Now, to create an audio file from the response you received from the Text-to-Speech API, run the following command from Cloud Shell:
This creates a new MP3 file named synthesize-text-audio.mp3 .
Of course, since the synthesize-text-audio.mp3 lives in the cloud, you can't just play it directly from Cloud Shell! To listen to the file, you create a Web server hosting a simple web page that embeds the file as playable audio (from an HTML < audio> control).
- Create a new file called index.html :
- Using a line editor (for example nano , vim , or emacs ) or the Cloud Shell code editor, add the following code into index.html :
Back in Cloud Shell, start a simple Python HTTP server from the command prompt:
Then select Preview on port 8080 from the displayed menu.
In the new browser window, you should see something like the following:
Play the audio embedded on the page. You'll hear the synthetic voice speak the text that you provided to it!
When you're done listening to the audio files, you can shut down the HTTP server by pressing CTRL + C in Cloud Shell.
In addition to using text, you can also provide input to the Text-to-Speech API in the form of Speech Synthesis Markup Language (SSML) . SSML defines an XML format for representing synthetic speech. Using SSML input, you can more precisely control pauses, emphasis, pronunciation, pitch, speed, and other qualities in the synthetic speech output.
- First, build your request to the Text-to-Speech API in a text file titled synthesize-ssml.json . Create this file in Cloud Shell by running the following command:
- Using a line editor (for example nano , vim , or emacs ) or the Cloud Shell code editor, paste the following JSON into synthesize-ssml.json :
Notice that the input object of the JSON payload to send includes some different stuff this time around. Rather than a text field, the input object has a ssml field instead. The ssml field contains XML-formatted content with the <speak> element as its root. Each of the elements present in this XML representation of the input affects the output of the synthetic speech.
Specifically, the elements in this sample have the following effects:
- <s> contains a sentence.
- <emphasis> adds stress on the enclosed word or phrase.
- <break> inserts a pause in the speech.
- <prosody> customizes the pitch, speaking rate, or volume of the enclosed text, as specified by the rate , pitch , or volume attributes.
- <say-as> provides more guidance about how to interpret and then say the enclosed text, for example, whether to speak a sequence of numbers as ordinal or cardinal.
- <sub> specifies a substitution value to speak for the enclosed text.
- In Cloud Shell use the following code to call the Text-to-Speech API, which saves the output to a file called synthesize-ssml.txt :
Again, you need to decode the output from the Text-to-Speech API before you can hear the audio.
- Run the following command to generate an audio file named synthesize-ssml-audio.mp3 using the tts_decode.py utility that you created previously:
- Next, open the index.html file that you created earlier. Replace the contents of the file with the following HTML:
- Then, start a simple Python HTTP server from the Cloud Shell command prompt:
- Play the two embedded audio files. Notice the differences in the SSML output: although both audio files say the same words, the SSML output speaks them a bit differently, adding pauses and different pronunciations for abbreviations.
Going beyond SSML, you can provide even more customization to your synthetic speech output created by the Text-to-Speech API. You can specify other audio encodings, change the pitch of the audio output, and even request that the output be optimized for a specific type of hardware.
Build your request to the Text-to-Speech API in a text file titled synthesize-with-settings.json :
- Using a line editor (for example nano , vim , or emacs ) or the Cloud Shell code editor, paste the following JSON into synthesize-with-settings.json :
Looking at this JSON payload, you notice that the audioConfig object contains some additional fields now:
- The speakingRate field specifies a speed at which the speaker says the voice. A value of 1.0 is the normal speed for the voice, 0.5 is half that fast, and 2.0 is twice as fast.
- The pitch field specifies a difference in tone to speak the words. The value here specifies a number of semitones lower (negative) or higher (positive) to speak the words.
- The audioEncoding field specifies the audio encoding to use for the data. The accepted values for this field are LINEAR16 , MP3 , and OGG_OPUS .
- The effectsProfileId field requests that the Text-to-Speech API optimizes the audio output for a specific playback device. The API applies an predefined audio profile to the output that enhances the audio quality on the specified class of devices.
The output of this call is saved to a file called synthesize-with-settings.txt .
- Run the following command to generate an audio file named synthesize-with-settings-audio.mp3 from the output received from the Text-to-Speech API:
- Next open the index.html file that you created earlier and replace the contents of the file with the following HTML:
- Now, restart the Python HTTP server from the Cloud Shell command prompt:
- Play the third embedded audio file. Notice that the voice on the audio speaks a bit faster and lower than the previous examples.
You have learned how to create synthetic speech using the Cloud Text-to-Speech API. You learned about:
- Listing all of the synthetic voices available through the Text-to-Speech API
- Creating a Text-to-Speech API request and calling the API with curl, providing both text and SSML
- Configuring the setting for audio output, including specifying a device profile for audio playback
Finish your quest
This self-paced lab is part of the Language, Speech, Text & Translation with Google CLoud APIs quest. A quest is a series of related labs that form a learning path. Completing this quest earns you a badge to recognize your achievement. You can make your badge or badges public and link to them in your online resume or social media account. Enroll in this quest and get immediate completion credit. Refer to the Google Cloud Skills Boost catalog for all available quests.
Take your next lab
Continue your quest with Translate Text with the Cloud Translation API or try one of these:
- Measuring and Improving Speech Accuracy
- Entity and Sentiment Analysis with the Natural Language API
Next steps / Learn more
- Check out the detailed documentation for the Text-to-Speech API on cloud.google.com.
- Learn how to create synthetic speech using the client libraries for the Text-to-Speech API .
Google Cloud training and certification
...helps you make the most of Google Cloud technologies. Our classes include technical skills and best practices to help you get up to speed quickly and continue your learning journey. We offer fundamental to advanced level training, with on-demand, live, and virtual options to suit your busy schedule. Certifications help you validate and prove your skill and expertise in Google Cloud technologies.
Manual Last Updated August 25, 2023
Lab Last Tested August 25, 2023
Copyright 2024 Google LLC All rights reserved. Google and the Google logo are trademarks of Google LLC. All other company and product names may be trademarks of the respective companies with which they are associated.
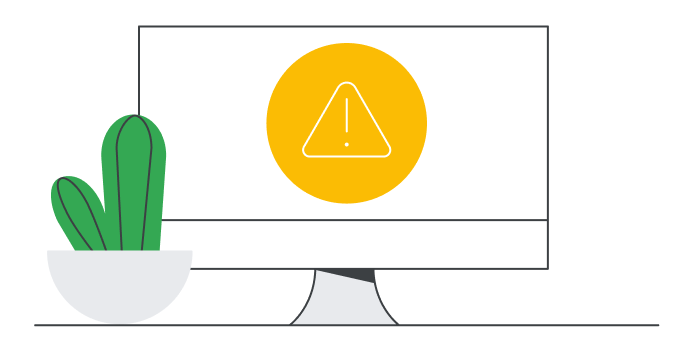
This content is not currently available
We will notify you via email, when it becomes available
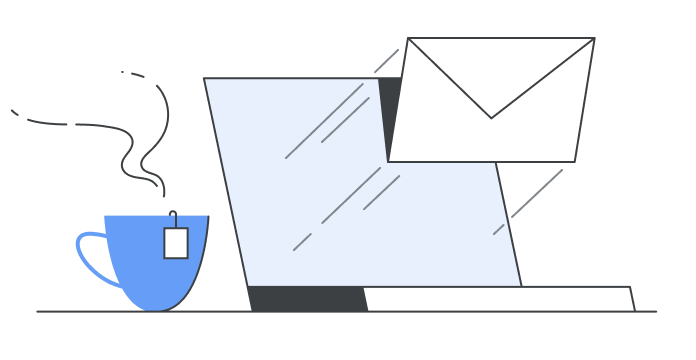
We will contact you via email, if it becomes available
In this lab, you create a series of audio files using the Text-to-Speech API, then listen to them to compare the differences.
Duration: 0m setup · 60m access · 60m completion
AWS Region: []
Levels: introductory
Permalink: https://www.cloudskillsboost.google/catalog_lab/1052
Something went wrong
Please try again later.
- Español – América Latina
- Português – Brasil
- Tiếng Việt
Using the Speech-to-Text API with Python
1. overview.
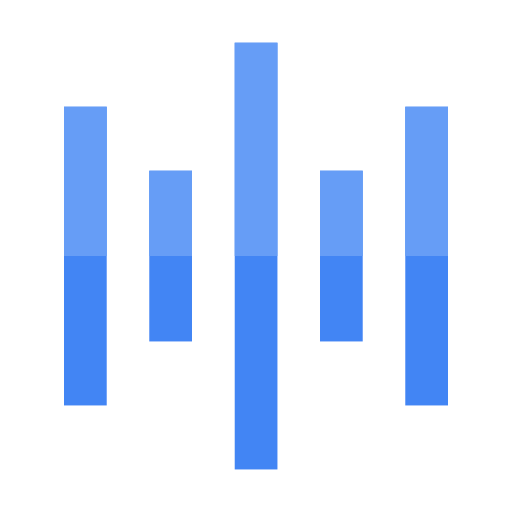
The Speech-to-Text API enables developers to convert audio to text in over 125 languages and variants, by applying powerful neural network models in an easy to use API.
In this tutorial, you will focus on using the Speech-to-Text API with Python.
What you'll learn
- How to set up your environment
- How to transcribe audio files in English
- How to transcribe audio files with word timestamps
- How to transcribe audio files in different languages
What you'll need
- A Google Cloud project
- A browser, such as Chrome or Firefox
- Familiarity using Python
How will you use this tutorial?
How would you rate your experience with python, how would you rate your experience with google cloud services, 2. setup and requirements, self-paced environment setup.
- Sign-in to the Google Cloud Console and create a new project or reuse an existing one. If you don't already have a Gmail or Google Workspace account, you must create one .

- The Project name is the display name for this project's participants. It is a character string not used by Google APIs. You can always update it.
- The Project ID is unique across all Google Cloud projects and is immutable (cannot be changed after it has been set). The Cloud Console auto-generates a unique string; usually you don't care what it is. In most codelabs, you'll need to reference your Project ID (typically identified as PROJECT_ID ). If you don't like the generated ID, you might generate another random one. Alternatively, you can try your own, and see if it's available. It can't be changed after this step and remains for the duration of the project.
- For your information, there is a third value, a Project Number , which some APIs use. Learn more about all three of these values in the documentation .
- Next, you'll need to enable billing in the Cloud Console to use Cloud resources/APIs. Running through this codelab won't cost much, if anything at all. To shut down resources to avoid incurring billing beyond this tutorial, you can delete the resources you created or delete the project. New Google Cloud users are eligible for the $300 USD Free Trial program.
Start Cloud Shell
While Google Cloud can be operated remotely from your laptop, in this codelab you will be using Cloud Shell , a command line environment running in the Cloud.
Activate Cloud Shell

If this is your first time starting Cloud Shell, you're presented with an intermediate screen describing what it is. If you were presented with an intermediate screen, click Continue .
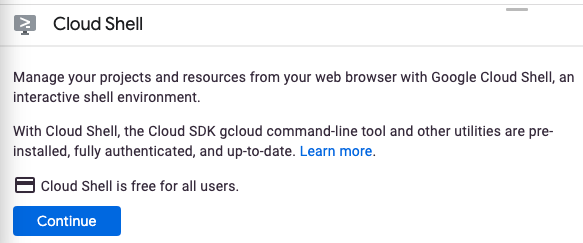
It should only take a few moments to provision and connect to Cloud Shell.

This virtual machine is loaded with all the development tools needed. It offers a persistent 5 GB home directory and runs in Google Cloud, greatly enhancing network performance and authentication. Much, if not all, of your work in this codelab can be done with a browser.
Once connected to Cloud Shell, you should see that you are authenticated and that the project is set to your project ID.
- Run the following command in Cloud Shell to confirm that you are authenticated:
Command output
- Run the following command in Cloud Shell to confirm that the gcloud command knows about your project:
If it is not, you can set it with this command:
3. Environment setup
Before you can begin using the Speech-to-Text API, run the following command in Cloud Shell to enable the API:
You should see something like this:
Now, you can use the Speech-to-Text API!
Navigate to your home directory:
Create a Python virtual environment to isolate the dependencies:
Activate the virtual environment:
Install IPython and the Speech-to-Text API client library:
Now, you're ready to use the Speech-to-Text API client library!
In the next steps, you'll use an interactive Python interpreter called IPython , which you installed in the previous step. Start a session by running ipython in Cloud Shell:
You're ready to make your first request...
4. Transcribe audio files
In this section, you will transcribe an English audio file.
Copy the following code into your IPython session:
Take a moment to study the code and see how it uses the recognize client library method to transcribe an audio file*.* The config parameter indicates how to process the request and the audio parameter specifies the audio data to be recognized.
Send a request:
You should see the following output:
Update the configuration to enable automatic punctuation and send a new request:
In this step, you were able to transcribe an audio file in English, using different parameters, and print out the result. You can read more about transcribing audio files .
5. Get word timestamps
Speech-to-Text can detect time offsets (timestamps) for the transcribed audio. Time offsets show the beginning and end of each spoken word in the supplied audio. A time offset value represents the amount of time that has elapsed from the beginning of the audio, in increments of 100ms.
To transcribe an audio file with word timestamps, update your code by copying the following into your IPython session:
Take a moment to study the code and see how it transcribes an audio file with word timestamps*.* The enable_word_time_offsets parameter tells the API to return the time offsets for each word (see the doc for more details).
In this step, you were able to transcribe an audio file in English with word timestamps and print the result. Read more about getting word timestamps .
6. Transcribe different languages
The Speech-to-Text API recognizes more than 125 languages and variants! You can find a list of supported languages here .
In this section, you will transcribe a French audio file.
To transcribe the French audio file, update your code by copying the following into your IPython session:
In this step, you were able to transcribe a French audio file and print the result. You can read more about the supported languages .
7. Congratulations!
You learned how to use the Speech-to-Text API using Python to perform different kinds of transcription on audio files!
To clean up your development environment, from Cloud Shell:
- If you're still in your IPython session, go back to the shell: exit
- Stop using the Python virtual environment: deactivate
- Delete your virtual environment folder: cd ~ ; rm -rf ./venv-speech
To delete your Google Cloud project, from Cloud Shell:
- Retrieve your current project ID: PROJECT_ID=$(gcloud config get-value core/project)
- Make sure this is the project you want to delete: echo $PROJECT_ID
- Delete the project: gcloud projects delete $PROJECT_ID
- Test the demo in your browser: https://cloud.google.com/speech-to-text
- Speech-to-Text documentation: https://cloud.google.com/speech-to-text/docs
- Python on Google Cloud: https://cloud.google.com/python
- Cloud Client Libraries for Python: https://github.com/googleapis/google-cloud-python
This work is licensed under a Creative Commons Attribution 2.0 Generic License.
Except as otherwise noted, the content of this page is licensed under the Creative Commons Attribution 4.0 License , and code samples are licensed under the Apache 2.0 License . For details, see the Google Developers Site Policies . Java is a registered trademark of Oracle and/or its affiliates.
- Arts, Design & Media
- Career Exploration
- Government, Policy & Social Impact
- Graduate Business (MBA & SMP)
- Health Care & Sciences
- Technology, Data & Engineering
- Career Assessments
- Career & Major Exploration
- Resumes, Cover Letters & Application Materials
- Graduate & Professional School Preparation
- Job & Internship Search Strategies
- Negotiation & Offer Evaluation
- Professional Conduct
- Bear Treks – Explore Industries
- Chancellor’s Career Fellows Program
- Federal Work-Study
- Pershing Fellowship in Non-Profit Leadership
- Stipends for Unpaid Summer Internships
- Schedule a Career Coaching Appointment
- Faculty & Staff
- Attend an Event
- Engage with Students
- Post a Position
- Hiring International Talent
- International Employer Resources in Asia
- Recruiting Calendar
- Recruitment and Offer Policy
- Visiting the Center for Career Engagement
- Hire WashU: Annual Employer Forum
- Career Outcomes
- Center for Career Engagement Leadership
- Academic Partnerships Team
- Career Development Team
- Employer Engagement Team
- Events, Operations & Programs Team
- Career Peer Student Team

Building a Video Transcriber with Node.js and Google AI Speech-To-Text API
- Share This: Share Building a Video Transcriber with Node.js and Google AI Speech-To-Text API on Facebook Share Building a Video Transcriber with Node.js and Google AI Speech-To-Text API on LinkedIn Share Building a Video Transcriber with Node.js and Google AI Speech-To-Text API on X
Instructor: Fikayo Adepoju
Formerly complicated tasks like audio transcription for videos have become much simpler thanks to the rise of APIs like Google’s Speech-to-Text. But while this exciting new tool can handle transcription, if you want to transcribe a lot of audio, your code still needs to set up connections and authentication and pipe the information back and forth. In this course, instructor Fikayo Adepoju shows you how to integrate Node.js applications with Google AI Speech-to-Text. Learn how to set up Google AI Speech-to-Text, build the video transcriber interface, develop the back end and connect to the AI, and then bring it all together.
This course is designed for intermediate and advanced developers interested in the future of development with generative AI and the integration of applications with AI models.
Speech Synthesis, Recognition, and More With SpeechT5
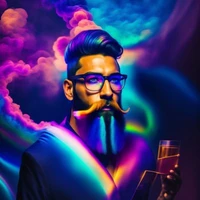
SpeechT5 was originally described in the paper SpeechT5: Unified-Modal Encoder-Decoder Pre-Training for Spoken Language Processing by Microsoft Research Asia. The official checkpoints published by the paper’s authors are available on the Hugging Face Hub.
If you want to jump right in, here are some demos on Spaces:
- Speech Synthesis (TTS)
- Voice Conversion
- Automatic Speech Recognition
Introduction
SpeechT5 is not one, not two, but three kinds of speech models in one architecture.
- speech-to-text for automatic speech recognition or speaker identification,
- text-to-speech to synthesize audio, and
- speech-to-speech for converting between different voices or performing speech enhancement.
The main idea behind SpeechT5 is to pre-train a single model on a mixture of text-to-speech, speech-to-text, text-to-text, and speech-to-speech data. This way, the model learns from text and speech at the same time. The result of this pre-training approach is a model that has a unified space of hidden representations shared by both text and speech.
At the heart of SpeechT5 is a regular Transformer encoder-decoder model. Just like any other Transformer, the encoder-decoder network models a sequence-to-sequence transformation using hidden representations. This Transformer backbone is the same for all SpeechT5 tasks.
To make it possible for the same Transformer to deal with both text and speech data, so-called pre-nets and post-nets were added. It is the job of the pre-net to convert the input text or speech into the hidden representations used by the Transformer. The post-net takes the outputs from the Transformer and turns them into text or speech again.
A figure illustrating SpeechT5’s architecture is depicted below (taken from the original paper ).
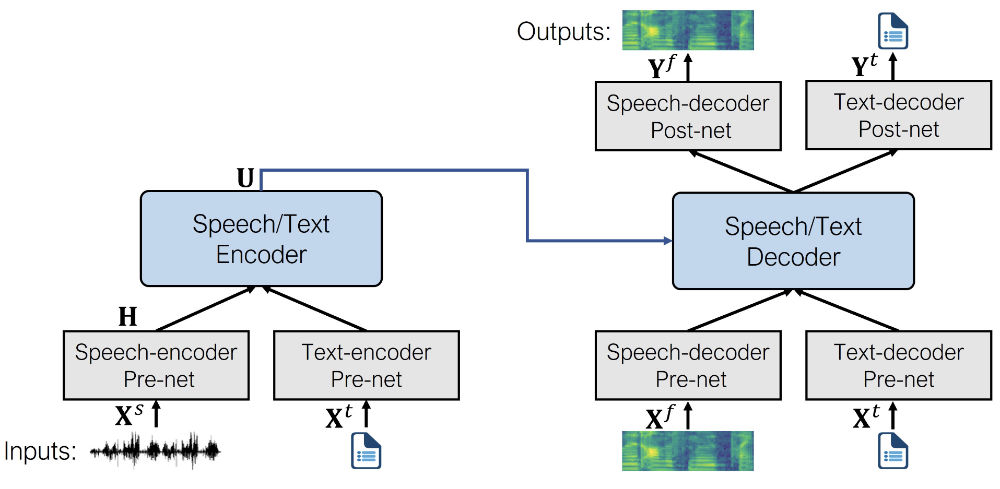
During pre-training, all of the pre-nets and post-nets are used simultaneously. After pre-training, the entire encoder-decoder backbone is fine-tuned on a single task. Such a fine-tuned model only uses the pre-nets and post-nets specific to the given task. For example, to use SpeechT5 for text-to-speech, you’d swap in the text encoder pre-net for the text inputs and the speech decoder pre and post-nets for the speech outputs.
Note: Even though the fine-tuned models start out using the same set of weights from the shared pre-trained model, the final versions are all quite different in the end. You can’t take a fine-tuned ASR model and swap out the pre-nets and post-net to get a working TTS model, for example. SpeechT5 is flexible, but not that flexible.
Text-to-speech
SpeechT5 is the first text-to-speech model we’ve added to 🤗 Transformers, and we plan to add more TTS models in the near future.
For the TTS task, the model uses the following pre-nets and post-nets:
Text encoder pre-net. A text embedding layer that maps text tokens to the hidden representations that the encoder expects. Similar to what happens in an NLP model such as BERT.
Speech decoder pre-net. This takes a log mel spectrogram as input and uses a sequence of linear layers to compress the spectrogram into hidden representations. This design is taken from the Tacotron 2 TTS model.
Speech decoder post-net. This predicts a residual to add to the output spectrogram and is used to refine the results, also from Tacotron 2.
The architecture of the fine-tuned model looks like the following.
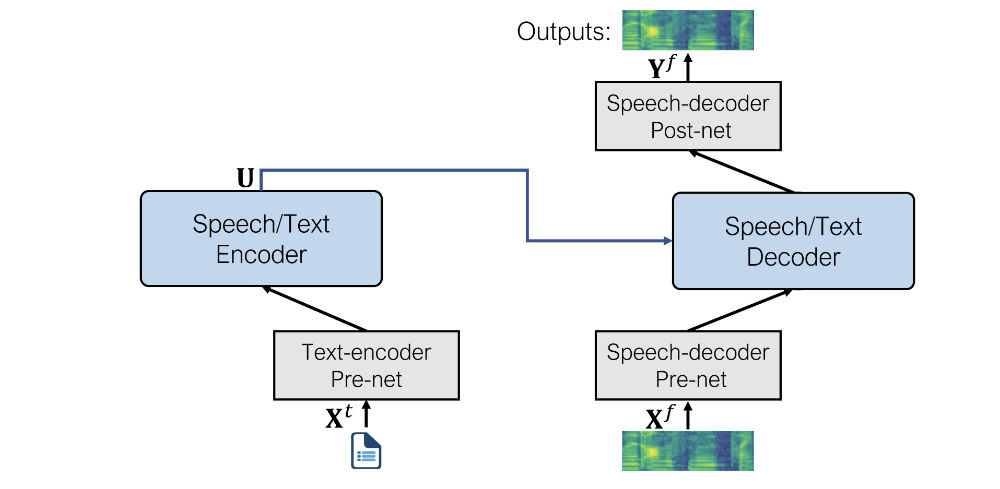
Here is a complete example of how to use the SpeechT5 text-to-speech model to synthesize speech. You can also follow along in this interactive Colab notebook .
SpeechT5 is not available in the latest release of Transformers yet, so you'll have to install it from GitHub. Also install the additional dependency sentencepiece and then restart your runtime.
First, we load the fine-tuned model from the Hub, along with the processor object used for tokenization and feature extraction. The class we’ll use is SpeechT5ForTextToSpeech .
Next, tokenize the input text.
The SpeechT5 TTS model is not limited to creating speech for a single speaker. Instead, it uses so-called speaker embeddings that capture a particular speaker’s voice characteristics. We’ll load such a speaker embedding from a dataset on the Hub.
The speaker embedding is a tensor of shape (1, 512). This particular speaker embedding describes a female voice. The embeddings were obtained from the CMU ARCTIC dataset using this script , but any X-Vector embedding should work.
Now we can tell the model to generate the speech, given the input tokens and the speaker embedding.
This outputs a tensor of shape (140, 80) containing a log mel spectrogram. The first dimension is the sequence length, and it may vary between runs as the speech decoder pre-net always applies dropout to the input sequence. This adds a bit of random variability to the generated speech.
To convert the predicted log mel spectrogram into an actual speech waveform, we need a vocoder . In theory, you can use any vocoder that works on 80-bin mel spectrograms, but for convenience, we’ve provided one in Transformers based on HiFi-GAN. The weights for this vocoder , as well as the weights for the fine-tuned TTS model, were kindly provided by the original authors of SpeechT5.
Loading the vocoder is as easy as any other 🤗 Transformers model.
To make audio from the spectrogram, do the following:
We’ve also provided a shortcut so you don’t need the intermediate step of making the spectrogram. When you pass the vocoder object into generate_speech , it directly outputs the speech waveform.
And finally, save the speech waveform to a file. The sample rate used by SpeechT5 is always 16 kHz.
The output sounds like this ( download audio ):
That’s it for the TTS model! The key to making this sound good is to use the right speaker embeddings.
You can play with an interactive demo on Spaces.
💡 Interested in learning how to fine-tune SpeechT5 TTS on your own dataset or language? Check out this Colab notebook with a detailed walk-through of the process.
Speech-to-speech for voice conversion
Conceptually, doing speech-to-speech modeling with SpeechT5 is the same as text-to-speech. Simply swap out the text encoder pre-net for the speech encoder pre-net. The rest of the model stays the same.
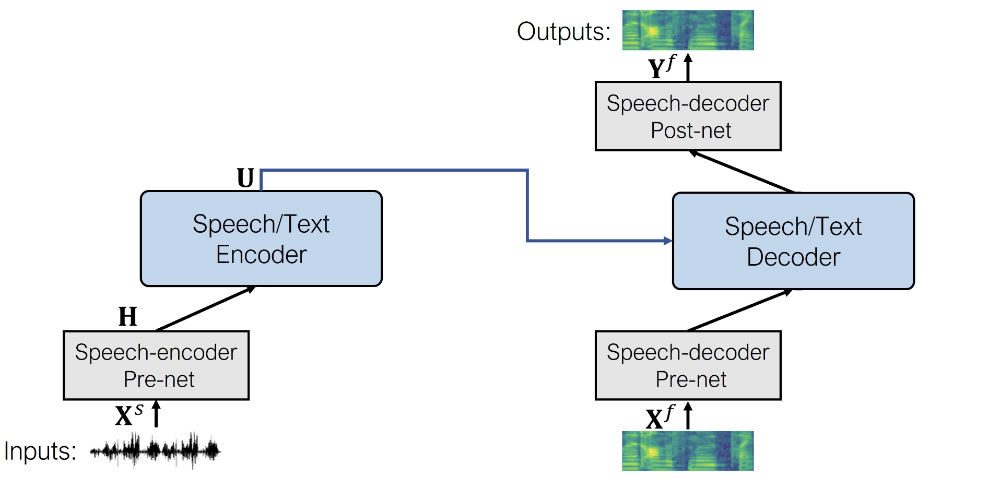
The speech encoder pre-net is the same as the feature encoding module from wav2vec 2.0 . It consists of convolution layers that downsample the input waveform into a sequence of audio frame representations.
As an example of a speech-to-speech task, the authors of SpeechT5 provide a fine-tuned checkpoint for doing voice conversion. To use this, first load the model from the Hub. Note that the model class now is SpeechT5ForSpeechToSpeech .
We will need some speech audio to use as input. For the purpose of this example, we’ll load the audio from a small speech dataset on the Hub. You can also load your own speech waveforms, as long as they are mono and use a sampling rate of 16 kHz. The samples from the dataset we’re using here are already in this format.
Next, preprocess the audio to put it in the format that the model expects.
As with the TTS model, we’ll need speaker embeddings. These describe what the target voice sounds like.
We also need to load the vocoder to turn the generated spectrograms into an audio waveform. Let’s use the same vocoder as with the TTS model.
Now we can perform the speech conversion by calling the model’s generate_speech method.
Changing to a different voice is as easy as loading a new speaker embedding. You could even make an embedding from your own voice!
The original input ( download ):
The converted voice ( download ):
Note that the converted audio in this example cuts off before the end of the sentence. This might be due to the pause between the two sentences, causing SpeechT5 to (wrongly) predict that the end of the sequence has been reached. Try it with another example, you’ll find that often the conversion is correct but sometimes it stops prematurely.
You can play with an interactive demo here . 🔥
Speech-to-text for automatic speech recognition
The ASR model uses the following pre-nets and post-net:
Speech encoder pre-net. This is the same pre-net used by the speech-to-speech model and consists of the CNN feature encoder layers from wav2vec 2.0.
Text decoder pre-net. Similar to the encoder pre-net used by the TTS model, this maps text tokens into the hidden representations using an embedding layer. (During pre-training, these embeddings are shared between the text encoder and decoder pre-nets.)
Text decoder post-net. This is the simplest of them all and consists of a single linear layer that projects the hidden representations to probabilities over the vocabulary.
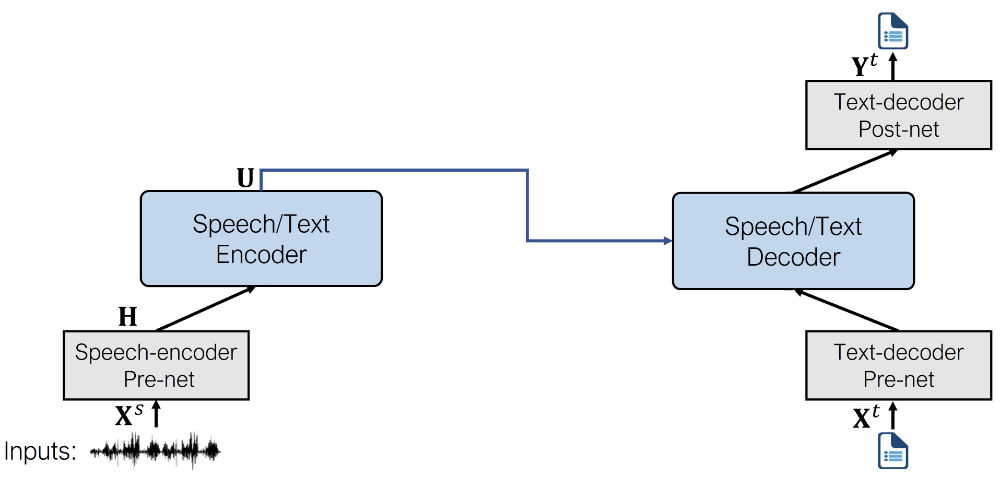
If you’ve tried any of the other 🤗 Transformers speech recognition models before, you’ll find SpeechT5 just as easy to use. The quickest way to get started is by using a pipeline.
As speech audio, we’ll use the same input as in the previous section, but any audio file will work, as the pipeline automatically converts the audio into the correct format.
Now we can ask the pipeline to process the speech and generate a text transcription.
Printing the transcription gives:
That sounds exactly right! The tokenizer used by SpeechT5 is very basic and works on the character level. The ASR model will therefore not output any punctuation or capitalization.
Of course it’s also possible to use the model class directly. First, load the fine-tuned model and the processor object. The class is now SpeechT5ForSpeechToText .
Preprocess the speech input:
Finally, tell the model to generate text tokens from the speech input, and then use the processor’s decoding function to turn these tokens into actual text.
Play with an interactive demo for the speech-to-text task .
SpeechT5 is an interesting model because — unlike most other models — it allows you to perform multiple tasks with the same architecture. Only the pre-nets and post-nets change. By pre-training the model on these combined tasks, it becomes more capable at doing each of the individual tasks when fine-tuned.
We have only included checkpoints for the speech recognition (ASR), speech synthesis (TTS), and voice conversion tasks but the paper also mentions the model was successfully used for speech translation, speech enhancement, and speaker identification. It’s very versatile!
More Articles from our Blog

Introduction to ggml
By ngxson August 13, 2024 • 76

Memory-efficient Diffusion Transformers with Quanto and Diffusers
By sayakpaul July 30, 2024 • 42
Navigation Menu
Search code, repositories, users, issues, pull requests..., provide feedback.
We read every piece of feedback, and take your input very seriously.
Saved searches
Use saved searches to filter your results more quickly.
To see all available qualifiers, see our documentation .
- Notifications You must be signed in to change notification settings
Speech To Speech: an effort for an open-sourced and modular GPT4-o
huggingface/speech-to-speech
Folders and files.
Name | Name | |||
---|---|---|---|---|
49 Commits | ||||
Repository files navigation

📖 Quick Index
- Server/Client approach
- Local approach
- Model parameters
- Generation parameters
- Notable parameters
This repository implements a speech-to-speech cascaded pipeline with consecutive parts:
- Voice Activity Detection (VAD) : silero VAD v5
- Speech to Text (STT) : Whisper checkpoints (including distilled versions )
- Language Model (LM) : Any instruct model available on the Hugging Face Hub ! 🤗
- Text to Speech (TTS) : Parler-TTS 🤗
The pipeline aims to provide a fully open and modular approach, leveraging models available on the Transformers library via the Hugging Face hub. The level of modularity intended for each part is as follows:
- VAD : Uses the implementation from Silero's repo .
- STT : Uses Whisper models exclusively; however, any Whisper checkpoint can be used, enabling options like Distil-Whisper and French Distil-Whisper .
- LM : This part is fully modular and can be changed by simply modifying the Hugging Face hub model ID. Users need to select an instruct model since the usage here involves interacting with it.
- TTS : The mini architecture of Parler-TTS is standard, but different checkpoints, including fine-tuned multilingual checkpoints, can be used.
The code is designed to facilitate easy modification. Each component is implemented as a class and can be re-implemented to match specific needs.
Clone the repository:
Install the required dependencies:
The pipeline can be run in two ways:
- Server/Client approach : Models run on a server, and audio input/output are streamed from a client.
- Local approach : Uses the same client/server method but with the loopback address.
Server/Client Approach
To run the pipeline on the server:
Then run the client locally to handle sending microphone input and receiving generated audio:
Local Approach
Simply use the loopback address:
You can pass --device mps to run it locally on a Mac.
Recommended usage
Leverage Torch Compile for Whisper and Parler-TTS:
For the moment, modes capturing CUDA Graphs are not compatible with streaming Parler-TTS ( reduce-overhead , max-autotune ).
Command-line Usage
Model parameters.
model_name , torch_dtype , and device are exposed for each part leveraging the Transformers' implementations: Speech to Text, Language Model, and Text to Speech. Specify the targeted pipeline part with the corresponding prefix:
- stt (Speech to Text)
- lm (Language Model)
- tts (Text to Speech)
For example:
Generation Parameters
Other generation parameters of the model's generate method can be set using the part's prefix + _gen_ , e.g., --stt_gen_max_new_tokens 128 . These parameters can be added to the pipeline part's arguments class if not already exposed (see LanguageModelHandlerArguments for example).
Notable Parameters
Vad parameters.
- --thresh : Threshold value to trigger voice activity detection.
- --min_speech_ms : Minimum duration of detected voice activity to be considered speech.
- --min_silence_ms : Minimum length of silence intervals for segmenting speech, balancing sentence cutting and latency reduction.
Language Model
- --init_chat_role : Defaults to None . Sets the initial role in the chat template, if applicable. Refer to the model's card to set this value (e.g. for Phi-3-mini-4k-instruct you have to set --init_chat_role system )
- --init_chat_prompt : Defaults to "You are a helpful AI assistant." Required when setting --init_chat_role .
Speech to Text
--description : Sets the description for Parler-TTS generated voice. Defaults to: "A female speaker with a slightly low-pitched voice delivers her words quite expressively, in a very confined sounding environment with clear audio quality. She speaks very fast."
--play_steps_s : Specifies the duration of the first chunk sent during streaming output from Parler-TTS, impacting readiness and decoding steps.
Distil-Whisper
Contributors 5.
- Python 100.0%
[affiliation=1]SamueleCornell* \name [affiliation=2]JordanDarefsky* \name [affiliation=2]ZhiyaoDuan \name [affiliation=1]ShinjiWatanabe
Generating Data with Text-to-Speech and Large-Language Models for Conversational Speech Recognition
Currently, a common approach in many speech processing tasks is to leverage large scale pre-trained models by fine-tuning them on in-domain data for a particular application. Yet obtaining even a small amount of such data can be problematic, especially for sensitive domains and conversational speech scenarios, due to both privacy issues and annotation costs. To address this, synthetic data generation using single speaker datasets has been employed. Yet, for multi-speaker cases, such an approach often requires extensive manual effort and is prone to domain mismatches. In this work, we propose a synthetic data generation pipeline for multi-speaker conversational ASR, leveraging a large language model (LLM) for content creation and a conversational multi-speaker text-to-speech (TTS) model for speech synthesis. We conduct evaluation by fine-tuning the Whisper ASR model for telephone and distant conversational speech settings, using both in-domain data and generated synthetic data. Our results show that the proposed method is able to significantly outperform classical multi-speaker generation approaches that use external, non-conversational speech datasets.
1 Introduction
Current robust speech processing methods are considerably data hungry. For example, state-of-the-art automatic speech recognition (ASR) systems require tens or even hundreds of thousands of hours of training data in order to achieve enough robustness in different domains [ 1 , 2 , 3 ] . Such a vast amount of training data is leveraged either explicitly by training from scratch on a large amount of data or implicitly by fine-tuning/adapting a pre-trained “foundation” model that was originally trained, in a supervised or unsupervised manner [ 4 , 5 , 6 , 1 ] , on a large dataset.
Nevertheless, for some domains, obtaining even a small portion of in-domain supervised data for fine-tuning can be problematic due to potential privacy concerns or prohibitive expense.
This is especially true for sensitive application scenarios, including medical, government, and law enforcement settings. Moreover, due to increasing regulatory attention, even scaling in-domain training data is potentially becoming more difficult.
Aside from privacy issues, applications that require recordings with multiple speakers are also inherently difficult, time-consuming and costly to annotate and thus obtain in scale. Prominent examples are meeting scenarios [ 7 , 8 ] including doctor-patient recordings, speech captioning, speech analytics and so on.
Despite the difficulties associated with obtaining data for multi-speaker scenarios, there are speech processing approaches that require multi-speaker conversational data for training. These approaches have proven to be effective as demonstrated in recent speech processing challenges [ 7 , 8 , 9 ] . Prominent examples are end-to-end neural diarization (EEND) and most target speaker voice activity detection (TS-VAD) approaches [ 10 , 11 , 12 , 13 , 14 ] , as well as multi-speaker ASR [ 15 , 16 , 17 , 18 , 19 ] . Lack of annotated in-domain conversational data at scale is a significant issue for these techniques, which is only partly mitigated by leveraging foundation models [ 17 , 18 , 19 ] . Consequently, many of these approaches have to rely on synthetic data to increase dataset size. This is commonly achieved by artificially overlapping clips from existing datasets and adding noise and reverberation.
While several toolkits have been proposed to ease the workload [ 20 , 21 ] , creating synthetic datasets remains more art than science, as it often requires extensive hand-tuning, domain knowledge, heuristics, and significant trial and error. Crucially, this process is highly prone to the introduction of unwanted biases in the resulting dataset, leading to a performance drop due to domain mismatch [ 12 ] .
The aforementioned difficulties motivate the development of more automated, machine learning based approaches for synthetic data creation. Several methods have in fact explored this direction, primarily focusing on improving ASR performance by leveraging synthetic data created with text-to-speech (TTS) models [ 22 , 23 , 24 , 25 , 26 , 27 , 28 , 27 , 29 , 30 , 31 ] or leveraging ASR and TTS cycle-consistency during training [ 32 , 33 ] for semi-supervised training. However, these approaches focus on single-speaker scenarios and thus cannot be directly applied to domains where multi-speaker conversational ASR is required. In parallel, recent works [ 34 , 35 ] on speech summarization and audio captioning have shown how large-language models (LLM) s can be leveraged effectively for synthetic audio data augmentation.
Building upon this previous research, in this work we explore using TTS models along with LLM s to generate multi-speaker conversational data. We focus on two-speaker ASR on real-world telephone (Fisher [ 36 ] ) and distant speech recognition settings (Mixer 6 Speech [ 37 ] ) by fine-tuning Whisper [ 1 ] . The contributions of this work are the following: 1) We propose a synthetic data generation pipeline for conversational ASR using LLMs for content generation and a conversational multi-speaker TTS model for speech generation; 2) We perform a systematic investigation on the use of synthetic data for training multi-speaker ASR models with three different approaches: using “classical” LibriSpeech based multi-speaker simulation, using a conventional state-of-the-art (SotA) TTS model, and using a recently proposed conversational TTS model [ 38 ] .
2 Method under study
Our approach is summarized in Figure 1 . We explore the use of a pre-trained chat-optimized LLM for creating short conversation transcripts between two participants from scratch for when in-domain conversational transcriptions are not available or would be costly to obtain. Specifically, we use the recently released Llama 3 8B Instruct model and few-shot prompt it with 8 text-prompt examples randomly selected from a 1000-example subset of Spotify Podcasts dataset [ 39 ] used to train Parakeet (the text data was transcribed using Whisper-D, described in [ 38 ] ). That is, for each new example we want to generate, we randomly select a subset of eight text samples from our Parakeet subset to use as the few-shot prompt. This procedure could also be used to augment existing in-domain text-only data. It could also be worth exploring fine-tuning on in-domain data instead of prompting.
These LLM obtained transcripts are then used to generate synthesized speech through a multi-speaker TTS model. The resulting data, consisting of ground truth multi-speaker transcripts and the synthesized multi-speaker mixture can then be used for training or fine-tuning purposes, i.e. in Sec. 4 for adapting Whisper to perform multi-speaker ASR.
2.1 Conversational TTS generation
The effectiveness of this approach will heavily depend on the capability of the TTS model used. While we expect LLM s will be proficient in generating conversational transcripts as shown in previous work on summarization [ 34 ] , most TTS models are not capable of synthesizing multi-speaker conversational data. Although one could naively generate each speaker’s utterances independently and then stitch them together, such an approach would fail to capture real conversational speech turn-taking dynamics and para-linguistic subtleties such as changes in intonation, etc., and would therefore potentially introduce a domain mismatch in the generated audio.
Recently, in [ 38 ] a conversational TTS model, Parakeet, has been proposed. Parakeet’s training dataset includes 60,000 hours of Spotify Podcasts data, much of which is multi-speaker. It is therefore able to directly generate two-speaker short conversations of up to 30 seconds when given a text prompt in the style of the one in Figure 1 , i.e. with speaker-id related tags [S1] and [S2]. We use a diffusion version of Parakeet that, similar to [ 40 ] autoregressively generates blocks of continuous latents using latent diffusion on each block. The autoencoder is trained to map 44,100 Hz audio to 16-channel dimensional latents, with a time downsampling factor of 1024. Each diffusion block consists of 128 (time-wise) latent vectors, which correspond to approximately three seconds of audio.
LLM-generated transcripts and speech examples are available online 1 1 1 popcornell.github.io/SynthConvASRDemo .

3 Experimental setup
3.1 evaluation data.
In this work, we focus on two-speaker multi-speaker conversational ASR. This focus is due to the limitations of Parakeet, whose generations tend to lose correctness as the number of unique speakers in the text prompt increases. Furthermore, we also consider scenarios with relatively high signal-to-noise ratio (SNR) ; tackling more complex settings such as CHiME-6 [ 7 ] requires modeling of background noise and dynamic acoustic conditions (as the participants move, reverberation can change significantly). We thus perform our experiments using two conversational speech datasets with these characteristics: Fisher Corpus (both Part 1 and Part 2) and Mixer 6 Speech.
3.1.1 Fisher
Fisher consists of 11699 11699 11699 11699 telephone conversations between two English speakers sampled at 8 8 8 8 kHz. Each conversation is around 10 10 10 10 minutes long. We use the train, validation, and test split from [ 41 ] ( 11577 11577 11577 11577 , 61 61 61 61 and 61 61 61 61 conversations of respectively 1960 1960 1960 1960 h, 7 7 7 7 h and 7 7 7 7 h). The Fisher recordings originally separate each of the speakers into different channels; however, since our focus is on general single-channel conversational speech processing, we mixdown the two channels to mono. We also resample the signal to 16 16 16 16 kHz as we use Whisper which was trained on 16 16 16 16 kHz data (see Sec. 3.3 ).
3.1.2 Mixer 6 6 6 6 Speech
As an additional scenario, we consider Mixer 6 Speech. Specifically we use the version re-annotated for the CHiME-7 challenge [ 8 ] . It consists of two-speaker interviews of approximately 15 15 15 15 minutes (sampled at 16 kHz) recorded by 14 different far-field recording devices. In this work we only use recordings from the tabletop microphone device (CH04). We use the splitting from [ 8 ] , where full long-form annotation is only available for the development ( 59 59 59 59 interviews, 15 15 15 15 h) and evaluation sets ( 23 23 23 23 interviews, 13 13 13 13 h). Here we further split the development set into an adaptation portion and a validation portion of respectively 2:30 h and 4 h after discarding utterance groups longer than 30 s as done in [ 19 ] . This further split allows us to compare the use of synthetic data versus in-domain data for fine-tuning.
3.2 Baseline Methods
3.2.1 nemo multi-speaker simulation tool.
We consider two baseline methods. The first method we consider is a “classical” synthetic speech generation method, where single speaker speech from one high quality speech dataset (e.g. LibriSpeech [ 42 ] ) is used to construct conversation-style synthetic recordings by artificially overlapping single speaker utterances and contaminating them by adding noise, artificial room impulse response (RIR) or other transforms (e.g. clipping, microphone transfer function etc.). We make use of the SotA NeMo multi-speaker simulation tool [ 21 ] (NeMo MSS in the following). We use LibriSpeech train-clean 360 360 360 360 and 100 100 100 100 portions and generate 100 100 100 100 h of short conversations between two speakers of up to 30 30 30 30 seconds in length. For Mixer 6 Speech experiments, we additionally use the built-in RIR simulation in order to generate simulated far-field speech.
3.2.2 xTTS-v2
The second baseline method we consider is the approach outlined in Section 2 , where a standard TTS model is used to generate the training data. We explore this using the Coqui xTTS-v2 model [ 43 ] (denoted simply as xTTS in Sec. 4 ) In detail, for each utterance group in the training dataset (either LLM-generated or taken from a text-only corpus) we sample two speaker ids from LibriSpeech train-clean 360 360 360 360 and 100 100 100 100 and then two corresponding LibriSpeech enrollment utterances to condition xTTS-v2 for the generated TTS id. We then generate each utterance in the utterance group independently via xTTS-v2 and truncate excessive leading and trailing silence regions using Silero VAD [ 44 ] . The generated audio is then resampled to 16 16 16 16 kHz and mixed together by randomly adding start time offsets based on the order of the sentences in the utterance group transcript, ensuring that utterances from the same speaker do not overlap.
3.3 ASR System
In our experiments, which focus on two-speaker conversational speech, we use the method proposed in [ 19 ] where Whisper [ 1 ] is adapted to perform multi-speaker ASR through fine-tuning with a serialized output training (SOT) [ 15 ] objective on utterance groups. This approach aligns with common practices in the field where a model pre-trained on a large amount of data (i.e. a foundation model) is fine-tuned/adapted for a particular domain or application of interest.
Compared to [ 19 ] , in our experiments we focus only on standard SOT without considering timestamps and use only Whisper medium. We use low-rank adapters (LoRA) [ 45 ] while the rest of the model is kept frozen. During each fine-tuning experiment a linear warm-up schedule is employed for the first N 𝑁 N italic_N epoch, then the learning rate is linearly decayed over a maximum of 20 20 20 20 epochs. The L 2 superscript 𝐿 2 L^{2} italic_L start_POSTSUPERSCRIPT 2 end_POSTSUPERSCRIPT norm of the gradients is clipped to 5 5 5 5 . One LoRA adapter for each linear layer in the model (i.e. for each query, key, value and feed-forward network layer) is used. For each adapter we set the LORA rank to 64 64 64 64 , alpha to 128 128 128 128 , and dropout to 0.1 0.1 0.1 0.1 . In our preliminary experiments on the full Fisher training set, we found that this configuration yields the best results, even when compared to fine-tuning the entire model. If validation loss does not improve for 2 2 2 2 consecutive epochs the training is stopped. We tune the batch size, number of warm-up epochs ( N 𝑁 N italic_N ) and the value of the maximum learning rate for each set of experiments. Parakeet synthesized audio is resampled to 16 16 16 16 kHz in our experiments. In Fisher experiments, for all synthetic data, we use on-the-fly resampling to simulate telephone 3400 3400 3400 3400 Hz band-limiting. In Mixer 6 experiments, only for xTTS and Parakeet, we contaminate the data with reverberation using random RIRs obtained from [ 46 ] . This of course is less realistic than the RIR simulation used in NeMo MSS as the RIR is the same for both speakers. We make our fine-tuning code publicly available 2 2 2 github.com/popcornell/ASRLightningFT .
3.4 Evaluation Setup
For each dataset, we run our experiments using the same setup as in [ 19 ] , where oracle voice activity detection (VAD) is used and the dataset is divided into several utterance groups [ 3 , 19 ] . Continuing to follow [ 19 ] , we then perform evaluation for each utterance group independently and accumulate word error rate (WER) statistics over the whole dataset (insertions, deletions etc.). We choose this evaluation method because we only focus on multi-speaker ASR, and an evaluation which considers the whole conversation (e.g. as in CHiME-6/7) would require a diarization component, which would add significant complexity.
We thus consider concatenated minimum permutation WER (cpWER) [ 7 ] . This is the same as WER in [ 19 ] , with the best permutation evaluated for each utterance group independently. We also consider multi-input multi-output WER (MIMO-WER) , which is more tolerant than cpWER to speaker assignment errors. We use the Meeteval toolkit [ 47 ] to compute both scores. Whisper text normalization is used both during training and scoring.
4 Experiments
In Table 1 we report results obtained on the Fisher test set as defined in Sec. 3.1.1 with different data used for fine-tuning. As a baseline, in the first row, we report the results with no adaptation. In the second panel, we report results on in-domain Fisher training data adaptation. We observe only a modest difference between using the full training set or a 80 h data subset, which is likely because we are leveraging a strong pre-trained model. In the third and fourth panels, we report results obtained with synthetic data approaches. In particular, for the two TTS approaches (xTTS and Parakeet), we consider two opposite situations: a best-case/oracle scenario where we use in-domain conversation transcriptions and another one where we suppose we have none and thus we use as input Llama-3 random generated utterance groups transcripts (LLM rnd ) as described in Sec. 2 .
We observe that xTTS-based generation outperforms NeMo MSS when Fisher only transcriptions (Fisher) are used. When LLM generated transcriptions are used (LLM rnd ), xTTS performance is on par/slightly worse than NeMo MSS. In contrast, when using Parakeet, the difference between using LLM generated transcripts versus the Fisher training set transcriptions is modest, and interestingly, the generated transcripts yield the best performance. In general, while the performance gain compared to the baseline synthetic data approaches (xTTS and NeMo MSS) is significant, there remains a substantial gap compared to using in-domain data (Fisher). It appears that this gap cannot be bridged solely by scaling the amount of synthetic data.
In Figure 2 we report cpWER on Fisher for different amounts of adaptation data, both from Fisher training set and from synthetic approaches. For modest amounts of data (less than 5 h) the proposed approach is competitive to using in-domain data; however, as the amount of adaptation data is scaled, performance saturates quickly: The improvement between 50 h and 5 h is marginal when compared to the one afforded by using in-domain data. This trend is also observed for the other synthetic data approaches and suggests that there is some inherent mismatch in all of the synthetic data approaches tested that prevents effective scaling. At least for Parakeet, results suggest that this mismatch seems to be more related to the signal/acoustic content rather than the transcription semantic content as the gap between using Fisher transcriptions and LLM-generated transcription is modest.
Adaptation Data | amount | cpWER | MIMO-WER |
---|---|---|---|
(hours) | (%) | (%) | |
- | 0 | 44.94 | 26.15 |
Fisher | 13.76 | 13.58 | |
Fisher | 15.43 | 14.94 | |
NeMo MSS | 34.37 | 26.51 | |
xTTS (Fisher) | 24.88 | 24.07 | |
xTTS (LLM ) | 34.65 | 28.31 | |
Parakeet (Fisher) | 21.44 | 21.00 | |
Parakeet (LLM ) | 20.41 | 19.48 | |
Parakeet (LLM ) | 19.93 | 19.45 |

4.2 Mixer 6 Speech
In Table 2 , we show results obtained on Mixer 6. The trends observed are consistent with the Fisher experiments, despite the rather naive artificial reverberation strategy used for xTTS and Parakeet experiments. This confirms that the proposed approach can also be effective for far-field multi-speaker synthetic data, at least when compared to the classical approach (NeMo MSS results) and when available in-domain data is very scarce (here 2:30 h). Parakeet (LLM rnd , 80 h) also compares favorably with the third and fourth rows, where we report the results of using the Fisher full 1960 h training set and a 80 h subset respectively for adaptation. For these Fisher experiments, to reduce the mismatch due to the telephone lower sampling frequency, we apply telephone band-limiting to Mixer 6 in the inference phase. We also contaminate the Fisher 6 training data with reverberation as done for Parakeet and xTTS as described in Sec. 3.3 .
4.3 Further discussion & remarks
Considering both Fisher and Mixer 6 experiments, the fact that Parakeet+LLM rnd improves considerably over NeMo MSS while xTTS fails suggests that turn-taking and para-linguistics may play a considerable role for multi-talker ASR.
Finally, for both Mixer 6 Speech and Fisher scenarios, we tried using 50 h 50 ℎ 50\,h 50 italic_h of synthetic LLM rnd data to augment a portion of in-domain data ( 5 h 5 ℎ 5\,h 5 italic_h and 50 h 50 ℎ 50\,h 50 italic_h ) by mixing the two or by training on synthetic data and then fine-tuning on in-domain data. However, in most instances, this approach does not result in any improvement over using solely the in-domain data; in the xTTS and NeMo MSS cases we even observe performance degradation. For example, by combining 50 h 50 ℎ 50\,h 50 italic_h of Parakeet (LLM rnd ) and 50 h 50 ℎ 50\,h 50 italic_h of original Fisher training data the model achieved a cpWER of 15.74 15.74 15.74 15.74 % which is only marginally better than the 16.36 16.36 16.36 16.36 % obtained with only 50 h 50 ℎ 50\,h 50 italic_h of Fisher (Figure 2 ). Interestingly, negligible or no improvement was also observed when the in-domain data was more modest (5 h). This may be due to the fact that we are leveraging a strong pre-trained model, and thus the quality of adaptation data rather than quantity matters most. Future work should explore adaptation of the TTS model to generate synthetic audio that better matches distribution of in-domain data.
Adaptation Data | amount | cpWER | MIMO-WER |
---|---|---|---|
(hours) | (%) | (%) | |
- | 0 | 43.67 | 32.16 |
Mixer6 | 2.30 | 20.36 | 19.77 |
Fisher | 1960 | 20.83 | 20.33 |
Fisher | 80 | 22.12 | 21.36 |
NeMo MSS | 80 | 36.71 | 28.21 |
xTTS (Mixer6) | 2.30 | 25.99 | 24.47 |
xTTS (LLM ) | 80 | 35.65 | 30.18 |
Parakeet (Mixer6) | 2.30 | 23.52 | 22.82 |
Parakeet (LLM ) | 2.30 | 23.70 | 22.12 |
Parakeet (LLM ) | 80 | 21.25 | 20.17 |
5 Conclusions
In this work, we study the use of synthetically generated data for multi-speaker ASR , focusing on the two-speaker case. We explore different strategies of generating synthetic data, comparing artificially overlapped data and SotA conventional TTS models with a novel conversational TTS model, Parakeet, capable of natively generating multi-speaker utterances. Our results show that our approach using Parakeet significantly outperforms previous SotA multi-speaker simulation techniques. Furthermore, when in-domain data is limited to only a few hours, our approach achieves performance reasonably close to that of using in-domain data; however, when more in-domain data is available, our approach lags behind using real data. For Mixer 6, our approach also obtains results comparable to using external real-world multi-speaker data (Fisher). Overall, our experiments suggest that the LLM generated transcripts are reliable but that there is currently a performance gap compared to using in-domain audio data (when enough in-domain data exists).
Limitations of our work include that we only consider two-speaker conversational speech, short 30-second conversations, and relatively high SNR scenarios. These constraints were primarily imposed by the current limitations of the Parakeet TTS model, and thus improvement of TTS capabilities is crucial to increasing synthetic data viability. For example, to tackle more complex noisy/reverberant scenarios, the TTS model needs to incorporate acoustic scenario modeling, e.g. via acoustic style transfer techniques or even few-shot adaptation on some in-domain data (e.g. via [ 48 ] ). Another possible limitation is that Parakeet itself is trained on text-audio pairs where the text is “synthetic”, i.e. Whisper-D [ 38 ] is used to generate multi-speaker transcriptions for Spotify podcast audio which is then used for Parakeet training. Since Whisper-D is fine-tuned from Whisper using a small number of annotated multi-speaker examples (and Whisper itself is likely trained on a sizeable quantity of multi-speaker data), there is an indirect but somewhat circular dependency on the existence of ground-truth annotations. Also, Parakeet’s weakness in generating consistent 3/4-speaker conversational data could in part be due to limitations of Whisper-D. Future work could potentially explore the joint bootstrapping of audio-to-text and text-to-audio models.
6 Acknowledgments
S. Cornell was supported by IC Postdoctoral Research Fellowship Program at CMU via ORISE through an agreement between U.S. DoE and ODNI. We’d like to thank Google’s TPU Research Cloud (TRC), which provided compute for generating synthetic Parakeet samples and Llama synthetic text utterances. Our work would not have been possible without their support.
- [1] A. Radford et al. , “Robust speech recognition via large-scale weak supervision,” in ICML . PMLR, 2023.
- [2] Y. Peng et al. , “Reproducing whisper-style training using an open-source toolkit and publicly available data,” in Proc. of ASRU . IEEE, 2023.
- [3] N. Kanda et al. , “Large-scale pre-training of end-to-end multi-talker asr for meeting transcription with single distant microphone,” arXiv preprint arXiv:2103.16776 , 2021.
- [4] A. Baevski et al. , “wav2vec 2.0: A framework for self-supervised learning of speech representations,” Advances in neural information processing systems , vol. 33, 2020.
- [5] W.-N. Hsu et al. , “Hubert: Self-supervised speech representation learning by masked prediction of hidden units,” IEEE/ACM TASLP , vol. 29, 2021.
- [6] S. Chen et al. , “WavLM: Large-scale self-supervised pre-training for full stack speech processing,” IEEE Journal of Selected Topics in Signal Processing , vol. 16, no. 6, 2022.
- [7] S. Watanabe et al. , “CHiME-6 challenge: Tackling multispeaker speech recognition for unsegmented recordings,” in CHiME Workshop , 2020.
- [8] S. Cornell et al. , “The CHiME-7 DASR challenge: Distant meeting transcription with multiple devices in diverse scenarios,” CHiME Workshop , 2023.
- [9] N. Ryant et al. , “The third dihard diarization challenge,” Proc. of Interspeech , 2021.
- [10] Y. Fujita et al. , “End-to-end neural speaker diarization with self-attention,” in Proc. of ASRU . IEEE, 2019.
- [11] K. Kinoshita, M. Delcroix, and N. Tawara, “Integrating end-to-end neural and clustering-based diarization: Getting the best of both worlds,” in Proc. of ICASSP . IEEE, 2021.
- [12] F. Landini et al. , “From simulated mixtures to simulated conversations as training data for end-to-end neural diarization,” 2022.
- [13] I. Medennikov et al. , “Target-speaker voice activity detection: a novel approach for multi-speaker diarization in a dinner party scenario,” Proc. of Interspeech , 2020.
- [14] N. Tawara et al. , “Ntt speaker diarization system for chime-7: multi-domain, multi-microphone end-to-end and vector clustering diarization,” CHiME Workshop , 2023.
- [15] N. Kanda et al. , “Serialized output training for end-to-end overlapped speech recognition,” Proc. of Interspeech , 2020.
- [16] ——, “Investigation of end-to-end speaker-attributed asr for continuous multi-talker recordings,” in Proc. of SLT . IEEE, 2021.
- [17] Z. Huang et al. , “Adapting self-supervised models to multi-talker speech recognition using speaker embeddings,” in Proc. of ICASSP . IEEE, 2023.
- [18] S. Cornell et al. , “One model to rule them all? towards end-to-end joint speaker diarization and speech recognition,” in Proc. of ICASSP . IEEE, 2024.
- [19] C. Li et al. , “Adapting multi-lingual asr models for handling multiple talkers,” Proc. of Interspeech , 2023.
- [20] T. Cord-Landwehr et al. , “Mms-msg: A multi-purpose multi-speaker mixture signal generator,” in Proc. of IWAENC . IEEE, 2022.
- [21] T. J. Park et al. , “Property-aware multi-speaker data simulation: A probabilistic modelling technique for synthetic data generation,” Proc. of Interspeech , 2023.
- [22] A. Rosenberg et al. , “Speech recognition with augmented synthesized speech,” in Proc. of ASRU . IEEE, 2019.
- [23] Z. Chen et al. , “Improving speech recognition using gan-based speech synthesis and contrastive unspoken text selection.” in Proc. of Interspeech , 2020.
- [24] N. Rossenbach et al. , “Generating synthetic audio data for attention-based speech recognition systems,” in Proc. of ICASSP . IEEE, 2020.
- [25] A. Tjandra, S. Sakti, and S. Nakamura, “Machine speech chain,” IEEE/ACM TASLP , vol. 28, 2020.
- [26] A. Fazel et al. , “SynthASR: Unlocking synthetic data for speech recognition,” Proc. of Interspeech , 2021.
- [27] X. Zheng et al. , “Using synthetic audio to improve the recognition of out-of-vocabulary words in end-to-end asr systems,” in Proc. of ICASSP . IEEE, 2021.
- [28] S. Ueno et al. , “Data augmentation for asr using tts via a discrete representation,” in Proc. of ASRU . IEEE, 2021.
- [29] T.-Y. Hu et al. , “Synt++: Utilizing imperfect synthetic data to improve speech recognition,” in Proc. of ICASSP . IEEE, 2022.
- [30] M. Soleymanpour et al. , “Synthesizing dysarthric speech using multi-speaker tts for dysarthric speech recognition,” in Proc. of ICASSP . IEEE, 2022.
- [31] E. Casanova et al. , “Asr data augmentation in low-resource settings using cross-lingual multi-speaker tts and cross-lingual voice conversion,” in Proc. of Interspeech , 2023.
- [32] T. Hori et al. , “Cycle-consistency training for end-to-end speech recognition,” in Proc. of ICASSP . IEEE, 2019.
- [33] M. K. Baskar et al. , “Eat: Enhanced asr-tts for self-supervised speech recognition,” in Proc. of ICASSP . IEEE, 2021.
- [34] J.-w. Jung et al. , “Augsumm: towards generalizable speech summarization using synthetic labels from large language model,” Proc. of ICASSP , 2024.
- [35] S.-L. Wu et al. , “Improving audio captioning models with fine-grained audio features, text embedding supervision, and llm mix-up augmentation,” in Proc. of ICASSP . IEEE, 2024.
- [36] C. Cieri, D. Miller, and K. Walker, “The Fisher corpus: A resource for the next generations of speech-to-text.” in LREC , 2004.
- [37] L. Brandschain et al. , “The Mixer 6 corpus: Resources for cross-channel and text independent speaker recognition,” in LREC , 2010.
- [38] J. Darefsky, G. Zhu, and Z. Duan, “Parakeet,” 2024. [Online]. Available: https://jordandarefsky.com/blog/2024/parakeet/
- [39] A. Clifton et al. , “100,000 podcasts: A spoken english document corpus,” in Proceedings of the 28th International Conference on Computational Linguistics , 2020.
- [40] Z. Liu et al. , “Autoregressive diffusion transformer for text-to-speech synthesis,” arXiv preprint arXiv:2406.05551 , 2024.
- [41] G. Morrone et al. , “End-to-end integration of speech separation and voice activity detection for low-latency diarization of telephone conversations,” Speech Communication , 2024.
- [42] V. Panayotov et al. , “Librispeech: an asr corpus based on public domain audio books,” in Proc. of ICASSP , 2015.
- [43] E. Casanova et al. , “Xtts: a massively multilingual zero-shot text-to-speech model,” arXiv e-prints , 2024.
- [44] S. Team, “Silero vad: pre-trained enterprise-grade voice activity detector (vad), number detector and language classifier,” https://github.com/snakers4/silero-vad , 2021.
- [45] E. J. Hu et al. , “Lora: Low-rank adaptation of large language models,” arXiv preprint arXiv:2106.09685 , 2021.
- [46] T. Ko et al. , “A study on data augmentation of reverberant speech for robust speech recognition,” in Proc. of ICASSP . IEEE, 2017.
- [47] T. von Neumann et al. , “MeetEval: A toolkit for computation of word error rates for meeting transcription systems,” CHiME Workshop , 2023.
- [48] L. Zhang, A. Rao, and M. Agrawala, “Adding conditional control to text-to-image diffusion models,” in Proceedings of the IEEE/CVF International Conference on Computer Vision , 2023.
- Español – América Latina
- Português – Brasil
- Documentation
- 2.17.1 (latest)
Google Cloud Text-to-Speech API
Overview of the APIs available for Google Cloud Text-to-Speech API.
All entries
Classes, methods and properties & attributes for Google Cloud Text-to-Speech API.
properties and attributes
Except as otherwise noted, the content of this page is licensed under the Creative Commons Attribution 4.0 License , and code samples are licensed under the Apache 2.0 License . For details, see the Google Developers Site Policies . Java is a registered trademark of Oracle and/or its affiliates.
Last updated 2024-08-21 UTC.
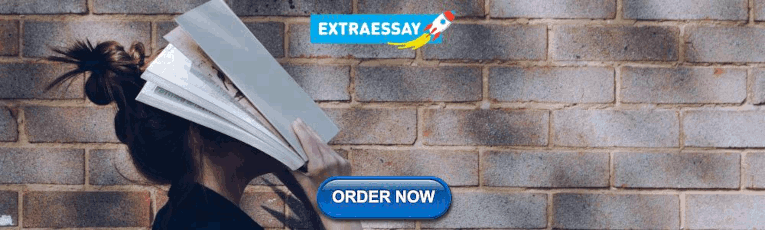
IMAGES
COMMENTS
To specify a specific model to use for audio transcription, you must set the model field to one of the allowed values—such as latest_long , latest_short, telephony, or telephony_short —in the RecognitionConfig parameters for the request. Speech-to-Text supports model selection for all speech recognition methods: speech:recognize , speech ...
A single model unifies data from multiple languages. However, users still specify the language in which the model should recognize speech. Chirp does not support some of the Google Speech features that other models have. See below for a complete list. Model identifiers. Chirp is available in the Speech-to-Text API v2.
Cloud Text-to-Speech lets you choose from 32 different voices from 12 languages and variants. Cloud Text-to-Speech correctly pronounces complex text such as names, dates, times and addresses for authentic sounding speech right out of the gate. Cloud Text-to-Speech also allows you to customize pitch, speaking rate, and volume gain, and supports ...
Our most capable generative video model. A tool to explore new applications and creative possibilities with video generation. With just text prompts, it creates high-quality, 1080P videos that can go beyond 60 seconds. Lets you control the camera, and prompt for things like time lapse or aerial shots of a landscape.
Voice Builder is an opensource text-to-speech (TTS) voice building tool that focuses on simplicity, flexibility, and collaboration. Our tool allows anyone with basic computer skills to run voice training experiments and listen to the resulting synthesized voice. We hope that this tool will reduce the barrier for creating new voices and ...
🐸TTS already provides tooling for the LJSpeech. if you use the same format, you can start training your models right away.. After you collect and format your dataset, you need to check two things. Whether you need a formatter and a text_cleaner. The formatter loads the text file (created above) as a list and the text_cleaner performs a sequence of text normalization operations that converts ...
Learning from human speech. WaveNet is a generative model trained on human speech samples. It creates waveforms of speech patterns by predicting which sounds are most likely to follow each other, each built one sample at a time, with up to 24,000 samples per second of sound. The model incorporates natural-sounding elements, such as lip-smacking ...
DeepVoice3: Single-speaker text-to-speech demo. In this notebook, you can try DeepVoice3-based single-speaker text-to-speech (en) using a model trained on LJSpeech dataset. The notebook is supposed to be executed on Google colab so you don't have to setup your machines locally. Estimated time to complete: 5 miniutes.
For more examples on what Bark and other pretrained TTS models can do, refer to our Audio course. If you are looking to fine-tune a TTS model, the only text-to-speech models currently available in 🤗 Transformers are SpeechT5 and FastSpeech2Conformer, though more will be added in the future.SpeechT5 is pre-trained on a combination of speech-to-text and text-to-speech data, allowing it to ...
Downloads. We encourage you to start with the Google Colab link above or run the provided notebook locally. If you want to download manually or train the models from scratch then both the WhisperSpeech pre-trained models as well as the converted datasets are available on HuggingFace. Roadmap.
The Text-to-Speech API enables developers to generate human-like speech. The API converts text into audio formats such as WAV, MP3, or Ogg Opus. It also supports Speech Synthesis Markup Language (SSML) inputs to specify pauses, numbers, date and time formatting, and other pronunciation instructions. In this tutorial, you will focus on using the ...
For example, Speech-to-Text has a transcription model trained to recognize speech recorded over the phone. When Speech-to-Text uses the telephony model to transcribe phone audio, it produces more accurate transcription results than if it had transcribed phone audio using the latest_long or medical_dictation models, for example. The following ...
Ephemeral Learning is a privacy preserving technique we use when the speech model runs on Google's servers. How ephemeral learning works to train speech models. As our systems convert incoming audio samples into text, those samples are sent to short-term memory (RAM).
GSP222. Overview. The Text-to-Speech API lets you create audio files of machine-generated, or synthetic, human speech.You provide the content as text or Speech Synthesis Markup Language (SSML), specify a voice (a unique 'speaker' of a language with a distinctive tone and accent), and configure the output; the Text-to-Speech API returns to you the content that you sent as spoken word, audio ...
Text-to-Speech. Text-to-Speech (TTS) is the task of generating natural sounding speech given text input. TTS models can be extended to have a single model that generates speech for multiple speakers and multiple languages.
MMS supports speech-to-text and text-to-speech for 1,107 languages and language identification for over 4,000 languages. Collecting audio data for thousands of languages was our first challenge because the largest existing speech datasets cover at most 100 languages. To overcome it, we turned to religious texts, such as the Bible, that have ...
1. Overview The Speech-to-Text API enables developers to convert audio to text in over 125 languages and variants, by applying powerful neural network models in an easy to use API.. In this tutorial, you will focus on using the Speech-to-Text API with Python. What you'll learn. How to set up your environment
Abstract. Recent progress in generative modeling has improved the naturalness of synthesized speech significantly. In this talk I will summarize these generative model-based approaches for speech synthesis and describe possible future directions.
The "latest" model tags in the Speech-to-Text API give access to two new model tags that can be used when you specify the model field. These models are designed to give you access to the latest speech technology and machine learning research from Google, and can provide higher accuracy for speech recognition over other available models.
Learn how to set up Google AI Speech-to-Text, build the video transcriber interface, develop the back end and connect to the AI, and then bring it all together. This course is designed for intermediate and advanced developers interested in the future of development with generative AI and the integration of applications with AI models.
Custom Voice Overview. Text-to-Speech now offers the Custom Voice feature. Custom Voice allows you to train a custom voice model using your own studio-quality audio recordings to create a unique voice. You can use your custom voice to synthesize audio using the Text-to-Speech API. Warning: Custom Voice is a private feature. The online ...
The main idea behind SpeechT5 is to pre-train a single model on a mixture of text-to-speech, speech-to-text, text-to-text, and speech-to-speech data. This way, the model learns from text and speech at the same time. The result of this pre-training approach is a model that has a unified space of hidden representations shared by both text and speech.
This repository implements a speech-to-speech cascaded pipeline with consecutive parts: Voice Activity Detection (VAD): silero VAD v5; Speech to Text (STT): Whisper checkpoints (including distilled versions) Language Model (LM): Any instruct model available on the Hugging Face Hub! 🤗; Text to Speech (TTS): Parler-TTS🤗
Cloud Computing Services | Google Cloud
Building upon this previous research, in this work we explore using TTS models along with LLM s to generate multi-speaker conversational data. We focus on two-speaker ASR on real-world telephone (Fisher []) and distant speech recognition settings (Mixer 6 Speech []) by fine-tuning Whisper [].The contributions of this work are the following: 1) We propose a synthetic data generation pipeline ...
Classes, methods and properties & attributes for Google Cloud Text-to-Speech API. classes. methods. properties and attributes. Send feedback Except as otherwise noted, the content of this page is licensed under the Creative Commons Attribution 4.0 License, and code samples are licensed under the Apache 2.0 License.