Thank you for visiting nature.com. You are using a browser version with limited support for CSS. To obtain the best experience, we recommend you use a more up to date browser (or turn off compatibility mode in Internet Explorer). In the meantime, to ensure continued support, we are displaying the site without styles and JavaScript.
- View all journals
- Explore content
- About the journal
- Publish with us
- Sign up for alerts
- Published: 17 June 2021
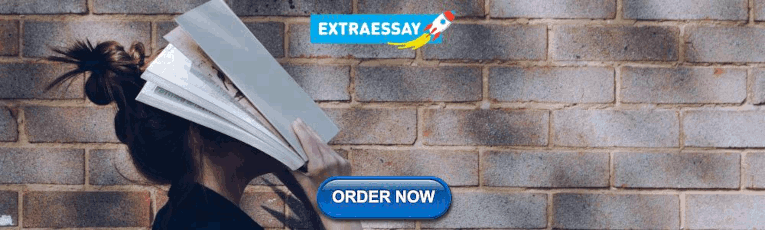
The impact of climate change on the productivity of conservation agriculture
- Yang Su ORCID: orcid.org/0000-0002-4717-9971 1 ,
- Benoit Gabrielle ORCID: orcid.org/0000-0002-9131-2549 1 &
- David Makowski ORCID: orcid.org/0000-0001-6385-3703 2
Nature Climate Change volume 11 , pages 628–633 ( 2021 ) Cite this article
5924 Accesses
36 Citations
55 Altmetric
Metrics details
- Agriculture
- Climate-change mitigation
Conservation agriculture (CA) is being promoted as a set of management practices that can sustain crop production while providing positive environmental benefits. However, its impact on crop productivity is hotly debated, and how this productivity will be affected by climate change remains uncertain. Here we compare the productivity of CA systems and their variants on the basis of no tillage versus conventional tillage systems for eight major crop species under current and future climate conditions using a probabilistic machine-learning approach at the global scale. We reveal large differences in the probability of yield gains with CA across crop types, agricultural management practices, climate zones and geographical regions. For most crops, CA performed better in continental, dry and temperate regions than in tropical ones. Under future climate conditions, the performance of CA is expected to mostly increase for maize over its tropical areas, improving the competitiveness of CA for this staple crop.
This is a preview of subscription content, access via your institution
Access options
Access Nature and 54 other Nature Portfolio journals
Get Nature+, our best-value online-access subscription
24,99 € / 30 days
cancel any time
Subscribe to this journal
Receive 12 print issues and online access
195,33 € per year
only 16,28 € per issue
Buy this article
- Purchase on Springer Link
- Instant access to full article PDF
Prices may be subject to local taxes which are calculated during checkout
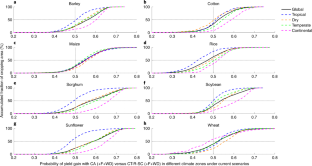
Similar content being viewed by others
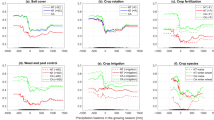
High probability of yield gain through conservation agriculture in dry regions for major staple crops
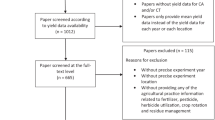
A global dataset for crop production under conventional tillage and no tillage systems
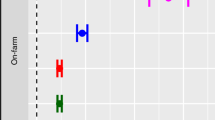
Conservation agriculture for sustainable intensification in South Asia
Data availability.
The dataset 10 is made fully available and described in a data paper 11 . The dataset can be accessed using the following link: https://doi.org/10.6084/m9.figshare.12155553 .
Code availability
All the R and MATLAB codes are available in figshare and can be accessed through the link https://doi.org/10.6084/m9.figshare.14427977 or on request from the corresponding author.
Bisht, J. K, Meena, V. S, Mishra, P. K. & Pattanayak, A. (eds) Conservation Agriculture: An Approach to Combat Climate Change in Indian Himalaya (Springer, 2016).
Farooq, M. & Siddique, K. H. M. (eds) Conservation Agriculture (Springer, 2015).
Giller, K. E. et al. Beyond conservation agriculture. Front. Plant Sci. 6 , 870 (2015).
Jat, S. L. et al. Differential response from nitrogen sources with and without residue management under conservation agriculture on crop yields, water-use and economics in maize-based rotations. Field Crops Res. 236 , 96–110 (2019).
Article Google Scholar
Steward, P. R. et al. The adaptive capacity of maize-based conservation agriculture systems to climate stress in tropical and subtropical environments: a meta-regression of yields. Agric. Ecosyst. Environ. 251 , 194–202 (2018).
Pittelkow, C. M. et al. When does no-till yield more? A global meta-analysis. Field Crops Res. 183 , 156–168 (2015).
Conservation Agriculture (FAO, 2020); http://www.fao.org/conservation-agriculture/en/
Pittelkow, C. M. et al. Productivity limits and potentials of the principles of conservation agriculture. Nature 517 , 365–368 (2015).
Article CAS Google Scholar
Nichols, V., Verhulst, N., Cox, R. & Govaerts, B. Weed dynamics and conservation agriculture principles: a review. Field Crops Res. 183 , 56–68 (2015).
Su, Y., Gabrielle, B. & Makowski, D. A global dataset for crop production under conventional tillage and no tillage practice. figshare https://doi.org/10.6084/m9.figshare.12155553 (2020).
Su, Y., Gabrielle, B. & Makowski, D. A global dataset for crop production under conventional tillage and no tillage systems. Sci. Data 8 , 33 (2020).
University of Delaware Air Temperature & Precipitation (NOAA/OAR/ESRL PSL, 2020); https://www.esrl.noaa.gov/psd/data/gridded/data.UDel_AirT_Precip.html
CPC Global Daily Temperature (NOAA/OAR/ESRL PSL, 2020); https://www.esrl.noaa.gov/psd/data/gridded/data.cpc.globaltemp.html
Martens, B. et al. GLEAM v3: satellite-based land evaporation and root-zone soil moisture. Geosci. Model Dev. 10 , 1903–1925 (2017).
Miralles, D. G. et al. Global land-surface evaporation estimated from satellite-based observations. Hydrol. Earth Syst. Sci. 15 , 453–469 (2011).
Crop Calendar Dataset: netCDF 5 degree (Univ. of Wisconsin-Madison, 2020); https://nelson.wisc.edu/sage/data-and-models/crop-calendar-dataset/netcdf0-5degree.php
Ho, T. K. Random decision forests. In Proc. 3rd International Conference on Document Analysis and Recognition 278–282 (IEEE, 1995).
Kottek, M., Grieser, J., Beck, C., Rudolf, B. & Rubel, F. World Map of the Köppen–Geiger climate classification updated. Meteorol. Z. 15 , 259–263 (2006).
Laborde, J. P., Wortmann, C. S., Blanco-Canqui, H., Baigorria, G. A. & Lindquist, J. L. Identifying the drivers and predicting the outcome of conservation agriculture globally. Agric. Syst. 177 , 102692 (2020).
Su, Z. et al. Effects of conservation tillage practices on winter wheat water-use efficiency and crop yield on the Loess Plateau, China. Agric. Water Manag. 87 , 307–314 (2007).
van Wie, J. B., Adam, J. C. & Ullman, J. L. Conservation tillage in dryland agriculture impacts watershed hydrology. J. Hydrol. 483 , 26–38 (2013).
Shukla, M. K., Lal, R. & Ebinger, M. Tillage effects on physical and hydrological properties of a typica argiaquoll in Central Ohio. Soil Sci. 168 , 802–811 (2003).
Shen, Y., McLaughlin, N., Zhang, X., Xu, M. & Liang, A. Effect of tillage and crop residue on soil temperature following planting for a Black soil in Northeast China. Sci. Rep. 8 , 4500 (2018).
Zuazo, V. H. D. & Pleguezuelo, C. R. R. Soil-erosion and runoff prevention by plant covers. A review. Agron. Sustain. Dev. 28 , 65–86 (2008).
Shaxson, F. & Barber, R. Optimizing Soil Moisture for Plant Production: The Significance of Soil Porosity (FAO, 2003).
Derpsch, R., Sidiras, N. & Roth, C. H. Results from studies made from 1977–1984 to control erosion by no-tillage techniques in Paraná, Brazil. Soil Tillage Res. 8 , 253–263 (1986).
TerAvest, D., Carpenter-Boggs, L., Thierfelder, C. & Reganold, J. P. Crop production and soil water management in conservation agriculture, no-till, and conventional tillage systems in Malawi. Agric. Ecosyst. Environ. 212 , 285–296 (2015).
Page, K. L., Dang, Y. P. & Dalal, R. C. The ability of conservation agriculture to conserve soil organic carbon and the subsequent impact on soil physical, chemical, and biological properties and yield. Front. Sust. Food Syst. 4 , 31 (2020).
Halvorson, A. D. et al. Spring wheat response to tillage and nitrogen fertilization in rotation with sunflower and winter wheat. Agron. J. 92 , 136–144 (2000).
Radford, B. J. & Thornton, C. M. Effects of 27 years of reduced tillage practices on soil properties and crop performance in the semi-arid subtropics of Australia. Int. J. Energy Environ. Econ. 19 , 565 (2011).
Google Scholar
García-Palacios, P., Alarcón, M. R., Tenorio, J. L. & Moreno, S. S. Ecological intensification of agriculture in drylands. J. Arid. Environ. 167 , 101–105 (2019).
Scopel, E. et al. Conservation agriculture cropping systems in temperate and tropical conditions, performances and impacts. A review. Agron. Sustain. Dev. 33 , 113–130 (2013).
Thierfelder, C. & Wall, P. C. Investigating conservation agriculture (CA) systems in Zambia and Zimbabwe to mitigate future effects of climate change. J. Crop Improve. 24 , 113–121 (2010).
Lal, R. The role of residues management in sustainable agricultural systems. J. Sustain. Agric. 5 , 51–78 (1995).
Thierfelder, C. et al. Complementary practices supporting conservation agriculture in southern Africa. A review. Agron. Sustain. Dev. 38 , 16 (2018).
Corbeels, M., Naudin, K., Whitbread, A. M., Kühne, R. & Letourmy, P. Limits of conservation agriculture to overcome low crop yields in sub-Saharan Africa. Nat. Food 1 , 447–454 (2020).
Smith, P., Powlson, D. S., Glendining, M. J. & Smith, J. U. Preliminary estimates of the potential for carbon mitigation in European soils through no‐till farming. Glob. Change Biol. 4 , 679–685 (1998).
Williams, A. et al. A regionally-adapted implementation of conservation agriculture delivers rapid improvements to soil properties associated with crop yield stability. Sci. Rep. 8 , 8467 (2018).
Kassam, A., Friedrich, T., Shaxson, F. & Pretty, J. The spread of conservation agriculture: justification, sustainability and uptake. Int. J. Agric. Sustain. 7 , 292–320 (2009).
Hobbs, P. R., Sayre, K. & Gupta, R. The role of conservation agriculture in sustainable agriculture. Phil. Trans. R. Soc. B 363 , 543–555 (2008).
Govaerts, B. et al. Conservation agriculture as a sustainable option for the central Mexican highlands. Soil Tillage Res. 103 , 222–230 (2009).
Su, Y., Gabrielle, B., Beillouin, D. & Makowski, D. High probability of yield gain through conservation agriculture in dry regions for major staple crops. Sci. Rep. 11 , 3344 (2021).
Soil Texture Map (Univ. of Tokyo, 2020); http://hydro.iis.u-tokyo.ac.jp/~sujan/research/gswp3/soil-texture-map.html
Breiman, L. Random Forests. Mach. Learn. 45 , 5–32 (2001).
Frieler, K. et al. Assessing the impacts of 1.5 °C global warming—simulation protocol of the Inter-Sectoral Impact Model Intercomparison Project (ISIMIP2b). Geosci. Model Dev. 10 , 4321–4345 (2017).
ESGF Project (Lawrence Livermore National Laboratory, 2020); https://esgf-node.llnl.gov/projects/esgf-llnl/
Portmann, F. T., Siebert, S. & Döll, P. MIRCA2000-Global monthly irrigated and rainfed crop areas around the year 2000: a new high-resolution data set for agricultural and hydrological modeling. Global Biogeochem. Cycles 24 , GB1011 (2010).
Download references
Acknowledgements
This work was supported by the ANR under the ‘Investissements d’avenir’ programme with the reference ANR-16-CONV-0003 (CLAND) and by the INRAE CIRAD meta-program ‘GloFoods’.
Author information
Authors and affiliations.
UMR ECOSYS, INRAE AgroParisTech, Université Paris-Saclay, Thiverval-Grignon, France
Yang Su & Benoit Gabrielle
UMR Applied Mathematics and Computer Science (MIA518), INRAE AgroParisTech, Université Paris-Saclay, Paris, France
- David Makowski
You can also search for this author in PubMed Google Scholar
Contributions
Y.S. wrote the main manuscript text and B.G. and D.M. modified it. Y.S., B.G. and D.M. worked together to prepare the figures and tables. Y.S. collected the data. Y.S., B.G. and D.M. designed and built the models to process the data. Y.S. worked on the model cross-validation. All authors reviewed the manuscripts.
Corresponding author
Correspondence to Yang Su .
Ethics declarations
Competing interests.
The authors declare no competing interests.
Additional information
Peer review information Nature Climate Change thanks Marc Corbeels, Krishna Naudin, Christian Thierfelder and the other, anonymous, reviewer(s) for their contribution to the peer review of this work.
Publisher’s note Springer Nature remains neutral with regard to jurisdictional claims in published maps and institutional affiliations.
Extended data
Extended data fig. 1 accumulated fraction of the cropping area as a function of the probability of yield gain under ca (+f+wd -irrigation) versus ct–r–sc (+f+wd -irrigation) systems in different climate regions..
Accumulated fraction of the cropping area as a function of the probability of yield gain under CA (+F+WD – Irrigation) versus CT–R–SC with fertilization (+F) and weed and pest control (+WD) without irrigation (–Irrigation) for eight major crops (a–h) and different climate zones. The results are based on the average climate conditions over 2021–2020 simulated by the Ipsl-cm5a-lr climate model and RCP 4.5 scenario.
Extended Data Fig. 2 Relative importance ranking of the model inputs.
The importance was defined by the mean decrease in accuracy in the ‘cforest’ model. Where ‘PB’ indicates precipitation balance over crop growing season; ‘Tmax’ indicates maximum air temperature over crop growing season;’Tave’ indicates average air temperature over crop growing season; ‘Tmin’ indicates minimum air temperature over crop growing season; ‘Crop’ indicates the crop species;’ST’ indicates soil texture; ‘SCNT’ indicates soil cover management under the variants of no tillage systems; ‘SCCT’ indicates soil cover management under CT systems; ‘RNT’ indicates crop rotation management under the variants of no tillage systems; ‘RCT’ indicates crop rotation management under CT systems; ‘FNT’ indicates management of crop fertilization under the variants of no tillage systems; ‘FCT’ indicates crop management of crop fertilization under CT systems; ‘WDNT’ indicates management of weed and pest control under the variants of no tillage systems; ‘WDCT’ indicates crop management of weed and pest control under CT systems.
Extended Data Fig. 3 The accumulated fraction of the cropping area in different level of change on the probability of yield gain under CA (+F+WD) versus CT–R–SC (+F+WD) under different crops, climate models and RCP scenarios.
The accumulated fraction of the cropping area in different level of change on the probability of yield gain under CA (+F+WD) versus CT–R–SC (+F+WD) for different crops, climate models and RCP scenarios. The results are based on the average climate data in different RCP scenarios (RCP 2.6, RCP 4.5, RCP 6.0, RCP 8.5) in Ipsl-cm5a-lr model, and RCP 4.5 scenario in different climate models (Ipsl-cm5a-lr, Gfdl-esm2m, Hadgem2-es, Miroc5) for both current (2021–2020) and future (2051–2060) scenarios.
Extended Data Fig. 4 Distributions of experiment site for each crop.
This map and the corresponding dataset are presented in ref. 11, 42. This figure was generated by MATLAB R2020a (Version 9.8.0.1451342, https://fr.mathworks.com/products/matlab.html ). In this meta-dataset (ref. 11 ), 4403 paired yield observations were extracted from NT and CT for 8 major crop species, including 370 observations for barley (232 observations for spring barley and 138 for winter barley), 94 observations for cotton, 1690 observations for maize, 195 observation for rice, 160 observations for sorghum, 583 observations for soybean, 61 observations for sunflower, 1250 observations for wheat (1041 observations for winter wheat and 209 observations for spring wheat) in 50 countries from 1980 to 2017.
Supplementary information
Supplementary information.
Supplementary Table 1, Figs. 1–8 and references.
Reporting Summary
Rights and permissions.
Reprints and permissions
About this article
Cite this article.
Su, Y., Gabrielle, B. & Makowski, D. The impact of climate change on the productivity of conservation agriculture. Nat. Clim. Chang. 11 , 628–633 (2021). https://doi.org/10.1038/s41558-021-01075-w
Download citation
Received : 21 August 2020
Accepted : 13 May 2021
Published : 17 June 2021
Issue Date : July 2021
DOI : https://doi.org/10.1038/s41558-021-01075-w
Share this article
Anyone you share the following link with will be able to read this content:
Sorry, a shareable link is not currently available for this article.
Provided by the Springer Nature SharedIt content-sharing initiative
This article is cited by
A 10-m annual grazing intensity dataset in 2015–2021 for the largest temperate meadow steppe in china.
- Chuchen Chang
- Yingjun Zhang
Scientific Data (2024)
Impact of climate change on the potential allocation of resources of rice cultivation in Yangtze-Huai Rivers region: a case study of Anhui Province, China
- Mingjie Chen
Theoretical and Applied Climatology (2024)
Synthesising results of meta-analyses to inform policy: a comparison of fast-track methods
- Rui Catarino
- Giovanni Tamburini
Environmental Evidence (2023)
Is Risk a Limit or an Opportunity to Mitigate Greenhouse Gas Emissions? The Case of fertilization in Agriculture
- Benjamin Dequiedt
- Marielle Brunette
- Emmanuel Servonnat
Environmental Modeling & Assessment (2023)
The effects of climate change and phenological variation on agricultural production and its risk pattern in the black soil area of northeast China
- Jiangbo Gao
- Shaohong Wu
Journal of Geographical Sciences (2023)
Quick links
- Explore articles by subject
- Guide to authors
- Editorial policies
Sign up for the Nature Briefing: Anthropocene newsletter — what matters in anthropocene research, free to your inbox weekly.

- Open access
- Published: 19 October 2018
Agricultural extension and its effects on farm productivity and income: insight from Northern Ghana
- Gideon Danso-Abbeam ORCID: orcid.org/0000-0002-7971-4676 1 ,
- Dennis Sedem Ehiakpor 1 &
- Robert Aidoo 2
Agriculture & Food Security volume 7 , Article number: 74 ( 2018 ) Cite this article
131k Accesses
124 Citations
3 Altmetric
Metrics details
In agricultural-dependent economies, extension programmes have been the main conduit for disseminating information on farm technologies, support rural adult learning and assist farmers in developing their farm technical and managerial skills. It is expected that extension programmes will help increase farm productivity, farm revenue, reduce poverty and minimize food insecurity. In this study, we estimate the effects of extension services on farm productivity and income with particular reference to agricultural extension services delivered by Association of Church-based Development NGOs (ACDEP).
The study used cross-sectional data collected from 200 farm households from two districts in the Northern region of Ghana. The robustness of the estimates was tested by the use of regression on covariates , regression on propensity scores and Heckman treatment effect model.
The study found positive economic gains from participating in the ACDEP agricultural extension programmes. Apart from the primary variable of interest (ACDEP agricultural extension programme), socio-economic, institutional and farm-specific variables were estimated to significantly affect farmers’ farm income depending on the estimation technique used.
Conclusions
The study has reaffirmed the critical role of extension programmes in enhancing farm productivity and household income. It is, therefore, recommended that agricultural extension service delivery should be boosted through timely recruitment, periodic training of agents and provision of adequate logistics.
The millennium development goals (MDGs) of reducing hunger and to promote food security are rooted in increasing agricultural productivity, especially from the crop sector. This is because agriculture is considered as the engine of growth in many developing economies, particularly in sub-Saharan Africa (SSA). The policy direction of growth and poverty reduction strategy (GPRS II) formulated by Ghana was to achieve accelerated and sustainable growth and poverty reduction through agricultural productivity. Although poverty had declined by 7.7% over the years (2005–2013), about 25% of Ghana’s population is still poor while under a tenth of the population are living in extreme poverty [ 1 ]. This suggests that the role of agriculture has not been sufficient to elevate many people above the poverty line, especially the rural folks who contribute immensely to agricultural production in Ghana. Ghana’s agricultural sector, which employs about 42% of the workforce, is dominated by smallholder farmers (about 90% farming on less than 2 hectares of land) who are using traditional production methods and farm inputs [ 2 ]. Asfaw et al. [ 3 ] argue that achieving productivity growth in the agricultural sector can only be successful through the development and dissemination of improved agricultural technologies to these smallholder farmers in the rural areas. Rural farmers farming on small hectares of land can be attributed to conditions such as lack of adequate credit, lack of access to product market, lack of adequate extension contacts, among others. Among these constraints, inadequate extension services have been identified as one of the main limiting factors to the growth of the agricultural sector and rural community development at large [ 3 ]. With recent threats of climate change and the rapid advancement in technology, more farmers require capital investment in agriculture and human capacity development to at least continue to make their living out of farming. Thus, the role of agricultural extension today goes beyond the transfer of technology and improvement in productivity, but also, it includes improvement in farmers’ managerial and technical skills through training, facilitation and coaching, among others.
Incognizant of these problems, the government of Ghana through the Ministry of Food and Agriculture (MoFA) over the years has invested so much in building the capacities of smallholder farmers through agricultural extension programmes. Other stakeholders in the agricultural sector such as international and local funding agencies, non-governmental organizations and financial institutions have and continue to make investments in the delivery of extension services to farmers. One of the prominent NGOs that have been implementing extension programmes in Northern Ghana is the Association of Church-based Development NGOs (ACDEP). ACDEP is a network of over 40, mostly, but not only church sponsored development NGOs in Northern Ghana with the vision of improving the economic well-being of its actors, particularly farmers through improved farm technology and access to input and output markets. The ACDEP NGOs are also engaged in other fields such as primary health care, HIV/AIDS, water and sanitation, rural enterprise development for women and value chains. One of the key components of ACDEP programmes in the field of agriculture is the delivery of agricultural extension services.
The ACDEP agricultural extension programmes include capacity building in good agricultural practices (GAPs), creating linkages among the value chain actors (input dealers, farmers, wholesalers and retailers) and other value addition techniques. Footnote 1 Thus, wider dissemination of information regarding farmer skill development, the use of improved farm technologies, general farm management practices and easy access to input and output markets have been the fundamental principles underlying delivery of ACDEP agricultural extension services. All these are geared towards improvement in productivity, reduction in poverty and enhancement in food security. Footnote 2 Given the scale of investment from ACDEP, the value for money regarding an increase in farm income is an important policy question. In this study, we hypothesized that participation in ACDEP agricultural extension programmes positively affects the welfare of the participating farm households through improvement in farm productivity and income. Many studies had dealt with issues relating to improving agricultural technologies such as improved crop varieties, adoption of fertilizer, etc. in Ghana [ 4 , 5 , 6 , 7 , 8 ]. Also, some other studies had focused on the impact of government extension programmes concerning the use of technology, adoption rates, farm productivity and efficiency, and farm output levels [ 9 ]. These studies had provided excellent information on factors shaping adoption and adoption intensities of farm technologies. However, rigorous studies on impact evaluation of agricultural extension services delivered by non-governmental organizations such as ACDEP on smallholders’ farm productivity and income remain very rare in the Ghanaian agricultural literature. Thus, there are many gaps with regard to what is known about the effects of extension services on the productivity and income of farmers—the body of empirical evidence does not match the scale of implementation, particularly in Ghana’s agricultural sector. Hence, the aim of the study is to estimate the effects of agricultural extension services with particular reference to ACDEP on the farm productivity and income of smallholder farmers in the ACDEP operational areas in the Northern region of Ghana.
The role of extension services in agriculture
Agricultural extension programmes have been one of the main conduits of addressing rural poverty and food insecurity. This is because, it has the means to transfer technology, support rural adult learning, assist farmers in problem-solving and getting farmers actively involved in the agricultural knowledge and information system [ 10 ]. Extension is defined by FAO [ 11 ] as; “systems that should facilitate the access of farmers, their organizations and other market actors to knowledge, information and technologies; facilitate their interaction with partners in research, education, agribusiness, and other relevant institutions; and assist them to develop their own technical, organizational and management skills and practices”. By this definition, an extension is deemed as a primary tool for making agriculture, its related activities as well as other economic activities more effective and efficient to meet the needs of the people. It is, therefore, regarded as a policy tool for promoting the safety and quality of agricultural products. Agricultural extension is aimed primarily at improving the knowledge of farmers for rural development; as such, it has been recognized as a critical component for technology transfer. Thus, agricultural extension is a major component to facilitate development since it plays a starring role in agricultural and rural development efforts [ 12 ].
Bonye et al. [ 12 ] argued that extension provides a source of information on new technologies for farming communities which when adopted can improve production, incomes and standards of living. Extension service providers make an innovation known to farm households, act as a catalyst to speed up adoption rate and also control change and attempt to prevent some individuals in the system from discontinuing the diffusion process [ 13 ]. In reaching farmers, extension officers demonstrate a technology to farmers but with much concentration on early adopters since the laggards would learn later from the early adopting farmers. Through extension services, farmers’ problems are identified for further investigation and policy direction. Swanson [ 14 ] argued that extension service goes beyond technology transfer to general community development through human and social capital development, improving skills and knowledge for production and processing, facilitating access to markets and trade, organizing farmers and producer groups, and working with farmers towards sustainable natural resource management. Where market failures such as limited access to credit and non-competitive market structures that provide a disincentive to farmers to produce exist, extension services tend to provide solutions.
The study area, data source and description of variables
The study analyses the effects of ACDEP extension programmes on farm productivity and income using a sample obtained from the farming communities in the Tolon and Kumbugu districts of the Northern region of Ghana. The two districts are predominantly rural communities with the majority being smallholder farm households. Tolon district has a population of 72,990 (males constitute 49.8% while females constitute 50.2%) of which 92.7% are engaged in agriculture, while Kumbugu has a population of 39,341 (equally distributed between males and females, i.e. 50%) of which 95.4% of the households are engaged in agriculture [ 15 ]. Crop farming is the main agricultural activities in the two districts with about 98% engaged in it [ 15 ]. The districts are characterized by a single rainy season, which starts in late April with little rainfall, rising to its peak in July–August and declining sharply after that and coming to a complete halt in October–November. The dry season starts from November to March with day temperatures ranging from 33 to 39 °C, while mean night temperature ranges from 20 to 26 °C. The mean annual rainfall ranges between 950 and 1200 mm.
The data were obtained mainly from primary sources, through the use of structured questionnaires. The study followed a multi-stage random sampling technique in selecting the two districts and communities from the Northern region and farm households from each community. In the first stage, the two districts were randomly selected from a number of ACDEP operational districts in the Northern region of Ghana. In the second stage, a random sampling was used to select four ACDEP operational communities from each district. Four non-ACDEP operational communities were selected from Tolon district, while three were selected from Kumbugu district. Thus, the survey covered fifteen communities, eight from Tolon and seven from Kumbugu. In the final stage, 10–15 maize farm households were randomly selected from each operational community, while 10–12 maize farm households were selected from each of the non-operational communities. The total sample size of the study is 200 maize farm households consisting of 110 farm households who had participated in ACDEP extension programmes and 90 maize farm households who did not take part in the programme. Key informants such as some heads of departments of ACDEP office in Tamale, Ghana and community leaders were approached to discuss challenges and opportunities relating to the programme and how the programme can aid in increasing productivity and farm income.
Table 1 shows the descriptive statistics of the sampled farm households. Two main categories of variables are described here: the dependent variables (outcome variables) and the explanatory variables.
Dependent variables
The dependent variables consist of maize farm productivity (yields), maize farm income per hectare, total household income and household income per capita. Farm productivity is defined as the total output of maize in kilograms per hectare. Footnote 3 The average yield of maize of the participating farm households is 1811 kg per hectare, while that of the non-participants is 1511 kg per hectare. Thus, there is no statistical difference between yields of participants and non-participants of the ACDEP agricultural extension programme. However, difference could partly be ascribed to the over-concentration of the programme on linking farmers to the output market with the adoption of farm technology lagging.
The income from maize (measured as the total sales per hectare) shows a difference of 20% in favour of the participants which is significant at 10% level of significance. One of the key components of the ACDEP agricultural extension programme is to link farmers to the output market, and so farmers who participated in the programme might have had their products sold at the right time compared with their counterparts who did not. As part of the programme, some of the participants were engaged in contract farming where their farm product was pre-negotiated. Hence, might be responsible for the significant difference in the farm income between the participants and non-participants. The total household income is the summation of the revenues from maize and other crops, revenues from the sales of livestock and income from non-farm economic activities such as wages, salaries and other self-employed businesses earned by members of the household (e.g. household head, spouse and other economically active members). The total household income per capita defines the total income of the households relative to their size. The total household income of the ACDEP participating households is GH¢ 13,710, while that of the non-participants is GH¢8239 showing a significant difference between the two groups. Footnote 4 Similarly, the total income per capita is GH¢2222 and GH¢788 for participants and non-participants, respectively. The large difference in household income is partly coming from the gap in the farm income, and probably the treated group were more engaged in other farming activities such as livestock rearing due to the knowledge they might have gained through the programme. The difference in household income per capita may emanate from the fact that non-participating households have a larger family size compared to the participating households. However, these descriptive statistics are limited regarding their implications for causality, as they fail to quantify and account for selectivity biases that may emanate from participation in the extension programmes.
Explanatory variables
Consistent with pieces of literature, the study hypothesized that participation in the ACDEP extension programme, as well as the determinants of farmers’ farm income, can be explained by socio-economic, farm-specific and institutional factors [ 9 , 15 , 16 , 17 ]. Some of the socio-economic factors include gender, age, household size, the number of years in formal education and the number of years in crop farming (experience). The farm-specific factors included in the empirical models of this study are the hectare of agricultural land allocated to maize cultivation (farm size) and the number of maize farm plots. The institutional and policy variables include membership of a farmer-based organization (FBO), distance to the local district market and distance to the main regional city market as well as access to agricultural credit.
Econometric technique
The study uses a combination of three econometric techniques to assess the effects of the ACDEP agricultural extension services on farm productivity and income of the smallholder maize farmers. First, we use a simple regression referred to as regression on covariates . Second, a regression on propensity scores was used to account for selectivity bias in estimating the effects of the extension services. In the third stage, we complement the results with the Heckman treatment effect model to test the robustness of the results.
We start by considering that the outcome variables (in our case maize farm yields, farm income per capita, total household income and total household income per capita) are a linear function. This linear function consists of a vector of households, farm-specific and institutional factors ( X i ). The simplest approach to assessing the effect of the ACDEP agricultural extension programme is to include in the outcome equation ACDEP agricultural extension participation variable ( AE i ) denoting one (1) if farmer participates in the ACDEP programme and zero (0) if otherwise, and then apply ordinary least squares (OLS) estimation technique. This can be specified as:
where Y i is the outcome variable, AE i is a dummy variable for ACDEP extension programme participation, X i represents other explanatory variables, α i and δ i are parameters to be estimated. The causal effect of AE i on the outcome variables can be measured by estimating the parameter α i . However, estimating Eq. ( 1 ) with OLS might lead to biased estimates because it assumes that AE i is random and exogenously given, while the selection of ACDEP participants is non-random and AE i variable is potentially endogenous [ 16 , 18 ]. The non-random sample selection problem arises from self-selection where the farmers themselves decide whether or not to participate in the extension programme, probably due to differences in resource endowments. Thus, the selection bias emanates from the fact that treated individuals may be systematically different from the non-treated for reasons other than the treatment status. The endogeneity problem may arise becuase the programme may target farmers with specific characteristics (smallholder farmers, commercial farmers, poor farmers or relatively wealthy farmers). These may result in biased estimates of the coefficient of AE i which measures the effects of extension programme participation on the outcome variables. Selection bias could also arise from the selection on observable or unobservable. Selection on observables could be controlled by including some set of variables in the model. However, selection on unobservables is usually difficult to control by adding variables. This is because unobserved variables such as farmers’ managerial ability, motivation, among others are not observed, hence, difficult to capture. Excluding these unobserved variables gives biased estimates of α i in Eq. ( 1 ). The study addresses the problem of selectivity bias in three ways.
The first is to include a set of observable covariates to account for potential selection bias due to selection on observables as applied in [ 13 ]. These variables include distance to local district market, distance to regional city market and farm size. We can then re-specify Eq. ( 1 ) as:
where Z i is a vector of variables to control for the selection bias and χ i is the parameter to be estimated. As stated earlier, this is called regression on covariates.
The second approach is to estimate the propensity score or conditional probability to participate in the ACDEP agricultural extension programme and use it as an additional control variable in the regression model. Footnote 5 This is referred to as regression on propensity scores as used in Alemu et al. [ 13 ] and Asres et al. [ 19 ]. The propensity score as a control variable in the regression model reduces the potential biases created by selection on observable characteristics [ 17 ]. The new regression model can be specified as:
where \(PS_{i} = p(AE_{i} = \frac{1}{X})\) and φ i is the estimate of the propensity score PS i . Other variables are defined earlier.
The third approach to deal with sample selection bias is the Heckman treatment effect model. The Heckman treatment effect model is one of the most widely used procedure to account for sample selection bias and offers a mean/way of correcting for biases that may arise from unobservable factors, and thus results in unbiased and consistent estimates [ 16 ]. The Heckman treatment effect model is an extension of the Heckman two-stage model. The only difference is that the dependent variable in the selection equation becomes one of the explanatory variables in the outcome equation of the former but not in the latter model. The principle behind the treatment effect model is to estimate the selection equation (usually a probit model) and use the predicted values of the dependent variable as a selection control factor called the inverse mills ratio (IMR). The IMR is then used as an additional regressor in the outcome equation to correct sample selection and free other explanatory variables from any biases. In this way, the true effects of participation in the ACDEP agricultural extension programme on outcome variables are measured [ 20 ]. Thus, in the treatment effect model, the treatment condition enters the outcome equations as an explanatory variable to measure the true effects on the outcome variables [ 18 ].
The model can be specified in two steps. The selection equation which is usually a probit is given as:
where AE i is a latent endogenous variable (participation in ACDEP agricultural extension programmes), X i is a set of exogenous variables determining the selection of farm households into the extension programme, δ i is a parameter to be estimated and ɛ i is the error term. The substantive equation can be specified as:
where α i measures the effect of ACDEP extension services on the outcome variables. To correct for self-selection biases in the substantive Eq. ( 5 ), an IMR denoted by the symbol λ was generated and added as an additional explanatory variable. The formulation process of IMR is given as:
where φ and Φ are normal probability density function and cumulative density function, respectively, of the standard normal distribution. Adding the IMR to Eq. ( 5 ) translates into Eq. ( 7 ) as:
where γ i is an estimate of the IMR ( λ i ) and μ i is a two-sided error term with \(N(0,\sigma_{v}^{2} ).\) The rest are as defined earlier. A significant coefficient of the IMR implies that there is self-selection problem, while a non-significant coefficient indicates the absence of sample selection. Ignoring the addition of the IMR will render the results from Eq. ( 5 ) as biased [ 21 ]. Thus, the inclusion of the selectivity term makes the coefficient α i (measuring the effects of the treatment variable on the outcome variables) unbiased, albeit it is inefficient as the disturbance term ( μ i ) is heteroscedastic [ 16 ]. The problem of heteroscedasticity can be corrected by the use of bootstrap standard errors or re-sampling. However, the STATA software package used in generating the estimates automatically adjusts for that bias in the standard errors [ 22 ].
Empirical results and discussions
Determinants of acdep agricultural extension programme participation.
The results from the probit model for the participation in ACDEP agricultural extension programme are presented in Table 2 . The model fits the data reasonably well as indicated by the Wald test that all the coefficients are jointly equal to zero is rejected [ Chi 2 (8) = 28.37 ; p = 0.000].
The probit model also correctly classified 69.37% of the maize farm households among the participants and 69.66% among the non-participants with a total accurate prediction rate of 69.50% for the entire sample.
From the table, the probability of participating in the ACDEP extension programmes is significantly influenced by the age of the household head, the number of years in crop farming, access to agricultural credit, membership of a farmer-based organization and the size of plots allocated to maize production (farm size). The positive and significant influence of age on the probability of participation in the ACDEP agricultural extension programme is against the notion that older farmers are usually reluctant to accept new information and ideas as reported by Asres et al. [ 19 ] and Genius et al. [ 23 ]. However, the result is consistent with the studies by Tiwari et al. [ 24 ] and Mendola [ 25 ]. Farmers with longer years in farming business have a higher likelihood of participating in the ACDEP programmes to optimize their farm productivity and income. Hence, the positive and significant effect of this variable is expected.
Similarly, access to agricultural credit will encourage farmers to participate in the extension programme to get more information that may help to maximize their yield to repay the credit on time. Group membership such as FBO enhances farmer-to-farmer extension services where knowledge and ideas on farm business and other off-farm activities are transferred from one farmer to the other. Thus, farmers who are members of FBOs are likely to get sufficient awareness and knowledge on farm technologies and, hence, are sensitized to join extension programme for more information on their farm business. Similarly, participants in the ACDEP agricultural extension programme tend to have larger farm sizes than their non-participants counterparts as supported by the descriptive statistics in Table 1 . Usually, members of such extension programmes are encouraged to consider their farm as a business entity rather than a cultural way of life and are, therefore, poised to achieve higher output through expansion and productivity. Gebreegziabher [ 26 ] reported a positive effect of plot size on the probability of participating in an extension programme in Ethiopia.
Effects of ACDEP extension programme on yield, farm income and household income
The econometric results of the effects of the extension programme on the four outcome variables (maize productivity, farm income per hectare, total household income and total household income per capita) are reported in Table 3 . These outcome variables are already defined in Table 1 . The following paragraphs discuss the effects of ACDEP agricultural extension programmes on each of the performance indicators across the three models.
Table 3 indicates that ACDEP agricultural extension programmes had positive effects on the productivity and farm income of the households in the study area. The results from different estimation approaches are quantitatively similar in terms of the direction, indicating the robustness of the results to changes in the estimation techniques. The estimated results show that the ACDEP extension programme had no significant effects on maize farm productivity when regression on covariates and regression on propensity scores were used. However, in the Heckman treatment effect model, the effect is positive and significant. Since all the variables are specified in logarithmic terms, it suggests that participating in the ACDEP extension programme has increased farm productivity by 11.3% points. The result from the Heckman model is at variance with the study of Feder et al. [ 27 ] who found no contribution of extension programmes to crop productivity. However, it is in line with other previous studies [ 28 , 29 , 30 , 31 ] reporting positive effects of extension programmes on crop farm productivity. The estimated effects for maize farm income per hectare vary from 0.113 to 1.13 depending on the estimation procedure. These coefficients imply that the extension programme has led to an increase in farm income by 11.3 to 111.3 percentage points. The effect is smaller in a regression on propensity score than the Heckman treatment effect model as indicated in Table 3 . The wide divergence in the magnitude of the effects may partly be attributed to the difference in the unobserved heterogeneity among the maize farm households. The regression on propensity score minimizes selection biases based on observed covariates, while Heckman treatment effects correct for selection bias arising from unobserved factors, hence, the two estimation techniques are most likely to produce different estimates. Moreover, given the market-oriented nature of the extension programmes nowadays, the large effect on farm income is not surprising. Thus, the focus is more on linking farmers to the market where products are purchased at the right time, rather than technology adoption.
For Heckman treatment effects, regression on propensity score and regression on covariate models, extension programmes lead to 85.3%, 23.3% and 36.1% increase in total household income, respectively. Gebrehiwot [ 9 ] observed that extension programmes lead to 7% and 10% points in farm household income when estimated with regression on covariates and propensity score matching using stratification technique, respectively. Similarly, Asres et al. [ 19 ] reported 6% and 18% increase in household income through participation in extension programme when estimated with OLS and Heckman treatment effects, respectively. The results also corroborate with that of [ 28 ] and [ 32 ]. The effects of ACDEP agricultural extension programmes on per capita income differ across the three models regarding the magnitudes of the effects. The extension programmes improve per capita income by 34.7% and 21.6% for regression on covariates and regression on the propensity scores, respectively. It increases by 110.4% in the Heckman treatment effect model. The greater impact of the ACDEP programme on the per capita income could emanate from the large effect of farm income coupled with the smaller household size of the participants compared with that of the non-participants as indicated in the descriptive statistics. Apart from participation in extension programme, some other factors have been estimated to affect farm households’ income. Table 4 presents the full results of the regression on covariates, regression on propensity scores and the second stage of the Heckman treatment model. The two regressions (regression on covariates and regression on propensity scores) are free from multicollinearity as indicated by the variance inflation factor (VIF) mean values of 1.39 and 2.65 for regression on covariates and regression on propensity scores, respectively. The VIF for each independent variable was less than the critical value of 10 indicating non-existence of multicollinearity [ 33 ]. The results are also free from heteroscedasticity as indicated by the small values of the Chi 2 generated by the Breusch–Pagan/Cook–Weisberg heteroscedasticity test.
The results indicate the significance of household locations and their effects on their farm income. The distance to market centres, both local and regional markets, is found to negatively affect their farm income. These findings may be attributed to the fact that ACDEP programmes are targeted at the remote districts and communities where poverty is pervasive. The other side is that farmers sometimes find it difficult in transporting their produce to the urban market centres as they are being constrained by finance and other factors such as road networks.
Other socio-economic factors such as the educational attainment (measured as the number of years in formal education), household size and farming experience (measured as the number of years in crop farming) significantly affect farm income with different estimation techniques. Similarly, institutional variables such as access to agricultural credit and social capital variable (FBO membership) are estimated to have positive and significant effects on farm income. The number of farm plots owned is positive and significant in the regression on propensity score but not significant in the regression on covariates and Heckman treatment effect model. Furthermore, the size of the plot allocated to maize production (farm size) had a positive and significant influence on farm income across the three estimation techniques. This underscores the importance of farm size to increasing farm income among smallholder rural farm households.
The study has assessed the effects of ACDEP agricultural extension programme on the productivity and income of farm households using primary data from two districts in the Northern region, Ghana. Since the agricultural extension programmes require a substantial amount of investment, understanding its effects on the beneficiaries (farmers) is very important. After controlling for selectivity bias, we found out that participation in the ACDEP agricultural extension programme improves welfare through an increase in farmers’ income. However, the effect levels are different depending on the empirical estimation procedure adopted. The central government and development partners should commit more human, financial and logistical resources to agricultural extension delivery in the country to boost agricultural productivity, farm incomes and total household income. Also, access to agricultural credit and formation of farmer groups such as farmer-based organizations should be promoted for agricultural extension service delivery to realize its full impact.
The study used “ACDEP extension programmes” and “ACDEP agricultural extension programmes” interchangeably.
Note: Information in this paragraph was sourced from ACDEP website ( http://acdep.org/site/index.php/home-7/14-acdep-home/24-about-acdep ) and its secretariat office in Tamale, Ghana.
In this study, farm productivity and farm yields are used interchangeably.
Average exchange in 2016: GH¢ = US$ 1.
Note: A probit model was used to generate the propensity scores used in Eq. ( 3 ).
Abbreviations
Association of Church-based Development NGOs
Food and Agriculture Organization
good agricultural practices
growth and poverty reduction strategy II
millennium development goals
ordinary least squares
sub-Saharan Africa
Ghana Statistical Service. Ghana living standards survey round 6 (GLSS6): poverty profile in Ghana (2005–2013). Ghana: Accra; 2014.
Google Scholar
Ghana Statistical Service. 2010 Population and housing census summary, report of final results. Ghana: Accra; 2012.
Asfaw S, Shiferaw B, Simtowe F, Lipper L. Impact of modern agricultural technologies on smallholder welfare: evidence from Tanzania and Ethiopia. Food Policy. 2012;37(3):283–95.
Article Google Scholar
Ehiakpor SD, Danso-Abbeam G, Zutah J, Hamdiyah A. Adoption of cocoa farm management practices in Prestea Huni-Valley District, Ghana. Russ J Agric Soc Sci. 2016;5(53):117–24.
Wiredu AN, Zeller M, Diagne A. What determines the adoption of fertilizers among rice-producing households in Northern Ghana? Q J Int Agric. 2015;54(3):263–83.
Danso-Abbeam G, Setsoafia ED, Ansah IGK. Modelling farmers investment in agrochemicals: the experience of smallholder cocoa farmers in Ghana. Res Appl Econ. 2014;6(4):12–27.
Bruce KKA, Donkoh SA, Ayamga M. Improved rice variety and its effect on farmers’ output in Ghana. J Dev Agric Econ. 2014;6(6):242–8.
Aneani F, Anchirinah VM, Owusu-Ansah F, Asamoah M. Adoption of some cocoa production technologies by cocoa farmers in Ghana. Sust Agric Res. 2012;1(1):103–17.
Gebrehiwot KG. The impact of agricultural extension on households’ welfare in Ethiopia. Int J Soc Econ. 2015;42(8):733–48.
Christoplos I, Kidd A. Guide for monitoring, evaluation and joint analyses of pluralistic extension support. Lindau: Neuchâtel Group; 2000.
Food and Agriculture Organization (FAO) of the United Nations. Ethiopia Country Brief; 2010. Retrieved from www.fao.org/countries/55528/en/eth/ .
Bonye SZ, Alfred KB, Jasaw GS. Promoting community-based extension agents as an alternative approach to formal agricultural extension service delivery in Northern Ghana. Asian J Agric Rural Dev. 2012;2(1):76–95.
Alemu AE, Maetens M, Deckers J, Bauer H, Mathijs E. Impact of supply chain coordination on honey farmers’ income in Tigray, Northern Ethiopia. Agric Food Econ. 2016;4:9.
Article CAS Google Scholar
Swanson BE. Global review of good agricultural extension and advisory service practices. Rome: Food and Agriculture Organization of the United Nations; 2008.
Ghana Statistical Service. 2010 Population and housing census, districts report. Ghana: Accra; 2014.
Greene WH. Econometric analysis. 5th ed. New Jersey: Prentice-Hall; 2003.
Imbens G. Non-parametric estimation of average treatment effects under exogeneity: a review. Rev Econ Stat. 2004;96(1):4–29.
Maddalla GS. Limited dependent and qualitative variables in econometrics. Cambridge: Cambridge University Press; 1983.
Book Google Scholar
Asres E, Makoto N, Kumi Y, Akira I. Effect of agricultural extension program on smallholders’ farm productivity: evidence from three peasant associations in the highlands of Ethiopia. J Agric Sci. 2013;5(8):163–81.
Smith J, Todd P. Does matching overcome Lalonde’s critique of non-experimental estimators? J Econ. 2005;125:305–53.
Heckman J. Sample selection bias as specification error. Econometrica. 1979;47:153–61.
Bushway S, Johnson BD, Slocum LA. Is the magic still there? The use of the Heckman two-step correction for selection bias in criminology. J Quant Criminol. 2007. https://doi.org/10.1007/s10940-007-9024-4 .
Genius MG, Pantzios CJ, Tzouvelekas V. Information acquisition and adoption of organic farming practices. J Agric Res Econ. 2006;31(1):93–113.
Tiwari KR, Sitaula BK, Nyborg LP, Paudel GS. Determinants of Farmers’ adoption of improved soil conservation technology in a middle mountain watershed of Central Nepal. Environ Manag. 2008;42(2):210–22.
Mendola M. Agricultural technology adoption and poverty reduction: a propensity score matching analysis for rural Bangladesh. Food Policy. 2007;32(33):372–93.
Gebregziabher G, Holden S. Does irrigation enhance and food deficits discourage fertilizer adoption in a risky environment: evidence from Tigray, Ethiopia. J Dev Agric Econ. 2011;30(10):514–28.
Feder G, Murgai R, Quizon JB. Sending farmers back to school: the impact of FFS in Indonesia. Rev Agric Econ. 2004;26(1):45–62.
Davis K, Nkonya E, Kato E, Mekonen DA, Odendo M, Miro R, Nkuba J. Impact of farmer field schools on agricultural productivity and poverty in East Africa. World Dev. 2012;40(2):402–13.
McGarry D. A synopsis of the measured outcomes of farmer field schools in integrated pest management for cabbage production, Timor Leste (2003–2005). FAO Brief, January 24, 2008.
Meti SK. Farmers field school strategies for effective diffusion of IPM technology for sustainable cotton yield: a critical analysis. Paper presented at the 23rd annual conference of the association for international agricultural and extension education, Polson, Montana, 20–24 May; 2007.
Geer T, Debipersaud R, Ramlall H, Settle W, Chakalall B, Joshi R. Introduction of aquaculture and other integrated pest management practices to rice farmers in Guyana and Suriname. FAN FAO Aquaculture Newsletter No. 35; 2006.
Kelemework, F. Impact evaluation of farmer field school: the case of integrated potato late blight management in the Central Highland of Ethiopia. University of Antwerp (Thesis); 2005.
Gujarati DN, Porter DC. Basic econometrics. 5th ed. Irwin: McGraw-Hill; 2009.
Download references
Authors’ contribution
GD-A designed the study and analysed the data. DSE collected the data and wrote the manuscript. RA revised the manuscript and conducted some additional analysis. All authors read and approved the final manuscript.
Acknowledgements
The authors are very grateful to the enumerators who collected and assisted in the data entry and all farmers who participated in the survey. We are also grateful to the anonymous reviewers for their useful comments.
Competing interests
The authors declare that they have no competing interests.
Availability of supporting data
The data set for this study is available from the corresponding author on request.
Consent for publication
All authors read, revised the earlier draft and approved the final manuscript.
Ethical approval and consent to participate
The authors obtained informed consent from each participant of the survey. Before starting the interview, all interviewees were informed about the context of the study and anonymous nature of the survey. Permission was sought from each respondent and they openly and freely answered the questions asked.
This study had no external funding. It was fully funded by the authors.
Publisher’s Note
Springer Nature remains neutral with regard to jurisdictional claims in published maps and institutional affiliations.
Author information
Authors and affiliations.
Department of Agricultural and Resource Economics, University for Development Studies, Tamale, Ghana
Gideon Danso-Abbeam & Dennis Sedem Ehiakpor
Department of Agricultural Economics, Agribusiness and Extension, Kwame Nkrumah University of Science and Technology, Kumasi, Ghana
Robert Aidoo
You can also search for this author in PubMed Google Scholar
Corresponding author
Correspondence to Gideon Danso-Abbeam .
Rights and permissions
Open Access This article is distributed under the terms of the Creative Commons Attribution 4.0 International License ( http://creativecommons.org/licenses/by/4.0/ ), which permits unrestricted use, distribution, and reproduction in any medium, provided you give appropriate credit to the original author(s) and the source, provide a link to the Creative Commons license, and indicate if changes were made. The Creative Commons Public Domain Dedication waiver ( http://creativecommons.org/publicdomain/zero/1.0/ ) applies to the data made available in this article, unless otherwise stated.
Reprints and permissions
About this article
Cite this article.
Danso-Abbeam, G., Ehiakpor, D.S. & Aidoo, R. Agricultural extension and its effects on farm productivity and income: insight from Northern Ghana. Agric & Food Secur 7 , 74 (2018). https://doi.org/10.1186/s40066-018-0225-x
Download citation
Received : 21 April 2017
Accepted : 09 October 2018
Published : 19 October 2018
DOI : https://doi.org/10.1186/s40066-018-0225-x
Share this article
Anyone you share the following link with will be able to read this content:
Sorry, a shareable link is not currently available for this article.
Provided by the Springer Nature SharedIt content-sharing initiative
- Agricultural extension
- Heckman treatment effect
- Productivity
- Regression on propensity scores
Agriculture & Food Security
ISSN: 2048-7010
- Submission enquiries: [email protected]
- General enquiries: [email protected]
- Systematic Map Protocol
- Open access
- Published: 29 October 2018
Evidence for the impacts of agroforestry on agricultural productivity, ecosystem services, and human well-being in high-income countries: a systematic map protocol
- Sarah E. Brown 1 ,
- Daniel C. Miller 1 ,
- Pablo J. Ordonez 2 &
- Kathy Baylis 2
Environmental Evidence volume 7 , Article number: 24 ( 2018 ) Cite this article
62k Accesses
70 Citations
9 Altmetric
Metrics details
A Systematic Map to this article was published on 17 March 2022
Agroforestry bridges the gap that often separates agriculture and forestry by building integrated systems that address both environmental and socio-economic objectives. Agroforestry can improve the resiliency of agricultural systems and mitigate the impacts of climate change. Existing research suggests that integrating trees on farms can prevent environmental degradation, improve agricultural productivity, increase carbon sequestration, generate cleaner water, and support healthy soil and healthy ecosystems while providing stable incomes and other benefits to human welfare. Although these claims are becoming more widely accepted as the body of agroforestry research increases, systematic understanding of the evidence supporting them remains lacking for high-income countries. This systematic map will address this research need by providing a tool for identifying and visualizing the existing evidence demonstrating the impacts of agroforestry practices and interventions on agricultural productivity, ecosystem services, and human well-being. The results will be useful for informing policy decisions and future research by making the evidence easily accessible and highlighting the gaps in knowledge as well as areas with enough evidence to conduct systematic reviews.
This systematic map will identify, collect, display, and describe available evidence on the impacts of agroforestry on agricultural productivity, ecosystem services, and human well-being in high-income countries. The search strategy will cover 5 primary databases and 24 organizational websites using a pre-defined search string designed to capture studies relating agroforestry practices and interventions to outcomes in high-income countries. The searches will all be conducted in English. We will screen the identified studies for inclusion or exclusion in stages, first on title and abstract and then on full-text. We will collect data from studies included at the full-text stage to form the map and associated database. For inclusion, the study in question must assess the impacts of the deliberate promotion and/or actual integration of woody perennials (trees, shrubs, palms, bamboos, etc.) on the same land management unit as agricultural crops and/or animals.
Agroforestry has risen to prominence as a land-use strategy to help address global climate change and provide other environmental, economic, and social benefits [ 1 , 2 , 3 , 4 , 5 , 6 ]. However, systematic knowledge on the human–environment impacts of agroforestry practices and interventions remains lacking. Agroforestry is promoted for its potential for carbon sequestration, soil erosion and runoff control, and improved nutrient and water cycling, as well as for offering socio-economic benefits and greater agricultural productivity [ 3 , 4 , 5 , 6 , 7 , 8 , 9 , 10 , 11 ]. While researchers and policy makers have long studied and supported agroforestry practices in low- and middle-income countries (L&MICs), particularly in tropical regions, recognition and promotion of agroforestry in the temperate climates typical of developed countries gained steam only more recently [ 2 , 12 ]. As the conversation discussing the potential and future for agroforestry continues to evolve, we note an increased study of and policy support for agroforestry in high-income countries (HICs) [ 2 , 8 , 13 , 14 ]. Evidence of the socio-economic and biophysical impacts of various agroforestry interventions and practices in HICs spans many disciplines and addresses a broad range of outcomes, thus creating an opportunity and need to synthesize the evidence for easier exchange of knowledge and ideas.
This study therefore aims to assemble the research showing the impacts of agroforestry practices and interventions in HICs to provide an evidence map of the literature to aid researchers and policy-makers in developing strategies for future research initiatives and policy formation. This systematic map (SM) directly parallels an evidence gap map (EGM) of the impacts of agroforestry in L&MICs that is currently in progress by members of same research group [ 15 ]. This protocol draws heavily from the L&MIC EGM protocol since the methods are intentionally aligned. Although these two maps are intended to directly parallel one another, we acknowledge that there are differences in the types of agroforestry practiced and studied between HICs and L&MICs. These differences may in part be explained by greater wealth and resources associated with the socio-political histories in HICs than in L&MICs as well as different types of climates, with HICs being predominantly in temperate climates and L&MICs being predominantly in tropical climates. Furthermore, we note that the L&MIC EGM has a stronger emphasis on agroforestry interventions since it was conducted through the International Initiative for Impact Evaluation (3ie), which focuses more on synthesizing evidence on the impacts of interventions. This SM intends to capture studies on the impacts of both agroforestry interventions as well as agroforestry practices in general, without placing emphasis on one or the other.
Simply defined, agroforestry is the intentional integration of woody vegetation, such as trees and shrubs, with crops and/or livestock simultaneously or sequentially on a land management unit. This integration is intended to diversify production systems to create environmental, economic, and social benefits through complementary interactions between the system components [ 16 , 17 , 18 ]. The general types of agroforestry include agrisilviculture (also called silvoarable, defined as trees integrated with cropping systems), silvopasture (trees integrated with livestock systems), agrosilvipasture (trees integrated with both crops and livestock as a system), forest farming (crop or livestock production within a forested area), urban agroforestry (often referred to as homegardens, defined as integrating trees with crops near the homestead), and other types, such as integrating trees in fisheries or beekeeping operations [ 18 ]. Common agroforestry practices are identified and presented in Table 1 . We note that these practices are meant to be mutually exclusive (i.e., an agrosilvopasture practice would not also be classified as an agrisilviculture practice and a silvopasture practice).
We further define several types of interventions that may be used to promote any one or more of these agroforestry practices. Agroforestry intervention types are described in Table 2 , and they represent types of support policy-makers could provide to promote adoption of one or more of the agroforestry practices described in Table 1 .
This systematic map will denote whether a study is an impact evaluation of an agroforestry-related intervention or is an evaluation of the impact only of an agroforestry practice.
Agroforestry research began with the study of the existing traditional practices of local populations, which formed the basis for conducting more rigorous experimental research [ 22 ]. As agroforestry research developed, researchers found a high potential for agroforestry to address many current environmental and social concerns, such as climate change and food security [ 22 ]. From this knowledge base, agroforestry advocates began pushing for the establishment of policies and programs to support the integration of trees on agricultural lands.
Broadly speaking, however, governmental policies for landowners have often lacked incentives to take up agroforestry practices [ 23 ]. Historically, there was the assumption that land must remain segregated between agriculture and other uses to optimize planning and productive efficiency (as opposed to establishing integrative land management techniques), which has limited the development of agroforestry [ 23 ]. To support the progress of industrial agriculture, governments designed national policies to promote specialization and intensification, which works to enforce this strategy of separation [ 24 ]. Industrial agriculture, however, is now associated with many negative social and environmental consequences [ 25 , 26 , 27 , 28 ]. Agroforestry has the potential to help address these consequences, and thus individuals familiar with agroforestry have started proposing and implementing a range of education and extension programs, financial incentives and cost-sharing initiatives, and support for the creation of markets for non-timber forest products to facilitate agroforestry adoption [ 16 , 21 , 29 , 30 ]. Such interventions have the potential to provide the incentives and support necessary to establish agroforestry as a thriving alternative land use strategy, by way of the following conceptual framework.
Figure 1 , developed and presented previously in [ 15 ], illustrates a generic theory of change which may underlie an effective agroforestry intervention. It identifies two initial preconditions: (1) successful mobilization and engagement of farmers; and (2) facilitating farmer capacity development and/or access to appropriate tree germplasm. The first of these and, in many cases, both, are required for significant and effective adoption of promoted agroforestry practices. Following such adoption, several intermediary outcomes are then expected. For example, farmers may see improved soil health and other ecosystem services, such as water infiltration, which then increase crop productivity or reduce production costs and, therefore, increase returns. Some participants may find that increased use and availability of tree/shrub fodder leads to increases in milk production and returns. Selling other agroforestry products such as timber, firewood, and fruit, can increase and diversify income and food sources [ 3 , 31 , 32 ]. These changes may have differential effects depending on gender, socio-economic status, race/ethnicity, or education/literacy level. Together, these intermediate outcomes are expected to bolster resilience to shocks, as well as boost overall household income and food security. These positive benefits along with features of the broader context in which participants operate will shape household investment in agroforestry. Our theory of change diagram presents positive pathways linking agroforestry interventions, adoption, and beneficial impacts; however, we also note that there are potentially negative tradeoffs to agroforestry, such as a reduction in area of crop production and negative tree-crop interactions.
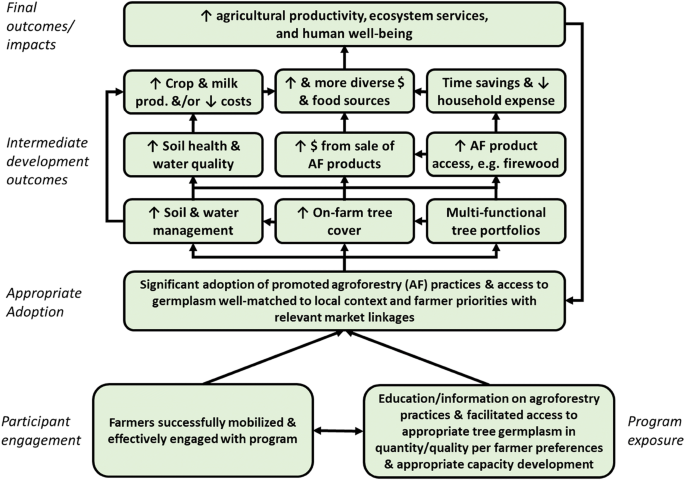
Illustrative theory of change for an agroforestry (AF) intervention, as adapted from [ 15 ]
By mapping the existing evidence of agroforestry practices in high-income countries with their impacts on agricultural productivity, ecosystem services, and human well-being, we will create an easily-navigable database of relevant research related to agroforestry impacts as well as form a clearer picture on key areas of interest for further research. The results will encompass research from all high-income countries, which will allow policymakers to utilize knowledge gained from around the globe as well as make the study relevant to all developed nations.
Why this systematic map is important to do for high income countries
A large body of evidence around agroforestry has accumulated over the past three decades through research across the high-income countries (HICs) of the world [ 5 , 6 , 7 , 33 ]. These HICs are listed in Additional file 1 , as defined by the World Bank for the 2018 fiscal year [ 34 ]. Figure 2 provides a map showing the global HICs.
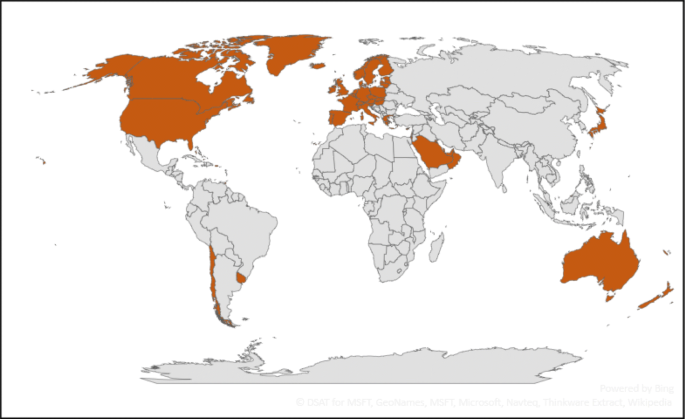
Geographic map showing high-income countries (HICs), as defined by [ 34 ]
To date, however, there has not been a comprehensive synthesis of evidence of what agroforestry practices and interventions have been effective, under what circumstances, and by what measures in HIC contexts. Recent literature reviews have given overviews of the evidence for the impacts of agroforestry on ecosystem services and environmental benefits, climate change adaptation and mitigation, carbon sequestration, biomass production, soil health, and food production [ 5 , 9 , 10 , 11 , 35 , 36 ]; however, they did not follow systematic review protocols. There are several recent efforts to systematically map and review aspects of agroforestry. Notably, one group mapped the evidence on agroforestry impacts on biodiversity and ecosystem services across Europe [ 7 , 37 ]. Other systematic reviews include aspects of agroforestry, such as a systematic map on the impacts of vegetated strips—including windbreaks, hedgerows, and shelterbelts—on nutrients, pollutants, socioeconomics, biodiversity, and soil retention in boreo-temperate systems [ 38 ]. Another study maps the impacts of Ecological Focus Area options (including agroforestry) in European farmed landscapes on climate regulation and pollination services [ 39 ]. Finally, we note that a systematic map of the effects of nature conservation on human well-being [ 40 ] and one on forests and poverty globally [ 41 ] include some studies on the impacts of agroforestry. We are not aware, however, of any effort to systematically map evidence on the impacts of agroforestry interventions and practices on the broad range of agricultural productivity, ecosystem services, and human well-being outcomes across HICs. Lack of such evidence synthesis constrains the ability of policymakers, practitioners, and researchers to make effective decisions relating to agroforestry.
Though it is easy to find examples of agroforestry practiced throughout the world, the initiatives to create policies and programs that formalize and promote agroforestry are relatively new in most HICs. International groups have invested in agroforestry projects in low- and middle-income countries (L&MICs) for decades (emerging in the 1960s and 1970s) as a solution to address environmental degradation, boost food security, and contribute to a range of other development policy objectives [ 3 , 42 ]. By contrast, agroforestry policy in the US, for instance, was first introduced in the mid-1980s (though promotion of windbreaks to reduce soil-erosion during the 1930s Dust Bowl era may be considered a precursor), with more formalized agroforestry policy emerging only in the 1990s with the Forest Stewardship Act of 1990 establishing a Center for Semiarid Agroforestry (renamed the National Agroforestry Center in 1994, broadening its scope to include the entire country). Similarly in the EU, agroforestry promotion began in the early 1990s with the 1992 reform of the EU Common Agricultural Policy (CAP), which formerly encouraged practices that discouraged farmers from integrating trees on farms [ 43 ]. Only within the last decade has there been a significant uptake of agroforestry projects in HICs in the context of institutionalized support for agroforestry as an alternative land use approach to address conservation and sustainable agricultural development objectives [ 2 ].
Major agroforestry initiatives in high-income countries include the USDA Agroforestry Strategic Framework Plan (FY 2011–2016) in the United States [ 16 ], the European Commission’s AGFORWARD program in Europe (FY 2014–2017) [ 21 ], Agriculture and Agri-Food Canada’s Agroforestry Development Centre in Canada [ 44 ], and the Farm Forestry National Action Statement and the Master TreeGrower Program (supported by the Australian Agroforestry Foundation) in Australia [ 45 ]. In Japan, the Satoyama Initiative includes agroforestry concepts, though it covers a broad range of practices [ 46 ].
One of the initial goals set out by these projects is to map out trees on farms and existing agroforestry practices within their respective countries. Several countries produced formalized documentation of the existing extent of agroforestry in their respective regions, such as the USDA in the United States [ 20 ] and the AGFORWARD project in Europe [ 47 ]. The USDA report, however, is limited to practices established with USDA technical and financial aid and a comprehensive mapping is yet to be completed and released. This SM will add to the toolset of resources supporting these initiatives by compiling existing knowledge of agroforestry impacts, identifying research needs, and making evidence accessible and customizable for diverse audiences. Furthermore, the SM will highlight any existing studies evaluating the impacts of these projects or any other agroforestry-related programs and policies in HICs.
There is evidence showing that agroforestry offers many ecological benefits—environmental, economic, and social—which give reason to incentivize and empower landowners to adopt such practices; however, it is also important to consider the evidence of the tradeoffs associated with agroforestry [ 4 , 5 , 6 , 7 , 8 , 9 , 10 , 11 , 23 , 48 ]. There is a growing interest in the potential of agroforestry and an increasing awareness of the role agroforestry can play in creating a diversified, multi-dimensional farming system [ 2 , 14 , 36 , 49 ]. Nevertheless, viewed in broader perspective, the integration of agroforestry into practice is still relatively low. For instance, the USDA estimates that agroforestry is applied on less than 1% of agricultural land with the potential for agroforestry through USDA assisted programs [ 20 ]. This SM will therefore provide important evidence synthesis that may support initiatives to disseminate agroforestry knowledge and promote broader adoption of agroforestry as an alternative land use strategy across different HIC contexts. Additionally, it will help to find evidence of potential tradeoffs that come with the establishment of agroforestry practices.
There are two primary audiences for this SM. First, we expect that researchers on agroforestry and broader sustainability issues will use the results to inform further investigations on these topics, including new empirical research, as well as systematic reviews of specific linkages and further evidence synthesis. Results should be of wide interest to researchers in a range of institutions, particularly national programs (USDA, AGFOWARD, etc.), national and regional agroforestry associations and extension programs, and universities. The second main anticipated audience is decision-makers for whom agroforestry is already or potentially of interest. This includes relevant government ministries and agencies, non-governmental organizations (NGOs), and other advocacy and implementing organization staff.
Stakeholder engagement
In developing the parallel L&MIC EGM, our team engaged with an advisory group comprised of 3ie members, donor agency staff, International Development Coordinating Group (IDCG) members and other evidence synthesis experts, International Centre for Research in Agroforestry (ICRAF) scientists and other agroforestry subject experts. We published the L&MIC EGM protocol with the Campbell Collaboration [ 15 ]. In preparing that protocol, we coordinated with the advisory group as well as colleagues involved in two related evidence maps [ 40 , 50 ], and we presented the work at several conferences with opportunities for discussion, see [ 15 ]. The HIC SM protocol was presented as a poster presentation at the Green Lands Blue Waters conference in Madison, Wisconsin in November 2017 and discussed with interested agroforestry experts. Feedback and suggestions given to the authors were incorporated into the HIC and L&MIC protocols. Finally, we expect to engage with additional reviewers through our efforts to publish this protocol and the resultant SM in peer-reviewed journals.
Objective of the map
The primary aim of this systematic map is to identify, map and describe existing evidence on the effects of agroforestry practices and interventions on agricultural productivity, ecosystem services, and human well-being in HICs.
In doing so, it addresses the following research questions:
What are the extents and characteristics of empirical evidence on the effects of agroforestry practices and interventions on agricultural productivity, ecosystem services, and human well-being in HICs?
What are the major gaps in the primary evidence base?
What are the agroforestry practice/intervention and outcome areas with potential for evidence synthesis?
To address these research questions, the scope is defined by the Population (Subject), Intervention (or Practice), Comparator, and Outcome (PICO) components to be examined, which are presented in Table 3 .
The methods for the searches, screening, and eligibility criteria replicates those used for the L&MIC EGM [ 15 ], with modifications to adapt the process to account for differences between HIC and L&MIC concepts of agroforestry.
Search strategy
We will undertake a comprehensive search across 5 bibliographic databases and 24 organizational websites for grey literature to best capture an unbiased representation of existing literature. Studies from January 1, 1990 to the time of the search (mid-2018) will be included in the search. We begin the study period in 1990 as this is roughly the time that HICs saw increased support for agroforestry and other approaches designed to further environmental goals, as discussed earlier. The search will be done through use of search engines, based on key words within the identified databases. When such a strategy is not possible (e.g. for some topical databases and organizational websites), hand searches will be performed to extract all potentially relevant studies. Due to resource constraints, the focus will be on studies published in English, which we acknowledge as a limitation of the comprehensiveness of this study.
The bibliographic databases that will be searched for publications are:
EBSCO: Agricola, Econlit
Web of Science: Core Collection
CAB Abstracts and Global Health
The search terms to be used in full to conduct a topic search in the Web of Science: Core Collection and CAB Abstracts and Global Health databases are presented in Table 4 . The search string includes each of the agroforestry practices from Table 1 . We note that the intervention types are more generic, including topics well beyond agroforestry. Our search terms will therefore focus on practices, but in doing this, we capture the range of relevant interventions studied as well. We include relevant study area terms (study country terms) to limit the number of search results returned, and this decision did not affect our study retrieve performance, as detailed in the section on Assessing Retrieval Performance below. We make use of the Boolean operators and wildcards where possible, as shown in Table 4 and described in further detail in Additional file 2 . Search strings are simplified for databases that have limited characters or lack Boolean functionality. The search strings used for each of the databases are detailed in Additional file 2 .
Additionally, to identify the existing grey literature, the websites of various organizations that are likely to produce published and unpublished research will be searched, using the search terms from We include relevant study area terms (study country terms) to limit the number of search results returned, and this decision did not affect our study retrieve performance, as detailed in the section on Assessing Retrieval Performance below. We make use of the Boolean operators and wildcards where possible, as shown in Table 4 and described in further detail in Additional file 2 . Search strings are simplified for databases that have limited characters or lack Boolean functionality. The search strings used for each of the databases are detailed in Additional file 2 .
The list of relevant research organizations (Table 5 ) has been constructed from cross-validation of websites listed in the systematic mapping protocols of agroforestry related studies [ 41 , 51 ]. To optimize the scope of the search while ensuring transparency in our methods, we will follow the approach developed by Haddaway et al. [ 52 ], which will allow us to search multiple websites simultaneously and to extract the relevant information from each website into a single database.
A search of literature through web-based search engines will also be performed. A search in Google Scholar, using the search terms from Table 4 will be performed and the first 300 results sorted by relevance will be reviewed, following the findings from Haddaway et al. [ 53 ]. The search string for Google Scholar will be simplified to not include the list of high-income countries (relevant study locations). The online literature review and reference management software, EPPI-Reviewer 4, will be used to upload relevant titles and abstracts for candidate studies identified through the search strategy. We will create a project workspace using Box ( https://www.box.com/home ; accessed 2 September 2018) to assist in organizing and managing documentation files as well as a project workspace using Slack ( https://slack.com/ ; accessed 2 September 2018; [ 54 ]) to manage communication on decisions, changes, and questions and provide a platform for all team members to have access to all relevant documents.
Assessing retrieval performance
The comprehensiveness of the search string was evaluated based on a test list of studies that meet the eligibility criteria. This test list consists of 44 studies, of which 22 are impact studies (18 primary studies, 4 systematic meta-analyses, and 1 farmer-managed field trial) and 22 researcher-managed field trials (which would not be included in this systematic map, per above, but which we would like to identify in the screening process for future work). The list was created based on personal knowledge and a snowballing method reviewing bibliographies and citations of known agroforestry papers. The test list was formed independently, without using the search string. The search string was tested and modified as needed by running it against this test list. The test list and results of the scoping process are reported in Additional file 2 . The search string retrieved 43 of the 44 test studies (97.7% of the studies in the test list), which was deemed acceptable.
We designed our search string to balance between specificity (proportion of relevant information) and sensitivity (finding all relevant information), per [ 55 ]. Our decision to include the country search terms is to avoid duplication of efforts from the parallel L&MIC EGM study and reduce the number of studies retrieved by our search to make the study feasible. We reviewed a sample of 500 studies omitted and found that the decision to include country terms omits primarily studies relating to L&MICs along with studies not relevant to our SM. Of the 500 omitted studies reviewed, we only found two relevant for inclusion (0.4%). Limiting the search by country terms reduces returned results from 92,293 to 30,014 (see Additional file 2 ). Furthermore, our retrieval rate of our test list studies does not change when the countries are removed from the search string (the one study that was missed did not contain any agroforestry-related terms that could be added to the search string). The topic search in Web of Science searches for the search terms in the title, abstract, keywords, and Web of Science KeyWords Plus. We found that with this type of topic search, a country term is almost always picked up, returning a sufficient percentage of the body of HIC agroforestry literature. Our assessment of study retrieval performance gives us confidence that we are capturing the majority of literature while not extending the scope of our study beyond feasibility.
Article screening and study eligibility criteria
We will first review search results at the level of title and abstract to determine inclusion or exclusion. Any study that we are unsure of whether it should be included or not during the title and abstract stage will be included for full-text review. We will keep a full list of excluded studies and record reasons for exclusion for each. Studies that meet the eligibility criteria at both the title and abstract stages will be reviewed at the full text stage. Those excluded at this stage will also be recorded along with reasons for the exclusion. A full list of studies excluded at full-text will be provided with the systematic map, along with the reasons for exclusion.
We will use double screening for a small subset of 100 training studies at the title and abstract stage and then use the approach in Snilstveit et al. for securing agreement among coders [ 56 ]. We will use a training set consisting of 100 studies randomly selected from an initial search using our search string in Web of Science to assess agreement among coders. The reviewers will discuss any discrepancies between coding for this subset to reach agreement. Based on a training set of studies screened by all reviewers, inter-rater reliability will be calculated using a Kappa statistic for all studies double screened at title and abstract levels [ 57 ]. If the Kappa test agreement falls below 0.6, indicating moderate agreement, an additional reviewer will be consulted and an additional set of 100 test studies will be screened by all reviewers, as in [ 39 , 51 ].
During the screening process, when a rater is uncertain about study eligibility, the relevant study will be marked for a second opinion and screening by a second rater will be conducted. The lead reviewer will check the consistency of coding periodically throughout the coding process for a subset of studies at the title and abstract screening phase and at the full-text screening stage. At both the title and abstract screening phase and the full-text data extraction stage, a subset of 10% of the studies will be assessed by at least two reviewers. Studies where there is inconsistency or disagreement will be marked as “Re-evaluate” in EPPI-Reviewer 4 and will be discussed by reviewers to reach agreement.
Eligibility criteria
Relevant type of study.
Given that we seek to provide a resource for decision-makers, as well as identify gaps and well-researched areas in the current evidence base, we will include both primary studies and systematic reviews. Primary studies that measure the effect of agroforestry practices and interventions on the different outcomes of interest will be included, as will systematic reviews of the literature that synthesize and analyze these same relationships. We will include English-language studies conducted from 1990 onwards, through until the time of search (mid-2018).
Included studies must explicitly examine the outcomes of specific agroforestry practices and interventions on farm settings. Further, they must use a comparator, which may be temporal, spatial, between group, or some combination of these (see below). We will exclude theoretical or modeling studies (unless they include a relevant empirical example with design that meets eligibility criteria), and editorials and commentaries. Experimental trials managed by researchers will not be included due to time and resource constraints and since the population of interest for this systematic map is farmers and farmer’s land. These experimental off-farm trials, however, will be excluded into a separate bin in EPPI-Reviewer 4 and be available as a base for future work and synthesis. On-farm field trials will be included if all other eligibility criteria are met.
Relevant types of study design
We will include four kinds of studies: (1) quantitative impact evaluations, (2) systematic reviews, (3) on-farm field trials that test specific agroforestry techniques and approaches, and (4) observational studies on the effect of agroforestry practices.
Impact evaluations are studies that measure changes that occur due to an intervention. Such studies will use an experimental or quasi-experimental study design to conduct a counterfactual analysis to allow for attribution of changes in an outcome to a specific intervention, or compare the effects of different types of programs [ 58 ]. Specifically, we will include the following types of impact evaluation studies:
Studies where participants or sites/plots of land (farmers, or land management areas on a farmer’s land) are randomly assigned to treatment and comparison group (experimental study designs);
Studies where assignment to treatment and comparison groups is based on other known allocation rules, including a threshold on a continuous variable (regression discontinuity designs) or exogenous geographical variation in the treatment allocation (natural experiments);
Studies with non-random assignment to treatment and comparison group that include pre-and post-test measures of the outcome variables of interest to ensure equity between groups on the baseline measure, and that use appropriate methods to control for selection bias and confounding. Such methods include statistical matching (for example, propensity score matching, or covariate matching), regression adjustment (for example, difference-in-differences, fixed effects regression, single difference regression analysis, instrumental variables, and ‘Heckman’ selection models).
Studies with non-random assignment to treatment and comparison group that include post-test measures of the outcome variables of interest only and use appropriate methods to control for selection bias and confounding, as above.
Ideally, studies would include baseline and post-intervention data, but due to our expectation of a small number of studies meeting this criterion, we will include studies with post-intervention outcome data only as long as they use some method to control for selection bias and confounding factors.
Reviews examine the effects of different interventions using transparent and systematic methods to identify, appraise and synthesize findings from studies addressing a specific issue [ 59 ]. We will include systematic reviews, meta-analyses, and literature reviews that describe methods used for search, data collection, and synthesis. Literature reviews that do not describe methods used for search, data collection and synthesis will not be included; however, they will be identified and included in a separate bin in EPPI-Reviewer 4 and made available as a list with the report. Systematic maps and evidence gap maps identified where agroforestry is an area of interest will also be noted in the final report.
Field trials in agroforestry are designed to test the effects of experimental treatments or other variables on crop yield or other outcomes of interest in conditions similar to the actual growing conditions experienced by farmers who may adopt the treatment [ 14 , 60 ]. While impact evaluations measure the changes due to an intervention, field trials measure the changes due to a practice. As for agronomy more generally, field trials can be divided into three types: (1) Researcher managed and researcher implemented; (2) researcher managed and farmer implemented, and (3) farmer managed and farmer implemented. We will include only studies implemented on a farmer’s land, and only if they pertain directly to some aspect of agroforestry, include an experimental research design, and describe the effects of an intervention, technique, or practice on an outcome category relevant to the current study.
Finally, we will include observational studies on the effects of agroforestry practices, provided they are quantitative and include at least one comparison as described below (e.g. before/after; study group/non-study group). We include such studies given that we anticipate a number of potentially interesting studies will not examine the impacts of an agroforestry intervention per se, but a specific practice or set of practices.
The SM will include both completed and ongoing studies, and the ongoing studies will be coded as ongoing and not yet completed.
Relevant subject
The subject of interest will be farms and/or the people that live and farm on them that are incorporating any agroforestry practices into their farming system within the high-income countries (Additional file 1 ).
Relevant intervention or practice
Our study will capture studies evaluating the impacts of interventions to support agroforestry as well as those evaluating agroforestry practices alone. From a policy perspective, it is especially useful to know what kinds of interventions might most effectively promote agroforestry practices to yield desired social-ecological outcomes. Although impact evaluations on agroforestry-related interventions (Table 2 ) are of particular interest for policy-makers, our study will also include studies on the impacts of specific agroforestry practices (Table 1 ) without a policy intervention, which will broadly capture the impacts of agroforestry practices. This SM will therefore include any study that meets our criteria that evaluates the impacts of one or more agroforestry practice or intervention. In our map, we will indicate studies that include an evaluation of an agroforestry-related intervention, versus studies that evaluate the impact of only an agroforestry practice without a policy intervention. We will conduct analyses on the body of agroforestry practice impact studies as well as on the body of studies evaluating the impacts of specific agroforestry interventions.
Relevant comparator
Farm or household that does not adopt a given practice identified in Table 1 , or is not exposed to a specific agroforestry intervention,
Farm or household before adopting a given agroforestry practice, or being exposed to a specific agroforestry intervention,
Farm or household that adopts a different agroforestry practice, and/or that is exposed to a different specific agroforestry intervention,
Primary forests, secondary forests, or managed forestry/plantations that not exposed to a specific agroforestry intervention,
A combination of two or more of the above. We will not include studies that only compare agroforestry practices with other agroforestry practices (i.e. studies that only evaluate different implementation of the same agroforestry practice, or studies that only evaluate multiple types of agroforestry practice).
Relevant outcomes
The columns of the SM matrix will be comprised of three broad outcome categories: (1) agricultural productivity, (2) ecosystem services, and (3) human well-being.
Studies that focus exclusively on the adoption of a particular agroforestry technique or species without reference to impact will be excluded. We will, however, note the number of adoption-related studies (and their geographic location) excluded due to lack of evidence on outcomes. The primary outcomes are the three stated above (agricultural productivity, ecosystem services, and human well-being), and secondary outcomes are adoption and behavior change, which will only be reported if the study also reports primary outcomes.
Specific outcome categories under agricultural productivity will comprise farm productivity, including yield, and profitability.
Ecosystem services outcomes will first be classified under three broad categories: (a) provisioning, (b) regulation and maintenance, and (c) cultural services. Outcomes will be further divided into a number of specific categories following the Common International Classification of Ecosystem Services (CICES) developed by the European Environment Agency [ 61 ] and presented in Table 6 . CICES builds from the seminal Millennium Ecosystem Assessment [ 62 ], The Economics of Ecosystems and Biodiversity [ 63 ], and other ecosystem services classification schemes.
For human well-being, the final broad outcome we will examine, we adapt the classification published in [ 40 ] to identify a set of key policy-relevant domains of human well-being (Table 7 ). Based on likely policy interest and goals typically articulated by proponents of agroforestry, we will focus on five dimensions of human well-being: income and household expenditure, housing and material assets, food security and nutrition, health, and cultural and subjective well-being. We will also include the category of “other” which may group some studies focusing on the other dimensions of human well-being identified in McKinnon et al. [ 40 ]. In this last category, we will note in particular any mention of adaptive capacity or resilience, especially with reference to the impacts of climate change.
We will present the three outcomes in the SM main matrix in two ways: (1) a simplified typology of broad agroforestry practice/intervention and outcome categories and (2) a more detailed version with the specific agroforestry practice/intervention and outcome categories.
Types of settings
We expect that the agroforestry interventions and outcomes will take place in a range of settings in HICs. These settings will cover a range of ecoregions and are likely to be primarily rural, but potentially also urban areas (e.g. city gardens). We also expect much of the evidence to pertain to smallholders, but some may describe agroforestry practices among larger landholders.
Study quality assessment
Systematic maps do not tend to provide much information on study quality, but rather simply provide the broad overview of knowledge and highlight areas where there is the potential for further review and literature quality assessment [ 55 ]. Therefore, we will not conduct study quality assessments on the studies included in this SM.
However, our study will include information about type of study design, referring to the types of study design presented above, including quantitative impact evaluations (experimental or quasi-experimental), systematic reviews, on-farm field trials (farmer-managed or researcher-managed), and observational studies on the effect of agroforestry practices. Furthermore, the type of quasi-experimental methods used, if applicable, will be documented. This data is not intended to offer an assessment of study quality, but rather provide basic information to get a broad perspective of the type of research being conducted in each area of the typology. As in the L&MIC EGM, we will break our results into three sections: a discussion of all included primary studies, a discussion of the subset consisting of only quantitative impact evaluation studies on interventions, and a discussion of included systematic reviews. We will present the distribution of study types for included studies and provide a list of all studies included at full-text with their assigned study type.
We do not expect to find any included studies authored by the coders of this systematic map. However, in the case that a study authored by one of the reviewers is included, those involved with authoring the studies will not be involved in decisions regarding inclusion or critical appraisal of that study.
Data coding strategy
Our research team will be led by the first author of this protocol (SEB), and the point of contact for any disputes on coding strategy will be the second author (DCM). The research team will consist of SEB, DCM, and between two and three hired research assistants, who are students at the University of Illinois at Urbana-Champaign. We will use a standardized data extraction form, attached as Additional file 3 , to extract descriptive data from all studies meeting our eligibility criteria. We will create a codebook describing the scope of each question in the data extraction form. We will conduct a pilot with a small subset of studies by everyone in the research team to ensure consistency and to resolve any issues or ambiguities. Given the likely volume of studies (based on previous SR and SM experience such as in [ 15 , 40 ]), we do not plan to carry out extensive side-by-side double extraction of data at the full text stage. Instead, we will conduct random spot checks of a small percentage of included articles to ensure consistency between raters. We will measure consistency using percent disagreement of spot-checking with the primary rater. We note that in our test studies, we found that some studies only specify a general practice (silvoarable, silvopasture, etc.) without detailing a specific practice (alley-cropping, improved fallow, riparian buffer strips, etc.). We address this in our data extraction spreadsheet by allowing a selection of “not specified” for the practice type.
Study mapping and presentation
We expect to perform several analyses based on the data collected and to summarize results visually and in various written forms to effectively communicate with intended audiences. A final report will present the map as a detailed data set with figures of descriptive statistics derived from the data set, as in [ 64 ]. We intend to publish our systematic map in Environmental Evidence and upload the dataset online as an open-access, interactive site, as in [ 41 ]. To communicate our results and visualize our data, we intend to create at minimum the following:
We will create a flow diagram of the systematic mapping process, detailing the number of studies returned by our search, included and excluded at each stage, and the number of studies included at the final data extraction stage.
We will create a tabular visualization reflecting agroforestry practices on the intervention axis and outcomes of the interventions on the outcome axis.
We will create a second tabular visualization reflecting agroforestry interventions on the intervention axis and outcomes of the interventions on the outcome axis.
We will also show the distribution of studies for each country on a geographic map, as in [ 40 , 50 , 64 ].
We will provide descriptive statistics on geographical distribution of study location by country and world region, the type of studies, and quality of the SRs.
We will visually present the included studies in a matrix. The matrix will be stylized as a topography that notes whether the study is a review/SR, an impact evaluation of agroforestry interventions, or if the paper studies the impacts of specific agroforestry practices.
Based on these maps, we will perform gap analysis to identify areas for systematic review or primary research.
We will create heatmaps as in [ 64 ] to visualize and identify knowledge gaps and clusters.
We will upload our dataset online on an open-access, interactive map server, as in [ 41 ]. Users will be able to visualize our results, filter the dataset per our data coding criteria, and automatically interact with the most updated version.
Furthermore, the reviewers will formally discuss and collectively identify areas of knowledge gaps and clusters of higher-quality literature based on their experience from screening full-texts after coding is completed. We will also comment specifically on the extent to which the literature examines interventions vs. specific practices or both simultaneously.
Smith J, Pearce BD, Wolfe MS. Reconciling productivity with protection of the environment: is temperate agroforestry the answer? Renew Agric Food Syst. 2012;28:80–92.
Article Google Scholar
Jose S, Gold MA, Garrett HE. The future of temperate agroforestry in the United States. In: Nair PKR, Garrity D, editors. Agroforestry—the future of global land use. Dordrecht: Springer Netherlands; 2012. p. 217–45.
Chapter Google Scholar
Waldron A, Garrity D, Malhi Y, Girardin C, Miller DC, Seddon N. Agroforestry can enhance food security while meeting other sustainable development goals. Trop Conserv Sci. 2017;10:1–6.
Garrity DP. Agroforestry and the achievement of the millennium development goals. Agrofor Syst. 2004;61:5–17.
Google Scholar
Jose S. Agroforestry for ecosystem services and environmental benefits: an overview. Agrofor Syst. 2009;76:1–10.
Jose S, Bardhan S. Agroforestry for biomass production and carbon sequestration: an overview. Agrofor Syst. 2012;86:105–11.
Fagerholm N, Torralba M, Burgess PJ, Plieninger T. A systematic map of ecosystem services assessments around European agroforestry. Ecol Ind. 2016;62:47–65.
Wilson M, Lovell S. Agroforestry—the next step in sustainable and resilient agriculture. Sustainability. 2016;8:574.
Holzmueller EJ, Jose S. Biomass production for biofuels using agroforestry: potential for the North Central Region of the United States. Agrofor Syst. 2012;85:305–14.
Tsonkova P, Böhm C, Quinkenstein A, Freese D. Ecological benefits provided by alley cropping systems for production of woody biomass in the temperate region: a review. Agrofor Syst. 2012;85:133–52.
Udawatta RP, Jose S. Agroforestry strategies to sequester carbon in temperate North America. Agrofor Syst. 2012;86:225–42.
Buck LE. Agroforestry policy issues and research directions in the US and less developed countries: insights and challenges from recent experience. Agrofor Syst. 1995;30:57–73.
Lassoie JP, Buck LE, Current D. The development of agroforestry as an integrated land use management strategy. In: Gene Garrett HE, editor. North American agroforestry: an integrated science and practice. 2nd ed. Washington: American Society of Agronomy; 2009. p. 1–24.
Lovell ST, Dupraz C, Gold M, Jose S, Revord R, Stanek E, Wolz KJ. Temperate agroforestry research: considering multifunctional woody polycultures and the design of long-term field trials. Agrofor Syst. 2018;92:1397–415.
Miller DC, Ordonez PJ, Baylis K, Hughes K, Rana P. Protocol for an evidence and gap map. The impacts of agroforestry on agricultural productivity, ecosystem services, and human well-being in low- and middle-income countries: an evidence and gap map. In: Campbell collaboration; 2017.
USDA. USDA agroforestry strategic framework, fiscal year 2011–2016. Washington, D.C.: U. S. Department of Agriculture; 2011.
Leakey RRB. Definition of agroforestry revisited. Agrofor Today. 1996;8:5–7.
Atangana A, Khasa D, Chang S, Degrande A. Definitions and classification of agroforestry systems. In Tropical agroforestry. Dordrecht: Springer; 2014. p. 35–47.
Huxley P, van Houten H. Glossary for agroforestry. Nairobi: World Agroforestry Center (ICRAF); 1997.
USDA. Agroforestry USDA reports to America, fiscal years 2011–2012—comprehensive version. Washington, D.C.: Department of Agriculture; 2013.
Mosquera-Losada MR, Santiago-Freijanes J, Pisanelli A, Rois M, Smith J, Herder M, Moreno G, Malignier N, Mirazo JR, Lamersdorf N, et al. Extent and success of current policy measures to promote agroforestry across Europe. 2016.
Nair PKR, Garrity D. Agroforestry—the future of global land use. 2012.
Garrett HE, Buck L. Agroforestry practice and policy in the United States of America. For Ecol Manag. 1997;91:5–15.
IPES-Food. From uniformity to diversity: a paradigm shift from industrial agriculture to diversified agroecological systems. International Panel of Experts on Sustainable Food Systems; 2016.
Matson PA, Parton WJ, Power AG, Swift MJ. Agricultural intensification and ecosystem properties. Science. 1997;277:504–9.
Article CAS Google Scholar
Tilman D, Cassman KG, Matson PA, Naylor R, Polasky S. Agricultural sustainability and intensive production practices. Nature. 2002;418:671.
Lobao L, Meyer K. The great agricultural transition: crisis, change, and social consequences of twentieth century US farming. Ann Rev Sociol. 2001;27:103–24.
Foley JA, DeFries R, Asner GP, Barford C, Bonan G, Carpenter SR, Chapin FS, Coe MT, Daily GC, Gibbs HK, et al. Global consequences of land use. Science. 2005;309:570–4.
Valdivia C, Barbieri C, Gold MA. Between forestry and farming: policy and environmental implications of the barriers to agroforestry adoption. Can J Agric Econ. 2012;60:155–75.
Jacobson M, Kar S. Extent of agroforestry extension programs in the United States. J Ext. 2013;51:4RIB4.
Mbow C, Van Noordwijk M, Luedeling E, Neufeldt H, Minang PA, Kowero G. Agroforestry solutions to address food security and climate change challenges in Africa. Curr Opin Environ Sustain. 2014;6:61–7.
Sharma N, Bohra B, Pragya N, Ciannella R, Dobie P, Lehmann S. Bioenergy from agroforestry can lead to improved food security, climate change, soil quality, and rural development. Food Energy Secur. 2016;5:165–83.
Garrett HE. North American agroforestry: an integrated science and practice. Washington: American Society of Agronomy; 2009.
Book Google Scholar
World Bank Country and Lending Groups. https://datahelpdesk.worldbank.org/knowledgebase/articles/906519-world-bank-country-and-lending-groups . Accessed 28 June 2018.
Wolz KJ, Lovell ST, Branham BE, Eddy WC, Keeley K, Revord RS, Wander MM, Yang WH, DeLucia EH. Frontiers in alley cropping: transformative solutions for temperate agriculture. Glob Chang Biol. 2018;24:883–94.
Dollinger J, Jose S. Agroforestry for soil health. Agrofor Syst. 2018;92:213–9.
Torralba M, Fagerholm N, Burgess PJ, Moreno G, Plieninger T. Do European agroforestry systems enhance biodiversity and ecosystem services? A meta-analysis. Agric Ecosyst Environ. 2016;230:150–61.
Haddaway NR, Brown C, Eales J, Eggers S, Josefsson J, Kronvang B, Randall NP, Uusi-Kämppä J. The multifunctional roles of vegetated strips around and within agricultural fields. Environ Evid. 2018;7:14.
Ottoy S, Angileri V, Gibert C, Paracchini ML, Pointereau P, Terres J-M, Van Orshoven J, Vranken L, Dicks LV. Impacts of selected ecological focus area options in European farmed landscapes on climate regulation and pollination services: a systematic map protocol. Environ Evid. 2018;7:10.
McKinnon MC, Cheng SH, Dupre S, Edmond J, Garside R, Glew L, Holland MB, Levine E, Masuda YJ, Miller DC, et al. What are the effects of nature conservation on human well-being? A systematic map of empirical evidence from developing countries. Environ Evid. 2016;5:8.
Cheng SH, Ahlroth S, Onder S, Shyamsundar P, Garside R, Kristjanson P, McKinnon MC, Miller DC. What is the evidence for the contribution of forests to poverty alleviation? A systematic map protocol. Environ Evid. 2017;6:10.
Garrity DP, Akinnifesi FK, Ajayi OC, Weldesemayat SG, Mowo JG, Kalinganire A, Larwanou M, Bayala J. Evergreen agriculture: a robust approach to sustainable food security in Africa. Food Secur. 2010;2:197–214.
Mosquera-Losada MR, Moreno G, Pardini A, McAdam JH, Papanastasis V, Burgess PJ, Lamersdorf N, Castro M, Liagre F, Rigueiro-Rodríguez A. Past, Present and Future of Agroforestry Systems in Europe. In: Nair PKR, Garrity D, editors. Agroforestry—the future of global land use. Dordrecht: Springer Netherlands; 2012. p. 285–312.
Thevathasan NV, Gordon AM, Bradley R, Cogliastro A, Folkard P, Grant R, Kort J, Liggins L, Njenga F, Olivier A, et al. Agroforestry research and development in Canada: the way forward. In: Nair PKR, Garrity D, editors. Agroforestry—the future of global land use. Dordrecht: Springer Netherlands; 2012. p. 247–83.
Reid R. Developing farmer and community capacity in agroforestry: is the Australian Master TreeGrower program transferable to other countries? Agrofor Syst. 2016;91:847–65.
Takeuchi K. Rebuilding the relationship between people and nature: the Satoyama initiative. Ecol Res. 2010;25:891–7.
den Herder M, Moreno G, Mosquera-Losada RM, Palma JHN, Sidiropoulou A, Santiago Freijanes JJ, Crous-Duran J, Paulo JA, Tomé M, Pantera A, et al. Current extent and stratification of agroforestry in the European Union. Agric Ecosyst Environ. 2017;241:121–32.
Montambault JR, Alavalapati JRR. Socioeconomic research in agroforestry: a decade in review. Agrofor Syst. 2005;65:151–61.
Jordan N, Warner KD. Towards multifunctional agricultural landscapes for the Upper Midwest Region of the USA. In: Steve Wratten HS, Ross C, Robert C, editors. Ecosystem services in agricultural and urban landscapes. New York: Wiley; 2013. p. 139–56.
Cheng SH, MacLeod K, Ahlroth S, Onder S, Perge E, Shyamsundar P, Rana P, Garside R, Kristjanson P, McKinnon MC, Miller DC. What is the contribution of forests to poverty alleviation? A systematic map of global evidence. Environmental Evidence in review.
Haddaway NR, Brown C, Eggers S, Josefsson J, Kronvang B, Randall N, Uusi-Kämppä J. The multifunctional roles of vegetated strips around and within agricultural fields. A systematic map protocol. Environ Evid. 2016;5:18.
Haddaway NR, Collins AM, Coughlin D, Kirk S. A rapid method to increase transparency and efficiency in web-based searches. Environ Evid. 2017;6:1.
Haddaway NR, Collins AM, Coughlin D, Kirk S, Larsen P, Ins MV, Pautasso M, Noorden R, Khabsa M, Giles C, et al. The role of google scholar in evidence reviews and its applicability to grey literature searching. PLoS ONE. 2015;10:e0138237.
Perkel JM. How scientists use Slack. Nat News. 2017;541:123.
James KL, Randall NP, Haddaway NR. A methodology for systematic mapping in environmental sciences. Environ Evid. 2016;5:7.
Snilstveit B, Stevenson J, Villar PF, Eyers J, Harvey C, Panfil S, Puri J, McKinnon MC. Land-use change and forestry programmes: evidence on the effects on greenhouse gas emissions and food security. In: Evidence gap map report 3. London; 2016.
Cohen J. A coefficient of agreement for nominal scales. Educ Psychol Meas. 1960;20:37–46.
Ferraro PJ. Counterfactual thinking and impact evaluation in environmental policy. New Dir Eval. 2009;2009:75–84.
Waddington H, White H, Snilstveit B, Hombrados JG, Vojtkova M, Davies P, Bhavsar A, Eyers J, Koehlmoos TP, Petticrew M, et al. How to do a good systematic review of effects in international development: a tool kit. J Dev Eff. 2012;4:359–87.
Nair PKR. State-of-the-art of agroforestry research and education. Agrofor Syst. 1993;23:95–119.
The Common International Classification of Ecosystem Services (CICES). http://cices.eu . Accessed 28 June 2018.
Millennium Ecosystem Assessment. Ecosystems and human well-being: synthesis. Washington, DC: Island Press; 2005.
Kumar P. The economics of ecosystems and biodiversity (TEEB) ecological and economic foundations. London, Washington: Earthscan; 2010.
Miller D, Ordonez P, Brown S, Forrest S, Nava N, Hughes K, Baylis K. The impacts of agroforestry on agricultural productivity, ecosystem services, and human well-being in low- and middle-income countries: an evidence gap map. In: 3ie evidence gap map report. London: International Initiative for Impact Evaluation (3ie); (in review) .
Download references
Authors’ contributions
All authors developed the original idea, participated in discussions defining the search and eligibility criteria and data coding strategy, and decided the study mapping and presentation design. SEB contributed to developing the background section and testing the search criteria. DCM, PJO, and KB developed the theory of change. PJO and SEB developed the data coding table. SEB had the lead of writing the manuscript, with contributions from all authors. All authors read and approved the final manuscript.
Acknowledgements
We thank Karl Hughes and Pushpendra Rana for helping shape this protocol through their development of a parallel project creating an Evidence Gap Map (EGM) for agroforestry impacts in low- and middle- income countries (L&MICs), supported by 3ie [ 15 ]. We also thank Festus Amadu and Katia Nakamura for comments on an earlier version of this protocol. Finally, we thank the editor of Environmental Evidence, Dr. Andrew Pullin, and three anonymous reviewers for comments on earlier drafts of this manuscript that have greatly improved this protocol.
Competing interests
The authors declare that they have no competing interests.
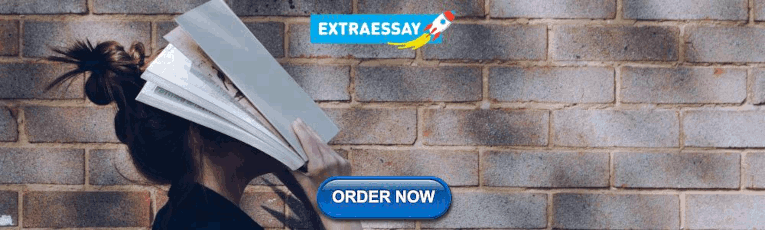
Availability of data and materials
Not applicable.
Consent for publication
Ethics approval and consent to participate.
This project is funded by the USDA National Institute of Food and Agriculture, Hatch Project #1009327.
Publisher’s Note
Springer Nature remains neutral with regard to jurisdictional claims in published maps and institutional affiliations.
Author information
Authors and affiliations.
Department of Natural Resources and Environmental Sciences, University of Illinois Urbana-Champaign, 1102 S Goodwin Ave, Urbana, IL, 61801, USA
Sarah E. Brown & Daniel C. Miller
Department of Agricultural and Consumer Economics, University of Illinois Urbana-Champaign, 1301 W Gregory Dr, Urbana, IL, 61801, USA
Pablo J. Ordonez & Kathy Baylis
You can also search for this author in PubMed Google Scholar
Corresponding author
Correspondence to Sarah E. Brown .
Additional files
Additional file 1..
List of high-income countries (according to the World Bank 2018 fiscal year classification [ 34 ]). Tabular list of high-income countries according to the World Bank 2018 fiscal year classification.
Additional file 2.
Test studies and scoping results. List of test studies and results of search string scoping process.
Additional file 3.
SM data extraction spreadsheet. Data extraction spreadsheet describing the data coding components for creating the systematic map.
Rights and permissions
Open Access This article is distributed under the terms of the Creative Commons Attribution 4.0 International License ( http://creativecommons.org/licenses/by/4.0/ ), which permits unrestricted use, distribution, and reproduction in any medium, provided you give appropriate credit to the original author(s) and the source, provide a link to the Creative Commons license, and indicate if changes were made. The Creative Commons Public Domain Dedication waiver ( http://creativecommons.org/publicdomain/zero/1.0/ ) applies to the data made available in this article, unless otherwise stated.
Reprints and permissions
About this article
Cite this article.
Brown, S.E., Miller, D.C., Ordonez, P.J. et al. Evidence for the impacts of agroforestry on agricultural productivity, ecosystem services, and human well-being in high-income countries: a systematic map protocol. Environ Evid 7 , 24 (2018). https://doi.org/10.1186/s13750-018-0136-0
Download citation
Received : 01 May 2018
Accepted : 11 October 2018
Published : 29 October 2018
DOI : https://doi.org/10.1186/s13750-018-0136-0
Share this article
Anyone you share the following link with will be able to read this content:
Sorry, a shareable link is not currently available for this article.
Provided by the Springer Nature SharedIt content-sharing initiative
- Alley cropping
- Silvopasture
- Riparian buffer
- Forest farming
- Agriculture
- Socio-economic impact
- Impact evaluation
Environmental Evidence
ISSN: 2047-2382
- Submission enquiries: Access here and click Contact Us
- General enquiries: [email protected]
Advertisement
Role of agricultural productivity growth in economic development: the neglected impact on institutional quality in Africa
- Published: 14 February 2022
- Volume 19 , pages 681–700, ( 2022 )
Cite this article
- Richard Grabowski ORCID: orcid.org/0000-0003-0617-1110 1 &
- Sharmistha Self 2
260 Accesses
3 Citations
2 Altmetric
Explore all metrics
Growing agricultural productivity has often been seen as the key to rapid economic development. In this paper, it is argued that agricultural productivity growth plays an important role in institutional development. It is hypothesized that agricultural productivity growth leads to structural change both inside the rural agricultural sector and between this sector and modern manufacturing and services. This structural change creates opportunities for institutional entrepreneurs to extend institutional protection to these new activities (improving institutional quality). It is also hypothesized that increases in inequality tend to lead to institutional innovation which reduces institutional quality. These hypotheses were tested utilizing data for eighteen countries in Sub-Saharan Africa. The main conclusion is that policies that promote agricultural productivity increases are found to be important in the improvement of institutional quality.
This is a preview of subscription content, log in via an institution to check access.
Access this article
Subscribe and save.
- Get 10 units per month
- Download Article/Chapter or eBook
- 1 Unit = 1 Article or 1 Chapter
- Cancel anytime
Price includes VAT (Russian Federation)
Instant access to the full article PDF.
Rent this article via DeepDyve
Institutional subscriptions
Similar content being viewed by others
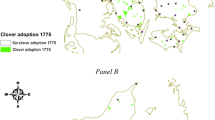
Agricultural productivity and economic development: the contribution of clover to structural transformation in Denmark
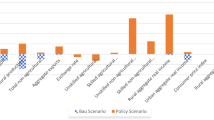
The impacts of agricultural productivity on structural transformation, and poverty alleviation in Africa: evidence from Guinea-Bissau
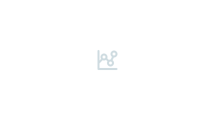
Economic strategy and agricultural productivity
Acemoglu D, Robinson J (2012) Why Nations Fail. Random House, New York
Google Scholar
Baba Y, Hendry DF, Starr RM (1992) The demand for m1 in the USA, 1960–1988. Rev Econ Stud 59:25–61. https://doi.org/10.2307/2297924
Article Google Scholar
Battlana J, Boxenbaum E, Lecca B (2009) How actors change institutions: towards a theory of institutional entrepreneurship. Acad Manag Ann 3(1):65–107
Carillo MF (2021) Agricultural policy and long-run development: evidence from Mussolini’s battle for grain. Econ J 634:566–597
Choi I (2001) Unit root tests for panel data. J Int Money Financ 20(2):249–272
Dercon S, Gollin D (2014) Agriculture in African development: a review of theories and strategies. Annual Review of Resource Economics 6:471–492
deVries G, Timmer MP, deVries K (2015) Structural transformation in africa: static gains, dynamic losses. J Dev Stud 51(6):674–688
Di Maggio PJ (1988) Interest and agency in institutional theory. In: Zucker L (ed) Institutional patterns and organization. Cambridge University Press, Cambridge
Food and Agricultural Organization (2020) FAOSTAT, ASTI Research and development indicators.
Hau MV (2012) State capacity and inclusive development. Effective State and Inclusive Development Research Center Working Paper 2, pp 1–39
Hodgson G (2006) What are institutions. J Econ Issues 40(1):1–25
Hsiao C (2005) Why Panel Data? 2005, IEPR Working Paper No. 05.33, Available at SSRN: https://ssrn.com/abstract=820204 or https://doi.org/10.2139/ssrn.820204
Huneeus F, Rogerson R (2020) Heterogeneous paths of industrialization. Cowles Foundation Discussion Paper No. 2253, pp 1–46
Im KS, Hashem Pesaran M, Shin Y (2003) Testing for unit roots in heterogeneous panels. J Economet 115(1):53–74
Johnston BF, Mellor JW (1961) The role of agriculture in economic development. Am Econ Rev 51(4):566–593
Kao C (1999) Spurious regression and residual-based tests for cointegration in panel data. J Economet 90:1–44
Kao C, Chiang MH (2000) On the estimation and inference of a cointegrated regression in panel data. In: Baltagi B (ed) nonstationary panels, panel cointegration, and dynamic panels (Advances in Econometrics). JAI Press, Amsterdam, pp 161–178
Krasner S (1984) Approaches to the state: alternative conceptions and historical dynamics. Comp Polit 16(2):223–246
Lashitew A, Werker E (2020) Do natural resources help or hinder development? Resource abundance, dependence, and the role of institutions. Resour Energy Econ 61:101–145
Levin A, Lin CF, Chu CSJ (2002) Unit root tests in panel data: asymptotic and finite-sample properties. J Economet 108(1):1–24
Lewis WA (1954) Economic development with unlimited supply of labour. Manch Sch 22(2):139–191
Maddala GS, Wu S (1999) A comparative study of unit root tests with panel data and a new simple test. Oxford Bull Econ Stat 61(S1):631–652
Mahoney J, Thelen K (2010) A theory of gradual institutional change. In: Mahoney J, Thelen K (eds) Exploring institutional change. Cambridge University Press, Cambridge
Malthus TR (1959) Population: the first essay. University of Michigan Press, Ann Arbor ( 1798 )
Book Google Scholar
Matsuyama K (1992) Agricultural productivity, comparative advantage, and economic growth. J Econ Theory 58(2):317–334
McArthur JW, McCord GC (2017) Fertilizing growth: agricultural inputs and their effects in economic development. J Dev Econ 127:133–152
McCullough E (2017) Labor productivity and employment gaps in sub-Saharan Africa. Food Policy 67:133–152
Mensah E, Szirmai A (2018) Africa database (ASD): expansion and update, 2018, UNU-MERIT Working Paper Series, #2018–20
Mensah E, Owusu S, Foster-McGregor N, Szirmai A (2018) Structural change, productivity growth, and labor-market turbulence in Africa. UNU-MERIT Working Papers #2018-025
Méon P, Sekkat K (2005) Does corruption grease or sand the wheels of growth? Public Choice 122(1/2):69–97
Moscona J (2019) Agricultural development and structural changes within and across nations. Working Paper, Department of Economics MIT, pp 1–45
North D (1981) Structure and change in economic history. Cambridge University Press, New York
North D (1990) Institutions, institutional change, and economic performance. Cambridge University Press, New York
Pedroni P (2001) Fully modified OLS for heterogeneous cointegrated panels. In: Baltagi BH, Fomby TB, Hill RC (eds) Nonstationary panels, panel cointegration, and dynamic panels; Advances in econometrics, vol 15. Emerald Group Publishing Limited, pp 93–130
Chapter Google Scholar
Pedroni P (1996) Fully modified OLS for heterogeneous cointegrated panels and the case of purchasing power parity; Manuscript; Department of Economics, Indiana University. http://web.williams.edu/Economics/pedroni/WP-96-20.pdf . Accessed 2021
Ranis G, Fei J (1961) A theory of economic development. Am Econ Rev 51(4):533–565
Ricardo D (1965) The principles of political economy and taxation. Everyman’s Library, London ( 1817 )
Sen K (2019) What explains the job creating potential of industrialization in the developing world. J Dev Stud 55(7):1565–1583
Stock JK, Watson M (1993) A simple estimator of cointegrating vectors in higher order integrated systems. Econometrica 61:783–820
Taylor M (2009) Institutional development through policy-making: a case study of the Brazilian Central Bank. World Polit 61(3):487–515
United States Department of Agriculture (2020) International agricultural productivity. https://www.ers.usda.gov/data-products/international-agricultural-productivity/
World Bank (2020) World development indicators
Download references
Author information
Authors and affiliations.
Department of Economics, Southern Illinois University, Carbondale, IL, 62901, USA
Richard Grabowski
Department of Economics, University of Northern Iowa, Cedar Falls, IA, 50613, USA
Sharmistha Self
You can also search for this author in PubMed Google Scholar
Corresponding author
Correspondence to Richard Grabowski .
Ethics declarations
Conflict of interest.
On behalf of all authors, the corresponding author states that there is no conflict of interest.
Additional information
Publisher's note.
Springer Nature remains neutral with regard to jurisdictional claims in published maps and institutional affiliations.
About this article
Grabowski, R., Self, S. Role of agricultural productivity growth in economic development: the neglected impact on institutional quality in Africa. Evolut Inst Econ Rev 19 , 681–700 (2022). https://doi.org/10.1007/s40844-022-00232-w
Download citation
Received : 17 July 2021
Accepted : 28 January 2022
Published : 14 February 2022
Issue Date : September 2022
DOI : https://doi.org/10.1007/s40844-022-00232-w
Share this article
Anyone you share the following link with will be able to read this content:
Sorry, a shareable link is not currently available for this article.
Provided by the Springer Nature SharedIt content-sharing initiative
- Agricultural productivity
- Institutions
JEL Classification
- Find a journal
- Publish with us
- Track your research

An official website of the United States government
The .gov means it’s official. Federal government websites often end in .gov or .mil. Before sharing sensitive information, make sure you’re on a federal government site.
The site is secure. The https:// ensures that you are connecting to the official website and that any information you provide is encrypted and transmitted securely.
- Publications
- Account settings
Preview improvements coming to the PMC website in October 2024. Learn More or Try it out now .
- Advanced Search
- Journal List
- Philos Trans R Soc Lond B Biol Sci
- v.365(1554); 2010 Sep 27
Implications of climate change for agricultural productivity in the early twenty-first century
This paper reviews recent literature concerning a wide range of processes through which climate change could potentially impact global-scale agricultural productivity, and presents projections of changes in relevant meteorological, hydrological and plant physiological quantities from a climate model ensemble to illustrate key areas of uncertainty. Few global-scale assessments have been carried out, and these are limited in their ability to capture the uncertainty in climate projections, and omit potentially important aspects such as extreme events and changes in pests and diseases. There is a lack of clarity on how climate change impacts on drought are best quantified from an agricultural perspective, with different metrics giving very different impressions of future risk. The dependence of some regional agriculture on remote rainfall, snowmelt and glaciers adds to the complexity. Indirect impacts via sea-level rise, storms and diseases have not been quantified. Perhaps most seriously, there is high uncertainty in the extent to which the direct effects of CO 2 rise on plant physiology will interact with climate change in affecting productivity. At present, the aggregate impacts of climate change on global-scale agricultural productivity cannot be reliably quantified.
1. Introduction
Agriculture is strongly influenced by weather and climate. While farmers are often flexible in dealing with weather and year-to-year variability, there is nevertheless a high degree of adaptation to the local climate in the form of established infrastructure, local farming practice and individual experience. Climate change can therefore be expected to impact on agriculture, potentially threatening established aspects of farming systems but also providing opportunities for improvements.
This paper reviews recent literature relevant to the impacts of climate change on global agricultural productivity through a wide range of processes. The aim is to provide a global-scale overview of all relevant impacts, rather than focusing on specific regions or processes, as the purpose of this review is to inform a wider assessment of the risks to global food security. Although there are a large number of studies which focus on the impact of a particular aspect of climate change in a specific location, there are relatively few studies which provide a global assessment. Moreover, these studies tend to focus more on the direct effect of changes in the mean climate state on crop growth and do not consider changes in extremes or in indirect effects of climate change such as sea-level rise or pests and diseases. A comprehensive, internally consistent assessment of all potential direct and indirect effects of climate change on agricultural productivity has not yet been carried out. As a step towards such a full-system assessment, we complement each stage of our review of the literature with presentation of projected changes in relevant climate-related quantities from the Met Office Hadley Centre (MOHC) models. This allows a comparison of the different aspects of climate change relevant to agricultural productivity, so that the relative importance of the different potential causes of impacts can be assessed. This provides some context to decision making in an area of high uncertainty, and also informs future research directions.
Most previous assessments of the impacts of climate change on agriculture (and indeed on other sectors) have focused on time horizons towards the end of the twenty-first century, illustrating the impacts of anthropogenic climate change that could be avoided by reducing greenhouse gas emissions. However, there is also a need to assess the impacts of climate change over the next few decades, which may now be largely unavoidable owing to inertia in the physical climate system and the time scales over which large-scale change in human social, economic and political influences on greenhouse gas emissions could be brought about. Even if greenhouse gas emissions began to be reduced immediately, there would still be some level of ongoing warming for decades and some sea-level rise continuing for centuries, as the climate system is slow to respond fully to imposed changes. There is relatively little information in the literature available on climate change impacts over these time horizons, so we present MOHC climate projections for approximately 2020 and 2050 in order to put the existing literature into context on these time scales.
This paper focuses on impacts on crop productivity, but many of the processes and impacts discussed may also apply to livestock. Some discussion of this is provided in the electronic supplementary material.
2. Direct impacts of climate change on agriculture
(a) changes in mean climate.
The nature of agriculture and farming practices in any particular location are strongly influenced by the long-term mean climate state—the experience and infrastructure of local farming communities are generally appropriate to particular types of farming and to a particular group of crops which are known to be productive under the current climate. Changes in the mean climate away from current states may require adjustments to current practices in order to maintain productivity, and in some cases the optimum type of farming may change.
Higher growing season temperatures can significantly impact agricultural productivity, farm incomes and food security ( Battisti & Naylor 2009 ). In mid and high latitudes, the suitability and productivity of crops are projected to increase and extend northwards, especially for cereals and cool season seed crops ( Maracchi et al . 2005 ; Tuck et al . 2006 ; Olesen et al . 2007 ). Crops prevalent in southern Europe such as maize, sunflower and soya beans could also become viable further north and at higher altitudes ( Hildén et al . 2005 ; Audsley et al . 2006 ; Olesen et al . 2007 ). Here, yields could increase by as much as 30 per cent by the 2050s, dependent on crop ( Alexandrov et al . 2002 ; Ewert et al . 2005 ; Richter & Semenov 2005 ; Audsley et al . 2006 ; Olesen et al . 2007 ). For the coming century, Fisher et al . (2005) simulated large gains in potential agricultural land for the regions such as the Russian Federation, owing to longer planting windows and generally more favourable growing conditions under warming, amounting to a 64 per cent increase over 245 million hectares by the 2080s. However, technological development could outweigh these effects, resulting in combined wheat yield increases of 37–101% by the 2050s ( Ewert et al . 2005 ).
Even moderate levels of climate change may not necessarily confer benefits to agriculture without adaptation by producers, as an increase in the mean seasonal temperature can bring forward the harvest time of current varieties of many crops and hence reduce final yield without adaptation to a longer growing season.
In areas where temperatures are already close to the physiological maxima for crops, such as seasonally arid and tropical regions, higher temperatures may be more immediately detrimental, increasing the heat stress on crops and water loss by evaporation. A 2°C local warming in the mid-latitudes could increase wheat production by nearly 10 per cent whereas at low latitudes the same amount of warming may decrease yields by nearly the same amount ( figure 1 ). Different crops show different sensitivities to warming. It is important to note the large uncertainties in crop yield changes for a given level of warming ( figure 1 ). By fitting statistical relationships between growing season temperature, precipitation and global average yield for six major crops, Lobell & Field (2007) estimated that warming since 1981 has resulted in annual combined losses of 40 million tonne or US$5 billion (negative relationships between wheat, maize & barley with temperature).

Sensitivity of cereal (( a , b ) maize (mid- to high-latitude and low latitude), ( c , d ) wheat (mid- to high-latitude and low latitude) and ( e , f ) rice (mid- to high-latitude)) to climate change as determined from the results of 69 studies, against temperature change. Results with (green), and without (red) adaptation are shown. Reproduced from Easterling et al . (2007) , fig. 5.2.
Figure 2 and table 1 show two scenarios for changes in mean annual temperature at 2020 and 2050 relative to present day. All areas of cropland are projected to experience some degree of warming, but the largest change in warming is projected in the high latitudes. However, small increases in temperature in low latitudes may have a greater impact than in high latitudes ( figure 1 ), possibly because agriculture in parts of these regions is already marginal.
Table 1.
Scenarios of future change in meteorological, hydrological and plant physiological variables relevant to agricultural productivity, selected from an ensemble of 17 scenarios with variants of the HadCM3 climate model. Results are presented as means over global cropland areas for 30-year periods centred on 2020 and 2050, relative to 1970–2000 (except for extreme temperature which is relative to 2000). Two scenarios are presented for each variable, spanning the range of results for each variable to illustrate uncertainties in the projections. For further details see the electronic supplementary material.
2020 | 2050 | |
---|---|---|
°C | ||
scenario T1 | 1.3 | 2.8 |
scenario T2 | 0.8 | 1.8 |
mm d | ||
scenario P1 | 0.05 | 0.05 |
scenario P2 | −0.04 | −0.08 |
°C | ||
scenario ET1 | 1.1 | 2.9 |
scenario ET2 | 0.5 | 1.7 |
kg C m y | ||
without CO fertilization | −0.03 | −0.07 |
with CO fertilization | 0.09 | 0.17 |
scenario WS1 | 0.003 | 0.004 |
scenario WS2 | 0.010 | 0.015 |
mm d | ||
scenario R1 | −0.02 | −0.01 |
scenario R2 | 0.03 | 0.07 |
% of baseline | ||
scenario D1 | 11 | 12 |
scenario D2 | 20 | 22 |

Two projections of change in annual mean temperature (°C) over global croplands for 30-year means centred around 2020 and 2050, relative to 1970–2000. The two projections are the members of the ensemble with the greatest and least change in annual mean temperature averaged over all global croplands. See the electronic supplementary material for further details.
Water is vital to plant growth, so varying precipitation patterns have a significant impact on agriculture. As over 80 per cent of total agriculture is rain-fed, projections of future precipitation changes often influence the magnitude and direction of climate impacts on crop production ( Olesen & Bindi 2002 ; Tubiello et al . 2002 ; Reilly et al . 2003 ). The impact of global warming on regional precipitation is difficult to predict owing to strong dependencies on changes in atmospheric circulation, although there is increasing confidence in projections of a general increase in high-latitude precipitation, especially in winter, and an overall decrease in many parts of the tropics and sub-tropics ( IPCC 2007 ). These uncertainties are reflected in two scenarios shown in figure 3 and table 1 , which project different signs of precipitation change averaged over all croplands, even though there is agreement in the sign of change in some regions. One scenario which predicts an overall increase in precipitation, shows large increases in southern USA and India but also significant decreases in the tropics and sub-tropics. The other scenario also shows the decreases in the low latitudes but without significant increases in India.

Two projections of change in annual mean precipitation (mm d −1 ) over global croplands for 30-year means centred around 2020 and 2050, relative to 1970–2000. The two projections are the members of the ensemble with the most positive and negative changes in annual mean precipitation averaged over all global croplands. See the electronic supplementary material for further details.
This reflects the wide range of projections of precipitation change from different climate models ( Christensen et al . 2007 ). The differences in precipitation projections arise for a number of reasons. A key factor is the strong dependence on changes in atmospheric circulation which itself depends on the relative rates of warming in different regions, but there are often a number of factors influencing precipitation change projections in a given location. For example, the uncertainty in precipitation change over India arises partly from the expected weakening of the dynamical monsoon circulation (decreasing the Indian monsoon precipitation) versus the increase in atmospheric water content associated with warming (increasing the Indian monsoon precipitation; Meehl et al . 2007 ).
However, changes in seasonal precipitation may be more relevant to agriculture than annual mean changes. In India, climate models generally project a decrease in dry season precipitation and an increase during the rest of the year including the monsoon season, but still with a large inter-model spread ( Christensen et al . 2007 ).
Precipitation is not the only influence on water availability. Increasing evaporative demand owing to rising temperatures and longer growing seasons could increase crop irrigation requirements globally by between 5 and 20 per cent, or possibly more, by the 2070s or 2080s ( Döll 2002 ; Fisher et al . 2006 ), but with large regional variations—South-East Asian irrigation requirements could increase by 15 per cent ( Döll 2002) . Regional studies project increasing irrigation demand in the Middle East and North Africa ( Abou-Hadid et al . 2003 ) and potentially 15 per cent increases in irrigation demand in South-East Asia ( Arnell et al . 2004 ; Fisher et al . 2006 ). However, decreased requirements are projected in China ( Tao et al . 2003 ). Clearly these projections also depend on uncertain changes in precipitation.
(b) Climate variability and extreme weather events
While change in long-term mean climate will have significance for global food production and may require ongoing adaptation, greater risks to food security may be posed by changes in year-to-year variability and extreme weather events. Historically, many of the largest falls in crop productivity have been attributed to anomalously low precipitation events ( Kumar et al . 2004 ; Sivakumar et al . 2005 ). However, even small changes in mean annual rainfall can impact on productivity. Lobell & Burke (2008) report that a change in growing season precipitation by one standard deviation can be associated with as much as a 10 per cent change in production (e.g. millet in South Asia).
For example, Indian agriculture is highly dependent on the spatial and temporal distribution of monsoon rainfall ( Kumar et al . 2004 ). Asada & Matsumoto (2009) analysed the relationship between district-level crop yield data (rainy season ‘kharif’ rice) and precipitation for 1960–2000. It was shown that different regions were sensitive to precipitation extremes in different ways. Crop yield in the upper Ganges basin is linked to total precipitation during the relatively short growing season and is thus sensitive to drought. Conversely, the lower Ganges basin was sensitive to pluvial flooding and the Brahmaputra basin demonstrated an increasing effect of precipitation variability on crop yield, in particular drought. These relationships were not consistent through time, in part owing to precipitation trends. Variation between districts implied the importance of social factors and the introduction of irrigation techniques.
Meteorological records suggest that heatwaves became more frequent over the twentieth century, and while individual events cannot be attributed to climate change, the change in probability of a heatwave can be attributed. Europe experienced a particularly extreme climate event during the summer of 2003, with average temperatures 6°C above normal and precipitation deficits of up to 300 mm. A record crop yield loss of 36 per cent occurred in Italy for corn grown in the Po valley where extremely high temperatures prevailed ( Ciais et al . 2005 ). It is estimated that such summer temperatures in Europe are now 50 per cent more likely to occur as a result of anthropogenic climate change ( Stott et al . 2004 ).
As current farming systems are highly adapted to local climate, growing suitable crops and varieties, the definition of what constitutes extreme weather depends on geographical location. For example, temperatures considered extreme for grain growers in the UK would be considered normal for cereal growers in central France. In many regions, farming may adapt to increases in extreme temperature events by moving to practices already used in warmer climate, for example by growing more tolerant crops. However, in regions where farming exists at the edge of key thresholds increases in extreme temperatures or drought may move the local climate into a state outside historical human experience. In these cases it is difficult to assess the extent to which adaptation will be possible.
(i) Extreme temperatures
Recent increases in climate variability may have affected crop yields in countries across Europe since around the mid-1980s ( Porter & Semenov 2005 ) causing higher inter-annual variability in wheat yields. This study suggested that such changes in annual yield variability would make wheat a high-risk crop in Spain. Even mid-latitude crops could suffer at very high temperatures in the absence of adaptation. In 1972, extremely high summer averaged temperature in the former Soviet Union (USSR) contributed to widespread disruptions in world cereal markets and food security ( Battisti & Naylor 2009 ).
Changes in short-term temperature extremes can be critical, especially if they coincide with key stages of development. Only a few days of extreme temperature (greater that 32°C) at the flowering stage of many crops can drastically reduce yield ( Wheeler et al . 2000 ). Crop responses to changes in growing conditions can be nonlinear, exhibit threshold responses and are subject to combinations of stress factors that affect their growth, development and eventual yield. Crop physiological processes related to growth such as photosynthesis and respiration show continuous and nonlinear responses to temperature, while rates of crop development often show a linear response to temperature to a certain level. Both growth and developmental processes, however, exhibit temperature optima. In the short-term high temperatures can affect enzyme reactions and gene expression. In the longer term these will impact on carbon assimilation and thus growth rates and eventual yield. The impact of high temperatures on final yield can depend on the stage of crop development. Wollenweber et al . (2003) found that the plants experience warming periods as independent events and that critical temperatures of 35°C for a short-period around anthesis had severe yield reducing effects. However, high temperatures during the vegetative stage did not seem to have significant effects on growth and development. Reviews of the literature ( Porter & Gawith 1999 ; Wheeler et al . 2000 ) suggest that temperature thresholds are well defined and highly conserved between species, especially for processes such as anthesis and grain filling.
Although groundnut is grown in semi-arid regions which regularly experience temperatures of 40°C, if after flowering the plants are exposed to temperatures exceeding 42°C, even for short periods, yield can be drastically reduced ( Vara Prasad et al . 2003 ). Maize exhibits reduced pollen viability for temperatures above 36°C. Rice grain sterility is brought on by temperatures in the mid-30s and similar temperatures can lead to the reverse of the vernalizing effects of cold temperatures in wheat. Increases in temperature above 29°C for corn, 30°C for soya bean and 32°C for cotton negatively impact on yields in the USA.
Figure 4 and table 1 show that in all cases and all regions, one in 20-year extreme temperature events is projected to be hotter. Events which today are considered extreme would be less unusual in the future. The impacts of extreme temperature events can be difficult to separate from those of drought. However, key temperature thresholds exist beyond which crop physiology is altered, potentially devastating yields.

Two projections of change in one in 20-year extreme temperature level (°C) over global croplands for 2020 and 2050, relative to 2000. The two projections are the members of the ensemble with the greatest and least change averaged over all global croplands. See the electronic supplementary material for further details.
(ii) Drought
There are a number of definitions of drought, which generally reflect different perspectives. Holton et al . (2003) point out that ‘the importance of drought lies in its impacts. Thus definitions should be region-specific and impact- or application-specific in order to be used in an operational mode by decision makers.’ It is common to distinguish between meteorological drought (broadly defined by low precipitation), agricultural drought (deficiency in soil moisture, increased plant water stress), hydrological drought (reduced streamflow) and socio-economic drought (balance of supply and demand of water to society; Holton et al . 2003 ). Globally, the areas sown for the major crops of barley, maize, rice, sorghum, soya bean and wheat have all seen an increase in the percentage of area affected by drought as defined in terms of the Palmer Drought Severity Index (PDSI; Palmer 1965 ) since the 1960s, from approximately 5–10% to approximately 15–25% ( Li et al . 2009 ). Global mean PDSI has also increased ( IPCC 2007 ), and a comparison of climate model simulations with observed data suggests that anthropogenic increases in greenhouse gas and aerosol concentrations have made a detectable contribution to the observed drying trend in PDSI ( Burke et al . 2006 ).
In climate-modelling studies, Burke et al . (2006) define drought as the 20th percentile of the PDSI distribution over time, for pre-industrial conditions; this definition is therefore regionally specific. Therefore at any given time, approximately 20 per cent of the land surface will be defined as being in drought, but the conditions in a normally wet area under drought may still be less dry than those in another region which is dry under normal conditions. Using this definition, the MOHC climate model simulates the proportion of the land surface under drought to have increased from 20 to 28 per cent over the twentieth century ( Burke et al . 2006 ).
Li et al . (2009) define a yield reduction rate (YRR) which takes a baseline of the long-term trend in yield (assumed to be owing to technological progress and infrastructure improvement) and compares this with actual annual yields to define a YRR owing to climate variability. Using national-scale data for the four major grains (barley, maize, rice and wheat), Li et al . (2009) suggested that 60–75% of observed YRRs can be explained by a linear relationship between YRR and a drought risk index based on the PDSI. Present-day mean YRR values are diagnosed as ranging from 5.82 per cent (rice) to 11.98 per cent (maize). By assuming the linear relationship between the drought risk index and YRR holds into the future, Li et al . (2009) estimated that drought related yield reductions would increase by more than 50 per cent by 2050 for the major crops.
The impacts of drought may offset benefits of increased temperature and season length observed at mid to high latitudes. Using models of global climate, crop production and water resources, Alcamo et al . (2007) suggested that decreased crop production in some Russian regions could be compensated by increased production in others, resulting in relatively small average changes. However, their results indicate that the frequency of food production shortfalls could double in many of the main crop growing areas in the 2020s, and triple in the 2070s ( Alcamo et al . 2007 ). Although water availability in Russia is increasing on average, the water resources model predicted more frequent low run-off events in the already dry crop growing regions in the south, and a significantly increased frequency of high run-off events in much of central Russia ( Alcamo et al . 2007 ).
(iii) Heavy rainfall and flooding
Food production can also be impacted by too much water. Heavy rainfall events leading to flooding can wipe out entire crops over wide areas, and excess water can also lead to other impacts including soil water logging, anaerobicity and reduced plant growth. Indirect impacts include delayed farming operations ( Falloon & Betts in press ). Agricultural machinery may simply not be adapted to wet soil conditions. In a study looking at the impacts of current climate variability, Kettlewell et al . (1999) showed that heavy rainfall in August was linked to lower grain quality which leads to sprouting of the grain in the ear and fungal disease infections of the grain. This was shown to affect the quality of the subsequent products such that it influenced the amount of milling wheat that was exported from the UK. The proportion of total rain falling in heavy rainfall events appears to be increasing, and this trend is expected to continue as the climate continues to warm. A doubling of CO 2 is projected to lead to an increase in intense rainfall over much of Europe. In the higher end projections, rainfall intensity increases by over 25 per cent in many areas important for agriculture ( figure 5 ).

( a ) Lower and ( b ) upper estimates covering the central 80% range of changes in precipitation intensity on wet days with a 1 year return period for a doubling of CO 2 .
(iv) Tropical storms
A tropical cyclone is the generic term for a non-frontal synoptic scale low-pressure system over tropical or sub-tropical waters with organized convection (i.e. thunderstorm activity) and definite cyclonic surface wind circulation ( Holland 1993 ). Severe tropical cyclones, with maximum sustained wind speeds of at least 74 mph, are known as ‘hurricanes’ in the eastern North Pacific and North Atlantic and ‘typhoons’ in the western North Pacific. The strongest tropical cyclones can reach wind speeds as large as 190 mph, as recorded in Typhoon Tip in the western North Pacific in October 1979. Tropical cyclones usually occur during the summer and early autumn: around May–November in the Northern Hemisphere and November–April in the Southern Hemisphere, although tropical cyclones are observed all year round in the western North Pacific. The North Indian Ocean is the only basin to have a two-part tropical cyclone season: before and after the onset of the South Asian monsoon, from April to May and October to November, respectively.
Figure 6 shows observed tropical cyclone tracks for all known storms over the period 1945–2008. In this context, the most vulnerable agricultural regions are found, among others, in the USA, China, Vietnam, India, Bangladesh, Myanmar and Madagascar.

Observed tropical cyclone tracks and intensity for all known storms over the period 1947–2008. Tracks are produced from the IBTrACS dataset of NOAA/NCDC ( Knapp et al . 2010 ).
Both societal and economic implications of tropical cyclones can be high, particularly in developing countries with high population growth rates in vulnerable tropical and subtropical regions. This is particularly the case in the North Indian Ocean, where the most vulnerable people live in the river deltas of Myanmar, Bangladesh, India and Pakistan; here population growth has resulted in increased farming in coastal regions most at risk from flooding ( Webster 2008 ). In 2007, cyclone Sidr hit Bangladesh costing 3500 lives ( United Nations 2007 ), and in 2008 cyclone Nargis caused 130 000 deaths in Myanmar. The agricultural impacts of these and other recent cyclones are shown in table 2 .
Table 2.
Selected tropical cyclones of the past decade, and their agricultural impacts.
date | location | cyclone name | agricultural impact |
---|---|---|---|
Feb–Apr 2000 | Madagascar | Eline, Gloria (Feb), Hudah (Apr) | combined losses owing to three cyclones: 149 441 hectares rice (7% of annual production), 5000 hectares maize, 155 000 hectares cereals ( ) |
2006–2007 | Madagascar | Bondo (Dec 2006), Clovis (Jan 2007), Favio (Jan 2007), Gamede (Feb 2007), Indlala (Mar 2007) | combined losses: 90 000 hectares of crop ( ); 80% of vanilla production lost to Indlala alone ( ) |
2007 | Mozambique | Favio | thousands of hectares of crop destroyed ( ) |
Nov 2007 | Bangladesh | Sidr | 1.6 million acres of cropland damaged; >25% winter rice crop destroyed ( ) |
May 2008 | Irrawaddy Delta, Myanmar (Burma) | Nargis | estimated 4 m storm surge inundated coastal areas and regions up to 40 km inland ( ). Soil salination made 50 000 acres of rice cropland now unfit for planting ( ). Loss of rice seed, fertilizers, farm machinery, and valuable land threatened the winter 2008/09 rice crop including exports to neighbouring countries ( ) |
Although many studies focus on the negative impacts, tropical cyclones can also bring benefits. In many arid regions in the tropics, a large portion of the annual rain comes from cyclones. It is estimated that tropical cyclones contribute to 15–20% of South Florida's annual rainfall ( Walther & Abtew 2006 ), which can temporarily end severe regional droughts. Examples of such storms are hurricane Gabrielle (2001) and tropical storm Fay (2008), which provided temporary relief from the 2000–2001 and 2006–2009 droughts, respectively. As much as 15 inches of rainfall was recorded in some regions from tropical storm Fay, without which, regions would have faced extreme water shortage, wildfires and potential saltwater intrusion into coastal freshwater aquifers ( Abtew et al . 2009 ). Tropical cyclones can also help replenish water supplies to inland regions: cyclone Eline, which devastated agriculture in Madagascar in February 2000, later made landfall in southern Africa and contributed significantly to the rainfall in the semi-desert region of southern Namibia.
There is much debate on the global change in tropical cyclone frequency and intensity under a warming climate. Climate modelling studies contributing to the IPCC's Fourth Assessment Report (AR4) suggest tropical cyclones may become more intense in the future with stronger winds and heavier precipitation ( Meehl et al . 2007 ). This is in agreement with more recent studies using high resolution models, which also indicate a possible decrease in future global tropical cyclone frequency ( McDonald et al . 2005 ; Bengtsson et al . 2007 ; Gualdi et al . 2008 ). However, there is limited consensus among the models on the regional variations in tropical cyclone frequency.
3. Indirect impacts of climate change on agricultural productivity
(a) pests and diseases.
Rising atmospheric CO 2 and climate change may also impact indirectly on crops through effects on pests and disease. These interactions are complex and as yet the full implications in terms of crop yield are uncertain. Indications suggest that pests, such as aphids ( Newman 2004 ) and weevil larvae ( Staley & Johnson 2008 ), respond positively to elevated CO 2 . Increased temperatures also reduced the overwintering mortality of aphids enabling earlier and potentially more widespread dispersion ( Zhou et al . 1995 ). Evidence suggests that in sub-Saharan Africa migration patterns of locusts may be influenced by rainfall patterns ( Cheke & Tratalos 2007 ) and thus potential exists for climate change to shape the impacts of this devastating pest. Pathogens and disease may also be affected by a changing climate. This may be through impacts of warming or drought on the resistance of crops to specific diseases and through the increased pathogenicity of organisms by mutation induced by environmental stress ( Gregory et al . 2009 ). Over the next 10–20 years, disease affecting oilseed rape could increase in severity within its existing range as well as spread to more northern regions where at present it is not observed ( Evans et al . 2008 ). Changes in climate variability may also be significant, affecting the predictability and amplitude of outbreaks.
(b) Changes in water availability owing to remote climate changes
Climate changes remote from production areas may also be critical. Irrigated agricultural land comprises less than one-fifth of all cropped area but produces between 40 and 45 per cent of the world's food ( Döll & Siebert 2002 ), and water for irrigation is often extracted from rivers which depend upon distant climatic conditions. For example, agriculture along the Nile in Egypt depends on rainfall in the upper reaches of the Nile such as the Ethiopian Highlands.
Figure 7 shows the projected changes in monthly river-flow for the 2020s and 2050s for selected key rivers of interest in this context. In some rivers such as the Nile, climate change increases flow throughout the year which could confer benefits to agriculture. However, in other catchments, e.g. the Ganges, the increase in run-off comes as an increase in peak flow around the monsoon. However, dry season river-flow is still very low. Without sufficient storage of peak season flow, water scarcity may affect agricultural productivity despite overall increases in annual water availability. Increases at peak flow may also cause damage to crop lands through flooding.

Projected mean monthly river flow (kg s −1 ) for 30 year means centred on 2000 (black), 2020 (green) and 2050 (blue) for the ( a ) Nile, ( b ) Ganges and ( c ) Volga. Projections are bias corrected ensemble means from the Hadley Centre models. See the electronic supplementary material for further details.
Figure 8 shows areas in the world where river flow is dominated by snow melt. These areas are mostly at mid to high latitudes where predictions for warming are greatest. Warming in winter means that less precipitation falls as snow and that which accumulates melts earlier in the year. Changing patterns of snow cover fundamentally alter how such systems store and release water. Changes in the amount of precipitation affect the volume of run-off, particularly near the end of the winter at the onset of snow melt. Temperature changes mostly affect the timing of run-off with earlier peak flow in the spring. Although additional river-flow can be considered beneficial to agriculture this is only true if there is an ability to store run-off during times of excess to use later in the growing season. Globally, only a few rivers currently have adequate storage to cope with large shifts in seasonality of run-off ( Barnett et al . 2005 ). Where storage capacities are not sufficient, much of the winter run-off will immediately be lost to the oceans. Figure 7 c shows the monthly river-flow from the Volga catchment in Russia. It shows an earlier and increased peak flow around snow melt with subsequently lower flow later in the year.

The fraction of run-off originating as snowfall. The red lines indicate the regions where streamflow is snowmelt-dominated, and where there is not adequate reservoir storage capacity to buffer shifts in the seasonal hydrograph. The black lines indicate additional areas where water availability is predominantly influenced by snowmelt generated upstream (but run-off generated within these areas is not snowmelt-dominated). Reproduced from Barnett et al . (2005) with permission from Macmillan Publishers Ltd: Nature.
Some major rivers, such as the Indus and Ganges, are fed by mountain glaciers, with approximately one-sixth of the world's population currently living in glacier-fed river basins ( Stern 2007 ). Populations are projected to rise significantly in major glacier-fed river basins such as the Indo-Gangetic plain. As such, changes in remote precipitation and the magnitude and seasonality of glacial melt waters could therefore potentially impact food production for many people.
The majority of observed glaciers around the globe are undergoing shrinkage ( Zemp et al. 2008 ). Formerly attributing this retreat to recent warming is not currently possible. However, there is a broad consensus that warming is a primary cause of retreat, although changes in atmospheric moisture particularly in the tropics may be contributing ( Bates et al . 2008 ). Melting glaciers will initially increase river-flow although the seasonality of flow will be enhanced ( Juen et al . 2007 ) bringing with it an increased flood risk. In the long term, glacial retreat is expected to be enhanced further leading to eventual decline in run-off, although the greater time scale of this decline is uncertain. The Chinese Glacier Inventory catalogued 46 377 glaciers in western China, with approximately 15 000 glaciers in the Himalayas. In total these glaciers store an estimated 12 000 km 3 of fresh water ( Ding et al . 2006 ; Cruz et al . 2007 ). Analysis of glaciers in the western Himalayas demonstrates evidence of glacial thinning ( Berthier et al . 2007 ), and radioactive bomb deposits from one high altitude glacier show no net accumulation since 1950 ( Kehrwald et al . 2008 ). The limited number of direct observations also supports evidence of a glacial retreat in the Himalayas ( Zemp et al. 2008 ). The water from these glaciers feeds large rivers such as the Indus, Ganges and Brahmaputra and is likely to be contributing a significant proportion of seasonal river flow although the exact magnitude is unknown. Currently nearly 500 million people are reliant on these rivers for domestic and agricultural water resources. Climate change may mean the Indus and Ganges become increasingly seasonal rivers, ceasing to flow during the dry season ( Kehrwald et al . 2008 ). Combined with a rising population this means that water scarcity in the region would be expected to increase in the future.
(c) Mean sea-level rise
Sea-level rise is an inevitable consequence of a warming climate owing to a combination of thermal expansion of the existing mass of ocean water and addition of extra water owing to the melting of land ice. This can be expected to eventually cause inundation of coastal land, especially where the capacity for introduction or modification of sea defences is relatively low or non-existent. Regarding crop productivity, vulnerability is clearly greatest where large sea-level rise occurs in conjunction with low-lying coastal agriculture. Many major river deltas provide important agricultural land owing to the fertility of fluvial soils, and many small island states are also low-lying. Increases in mean sea level threaten to inundate agricultural lands and salinize groundwater in the coming decades to centuries, although the largest impacts may not be seen for many centuries owing to the time required to melt large ice sheets and for warming to penetrate into the deep ocean.
The potential sea-level rise associated with melting of the main ice sheets would be 5 m for West Antarctic Ice Sheet (WAIS), 60 m for East Antarctic Ice Sheet (EAIS), and 7 m for Greenland Ice Sheet (GIS), with both the GIS and WAIS considered vulnerable. Due to the possible rate of discharge of these ice sheets, and past maximal sea-level rise (under similar climatic conditions) a maximum eustatic sea-level rise of approximately 2 m by 2100 is considered physically plausible, but very unlikely ( Pfeffer et al . 2008 ; Rohling et al . 2008 ; Lowe et al . 2009 ).
Short-lived storm surges can also cause great devastation, even if land is not permanently lost. There has been relatively little work assessing the impacts of either mean sea-level rise or storm surges on agriculture.
4. Non-climate impacts related to greenhouse gas emissions: impacts of changes in atmospheric composition
(a) co 2 fertilization.
As well as influencing climate through radiative forcing, increasing atmospheric CO 2 concentrations can also directly affect plant physiological processes of photosynthesis and transpiration ( Field et al . 1995 ). Therefore any assessment of the impacts of CO 2 -induced climate change on crop productivity should account for the modification of the climate impact by the CO 2 physiological impact. The CO 2 physiological response varies between species, and in particular, two different pathways of photosynthesis (named C 3 and C 4 ) have evolved and these affect the overall response. The difference lies in whether ribulose-1,5-bisphosphate carboxylase–oxygenase (RuBisCO) within the plant cells is saturated by CO 2 or not. In C 3 plants, RuBisCO is not CO 2 -saturated in present day atmospheric conditions, so rising CO 2 concentrations increase net uptake of carbon and thus growth. The RuBisCO enzyme is highly conserved in plants and as such it is thought that the response of all C 3 crops including wheat and soya beans will be comparable. Theoretical estimates suggest that increasing atmospheric CO 2 concentrations to 550 ppm, could increase photosynthesis in such C 3 crops by nearly 40 per cent ( Long et al . 2004 ). The physiology of C 4 crops, such as maize, millet, sorghum and sugarcane is different. In these plants CO 2 is concentrated to three to six times atmospheric concentrations and thus RuBisCO is already saturated ( von Caemmerer & Furbank 2003 ). Thus, rising CO 2 concentrations confer no additional physiological benefits. These crops may, however, become more water-use efficient at elevated CO 2 concentrations as stomata do not need to stay open as long for the plant to receive the required CO 2. Thus yields may increase marginally as a result ( Long et al . 2004 ).
Many studies suggest that yield rises owing to this CO 2 -fertilization effect and these results are consistent across a range of experimental approaches including controlled environment closed chambers, greenhouse, open and closed field top chambers, and free-air carbon dioxide enrichment (FACE) experiments ( Tubiello et al . 2007 ). Experiments under idealized conditions show that a doubling of atmospheric CO 2 concentration increases photosynthesis by 30–50% in C 3 plant species and 10–25% in C 4 species ( Ainsworth & Long 2005 ). Crop yield increase is lower than the photosynthetic response; increases of atmospheric CO 2 to 550 ppm would on average increase C 3 crop yields by 10–20% and C 4 crop yields by 0–10% ( Gifford 2004 ; Long et al . 2004 ; Ainsworth & Long 2005 ).
Some authors argue that crop response to elevated CO 2 may be lower than previously thought, with consequences for crop modelling and projections of food supply (Long et al . 2004 , 2009 ). Plant physiologists and modellers alike recognize that the effects of elevated CO 2 , as measured in experimental settings and subsequently implemented in models, may overestimate actual field and farm level responses. This is because of many limiting factors such as pests and weeds, nutrients, competition for resources, soil water and air quality which are neither well understood at large scales, nor well implemented in leading models.
Despite the potential positive effects on yield quantities, elevated CO 2 may, however, be detrimental to yield quality of certain crops. For example, elevated CO 2 is detrimental to wheat flour quality through reductions in protein content ( Sinclair et al . 2000 ).
Figure 9 and table 1 show the impact of including CO 2 physiological effects in projections of plant productivity in agricultural regions. Without CO 2 fertilization, many regions, especially in the low latitudes, suffer a decrease in productivity by 2050. In contrast, by including CO 2 fertilization all but the very driest regions show increases in productivity.

Two projections of future change in net primary productivity (kg C m −2 yr −1 ) over global croplands for 30-year means centred around 2020 and 2050, relative to 1970–2000. The two projections show the impact of including CO 2 physiological effects and are the members of the ensemble with the most positive and negative changes in productivity averaged over all global croplands. See the electronic supplementary material for further details.
Global-scale comparisons of the impacts of CO 2 fertilization with those of changes in mean climate ( Parry et al . 2004 ; Nelson et al . 2009 ) show that the strength of CO 2 fertilization effects is a critical factor in determining whether global-scale yields are projected to increase or decrease. If CO 2 fertilization is strong, North America and Europe may benefit from climate change at least in the short term ( figure 10 ). However, regions such as Africa and India are nevertheless still projected to experience up to 5 per cent losses by 2050, even with strong CO 2 fertilization. These losses increase to up to 30 per cent if the effects of CO 2 fertilization are omitted. In fact without CO 2 fertilization all regions are projected to experience a loss in productivity owing to climate change by 2050. However, existing global scale studies ( Parry et al . 2004 ; Nelson et al . 2009 ) have only used a limited sample of available climate model projections.

Potential changes (%) in national cereal yields for the 2020s and 2050s relative to 1990, with climate change projected by the HadCM3 model under the A1FI scenario ( a ) with and ( b ) without CO 2 fertilization. Reproduced from Parry et al . (2004) with permission from Elsevier.
A reduction in CO 2 emissions would be expected to reduce the positive effect of CO 2 fertilization on crop yields more rapidly than it would mitigate the negative impacts of climate change. Even if GHG concentrations rose no further, there is a commitment to a certain amount of further global warming ( IPCC 2007 ). Stabilization of CO 2 concentrations would therefore halt any increase in the impacts of CO 2 fertilization, while the impacts of climate change could still continue to grow. Therefore in the short term the impacts on global food production could be negative. However, estimates suggest that stabilizing CO 2 concentrations at 550 ppm would significantly reduce production losses by the end of the century ( Arnell et al . 2002 ; Tubiello & Fisher 2006 ).
For all species higher water-use efficiencies and greater root densities under elevated CO 2 in field systems may, in some cases, alleviate drought pressures, yet their large-scale implications are not well understood ( Wullschleger et al . 2002 ; Norby et al . 2004 ; Centritto 2005 ). This could offset some of the expected warming-induced increase in evaporative demand, thus easing the pressure for more irrigation water. This may also alter the relationship between meteorological drought and agricultural/hydrological drought; an increase in meteorological drought may result in a smaller increase in agricultural or hydrological drought owing to increased water-use efficiency of plants ( Betts et al . 2007 ).
Soil moisture and run-off may be more relevant than precipitation and meteorological drought indices as metrics of water resource availability, as these represent the water actually available for agricultural use. These quantities are routinely simulated by physically based climate models as a necessary component of the hydrological cycle. Figure 11 and table 1 show two scenarios of projected changes in soil moisture as a fraction of that required to prevent plant stress. The available soil moisture fraction is projected to increase on average across global croplands ( table 1 ), with increases in some regions, particularly the mid-latitudes, but decrease in others, particularly in the tropics. Similarly, run-off increases in some regions and decreases in others ( figure 12 ), but the mean change across global croplands varies in sign between scenarios ( table 1 ). Importantly, the scenarios with an increase in mean run-off and the greatest increase in available soil moisture included the effects of CO 2 fertilization in the model, while those with a decrease in mean run-off and the smallest increase in soil moisture availability did not include this effect ( Betts et al . 2007 ).

Two projections of future change in soil moisture as a fraction of that required to prevent plant water stress over global croplands for 30-year means centred around 2020 and 2050, relative to 1970–2000. Positive values indicate increased water availability. The two projections are the members of the ensemble with the greatest and least change averaged over all global croplands. See the electronic supplementary material for further details.

Two projections of future change in annual mean run-off (mm d −1 ) over global croplands for 30-year means centred around 2020 and 2050, relative to 1970–2000. The two projections are the members of the ensemble with the most positive and negative changes in annual mean run-off averaged over all global croplands. See the electronic supplementary material for further details.
However, as discussed in §2 b , changes in extremes are also important, and agricultural drought may be more critical than annual mean soil moisture availability. With drought defined as the driest 20th percentile of the distribution in soil moisture over time in any given location, the model ensemble used here consistently projects an increase in the time spent under drought in most regions for the first half of the twenty-first century ( figure 13 and table 1 ).

Two projections of percentage change in time spent under meteorological drought as defined in terms of soil moisture in global croplands for 30-year means centred around 2020 and 2050, relative to 2000. The two projections are the members of the ensemble with the greatest and least percentage change averaged over all global croplands. See the electronic supplementary material for further details.
Ozone is a major secondary air-pollutant, which at current concentrations has been shown to have significant negative impacts on crop yields ( Van Dingenen et al . 2009 ). Whereas in North America and Europe, emissions of ozone precursors are decreasing, in other regions of the world, especially Asia, they are increasing rapidly ( Van Dingenen et al . 2009 ).
Ozone reduces agricultural yield through several mechanisms. Firstly, acute and visible injury to products such as horticultural crops reduces market value. Secondly, ozone reduces photosynthetic rates and accelerates leaf senescence which in turn impacts on final yield. In Europe and North America many studies have investigated such yield reductions (e.g. Morgan et al . 2003 ). However, in other regions, such as Asia, little evidence currently exists. Thus, our understanding of the impacts in such regions is limited.
5. Conclusions
Anthropogenic greenhouse gas emissions and climate change have a number of implications for agricultural productivity, but the aggregate impact of these is not yet known and indeed many such impacts and their interactions have not yet been reliably quantified, especially at the global scale. An increase in mean temperature can be confidently expected, but the impacts on productivity may depend more on the magnitude and timing of extreme temperatures. Mean sea-level rise can also be confidently expected, which could eventually result in the loss of agricultural land through permanent inundation, but the impacts of temporary flooding through storm surges may be large although less predictable.
Freshwater availability is critical, but predictability of precipitation is highly uncertain and there is an added problem of lack of clarity on the relevant metric for drought—some studies including IPCC consider metrics based on local precipitation and temperature such as the Palmer Drought Severity Index, but this does not include all relevant factors. Agricultural impacts in some regions may arise from climate changes in other regions, owing to the dependency on rivers fed by precipitation, snowmelt and glaciers some distance away. Drought may also be offset to some extent by an increased efficiency of water use by plants under higher CO 2 concentrations, although the impact of this again is uncertain especially at large scales. The climate models used here project an increase in annual mean soil moisture availability and run-off in many regions, but nevertheless across most agricultural areas there is a projected increase in the time spent under drought as defined in terms of soil moisture.
Moreover, even the sign of crop yield projections is uncertain as this depends critically on the strength of CO 2 fertilization and also O 3 damage. Few studies have assessed the response of crop yields to CO 2 fertilization and O 3 pollution under actual growing conditions, and consequently model projections are poorly constrained. Indirect effects of climate change through pests and diseases have been studied locally but a global assessment is not yet available. Overall, it does not appear to be possible at the present time to provide a robust assessment of the impacts of anthropogenic climate change on global-scale agricultural productivity.
Acknowledgements
We are grateful to Simon Brown, Ian Crute, Diogo de Gusmão, Keith Jaggard, Doug McNeall, Erika Palin, Doug Smith and Jonathan Tinker for useful discussions.
One contribution of 23 to a Theme Issue ‘ Food security: feeding the world in 2050 '.
- Abou-Hadid A. F., Mougou R., Mokssit A., Iglesias A.2003 Assessment of impacts, adaptation and vulnerability to climate change in North Africa: food production and water resources. AIACC AF90 Semi-Annual Progress Report . [ Google Scholar ]
- Abtew W., Pathak C., Scott Huebner R., Ciuca V.2009 Hydrology of the South Florida Environment. In 2009 South Florida Environmental Report, South Florida Water Management District, West Palm Beach, FL. vol. I, ch. 2 . [ Google Scholar ]
- Ainsworth E. A., Long S. P.2005 What have we learned from 15 years of free-air CO 2 enrichment (FACE)? A meta-analytic review of the responses of photosynthesis, canopy . New Phytol. 165 , 351–371 ( doi:10.1111/j.1469-8137.2004.01224.x ) [ PubMed ] [ Google Scholar ]
- Alcamo J., Dronin N., Endejan M., Golubev G., Kirilenkoc A.2007 A new assessment of climate change impacts on food production shortfalls and water availability in Russia . Global Environ. Change—Hum. Policy Dimens. 17 , 429–444 [ Google Scholar ]
- Alexandrov V., Eitzinger J., Cajic V., Oberforster M.2002 Potential impact of climate change on selected agricultural crops in north-eastern Austria . Global Change Biol. 8 , 372–389 ( doi:10.1046/j.1354-1013.2002.00484.x ) [ Google Scholar ]
- Arnell N. W., Cannell M. G. R., Hulme M., Kovats R. S., Mitchell J. F. B., Nicholls R. J., Parry M. L., Livermore M. T. J., White A.2002 The consequences of CO 2 stabilisation for the impacts of climate change . Clim. Change 53 , 413–446 ( doi:10.1023/A:1015277014327 ) [ Google Scholar ]
- Arnell N. W., Livermore M. J. L., Kovats S., Levy P. E., Nicholls R., Parry M. L., Gaffin S. R.2004 Climate and socio-economic scenarios for global-scale climate change impacts assessments: characterising the SRES storylines . Global Environ. Change—Hum. Policy Dimens. 14 , 3–20 [ Google Scholar ]
- Asada H., Matsumoto J.2009 Effects of rainfall variation on rice production in the Ganges–Brahmaputra Basin . Clim. Res. 38 , 249–260 [ Google Scholar ]
- Audsley E., Pearn K. R., Simota C., Cojocaru G., Koutsidou E., Rounsevell M. D. A., Trnka M., Alexandrov V.2006 What can scenario modelling tell us about future European scale agricultural land-use, and what not? Environ. Sci. Policy 9 , 148–162 ( doi:10.1016/j.envsci.2005.11.008 ) [ Google Scholar ]
- Barnett T. P., Adam J. C., Lettenmaier D. P.2005 Potential impacts of a warming climate on water availability in snow-dominated regions . Nature 438 , 303–309 ( doi:10.1038/nature04141 ) [ PubMed ] [ Google Scholar ]
- Bates B. C., Kundzewicz Z. W., Wu S., Palutikof J. P.2008 Climate change and water. Technical Paper of the Intergovernmental Panel on Climate Change . Geneva, Switzerland: IPCC Secretariat [ Google Scholar ]
- Battisti D. S., Naylor R. L.2009 Historical warnings of future food insecurity with unprecedented seasonal heat . Science 323 , 240–244 ( doi:10.1126/science.1164363 ) [ PubMed ] [ Google Scholar ]
- Bengtsson L., Hodges K. I., Esch M., Keenlyside N., Kornblueh L., Luo J. J., Yamagata T.2007 How may tropical cyclones change in a warmer climate? Tellus 59 , 539–561 [ Google Scholar ]
- Berthier E., Arnaud Y., Kumar R., Ahmad S., Wagnon P., Chevallier P.2007 Remote sensing estimates of glacier mass balances in the Himachal Pradesh (Western Himalaya, India) . Remote Sensing Environ. 108 , 327–338 ( doi:10.1016/j.rse.2006.11.017 ) [ Google Scholar ]
- Betts R. A., et al.2007 Projected increase in future river runoff through plant responses to carbon dioxide rise . Nature 448 , 1037–1042 ( doi:10.1038/nature06045 ) [ PubMed ] [ Google Scholar ]
- Burke E. J., Brown S. J., Christidis N.2006 Modeling the recent evolution of global drought and projections for the twenty-first century with the Hadley Centre climate model . J. Hydrometeorol. 7 , 1113–1125 ( doi:10.1175/JHM544.1 ) [ Google Scholar ]
- Centritto M.2005 Photosynthetic limitations and carbon partitioning in cherry in response to water deficit and elevated [CO 2 ] . Agric. Ecosyst. Environ. 106 , 233–242 ( doi:10.1016/j.agee.2004.10.011 ) [ Google Scholar ]
- Cheke R. A., Tratalos J. A.2007 Migration, patchiness, and population processes illustrated by two migrant pests . Bioscience 57 , 145–154 ( doi:10.1641/B570209 ) [ Google Scholar ]
- Christensen J. H., et al.2007 Regional climate projections . In Climate change 2007: the physical science basis. Contribution of Working Group I to the Fourth Assessment Report of the Intergovernmental Panel on Climate Change (eds Solomon S., Qin D., Manning M., Chen Z., Marquis M., Averyt K. B., Tignor M., Miller H. L.), pp. 847–940 Cambridge, UK: Cambridge University Press [ Google Scholar ]
- Ciais P., et al.2005 Europe-wide reduction in primary productivity caused by the heat and drought in 2003 . Nature 437 , 529–533 ( doi:10.1038/nature03972 ) [ PubMed ] [ Google Scholar ]
- Cruz R. V., et al.2007 Asia. Climate change 2007: impacts, adaptation and vulnerability . In Contribution of Working Group II to the Fourth Assessment Report of the Intergovernmental Panel on Climate Change (eds Parry M. L., Canziani O. F., Palutikof J. P., van der Linden P. J., Hanson C. E.), pp. 469–506 Cambridge, UK: Cambridge University Press [ Google Scholar ]
- Ding Y., Liu S., Li J., Shangguan D.2006 The retreat of glaciers in response to recent climate warming in western China . Ann. Glaciol. 43 , 97–105 ( doi:10.3189/172756406781812005 ) [ Google Scholar ]
- Döll P.2002 Impact of climate change and variability on irrigation requirements: a global perspective . Clim. Change 54 , 269–293 ( doi:10.1023/A:1016124032231 ) [ Google Scholar ]
- Döll P., Siebert S.2002 Global modeling of irrigation water requirements . Water Resour. Res. 38 ( doi:10.1029/2001WR000355 ) [ Google Scholar ]
- Easterling W. E., et al.2007 Food, fibre and forest products . In Climate change 2007: impacts, adaptation and vulnerability. Contribution of Working Group II to the Fourth Assessment Report of the Intergovernmental Panel on Climate Change (eds Parry M. L., Canziani O. F., Palutikof J. P., Linden P. J. v. d., Hanson C. E.), pp. 273–313 Cambridge, UK: Cambridge University Press [ Google Scholar ]
- Evans N., Baierl A., Semenov M. A., Gladders P., Fitt B. D. L.2008 Range and severity of a plant disease increased by global warming . J. R. Soc. Interface 5 , 525–531 ( doi:10.1098/rsif.2007.1136 ) [ PMC free article ] [ PubMed ] [ Google Scholar ]
- Ewert F., Rounsevell M. D. A., Reginster I., Metzger M. J., Leemans R.2005 Future scenarios of European agricultural land use I. Estimating changes in crop productivity . Agric. Ecosyst. Environ. 107 , 101–116 ( doi:10.1016/j.agee.2004.12.003 ) [ Google Scholar ]
- Falloon P. D., Betts R.In press Climate impacts on European agriculture and water management in the context of adaptation and mitigation—the importance of an integrated approach . Sci. Total Environ. ( doi:10.1016/j.scitotenv.2009.05.002 ) [ PubMed ] [ Google Scholar ]
- FAO 2000 FAO/WFP mission to assess the impact of cyclones and drought on the food supply situation in Madagascar . See http://www.fao.org/docrep/004/X7379E/X7379E00.HTM
- FAO 2007 Floods and cyclones cause heavy crop losses in parts of southern Africa . See http://www.fao.org/newsroom/en/news/2007/1000518/index.html
- FAO 2009 FAO/WFP crop and food security assessment mission to Myanmar . See http://www.fao.org/docrep/011/ai478e/ai478e00.htm
- Field C., Jackson R., Mooney H.1995 Stomatal responses to increased CO 2 : implications from the plant to the global scale . Plant Cell Environ. 18 , 1214–1255 ( doi:10.1111/j.1365-3040.1995.tb00630.x ) [ Google Scholar ]
- Fischer G., Shah M., Tubiello F. N., van Velhuizen H.2005 Socio-economic and climate change impacts on agriculture: an integrated assessment, 1990–2080 . Phil. Trans. R. Soc. B 360 , 2067–2083 ( doi:10.1098/rstb.2005.1744 ) [ PMC free article ] [ PubMed ] [ Google Scholar ]
- Fischer G., Tubiello F., Van Velhuizen H., Wiberg D.2006 Climate change impacts on irrigation water requirements: effects of mitigation, 1990–2989 . Technol. Forecasting Soc. Change 74 , 1083–1107 ( doi:10.1016/j.techfore.2006.05.021 ) [ Google Scholar ]
- Gifford R. M.2004 The CO 2 fertilising effect—does it occur in the real world? The international free air CO 2 enrichment (FACE) workshop: short- and long-term effects of elevated atmospheric CO 2 on managed ecosystems, Ascona, Switzerland, March 2004 . New Phytol. 163 , 221–225 ( doi:10.1111/j.1469-8137.2004.01133.x ) [ PubMed ] [ Google Scholar ]
- Gregory P. J., Johnson S. N., Newton A. C., Ingram J. S. I.2009 Integrating pests and pathogens into the climate change/food security debate . J. Exp. Bot. 60 , 2827–2838 ( doi:10.1093/jxb/erp080 ) [ PubMed ] [ Google Scholar ]
- Gualdi S., Scoccimarro E., Navarra A.2008 Changes in tropical cyclone activity due to global warming: results from a high-resolution coupled general circulation model . J. Clim. 21 , 5204–5228 ( doi:10.1175/2008JCLI1921.1 ) [ Google Scholar ]
- Hildén M., Lehtonen H., Bärlund I., Hakala K., Kaukoranta T., Tattari S.2005 The practice and process of adaptation in Finnish agriculture . FINADAPT Working Paper 5, Finnish Environment Institute Mimeographs , no. 335 Helsinki, Finland:Finnish Environment Institute [ Google Scholar ]
- Holland G. J.1993 The global guide to tropical cyclone forecasting . Geneva, Switzerland: World Meteorological Organization [ Google Scholar ]
- Holton J. R., Curry J. A., Pyle J. A.2003 Encyclopedia of atmospheric sciences . New York, NY: Academic Press [ Google Scholar ]
- IFRC 2007 Madagascar: cyclone Indlala . See http://www.reliefweb.int/rw/rwb.nsf/db900sid/JBRN-6ZRGP7?OpenDocument&query=cyclone%20indlala [ Google Scholar ]
- IPCC 2007 Climate change 2007: the physical science basis. Contribution of Working Group I to the Fourth Assessment Report of the Intergovernmental Panel on Climate Change . Cambridge, UK: Cambridge University Press [ Google Scholar ]
- Juen I., Kaser G., Georges C.2007 Modelling observed and future runoff from a glacierized tropical catchment (Cordillera Blanca, Peru) . Global Planet. Change 59 , 37–48 ( doi:10.1016/j.gloplacha.2006.11.038 ) [ Google Scholar ]
- Kehrwald N. M., Thompson L. G., Yao T. D., Mosley-Thompson E., Schotterer U., Alfimov V., Beer J., Eikenberg J., Davis M. E.2008 Mass loss on Himalayan glacier endangers water resources . Geophys. Res. Lett. 35 , L22503 ( doi:10.1029/2008GL035556 ) [ Google Scholar ]
- Kettlewell P. S., Sothern R. B., Koukkari W. L.1999 UK wheat quality and economic value are dependent on the North Atlantic oscillation . J. Cereal Sci. 29 , 205–209 ( doi:10.1006/jcrs.1999.0258 ) [ Google Scholar ]
- Knapp K. R., Kruk M. C., Levinson D. H., Diamond H. J., Neumann C. J.2010 The international best track archive for climate stewardship (IBTrACS): unifying tropical cyclone best track data . Bull. Am. Meteorol. Soc . 91 , 363–376 [ Google Scholar ]
- Kumar K. K., Kumar K. R., Ashrit R. G., Deshpande N. R., Hansen J. W.2004 Climate impacts on Indian agriculture . Int. J. Climatol. 24 , 1375–1393 [ Google Scholar ]
- Li Y. P., Ye W., Wang M., Yan X. D.2009 Climate change and drought: a risk assessment of crop-yield impacts . Clim. Res. 39 , 31–46 [ Google Scholar ]
- Lobell D. B., Field C. B.2007 Global scale climate–crop yield relationships and the impacts of recent warming . Environ. Res. Lett. 2 , 1–7 [ Google Scholar ]
- Lobell D. B., Burke M. B.2008 Why are agricultural impacts of climate change so uncertain? The importance of temperature relative to precipitation . Environ. Res. Lett. 3 , 1–8 [ Google Scholar ]
- Long S. P., Ainsworth E. A., Rogers A., Ort D. R.2004 Rising atmospheric carbon dioxide: plants face the future . Annu. Rev. Plant Biol. 55 , 591–628 ( doi:10.1146/annurev.arplant.55.031903.141610 ) [ PubMed ] [ Google Scholar ]
- Long S. P., Ainsworth E. A., Leakey A. D. B., Morgan P. B.2009 Global food insecurity. Treatment of major food crops with elevated carbon dioxide or ozone under large-scale fully open-air conditions suggests recent models may have overestimated future yields . Phil. Trans. R. Soc. B 360 , 2011–2020 ( doi:10.1098/rstb.2005.1749 ) [ PMC free article ] [ PubMed ] [ Google Scholar ]
- Lowe J. A., et al.2009 UK Climate Projections science report: marine and coastal projections . Exeter, UK: Met Office Hadley Centre [ Google Scholar ]
- Maracchi G., Sirotenko O., Bindi M.2005 Impacts of present and future climate variability on agriculture and forestry in the temperate regions: Europe . Clim. Change 70 , 117–135 ( doi:10.1007/s10584-005-5939-7 ) [ Google Scholar ]
- McDonald R. E., Bleaken D. G., Cresswell D. R., Pope V. D., Senior C. A.2005 Tropical storms: representation and diagnosis in climate models and the impacts of climate change . Clim. Dyn. 25 , 19–36 ( doi:10.1007/s00382-004-0491-0 ) [ Google Scholar ]
- Meehl G. A., et al.2007 Global climate projections . In Climate change 2007: the physical science basis. Contribution of Working Group I to the Fourth Assessment Report of the Intergovernmental Panel on Climate Change (eds Solomon S., Qin D., Manning M., Chen Z., Marquis M., Averyt K. B., Tignor M., Miller H. L.), pp. 747–846 Cambridge, UK: Cambridge University Press [ Google Scholar ]
- Morgan P. B., Ainsworth E. A., Long S. P.2003 How does elevated ozone impact soybean? A meta-analysis of photosynthesis, growth and yield . Plant Cell Environ. 26 , 1317–1328 ( doi:10.1046/j.0016-8025.2003.01056.x ) [ Google Scholar ]
- Nelson G. C., et al.2009 Climate change impact on agriculture and costs of adaptation . Washington, DC: International Food Policy Research Institute [ Google Scholar ]
- Newman J. A.2004 Climate change and cereal aphids: the relative effects of increasing CO 2 and temperature on aphid population dynamics . Global Change Biol. 10 , 5–15 ( doi:10.1111/j.1365-2486.2003.00709.x ) [ Google Scholar ]
- Norby R. J., Ledford J., Reilly C. D., Miller N. E., O'Neill E. G.2004 Fine-root production dominates response of a deciduous forest to atmospheric CO 2 enrichment . Proc. Natl Acad. Sci. USA 101 , 9689–9693 ( doi:10.1073/pnas.0403491101 ) [ PMC free article ] [ PubMed ] [ Google Scholar ]
- Olesen J. E., Bindi M.2002 Consequences of climate change for European agricultural productivity, land use and policy . Eur. J. Agron. 16 , 239–262 ( doi:10.1016/S1161-0301(02)00004-7 ) [ Google Scholar ]
- Olesen J. E., et al.2007 Uncertainties in projected impacts of climate change on European agriculture and terrestrial ecosystems based on scenarios from regional climate models . Clim. Change 81 , 123–143 ( doi:10.1007/s10584-006-9216-1 ) [ Google Scholar ]
- Palmer W. C.1965 Meteorological drought. Research paper 45 . Washington, DC: US Weather Bureau [ Google Scholar ]
- Parry M. L., Rosenzweig C., Iglesias A., Livermore M., Fischer G.2004 Effects of climate change on global food production under SRES emissions and socio-economic scenarios . Global Environ. Change—Hum. Policy Dimens. 14 , 53–67 [ Google Scholar ]
- Pfeffer W. T., Harper J. T., O'Neel S.2008 Kinematic constraints on glacier contributions to 21st-century sea-level rise . Science 321 , 1340–1343 ( doi:10.1126/science.1159099 ) [ PubMed ] [ Google Scholar ]
- Porter J. R., Gawith M.1999 Temperatures and the growth and development of wheat: a review . Eur. J. Agron. 10 , 23–36 ( doi:10.1016/S1161-0301(98)00047-1 ) [ Google Scholar ]
- Porter J. R., Semenov M. A.2005 Crop responses to climatic variation . Phil. Trans. R. Soc. B 360 , 2021–2035 ( doi:10.1098/rstb.2005.1752 ) [ PMC free article ] [ PubMed ] [ Google Scholar ]
- Reilly J., et al.2003 US agriculture and climate change: new results . Clim. Change 57 , 43–69 [ Google Scholar ]
- Richter G. M., Semenov M. A.2005 Modelling impacts of climate change on wheat yields in England and Wales: assessing drought risks . Agric. Syst. 84 , 77–97 ( doi:10.1016/j.agsy.2004.06.011 ) [ Google Scholar ]
- Rohling E. J., Grant K., Hemleben C., Siddall M., Hoogakker B. A. A., Bolshaw M., Kucera M.2008 High rates of sea-level rise during the last interglacial period . Nat. Geosci. 1 , 38–42 ( doi:10.1038/ngeo.2007.28 ) [ Google Scholar ]
- Sinclair T. R., et al.2000 Leaf nitrogen concentration of wheat subjected to elevated (CO 2 ) and either water or N deficits . Agric. Ecosyst. Environ. 79 , 53–60 ( doi:10.1016/S0167-8809(99)00146-2 ) [ Google Scholar ]
- Sivakumar M. V. K., Das H. P., Brunini O.2005 Impacts of present and future climate variability and change on agriculture and forestry in the arid and semi-arid tropics . Clim. Change 70 , 31–72 ( doi:10.1007/s10584-005-5937-9 ) [ Google Scholar ]
- Staley J. T., Johnson S. N.2008 Climate change impacts on root herbivores . In Root Feeders: an ecosystem perspective (eds Johnson S. N., Murray P. J.). Wallingford, UK: CABI [ Google Scholar ]
- Stern N.2007 The economics of climate change: the Stern review . Cambridge, UK: Cambridge University Press [ Google Scholar ]
- Stott P. A., Stone D. A., Allen M. R.2004 Human contribution to the European heatwave of 2003 . Nature 432 , 610–614 ( doi:10.1038/nature03089 ) [ PubMed ] [ Google Scholar ]
- Stover E., Vinck P.2008 Cyclone Nargis and the politics of relief and reconstruction aid in Burma (Myanmar) . J. Am. Med. Assoc. 300 , 729–731 ( doi:10.1001/jama.300.6.729 ) [ PubMed ] [ Google Scholar ]
- Tao F. L., Yokozawa M., Hayashi Y., Lin E. D.2003 Future climate change, the agricultural water cycle, and agricultural production in China . Agric. Ecosyst. Environ. 97 , 361–361 ( doi:10.1016/S0167-8809(03)00174-9 ) [ Google Scholar ]
- Tubiello F., Fischer G.2006 Reducing climate change impacts on agriculture: global and regional effects of mitigation, 2000–2080 . Technol. Forecasting Soc. Change 74 , 1030–1056 ( doi:10.1016/j.techfore.2006.05.027 ) [ Google Scholar ]
- Tubiello F. N., Rosenzweig C., Goldberg R. A., Jagtap S., Jones J. W.2002 Effects of climate change on US crop production: simulation results using two different GCM scenarios. Part I: wheat, potato, maize, and citrus . Clim. Res. 20 , 259–270 [ Google Scholar ]
- Tubiello F. N., et al.2007 Crop response to elevated CO 2 and world food supply—a comment on ‘food for thought …’ by Long et al . Science 312: 1918–1921, 2006 . Eur. J. Agron. 26 , 215–223 ( doi:10.1016/j.eja.2006.10.002 ) [ Google Scholar ]
- Tuck G., Glendining M. J., Smith P., House J. I., Wattenbach M.2006 The potential distribution of bioenergy crops in Europe under present and future climate . Biomass Bioenergy 30 , 183–197 ( doi:10.1016/j.biombioe.2005.11.019 ) [ Google Scholar ]
- United Nations 2007 Cyclone Sidr United Nations Rapid Initial Assessment Report . See http://ochaonline.un.org/News/NaturalDisasters/Bangladesh/tabid/2707/Default.aspx
- Van Dingenen R., Dentener F. J., Raes F., Krol M. C., Emberson L., Cofala J.2009 The global impact of ozone on agricultural crop yields under current and future air quality legislation . Atmos. Environ. 43 , 604–618 [ Google Scholar ]
- Vara Prasad P. V., Boote K. J., Allen L. H., Thomas J. M. G.2003 Super-optimal temperatures are detrimental to peanut ( Arachis hypogaea L.) reproductive processes and yield at both ambient and elevated carbon dioxide . Global Change Biol. 9 , 1775–1787 ( doi:10.1046/j.1365-2486.2003.00708.x ) [ Google Scholar ]
- von Caemmerer S., Furbank R. T.2003 The C-4 pathway: an efficient CO 2 pump . Photosynth. Res. 77 , 191–207 [ PubMed ] [ Google Scholar ]
- Walther S., Abtew W.2006 Contribution of rainfall from tropical systems in South Florida . In South Florida Water Management District, West Palm Beach . Palm Beach, FL: Environmental Resources Department [ Google Scholar ]
- Webster P. J.2008 Myanmar's deadly daffodil . Nat. Geosci. 1 , 488–490 ( doi:10.1038/ngeo257 ) [ Google Scholar ]
- Wheeler T. R., Craufurd P. Q., Ellis R. H., Porter J. R., Prasad P. V. V.2000 Temperature variability and the yield of annual crops . Agric. Ecosyst. Environ. 82 , 159–167 ( doi:10.1016/S0167-8809(00)00224-3 ) [ Google Scholar ]
- Wollenweber B., Porter J. R., Schellberg J.2003 Lack of interaction between extreme high-temperature events at vegetative and reproductive growth stages in wheat . J. Agron. Crop Sci. 189 , 142–150 ( doi:10.1046/j.1439-037X.2003.00025.x ) [ Google Scholar ]
- Wullschleger S. D., Tschaplinski T. J., Norby R. J.2002 Plant water relations at elevated CO 2 —implications for water-limited environments . Plant Cell Environ. 25 , 319–331 ( doi:10.1046/j.1365-3040.2002.00796.x ) [ PubMed ] [ Google Scholar ]
- Zemp M., Roer I., Kääb A., Hoelzle M., Paul F., Haeberli W.2008 Global glacier changes: facts and figures . Zurich, Switzerland: UNEP, World Glacier Monitoring Service [ Google Scholar ]
- Zhou X. L., Harrington R., Woiwod I. P., Perry J. N., Bale J. S., Clark S. J.1995 Effects of temperature on aphid phenology . Global Change Biol. 1 , 303–313 ( doi:10.1111/j.1365-2486.1995.tb00029.x ) [ Google Scholar ]
- Open access
- Published: 02 May 2018
Impact of farmer education on farm productivity under varying technologies: case of paddy growers in India
- Kirtti Ranjan Paltasingh 1 &
- Phanindra Goyari 2
Agricultural and Food Economics volume 6 , Article number: 7 ( 2018 ) Cite this article
32k Accesses
62 Citations
8 Altmetric
Metrics details
This paper analyzes the effects of education on farm productivity in the case of growers of modern and traditional varieties of paddy in Odisha, Eastern India. Using an endogenous switching regression model, the study has found that a minimum threshold level of education is significantly influencing the adoption of modern varieties of paddy and thereby the farm productivity of adopters only. So, the study finds the evidence in support of Schultz hypothesis that says education enhances farm productivity in the case of adopters of modern technology. The study suggests that farmers’ field school program must be implemented along with a strong extension network in the study region for a wider dissemination modern technology.
After the seminal work of Schultz ( 1964 ), the importance of education in agricultural development has been widely affirmed. Education enhances the farming skills and productive capabilities of the farmers (Weir 1999 ). It enables them to follow some written instructions about the application of adequate and recommended doses of chemical and other inputs (Appleton and Balihuta 1996 ; Huang and Luh 2009 ). Again, numeracy helps them to calculate the costs and benefits of adopting a particular farming technology. But, the empirical assessment of that hypothesis has remained inconclusive (Asadullah and Rahman 2009 ; Hojo 2004 ; Lockheed et al. 1980 ; Narayanmoorthy 2000 ). No consensus has so far been agreed upon among the experts who studied the impact of farmer education on farm productivity. A group of studies (e.g., Abdulai and Huffman 2014 ; Asadullah and Rahman 2009 ; Azhar 1991 ; Chaudhri 1979 ; Duraisamy 1992 ; Mellor 1976 ; Pudasaini 1983 ; Ram 1980 ; Singh 1974 ; Young and Deng 1999 ) found a significant role of education in augmentation of agricultural productivity. On the other hand, studies like Battese and Coelli ( 1995 ), Coelli et al. ( 2002 ), Deb ( 1995 ), Kalirajan and Shand ( 1985 ), Llewellyn and Williams ( 1996 ), Narayanamoorthy ( 2000 ), and Wadud and White ( 2000 ) did not find any significant impact of education on farm productivity and efficiency. Some studies (e.g., Hasnah and Coelli 2004 ) found even a significant negative impact of education on farm efficiency. Again, studies like Lockheed et al. ( 1980 ), Phillips ( 1994 ), and Tilak ( 1993 ) reported mixed results. Footnote 1
The possible reasons for the inconclusive empirical results of the impact of education on agricultural productivity arise mainly due to four issues—(i) how to quantify the variable “education”, (ii) whose education needs to be taken into account, (iii) where it matters, and (iv) whom it matters to? The former two issues are related to the construction of the variable “education” used in empirical models. The latter two issues are concerned with the importance of formal education in varying farm environments and also to different farmers in the same environment. The first two issues are discussed at length and breadth by Lockheed et al. ( 1980 ) and Phillips ( 1994 ). On the question of how should education be measured, many authors took years of schooling attained or completed (Asadullah and Rahman 2009 ; Asfaw and Admassie 2004 ; Chaudhri 1979 ; Hojo 2004 ; Jamison and Lau 1982 ; Narayanamoorthy 2000 ; Reimers and Klasen 2013 ; Rahman et al. 2012 ), but some authors used the dummy variable showing attainment of a threshold level of certain years of schooling (Hojo 2004 ; Huang and Luh 2009 ; Jamison and Moock 1984 ; Moock 1973 ). Footnote 2 Again, the number of grades attended was also taken in studies like Haller ( 1972 ) or sometimes a simple indicator of literacy (Sharma 1974 ). Coming to the question of “whose education matters” in agricultural development, we find different studies taking education of different persons like education of the head of the farming household, average education of the household, maximum education of any member of the household, and minimum level of education of any household member above 14 years of age. Footnote 3 These two issues are well taken up in many studies (see Alene and Manyong 2007 ; Asadullah and Rahman 2009 ; Hojo 2004 ) as they incorporated more than one measure of education in the empirical model that covers both household head’s education as well as the education level of other members. Again, in those studies, education is defined as years of schooling or a dummy variable showing a minimum threshold level.
The third important reason is ascribed to the wrong assumption of a homogeneous farming environment that all farmers operate in. Schultz ( 1975 ) argued that education plays a significant role in modernized farm environment rather than the traditional one. The ability to deal with the disequilibria caused by the adoption of modern technology is largely a function of farmer’s education. Thus, more educated farmers adjust better and quicker than the less educated or illiterate farmers (Ali and Byerlee 1991 ; Hojo 2004 ). However, most of the past studies assumed that farmers operate in a uniform farm environment. So, the effects of conventional and non-conventional inputs on farm productivity are independent of the type of farm environments (Appleton and Balihuta 1996 ; Jamison and Moock 1984 ; Moock 1981 ). This again leads to another issue (that is, the fourth concern) that even in the same farm environment, as argued by Alene and Manyong ( 2007 ), farmers are not exposed to a homogeneous technology. Some farmers are adopters of modern technology while others are not. In their study, they found a significant impact of farmer education on adoption decision of modern cowpea varieties and thereby on-farm productivity of adopters in Nigeria. Thus, as they argue “the failure to account for differences in technology available to farmers, even in the same farm environment, is likely to confound the true effects of education on agricultural productivity” (Alene and Manyong 2007 , p.156). So, the empirical specifications and subsequent analyses without taking care of all these issues fully may provide incomprehensible and confounding results. Hence, many studies found insignificant or even negative impact of education on agricultural productivity.
Coming to Indian agriculture, there is a dearth of research on this particular issue. Very few studies have been conducted on the present issue, and there is a lack of consistent and concrete evidence of a significant positive relationship between farmer education and farm productivity. Most of the studies suffer from the methodological deficiencies discussed above. Moreover, none of them tried to test the Schultz hypothesis (Schultz 1975 ) by taking care of the issues of differences in farming environments and technologies available to different groups of farmers within the same environment. Footnote 4 Among the available studies in India, only Narayanamoorthy ( 2000 ) analyzed the impact of education on farm productivity in the case of two high yielding paddy varieties (CR1009 and ADT 36) by taking average household education in terms of years of schooling, but the results were insignificant. Footnote 5 This illustrates the need for further investigation on the topic after taking care of all the methodological issues discussed above. Therefore, the present study sets out to examine the impact of farmer education on farm productivity in the case of modern and traditional paddy growers in Odisha, a major rice-producing state in Eastern India. More specifically, the study aims at testing the Schultz hypothesis and also try to find out which specification of education variable is a better measure to delineate the impact of education on farm productivity. Following Alene and Manyong ( 2007 ), Asfaw et al. ( 2012 ), Di Falco et al. ( 2011 ), and Khonje et al. ( 2015 ), an efficient version of endogenous switching regression model is used, after controlling for different exogenous factors and other farm and farmers’ characteristics, to test the differential impact of education on farm productivity under both technologies. Only the paddy growers are taken as a case study since paddy is the major crop and staple food for the state of Odisha.
The paper is organized in the following manner. After a brief background in the “ Background ” section, the “ Methods ” section provides an outline of the analytical framework and also describes the study area, data collection, and empirical model specification with emphasis on the hypothesized relations. The “ Results and discussion ” section presents the empirical results, their analysis, and discussion. The “ Conclusion ” section concludes with some policy implications.
Materials and methods
Endogenous switching regression is a suitable method to analyze the core objective of the paper, namely, the impact of farmer education on farm productivity, given the technology adoption status. This method takes care of two econometric problems—endogeneity and sample selection bias. The analysis of the impact of technology adoption on productivity and other welfare outcomes poses a problem of possible endogeneity (Asfaw et al. 2012 ; Hausman 1978 ) because there is likely to be a two-way relationship between technology adoption and productivity enhancement (Alene and Manyong 2007 ). First, technology adoption may cause productivity enhancement for some farmers. However, at the same time, enhanced productivity also induces more technology adoption. Thus, adoption decision is either voluntary, or some technologies are targeted to a group of farmers. Farmers, who are more productive, adopt more technologies because of their self-decision. This self-selection decision into technology intervention is a potential source of endogeneity, and it results in an overstatement of the true impact of technology on productivity if not accounted for properly. Again, the less productive farmers are also encouraged to adopt technology because of its productivity enhancement capabilities. This, if not accounted for properly, will result in an understatement of the true impact of technology adoption on productivity. Therefore, the productivity outcome between the adopters of modern technology and non-adopters could be due to unobserved heterogeneity caused by innate abilities and other unobserved farmers and farm-specific characteristics. This leads to inconsistent estimates of the impact of technology adoption. Because of this difference in initial conditions between adopters and non-adopters caused by unobserved farm and farmer characteristics being only known to farmers, but not to the researchers, the problem of endogeneity in adoption behavior can be solved by adopting simultaneous equation models (Alene and Manyong 2007 ; Hausman 1983 ).
The other econometric issue involved here, even after accounting for endogeneity, is the sample selection bias. The vital query that remains is whether is it appropriate to use a pooled sample of adopters and non-adopters (where a dummy variable can be used in the regression model to evaluate the impact of adoption of modern technology on productivity)? If the entire sample is selected, it implicitly assumes that technology will have an average impact on productivity over the entire sample of farmers by the way of an intercept shift in the production function. The pooled-sample estimation assumes the same impact of the set of covariates on adopters and non-adopters (Kassie et al. 2007 , 2009 ). This implies that the marginal impact of other conventional factors (land, labor, fertilizer, irrigation, and others) and non-conventional factors (education, extension, experience, and so on) is independent of the status of adoption (Asfaw et al. 2012 ). But, if we assume that the marginal impact of those factors (slope parameters) is not independent of technology adoption, and they have different impacts on productivity based on technology adoption, then different production functions should be used for adopters and non-adopters separately. Thus, the econometric problems involved here are both endogeneity (Hausman 1978 ) and sample selection bias (Heckman 1979 ). This motivates us to use an endogenous switching regression model that takes care of both endogeneity and sample selection problem (Asfaw et al. 2012 ; Di Falco et al. 2011 ; Freeman et al. 2001 ).
- Endogenous switching regression
In endogenous switching regression model, the first stage involves modeling of the adoption behavior with the limited-dependent variable method. In the second stage, another decision variable (productivity) is estimated separately for each group (adopters and non-adopters), conditional on the adoption decision. So, a binary probit model is used in the first stage to model the adoption behavior, and in the second stage, separate regression models are used to model agricultural production function conditional on a specified criterion function.
Following Ali and Abdulai ( 2010 ) and Asfaw et al. ( 2012 ), the decision to adopt can be modeled in the framework of utility maximization. The difference between the utilities from adoption ( U Ai ) and non-adoption ( U Ni ) of modern varieties (MVs) of paddy may be denoted as G * , such that the i th household would like to adopt the given technology if U Ai is greater than U Ni . In other words, the i th household will adopt when G ∗ = U Ai − U Ni > 0. But, G * is unobservable. So, we can express it as a function of observable factors in this latent variable model (probit model) as follows:
Where G is the dichotomous variable that takes the value 1 if farmer is an adopter of MVs of paddy and 0 otherwise; β is the vector of unknown parameters to be estimated, and X is the vector of explanatory variables comprising farmer, farm, and technology-specific characteristics; and u is the random error term with 0 mean and variance as σ 2 . Maximum likelihood estimation procedure is employed to estimate the vector of probit coefficients β .
Now, the adoption of technology also affects the farm productivity ( Y ). Let the farm productivity ( Y ) be a function of conventional and non-conventional factors then J i is the vector of those exogenous factors. In switching regression method, as farm productivity (Y) is conditional on technology adoption status, we use two separate production functions for adopters and non-adopters as follows:
The variables Y 1 and Y 0 are the agricultural productivity (paddy yield) under new technology (MVs) and traditional technology (local), respectively. For a given household, Y 1 or Y 0 is observable depending on the values of the criterion function in Eq. ( 1 ). Therefore, the ordinary least squares (OLS) estimates of parameter vector J 1 or J 0 will be biased as they suffer from sample selection bias. The errors ϵ 1 and ϵ 0 , conditional on sample selection criterion will have non-zero expected values (Lee and Trost 1978 ; Maddala 1983 ). Finally, the error terms u , ϵ 1 , and ϵ 0 are assumed to have a trivariate normal distribution with 0 mean and non-singular covariance matrix expressed as follows:
Where \( {\sigma}_u^2 \) is the variance of the error in the criterion Eq. ( 1 ); \( {\sigma}_{\epsilon 1}^2 \) and \( {\sigma}_{\epsilon 0}^2 \) are the variance of the errors ϵ 1 and ϵ 0 , respectively, in productivity outcome functions in Eq. ( 2 ); and σ ϵ 1 u and σ ϵ 0 u are the covariance of error terms u , ϵ 1 , and ϵ 0 . The outcome functions in Eq. ( 2 ) are not observed simultaneously. So, the covariance between ϵ 1 and ϵ 0 is not defined (Maddala 1983 ). However, a significant inference of the error structure is that as the error u of criterion function is correlated with the error terms of the productivity functions in Eq. ( 2 ), the expected values of the error terms are non-zero, conditional on the sample selection, and expressed as:
where ϕ (.) and Φ (.) are the standard normal probability density function and standard normal cumulative density function, respectively. λ 1 i and λ 0 i , i.e., the estimated ratio of ϕ (.), and Φ (.) evaluated at βX i is the inverse Mills ratio. If the estimated covariance σ ϵ 1 u and σ ϵ 0 u are statistically significant, it implies that adoption decision and productivity outcome variable are correlated. So, we find the evidence of endogenous switching and reject the null hypothesis of no sample selection bias (Maddala and Nelson 1975 ).
The full information maximum likelihood (FIML) estimation method is considered to be the most efficient one to estimate the endogenous switching regression model (Asfaw et al. 2012 ; Di Falco et al. 2011 ; Lokshin and Sajaia 2004 ). It estimates simultaneously the criterion equation (probit model) and the productivity outcome functions to give consistent standard errors. On the basis of the trivariate normal distribution for the error terms, the logarithmic likelihood function for the system of Eqs. ( 1 ) and ( 2 ) can be given as:
Where \( {\varphi}_{ji}=\frac{\left({\beta X}_i+{\gamma}_j{\epsilon}_{ji}/{\sigma}_j\right)}{\sqrt{1-{\gamma}_j^2}} \) , j = 0, 1 with γ j denoting the correlation coefficient between the error term of criterion function, i.e., u i and the errors of outcome functions, i.e., ϵ ji . The entire system of the equations is jointly estimated by full information likelihood method.
Study area and sampling strategy
The study is based on the primary data at the household level collected from two districts of Odisha, Cuttack, and Khordha during 2012 to 2013 cropping season. It focused only on the kharif season which is a major paddy-producing season in Odisha. Footnote 6 A multistage purposive sampling method was used to pin down the households surveyed. First, the two districts, Cuttack and Khordha, were selected purposively based on the scale of paddy production and intensity of agricultural research and development (R&D) activities being carried out. The paddy is a major crop grown in these two districts, and more importantly, two major agricultural research institutes, that is, Central Rice Research Institute (CRRI) and Odisha University of Agriculture and Technology (OUAT), are situated in Cuttack and Khordha district, respectively. Thus, the farmers in these two districts are equally exposed to different R&D activities in agriculture. However, we hypothesize that the educated farmers will capture the information better than uneducated or less educated farmers. In the next step, two different development blocks are chosen randomly. From each block, three villages were chosen, and from each village, households were surveyed randomly. The total sample included 300 farming households, 152 from Cuttack and 148 from Khordha.
The survey collected information on farmers’ specific socioeconomic characteristics along with farm-specific factors. Table 1 shows the construction of all variables, and Table 2 shows the significance of the difference between the mean values of some continuous variables pertaining to adopters and non-adopters of MVs. Footnote 7 In this study, an adopter is defined as one who cultivates any type of modern varieties of paddy, Footnote 8 no matter how much land of total holding is being devoted to the adoption of MVs of paddy. Some adopters were found to allocate some land for traditional varieties (TVs) of paddy also.
Variables and estimation strategy
The adoption decision is a dichotomous one. The dependent variable (ADOPTION) takes the value 1 if the farmer adopts the MVs of paddy and 0 otherwise. The probit model is used to estimate the adoption behavior (criterion equation).
The independent variables are farm-specific and other institutional factors. Farmer-specific variables are farming experience in years (EXP), farmer’s education (EDN), and his household size (HS) in numbers. Educational status variable (EDN) is defined differently by using different specifications as (i) first, years of schooling of household head; (ii) second, the average years of education of the household; and (iii) third, two dummy variables defined as 5 years of schooling or more and 10 years of schooling or more. The first dummy variable takes the value 1 if the head’s education is of 5 years of schooling or more and 0 otherwise. The second dummy variable takes the value 1 if it is 10 years of schooling or more and 0 otherwise. Footnote 9 The reason for taking 5 and 10 years of schooling spawns from the argument that in a basic education cycle, 5 years of schooling is considered as minimum education level as it completes primary education, and 10 years of schooling completes the secondary level of education or high school education in India. Footnote 10 All these specifications of education (EDN) variable are hypothesized to have positive impact on adoption. There is a significant mean difference in the level of education between adopters and non-adopters. The farm-specific factors include total paddy area (ARPDY) in hectare, soil quality (SQ), and total cost of hired labour (TLC) in thousand rupees and ecosystem dummy (ECDMY). We hypothesized a positive relation between these farm-specific factors and adoption. The mean values of total paddy area (ARPDY) vary significantly in-between adopters and non-adopters of MVs. The set of institutional variables includes social capital (SOC), tenancy structure (TNCY), access to credit (CRDIT), extension visits dummy (EXTN), ownership of livestock (LIVSTCK), distance to market (MKTD), and access to media dummy (MEDIA). The tenancy structure is constructed as the proportion of leased-in operated area in total operational landholding. In our study region, generally, some farmers leased in some lands from other relatively big farmers who are either staying away from villages or not doing cultivation since their main source of livelihood is non-farm activities. So, this is not sharecropping where a farmer allies with another farmer. Similarly, we have constructed the dummy variable “MEDIA” as the one that takes value 1 if a farmer owns a television and 0 otherwise. In the existing literature, many studies have taken a radio or both radio and television. But, intuition tells that audiovisual source of information is more effective than only audio. The set of technology characteristic-related variables includes farmer’s perception about the maturity period of the crop (MATC), higher productivity characteristic (PRDTYC), and the availability of seeds at a cheaper price (CHEPSC). All these technology characteristics related variables are dummy variables taking the value 1 if the farmer perceives so about the MVs and 0 if not.
In order to estimate the yield equations (outcome function) for the adopters as well as non-adopters separately on the basis of criterion equation, the following functional form of the equations is specified here as:
The dependent variable Ln (YIELD) is natural logarithm of paddy yield expressed as kilogram per hectare. Footnote 11 There is a significant mean difference of yield in-between adopters and non-adopters of MVs. The set of conventional and non-conventional factors included here in the outcome equation are education, extension, total area under paddy, ecosystem dummy, total fertilizer use, total labor cost, and soil quality. There is a significant mean difference between adopters and non-adopters in the case of labor cost and total fertilizer use. All these conventional and non-conventional factors of production are hypothesized to have yield-augmenting effects. However, it is hypothesized that formal education (EDN) will have a differential impact on agricultural productivity under modern and traditional technologies.
Following Asfaw et al. ( 2012 ), Di Falco et al. ( 2011 ), and Maddala ( 1983 ), the three technology characteristic variables along with some others are not included in yield equations on the basis of the assumption that these variables are not likely to influence paddy production directly. Rather, they influence through technology adoption. Thus, the usual order condition for identification purpose is followed so that the X i in the adoption equation (criterion equation) contains at least one factor not in J i in the yield equation (outcome equation). The FIML method is employed to estimate the system of equations jointly.
Results and discussion
Determinants of modern varieties of paddy adoption.
Table 3 gives the results of the first stage estimation of endogenous switching regression model for various factors affecting adoption of modern varieties of paddy (criterion equation). Different specifications were tried on the basis of various measures of education variable. All the model specifications fit well to the data set as indicated by the likelihood ratio test that the coefficient parameters are statistically significantly different from 0 at 1% significance level and the pseudo R-squared value is quite high. Four different measures for education variable, i.e., years of schooling of the farmer, two dummy variables for head’s education, and the average years of schooling of the household, were incorporated in the analysis. Footnote 12 The four measures were incorporated in four model specifications. The measure of an average year of schooling in the fourth model specification did not come out significant. But, the other three measures are statistically significant. The variable “years of schooling of the farmers” is significant at 10% probability level while both education dummy variables (EDNDMY) as defined earlier are significant at 5% and 1% probability levels, respectively. So, this confirms our hypothesis that education has a strong threshold effect on the probability of adoption of modern technology. This result is supported by many studies like Alene and Manyong ( 2007 ) and Phillips ( 1994 ). Farming experience (EXP) is positive but insignificant. The household size (HS) is negative though insignificant. The hypothetical relation was assumed to be positive. But, empirical evidence shows the reverse relation. The reason may be that big family spends more on consumption durables and developmental activities rather than modernization of agriculture. Other significant variables are total paddy area (ARPDY), ecosystem dummy (ECDMY), contact with agricultural extension service (EXTN), social capital (SOC), distance to local market (MKTD), and one technology characteristic variables, i.e., short maturity of MVs (SRTMC). The adoption of new technology is usually initiated by the large farmers as they can withstand both subjective and objective risks attached with modern technology. Therefore, as the total paddy area (ARPDY) increases, there is a better prospect for adoption. Ecosystem dummy is highly significant indicating thereby that if the farmers have irrigated land, then they are more likely to adopt modern technology. Contact with agricultural extension services lends a great deal of help to the farmers. The farmers learn more about the new technology from the extension service officers. So, farmers who frequently visit the extension office and keep regular contact with them are usually more progressive and quickly adopt the new technology. Thus, it was hypothesized to be positive, and we found results in line with that theoretical hypothesis. Soil quality retains its positive sign but insignificant. Though, a fertile soil is quite an important factor making a conducive environment for adoption of MVs of paddy but, it does not affect the adoption behavior significantly here in the study region because a major portion of the farmland belongs to the same quality of soil fertility. So, it does not make a big difference. Social capital in the form of membership of developmental groups influences the probability of adoption significantly. It breaks the barrier of information asymmetry and helps in the cross-fertilization of ideas. The existence of local market acts as an information hub to the farmers. If the distance to local market is more, then farmers are less likely to get the information about new farm practices. So, it hampers technology adoption. We get significant and negative results as expected. Out of two technology characteristics, only farmer’s perception about the short maturity period of MVs is significant.
Determinants of agricultural productivity
Table 4 presents the results of the second stage of endogenous switching regression model. We estimated four different specifications of the outcome equation (yield equation) corresponding to each specification of criterion equation on the basis of various measures of education variable. The correlation coefficients between the error of criterion equation (adoption) and errors of outcome equations (yield equations) in each specification are given in the last row. The estimated correlation coefficients in all four specifications are significant indicating that both observed and unobserved factors are influencing the decision to adopt. Thus, the results of the adoption model and switching regression model together suggest the existence of endogeneity and the problem of self-selection. In other words, the decision to adopt and the impact of technology on yield given the adoption decision, are influenced by both observed and unobserved factors. The alternative signs of both correlation coefficients suggest that adopters adopt modern varieties on the basis of their comparative advantages. In other words, those who adopted have above-average returns from adoption, and those who did not choose to adopt have above-average returns from non-adoption (Alene and Manyong 2007 ; Fuglie and Bosch 1995 ). The insignificance of the correlation coefficient between adoption equation and non-adopters’ yield function in all four specifications indicates that adopters and non-adopters obtain the same mean yield of paddy using local varieties, given their observed characteristics. Thus, the initial difference between the adopters and non-adopters is insignificant, but the differential effects of technology on the two groups are brought by some unobserved factors.
The switching regression results in Table 4 demonstrate that majority of the conventional factors of production have expected signs. But, the marginal effects are different in the case of both adopters and non-adopters confirming the differential marginal impacts of factors on paddy yield between adopters and non-adopters. The ecosystem dummy is significant in the case of both modern and traditional paddy varieties. This reveals that overall paddy yield is positively and significantly influenced by assured irrigation being represented by ecosystem dummy (ECDMY), no matter whether it is modern varieties or traditional varieties. Of course, paddy is water-intensive crop and it consumes plenty of water. So, assured irrigation is a must for paddy cultivation. Similarly, the total area under paddy (ARPDY) is also positively and significantly influencing paddy yields in the case of both MVs and TVs of paddy. Bigger farm size brings many economies of scale in farm production process. Optimal use of many farm inputs can be achieved if the size of holding is a big one. So, bigger farm size is always hypothesized to have a positive impact on productivity. Total fertilizer used (FERT) was being hypothesized to have yield augmenting effects in the case of both varieties, and it retains its expected sign. But, it is significant only in the case of modern varieties. In fact, both fertilizer and MVs are complementary to each other, and both were introduced to Indian farmers as a package during the 1960s that marked the onset of green revolution in Indian agriculture (Bezbaruah 1994 ). Even, the output elasticity of fertilizer is bigger in the case of MVs of paddy in comparison to TVs. However, soil quality (SQ) is positive but insignificantly affecting farm production under both technologies. Contact with agricultural extension services is only significant in the second and third specification in the case of adopters only. So it is not that much of an influential factor here.
The main objective of this study is to analyze the impact of education on paddy yield in the case of MVs and TVs to test the Schultz hypothesis. The average years of schooling of the household did not reveal any significant effects on paddy yield in the case of both adopters and non-adopters (specification 4(a)). This is consistent with other Indian studies such as Narayanamoorthy ( 2000 ) who also concluded the same insignificant effects of average years of schooling of households on paddy yields. Lack of significant influence of average education implies the absence of centralized decision-making in farming as evidenced by Asadullah and Rahman ( 2009 ) and Mussa ( 2015 ). It is because of the reason that distribution of landholding in Odisha is severely skewed towards marginal farmers (77.17% out of total number of farmers) and small farmers (20%) with the average size of holding in the state being 1.04 ha as per 2010 to 2011 census (Odisha Agriculture Statistics 2013–2014 ). In our sample also, most of the farmers belong to these two categories. Therefore, in a subsistence agriculture, the decision-making solely rests with the farmer himself. The other educated members of the household prefer other non-farm activities rather than being engaged in agriculture. However, as expected, the other three measures of education variable, that is, years of schooling of the head and both dummy variables, revealed a significant impact on paddy yield in the case of adopters only. Again, the level of statistical significance of education dummies is higher than the years of schooling (specification 1(a), 2(a), and 3(a) of Table 4 ). The second dummy variable having the threshold level as 10 years of schooling is significant at 1% probability level in comparison to other two measures. Thus, this indicates that farmer education affects farm productivity significantly under modern technology, and it has a strong threshold effect on farm productivity. This result is supported by many studies like Alene and Manyong ( 2007 ), Asfaw et al. ( 2012 ), Lockheed et al. ( 1980 ), and Phillips ( 1994 ). Footnote 13 Thus, the farmers who completed minimum education of secondary level are most likely to adopt MVs and experience the yield augmenting effects of education. This indicates that attaining a minimum threshold level of education helps farmers in ways of enhancing their skills and capabilities to collect and analyze the information and execute that on the field. It creates a conducive environment to go for modern technology adoption and thereby augments the productivity. Unless that minimum basic education is attained, formal education does not influence productivity. The results clearly demonstrate that coefficients of the two measures of education are positive in the case of both adopters and non-adopters but significant only in the case of adopters. Even, the differential impact is also noticed in-between the two paddy varieties. The output elasticities of education in the case of adopters of modern varieties are 0.019, 0.048, and 0.036, respectively, in the first three model specifications while they are 0.004, 0.010, and 0.016 for non-adopters, respectively . Hence, the results support Schultz’s argument that formal education has a significant marginal contribution to farm production only under modern technology. This result is consistent with the earlier studies like Asfaw et al. ( 2012 ), Alene and Manyong ( 2007 ), Lockheed et al. ( 1980 ), Phillips ( 1994 ), and others. The reason for this as argued by Schultz ( 1964 ) is that farmers in the traditional environment are already efficient. Thus, educational efforts offer little or no marginal contribution to production (cited in Alene and Manyong 2007 , p. 156). But, the modern mode of production requires the formal education which may not be necessary for traditional agriculture. So, we are likely to get confounding results if we fail to take into account the technology differences available to farmers even in the same farming environment.
We view our study as fulfilling a couple of important research gaps in the literature. First, the method used in this study is an improvement over the methods used in the past literature. Many earlier studies understated the true marginal contribution of education to agricultural productivity by adopting an OLS method of estimation of the single production function. Because the OLS method of estimation of the single production function for all farmers does not take into account the heterogeneity that exists among the farmers so far as technology adoption is concerned. Even if a dummy variable is incorporated in the OLS model to account for technology adoption, it falls into the trap of endogeneity problem as technology adoption is highly endogenous. But, in this study, the endogenous switching regression model is used which is free from endogeneity and sample selection bias. So, the results are unbiased and robust. Second, the use of years of schooling of the head or average years of schooling of the household as the education variable in regression model acts as the major source of confounding results. Because these two variables do not reveal any strong significant effect on productivity as there is a strong threshold effect of education on farm productivity. Even in this study, we also got insignificant influence of average years of schooling of household and very low level of significance for years of schooling of the farmer. But, both education dummies defined as 5 years of schooling or more and 10 years of schooling or more revealed highly significant impact of education on agricultural productivity. Third, the results of endogenous switching regression model confirmed that formal education has a differential impact on farm productivity of adopters and non-adopters of modern technology, even in the same physical environment that they all operate in together. Thus, it supports the Schultz’s hypothesis that in the modernized agriculture, education does play a significant role.
The effects of education will be much more if suitable policies are implemented properly. As evidenced from the analysis, education has a strong threshold effect in the successful adoption of MVs of paddy and thereby paves the way for achieving better yields. Farmer education is complementary to the research and development activities that take place in the farm sector because the application of scientific knowledge for agricultural development becomes a major concern when the human resources involved in it are unskilled and incompetent due to mass illiteracy. Therefore, educating farmers about the application of various modern technologies needs to be emphasized. The implementation of farmer field school (FFS) program along with strong extension service could help in this direction as it is an innovative, participatory, and interactive model approach to educate the farmer about various farm practices, crop management strategies, and technological up-gradation.
Lockheed et al. ( 1980 ) and Phillips ( 1994 ) surveyed some early studies conducted worldwide while Tilak ( 1993 ) surveyed some studies conducted in Asia, and Appleton and Balihuta ( 1996 ) surveyed comprehensively on the same issue in the case of African countries only.
The dummy variable shows threshold level of education where the threshold is mostly taken as 4 years of schooling. It has been argued that the 4 years of schooling constitute the minimum level in the basic education cycle (Lockheed et al. 1980 ). However, some studies have taken different thresholds, for instance, Jamison and Moock ( 1984 ) have taken 6 years or more. Recent studies like Narayanamoorthy ( 2000 ) and Asadullah and Rahman ( 2009 ) have taken the dummy of 5 years of farmer education as minimum threshold. But, Narayanamoorthy did not include that variable in regression analysis.
Lockheed et al. ( 1980 ) and Phillips ( 1994 ) discussed the issue of 'whose education matters' and surveyed many studies in detail in this regard. Recently, Mussa ( 2015 ) has taken average years of schooling of household and maximum and minimum years of schooling any member of household in his analysis.
Kalirajan and Shand ( 1985 ) in their study argued that in technologically advanced area, where there are no serious production constraints like input unavailability, extension service, schooling of farmer is not necessarily a major factor of efficient production performance. Rather, they argued that no difference exists between educated farmers and illiterate farmers if the technology is communicated properly. Thus, understanding of the technology is more important than the formal education.
Duraisamy ( 1992 ) used both years of schooling of the farmer and average education of the household and some dummy variable. He found all the variables significantly and positively influencing crop yield. But the issues of differences in farming environments and technologies available to the farmers are not taken into consideration.
In Odisha, paddy is cultivated in three different cropping seasons, that is, kharif season (June to September), rabi season (October to February), and the summer season (March to May). However, the kharif season is the major rice-producing season that accounts for 94% of total rice area and 92% of total rice production (Odisha Agriculture Statistics 2013–2014 ).
The t statistic used here to demonstrate the mean difference between adopters and non-adopters is computed as: \( t=\left({y}_{\mathrm{A}}-{y}_{\mathrm{N}}\right)/\sqrt{\mathrm{Var}\left({y}_{\mathrm{A}}\right)/{n}_{\mathrm{A}}+\mathrm{Var}\left({y}_{\mathrm{N}}\right)/{n}_{\mathrm{N}}} \) , where n A and n N are the number of adopters and non-adopters of MVs, respectively, and y A and y N are the sample means of a respective variable for adopters and non-adopters. The degree of freedom is n A + n N − 2.
Modern varieties of paddy include the new generation of high yielding varieties (HYV) as well as hybrid seeds and recently developed stress tolerant varieties. Details can be found in Paltasingh ( 2014 ). Though, a farmer is considered as an adopter irrespective of the amount of land being devoted to adoption of MVs. However, observation shows that majority of farmers who adopt MVs devote a major portion of their landholding to MVs adoption.
Many studies have taken different thresholds. For details, see Lockheed et al. ( 1980 ), Phillips ( 1994 ), and Tilak ( 1993 ).
In India, education up to 10th class is considered as secondary level which comes under the “Board of Secondary Education,” and 11th and 12th classes are considered as higher secondary education which comes under “Council of Higher Secondary Education.” This system is uniform broadly across the states. Therefore, 10 years of schooling marks the completion of the secondary level of education.
The dependent variable in the outcome function is overall paddy yield. In fact, there are some farmers who adopt both varieties, and when we take yield in outcome function for adopters, it is the overall yield of adopters, no matter whether he is a complete adopter or partial adopter.
Some other specifications of education variable like proportion of other members who completed primary education, spouse’s education, and the highest level of education of any member of the household are also tried, but all of them are found to be insignificant. So they are not reported here.
First two studies have taken dummy of 4 years of schooling while the last study tried different thresholds. However, many other studies have taken dummy variable indicating a threshold level of education. Details can be found in Lockheed et al. ( 1980 ) and Phillips ( 1994 ).
Abdulai A, Huffman W (2014) The adoption and impact of soil and water conservation technology: an endogenous switching regression application. Land Econ 90(1):26–43
Article Google Scholar
Alene AD, Manyong VM (2007) The effect of education on agricultural productivity under traditional and improved technology in northern Nigeria: an endogenous switching regression analysis. Empir Econ 32(1):141–159
Ali A, Abdulai A (2010) The adoption of genetically modified cotton and poverty reduction in Pakistan. J Agr Econ 61(1):175–192
Ali M, Byerlee D (1991) Economic efficiency of small farmers in a changing world: a survey of recent evidence. J Int Dev 3(1):1–27
Appleton S, Balihuta A (1996) Education and agricultural productivity: evidence from Uganda. J Int Dev 8(3):415–444
Asadullah MN, Rahman S (2009) Farm productivity and efficiency in rural Bangladesh: the role of education revisited. Appl Econ 41(1):17–33
Asfaw A, Admassie A (2004) The role of education on the adoption of chemical fertilizer under different socioeconomic environments in Ethiopia. Agric Econ 30(3):215–228
Asfaw S, Shiferaw B, Simtowe F, Lipper L (2012) Impact of modern agricultural technologies on smallholder welfare: evidence from Tanzania and Ethiopia. Food Policy 37(3):283–295
Azhar RA (1991) Education and technical efficiency during the green revolution in Pakistan. Econ Dev Cult Change 39(3):651–665
Battese GE, Coelli TJ (1995) A model for technical inefficiency effects in a stochastic frontier production function for panel data. Empir Econ 20(2):325–332
Bezbaruah MP (1994) Technological transformation of agriculture: a study from Assam. Mittal Publications, New Delhi
Google Scholar
Chaudhri DP (1979) Education, innovations and agricultural development. Croom Helm, London
Coelli T, Rahman S, Thirtle C (2002) Technical, allocative, cost and scale efficiencies in Bangladesh rice cultivation: a non-parametric approach. J Agr Econ 53(3):607–626
Deb UK (1995) Human capital and agricultural growth in Bangladesh. Dissertation, University of the Philippines, Philippines
Di Falco S, Veronesi M, Yesuf M (2011) Does adaptation to climate change provide food security? A micro-perspective from Ethiopia. Am J Agr Econ 93(3):829–846
Duraisamy P (1992) Effects of education and extension contacts on agricultural production. Ind J Agr Econ 47(2):205–214
Freeman HA, van der Merwe PJA et al (2001) Assessing the adoption potential of new groundnut varieties in Malawi. Working paper 11. ICRISAT, Patancheru
Fuglie KO, Bosch DJ (1995) Economic and environmental implications of soil nitrogen testing: a switching regression analysis. Am J Agr Econ 77(4):891–900
Haller TE (1972) Education and rural development in Colombia. Dissertation, Purdue University, Indiana
Hasnah EF, Coelli T (2004) Assessing the performance of a nucleus estate and smallholder scheme for oil palm production in West Sumatra: a stochastic frontier analysis. Agric Syst 79(1):17–30
Hausman JA (1978) Specification tests in econometrics. Econometrica 46(6):1251–1272
Hausman JA (1983) Specification and estimation of simultaneous equation models. In: Griliches Z, Intriligator MD (eds) Handbook of econometrics, vol I. North Holland Press, Amsterdam
Heckman J (1979) Sample selection bias as a specification error. Econometrica 47(1):153–161
Hojo M (2004) Measuring education levels of farmers: evidence from innovation adoption in Bangladesh. Discussion Paper 04–06, Osaka University, Japan
Huang F, Luh Y (2009) The economic value of education in agricultural production: a switching regression analysis of selected East-Asian countries. A paper presented in International Association of Agricultural Economists Conference, 16–22 August, Beijing
Jamison DT, Lau LJ (1982) Farmer education and farm efficiency. Johns Hopkins University Press, Baltimore
Jamison DT, Moock PR (1984) Farmer education and farm efficiency in Nepal: the role of schooling, extension services and cognitive skills. World Dev 12(1):67–86
Kalirajan KP, Shand RT (1985) Types of education and agricultural productivity: a quantitative analysis of Tamil Nadu rice farming. J Dev Stud 21(2):232–243
Kassie M, Pender J et al (2007) Impact of soil conservation on crop production in the northern Ethiopian highlands. IFPRI Discussion Paper 00733, International Food Policy Research Institute, Washington, D. C
Kassie M, Zikhali P, Pender J, Kohlin G (2009) Sustainable agricultural practices and agricultural productivity in Ethiopia: does agroecology matter? EfD Discussion paper 09–12, Environment for the Development Initiative (EfD)/Resources for the Future (RfF), Washington, D.C
Khonje M, Manda J, Alene AD, Kassie M (2015) Analysis of adoption and impacts of improved maize varieties in eastern Zambia. World Dev 66:695–706
Lee LF, Trost RP (1978) Estimation of some limited dependent variable models with application to housing demand. J Econometrics 8(3):357–382
Llewellyn RV, Williams JR (1996) Nonparametric analysis of technical, pure technical, and scale efficiencies for food crop production in East Java, Indonesia. Agric Econ 15(2):113–126
Lockheed ME, Jamison DT, Lau LJ (1980) Farmer education and farm efficiency: a survey. Econ Dev Cult Change 29(1):37–76
Lokshin M, Sajaia Z (2004) Maximum likelihood estimation of endogenous switching regression models. The Stata J 4(3):282–289
Maddala GS (1983) Limited dependent and qualitative variables in econometrics. Cambridge University Press, UK
Maddala GS, Nelson FD (1975) Switching regression models with exogenous and endogenous switching. In Proceeding of the American Statistical Association (Business and Economics Section), pp. 423–426
Mellor JW (1976) The new economics of growth. Cornell University Press, New York
Moock PR (1973) Managerial ability in small farm production: an analysis of maize yields in the Vihiga division of Kenya. Dissertation, Columbia University, USA
Moock PR (1981) Education and technical efficiency in small-farm production. Econ Dev Cult Change 29(4):723–739
Mussa R (2015) The effects of educational externalities on maize production in rural Malawi. Oxford Dev Stud:1–25. https://doi.org/10.1080/13600818.2015.1046826
Narayanamoorthy A (2000) Farmers’ education and productivity of crops: a new approach. Ind J Agr Econ 55(3):511–519
Odisha Agriculture Statistics (2013–2014) Directorate of agriculture and food production, Government of Odisha, Bhubaneswar
Paltasingh KR (2014) Technology adoption and climatic risks in agriculture: a study of Odisha. Dissertation, University of Hyderabad, India
Phillips JM (1994) Farmer education and farmer efficiency: a meta-analysis. Econ Dev Cult Change 43(1):149–165
Pudasaini SP (1983) The effects of education in agriculture: evidence from Nepal. Am J Agr Econ 65(3):509–515
Rahman KM, Mia MA et al (2012) A stochastic frontier approach to technical efficiency of rice farmers in Bangladesh: an empirical analysis. Agriculturalists 10(2):9–19
Ram R (1980) Role of education in production: a slightly new approach. Q J Econ 95(2):365–373
Reimers M, Klasen S (2013) Revisiting the role of education for agricultural productivity. Am J Agr Econ 95(1):131–152
Schultz TW (1964) Transforming traditional agriculture. Yale University Press, New Haven
Schultz TW (1975) The value of the a bility to deal with disequilibria. J Econ Lit 13(3):827–896
Sharma SR (1974) Technical efficiency in traditional agriculture: an econometric analysis of the Rupandehi district of Nepal. Dissertation, Australian National University, Australia
Singh B (1974) Impact of education on farm production. Econ Polit Weekly 9(39):A92–A96
Tilak JBG (1993) Education and agricultural productivity in Asia: a review. Ind J Agr Econ 48(2):187–200
Wadud A, White B (2000) Farm household efficiency in Bangladesh: a comparison of stochastic frontier and DEA methods. Appl Econ 32(13):1665–1673
Weir S (1999) The effects of education on farmer productivity in rural Ethiopia. Centre for the Study of African Economies Working Paper no. WPS/99–7, Oxford University, Oxford
Young D, Deng H (1999) The effects of education in early-stage agriculture: some evidence from China. Appl Econ 31(11):1315–1323
Download references
Acknowledgements
The authors are thankful to Pratap Singh Birthal, M. P. Bezbaruah, Sarthak Gaurav, Priyedarshi Jetli, Mohd. Kashif Khan and two anonymous reviewers for their insightful comments on the earlier draft of the paper. Special thanks are also in place for those farmers who participated in our survey. However, the usual disclaimer applies.
Author information
Authors and affiliations.
School of Economics, SMVD University, Jammu, 182 320, India
Kirtti Ranjan Paltasingh
School of Economics, University of Hyderabad, Hyderabad, 500 046, India
Phanindra Goyari
You can also search for this author in PubMed Google Scholar
Contributions
KRP acquired the data and contributed in the development of the model, drafting the article, and analysis of the results. PG revised the paper critically for important intellectual content and rechecked the results. Both authors read and approved the final manuscript.
Corresponding author
Correspondence to Kirtti Ranjan Paltasingh .
Ethics declarations
Authors’ information.
KRP is an assistant professor in the School of Economics, SMVD University, India.
PG is a professor in the School of Economics, University of Hyderabad, India.
Competing interests
Both authors declare that they have no competing interests.
Publisher’s Note
Springer Nature remains neutral with regard to jurisdictional claims in published maps and institutional affiliations.
Rights and permissions
Open Access This article is distributed under the terms of the Creative Commons Attribution 4.0 International License ( http://creativecommons.org/licenses/by/4.0/ ), which permits unrestricted use, distribution, and reproduction in any medium, provided you give appropriate credit to the original author(s) and the source, provide a link to the Creative Commons license, and indicate if changes were made.
Reprints and permissions
About this article
Cite this article.
Paltasingh, K.R., Goyari, P. Impact of farmer education on farm productivity under varying technologies: case of paddy growers in India. Agric Econ 6 , 7 (2018). https://doi.org/10.1186/s40100-018-0101-9
Download citation
Received : 31 March 2017
Accepted : 14 February 2018
Published : 02 May 2018
DOI : https://doi.org/10.1186/s40100-018-0101-9
Share this article
Anyone you share the following link with will be able to read this content:
Sorry, a shareable link is not currently available for this article.
Provided by the Springer Nature SharedIt content-sharing initiative
- Technology adoption
- Farm productivity
JEL classification
- DOI: 10.17221/74/2024-agricecon
- Corpus ID: 271474398
The effect of climate-smart agriculture on productivity and cost efficiency: Insights from smallholder wheat producers in Pakistan
- Panhwar Ghulam Mustafa , Shangao Wang , +2 authors Zhou Li
- Published in Agricultural Economics (Zeměd… 26 July 2024
- Agricultural and Food Sciences, Economics, Environmental Science
- Agricultural Economics (Zemědělská ekonomika)
54 References
Income and yield effects of climate-smart agriculture (csa) adoption in flood prone areas of bangladesh: farm level evidence, summary for policymakers, does adoption of climate-smart agriculture (csa) practices improve farmers’ crop income assessing the determinants and its impacts in punjab province, pakistan, what makes farmers exit farming: a case study of sindh province, pakistan, women participation: a productivity strategy in rice production, rethinking food production: nexus of mobile phones and production cost minimization, yield effects of climate-smart agriculture aid investment in southern malawi, towards cleaner production: certified seed adoption and its effect on technical efficiency, climate change, vulnerability, and its impacts in rural pakistan: a review, recuperating dynamism in agriculture through adoption of sustainable agricultural technology - implications for cleaner production, related papers.
Showing 1 through 3 of 0 Related Papers
Geo-spatial analysis: the impact of agriculture productivity, drought, and irrigation on poverty in East Java, Indonesia
- Rahmawati, Yessi
- Ichsan, Andiga Kusuma Nur
- Brintanti, Annisaa Rizky Dwi
- Jamil, Iqram Ramadhan
The aim of the present study is to empirically measure the contribution of drought and productivity of agriculture to the poverty in East Java, Indonesia. The study uses data from 2010 to 2020 by using Rice productivity, Drought as independent variable and poverty as dependent variable. The study includes other important explanatory variables such as human development indexes, irrigation, and GDRP. The present research utilizes the Spatial Econometrics-Random Effect Model which allows for controlling for regional dependency. The results confirm the presence of spatial autocorrelation in poverty, indicating that poverty can spread across the region. The study also reveals a positive and significant relationship between poverty and agricultural productivity growth. However, only wealthier persons, such as middlemen and farmers, may benefit from productivity development while small-scale farmers and vulnerable communities continue to live in poverty. As a consequence, impoverished individuals may need to resort to selling their assets or borrowing money to cover their daily expenses. The study shows a significant negative relationship between irrigation and poverty, highlighting the critical role irrigation plays in helping small-scale farmers increase their income. Therefore, the authors suggest that sustaining the Ministry of Public Works and Public Housing's irrigation policy could be an effective poverty alleviation strategy.
- Irrigation system;
- Rice productivity;
- Spatial autocorrelation;
Information
- Author Services
Initiatives
You are accessing a machine-readable page. In order to be human-readable, please install an RSS reader.
All articles published by MDPI are made immediately available worldwide under an open access license. No special permission is required to reuse all or part of the article published by MDPI, including figures and tables. For articles published under an open access Creative Common CC BY license, any part of the article may be reused without permission provided that the original article is clearly cited. For more information, please refer to https://www.mdpi.com/openaccess .
Feature papers represent the most advanced research with significant potential for high impact in the field. A Feature Paper should be a substantial original Article that involves several techniques or approaches, provides an outlook for future research directions and describes possible research applications.
Feature papers are submitted upon individual invitation or recommendation by the scientific editors and must receive positive feedback from the reviewers.
Editor’s Choice articles are based on recommendations by the scientific editors of MDPI journals from around the world. Editors select a small number of articles recently published in the journal that they believe will be particularly interesting to readers, or important in the respective research area. The aim is to provide a snapshot of some of the most exciting work published in the various research areas of the journal.
Original Submission Date Received: .
- Active Journals
- Find a Journal
- Proceedings Series
- For Authors
- For Reviewers
- For Editors
- For Librarians
- For Publishers
- For Societies
- For Conference Organizers
- Open Access Policy
- Institutional Open Access Program
- Special Issues Guidelines
- Editorial Process
- Research and Publication Ethics
- Article Processing Charges
- Testimonials
- Preprints.org
- SciProfiles
- Encyclopedia
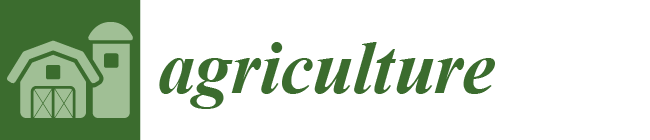
Article Menu
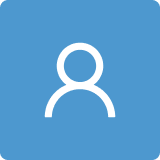
- Subscribe SciFeed
- Recommended Articles
- Google Scholar
- on Google Scholar
- Table of Contents
Find support for a specific problem in the support section of our website.
Please let us know what you think of our products and services.
Visit our dedicated information section to learn more about MDPI.
JSmol Viewer
Abiotic and biotic factors affecting crop growth and productivity: unique buckwheat production in egypt, 1. introduction, 2. materials and methods, 2.1. materials, sowing dates, 2.2. methods, 2.2.1. seeds, 2.2.2. experimental site analysis, 2.2.3. experimental site preparation, 2.2.4. data recorded, plant vegetative growth parameters, plant height (cm), number of branches per plant, whole-plant fresh weight (gm), harvesting of buckwheat grain samples, number of seeds per plant, total grain weight per square meter (kg), total grain yield per hectare (kg/ha), 2.3. the statistical analysis, 3.1. evaluation of the 2-way interaction effect of biotic and abiotic factors on the growth of buckwheat, 3.1.1. interaction between location and species, 3.1.2. interaction of location × planting date, 3.1.3. interaction effect of species × sowing time, 3.2. evaluation of the 2-way interaction between biotic and abiotic factors on the productivity of buckwheat, 3.2.1. interaction between location and species, 3.2.2. interaction of location × sowing time, 3.2.3. interaction of species × sowing date, 4. discussion, 4.1. evaluation of the 2-way interaction effect of biotic and abiotic factors on the growth of buckwheat, 4.2. evaluation of the 2-way interaction between biotic and abiotic factors on the productivity of buckwheat, 5. conclusions, author contributions, data availability statement, conflicts of interest.
- Barrett, C.B. Overcoming global food security challenges through science and solidarity. Am. J. Agric. Econ. 2021 , 103 , 422–447. [ Google Scholar ] [ CrossRef ]
- Keler, V.V.; Demeneva, A.A.; Martynova, O.V. Influence of various elements from cultivation technology on the buckwheat yield. IOP Conf. Ser. Earth Environ. Sci. 2021 , 659 , 12061. [ Google Scholar ] [ CrossRef ]
- Siracusa, L.; Gresta, F.; Sperlinga, E.; Ruberto, G. Effect of sowing time and soil water content on grain yield and phenolic profile of four buckwheat (Fagopyrum esculentum Moench.) varieties in a Mediterranean environment. J. Food Compos. Anal. 2017 , 62 , 1–7. [ Google Scholar ] [ CrossRef ]
- Song, J.N.; Liu, X.H.; Wang, Y.Q.; Yang, H.B. Transcriptome analysis reveals salinity responses in four Tartary buckwheat cultivars. J. Plant Biochem. Biotechnol. 2021 , 30 , 564–578. [ Google Scholar ] [ CrossRef ]
- Sowmya, S.A.; Vijayakumar, B.; Vishwanath, Y.C.; Ganiger, V.M.; Hegde, L.; Lokesh, M.S. Evaluation of buckwheat (Fagopyrum esculentum Moench.) genotypes under northern dry zone of Karnataka. Pharma Innov. J. 2021 , 10 , 1974–1979. [ Google Scholar ]
- Zhang, K.; He, M.; Fan, Y.; Zhao, H.; Gao, B.; Yang, K.; Zhou, M. Resequencing of global Tartary buckwheat accessions reveals multiple domestication events and key loci associated with agronomic traits. Genome Biol. 2021 , 22 , 23. [ Google Scholar ] [ CrossRef ] [ PubMed ]
- Tandon, H.L.S. Methods of Analysis of Soils, Plants, Waters, Fertilisers & Organic Manures ; Fertiliser Development and Consultation Organisation: New Delhi, India, 2005. [ Google Scholar ]
- Calegari, A.; Tiecher, T.; Wutke, E.B.; Canalli, L.D.S.; Bunch, R.; Rheinheimer, D.D.S. The role and management of soil mulch and cover crops in Conservation Agriculture systems. In Advances in Conservation Agriculture ; Burleigh Dodds Science Publishing: Cambridge, UK, 2020; pp. 179–248. [ Google Scholar ]
- Germ, M.; Gaberščik, A. The effect of environmental factors on buckwheat. In Molecular Breeding and Nutritional Aspects of Buckwheat ; Academic Press: Cambridge, MA, USA, 2016; pp. 273–281. [ Google Scholar ]
- Fesenko, N.N.; Glazova, Z.I.; Fesenko, I.N. Cold stress at seedlings stage of buckwheat optimizes development of both roots and aboveground biomass and limits the excessive vegetative growth interfering with seed formation (an analytical review). Acta Agric. Slov. 2020 , 116 , 5–10. [ Google Scholar ] [ CrossRef ]
- Hassona, M.M.; Hussein, A.S.; Morsy, N.; AAbd El-Aal, H. Chemical, rheological, sensorial and functional properties buckwheat semolina flour composite pasta. Egypt. J. Chem. 2023 , 66 , 467–478. [ Google Scholar ] [ CrossRef ]
- Mariotti, M.; Macchia, M.; Cerri, D.; Gatta, D.; Arduini, I.; Saccomanni, G. Rutin content in the forage and grain of common buckwheat (Fagopyrum esculentum) as affected by sowing time and irrigation in a Mediterranean environment. Crop Pasture Sci. 2020 , 71 , 171–182. [ Google Scholar ] [ CrossRef ]
- Salehi, A.; Fallah, S.; Neugschwandtner, R.W.; Mehdi, B.; Kaul, H.P. Growth analysis and land equivalent ratio of fenugreek-buckwheat intercrops at different fertilizer types. Die Bodenkult. J. Land Manag. Food Environ. 2018 , 69 , 105–119. [ Google Scholar ] [ CrossRef ]
- Khazaei, H.; Link, W.; Street, K.; Stoddard, F.L. ILB 938, a valuable faba bean ( Vicia faba L.) accession. Plant Genet. Resour. 2018 , 16 , 478–482. [ Google Scholar ] [ CrossRef ]
- Oomah, B.D.; Mazza, G. Flavonoids and antioxidative activities in buckwheat. J. Agric. Food Chem. 1996 , 44 , 1746–1750. [ Google Scholar ] [ CrossRef ]
- Jung, G.H.; Kim, S.L.; Kim, M.J.; Kim, S.K.; Park, J.H.; Kim, C.G.; Heu, S. Effect of sowing time on buckwheat (Fagopyrum esculentum Moench) growth and yield in central Korea. J. Crop Sci. Biotechnol. 2015 , 18 , 285–291. [ Google Scholar ] [ CrossRef ]
- Maletić, R.O.; Jevđović, R. The influence of meteorological conditions on major quantitative and qualitative traits of buckwheat (Fagopyrum esculentum Moench). J. Agric. Sci. 2003 , 48 , 11–19. [ Google Scholar ] [ CrossRef ]
- Facho, Z.H.; Khan, F.; Tao, W.; Ali, S. Species divergence and diversity in buckwheat landraces collected from the western himalayan region of pakistan. Pak. J. Bot 2019 , 51 , 2215–2224. [ Google Scholar ] [ CrossRef ] [ PubMed ]
- Brunori, A.; Végvári, G. Rutin content of the grain of buckwheat (Fagopyrum esculentum Moench. and Fagopyrum tataricum Gaertn.) varieties growtn in southern Italy. Acta Agron. Hung. 2007 , 55 , 265–272. [ Google Scholar ] [ CrossRef ]
- Larney, F.J.; Blackshaw, R.E. Weed seed viability in composted beef cattle feedlot manure. J. Environ. Qual. 2003 , 32 , 1105–1113. [ Google Scholar ] [ CrossRef ]
- Guo, X.D.; Ma, Y.J.; Parry, J.; Gao, J.M.; Yu, L.L.; Wang, M. Phenolics content and antioxidant activity of tartary buckwheat from different locations. Molecules 2011 , 16 , 9850–9867. [ Google Scholar ] [ CrossRef ]
- JoJohansson, E.; Kuktaite, R.; Labuschagne, M.; Lama, S.; Lan, Y.; Nakimbugwe, D.; Repo-Carrasco-Valencia, R.; Tafesse, F.; Tesfaye, K.; Vazquez, D. Adaptation to abiotic stress factors and their effects on cereal and pseudocereal grain quality. In Developing Sustainable and Health Promoting Cereals and Pseudocereals ; Academic Press: Cambridge, MA, USA, 2023; pp. 339–358. [ Google Scholar ]
- Boglaienko, D.; Soti, P.; Shetty, K.G.; Jayachandran, K. Buckwheat as a cover crop in Florida: Mycorrhizal Status and soil analysis. Agroecol. Sustain. Food Syst. 2014 , 38 , 1033–1046. [ Google Scholar ] [ CrossRef ]
- Omidbaigi, R.; Mastro, G.D.E. Influence of sowing time on the biological behaviour, biomass production, and rutin content of buckwheat (Fagopyrum esculentum Moench). Ital. J. Agron. 2004 , 8 , 47–50. [ Google Scholar ]
- Björkman, T.; Shail, J.W. Using a buckwheat cover crop for maximum weed suppression after early vegetables. HortTechnology 2013 , 23 , 575–580. [ Google Scholar ] [ CrossRef ]
- Snedecor, G.W.; Cochran, W.G. Statistical Methods , 8th ed.; Iowa State University Press: Ames, IA, USA, 1994. [ Google Scholar ]
- Bulan, M.T.S.; Stoltenberg, D.E.; Posner, J.L. Buckwheat species as summer cover crops for weed suppression in no-tillage vegetable cropping systems. Weed Sci. 2015 , 63 , 690–702. [ Google Scholar ] [ CrossRef ]
- Mariotti, M.; Masoni, A.; Arduini, I. Forage and grain yield of common buckwheat in Mediterranean conditions: Response to sowing time and irrigation. Crop Pasture Sci. 2016 , 67 , 1000–1008. [ Google Scholar ] [ CrossRef ]
Click here to enlarge figure
Location | Parameter | Belbies City Site | Sadat City Site |
---|---|---|---|
Soil Properties | |||
pH | - | 7.83 | 8.57 |
EC (dS/m) | Electrical Conductivity | 1.48 | 1.11 |
SP % | Saturation Percentage | 25 | - |
Soluble Anions (meq/L) | |||
CO | Carbonate | - | - |
HCO | Bicarbonate | 0.25 | 1.9 |
Cl | Chloride | 12.1 | 7.4 |
SO₄ | Sulfate | 1.56 | 3.4 |
Soluble Cations (meq/L) | |||
Ca | Calcium | 3.7 | 1.2 |
Mg | Magnesium | 1.8 | 1.2 |
Na | Sodium | 8 | 3.3 |
K | Potassium | 0.5 | 0.18 |
Soil Composition | |||
CaCO % | Calcium Carbonate | 3.9 | 1.7 |
OM % | Organic Matter | 1.2 | 0.35 |
Available Nutrients (mg/kg) | |||
N | Nitrogen | 175 | - |
P | Phosphorus | 183.6 | 5.3 |
K | Potassium | 7.96 | 508 |
Cu | Copper | 0.75 | 1.43 |
Fe | Iron | 5.25 | 1.11 |
Mn | Manganese | 2.43 | 20 |
Zn | Zinc | 2 | 2.75 |
Mechanical Analysis (%) | |||
Sand | - | 83 | 83 |
Silt | - | 15.5 | 10 |
Clay | - | 1.2 | 7 |
Texture Grade | - | Clay sandy | Sandy loamy |
Parameter | Unit | Belbies City Site | Sadat City Site |
---|---|---|---|
pH | - | 7.41 | 7.98 |
EC | dS/m | 0.64 | 1.26 |
Chemical Parameters | |||
Ca | meq/L | 1.70 | 4.20 |
Mg | meq/L | 1.00 | 2.60 |
Na | meq/L | 3.20 | 6.50 |
K | meq/L | 0.01 | 0.20 |
CO | meq/L | 0.00 | 0.00 |
HCO | meq/L | 0.21 | 4.00 |
Cl | meq/L | 4.70 | 7.00 |
SO₄ | meq/L | 10.9 | 2.50 |
SAR | - | 2.76 | 3.50 |
TDS | mg/L | 410.00 | 896.00 |
Trace Elements | |||
Fe | ppm | 0.27 | 0.11 |
Zn | ppm | 0.01 | 0.18 |
Mn | ppm | 0.20 | <0.01 |
Cu | ppm | 0.02 | <0.01 |
Location | Belbies City Site (BCS) | Sadat City Site (SCS) | |||
---|---|---|---|---|---|
Parameter | Season 1 (2018–2019) | Season 2 (2019–2020) | Season 1 (2018–2019) | Season 2 (2019–2020) | |
Temperature [°C] Max | 27.31–36.71 | 19.04–35.8 | 18.37–35.86 | 17.9–33.92 | |
Temperature [°C] Min | 8.51–24.48 | 9.75–21.27 | 7.97–23.87 | 9.57–20.64 | |
Temperature [°C] Mean | 13.65–30.37 | 14.18–28.24 | 12.92–29.33 | 13.61–26.81 | |
Precipitation [mm] Total | 0.00–0.47 | 0.00–1.42 | 0.00–2.00 | 0.00–1.12 | |
Relative Humidity [%] Max | 68.3–85.94 | 75.52–81.57 | 70.88–89.44 | 79.23–84.67 | |
Relative Humidity [%] Min | 19.63–33.3 | 22.22–35.56 | 21.26–37.89 | 24.26–42.92 | |
Wind Gust [km/h] Max | 27.53–40.62 | 30.6–39.4 | 28.36–41.82 | 30.7–41.12 | |
Sun Duration [min] Total | 492.23–777.84 | 413.8–804.67 | 514.65–788.43 | 402.65–802.5 | |
Soil Temperature [°C] Max | 28.01–40.08 | 19.7–38.58 | 20.32–43.27 | 20.31–40.71 | |
Soil Temperature [°C] Min | 11.27–28.89 | 12.57–26.29 | 9.00–26.96 | 10.87–23.56 | |
Soil Temperature [°C] Mean | 15.22–34.03 | 15.09–31.85 | 13.98–33.97 | 15.02–31.14 |
Treatments | Fagopyrum tataricum | Fagopyrum esculentum | L.S.D. at 0.05 | |||
---|---|---|---|---|---|---|
Parameters | Belbies City | Sadat City | Belbies City | Sadat City | ||
Plant height cm per plant | 1st Season | 97.704 a | 85.667 b | 75.296 c | 35.463 d | 6.6183 |
2nd Season | 90.741 a | 78.333 b | 63.185 c | 41.704 d | 3.6779 | |
Branch number per plant | 1st Season | 12.000 a | 10.111 b | 8.000 c | 6.185 d | 0.476 |
2nd Season | 10.704 a | 9.445 b | 7.778 c | 6.815 d | 0.334 | |
Internode number per plant | 1st Season | 14.111 a | 11.037 b | 8.889 c | 7.185 d | 0.597 |
2nd Season | 12.259 a | 10.889 b | 9.037 c | 7.296 d | 0.3517 | |
Number of leaves per plant | 1st Season | 46.852 a | 27.889 b | 18.778 c | 11.037 d | 2.6427 |
2nd Season | 31.778 a | 19.630 b | 14.370 c | 8.556 d | 2.872 | |
Fresh weight per plant gm | 1st Season | 36.188 a | 22.187 b | 14.330 c | 10.171 d | 1.6143 |
2nd Season | 39.356 a | 24.771 b | 16.013 c | 11.31 d | 1.881 |
Treatments | Belbies City Site | Sadat City Site | L.S.D. at 0.05 | |||||
---|---|---|---|---|---|---|---|---|
Parameters | Mid-Jan | Mid-Nov | Mid-Mar | Mid-Jan | Mid-Nov | Mid-Mar | ||
Plant height cm per plant | 1st Season | 88.222 a | 86.389 a | 84.889 a | 62.806 b | 61.389 b | 57.500 b | 8.106 |
2nd Season | 77.667 a | 77.778 a | 75.444 a | 60.444 b | 61.945 b | 57.667 b | 4.504 | |
Branch number per plant | 1st Season | 10.056 a | 9.944 a | 10.000 a | 8.222 b | 8.111 b | 8.111 b | 0.583 |
2nd Season | 9.223 a | 9.111 a | 9.389 a | 8.111 b | 8.222 b | 8.056 b | 0.409 | |
Internode number per plant | 1st Season | 11.611 a | 11.444 a | 11.445 a | 9.000 b | 9.056 b | 9.278 b | 0.731 |
2nd Season | 10.778 a | 10.778 a | 10.389 a | 9.222 b | 9.222 b | 8.833 b | 0.431 | |
Number of leaves per plant | 1st Season | 32.500 a | 30.167 b | 35.778 b | 17.722 c | 19.444 cd | 21.222 d | 3.237 |
2nd Season | 21.889 b | 21.889 b | 25.444 a | 13.834 c | 14.500 c | 13.944 c | 3.517 | |
Fresh weight per plant gm | 1st Season | 24.817 a | 24.323 ab | 26.637 b | 15.433 c | 15.239 d | 17.810 d | 1.977 |
2nd Season | 26.591 | 27.604 a | 28.857 a | 16.222 c | 16.979 c | 20.921 b | 2.304 |
Treatments | Fagopyrum tataricum | Fagopyrum esculentum | L.S.D. at 0.05 | |||||
---|---|---|---|---|---|---|---|---|
Parameters | Mid-Mar | Mid-Jan | Mid-Nov | Mid-Jan | Mid-Nov | Mid-Mar | ||
Plant height cm per plant | 1st Season | 95.778 a | 90.833 a | 88.444 a | 60.195 b | 59.333 b | 46.611 b | 8.1057 |
2nd Season | 89.389 a | 81.056 b | 83.167 b | 57.056 c | 56.556 c | 43.722 d | 4.5045 | |
Branch number per plant | 1st Season | 11.722 a | 10.833 b | 10.611 b | 7.445 c | 7.445 c | 6.389 d | 0.583 |
2nd Season | 10.500 a | 9.778 b | 9.945 b | 7.556 c | 7.389 c | 6.945 d | 0.4091 | |
Internode number per plant | 1st Season | 13.334 a | 12.278 b | 12.111 b | 8.334 c | 8.389 c | 7.389 d | 0.7312 |
2nd Season | 11.944 a | 11.389 b | 11.389 b | 8.611 c | 8.611 c | 7.278 d | 0.4307 | |
Leaf number per plant | 1st Season | 44.833 a | 33.722 b | 33.556 b | 16.500 c | 16.055 c | 12.166 d | 3.3267 |
2nd Season | 30.444 a | 23.278 b | 23.389 b | 12.445 c | 13.000 cd | 8.944 d | 3.5174 | |
Fresh weight per plant gm | 1st Season | 33.495 a | 27.483 b | 26.584 b | 12.767 c | 13.032 cd | 10.952 d | 1.977 |
2nd Season | 37.697 a | 28.418 b | 30.075 b | 14.395 c | 14.508 c | 12.081 d | 2.3037 |
Parameter | Number of Seeds Per Plant | Grains of 1 Meter (kg) | Productivity Rate kg/Hectare | ||||
---|---|---|---|---|---|---|---|
Treatment | 1st Season | 2nd Season | 1st Season | 2nd Season | 1st Season | 2nd Season | |
Fagopyrum tataricum | Belbies | 48.07 a | 49.63 a | 0.088.6 a | 0.0867 a | 859.38 a | 832.27 a |
Sadat | 25.04 b | 26.74 b | 0.0663 b | 0.0596 b | 661.43 b | 594.43 b | |
Fagopyrum esculentum | Belbies | 17.74 c | 18.59 c | 0.0499 c | 0.0467 c | 498.02 c | 467.72 c |
Sadat | 13.67 d | 14.63 d | 0.0314 d | 0.032 d | 316.32 d | 319.12 d | |
LSD at 0.05 | 3.407 | 3.1064 | 3.85 × 10 | 5.82 × 10 | 39.714 | 60.144 |
Parameter | Number of Seeds Per Plant | Grains of 1 Meter (kg) | Productivity Rate kg/Hectare | ||||
---|---|---|---|---|---|---|---|
Treatment | 1st Season | 2nd Season | 1st Season | 2nd Season | 1st Season | 2nd Season | |
Belbies City Site | Mid-Jan | 29.67 b | 32.78 b | 0.0673 b | 0.0655 b | 648.76 b | 627.3 b |
Mid-Nov | 31.33 b | 31.72 b | 0.0680 ab | 0.0615 b | 684.46 ab | 614.48 b | |
Mid-Mar | 37.72 a | 37.83 a | 0.0723 a | 0.073 a | 702.88 a | 708.21 a | |
Sadat City Site | Mid-Jan | 17.72 c | 19.61 cd | 0.0495 c | 0.0472 c | 481.99 c | 448.23 c |
Mid-Nov | 18.61 c | 19.22 d | 0.0482 c | 0.0457 c | 493.96 c | 477.56 c | |
Mid-Mar | 21.72 c | 23.22 c | 0.0490 c | 0.0445 c | 490.67 c | 444.55 c | |
LSD at 0.05 | 4.1727 | 3.8045 | 4.71 × 10 | 7.13 × 10 | 48.639 | 73.662 |
Parameter | Number of Seeds Per Plant | Grains of 1 Meter (kg) | Productivity Rate kg/Hectare | ||||
---|---|---|---|---|---|---|---|
Treatment | 1st Season | 2nd Season | 1st Season | 2nd Season | 1st Season | 2nd Season | |
Fagopyrum tataricum | Mid-Mar | 45.17 a | 45.72 a | 0.0858 a | 0.084 a | 837.55 a | 818.36 a |
Mid-Jan | 31.00 b | 35.39 b | 0.0743 b | 0.0698 b | 724.91 b | 663.09 b | |
Mid-Nov | 33.50 b | 33.44 b | 0.0722 b | 0.0655 b | 718.75 b | 658.60 b | |
Fagopyrum esculentum | Mid-Jan | 16.39 c | 17.00 c | 0.0425 c | 0.0428 c | 405.83 d | 412.44 c |
Mid-Nov | 16.44 c | 17.50 c | 0.0440 c | 0.0417 c | 459.68 c | 433.44 c | |
Mid-Mar | 14.28 c | 15.33 c | 0.0355 d | 0.0335 d | 356.00 e | 334.39 d | |
LSD at 0.05 | 4.1727 | 3.8045 | 4.71 × 10 | 7.13 × 10 | 48.639 | 73.662 |
The statements, opinions and data contained in all publications are solely those of the individual author(s) and contributor(s) and not of MDPI and/or the editor(s). MDPI and/or the editor(s) disclaim responsibility for any injury to people or property resulting from any ideas, methods, instructions or products referred to in the content. |
Share and Cite
Hassona, M.M.; Abd El-Aal, H.A.; Morsy, N.M.; Hussein, A.M.S. Abiotic and Biotic Factors Affecting Crop Growth and Productivity: Unique Buckwheat Production in Egypt. Agriculture 2024 , 14 , 1280. https://doi.org/10.3390/agriculture14081280
Hassona MM, Abd El-Aal HA, Morsy NM, Hussein AMS. Abiotic and Biotic Factors Affecting Crop Growth and Productivity: Unique Buckwheat Production in Egypt. Agriculture . 2024; 14(8):1280. https://doi.org/10.3390/agriculture14081280
Hassona, Mohamed M., Hala A. Abd El-Aal, Nahla M. Morsy, and Ahmed M. S. Hussein. 2024. "Abiotic and Biotic Factors Affecting Crop Growth and Productivity: Unique Buckwheat Production in Egypt" Agriculture 14, no. 8: 1280. https://doi.org/10.3390/agriculture14081280
Article Metrics
Article access statistics, further information, mdpi initiatives, follow mdpi.
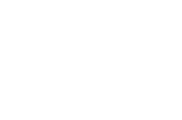
Subscribe to receive issue release notifications and newsletters from MDPI journals
Water Productivity in Agriculture: A Key to Sustainable Food Production
- December 2023
- 5(12):326-329

- Indian Institute of Technology Kharagpur

- Indian Institute of Technology Hyderabad

Discover the world's research
- 25+ million members
- 160+ million publication pages
- 2.3+ billion citations
- Mohamed E. A. El-Sayed

- Ahmed S. K. Fayed
- Rasha M. Badr Eldin

- Shashi Bhushan Kumar

- Damodhara Rao Mailapalli

- Dongping Shen
- ENVIRON RES LETT

- Francesco Fuso Nerini

- Qianmin Jia

- Zhikuan Jia
- AGR WATER MANAGE

- Recruit researchers
- Join for free
- Login Email Tip: Most researchers use their institutional email address as their ResearchGate login Password Forgot password? Keep me logged in Log in or Continue with Google Welcome back! Please log in. Email · Hint Tip: Most researchers use their institutional email address as their ResearchGate login Password Forgot password? Keep me logged in Log in or Continue with Google No account? Sign up
- Affiliated Professors
- Invited Researchers
- J-PAL Scholars
- Diversity, Equity, and Inclusion
- Code of Conduct
- Initiatives
- Latin America and the Caribbean
- Middle East and North Africa
- North America
- Southeast Asia
- Agriculture
- Crime, Violence, and Conflict
- Environment, Energy, and Climate Change
- Labor Markets
- Political Economy and Governance
- Social Protection
- Evaluations
- Research Resources
- Policy Insights
- Evidence to Policy
- For Affiliates
- Support J-PAL
The Abdul Latif Jameel Poverty Action Lab (J-PAL) is a global research center working to reduce poverty by ensuring that policy is informed by scientific evidence. Anchored by a network of more than 900 researchers at universities around the world, J-PAL conducts randomized impact evaluations to answer critical questions in the fight against poverty.
- Affiliated Professors Our affiliated professors are based at 97 universities and conduct randomized evaluations around the world to design, evaluate, and improve programs and policies aimed at reducing poverty. They set their own research agendas, raise funds to support their evaluations, and work with J-PAL staff on research, policy outreach, and training.
- Board Our Board of Directors, which is composed of J-PAL affiliated professors and senior management, provides overall strategic guidance to J-PAL, our sector programs, and regional offices.
- Diversity, Equity, and Inclusion J-PAL recognizes that there is a lack of diversity, equity, and inclusion in the field of economics and in our field of work. Read about what actions we are taking to address this.
- Initiatives J-PAL initiatives concentrate funding and other resources around priority topics for which rigorous policy-relevant research is urgently needed.
- Events We host events around the world and online to share results and policy lessons from randomized evaluations, to build new partnerships between researchers and practitioners, and to train organizations on how to design and conduct randomized evaluations, and use evidence from impact evaluations.
- Blog News, ideas, and analysis from J-PAL staff and affiliated professors.
- News Browse news articles about J-PAL and our affiliated professors, read our press releases and monthly global and research newsletters, and connect with us for media inquiries.
- Press Room Based at leading universities around the world, our experts are economists who use randomized evaluations to answer critical questions in the fight against poverty. Connect with us for all media inquiries and we'll help you find the right person to shed insight on your story.
- Overview J-PAL is based at MIT in Cambridge, MA and has seven regional offices at leading universities in Africa, Europe, Latin America and the Caribbean, Middle East and North Africa, North America, South Asia, and Southeast Asia.
- Global Our global office is based at the Department of Economics at the Massachusetts Institute of Technology. It serves as the head office for our network of seven independent regional offices.
- Africa J-PAL Africa is based at the Southern Africa Labour & Development Research Unit (SALDRU) at the University of Cape Town in South Africa.
- Europe J-PAL Europe is based at the Paris School of Economics in France.
- Latin America and the Caribbean J-PAL Latin America and the Caribbean is based at the Pontificia Universidad Católica de Chile.
- Middle East and North Africa J-PAL MENA is based at the American University in Cairo, Egypt.
- North America J-PAL North America is based at the Massachusetts Institute of Technology in the United States.
- South Asia J-PAL South Asia is based at the Institute for Financial Management and Research (IFMR) in India.
- Southeast Asia J-PAL Southeast Asia is based at the Faculty of Economics and Business at the University of Indonesia (FEB UI).
- Overview Led by affiliated professors, J-PAL sectors guide our research and policy work by conducting literature reviews; by managing research initiatives that promote the rigorous evaluation of innovative interventions by affiliates; and by summarizing findings and lessons from randomized evaluations and producing cost-effectiveness analyses to help inform relevant policy debates.
- Agriculture How can we encourage small farmers to adopt proven agricultural practices and improve their yields and profitability?
- Crime, Violence, and Conflict What are the causes and consequences of crime, violence, and conflict and how can policy responses improve outcomes for those affected?
- Education How can students receive high-quality schooling that will help them, their families, and their communities truly realize the promise of education?
- Environment, Energy, and Climate Change How can we increase access to energy, reduce pollution, and mitigate and build resilience to climate change?
- Finance How can financial products and services be more affordable, appropriate, and accessible to underserved households and businesses?
- Firms How do policies affecting private sector firms impact productivity gaps between higher-income and lower-income countries? How do firms’ own policies impact economic growth and worker welfare?
- Gender How can we reduce gender inequality and ensure that social programs are sensitive to existing gender dynamics?
- Health How can we increase access to and delivery of quality health care services and effectively promote healthy behaviors?
- Labor Markets How can we help people find and keep work, particularly young people entering the workforce?
- Political Economy and Governance What are the causes and consequences of poor governance and how can policy improve public service delivery?
- Social Protection How can we identify effective policies and programs in low- and middle-income countries that provide financial assistance to low-income families, insuring against shocks and breaking poverty traps?
Leveraging Private Sector Innovation to Generate Evidence on Farmers' Productivity, Profitability, and Food Security in Africa: Framing a Research Agenda for the UM6P-J-PAL Agricultural Lab for Africa (UJALA)
The UM6P-J-PAL Agricultural Lab for Africa (UJALA) aims to generate rigorous, policy-relevant evidence to answer critical questions around small-scale agriculture and food security in Africa.
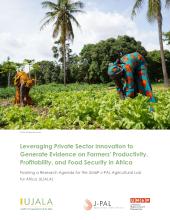
Post-Doctoral Fellow in Agricultural Economics, Sean Kiely 2024-2025
Investigator, supported by the us department of agriculture, more from nber.
In addition to working papers , the NBER disseminates affiliates’ latest findings through a range of free periodicals — the NBER Reporter , the NBER Digest , the Bulletin on Retirement and Disability , the Bulletin on Health , and the Bulletin on Entrepreneurship — as well as online conference reports , video lectures , and interviews .
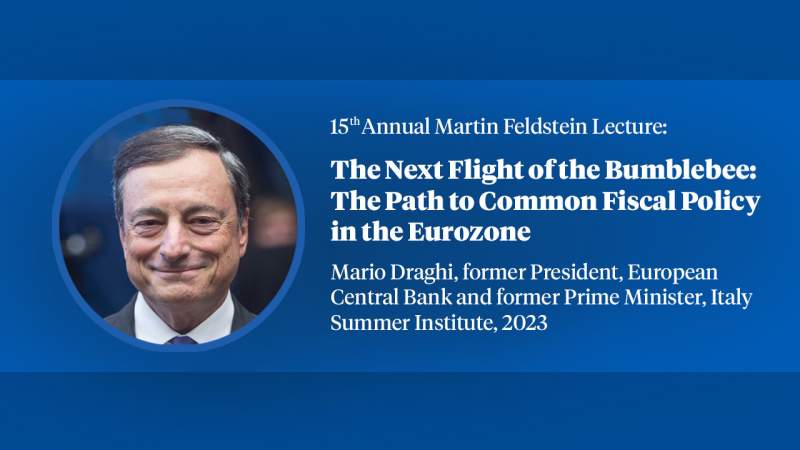
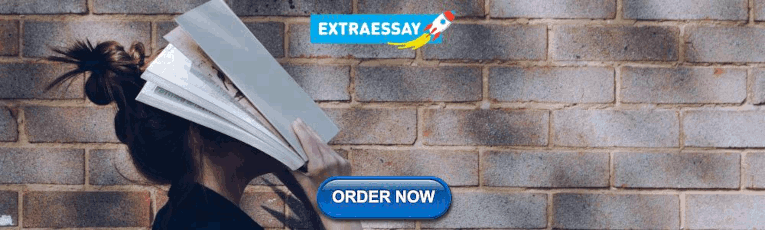
IMAGES
VIDEO
COMMENTS
The European Innovation Partnership Agricultural Productivity and Sustainability's SunBot project is an illustration of this strategy in action (EIP-AGRI). The project, which started in late 2018, has focused on automating a solar-powered tractor and mower for efficient utilization of resources in berry orchards [27]. The goal of the project is ...
Sectoral gaps and cross-country productivity differences. The importance of agricultural productivity differences in explaining cross-country income differences was the focus of accounting work by Córdoba and Ripoll (Citation 2009), Gollin et al. (Citation 2007), Restuccia et al. (Citation 2008), Vollrath (Citation 2009), and others.These papers underscored that, in a proximate sense, cross ...
This paper projects and analyzes the regional impacts of climate change on the agricultural productivity of family farming and large-scale agriculture in Brazil between 2021 and 2050, using the ...
To contribute to these debates, this study employs a panel data spanning 26 year (1990-2015) and including 13 ECOWAS countries to analyze the impact of production factors on agricultural ...
Agricultural Economics is an international journal publishing research results and policy analyses from across disciplines, from food consumption to land use. ... Search for more papers by this author. Abdullah Mamun ... This study examines the impact of subsidies on productivity growth in agriculture globally using a long time series on the ...
1 Introduction. Uncertainty in projections makes it difficult to determine the precise impact of climate change on future agricultural productivity, but studies have consistently found that under most scenarios significant negative effects should be expected worldwide [1-6] and especially in economically underdeveloped regions [7-9].Importantly, agricultural production is not only affected ...
Farooq et al. (2020) surveyed 67 research papers that published through 2006 to 2019 on the use of IoT in different agricultural applications; ... Furthermore, one of the significant natural problems influencing agricultural productivity is climate change (Srivastava and Singh,2016). The same authors used GIS-based integrated modeling, which ...
In recent decades, world agriculture has undergone a vast transformation. Between 1961 and 2020, global agricultural output increased nearly four‐fold while population grew 2.6 times, leading to a 53 per cent increase in output per capita. Real food prices declined, providing for more affordable and diverse diets. There was a pronounced and sustained shift in the location of production to ...
Journal of Geographical Sciences (2023) Conservation agriculture (CA) is being promoted as a set of management practices that can sustain crop production while providing positive environmental ...
Precision agriculture employs cutting-edge technologies to increase agricultural productivity while reducing adverse impacts on the environment. Precision agriculture is a farming approach that uses advanced technology and data analysis to maximize crop yields, cut waste, and increase productivity. It is a potential strategy for tackling some of the major issues confronting contemporary ...
Background In agricultural-dependent economies, extension programmes have been the main conduit for disseminating information on farm technologies, support rural adult learning and assist farmers in developing their farm technical and managerial skills. It is expected that extension programmes will help increase farm productivity, farm revenue, reduce poverty and minimize food insecurity. In ...
Agricultural productivity growth is key to rapid structural transformation of the economy. More than 50 years after the start of the green revolution, India's reliance on agriculture as the mainstay of livelihoods continues to be high. Against this backdrop, this paper examines trends in labour and total factor productivity in Indian agriculture. We first examine trends in the differences in ...
This paper considers the relationship between farm size and productivity. It begins by discussing measurement issues and conceptual issues related to agricultural productivity, including the well-documented difficulty of measuring inputs and outputs in smallholder production systems. The paper then considers the relationship between farm size and
Background Agroforestry bridges the gap that often separates agriculture and forestry by building integrated systems that address both environmental and socio-economic objectives. Agroforestry can improve the resiliency of agricultural systems and mitigate the impacts of climate change. Existing research suggests that integrating trees on farms can prevent environmental degradation, improve ...
Growing agricultural productivity has often been seen as the key to rapid economic development. In this paper, it is argued that agricultural productivity growth plays an important role in institutional development. It is hypothesized that agricultural productivity growth leads to structural change both inside the rural agricultural sector and between this sector and modern manufacturing and ...
This paper reviews recent literature relevant to the impacts of climate change on global agricultural productivity through a wide range of processes. The aim is to provide a global-scale overview of all relevant impacts, rather than focusing on specific regions or processes, as the purpose of this review is to inform a wider assessment of the ...
In developing countries, poverty, food insecurity and declined agricultural productivity are common issues, which indicate the need for mitigation and adaptation measures to sustain productivity (Clair and Lynch, 2010; Lybbert and Sumner, 2012; Mbow et al., 2014). At the national and regional level, the insurance of food security is the major ...
Climate change and its impact on agriculture productivity vary among crops and regions. The southeastern United States (SE-US) is agro-ecologically diversified, economically dependent on ...
For this reason, Kassie et al. (Citation 2011) argue that the adoption of research and improved technology is key to expanding agricultural productivity and production while mitigating rural poverty for sustained livelihoods. In many developed countries, "extension" is the motive force for yield enhancements (Labarthe & Laurent, Citation 2013).
This paper reviews recent literature relevant to the impacts of climate change on global agricultural productivity through a wide range of processes. The aim is to provide a global-scale overview of all relevant impacts, rather than focusing on specific regions or processes, as the purpose of this review is to inform a wider assessment of the ...
sion services on the productivity of farmers. This study investigates the efects of agricultura. extension services on farm yields in Uganda.Available empirical evidence from previous studies on the efect of extension services on agricultural productivity in Uganda provides mixed results (see, e.g., Hasan et al., 2013; Nkonya et al., 2009 ...
This paper analyzes the effects of education on farm productivity in the case of growers of modern and traditional varieties of paddy in Odisha, Eastern India. Using an endogenous switching regression model, the study has found that a minimum threshold level of education is significantly influencing the adoption of modern varieties of paddy and thereby the farm productivity of adopters only.
@article{Mustafa2024TheEO, title={The effect of climate-smart agriculture on productivity and cost efficiency: Insights from smallholder wheat producers in Pakistan}, author={Panhwar Ghulam Mustafa and Shangao Wang and Gershom Endelani Mwalupaso and Yi Yu and Zhou Li}, journal={Agricultural Economics (Zemědělsk{\'a} ekonomika)}, year={2024 ...
The geographical distribution of the research papers by states/regions and the publication trends are shown in Table S1 and Fig. S1, respectively. Maximum study sites are located in the Indian state of Uttarakhand, followed by Himachal Pradesh, Karnataka, Odisha, Maharashtra, Punjab, Madhya Pradesh, and Uttar Pradesh.
The aim of the present study is to empirically measure the contribution of drought and productivity of agriculture to the poverty in East Java, Indonesia. The study uses data from 2010 to 2020 by using Rice productivity, Drought as independent variable and poverty as dependent variable. The study includes other important explanatory variables such as human development indexes, irrigation, and ...
Egypt did not previously grow buckwheat, due to the belief that the environment does not meet the factors of growth, development, and productivity in an arid or semiarid region. The present study investigated two species of buckwheat, Fagopyrum tataricum (FT) and Fagopyrum esculentum (FE), which were planted in two different soil, weather, and water property sites, including the first in ...
Empirically, less developed countries feature higher distortions and larger dispersion in firm-level productivity, mostly resulting from the higher prevalence of unproductive firms. Quantitatively, measured cross-country differences in the elasticity of distortions with respect to firm productivity generate the bulk of empirical patterns and ...
water productivity — the net return per unit of water used — is essential. The goal is to produce more food, income, livelihoods, and ecosystem services while using less water (Molden and ...
Framing a Research Agenda for the UM6P-J-PAL Agricultural Lab for Africa (UJALA). Leveraging Private Sector Innovation to Generate Evidence on Farmers' Productivity, Profitability, and Food Security in Africa: Framing a Research Agenda for the UM6P-J-PAL Agricultural Lab for Africa (UJALA) | The Abdul Latif Jameel Poverty Action Lab
In addition to working papers, the NBER disseminates affiliates' latest findings through a range of free periodicals — the NBER Reporter, the NBER Digest, the Bulletin on Retirement and Disability, the Bulletin on Health, and the Bulletin on Entrepreneurship — as well as online conference reports, video lectures, and interviews.