9 Great Ways to Teach Variables in Science Experiments
by Katrina | Feb 17, 2024 | Pedagogy , Science | 1 comment
Science is a journey of exploration and discovery, and at the heart of every scientific experiment lies the concept of variables. Variables in science experiments are the building blocks of experimentation, allowing scientists to manipulate and measure different elements to draw meaningful conclusions.
Teaching students about variables is crucial for developing their scientific inquiry skills and fostering a deeper understanding of the scientific method.
In this blog post, we’ll explore the importance of teaching variables in science experiments, delve into the distinctions between independent, dependent, and controlled variables, and provide creative ideas on how to effectively teach these variable types.
So grab a coffee, find a comfy seat, and relax while we explore fun ways to teach variables in science experiments!

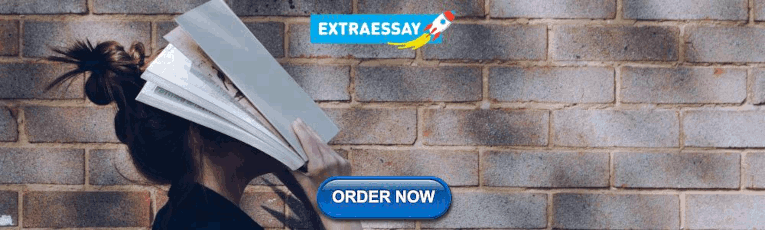
The Importance of Teaching Variables in Science Experiments:
Foundation of Scientific Inquiry: Variables form the bedrock of the scientific method. Teaching students about variables helps them grasp the fundamental principles of scientific inquiry, enabling them to formulate hypotheses, design experiments, and draw valid conclusions.
Critical Thinking Skills: Understanding variables cultivates critical thinking skills in students. It encourages them to analyze the relationships between different factors, question assumptions, and think systematically when designing and conducting experiments.
Real-world Application: Variables are not confined to the laboratory; they exist in everyday life. Teaching students about variables equips them with the skills to critically assess and interpret the multitude of factors influencing phenomena in the real world, fostering a scientific mindset beyond the classroom.
In addition to the above, understanding scientific variables is crucial for designing an experiment and collecting valid results because variables are the building blocks of the scientific method.
A well-designed experiment involves the careful manipulation and measurement of variables to test hypotheses and draw meaningful conclusions about the relationships between different factors. Here are several reasons why a clear understanding of scientific variables is essential for the experimental process:
1. Precision and Accuracy: By identifying and defining variables, researchers can design experiments with precision and accuracy. This clarity helps ensure that the measurements and observations made during the experiment are relevant to the research question, reducing the likelihood of errors or misinterpretations.
2. Hypothesis Testing: Variables in science experiments are central to hypothesis formulation and testing. A hypothesis typically involves predicting the relationship between an independent variable (the one manipulated) and a dependent variable (the one measured). Understanding these variables is essential for constructing a hypothesis that can be tested through experimentation.
3. Controlled Experiments: Variables, especially controlled variables, enable researchers to conduct controlled experiments. By keeping certain factors constant (controlled variables) while manipulating others (independent variable), scientists can isolate the impact of the independent variable on the dependent variable. This control is essential for drawing valid conclusions about cause-and-effect relationships.
4. Reproducibility: Clear identification and understanding of variables enhance the reproducibility of experiments. When other researchers attempt to replicate an experiment, a detailed understanding of the variables involved ensures that they can accurately reproduce the conditions and obtain similar results.
5. Data Interpretation: Knowing the variables in science experiments allows for a more accurate interpretation of the collected data. Researchers can attribute changes in the dependent variable to the manipulation of the independent variable and rule out alternative explanations. This is crucial for drawing reliable conclusions from the experimental results.
6. Elimination of Confounding Factors: Without a proper understanding of variables, experiments are susceptible to confounding factors—unintended variables that may influence the results. Through careful consideration of all relevant variables, researchers can minimize the impact of confounding factors and increase the internal validity of their experiments.
7. Optimization of Experimental Design: Understanding variables in science experiments helps researchers optimize the design of their experiments. They can choose the most relevant and influential variables to manipulate and measure, ensuring that the experiment is focused on addressing the specific research question.
8. Applicability to Real-world Situations: A thorough understanding of variables enhances the applicability of experimental results to real-world situations. It allows researchers to draw connections between laboratory findings and broader phenomena, contributing to the advancement of scientific knowledge and its practical applications.
The Different Types of Variables in Science Experiments:
There are 3 main types of variables in science experiments; independent, dependent, and controlled variables.
1. Independent Variable:
The independent variable is the factor that is deliberately manipulated or changed in an experiment. The independent variable affects the dependent variable (the one being measured).
Example : In a plant growth experiment, the amount of sunlight the plants receive can be the independent variable. Researchers might expose one group of plants to more sunlight than another group.
2. Dependent Variable:
The dependent variable is the outcome or response that is measured in an experiment. It depends on the changes made to the independent variable.
Example : In the same plant growth experiment, the height of the plants would be the dependent variable. This is what researchers would measure to determine the effect of sunlight on plant growth.
3. Controlled Variable:
Controlled variables, also called constant variables, are the factors in an experiment that are kept constant to ensure that any observed changes in the dependent variable are a result of the manipulation of the independent variable. These are not to be confused with control groups.
In a scientific experiment in chemistry, a control group is a crucial element that serves as a baseline for comparison. The control group is designed to remain unchanged or unaffected by the independent variable, which is the variable being manipulated in the experiment.
The purpose of including a control group is to provide a reference point against which the experimental results can be compared, helping scientists determine whether the observed effects are a result of the independent variable or other external factors.
Example : In the plant growth experiment, factors like soil type, amount of water, type of plant and temperature would be control variables. Keeping these constant ensures that any differences in plant height can be attributed to changes in sunlight.
Science variables in science experiments

Want a FREE digital escape room?
Enter your email address here and I'll send you a FREE no-prep digital escape room on the topic 'Scientific method & science equipment'.
You have successfully joined our subscriber list.
We will not send you spam.
You can unsubscribe at any time.
Best resources for reviewing variables in science experiments:
If you’re short on time and would rather buy your resources, then I’ve compiled a list of my favorite resources for teaching and reviewing variables in science experiments below. While there is nothing better than actually doing science experiments, this isn’t feasible every lesson and these resources are great for consolidation of learning:
1. FREE Science Variables Posters : These are perfect as a visual aide in your classroom while also providing lab decorations! Print in A4 or A3 size to make an impact.
2. Variable scenarios worksheet printable : Get your students thinking about variable with these train your pet dragon themed scenarios. Students identify the independent variable, dependent variable and controlled variables in each scenario.
3. Variable Valentines scenarios worksheet printable : Get your students thinking about variables with these cupid Valentine’s Day scenarios. Students identify the independent variable, dependent variable and controlled variables in each scenario.
4. Variable Halloween scenarios worksheet printable : Spook your students with these Halloween themed scenarios. Students identify the independent variable, dependent variable and controlled variables in each scenario.
5. Scientific Method Digital Escape Room : Review all parts of the scientific method with this fun (zero prep) digital escape room!
6. Scientific Method Stations Printable or Sub Lesson : The worst part of being a teacher? Having to still work when you are sick! This science sub lesson plan includes a fully editable lesson plan designed for a substitute teacher to take, including differentiated student worksheets and full teacher answers. This lesson involves learning about all parts of the scientific method, including variables.

Digital Lab Equipment Escape Room Middle School Science

Scientific Method Digital Escape Room Science Middle School

Science Graphing Skills Escape Room Middle School
9 teaching strategies for variables in science experiments.
To help engage students in learning about the different types of scientific variables, it is important to include a range of activities and teaching strategies. Here are some suggestions:
1. Hands-on Experiments: Conducting hands-on experiments is one of the most effective ways to teach students about variables. Provide students with the opportunity to design and conduct their experiments, manipulating and measuring variables to observe outcomes.
Easy science experiments you could include might relate to student heart rate (e.g. before and after exercise), type of ball vs height it bounces, amount of sunlight on the growth of a plant, the strength of an electromagnet (copper wire around a nail) vs the number of coils.
Change things up by sometimes having students identify the independent variable, dependent variable and controlled variables before the experiment, or sometimes afterwards.
Consolidate by graphing results and reinforcing that the independent variable goes alone the x-axis while the dependent variable goes on the y-axis.
2. Teacher Demonstrations:
Use demonstrations to illustrate the concepts of independent, dependent, and controlled variables. For instance, use a simple chemical reaction where the amount of reactant (independent variable) influences the amount of product formed (dependent variable), with temperature and pressure controlled.
3. Case Studies:
Introduce case studies that highlight real-world applications of variables in science experiments. Discuss famous experiments or breakthroughs in science where variables played a crucial role. This approach helps students connect theoretical knowledge to practical situations.
4. Imaginary Situations:
Spark student curiosity and test their understanding of the concept of variables in science experiments by providing imaginary situations or contexts for students to apply their knowledge. Some of my favorites to use are this train your pet dragon and Halloween themed variables in science worksheets.
5. Variable Sorting Activities:
Engage students with sorting activities where they categorize different variables in science experiments into independent, dependent, and controlled variables. This hands-on approach encourages active learning and reinforces their understanding of variable types.
6. Visual Aids:
Utilize visual aids such as charts, graphs, and diagrams to visually represent the relationships between variables. Visualizations can make abstract concepts more tangible and aid in the comprehension of complex ideas.
7. Technology Integration:
Leverage technology to enhance variable teaching. Virtual simulations and interactive apps can provide a dynamic platform for students to manipulate variables in a controlled environment, fostering a deeper understanding of the cause-and-effect relationships.
Websites such as Phet are a great tool to use to model these types of scientific experiments and to identify and manipulate the different variables
8. Group Discussions:
Encourage group discussions where students can share their insights and experiences related to variables in science experiments. This collaborative approach promotes peer learning and allows students to learn from each other’s perspectives.
9. Digital Escape Rooms:
Reinforce learning by using a fun interactive activity like this scientific method digital escape room.

Body Systems Worksheets, Stations and Activities Bundle

The Musculoskeletal System Worksheet Stations

Engaging Cardiovascular System Worksheet Stations
Teaching variables in science experiments is an essential component of science education, laying the groundwork for critical thinking, inquiry skills, and a lifelong appreciation for the scientific method.
By emphasizing the distinctions between independent, dependent, and controlled variables and employing creative teaching strategies, educators can inspire students to become curious, analytical, and scientifically literate individuals.
What are your favorite ways to engage students in learning about the different types of variables in science experiments? Comment below!
Note: Always consult your school’s specific safety guidelines and policies, and seek guidance from experienced colleagues or administrators when in doubt about safety protocols.
Teaching variables in science experiments
About the Author

Katrina Harte is a multi-award winning educator from Sydney, Australia who specialises in creating resources that support teachers and engage students.
Station activities for middle school
Great web site you’ve got here.. It’s difficult to find excellent writing like yours nowadays. I truly appreciate individuals like you! Take care!!
Recent Posts

Check out my best selling no-prep lesson activities!
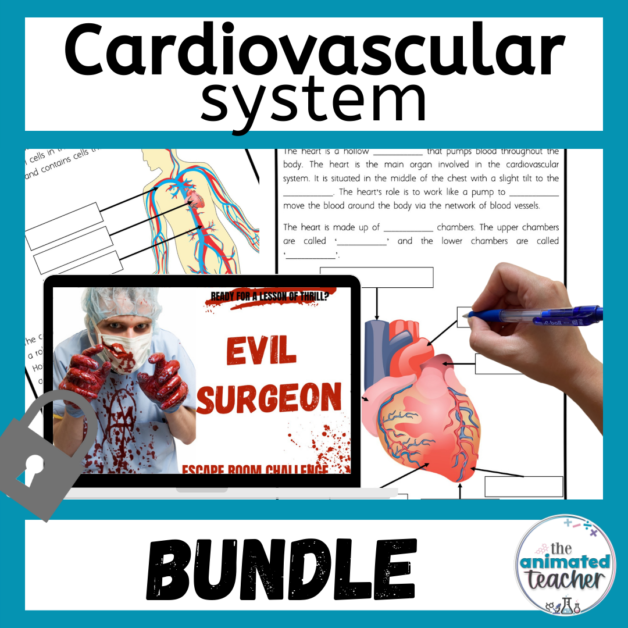

15 Independent and Dependent Variable Examples
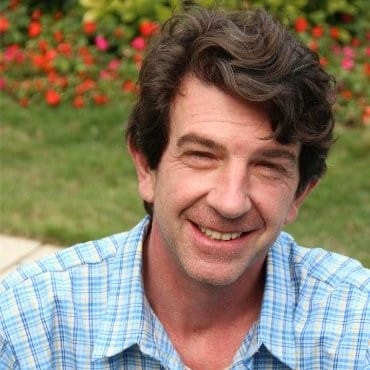
Dave Cornell (PhD)
Dr. Cornell has worked in education for more than 20 years. His work has involved designing teacher certification for Trinity College in London and in-service training for state governments in the United States. He has trained kindergarten teachers in 8 countries and helped businessmen and women open baby centers and kindergartens in 3 countries.
Learn about our Editorial Process
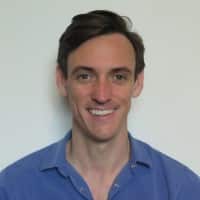
Chris Drew (PhD)
This article was peer-reviewed and edited by Chris Drew (PhD). The review process on Helpful Professor involves having a PhD level expert fact check, edit, and contribute to articles. Reviewers ensure all content reflects expert academic consensus and is backed up with reference to academic studies. Dr. Drew has published over 20 academic articles in scholarly journals. He is the former editor of the Journal of Learning Development in Higher Education and holds a PhD in Education from ACU.

An independent variable (IV) is what is manipulated in a scientific experiment to determine its effect on the dependent variable (DV).
By varying the level of the independent variable and observing associated changes in the dependent variable, a researcher can conclude whether the independent variable affects the dependent variable or not.
This can provide very valuable information when studying just about any subject.
Because the researcher controls the level of the independent variable, it can be determined if the independent variable has a causal effect on the dependent variable.
The term causation is vitally important. Scientists want to know what causes changes in the dependent variable. The only way to do that is to manipulate the independent variable and observe any changes in the dependent variable.
Definition of Independent and Dependent Variables
The independent variable and dependent variable are used in a very specific type of scientific study called the experiment .
Although there are many variations of the experiment, generally speaking, it involves either the presence or absence of the independent variable and the observation of what happens to the dependent variable.
The research participants are randomly assigned to either receive the independent variable (called the treatment condition), or not receive the independent variable (called the control condition).
Other variations of an experiment might include having multiple levels of the independent variable.
If the independent variable affects the dependent variable, then it should be possible to observe changes in the dependent variable based on the presence or absence of the independent variable.
Of course, there are a lot of issues to consider when conducting an experiment, but these are the basic principles.
These concepts should not be confused with predictor and outcome variables .
Examples of Independent and Dependent Variables
1. gatorade and improved athletic performance.
A sports medicine researcher has been hired by Gatorade to test the effects of its sports drink on athletic performance. The company wants to claim that when an athlete drinks Gatorade, their performance will improve.
If they can back up that claim with hard scientific data, that would be great for sales.
So, the researcher goes to a nearby university and randomly selects both male and female athletes from several sports: track and field, volleyball, basketball, and football. Each athlete will run on a treadmill for one hour while their heart rate is tracked.
All of the athletes are given the exact same amount of liquid to consume 30-minutes before and during their run. Half are given Gatorade, and the other half are given water, but no one knows what they are given because both liquids have been colored.
In this example, the independent variable is Gatorade, and the dependent variable is heart rate.
2. Chemotherapy and Cancer
A hospital is investigating the effectiveness of a new type of chemotherapy on cancer. The researchers identified 120 patients with relatively similar types of cancerous tumors in both size and stage of progression.
The patients are randomly assigned to one of three groups: one group receives no chemotherapy, one group receives a low dose of chemotherapy, and one group receives a high dose of chemotherapy.
Each group receives chemotherapy treatment three times a week for two months, except for the no-treatment group. At the end of two months, the doctors measure the size of each patient’s tumor.
In this study, despite the ethical issues (remember this is just a hypothetical example), the independent variable is chemotherapy, and the dependent variable is tumor size.
3. Interior Design Color and Eating Rate
A well-known fast-food corporation wants to know if the color of the interior of their restaurants will affect how fast people eat. Of course, they would prefer that consumers enter and exit quickly to increase sales volume and profit.
So, they rent space in a large shopping mall and create three different simulated restaurant interiors of different colors. One room is painted mostly white with red trim and seats; one room is painted mostly white with blue trim and seats; and one room is painted mostly white with off-white trim and seats.
Next, they randomly select shoppers on Saturdays and Sundays to eat for free in one of the three rooms. Each shopper is given a box of the same food and drink items and sent to one of the rooms. The researchers record how much time elapses from the moment they enter the room to the moment they leave.
The independent variable is the color of the room, and the dependent variable is the amount of time spent in the room eating.
4. Hair Color and Attraction
A large multinational cosmetics company wants to know if the color of a woman’s hair affects the level of perceived attractiveness in males. So, they use Photoshop to manipulate the same image of a female by altering the color of her hair: blonde, brunette, red, and brown.
Next, they randomly select university males to enter their testing facilities. Each participant sits in front of a computer screen and responds to questions on a survey. At the end of the survey, the screen shows one of the photos of the female.
At the same time, software on the computer that utilizes the computer’s camera is measuring each male’s pupil dilation. The researchers believe that larger dilation indicates greater perceived attractiveness.
The independent variable is hair color, and the dependent variable is pupil dilation.
5. Mozart and Math
After many claims that listening to Mozart will make you smarter, a group of education specialists decides to put it to the test. So, first, they go to a nearby school in a middle-class neighborhood.
During the first three months of the academic year, they randomly select some 5th-grade classrooms to listen to Mozart during their lessons and exams. Other 5 th grade classrooms will not listen to any music during their lessons and exams.
The researchers then compare the scores of the exams between the two groups of classrooms.
Although there are a lot of obvious limitations to this hypothetical, it is the first step.
The independent variable is Mozart, and the dependent variable is exam scores.
6. Essential Oils and Sleep
A company that specializes in essential oils wants to examine the effects of lavender on sleep quality. They hire a sleep research lab to conduct the study. The researchers at the lab have their usual test volunteers sleep in individual rooms every night for one week.
The conditions of each room are all exactly the same, except that half of the rooms have lavender released into the rooms and half do not. While the study participants are sleeping, their heart rates and amount of time spent in deep sleep are recorded with high-tech equipment.
At the end of the study, the researchers compare the total amount of time spent in deep sleep of the lavender-room participants with the no lavender-room participants.
The independent variable in this sleep study is lavender, and the dependent variable is the total amount of time spent in deep sleep.
7. Teaching Style and Learning
A group of teachers is interested in which teaching method will work best for developing critical thinking skills.
So, they train a group of teachers in three different teaching styles : teacher-centered, where the teacher tells the students all about critical thinking; student-centered, where the students practice critical thinking and receive teacher feedback; and AI-assisted teaching, where the teacher uses a special software program to teach critical thinking.
At the end of three months, all the students take the same test that assesses critical thinking skills. The teachers then compare the scores of each of the three groups of students.
The independent variable is the teaching method, and the dependent variable is performance on the critical thinking test.
8. Concrete Mix and Bridge Strength
A chemicals company has developed three different versions of their concrete mix. Each version contains a different blend of specially developed chemicals. The company wants to know which version is the strongest.
So, they create three bridge molds that are identical in every way. They fill each mold with one of the different concrete mixtures. Next, they test the strength of each bridge by placing progressively more weight on its center until the bridge collapses.
In this study, the independent variable is the concrete mixture, and the dependent variable is the amount of weight at collapse.
9. Recipe and Consumer Preferences
People in the pizza business know that the crust is key. Many companies, large and small, will keep their recipe a top secret. Before rolling out a new type of crust, the company decides to conduct some research on consumer preferences.
The company has prepared three versions of their crust that vary in crunchiness, they are: a little crunchy, very crunchy, and super crunchy. They already have a pool of consumers that fit their customer profile and they often use them for testing.
Each participant sits in a booth and takes a bite of one version of the crust. They then indicate how much they liked it by pressing one of 5 buttons: didn’t like at all, liked, somewhat liked, liked very much, loved it.
The independent variable is the level of crust crunchiness, and the dependent variable is how much it was liked.
10. Protein Supplements and Muscle Mass
A large food company is considering entering the health and nutrition sector. Their R&D food scientists have developed a protein supplement that is designed to help build muscle mass for people that work out regularly.
The company approaches several gyms near its headquarters. They enlist the cooperation of over 120 gym rats that work out 5 days a week. Their muscle mass is measured, and only those with a lower level are selected for the study, leaving a total of 80 study participants.
They randomly assign half of the participants to take the recommended dosage of their supplement every day for three months after each workout. The other half takes the same amount of something that looks the same but actually does nothing to the body.
At the end of three months, the muscle mass of all participants is measured.
The independent variable is the supplement, and the dependent variable is muscle mass.
11. Air Bags and Skull Fractures
In the early days of airbags , automobile companies conducted a great deal of testing. At first, many people in the industry didn’t think airbags would be effective at all. Fortunately, there was a way to test this theory objectively.
In a representative example: Several crash cars were outfitted with an airbag, and an equal number were not. All crash cars were of the same make, year, and model. Then the crash experts rammed each car into a crash wall at the same speed. Sensors on the crash dummy skulls allowed for a scientific analysis of how much damage a human skull would incur.
The amount of skull damage of dummies in cars with airbags was then compared with those without airbags.
The independent variable was the airbag and the dependent variable was the amount of skull damage.
12. Vitamins and Health
Some people take vitamins every day. A group of health scientists decides to conduct a study to determine if taking vitamins improves health.
They randomly select 1,000 people that are relatively similar in terms of their physical health. The key word here is “similar.”
Because the scientists have an unlimited budget (and because this is a hypothetical example, all of the participants have the same meals delivered to their homes (breakfast, lunch, and dinner), every day for one year.
In addition, the scientists randomly assign half of the participants to take a set of vitamins, supplied by the researchers every day for 1 year. The other half do not take the vitamins.
At the end of one year, the health of all participants is assessed, using blood pressure and cholesterol level as the key measurements.
In this highly unrealistic study, the independent variable is vitamins, and the dependent variable is health, as measured by blood pressure and cholesterol levels.
13. Meditation and Stress
Does practicing meditation reduce stress? If you have ever wondered if this is true or not, then you are in luck because there is a way to know one way or the other.
All we have to do is find 90 people that are similar in age, stress levels, diet and exercise, and as many other factors as we can think of.
Next, we randomly assign each person to either practice meditation every day, three days a week, or not at all. After three months, we measure the stress levels of each person and compare the groups.
How should we measure stress? Well, there are a lot of ways. We could measure blood pressure, or the amount of the stress hormone cortisol in their blood, or by using a paper and pencil measure such as a questionnaire that asks them how much stress they feel.
In this study, the independent variable is meditation and the dependent variable is the amount of stress (however it is measured).
14. Video Games and Aggression
When video games started to become increasingly graphic, it was a huge concern in many countries in the world. Educators, social scientists, and parents were shocked at how graphic games were becoming.
Since then, there have been hundreds of studies conducted by psychologists and other researchers. A lot of those studies used an experimental design that involved males of various ages randomly assigned to play a graphic or non-graphic video game.
Afterward, their level of aggression was measured via a wide range of methods, including direct observations of their behavior, their actions when given the opportunity to be aggressive, or a variety of other measures.
So many studies have used so many different ways of measuring aggression.
In these experimental studies, the independent variable was graphic video games, and the dependent variable was observed level of aggression.
15. Vehicle Exhaust and Cognitive Performance
Car pollution is a concern for a lot of reasons. In addition to being bad for the environment, car exhaust may cause damage to the brain and impair cognitive performance.
One way to examine this possibility would be to conduct an animal study. The research would look something like this: laboratory rats would be raised in three different rooms that varied in the degree of car exhaust circulating in the room: no exhaust, little exhaust, or a lot of exhaust.
After a certain period of time, perhaps several months, the effects on cognitive performance could be measured.
One common way of assessing cognitive performance in laboratory rats is by measuring the amount of time it takes to run a maze successfully. It would also be possible to examine the physical effects of car exhaust on the brain by conducting an autopsy.
In this animal study, the independent variable would be car exhaust and the dependent variable would be amount of time to run a maze.
Read Next: Extraneous Variables Examples
The experiment is an incredibly valuable way to answer scientific questions regarding the cause and effect of certain variables. By manipulating the level of an independent variable and observing corresponding changes in a dependent variable, scientists can gain an understanding of many phenomena.
For example, scientists can learn if graphic video games make people more aggressive, if mediation reduces stress, if Gatorade improves athletic performance, and even if certain medical treatments can cure cancer.
The determination of causality is the key benefit of manipulating the independent variable and them observing changes in the dependent variable. Other research methodologies can reveal factors that are related to the dependent variable or associated with the dependent variable, but only when the independent variable is controlled by the researcher can causality be determined.
Ferguson, C. J. (2010). Blazing Angels or Resident Evil? Can graphic video games be a force for good? Review of General Psychology, 14 (2), 68-81. https://doi.org/10.1037/a0018941
Flannelly, L. T., Flannelly, K. J., & Jankowski, K. R. (2014). Independent, dependent, and other variables in healthcare and chaplaincy research. Journal of Health Care Chaplaincy , 20 (4), 161–170. https://doi.org/10.1080/08854726.2014.959374
Manocha, R., Black, D., Sarris, J., & Stough, C.(2011). A randomized, controlled trial of meditation for work stress, anxiety and depressed mood in full-time workers. Evidence-Based Complementary and Alternative Medicine , vol. 2011, Article ID 960583. https://doi.org/10.1155/2011/960583
Rumrill, P. D., Jr. (2004). Non-manipulation quantitative designs. Work (Reading, Mass.) , 22 (3), 255–260.
Taylor, J. M., & Rowe, B. J. (2012). The “Mozart Effect” and the mathematical connection, Journal of College Reading and Learning, 42 (2), 51-66. https://doi.org/10.1080/10790195.2012.10850354
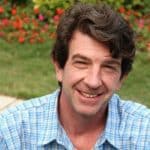
- Dave Cornell (PhD) https://helpfulprofessor.com/author/dave-cornell-phd/ 23 Achieved Status Examples
- Dave Cornell (PhD) https://helpfulprofessor.com/author/dave-cornell-phd/ 25 Defense Mechanisms Examples
- Dave Cornell (PhD) https://helpfulprofessor.com/author/dave-cornell-phd/ 15 Theory of Planned Behavior Examples
- Dave Cornell (PhD) https://helpfulprofessor.com/author/dave-cornell-phd/ 18 Adaptive Behavior Examples
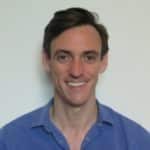
- Chris Drew (PhD) https://helpfulprofessor.com/author/chris-drew-phd/ 23 Achieved Status Examples
- Chris Drew (PhD) https://helpfulprofessor.com/author/chris-drew-phd/ 15 Ableism Examples
- Chris Drew (PhD) https://helpfulprofessor.com/author/chris-drew-phd/ 25 Defense Mechanisms Examples
- Chris Drew (PhD) https://helpfulprofessor.com/author/chris-drew-phd/ 15 Theory of Planned Behavior Examples
Leave a Comment Cancel Reply
Your email address will not be published. Required fields are marked *
Have a language expert improve your writing
Run a free plagiarism check in 10 minutes, generate accurate citations for free.
- Knowledge Base
Methodology
- Independent vs. Dependent Variables | Definition & Examples
Independent vs. Dependent Variables | Definition & Examples
Published on February 3, 2022 by Pritha Bhandari . Revised on June 22, 2023.
In research, variables are any characteristics that can take on different values, such as height, age, temperature, or test scores.
Researchers often manipulate or measure independent and dependent variables in studies to test cause-and-effect relationships.
- The independent variable is the cause. Its value is independent of other variables in your study.
- The dependent variable is the effect. Its value depends on changes in the independent variable.
Your independent variable is the temperature of the room. You vary the room temperature by making it cooler for half the participants, and warmer for the other half.
Table of contents
What is an independent variable, types of independent variables, what is a dependent variable, identifying independent vs. dependent variables, independent and dependent variables in research, visualizing independent and dependent variables, other interesting articles, frequently asked questions about independent and dependent variables.
An independent variable is the variable you manipulate or vary in an experimental study to explore its effects. It’s called “independent” because it’s not influenced by any other variables in the study.
Independent variables are also called:
- Explanatory variables (they explain an event or outcome)
- Predictor variables (they can be used to predict the value of a dependent variable)
- Right-hand-side variables (they appear on the right-hand side of a regression equation).
These terms are especially used in statistics , where you estimate the extent to which an independent variable change can explain or predict changes in the dependent variable.
Receive feedback on language, structure, and formatting
Professional editors proofread and edit your paper by focusing on:
- Academic style
- Vague sentences
- Style consistency
See an example
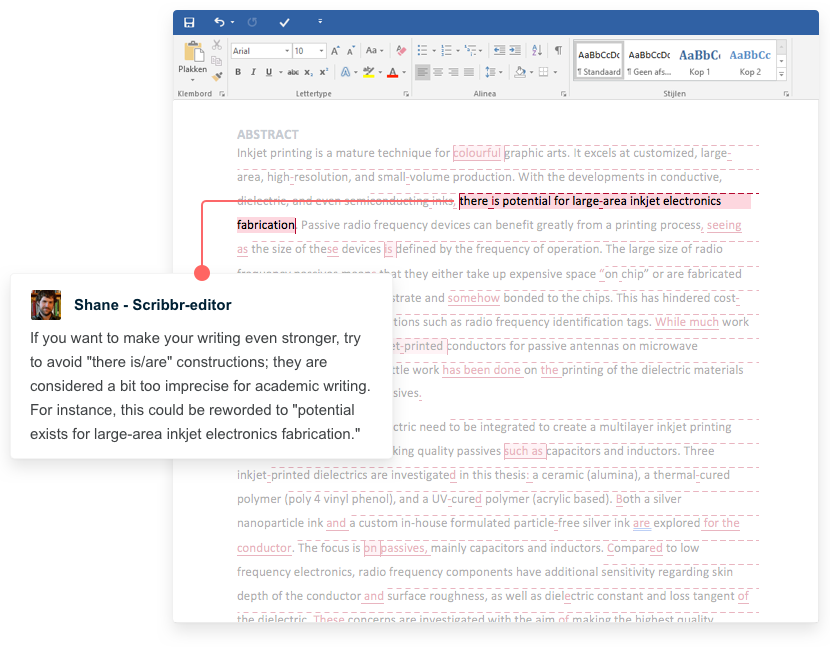
There are two main types of independent variables.
- Experimental independent variables can be directly manipulated by researchers.
- Subject variables cannot be manipulated by researchers, but they can be used to group research subjects categorically.
Experimental variables
In experiments, you manipulate independent variables directly to see how they affect your dependent variable. The independent variable is usually applied at different levels to see how the outcomes differ.
You can apply just two levels in order to find out if an independent variable has an effect at all.
You can also apply multiple levels to find out how the independent variable affects the dependent variable.
You have three independent variable levels, and each group gets a different level of treatment.
You randomly assign your patients to one of the three groups:
- A low-dose experimental group
- A high-dose experimental group
- A placebo group (to research a possible placebo effect )
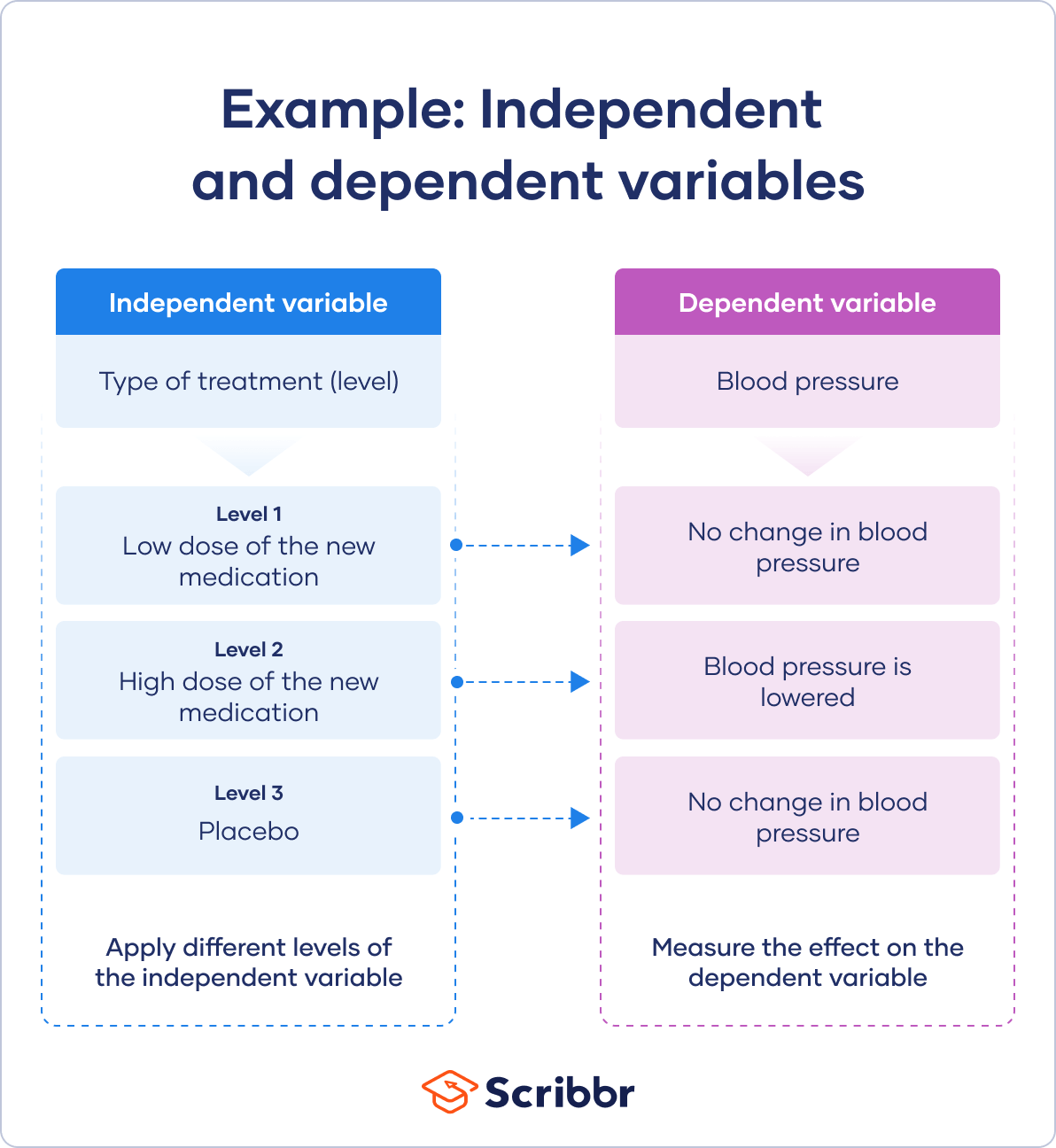
A true experiment requires you to randomly assign different levels of an independent variable to your participants.
Random assignment helps you control participant characteristics, so that they don’t affect your experimental results. This helps you to have confidence that your dependent variable results come solely from the independent variable manipulation.
Subject variables
Subject variables are characteristics that vary across participants, and they can’t be manipulated by researchers. For example, gender identity, ethnicity, race, income, and education are all important subject variables that social researchers treat as independent variables.
It’s not possible to randomly assign these to participants, since these are characteristics of already existing groups. Instead, you can create a research design where you compare the outcomes of groups of participants with characteristics. This is a quasi-experimental design because there’s no random assignment. Note that any research methods that use non-random assignment are at risk for research biases like selection bias and sampling bias .
Your independent variable is a subject variable, namely the gender identity of the participants. You have three groups: men, women and other.
Your dependent variable is the brain activity response to hearing infant cries. You record brain activity with fMRI scans when participants hear infant cries without their awareness.
A dependent variable is the variable that changes as a result of the independent variable manipulation. It’s the outcome you’re interested in measuring, and it “depends” on your independent variable.
In statistics , dependent variables are also called:
- Response variables (they respond to a change in another variable)
- Outcome variables (they represent the outcome you want to measure)
- Left-hand-side variables (they appear on the left-hand side of a regression equation)
The dependent variable is what you record after you’ve manipulated the independent variable. You use this measurement data to check whether and to what extent your independent variable influences the dependent variable by conducting statistical analyses.
Based on your findings, you can estimate the degree to which your independent variable variation drives changes in your dependent variable. You can also predict how much your dependent variable will change as a result of variation in the independent variable.
Distinguishing between independent and dependent variables can be tricky when designing a complex study or reading an academic research paper .
A dependent variable from one study can be the independent variable in another study, so it’s important to pay attention to research design .
Here are some tips for identifying each variable type.
Recognizing independent variables
Use this list of questions to check whether you’re dealing with an independent variable:
- Is the variable manipulated, controlled, or used as a subject grouping method by the researcher?
- Does this variable come before the other variable in time?
- Is the researcher trying to understand whether or how this variable affects another variable?
Recognizing dependent variables
Check whether you’re dealing with a dependent variable:
- Is this variable measured as an outcome of the study?
- Is this variable dependent on another variable in the study?
- Does this variable get measured only after other variables are altered?
Here's why students love Scribbr's proofreading services
Discover proofreading & editing
Independent and dependent variables are generally used in experimental and quasi-experimental research.
Here are some examples of research questions and corresponding independent and dependent variables.
Research question | Independent variable | Dependent variable(s) |
---|---|---|
Do tomatoes grow fastest under fluorescent, incandescent, or natural light? | ||
What is the effect of intermittent fasting on blood sugar levels? | ||
Is medical marijuana effective for pain reduction in people with chronic pain? | ||
To what extent does remote working increase job satisfaction? |
For experimental data, you analyze your results by generating descriptive statistics and visualizing your findings. Then, you select an appropriate statistical test to test your hypothesis .
The type of test is determined by:
- your variable types
- level of measurement
- number of independent variable levels.
You’ll often use t tests or ANOVAs to analyze your data and answer your research questions.
In quantitative research , it’s good practice to use charts or graphs to visualize the results of studies. Generally, the independent variable goes on the x -axis (horizontal) and the dependent variable on the y -axis (vertical).
The type of visualization you use depends on the variable types in your research questions:
- A bar chart is ideal when you have a categorical independent variable.
- A scatter plot or line graph is best when your independent and dependent variables are both quantitative.
To inspect your data, you place your independent variable of treatment level on the x -axis and the dependent variable of blood pressure on the y -axis.
You plot bars for each treatment group before and after the treatment to show the difference in blood pressure.
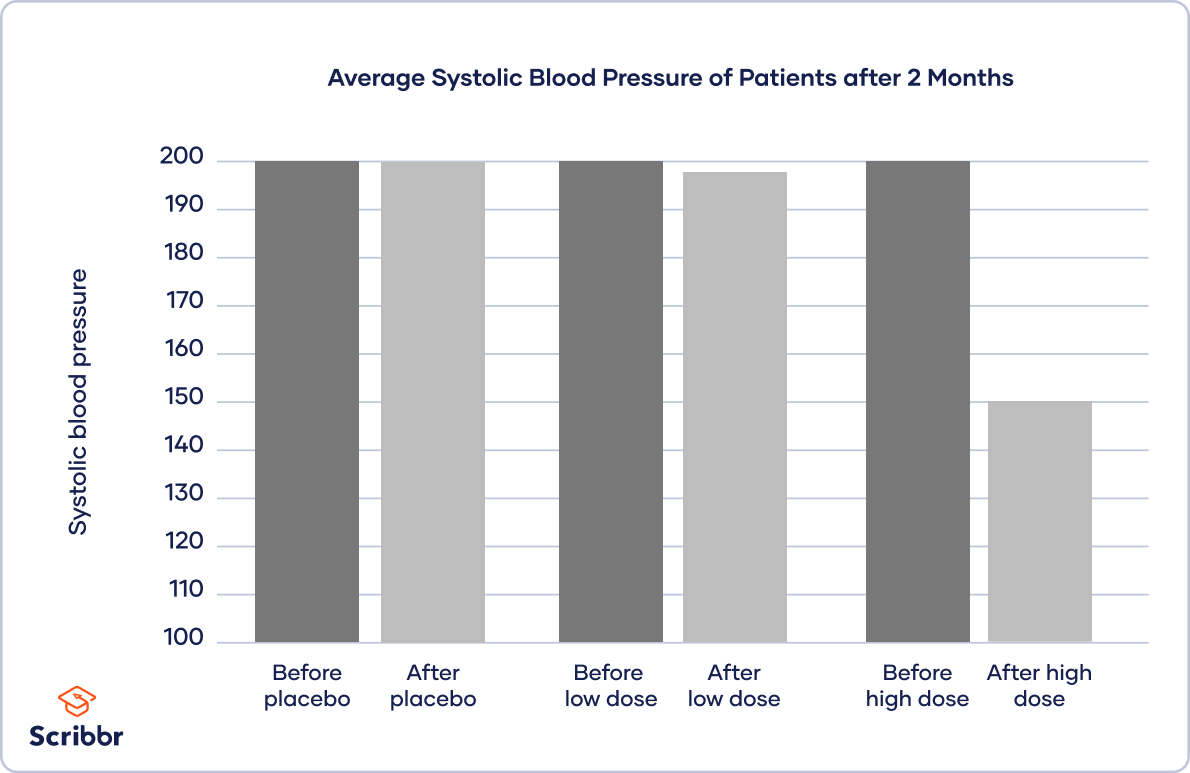
If you want to know more about statistics , methodology , or research bias , make sure to check out some of our other articles with explanations and examples.
- Normal distribution
- Degrees of freedom
- Null hypothesis
- Discourse analysis
- Control groups
- Mixed methods research
- Non-probability sampling
- Quantitative research
- Ecological validity
Research bias
- Rosenthal effect
- Implicit bias
- Cognitive bias
- Selection bias
- Negativity bias
- Status quo bias
An independent variable is the variable you manipulate, control, or vary in an experimental study to explore its effects. It’s called “independent” because it’s not influenced by any other variables in the study.
A dependent variable is what changes as a result of the independent variable manipulation in experiments . It’s what you’re interested in measuring, and it “depends” on your independent variable.
In statistics, dependent variables are also called:
Determining cause and effect is one of the most important parts of scientific research. It’s essential to know which is the cause – the independent variable – and which is the effect – the dependent variable.
You want to find out how blood sugar levels are affected by drinking diet soda and regular soda, so you conduct an experiment .
- The type of soda – diet or regular – is the independent variable .
- The level of blood sugar that you measure is the dependent variable – it changes depending on the type of soda.
No. The value of a dependent variable depends on an independent variable, so a variable cannot be both independent and dependent at the same time. It must be either the cause or the effect, not both!
Yes, but including more than one of either type requires multiple research questions .
For example, if you are interested in the effect of a diet on health, you can use multiple measures of health: blood sugar, blood pressure, weight, pulse, and many more. Each of these is its own dependent variable with its own research question.
You could also choose to look at the effect of exercise levels as well as diet, or even the additional effect of the two combined. Each of these is a separate independent variable .
To ensure the internal validity of an experiment , you should only change one independent variable at a time.
Cite this Scribbr article
If you want to cite this source, you can copy and paste the citation or click the “Cite this Scribbr article” button to automatically add the citation to our free Citation Generator.
Bhandari, P. (2023, June 22). Independent vs. Dependent Variables | Definition & Examples. Scribbr. Retrieved September 3, 2024, from https://www.scribbr.com/methodology/independent-and-dependent-variables/
Is this article helpful?
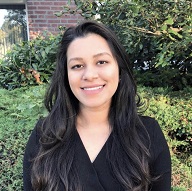
Pritha Bhandari
Other students also liked, guide to experimental design | overview, steps, & examples, explanatory and response variables | definitions & examples, confounding variables | definition, examples & controls, "i thought ai proofreading was useless but..".
I've been using Scribbr for years now and I know it's a service that won't disappoint. It does a good job spotting mistakes”
Want FREE Math Games?
Chloe Campbell Education
Resources for your classroom
Dependent & Independent Variables in Science Experiments
by Chloe Campbell Leave a Comment
Understanding how variables in science experiments work is an important skill for our students to understand. We do a lot of science experiments in my classroom, and knowing how different factors can change the outcome of a scientific experiment is always something I want them to be able to discover and explain. It’s also great practice for the scientific method. Here are some of the best ways to teach dependent and independent variables in your science classroom.
VARIABLES IN SCIENCE EXPERIMENTS: WHAT ARE THEY?
Here are definitions you can use with your students, using a plant growth experiment as a base:
- Example: If you are testing how different amounts of water affect plant growth, the amount of water is the independent variable because it’s what you change in your experiment.
- Example: In the plant experiment, the growth of the plant is the dependent variable because it’s what you measure to see how much the plant has grown based on the different amounts of water.
My Independent and Dependent Variables Resource has a foldable, interactive vocabulary activity that helps students understand the concept of variables. In the resource, students also define what control variables are.
It’s important for our students to know the variable that we are changing and the variables that occur because of that one change. It’s also so important to make sure the kids understand how important changing only one thing is. We need to know what caused the outcome of the experiment, and that’s difficult if we change different variables.

DESIGNING EXPERIMENTS
Once students understand what variables are, we need to help them put this new vocabulary into action. That’s where experiments come in! I like to start with a premade experiment that guide students through how variables work in a real-world context. An easy experiment that I like to use with my students is W hat Will Make Ice Melt the Fastest? . Students work with three different materials that we have on hand in class, and they predict which substance will make ice melt the fastest. I like to use sand, water, salt, sugar, or anything similar. I also make sure students know we need a control group to see what happens when no substance is applied to the ice.

FOCUS ON THE VARIABLES
Students can sometimes get lost in the steps of an experiment and forget what brought the results about. For this reason, I make sure that my students can communicate to each other what the variables were and, more importantly, why each variable exists. For example, in the plant growth experiment, the goal is for my students to be able to explain that:
- the independent variable is the amount of water we’re using, because we are changing the amount on purpose;
- the dependent variable is the plant’s growth, because that will change based on the water we give it;
- the controlled variables are anything we don’t intend to change, which in this case could be the type of soil used, the type of plant used, the amount of light each plant gets, the type of liquid (we always use the same tap water), and so on.
To keep the focus even stronger, the students know that their exit ticket for the class will be for them to explain what an independent, dependent, and controlled variable is. You can have students define in it general, or you can have them provide examples based on the results of the experiment.
ANALYZE THE DATA
Once my students have correctly identified the different types of variables in an experiment, we analyze the data we collected. I want them to understand, and then be able to explain to someone else, how the independent variable affects the dependent variable. For example, in my What Will Make Ice Melt the Fastest? lab, students conclude that the salt melted the ice fastest. The constant variables were anything we didn’t change, such as how long we timed them melting and the temperature of the room. The final outcome of an experiment is important, and knowing the why behind the outcome is important too.

Understanding these variables helps students design good experiments and understand the results better when they go off and create their own scientific investigations. When our students know what we are changing (independent variable) and what we are measuring (dependent variable), they can make better observations and conclusions. Being able to analyze the results of an experiment is a great critical thinking developer, and students pick up scientific inquiry skills they can use throughout the year.
You can grab this entire lesson with everything made for you by clicking the picture below.

Save this post for later by clicking on the picture below.

Leave a Reply Cancel reply
Your email address will not be published. Required fields are marked *
Notify me of follow-up comments by email.
Notify me of new posts by email.
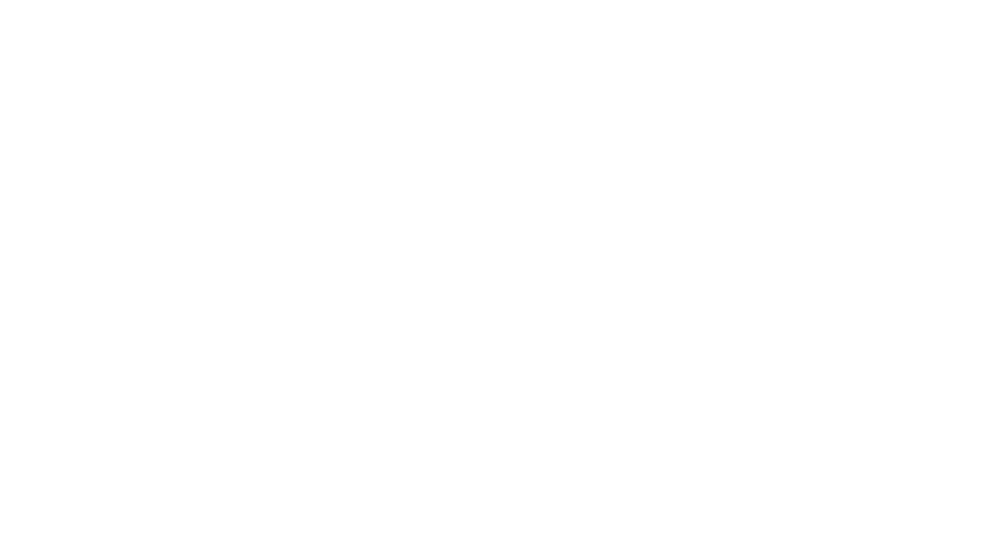
Get Your ALL ACCESS Shop Pass here →
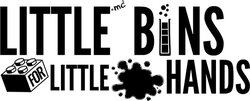
What Are Variables In Science
Whether setting up a science experiment for a science project, or learning more about the scientific method , variables in science are important. Find out what variables mean, what are three types of variables you need to know, plus examples of independent and dependent variables in experiments. Enjoy hands-on and easy science experiments for kids today!
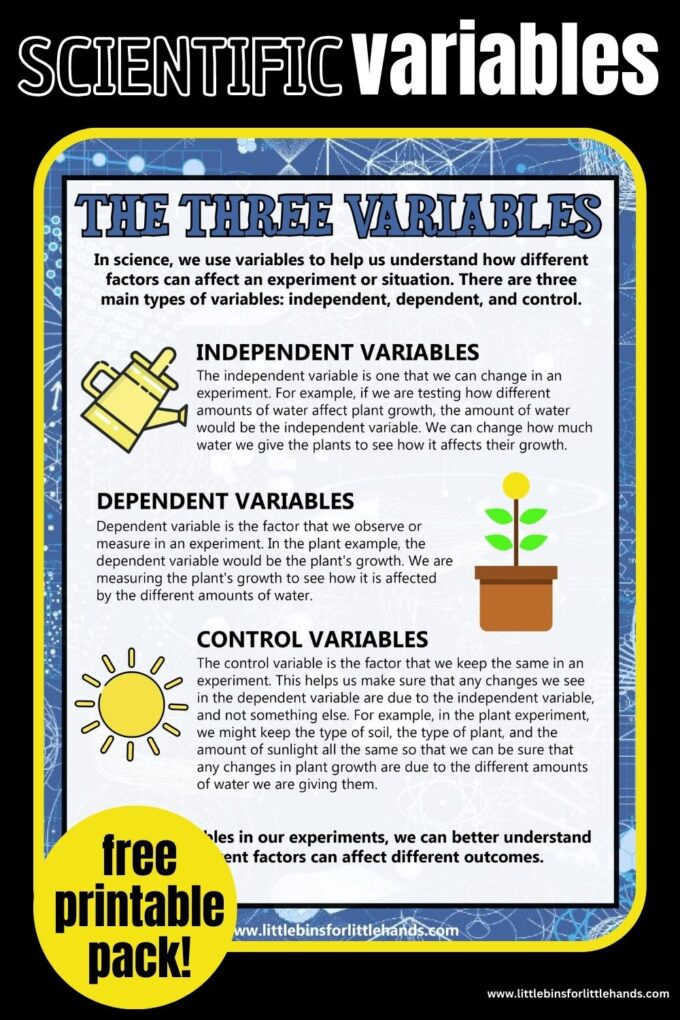
What Are Scientific Variables?
In science, variables are any factor that can be changed in the experiment to help us understand how different factors can affect an experiment or situation.
Specifically, three different variables help us answer the question we are investigating. Identifying these variables before you start will guide your decisions about how to conduct your experiment and how to measure the results.
Three Types of Variables
The three main types of variables are independent variable, dependent variable, and controlled variables.
Independent Variable
The independent variable in a science experiment is the factor that you will change. The independent variable affects the dependent variable.
You can identify the independent variable by looking at what can exist in differing amounts or types, and what is directly related to the question of your experiment.
For example, if you are testing how different amounts of water affect plant growth, the amount of water would be the independent variable. You can change how much water you give the plants to see how it affects their growth.
Remember, choose only one independent variable for your experiment!
Dependent Variable
The dependent variable is the factor that you observe or measure in an experiment. It is the variable that is affected by changes made to the independent variable.
In the plant example, the dependent variable would be the plant’s growth. We are measuring the plant’s growth to see how it is affected by the different amounts of water.
Controlled Variables
Control variables are the factors that you keep the same in the science experiment. This helps you make sure that any changes you see in the dependent variable are due to the independent variable and not something else.
With some experiments, you may choose to set up a control that has no amount of the independent variable added to it. All other factors are the same. This is great for comparison.
For example, in the plant experiment, you would keep the type of soil, the type of plant, and the amount of sunlight all the same so that you can be sure that any changes in plant growth are only due to the different amounts of water you are giving them. You could also have one plant that you give no water.
Grab the FREE printable variables sheet!
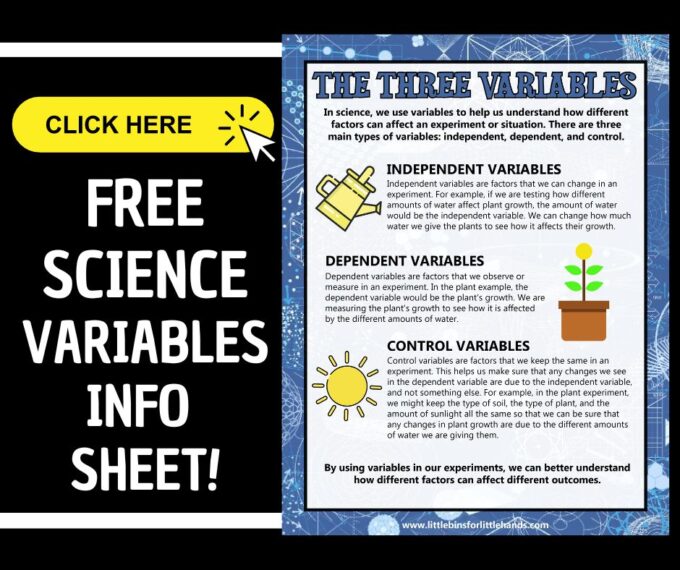
Easy Science Experiments With Variables
Here are a few examples of independent and dependent variables in science experiments . All of these experiments are very easy to do, and use simple supplies! Of course, you could change out the variables in these examples by asking a different question.
Apple Browning Experiment
Investigate what stops cut apples from turning brown. Does lemon juice work the best or something else? The independent variable is the type of substance you apply to the apples to stop or slow down browning. The dependent variable is the amount of browning on each apple slice.
Balloon Experiment
Kids love this easy science experiment. Blow up a balloon with a vinegar and baking soda chemical reaction. Find out what amount of baking soda makes for the biggest balloon. The independent variable is the amount of baking soda added to the vinegar, and the dependent variable is the size of the balloon.
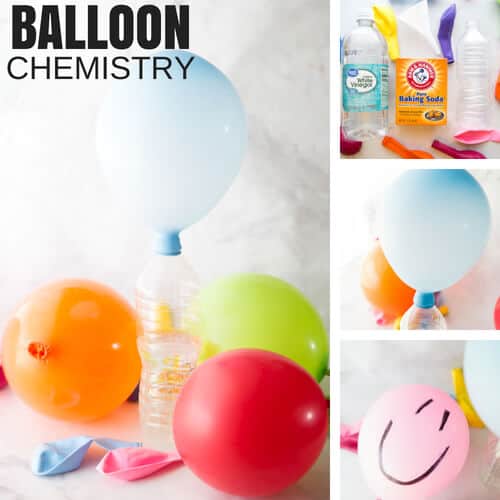
Celery and Food Coloring
Demonstrate how water travels through plants via capillary action with cut celery. The independent variable could be the length of time the celery is immersed in the water. The dependent variable is how far the food coloring has traveled throughout the celery.
Color Taste Test
Does the color of food or drink affect how it tastes? Explore whether the color of a drink affects how it is perceived in terms of taste. The independent variable would be the color of the drink and your dependent variable the perceived taste.
Floating Orange
A fun and easy experiment that explores the density of peeled and unpeeled oranges in water. The independent variable could be the type of orange you use. The dependent variable is how much of the orange floats in water.
Grow Crystals
Turn your crystal growing activity into an experiment that explores how temperature of the solution affects the size of the crystals. The independent variable is the temperature and the dependent variable is the size of the crystals.
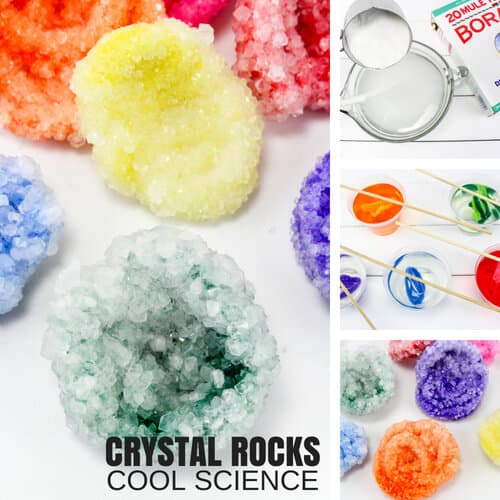
Gummy Bear Experiment
A dissolving candy experiment is fun to do! Here we used gummy bears to explore what liquid they dissolve the fastest in. You could also do this with candy hearts , candy corn , candy fish , or candy canes for fun variations.
The independent variable is the type of liquid you use to dissolve your gummy bears. You could use water, salt water, vinegar, oil or other household liquids. The dependent variable is the time it takes to dissolve the candy.
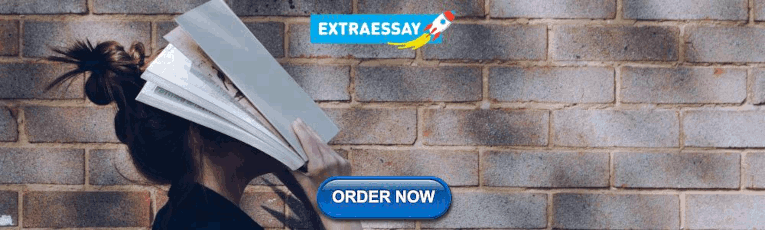
Ice Melting Experiment
Explore what makes ice melt faster. The independent variable is the type of substance added to the ice. You could try salt, sand and sugar. The dependent variable is the time it takes to melt the ice.
Magic Milk Experiment
Kids will love this color changing milk experiment with food coloring and dish soap. Investigate what happens when you use different types of milk. The independent variable is the type of milk and the dependent variable is how the food coloring moves through the milk.
Popsicle Stick Catapult
This is a fun physics activity especially for kids who love tinkering and building stuff, and you can turn it into a science experiment. Investigate how far an object travels as it weighs more.
The independent variable is the type of object you use on your catapult (vary by weight). The dependent variable is the distance it travels. This is a good experiment for repeating several times so you can average the results.
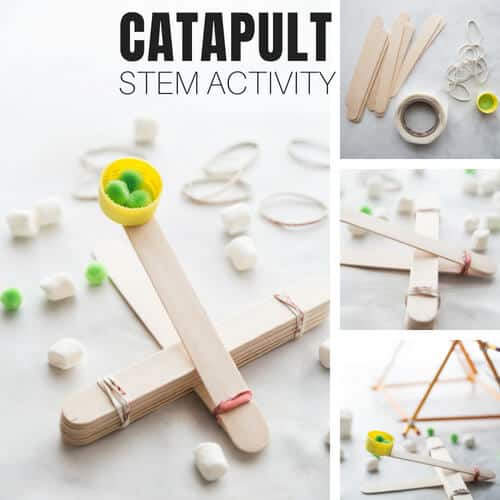
Salt Water Density Experiment
Explore the density of salt water vs fresh water with this simple science experiment. What happens to an egg in salt water? Will the egg float or sink? The independent variable is the amount of salt added to fresh water. The dependent variable is the distance of the egg from the bottom of the glass.
Seed Germination Experiment
Turn this seed germination jar into an easy science experiment by exploring what happens to seed growth when you change the amount of water used. The independent variable is the amount of the water used for each seed jar. The dependent variable is the length of the seedling over a period of time.

Helpful Science Resources
Here are a few resources that will help you introduce science more effectively to your kiddos or students and feel confident when presenting materials. You’ll find helpful free printables throughout.
Science Vocabulary: Introducing some fantastic science words to kids is never too early. Get them started with a printable science vocabulary word list .
What Is A Scientist? Learn about the different types of scientists and what they do. Read What Is A Scientist
Science Books: Sometimes, the best way to introduce science concepts is through a colorfully illustrated book with characters your kids can relate to! Check out this fantastic list of science books .
Science Practices: These eight science and engineering practices are less structured and allow for a more free – flowing approach to problem-solving and finding answers to questions.
Fun Science Experiments To Try: Don’t just read about science, go ahead and enjoy one of these fantastic kids science experiments !
Science Experiments By Age Group
We’ve put together a few separate resources for different age groups, but remember that many experiments will cross over and can be re-tried at several different age levels. Younger kiddos can enjoy the simplicity and hands-on fun. At the same time, you can talk back and forth about what is happening.
- Science for Toddlers
- Science for Preschoolers
- Science for Kindergarten
- Science for Early Elementary Grades
- Science for 3rd Grade
- Science for Middle School
Printable Science Projects For Kids
If you’re looking to grab all of our printable science projects in one convenient place plus exclusive worksheets and bonuses like a STEAM Project pack, our Science Project Pack is what you need! Over 300+ Pages!
- 90+ classic science activities with journal pages, supply lists, set up and process, and science information. NEW! Activity-specific observation pages!
- Best science practices posters and our original science method process folders for extra alternatives!
- Be a Collector activities pack introduces kids to the world of making collections through the eyes of a scientist. What will they collect first?
- Know the Words Science vocabulary pack includes flashcards, crosswords, and word searches that illuminate keywords in the experiments!
- My science journal writing prompts explore what it means to be a scientist!!
- Bonus STEAM Project Pack: Art meets science with doable projects!
- Bonus Quick Grab Packs for Biology, Earth Science, Chemistry, and Physics
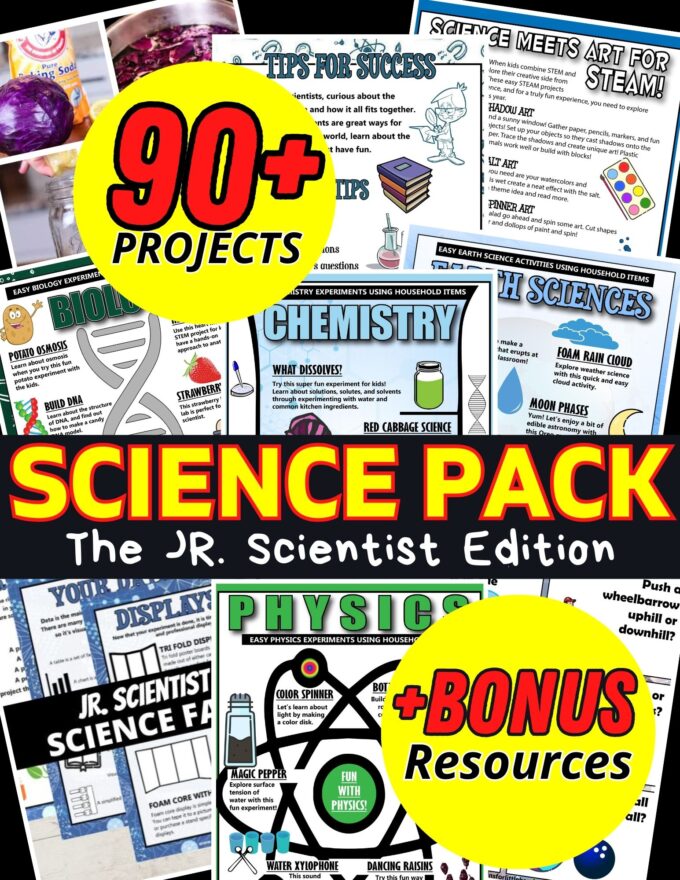
Subscribe to receive a free 5-Day STEM Challenge Guide
~ projects to try now ~.
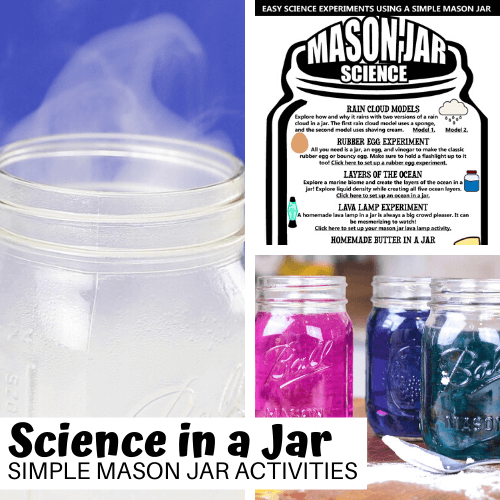
Independent Variable Definition and Examples
Understand the Independent Variable in an Experiment
- Chemical Laws
- Periodic Table
- Projects & Experiments
- Scientific Method
- Biochemistry
- Physical Chemistry
- Medical Chemistry
- Chemistry In Everyday Life
- Famous Chemists
- Activities for Kids
- Abbreviations & Acronyms
- Weather & Climate
- Ph.D., Biomedical Sciences, University of Tennessee at Knoxville
- B.A., Physics and Mathematics, Hastings College
The independent variable and the dependent variable are the two main variables in a science experiment. Below is the definition of an independent variable and a look at how you might use it.
Key Takeaways: Independent Variable
- The independent variable is the factor that you purposely change or control to see what effect it has.
- The variable that responds to the change in the independent variable is called the dependent variable. The dependent variable depends on the independent variable.
- The independent variable is graphed on the x-axis.
Independent Variable Definition
An independent variable is defined as a variable that is changed or controlled in a scientific experiment. The independent variable represents the cause or reason for an outcome. Independent variables are the variables that the experimenter changes to test his or her dependent variable . A change in the independent variable directly causes a change in the dependent variable. The effect on the dependent variable is measured and recorded.
Common misspellings: independant variable
Independent Variable Examples
Here are some examples of an independent variable.
- A scientist is testing the effect of light and dark on the behavior of moths by turning a light on and off. The independent variable is the amount of light (cause) and the moth's reaction is the dependent variable (the effect).
- In a study to determine the effect of temperature on plant pigmentation , the independent variable is the temperature, while the amount of pigment or color is the dependent variable.
Graphing the Independent Variable
When graphing data for an experiment, the independent variable is plotted on the x-axis, while the dependent variable is recorded on the y-axis. An easy way to keep the two variables straight is to use the acronym DRY MIX , which stands for:
- Dependent variable that Responds to change goes on the Y axis
- Manipulated or Independent variable goes on the X axis
Practice Identifying the Independent Variable
Students are often asked to identify the independent and dependent variable in an experiment. The difficulty is that the value of both of these variables can change. It is even possible for the dependent variable to remain unchanged in response to controlling the independent variable.
Example : You are asked to identify the independent and dependent variable in an experiment to see if there is a relationship between hours of sleep and student test scores.
There are two ways to identify the independent variable. The first is to write the hypothesis and see if it makes sense.
For example:
- Student test scores do not affect the number of hours the students sleep.
- The number of hours students sleep do not affect their test scores.
Only one of these statements makes sense. This type of hypothesis is constructed to state the independent variable followed by the predicted impact on the dependent variable. So, the number of hours of sleep is the independent variable.
The other way to identify the independent variable is more intuitive. Remember, the independent variable is the one the experimenter controls to measure its effect on the dependent variable. A researcher can control the number of hours a student sleeps. On the other hand, the scientist has no control over the students' test scores.
The independent variable always changes in an experiment, even if there is just a control and an experimental group. The dependent variable may or may not change in response to the independent variable. In the example regarding sleep and student test scores, the data might show no change in test scores, no matter how much sleep students get (although this outcome seems unlikely). The point is that a researcher knows the values of the independent variable. The value of the dependent variable is measured .
- Babbie, Earl R. (2009). The Practice of Social Research (12th ed.). Wadsworth Publishing. ISBN 0-495-59841-0.
- Dodge, Y. (2003). The Oxford Dictionary of Statistical Terms . OUP. ISBN 0-19-920613-9.
- Everitt, B. S. (2002). The Cambridge Dictionary of Statistics (2nd ed.). Cambridge UP. ISBN 0-521-81099-X.
- Gujarati, Damodar N.; Porter, Dawn C. (2009). "Terminology and Notation". Basic Econometrics (5th international ed.). New York: McGraw-Hill. p. 21. ISBN 978-007-127625-2.
- Shadish, William R.; Cook, Thomas D.; Campbell, Donald T. (2002). Experimental and quasi-experimental designs for generalized causal inference . (Nachdr. ed.). Boston: Houghton Mifflin. ISBN 0-395-61556-9.
- Oxidation Definition and Example in Chemistry
- Dependent Variable vs. Independent Variable: What Is the Difference?
- Heavy Metal Definition and List
- Melting Definition in Chemistry
- Stoichiometry Definition in Chemistry
- The Combined Gas Law in Chemistry
- pKa Definition in Chemistry
- Element Symbol Definition in Chemistry
- What Is an Element in Chemistry? Definition and Examples
- Absolute Temperature Definition
- 4 Types and Examples of Chemical Weathering
- Ionization Energy Definition and Trend
- The Chemical Composition of Air
- Why Is the Carbon Cycle Important?
- Definition of Air in Science
- Null Hypothesis Definition and Examples

- Science Notes Posts
- Contact Science Notes
- Todd Helmenstine Biography
- Anne Helmenstine Biography
- Free Printable Periodic Tables (PDF and PNG)
- Periodic Table Wallpapers
- Interactive Periodic Table
- Periodic Table Posters
- Science Experiments for Kids
- How to Grow Crystals
- Chemistry Projects
- Fire and Flames Projects
- Holiday Science
- Chemistry Problems With Answers
- Physics Problems
- Unit Conversion Example Problems
- Chemistry Worksheets
- Biology Worksheets
- Periodic Table Worksheets
- Physical Science Worksheets
- Science Lab Worksheets
- My Amazon Books
What Is an Independent Variable? Definition and Examples

The independent variable is the variable that is controlled or changed in a scientific experiment to test its effect on the dependent variable . It doesn’t depend on another variable and isn’t changed by any factors an experimenter is trying to measure. The independent variable is denoted by the letter x in an experiment or graph.
INDEPENDENT VARIABLE EXAMPLE
Two classic examples of independent variables are age and time. They may be measured, but not controlled. In experiments, even if measured time isn’t the variable, it may relate to duration or intensity.
For example, a scientist is testing the effect of light and dark on the behavior of moths by turning a light on and off. The independent variable is the amount of light and the moth’s reaction is the dependent variable.
For another example, say you are measuring whether amount of sleep affects test scores. The hours of sleep would be the independent variable while the test scores would be dependent variable.
A change in the independent variable directly causes a change in the dependent variable. If you have a hypothesis written such that you’re looking at whether x affects y , the x is always the independent variable and the y is the dependent variable.
GRAPHING THE INDEPENDENT VARIABLE
If the dependent and independent variables are plotted on a graph, the x-axis would be the independent variable and the y-axis would be the dependent variable. You can remember this using the DRY MIX acronym, where DRY means dependent or responsive variable is on the y-axis, while MIX means the manipulated or independent variable is on the x-axis.
Related Posts
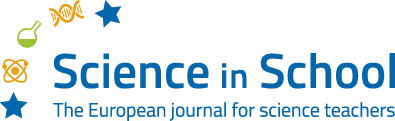
Science in School
Simple gravimetric chemical analysis – weighing molecules the microscale way teach article.
Author(s): Bob Worley and Adrian Allan
Learn how to do quantitative chemistry using microscale techniques with bottle tops and inexpensive spirit burners that are relatively easy and quick to set up.
Quantitative chemistry using gravimetric analysis gives students the opportunity to experience chemical reactions, observe chemical changes, and use measurements of masses to determine the formula of a compound. This can be done using a combustion reaction, which results in a gain of mass (such as the reaction of magnesium with oxygen), or removing the water from a hydrated salt by heating, which results in a loss of mass. [ 1 ] These microscale practical activities are relatively simple and quick to do and can help students focus on the chemistry and reduce the load on working memory. Despite the small masses involved, the data generated from microscale experiments shows equivalent or better results than those obtained with traditional equipment, although a comparison of techniques is a useful exercise in error analysis. The advent of inexpensive, robust digital balances, measuring accurately to 0.01 g, has also allowed these methods to be more accessible and affordable than before.
Activity 1: Determining the formula of magnesium oxide
The determination of the formula of magnesium oxide by combustion of magnesium can yield variable results. Porcelain crucibles can be costly and can break during the experiment, and magnesium can escape when the lid is lifted. This product loss can reduce the accuracy of the result.
The microscale method uses an inexpensive alternative to expensive crucibles. The natural design of bottle tops allows a good flow of air with minimal loss of product.
This activity will take about 30 minutes and suitable for students aged 14–18.
- Magnesium ribbon, about 10–15 cm long (danger: flammable)
- Bunsen burner
- Two crown bottle tops (make sure the plastic coating has been removed from the bottle tops; this is easily done with a Bunsen burner and pair of tongs in a working fume cupboard)
- Mass balance
- Eye protection
- Nichrome wire, about 15 cm
- Small pipe-clay triangle
- Heating mat
- Activity 1 worksheet
- Find the total mass of two bottle tops and 15 cm of nichrome wire (M1) on a balance. Record the mass on the student worksheet.
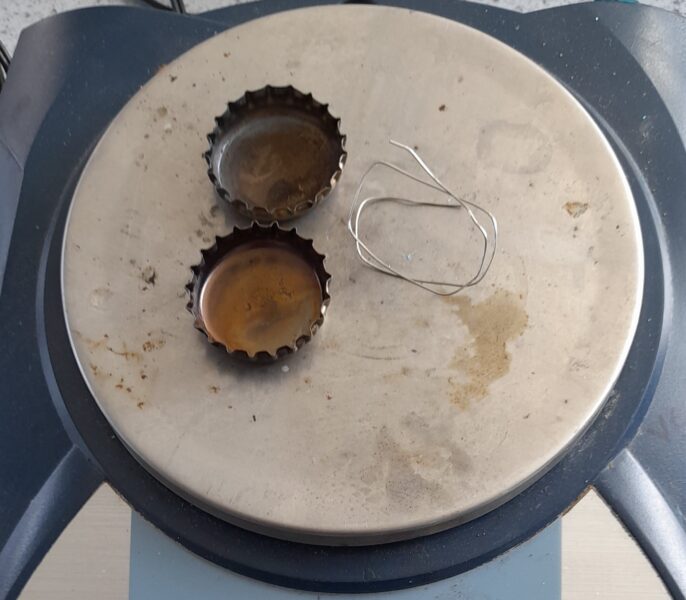
- Roll a 10–15 cm length of magnesium ribbon around a pencil and place the ribbon on one of the bottle tops.
- Find the mass of the two bottle tops, nichrome wire, and magnesium ribbon (M2) and record on the worksheet.
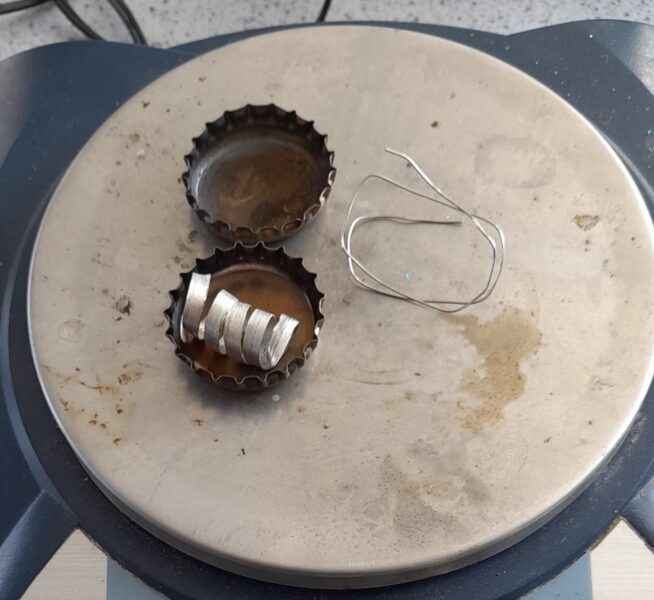
- Set up a Bunsen burner and tripod on a heatproof mat. On the tripod, place a pipe-clay triangle small enough to support the bottle top ‘parcel’.
- Sandwich the magnesium between the two bottle tops (serrated edges together). Wrap the wire round the bottle tops to keep them together.
- Place the bottle tops securely on the pipe clay.
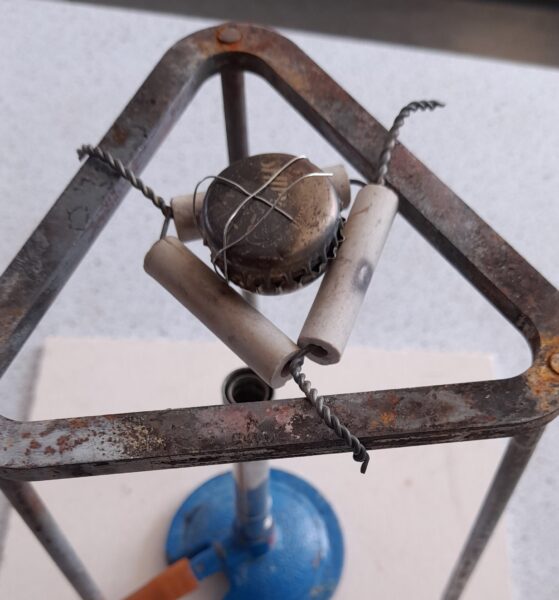
- Heat the bottle tops with a strong blue flame for 10 minutes.
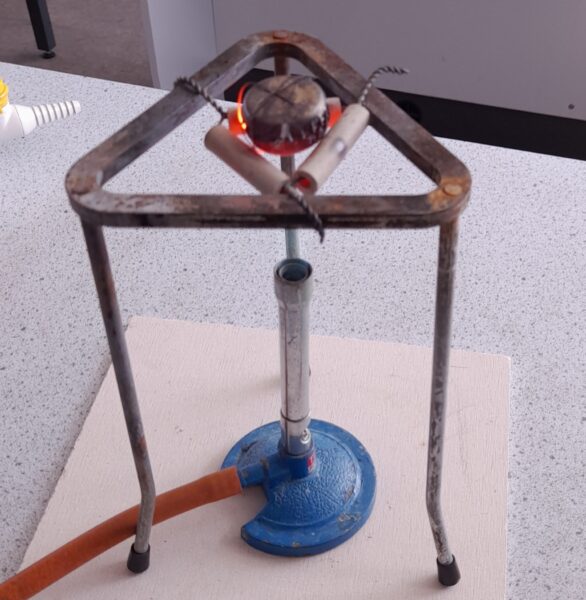
- Switch off the Bunsen burner and allow the bottle tops to cool (for about 5 minutes).
- Find the mass of the bottle tops plus nichrome wire and magnesium oxide. Record this mass as M3.
- Use the masses of magnesium and magnesium oxide to calculate the number of moles of each substance. The molar ratio can be used to determine the formula of the compound.
Results and discussion
This activity can also be used with younger students who have not yet been taught mole calculations as way of introducing conservation of mass. Ask them to predict whether the mass of magnesium will get lighter, stay the same, or get heavier when heated, and test their prediction. Some will think the magnesium will get lighter, as they assume it will be ‘burnt away’ like carbon when it reacts with oxygen to form carbon dioxide. They are often surprised that oxygen atoms have mass, which can be measured on a balance after a combustion reaction with a metal.
A full explanation of the calculations can be found in the supporting material.
Sample result and calculation:
M1 = mass of bottle tops plus nichrome wire
M2 = mass of magnesium plus nichrome wire and magnesium
M3 = mass of the bottle top plus nichrome wire and magnesium oxide
Mass of magnesium ribbon used (M2 − M1 = 4.11 − 3.87)
Moles of magnesium = mass of Mg/gram formula mass of Mg = 0.24 ⁄ 24.5
Mass of oxygen used = M3 − M2 = 4.26 − 4.11
Moles of oxygen = mass of O/gram formula mass of O = 0.15 ⁄ 16
Ratio of magnesium to oxygen = moles Mg/moles O = 0.0098 ⁄ 0.0094
The value should be close to one, giving a molar ratio of approximately one magnesium to one oxygen, which suggests the formula of magnesium oxide is indeed MgO.
Activity 2: Gravimetric determination of the formula of hydrated copper(II) sulfate
Gravimetric analysis to determine the moles of water present in a hydrated complex usually requires preweighing of a sample and heating to constant mass over a Bunsen burner using a crucible and a desiccator to prevent water from being reabsorbed from the air.
This method is quicker and uses a bottle top instead of a crucible, as described in Activity 1, along with spirit burners.
Spirit burners
Spirit burners burn cooler than Bunsen flames, which is advantageous for some experiments. A cheaper alternative to buying them from laboratory suppliers is to construct a homemade version made from small-scale jam jars.
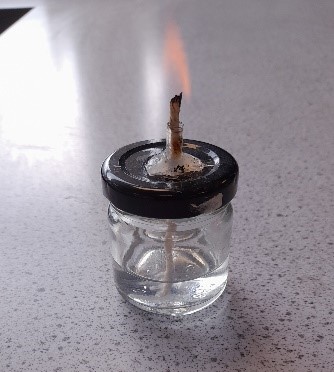
A full guide on how to make a spirit burner is available in the supporting material. The assembly process can be observed in this video: https://www.youtube.com/watch?v=ndlycDnCM8c
The spirit burners can be used for other microscale practical applications, such as flame tests and determining the melting points of covalent molecular and ionic substances, [ 2 ] as well as for the cracking of hydrocarbons. [ 3 ]
In this experiment, the use of a spirit burner limits the extent to which copper sulfate will decompose to release toxic sulfur dioxide:
CuSO 4 ·5H 2 O(s) (pale-blue solid) ⇌ CuSO 4 (s) (white solid) + 5H 2 O(s)
Copper(II) sulfate pentahydrate (CuSO 4 ·5H 2 O) loses four of its water molecules at about 100 °C. The final water molecule is lost at 150 °C. With a Bunsen flame, a temperature of over 650 °C is reached, which causes the hydrated copper sulfate to decompose; the solid darkens and toxic sulfur dioxide and trioxide gases are released. As well as being hazardous, the decomposition affects the accuracy of the results. Using a cooler flame produced by a spirit burner prevents this decomposition.
Safety note
Wear eye protection.
- Bottle tops with plastic removed, as described in Activity 1
- Spirit burner (details of how to make one are given in the supporting material)
- Copper(II) sulfate pentahydrate (CuSO 4 ·5H 2 O)
- Spirit burner
- Optional: a muselet (this is a wire cage from a bottle of champagne or other sparkling wine) to act as a microscale tripod
- Activity 2 worksheet
- Put the bottle-top crucible on the balance and set it to 0.0 g using the tare button.
- Add about 1.2 g of hydrated copper sulfate to the crucible. Record the mass on the student worksheet.
- Optional: place the muselet on the spirit burner. This will act as a microscale tripod for the bottle top.
- Place the bottle-top crucible on the muselet tripod and heat with an ignited spirit burner until the blue colour has been lost and white/colourless solid remains. Alternatively, hold the bottle top with tongs and heat as above.
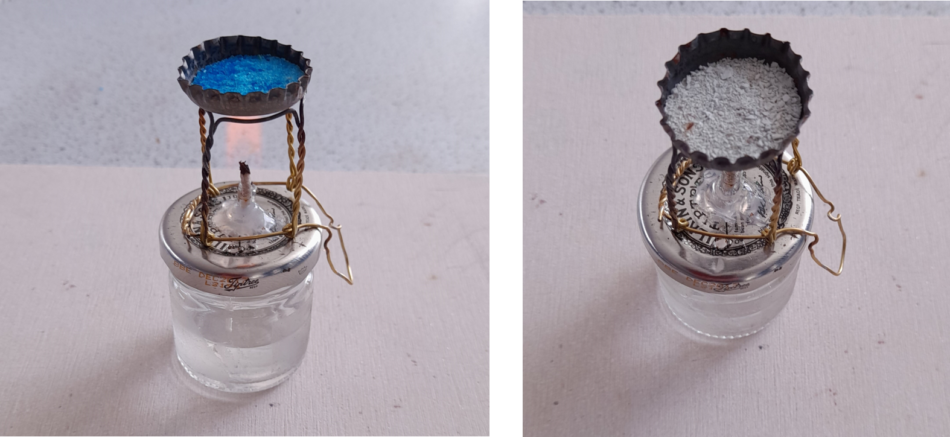
- Remove the crucible from the spirit burner (and extinguish the flame).
- Allow the crucible to cool.
- Find the mass of the crucible plus anhydrous salt and record this.
- Use the masses of hydrated and anhydrous copper sulfate to calculate the number of moles of copper sulfate and water present in the hydrated complex. The molar ratio can be used to determine the formula of hydrated copper(II) sulfate.
Mass of hydrated copper(II) sulfate used =
Mass of anhydrous copper(II) sulfate after heating =
Mass of water removed by heating = 1.20 − 0.78 =
Number of moles of copper sulfate (CuSO 4 ) left after water was removed = 0.78 ⁄ 159.6 =
Number of moles of water removed by heating = 0.42 ⁄ 18 =
Ratio of moles of water to copper sulfate = 0.023 ⁄ 0.0049 = 4.9, which is 5 when rounded to nearest whole number.
The students can be shown the label of a bottle of hydrated copper sulfate and compare their result with the label to verify their result. The value should be close to five, giving a molar ratio of approximately five moles of water to one mole of copper(II) sulfate, which suggests the formula of the hydrated copper(II) sulfate is CuSO 4 ·5H 2 O.
Adrian and Bob have now reached the end of this series of articles on microscale chemistry. What started out as an antidote to the safety concerns of dealing with chemicals in schools, (storage, use, disposal) by education managers and the UK Health and Safety executive, in around 1993, has now attracted more enthusiasts because of the educational and economic benefits the techniques bring. Now we can add the promotion of sustainability, as directed by the United Nations, using the principles of green chemistry, [ 4 ] as formulated in 1998 by Paul Anastas and John C. Warner. At least 6 of the 12 principles of green chemistry can apply to school-taught chemistry. [ 5 ]
- Prevention of waste : droplets of solutions added to a plastic surface using transfer pipettes are just wiped away with a paper towel.
- Less-hazardous chemical syntheses : preparing copper sulfate crystals while avoiding scalds, burns, and the evolution of toxic gases; microelectrolysis of copper chloride solution.
- Safer solvents and auxiliaries : using water as the main solvent, as well as adopting salting-out procedures.
- Design for energy efficiency : spirit burners and hot water from a kettle can be used to avoid the use of fossil fuels (e.g., the Bunsen burner); using more energy efficient LEDs.
- Catalysis : using yeast to produce oxygen from hydrogen peroxide.
- Inherently safer chemistry for accident prevention :reducing concentrations, finding an alternative procedure to carry out the electrolysis of a molten lead bromide, and conducting small-scale catalytic cracking to avoid suck back.
This last principle is what CLEAPSS and SSERC in the UK have been doing since 1963.
We are often accused of removing the ‘wow’ moments that school chemistry brings. With the microchemistry approach, there are still explosions (dynamite soap bubbles), and there are more wow moments, such as the beauty of an array of colours in droplet art. [ 6 ] There are completely new demonstrations. Bob recently carried out a demonstration showing the electrical conductivity of molten sodium chloride , an observation that is quoted in many school texts as evidence of ionic bonding, but never easily demonstrated until now, by using microscale techniques [ 7 ] and the bottle-top crucible described in this article.
Acknowledgements
We would like to thank and acknowledge Howard Tolliday at Dornoch Academy, UK, for his advice and assistance in developing the equipment and his help in collecting the images and video accompanying this article.
[1] Worley B, Paterson D (2021) Understanding Chemistry through Microscale Practical Work pp 38-41. Association for Science Education. ISBN: 978-0863574788
[2] The Science on Stage webinar on microscale chemistry: https://youtu.be/LM97yXJlotQ?si=e_IGnqLuTiJdPV84
[3] The Royal Society of Chemistry resource to teach the cracking of long-chain hydrocarbons: https://edu.rsc.org/exhibition-chemistry/cracking/4010515.article
[4] The 12 principles of green chemistry: https://www.compoundchem.com/2015/09/24/green-chemistry/
[5] Green chemistry principles applicable in school chemistry: https://microchemuk.weebly.com/green-chemistry.html
[6] An article on chemical droplet art: https://uwaterloo.ca/chem13-news-magazine/september-2019/feature/indicator-droplet-art
[7] A video on the electrolysis of molten sodium chloride: https://www.youtube.com/watch?v=wKgDJYY6Vkk&t=60s
- Watch a webinar on microscale-chemistry techniques.
- Read about the 12 principles of green chemistry .
- Read an introduction to microscale chemistry in the classroom: Worley B (2021) Little wonder: microscale chemistry in the classroom . Science in School 53 .
- Discover simple adaptations of experiments to make chemistry accessible to students with vision impairment: Chataway-Green R, Schnepp Z (2023) Making chemistry accessible for students with vision impairment . Science in School 64 .
- Enhance your students’ understanding of electrolysis using microscale chemistry techniques: Worley B, Allan A (2022) Elegant electrolysis – the microscale way . Science in School 60 .
- Use microscale techniques to do quantitative chemistry experiments: Worley B, Allan A (2023) Quick quantitative chemistry – the microscale way . Science in School 63 .
- Teach the chemistry of precipitation using microscale-chemistry methods: Worley B, Allan A (2022) Pleasing precipitation performances – the microscale way . Science in School 57 .
- Make chemistry practice fun with chemical card games: Johnson P (2024) Stealth learning – how chemical card games can improve student participation . Science in School 68 .
- Use geometry to estimate the CO 2 absorbed by a tree in the schoolyard: Schwarz A et al. (2024) How much carbon is locked in that tree? Science in School 67 .
- Try some experiments with gases to illustrate stoichiometric reactions and combustion: Paternotte I, Wilock P (2022) Playing with fire: stoichiometric reactions and gas combustion . Science in School 59 .
- Promote critical thinking by adding some variables to the classic candle-mystery experiment: Ka Kit Yu S (2024) A twist on the candle mystery . Science in School 66 .
- Explore laboratory safety with creative horror stories about lab disasters: Havaste P, Hlaj J (2024) Lab disasters: creative learning through storytelling . Science in School 68 .
- Try a classroom activity to extract essential oils from fragrant plants: Allan A, Worley B, Owen M (2018) Perfumes with a pop: aroma chemistry with essential oils . Science in School 44 : 40–46.
- Read about the environmental costs of fireworks: Le Guillou I (2021) The dark side of fireworks . Science in School 55 .
Dr Adrian Allan is a teacher of chemistry at Dornoch Academy, UK. He was selected to represent the UK at the Science on Stage conferences in 2017 and 2019. He has presented Science on Stage webinars and workshops around Europe on microscale chemistry and using magic to teach science.
Bob Worley, FRSC, is the (semiretired) chemistry advisor for CLEAPSS in the UK. He taught chemistry for 20 years, and in 1991, he joined CLEAPSS, which provides safety and advisory support for classroom experiments. In carrying out these duties, he gained an interest in miniaturizing experiments to improve safety and convenience. He was awarded the 2021 Excellence in Secondary and Further Education Prize for significant and sustained contributions to the development and promotion of safe practical resources for teachers worldwide.
Microscale chemistry (and science in general!) is an incredibly important field. The work done by Bob and Adrian is second to none, and will allow teacher (and learners) from all areas to get “stuck in” with micro. From a budget and safety stand point, it makes all the sense in the world to approach thing on a microscale, especially in the current financial climate.
John Cochrane, Chemistry Teacher. Greenfaulds High School, Scotland.
Supporting materials
Activity 1 Student worksheet
Activity 1 Calculation details
How to make a spirit burner
Activity 2 Student worksheet
Activity 2 Calculation details
Download this article as a PDF
Share this article
Subscribe to our newsletter, related articles.
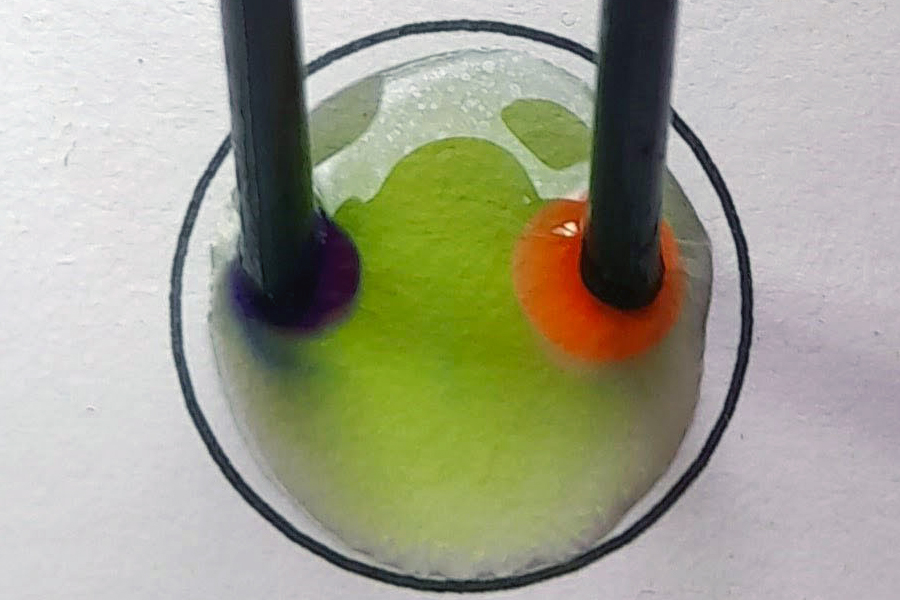
Elegant electrolysis – the microscale way
Enhance your students’ knowledge of electrolysis using quick, safe, and easy microscale chemistry…

Making chemistry accessible for students with vision impairment
Discover simple adaptations to apparatus and experiments that make practical chemistry more accessible to students with vision impairment.
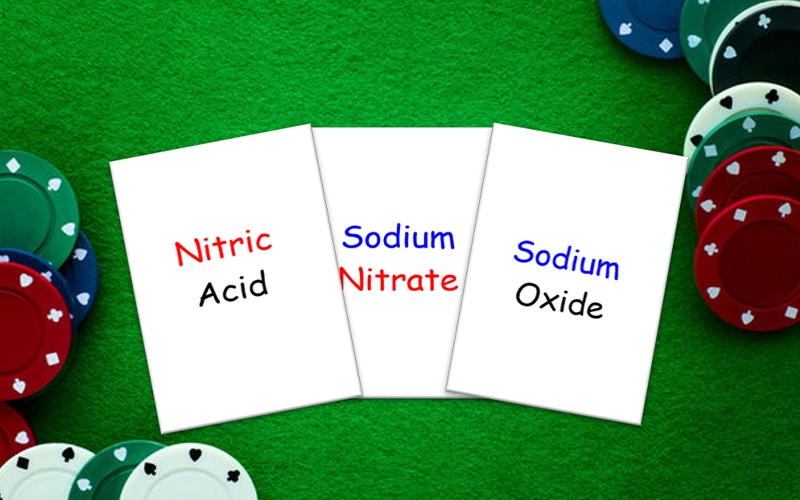
Stealth learning – how chemical card games can improve student participation
Play your cards right: Everyone enjoys playing games, so use chemical card games to get students to learn through play without them realising.
Thank you for visiting nature.com. You are using a browser version with limited support for CSS. To obtain the best experience, we recommend you use a more up to date browser (or turn off compatibility mode in Internet Explorer). In the meantime, to ensure continued support, we are displaying the site without styles and JavaScript.
- View all journals
- Explore content
- About the journal
- Publish with us
- Sign up for alerts
- Open access
- Published: 02 September 2024
The carbon emission reduction effect of green fiscal policy: a quasi-natural experiment
- Shuguang Wang 1 ,
- Zequn Zhang 1 ,
- Zhicheng Zhou 2 &
- Shen Zhong 2
Scientific Reports volume 14 , Article number: 20317 ( 2024 ) Cite this article
1 Altmetric
Metrics details
- Climate-change impacts
- Climate-change mitigation
- Environmental impact
Carbon emission reduction is crucial for mitigating global climate change, and green fiscal policies, through providing economic incentives and reallocating resources, are key means to achieve carbon reduction targets. This paper uses data covering 248 cities from 2003 to 2019 and applies a multi-period difference-in-differences model (DID) to thoroughly assess the impact of energy conservation and emission reduction ( ECER ) fiscal policies on enhancing carbon emission ( CE 1 ) reduction and carbon efficiency ( CE 2 ). It further analyzes the mediating role of Green Innovation ( GI ), exploring how it strengthens the impact of ECER policies. We find that: (1) ECER policies significantly promote the improvement of carbon reduction and CE 2 , a conclusion that remains robust after excluding the impacts of concurrent policy influences, sample selection biases, outliers, and other random factors. (2) ECER policies enhance CE 1 reduction and CE 2 in pilot cities by promoting green innovation, and this conclusion is confirmed by Sobel Z tests. (3) The effects of ECER policies on CE 1 reduction and the improvement of CE 2 are more pronounced in higher-level cities, the eastern regions and non-resource cities. This research provides policy makers with suggestions, highlighting that incentivizing green innovation through green fiscal policies is an effective path to achieving carbon reduction goals.
Similar content being viewed by others
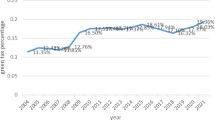
Green taxation, regional green development and innovation: Mechanisms of influence and policy optimization
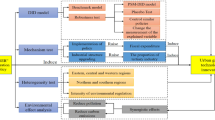
The impact of China’s energy saving and emission reduction demonstration city policy on urban green technology innovation
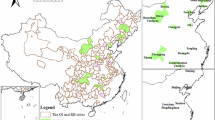
Can place-based policy reduce carbon emissions? Evidence from industrial transformation and upgrading exemplary zone in China
Introduction.
Efforts to mitigate global climate change through the reduction of CE 1 have emerged as a shared objective among nations globally 1 . From the initiation of the United Nations Framework Convention on Climate Change to the enactment of the Kyoto Protocol and the adoption of the Paris Agreement, these pacts reflect the unified resolve of nations to tackle global climate change 2 , 3 . With the acceleration of global industrialization and the continuous increase in energy demand, there has been a significant rise in the emissions of greenhouse gases, especially carbon dioxide, posing an unprecedented challenge to the Earth’s climate system 4 . These issues encompass the escalation of average global temperatures, a surge in severe weather occurrences, accelerated glacier melt, and a persistent increase in sea levels 5 , 6 , 7 , which threaten the balance of natural ecosystems and have profound impacts on the economic development and well-being of human societies. Therefore, adopting effective carbon reduction strategies to slow these climate change trends has become an urgent task faced globally.
In the current field of CE 1 reduction research, the focus is mainly on implementing policies such as carbon emission trading 8 , smart city pilot policies 9 , and low-carbon city pilot policies 10 . Among these policies, green fiscal policy, as a core strategy to mitigate the impact of climate change, is increasingly recognized by the academic community and policymakers for its importance in promoting CE 1 reduction 11 , 12 . This policy directly impacts CE 1 in economic activities through adjustments in the tax system, provision of fiscal subsidies, and increased investments in renewable energy and low-carbon technologies 13 . Green fiscal policies differ from traditional environmental protection measures by employing a mechanism that combines incentives and constraints, aiming to encourage enterprises to adopt emission reduction measures. In the implementation process of green fiscal policies, governments encourage enterprises to reduce CE 1 by adjusting tax policies 14 . Specifically, the ECER policy impacts the carbon emissions of demonstration cities through a combination of financial incentives and target constraints. The demonstration period lasts for three years, during which the central government provides reward funds for demonstration projects. The amount of these rewards is determined by the category of the city: 600 million RMB annually for municipalities and city clusters, 500 million RMB annually for sub-provincial cities and provincial capitals, and 400 million RMB annually for other cities. Local governments have the discretion to decide how to utilize these funds, while the central government is responsible solely for project record management. Additionally, the central government conducts annual and overall target assessments of the demonstration cities. The results of the annual assessment influence the reward funds for the following year: cities that perform excellently will receive an additional 20% of reward funds, while those that fail to meet the standards will have 20% of their funds withdrawn. The overall assessment results are linked to the demonstration qualification and reward funds; cities that fail to meet the overall targets or have serious issues will lose their demonstration status and have all reward funds withdrawn. This financial incentive mechanism ensures that local governments have sufficient financial support when implementing green technologies and projects, promoting increased energy efficiency and the widespread adoption of clean energy. Simultaneously, through the target constraint mechanism, the central government strictly supervises and incentivizes local governments’ efforts to reduce emissions, ensuring effective policy implementation. Under the dual pressure of financial incentives and performance assessments, local governments actively adopt various measures to promote energy conservation and emission reduction, including investing in green infrastructure, promoting energy-saving technologies, and optimizing energy structures, thereby achieving significant reductions in carbon emissions.
Furthermore, innovation and technological breakthroughs significantly enhance the effectiveness of green fiscal policies in reducing carbon emissions. Specifically, technological advancements improve energy efficiency, reducing the energy consumption per unit of output; they lower the production costs of clean energy, promoting its widespread adoption; and they advance carbon capture and storage technologies, directly reducing industrial carbon dioxide emissions. These technological improvements bolster the impact of green fiscal policies, making them more effective in achieving carbon reduction targets. However, the implementation of green fiscal policies also faces some challenges. Firstly, balancing the relationship between economic development and environmental protection to avoid potential negative impacts such as job losses and industrial relocation during policy execution is an issue that policymakers need to consider. Secondly, the effective implementation of green fiscal policies requires strong policy support and regulatory mechanisms to ensure that policy measures are effectively executed and can adapt to constantly changing economic and environmental conditions. Therefore, evaluating the carbon reduction effect of such policies is of significant importance for achieving long-term environmental sustainability and promoting the green economic transformation.
This paper analyzes the impact of green fiscal policies on carbon emissions and carbon efficiency. Relevant research mainly focuses on the following two areas: studies on the factors influencing carbon emissions, and research related to environmental regulations and energy conservation and emission reduction fiscal policies.
Firstly, a substantial body of literature focuses on the factors influencing carbon emissions, with some studies specifically examining the impact of government intervention and environmental regulation on CO2 emissions. These studies are closely related to the theme of this paper. From an economic perspective, numerous studies have demonstrated that economic growth significantly impacts carbon emissions 15 , 16 , 17 . Generally, increased economic activity is associated with higher energy consumption, leading to higher carbon emissions. However, as economies reach a certain level of development, the Environmental Kuznets Curve (EKC) phenomenon may occur, where carbon emissions begin to decrease after reaching a certain economic threshold 18 , 19 . Research has also confirmed that economic growth increases the ecological footprint, leading to environmental degradation 20 . For example, economic growth, income inequality, and energy poverty have increased environmental pressure in BRICS countries 21 . In Pakistan, institutional quality has led to higher CO 2 emissions, but economic development can help reduce these emissions 22 . From a social perspective, the acceleration of urbanization is typically accompanied by increased energy consumption, thereby raising carbon emissions. There is a long-term and short-term U-shaped relationship between urbanization and the environment 23 . Upgrading existing infrastructure can enable various sectors to produce minimal waste that impacts emissions 24 . Changes in consumption levels and population structure also significantly affect carbon emissions 25 . From a policy perspective, government-enacted environmental regulations and policies, such as carbon taxes, carbon trading markets, emission standards, and renewable energy subsidies, play a crucial role in reducing carbon emissions. Innovations and environmental policies contribute to emission reductions both in the long and short term. Additionally, carbon pricing can reduce emissions in specific regions, although its impact is often more targeted at specific countries 26 . Carbon taxes and mitigation technologies are helping to achieve sustainable development goals for carbon mitigation 27 . Green energy investments are significantly associated with greenhouse gas emissions and support environmental quality 28 . However, these studies often overlook the impact of energy conservation and emission reduction fiscal policies on carbon emissions.
Secondly, there is a body of literature focusing on environmental regulation, which can be divided into two main areas: the impact of environmental regulation on the environment and its impact on the economy. On the one hand, extensive research has explored the environmental impact of regulation. Studies generally agree that stringent environmental regulations help reduce pollutant emissions and improve environmental quality. Environmental regulations significantly enhance the synergy between carbon reduction and air pollution control 29 . Target-based pollutant reduction policies effectively constrain the sulfur dioxide emissions of regulated enterprises, lowering their sulfur dioxide emission intensity, thereby demonstrating that stringent environmental regulations facilitate green transitions for businesses 30 . However, in some developing countries or regions with weak enforcement, the effectiveness of environmental regulations may be compromised. Despite strict regulatory policies being in place, inadequate enforcement or a lack of regulatory capacity may result in actual pollutant reduction falling short of expectations. On the other hand, part of the literature examines the economic impact of environmental regulation. Some studies suggest that environmental regulation can drive technological innovation and industrial upgrading, thereby promoting economic growth 31 . Strict environmental standards force companies to improve production processes and develop new environmental technologies, which can create new economic opportunities and growth points 32 . Environmental regulations significantly enhance green technological innovation 33 , and they have notably promoted green innovation across European countries 34 . Conversely, environmental regulations may increase operational costs for businesses, particularly in the short term due to compliance costs, which could inhibit economic growth. This is especially true for regions or countries that rely heavily on high-pollution, high-energy-consumption industries, where environmental regulation might lead to a slowdown in economic growth. Given that energy conservation and emission reduction fiscal policies are a form of environmental regulation, it is necessary to evaluate their effectiveness.
Thirdly, some literature evaluates the governance effectiveness of energy conservation and emission reduction fiscal policies. From an environmental perspective, these policies can reduce pollutants and enhance efficiency. On average, such policies have reduced industrial SO2 (sulfur dioxide) emissions by 23.8% and industrial wastewater discharge by 17.5% 35 . Additionally, energy conservation and emission reduction fiscal policies can effectively improve green total factor carbon efficiency 36 . From an economic perspective, these policies can promote investment and economic growth 37 . They have significantly improved green credit for enterprises and can facilitate sustainable urban development 38 .
In summary, there are two significant gaps in the existing literature. Firstly, although numerous studies have extensively explored the factors influencing carbon emissions from economic, social, and policy perspectives, relatively few have examined the relationship between ECER policies and carbon emissions. Specifically, most of the existing literature focuses on the impact of macroeconomic policies, industrial structure adjustments, and technological innovation on carbon emissions. However, there is a lack of systematic empirical analysis on how specific fiscal incentives directly affect carbon emissions, limiting our comprehensive understanding of the actual effects of fiscal policies on emission reduction. Secondly, most of the existing studies investigate carbon dioxide emissions from a single perspective, such as focusing on total carbon emissions, carbon intensity, or carbon efficiency. These studies lack a multi-faceted exploration of the relationship between a single policy and carbon emissions. Typically, research adopts a specific metric to measure policy effects, but this approach overlooks how different metrics might reveal various aspects of policy impact. Consequently, these studies fail to capture the multi-dimensional effects of policies on reducing carbon emissions comprehensively. This single-perspective research methodology cannot adequately reflect the multiple impacts of policies on carbon emissions across different scenarios and time periods. This paper aims to evaluate the impact of the ECER policy, jointly introduced by the Ministry of Finance and the National Development and Reform Commission in 2011, on CE1 and CE2. Given that the ECER policy was implemented in three batches of pilot cities, this study employs a multi-period Difference-in-Differences (DID) model for analysis. The advantage of this model lies in its ability to compare the effects of the policy before and after its implementation across multiple time points, thereby capturing the dynamic impacts of the policy. Furthermore, this article explores the mediating role of green innovation in the impact process of the ECER policy, revealing the policy’s varying effects on CE 1 and CE2 across different regions through heterogeneity analysis.The marginal contributions of this article: Firstly, this paper evaluates the relationship between ECER policies and carbon emissions, addressing a significant gap in the existing research. Although numerous studies have explored various factors influencing carbon emissions from different perspectives, there is a lack of systematic research on the actual effects of specific fiscal policies on energy conservation and emission reduction, particularly their direct impact on carbon emissions. Through empirical analysis and data validation, this study thoroughly investigates the specific mechanisms and effects of ECER policies on carbon emissions in practice, thus filling this research gap. Secondly, this paper systematically assesses the relationship between ECER policies and carbon emissions from two key perspectives: total carbon emissions and carbon efficiency. By considering these two important indicators, this study not only examines the impact of ECER fiscal policies on overall carbon emissions but also analyzes their role in improving carbon efficiency. Through an in-depth analysis of these two metrics, this paper provides a more comprehensive and multi-dimensional view, systematically evaluating the effectiveness and mechanisms of ECER policies.
The remainder of the article is organized as follows: the second part discusses the policy background and theoretical analysis; the third part details the model settings and variable explanations; the fourth part presents the empirical analysis; the fifth part analyzes regional heterogeneity; and the last part concludes with conclusions and policy recommendations.
Policy background and theoretical analysis
Policy background.
In 2011, the Ministry of Finance and the National Development and Reform Commission issued the “Notice on Conducting Comprehensive Demonstration Work of Fiscal Policies for Energy Conservation and Emission Reduction,” deciding to carry out comprehensive demonstrations of fiscal policies for ECER in some cities during the “Twelfth Five-Year” period. Beijing, Shenzhen, Chongqing, Hangzhou, Changsha, Guiyang, Jilin, and Xinyu were selected as the first batch of demonstration cities. In the subsequent years of 2013 and 2014, 10 and 12 cities were respectively chosen as pilot cities for the fiscal policies on ECER . Specifically, this policy uses cities as platforms and integrates fiscal policies as a means to comprehensively carry out urban ECER demonstrations in various aspects, including industrial decarbonization, transportation clean-up, building greening, service intensification, major pollutant reduction, and large-scale utilization of renewable energy. Its main goal in terms of CE 1 reduction is to establish a concept of green, circular, and low-carbon development in the demonstration cities, achieve widespread promotion of low-carbon technologies in industries, construction, transportation, and other fields, lead the pilot cities in ECER efforts across society, and significantly enhance their capacity for sustainable development. Figure 1 presents the spatial distribution of ECER policy pilot cities in the years 2011, 2013, and 2014 (This figure was created using ArcMap software).
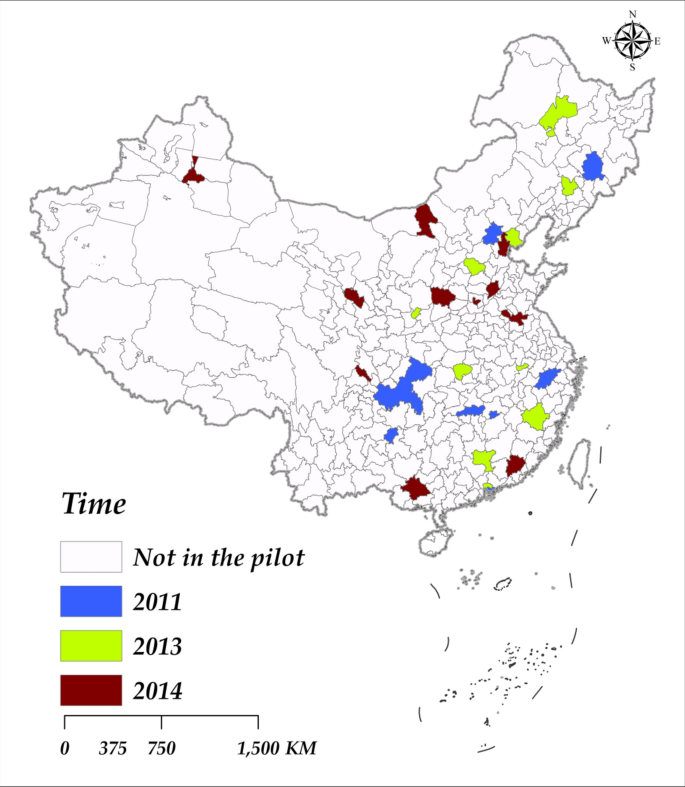
Distribution of ECER Policy Pilot Areas (Plan Approval Number GS(2019)1822).
Theoretical analysis
Carbon emission reduction effect of green fiscal policy.
Green fiscal policy, as a significant environmental governance tool, promotes the transformation of the economic and social system towards low-carbon, sustainable development through fiscal measures 39 . Its CE 1 reduction effects can be described from the following aspects. Firstly, green fiscal policy encourages the research and application of green technologies through economic incentives (such as tax reductions and fiscal subsidies) 40 . These technologies include energy efficiency improvement technologies, clean energy technologies, and carbon capture and storage technologies, which directly reduce energy consumption and CE 1 in economic activities. Secondly, green fiscal policy influences the behavior of consumers and producers by affecting the price mechanism. The imposition of a carbon tax raises the cost of CE 1 , reflecting the external cost of CE 1 on the environment, encouraging enterprises to take emission reduction measures, and prompting consumers to prefer low-carbon products and services 41 . The change in price signals promotes the transformation of the entire society’s energy consumption structure towards more efficient and low-carbon directions. Furthermore, green fiscal policy can support CE 1 reduction-related infrastructure construction and public service improvements through the guidance and redistribution of funds. This includes the construction and optimization of public transportation systems, urban greening, and forest conservation projects, which not only directly or indirectly reduce CE 1 but also enhance the carbon absorption capacity of cities and regions. Lastly, green fiscal policies, by raising public environmental awareness and participation, create a conducive atmosphere for all sectors of society to join in carbon reduction efforts 42 . Governments can increase public awareness of climate change and inspire a low-carbon lifestyle through the promotion and education of fiscal policies, providing broader social support for carbon reduction 43 .
Green fiscal policies not only drive a reduction in CE 1 but also stimulate sustainable economic growth. By taxing high-carbon activities, offering financial subsidies and incentives for green projects, these policies channel capital towards low-carbon and green industries. This not only mitigates negative environmental impacts but also fosters the development of emerging green technologies and sectors. As the green industry expands and low-carbon technologies become more widespread, economic growth increasingly relies on clean and efficient energy use 44 , thereby enhancing the CE 2 . Thus, the implementation of green fiscal policies demonstrates a commitment to transitioning towards a low-carbon economy, playing a crucial role in the global response to climate change, achieving a win–win for environmental protection and economic growth.
Based on this, the article proposes hypothesis 1: Green fiscal policies can promote CE 1 reduction effects and enhance CE 2 .
Mechanism analysis
Green innovation is a key factor in driving sustainable development, particularly playing a significant role in CE 1 reduction and efficiency enhancement. By introducing and adopting new environmentally friendly technologies and processes, green innovation not only significantly reduces greenhouse gas emissions but also enhances the efficiency of energy use and resource management, thus promoting a harmonious coexistence between economic activity and environmental protection. Green innovation, through the development and adoption of renewable energy technologies such as solar, wind, and biomass energy, directly reduces reliance on fossil fuels and the corresponding CE 1 . The application of these technologies not only reduces the carbon footprint but also promotes the diversification of energy supply and enhances energy security 45 . Green innovation also plays an essential role in improving energy efficiency. By adopting more efficient production processes and energy-using equipment, businesses and households can accomplish the same tasks or meet the same living needs with lower energy consumption, thus reducing CE 1 46 . Additionally, green innovation encompasses the concepts and practices of the circular economy, which encourages the reuse, recycling, and recovery of materials, reducing the extraction and processing of new materials and further lowering CE1s in the production process 47 . Green innovation includes the development of Carbon Capture, Utilization, and Storage (CCUS) technologies, which can directly capture carbon dioxide from industrial emissions and either convert it into useful products or safely store it, thereby reducing the carbon content in the atmosphere 48 . On the policy and management level, green innovation also involves establishing and refining mechanisms such as carbon pricing, green taxes, and carbon trading, which promote the adoption of low-carbon and environmentally friendly technologies and behaviors among businesses and individuals through economic incentives 49 . Based on this, the article proposes hypothesis H2: Green fiscal policies can promote CE 1 reduction effects and CE 2 by fostering green innovation.
In conclusion, the theoretical framework, as shown in Fig. 2 .

Theoretical framework.
Model setting and variable description
To address the limitations faced by traditional regression models in evaluating policy implementation effects, this study utilizes DID model for analysis. Given the variation in the policy implementation years in this paper, the traditional DID model cannot be used 50 . Accordingly, this paper draws on the approach of Beck et al. 51 , employing a DID with multiple time periods to assess the policy effects, with the model set up as follows:
Y in the model is the explained variable, indicating CE 1 and CE 2 of the city i in the annual t . Treated i is the group variable, where it takes the value 1 if city i belongs to the treatment group, and 0 if it belongs to the control group; Post it is the post-treatment period dummy variable, where it takes the value 1 for city i in year t if ECER policy has been officially implemented, and 0 if it has not been officially implemented. This study investigates the impact of energy conservation and emission reduction fiscal policies on urban CE 1 and CE 2 by examining the effect of the interaction term Treated × Post it on the dependent variable. The coefficient β 1 measures the impact of the policy on the dependent variable. Controls in this study represent control variables, specifically urbanization rate ( lnur ), foreign direct investment level ( lnfdi ), industrial structure ( lnis ), level of scientific and technological expenditure ( lnsst ), and fiscal revenue and expenditure level ( lnfre ), among others. \(\nu\) , \(\tau\) and \(\varepsilon\) represent city fixed effects, time fixed effects, and random error terms, respectively.
Considering the three-year implementation period of green fiscal policies, it is necessary to establish an exit mechanism for the treatment group. Drawing on existing literature 12 , this paper constructs the following treatment groups: the first batch of pilot cities from 2011 to 2014 is set to 1; the second batch of pilot cities from 2013 to 2016 is set to 1; the third batch of pilot cities from 2014 to 2017 is set to 1, with other years set to 0. The pilot cities are shown in Fig. 3 .
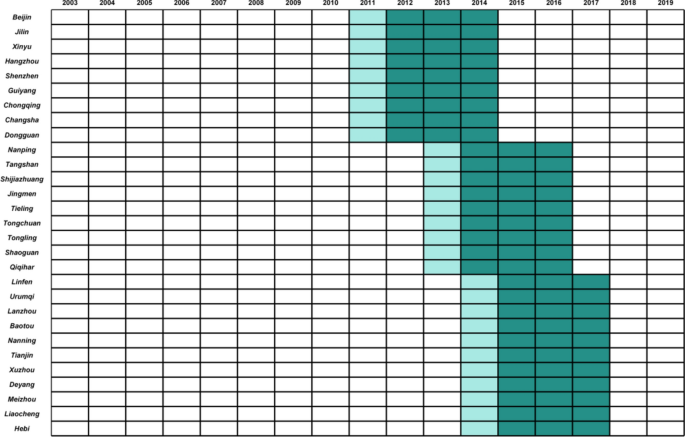
ECER policy implementation period.
Variables and data sources
Explained variables.
Carbon Emissions: Drawing from existing literature, this article utilizes current CE 1 data to calculate CE 1 52 , 53 . It follows the guidelines on greenhouse gas emission allocations by the IPCC , taking into account the emissions of carbon dioxide within the administrative boundaries of each city. Territorial emissions refer to emissions occurring within the managed territory and maritime areas under the jurisdiction of a region 54 , including emissions from socio-economic sectors and direct residential activities within regional boundaries 55 .
Carbon Efficiency: Following existing literature, this paper measures CE 2 using the ratio of CE 1 to GDP 56 .
In examining the correlation between CE 1 and economic efficiency, Fig. 4 a provides an overview of the evolution of CE 1 from 2003 to 2019, while Fig. 4 b offers a detailed portrayal of the progress in CE 2 over the same period. Figure 4 a reveals a steady increase in total CE 1 beginning in 2002, with a notable acceleration post-2009, peaking in 2017. Despite some fluctuations and a slight dip in 2018, the figures for 2019 remained just below the peak, overall indicating an upward trajectory. In contrast, Fig. 4 b demonstrates a year-on-year improvement in CE 2 , measured in tens of thousands of yuan output per ton of carbon emitted, starting in 2003. The pace of growth accelerated significantly after 2011, reaching its zenith in 2019. This signifies a substantial rise in the economic output efficiency per unit of carbon emitted, revealing a reduction in carbon dependency within economic activities. The combined analysis of both figures indicates that, alongside economic growth, there has been a notable advancement in optimizing CE 2 .
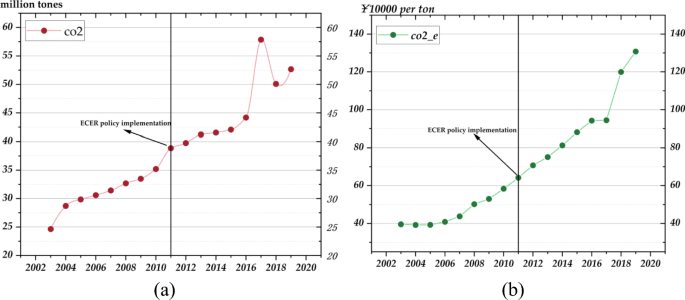
Trends in CE 1 ( a ) and CE 2 ( b ) (2003–2019).
Control variables
To eliminate the interference of omitted variables on the research results, this article selects the following control variables 57 , 58 : Urbanization rate ( lnur ), which refers to the ratio of urban population to total population; Level of foreign direct investment ( lnfdi ), the ratio of actual foreign investment to the GDP ; Industrial structure ( lnis ), the proportion of the secondary industry in GDP ; Level of science and technology expenditure ( lnsst ), the ratio of science and technology expenditure in ten thousand to GDP in hundred billion; Fiscal revenue and expenditure level ( lnfre ), the sum of local fiscal budget revenue and expenditure to GDP . To reduce heteroscedasticity in the data, this article takes the logarithm of all control variables. Table 1 reports the definitions of the main variables in this paper.
Sample selection and data source
We selects cities at the prefecture level in China from 2003 to 2019 as the research sample. Considering that missing data can affect the results, this paper excludes samples with missing data, ultimately obtaining 3134 samples. The CE 1 data in this paper comes from the China Emissions Accounts and Datasets (CEADs), which provides CE 1 data from 1997 to 2019, so the sample period for this paper ends in 2019. The control variable data are all sourced from the China City Statistical Yearbook covering the years 2004 to 2020. Table 2 provides descriptive statistics for the main variables in this paper.
Eliminating interference
In a quasi-natural experiment, various factors may influence the relationship between the implementation of green fiscal policies and the reduction of carbon emissions. To address this, we employed multiple methods to control for these potential confounding variables. Firstly, we introduced control variables to eliminate or reduce the interference of external factors on the main research relationship, ensuring the accurate estimation of the effects of green fiscal policies. Secondly, we adopted a two-way fixed effects model to control for time-invariant city characteristics and potential common time trends. Thirdly, we conducted parallel trend tests to verify whether the trends of the treatment and control groups were consistent before the policy implementation, ensuring the validity of the Difference-in-Differences (DID) estimates. Additionally, we performed multiple robustness checks, including propensity score matching and excluding the effects of other concurrent policies, to test the robustness of the results. Finally, we confirmed the reliability of the results through placebo tests. These methods collectively help to effectively reduce the interference of external variables, ensuring the accuracy and reliability of the research findings.
Empirical results
Benchmark regression analysis.
We employs a two-way fixed effects model for the empirical analysis of the CE 1 reduction effects of ECER policies, with the estimation results presented in Table 3 . Columns (1) to (3) of Table 3 report the estimation results of green fiscal policies on CE 1 . The results show that, when the model does not include control variables, the implementation of green fiscal policies has an estimated coefficient of − 0.070 for CE 1 , significant at the 1% level, indicating that the CE 1 of pilot cities are 7.0% lower than those of non-pilot cities. After adding control variables, the results do not change significantly. Columns (4) to (6) report the estimation results of green fiscal policies on CE 2 . The results indicate that, when the model does not include control variables, the implementation of green fiscal policies has an estimated coefficient of 0.099 for CE 2 , significant at the 1% level, suggesting that the CE 2 of pilot cities is 9.9% higher than that of non-pilot cities. After including control variables, the results remain largely unchanged. This provides evidence for Hypothesis 1: ECER policies have a significant CE 1 reduction effect and also significantly promote CE 2 .
To further illustrate the step-by-step changes in the coefficients, this paper presents Fig. 5 . The horizontal axis of Fig. 5 represents the number of control variables, while the vertical axis indicates the coefficients, with the grey area denoting the error bars. As evident from Fig. 5 , the coefficients and error bars exhibit minimal variation with the increase in control variables, indicating a negligible impact of the number of control variables on the coefficients and highlighting their stability. This finding suggests that the primary regression coefficients remain consistent even when more control variables are included in the analysis, underscoring the model’s robustness.
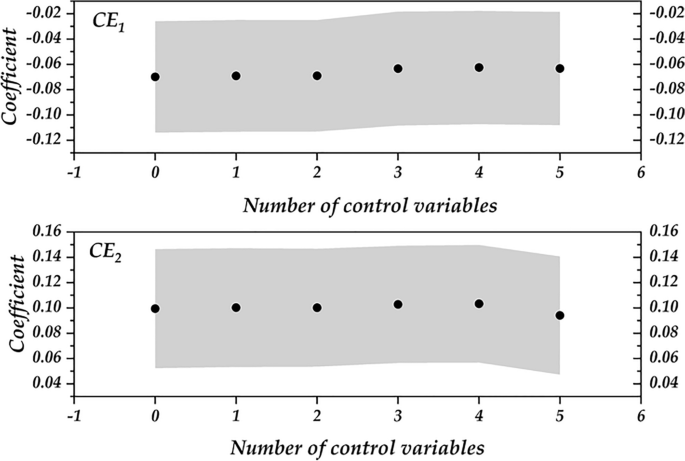
Plot of coefficient variation based on the step by step method.
Parallel trend test
The prerequisite for using DID model to evaluate policies is the parallel trends assumption. This implies that, before the policy intervention, the treatment group and the control group should exhibit similar trends without systematic differences. After the policy intervention, the trends between these two groups should diverge significantly. Following existing literature 50 , 59 , 60 , this paper employs an event study approach to analyze the effects before and after the policy implementation.
In Eq. ( 2 ), the variable Treated still represents cities that have been approved to establish pilot ECER policies. To avoid perfect multicollinearity, this paper uses the year before policy implementation as the baseline group, meaning that k = − 1 is not included in the regression equation, and the other parts of the model are consistent with the baseline model. If the coefficient is not significant when k < 0 , it indicates that the estimated results satisfy the parallel trends assumption. Figure 6 shows that, before the implementation of the policy, all coefficients are not significant, and in the fifth year after policy implementation, the coefficients start to become significant. This indicates that the implementation of ECER policies has a significant promotional effect on CE 1 reduction and CE 2 in the pilot areas, but this effect has some lag.
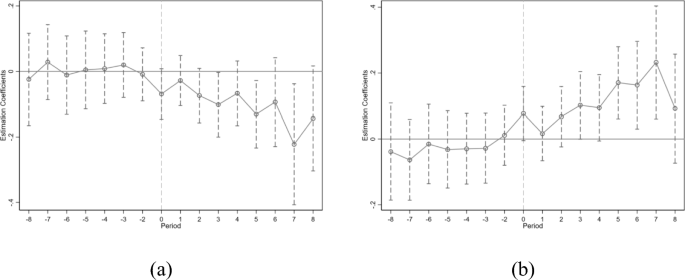
Parallel trend test of CE 1 ( a ) and CE 2 ( b ).
Robustness test
Exclusion of contemporaneous policies.
The smart city construction policy began with the “Notice on Carrying out the National Smart City Pilot Work” issued by the Ministry of Housing and Urban–Rural Development in 2012, with smart city pilots being established in 2012, 2013, and 2014 61 . This paper excludes all smart pilot cities and re-runs the regression, with results shown in columns (1) and (2) of Table 4 . The results indicate that contemporaneous policies during the sample period caused some interference with the estimated coefficients, but the extent is very limited. The implementation of ECER policies still has statistically and economically significant effects on promoting CE 1 reduction and CE 2 in pilot cities.
We employs the Propensity Score Matching (PSM) method to process the data, aiming to reduce data bias and the impact of confounding factors 62 , 63 . Through PSM-DID analysis, the results show that after matching, the absolute bias (|bias|) of all variables decreases by more than 70%, and the p -values are not statistically significant. This comparative analysis reveals the effectiveness of PSM in reducing the initial bias between the treatment and control groups. Therefore, the matching process successfully achieves balance in characteristics between the two groups across key indicators, making the assessment of the treatment effect more accurate and reliable.
Table 4 reports the results of the PSM. The propensity score matching results show a substantial decrease in |bias| for variables, highlighting an enhanced balance between treated and control groups post-matching. For instance, the absolute bias for “lnur” dropped from 86.0% to just 3.3%, showcasing a 96.2% reduction in bias, which underscores the effectiveness of the matching process. Similarly, other variables like “lnfdi”, “lnis”, and “lnsst” experienced significant reductions in bias. The p >|t| values, mostly above 0.05 post-matching, indicate that the differences between groups are not statistically significant, affirming the success of the matching in minimizing discrepancies and improving comparability.
Figure 7 displays the matching results of PSM. The results indicate that after the matching process, the percentage bias (%bias) for the control variables all remain below 10%. This finding fully confirms the effectiveness of the PSM method in balancing key characteristics between the experimental and control groups, thereby ensuring the accuracy and reliability of subsequent analyses.
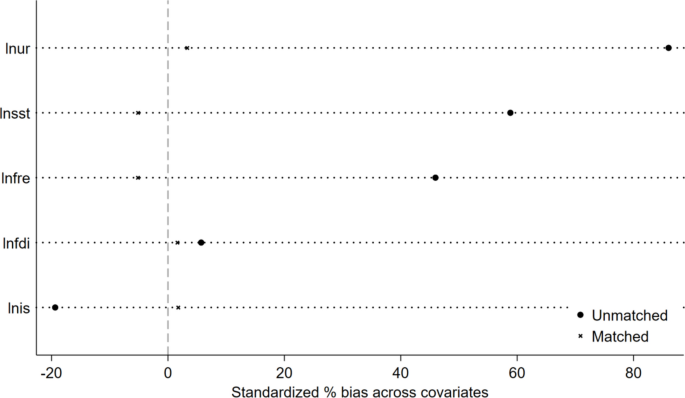
Balance test.
This paper conducts an empirical analysis using matched data, with the results shown in columns (3) and (4) of Table 5 . The results indicate that ECER policy still has a significant CE 1 reduction effect and also significantly promotes CE 2 . This suggests that there is no significant impact of self-selection bias on the regression results in this study.
To reduce the impact of outliers on regression analysis, this paper adopts a winsorization process 39 , 64 , which involves replacing observations below a certain threshold with the 1st percentile and those above the threshold with the 99th percentile before conducting the regression. Columns (5) and (6) of Table 5 display the analysis results after this treatment, showing that the impact of outliers on the regression results is not significant.
Replacement sample time
Considering the potential unique impact of the COVID-19 pandemic on CE 1 and CE 2 in 2019, this paper decided to exclude data from 2019 to ensure the robustness of the research results, thus avoiding the interference of pandemic-related outliers in the analysis. Subsequently, the paper conducted an empirical analysis based on the updated dataset, with the analysis results presented in columns (7) and (8) of Table 5 . The analysis results indicate that after excluding the special impact of the COVID-19 pandemic, the CE 1 reduction effect of the green fiscal policy remains significant, and there is still a significant promotional effect on CE 2 .
Placebo test
The DID model is based on the common trends assumption, which posits that, in the absence of an intervention, the trends of the treatment and control groups would have been similar 65 . By conducting a placebo test on data from before the intervention, this assumption can be tested for validity. If significant ‘intervention effects’ are also found during the placebo test conducted before the intervention or at irrelevant time points, this indicates that the effects estimated by DID are actually caused by other unobserved factors, rather than the intervention itself 66 . Referencing the placebo practices in existing literature 59 , this paper tests for the impact of unobservable factors on the estimation results. The study randomizes the impact of ECER policies across cities, selecting treatment groups randomly from 248 cities, with the remaining cities serving as control groups. This randomization process is repeated 500 times to generate a distribution graph of the regression coefficients, where the dashed line in the graph represents the actual regression coefficient, as specifically shown in Fig. 8 . Figure 8 a represents the placebo test for CE 1 , and Fig. 8 b for CE 2 . From Fig. 8 , it is evident that after randomizing the core explanatory variables, the mean of the coefficients is close to 0, and the mean of the coefficients after randomization significantly deviates from their true values. This indicates that, excluding the interference of other random factors on the empirical results, the green fiscal policy has a significant effect on CE 1 reduction and significantly promotes CE 2 .
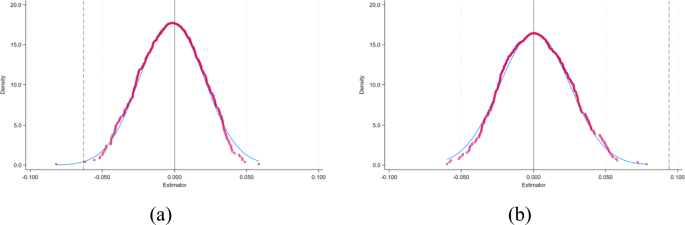
Placebo test of CE 1 ( a ) and CE 2 ( b ).
Mechanism test
The analysis results presented earlier indicate that the ECER policy has significantly promoted CE 1 reduction and the improvement of CE 2 in pilot cities. Accordingly, this study will further explore the mechanism of action of ECER policy and has constructed the following model:
GI refers to green innovation. Following existing literature, this study uses the number of green invention patent grants ( lngi_invention ) and the total number of green patents per 10,000 people ( lnpgi_total ) as proxy variables for green innovation 67 , 68 . Due to the evident causal inference flaws in the three-stage mediation mechanism test 69 , this study refers to the mediation effect test model by Niu et al. 70 and employs the Sobel test to further evaluate the regression results, thereby enhancing the completeness and credibility of the mechanism test 71 . The regression results are shown in Table 6 . Columns (1) and (4) report the impact of the ECER policy on green innovation, with significant results. This confirms hypothesis H2: green fiscal policies can promote CE 1 reduction effects and CE 2 by fostering green innovation. Moreover, the Sobel Z coefficients are greater than 2.58, indicating that the mediating variable has a sufficiently strong explanatory power for the total effect.
Heterogeneity analysis
By city grade.
In the process of urbanization and industrialization, a city’s level often reflects its level of economic development, capacity for technological innovation, infrastructure completeness, and the comprehensiveness of its public services. This paper categorizes the sample cities based on their tier into higher-level cities (provincial capitals, sub-provincial cities, and municipalities directly under the Central Government) and general cities, and conducts regression analysis. The regression results shown in Table 7 , specifically in columns (1), (2), (6), and (7), indicate that in higher-tier cities, the coefficients of the ECER policy on CE 1 and CE 2 for pilot cities are -0.098 and 0.118, respectively, significant at the 1% level. However, in general cities, the absolute values of the coefficients are smaller and not significant. From this, we can conclude that the ECER policy’s effect on CE 1 reduction and the enhancement of CE 2 is more significant in higher-tier cities compared to general cities. Higher-level cities, with their advanced economic structures, abundant fiscal resources, high levels of technological innovation, and strong policy enforcement capabilities, make the green fiscal policy more effective in these areas in terms of CE 1 reduction and the promotion of CE 2 . Firstly, economically developed higher-tier cities have more sufficient fiscal funds and investment capacity, which can support large-scale green infrastructure construction and green technology R&D, thereby directly reducing urban CE 1 and improving energy use efficiency. Secondly, technological innovation is a key factor in improving CE 2 . As centers of technological innovation and information exchange, higher-level cities are more likely to attract and gather high-tech companies and research institutions, promoting the development and application of green technologies, and effectively reducing CE 1 . Additionally, higher-tier cities usually have more comprehensive laws, regulations, and policy enforcement mechanisms, ensuring the effective implementation and regulation of green fiscal policies. Also, residents in these cities often have higher environmental awareness and a preference for green consumption, which helps to create a favorable social atmosphere for the implementation of green fiscal policies. Finally, due to their strong regional influence and exemplary role, higher-tier cities can promote green transformation and low-carbon development in surrounding areas and even the entire country through policy guidance and market incentives, further amplifying the CE 1 reduction effect and enhancing the impact on CE 2 of green fiscal policies.
By geographic location
Given the significant differences in economic development levels, resource endowments, and institutional environments across regions in China, the implementation effects of the ECER policy may exhibit heterogeneity. Therefore, this paper divides the sample into eastern, central, and western regions for analysis and conducts regressions separately. The regression results are presented in Table 7 . Columns (3) to (5) and (8) to (9) of Table 7 show the regression results for CE1s and CE 2 , respectively, with columns (3) and (8) representing the results for the eastern region. The analysis indicates that, in the eastern region, the ECER policy significantly promotes carbon reduction and CE 2 . Although the policy’s effects in the central region are less than those in the eastern region, they still exhibit a positive impact. In contrast, in the western region, the ECER policy’s promotional effects on carbon reduction and CE 2 are not significant.
This analysis reveals that, within the regional development pattern of China, the eastern regions exhibit more significant outcomes in terms of the CE 1 reduction effect and the enhancement of CE 2 under green fiscal policies compared to the central and western regions. Firstly, as the most economically developed area in China, the eastern region, with its leading total economic output, industrialization, and urbanization levels, provides a solid fiscal support and technological foundation for the implementation of green fiscal policies. This economic advantage enables the eastern region to allocate more resources to the research, development, and application of green technologies, as well as related infrastructure construction, thereby effectively promoting CE 1 reduction and energy efficiency improvement. Secondly, environmental policies and regulations in the eastern region are generally stricter and more advanced. Coupled with a higher public awareness of environmental protection, this creates a favorable social environment and policy atmosphere for the implementation of green fiscal policies and carbon reduction. Additionally, the industrial structure in the eastern region is more optimized and high-end compared to the central and western regions, with a larger proportion of the service industry and high-tech industries, which typically have lower energy consumption intensity and CE 1 , facilitating the improvement of overall CE 2 . Furthermore, as an important gateway for international trade and investment, the eastern region is more open to adopting and introducing advanced green technologies and management practices from abroad, accelerating the pace of green transformation. Lastly, the dense urban network and well-developed transportation and logistics systems in the eastern region provide convenient conditions for the effective implementation of green fiscal policies. Therefore, due to comprehensive advantages in economic development level, industrial structure, policy environment, technological innovation capability, and infrastructure, the eastern region demonstrates more significant performance in the CE 1 reduction effect and the promotion of CE 2 under green fiscal policies.
Figure 9 reports the main regression coefficients and error bars from the heterogeneity analysis, clearly illustrating the distribution of coefficients.
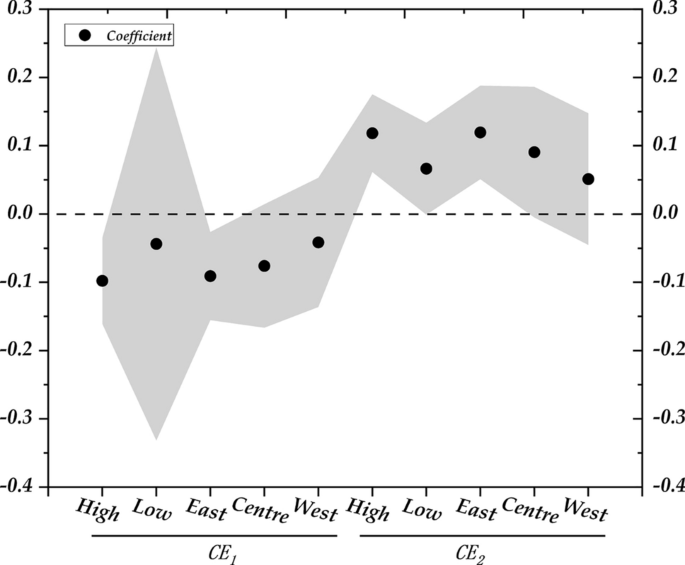
Results of heterogeneity analysis.
Classification by resource-based city
Resource-based cities center on industries involved in the extraction and processing of local natural resources, including minerals and forests 72 , 73 , 74 . Due to their unique urban characteristics, these cities may have a specific impact on the efficacy of ECEP policy. Consequently, this paper follows the guidelines set forth by the State Council in the “National Plan for Sustainable Development of Resource-based Cities (2013–2020),” dividing the sample into resource-based and non-resource-based cities for separate regression analyses, the results of which are presented in Table 8 . Columns (1) and (2) detail the regression outcomes for CE 1 , while columns (3) and (4) address CE 2 . The findings reveal that, compared to resource-based cities, the effect of ECEP policies on carbon reduction is more pronounced in non-resource-based cities, with a similarly more substantial impact on the promotion of CE 2 .
Upon conducting a thorough analysis of the disparities in how non-resource-based cities and resource-based cities respond to ECER policies, a significant finding emerges: non-resource-based cities, due to their diversified industrial structures and lower reliance on highly polluting and energy-intensive heavy industries and mineral resource extraction, demonstrate a stronger capacity to adopt and promote new energy, clean energy, and energy-efficient technologies. This characteristic of their industrial structure not only facilitates effective carbon reduction efforts but also propels a shift in economic growth models towards services, high-tech industries, and innovation-driven sectors, which are associated with lower energy consumption and carbon intensities. Therefore, the potential for ECER policies to enhance CE 2 and reduce CE 1 is greater in these cities. In contrast, resource-based cities, due to their long-standing dependence on resource extraction, exhibit significant inertia in their economic structure, technological levels, and employment opportunities. This inertia not only complicates their transition and industrial restructuring but also increases the associated costs. Against this backdrop, non-resource-based cities are more likely to achieve notable successes in implementing ECER policies compared to their resource-based counterparts.
Conclusions and policy recommendations
Conclusions.
Based on the city-level dataset from 2003 to 2019, this paper employs a multi-time point difference-in-differences model to thoroughly explore the impact of the ECER policy on CE 1 reduction and CE 2 , reaching the following conclusions:
The ECER policy is confirmed to play a significant role in promoting the reduction of CE 1 and enhancing CE 2 . This conclusion remains robust even after controlling for factors that might affect the accuracy of the assessment, such as contemporaneous policy interferences, sample selection biases, extreme value treatments, and other random factors. This indicates that the ECER policy has important practical implications in mitigating climate change impacts, and its effects are not significantly influenced by the aforementioned potential interferences. The ECER policy effectively promotes CE 1 reduction and CE 2 improvements by incentivizing the research and application of green technologies. This finding underscores the mediating role of green innovation in environmental policies, highlighting that fiscal incentives such as tax breaks and subsidies are crucial for promoting technological innovation and application, and further achieving environmental benefits. The CE 1 reduction effect and CE 2 enhancement of the ECER policy are more pronounced in economically developed, higher-tier cities and in the eastern regions. This may be due to these areas having better infrastructure, higher technological innovation capabilities, more abundant fiscal resources, and stronger public environmental awareness, which all provide strong support for the effective implementation of the ECER policy. Moreover, this variation also suggests that policymakers need to consider regional characteristics when implementing relevant policies to maximize policy effectiveness.
Existing literature has explored the role of energy conservation and emission reduction fiscal policies in environmental protection, such as green credit 37 , ESG performance 75 , green total factor carbon efficiency 36 , and sustainable urban development 38 . These studies report the positive impact of such policies on the environment. However, they do not directly examine the impact of these policies on pollutants. Our study extends the existing literature by investigating the relationship between these policies and carbon emissions. Green fiscal policies significantly promote the reduction of carbon emissions (CE1) and the improvement of carbon efficiency (CE2) through economic incentives, price mechanisms, infrastructure support, and increasing public environmental awareness. Specifically, these policies encourage the research and application of green technologies, change consumer and producer behavior, optimize energy consumption structures, support related infrastructure construction, and increase public participation in low-carbon living. Additionally, green fiscal policies promote sustainable economic growth by directing funds towards low-carbon and green industries, fostering the development of green technologies and industries. Overall, green fiscal policies have not only achieved significant environmental protection results but also played a crucial role in realizing the dual goals of economic growth and environmental protection.
Despite the significant findings, our study has some limitations. Firstly, the data is limited to 248 cities from 2003 to 2019, which may not fully capture the long-term impact of ECER policies. Secondly, reliance on existing data may introduce biases, as not all relevant factors could be considered. Future research could address these limitations by expanding the dataset, including more diverse regions, and employing alternative methods to validate these findings.
Policy recommendations
Based on the above analysis, the policy recommendations of this paper are as follows:
Continue to increase fiscal support. The government should continue to enhance fiscal support for the ECER policy, including expanding the scope of tax reductions and increasing the level of fiscal subsidies, especially for those projects and technologies that can significantly improve energy efficiency and reduce CE 1 . This will further stimulate the innovation motivation of enterprises and research institutions, accelerating the research and development (R&D) and application of low-carbon technologies.
Optimize policy design and implementation mechanisms. Considering the robustness of the ECER policy effects, the government should further refine the policy design to ensure that measures precisely target sectors and aspects with high CE 1 . Concurrently, it is crucial to establish and enhance the supervision mechanism for policy execution, ensuring effective implementation of policy measures. This approach also necessitates timely adjustments and optimizations of the policy to tackle new challenges effectively.
Establish a dedicated Green Technology Innovation Fund. This fund aims to provide financial support specifically for R&D and promotion of green technologies with high CE 2 . By offering startup capital, R&D subsidies, and rewards for the successful commercialization of green technologies, the fund can not only stimulate the innovation drive of enterprises and research institutions but also accelerate the transformation of green technologies from theory to practice. Consequently, this will promote CE 1 reduction and CE 2 enhancement on a broader scale. This initiative directly responds to the importance of fiscal incentive measures for promoting technological innovation and application emphasized in the research, ensuring the ECER policy maximizes its benefits in promoting green development.
Differentiated policy design. Given the variations in the effects of the ECER policy across different regions, policymakers should design and implement differentiated energy-saving and emission reduction policies based on regional factors such as economic development level, industrial structure, and resource endowment. For economically more developed areas with a stronger technological foundation, CE 1 reduction can be promoted by introducing higher standards for environmental protection and mechanisms for rewarding technological innovation. For regions that are relatively less economically developed, the focus should be on providing technical support and financial assistance to enhance their capacity for CE 1 reduction.
Green fiscal policies play a crucial role in reducing carbon emissions and promoting sustainable economic growth, but their impact on social and income inequality needs careful consideration. Firstly, while policies like carbon taxes are effective in reducing emissions, they may place a significant burden on low-income households, as a larger proportion of their income goes towards energy and basic necessities. To mitigate this inequality, governments can implement redistributive measures, such as using carbon tax revenues for direct subsidies or tax reductions for low-income families, ensuring social equity while achieving emission reductions. Secondly, green fiscal policies encourage investment in green technologies and the implementation of green projects. However, these incentives often favor businesses and wealthy families capable of making such investments, potentially widening income disparities. Therefore, policy design should consider inclusive growth by providing green job training and encouraging small and medium-sized enterprises to participate in green projects, ensuring that various social strata benefit from the green economy. Furthermore, in terms of public investment, governments should prioritize low-income and marginalized communities, ensuring they also benefit from the construction of green infrastructure. This includes prioritizing the development of public transportation and renewable energy projects in these areas, thereby reducing living costs and improving the quality of life for these communities. By adopting these redistributive measures and inclusive policy designs, green fiscal policies can achieve the goals of environmental protection and economic growth while effectively mitigating their negative impacts on social and income inequality, promoting sustainable and inclusive development.
When evaluating various policy tools for achieving carbon reduction goals, it is evident that carbon taxes, renewable energy subsidies, ECER policies, emissions trading systems, and energy efficiency standards each have their unique advantages (see Table 9 ). Carbon taxes leverage price mechanisms to encourage emissions reduction and provide redistribution opportunities, while renewable energy subsidies promote technological advancement and market development. ECER policies offer direct incentives and support for infrastructure, resulting in long-term environmental benefits. Emissions trading systems combine cap-and-trade controls with market flexibility, and energy efficiency standards provide direct pathways to emissions reduction. In practical applications, the integrated use of multiple policy tools, fully utilizing their respective advantages, can more effectively achieve carbon reduction goals and drive the transition to a low-carbon economy. Policymakers must consider equity, economic impact, and public acceptance when designing these policies to balance environmental protection with economic growth. Through careful integration and balanced implementation, green fiscal policies can significantly reduce carbon emissions while promoting sustainable and inclusive economic development.
Data availability
The datasets used and/or analysed during the current study available from the corresponding author on reasonable request.
Martin, G. & Saikawa, E. Effectiveness of state climate and energy policies in reducing power-sector CO2 emissions. Nat. Clim. Change 7 , 912–919 (2017).
Article ADS Google Scholar
Soergel, B. et al. A sustainable development pathway for climate action within the UN 2030 Agenda. Nat. Clim. Change 11 , 656–664 (2021).
Terhaar, J., Frölicher, T. L., Aschwanden, M. T., Friedlingstein, P. & Joos, F. Adaptive emission reduction approach to reach any global warming target. Nat. Clim. Change 12 , 1136–1142 (2022).
Gidden, M. J. et al. Aligning climate scenarios to emissions inventories shifts global benchmarks. Nature 624 , 102–108 (2023).
Article ADS PubMed PubMed Central Google Scholar
Brown, P. T. et al. Climate warming increases extreme daily wildfire growth risk in California. Nature 621 , 760–766 (2023).
Article ADS PubMed Google Scholar
Liu, Y., Cai, W., Lin, X. & Li, Z. Increased extreme swings of Atlantic intertropical convergence zone in a warming climate. Nat. Clim. Change 12 , 828–833 (2022).
Tebaldi, C. et al. Extreme sea levels at different global warming levels. Nat. Clim. Change 11 , 746–751 (2021).
Chaobo, Z. & Qi, S. Can carbon emission trading policy break China’s urban carbon lock-in?. J. Environ. Manag. 353 , 120129 (2024).
Article Google Scholar
Wu, S. Smart cities and urban household carbon emissions: A perspective on smart city development policy in China. J. Clean Prod. 373 , 133877 (2022).
Zhang, H., Feng, C. & Zhou, X. Going carbon-neutral in China: Does the low-carbon city pilot policy improve carbon emission efficiency. Sustain. Prod. Consump. 33 , 312–329 (2022).
Shan, Y. et al. Impacts of COVID-19 and fiscal stimuli on global emissions and the Paris Agreement. Nat. Clim. Chang. 11 , 200–206 (2021).
Sun, L. & Feng, N. Research on fiscal policies supporting green and low-carbon transition to promote energy conservation and emission reduction in cities: Empirical evidence from China. J. Clean. Prod. 430 , 139688 (2023).
Zhu, X. & Lu, Y. Open economy, fiscal expenditure of environmental protection and pollution governance: Evidences from China’s provincial and industrial panel data. China Popul. Resour. Environ. 27 , 10–18 (2017).
Google Scholar
Fan, H. & Liang, C. The pollutant and carbon emissions reduction synergistic effect of green fiscal policy: Evidence from China. Financ. Res. Lett. 58 , 104446 (2023).
Waheed, R., Sarwar, S. & Wei, C. The survey of economic growth, energy consumption and carbon emission. Energy Rep. 5 , 1103–1115 (2019).
Esso, L. J. & Keho, Y. Energy consumption, economic growth and carbon emissions: Cointegration and causality evidence from selected African countries. Energy 114 , 492–497 (2016).
Li, J. & Li, S. Energy investment, economic growth and carbon emissions in China-Empirical analysis based on spatial Durbin model. Energy Policy 140 , 111425 (2020).
Yin, J., Zheng, M. & Chen, J. The effects of environmental regulation and technical progress on CO 2 Kuznets curve: An evidence from China. Energy Policy 77 , 97–108 (2015).
Al-Mulali, U., Saboori, B. & Ozturk, I. Investigating the environmental Kuznets curve hypothesis in Vietnam. Energy Policy 76 , 123–131 (2015).
Danish, Hassan, S. T., Baloch, M. A., Mahmood, N. & Zhang, J. Linking economic growth and ecological footprint through human capital and biocapacity. Sustain. Cities Soc. 47 , 101516 (2019).
Hassan, S. T., Batool, B., Zhu, B. & Khan, I. Environmental complexity of globalization, education, and income inequalities: New insights of energy poverty. J. Clean. Prod. 340 , 130735 (2022).
Hassan, S. T., Danish, Khan, S.U.-D., Xia, E. & Fatima, H. Role of institutions in correcting environmental pollution: An empirical investigation. Sustain. Cities Soc. 53 , 101901 (2020).
Awan, A., Sadiq, M., Hassan, S. T., Khan, I. & Khan, N. H. Combined nonlinear effects of urbanization and economic growth on CO2 emissions in Malaysia. An application of QARDL and KRLS. Urban Clim. 46 , 101342 (2022).
Khan, K. & Khurshid, A. Are technology innovation and circular economy remedy for emissions? Evidence from the Netherlands. Environ. Dev. Sustain. 26 , 1435–1449 (2024).
Zhu, Q. & Peng, X. The impacts of population change on carbon emissions in China during 1978–2008. Environ. Impact Assess. Rev. 36 , 1–8 (2012).
Khurshid, A., Rauf, A., Qayyum, S., Calin, A. C. & Duan, W. Green innovation and carbon emissions: the role of carbon pricing and environmental policies in attaining sustainable development targets of carbon mitigation—Evidence from Central-Eastern Europe. Environ. Dev. Sustain. 25 , 8777–8798 (2023).
Li, Z. et al. Climate change and the UN-2030 agenda: Do mitigation technologies represent a driving factor? New evidence from OECD economies. Clean Technol. Environ. Policy 25 , 195–209 (2023).
Hassan, S. T., Batool, B., Sadiq, M. & Zhu, B. How do green energy investment, economic policy uncertainty, and natural resources affect greenhouse gas emissions? A Markov-switching equilibrium approach. Environ. Impact Assess. Rev. 97 , 106887 (2022).
Liao, N., Luo, X. & He, Y. Could environmental regulation effectively boost the synergy level of carbon emission reduction and air pollutants control? Evidence from industrial sector in China. Atmos. Pollut. Res. 15 , 102173 (2024).
Meng, X., Zhang, M. & Zhao, Y. Environmental regulation and green transition: Quasi-natural experiment from China’s efforts in sulfur dioxide emissions control. J. Clean Prod. 434 , 139741 (2024).
Chen, J. & Hu, L. Does environmental regulation drive economic growth through technological innovation: Application of nonlinear and spatial spillover effect. Sustainability 14 , 16455 (2022).
Zhang, Y. & Li, X. Environmental regulation and high-quality economic growth: Quasi-natural experimental evidence from China. Environ. Sci. Pollut. Res. 29 , 85389–85401 (2022).
Wang, X., Chai, Y., Wu, W. & Khurshid, A. The empirical analysis of environmental regulation’s spatial spillover effects on green technology innovation in China. Int. J. Environ. Res. Public Health 20 , 1069 (2023).
Article PubMed PubMed Central Google Scholar
Khurshid, A., Huang, Y., Cifuentes-Faura, J. & Khan, K. Beyond borders: Assessing the transboundary effects of environmental regulation on technological development in Europe. Technol. Forecast. Soc. Change 200 , 123212 (2024).
Zhu, Y., Han, S., Zhang, Y. & Huang, Q. Evaluating the effect of government emission reduction policy: Evidence from demonstration cities in China. Int. J. Environ. Res. Public Health 18 , 4649 (2021).
Li, G. & Wang, X. Can green fiscal policy improve green total factor carbon efficiency? Evidence from China. J. Environ. Plan. Manag. https://doi.org/10.1080/09640568.2024.2352554 (2024).
Cheng, Y. & Xu, Z. Fiscal policy promotes corporate green credit: Experience from the construction of energy conservation and emission reduction demonstration cities in China. Green Financ. 6 , 1–23 (2024).
Lin, B. & Zhu, J. Impact of energy saving and emission reduction policy on urban sustainable development: Empirical evidence from China. Appl. Energy 239 , 12–22 (2019).
Liu, S., Xu, P. & Chen, X. Green fiscal policy and enterprise green innovation: evidence from quasi-natural experiment of China. Environ. Sci. Pollut. Res. 30 , 94576–94593 (2023).
Nordhaus, W. The Climate Casino: Risk, Uncertainty, and Economics for a Warming World (Yale University Press, 2013). https://doi.org/10.2307/j.ctt5vkrpp .
Book Google Scholar
Green, J. F. Does carbon pricing reduce emissions? A review of ex-post analyses. Environ. Res. Lett. 16 , 043004 (2021).
Ma, B., Sharif, A., Bashir, M. & Bashir, M. F. The dynamic influence of energy consumption, fiscal policy and green innovation on environmental degradation in BRICST economies. Energy Policy 183 , 113823 (2023).
Hu, Y., Ding, Y., Liu, J., Zhang, Q. & Pan, Z. Does carbon mitigation depend on green fiscal policy or green investment?. Environ. Res. Lett. 18 , 045005 (2023).
Nie, C., Li, R., Feng, Y. & Chen, Z. The impact of China’s energy saving and emission reduction demonstration city policy on urban green technology innovation. Sci. Rep. 13 , 15168 (2023).
Fischedick, M. et al. Industry. In: Climate Change 2014: Mitigation of Climate Change. Contribution of Working Group III to the Fifth Assessment Report of the Intergovernmental Panel on Climate Change. Technical Report. (2014).
Sorrell, S. Reducing energy demand: A review of issues, challenges and approaches. Renew. Sustain. Energy Rev. 47 , 74–82 (2015).
Ghisellini, P., Cialani, C. & Ulgiati, S. A review on circular economy: The expected transition to a balanced interplay of environmental and economic systems. J. Clean. Prod. 114 , 11–32 (2016).
Leung, D. Y. C., Caramanna, G. & Maroto-Valer, M. M. An overview of current status of carbon dioxide capture and storage technologies. Renew. Sustain. Energy Rev. 39 , 426–443 (2014).
Stiglitz, J. E. et al. Report of the High-Level Commission on Carbon Prices. 1–61 (2017) https://doi.org/10.7916/d8-w2nc-4103 .
Callaway, B. & Sant’Anna, P. H. C. Difference-in-differences with multiple time periods. J. Econom. 225 , 200–230 (2021).
Article MathSciNet Google Scholar
Beck, T., Levine, R. & Levkov, A. Big bad banks? The winners and losers from bank deregulation in the United States. J. Financ. 65 , 1637–1667 (2010).
Shan, Y. et al. City-level emission peak and drivers in China. Sci. Bull. 67 , 1910–1920 (2022).
Shan, Y. et al. City-level climate change mitigation in China. Sci. Adv. 4 , eaaq0390 (2018).
Eggleston, H. S., Buendia, L., Miwa, K., Ngara, T. & Tanabe, K. 2006 IPCC Guidelines for National Greenhouse Gas Inventories. (2006).
Shan, Y. et al. Methodology and applications of city level CO2 emission accounts in China. J. Clean. Prod. 161 , 1215–1225 (2017).
Xu, Q., Zhong, M. & Cao, M. Does digital investment affect carbon efficiency? Spatial effect and mechanism discussion. Sci. Total Environ. 827 , 154321 (2022).
Article PubMed Google Scholar
Sun, W. & Huang, C. How does urbanization affect carbon emission efficiency? Evidence from China. J. Clean. Prod. 272 , 122828 (2020).
Opoku, E. E. O. & Boachie, M. K. The environmental impact of industrialization and foreign direct investment. Energy Policy 137 , 111178 (2020).
Li, P., Lu, Y. & Wang, J. Does flattening government improve economic performance? Evidence from China. J. Dev. Econ. 123 , 18–37 (2016).
Guo, Q. & Zhong, J. The effect of urban innovation performance of smart city construction policies: Evaluate by using a multiple period difference-in-differences model. Technol. Forecast. Soc. Chang. 184 , 122003 (2022).
Guo, Q., Wang, Y. & Dong, X. Effects of smart city construction on energy saving and CO 2 emission reduction: Evidence from China. Appl. Energy 313 , 118879 (2022).
Abadie, A. & Imbens, G. W. Matching on the estimated propensity score. Econometrica 84 , 781–807 (2016).
Lyu, C., Xie, Z. & Li, Z. Market supervision, innovation offsets and energy efficiency: Evidence from environmental pollution liability insurance in China. Energy Policy 171 , 113267 (2022).
Park, B. U., Simar, L. & Zelenyuk, V. Local likelihood estimation of truncated regression and its partial derivatives: Theory and application. J. Econ. 146 , 185–198 (2008).
Baker, A. C., Larcker, D. F. & Wang, C. C. Y. How much should we trust staggered difference-in-differences estimates?. J. Financ. Econ. 144 , 370–395 (2022).
Arkhangelsky, D., Athey, S., Hirshberg, D. A., Imbens, G. W. & Wager, S. Synthetic difference-in-differences. Am. Econ. Rev. 111 , 4088–4118 (2021).
Cho, J. H. & Sohn, S. Y. A novel decomposition analysis of green patent applications for the evaluation of R&D efforts to reduce CO2 emissions from fossil fuel energy consumption. J. Clean. Prod. 193 , 290–299 (2018).
Zhao, P., Lu, Z., Kou, J. & Du, J. Regional differences and convergence of green innovation efficiency in China. J. Environ. Manag. 325 , 116618 (2023).
Jiang, T. Mediating and moderating effects in empirical studies of causal inference. China Ind. Econ. https://doi.org/10.19581/j.cnki.ciejournal.2022.05.005 (2022).
Niu, Z., Xu, C. & Wu, Y. Business environment optimization, human capital effect and firm labor productivity. J. Manag. World 39 , 83–100 (2023).
Aguinis, H., Edwards, J. R. & Bradley, K. J. Improving our understanding of moderation and mediation in strategic management research. Org. Res. Methods 20 , 665–685 (2017).
Chen, W. et al. Exploring the industrial land use efficiency of China’s resource-based cities. Cities 93 , 215–223 (2019).
Li, B., Han, Y., Wang, C. & Sun, W. Did civilized city policy improve energy efficiency of resource-based cities? Prefecture-level evidence from China. Energy Policy 167 , 113081 (2022).
Wang, Y. et al. Has the sustainable development planning policy promoted the green transformation in China’s resource-based cities. Resour. Conserv. Recycl. 180 , 106181 (2022).
Miao, S., Tuo, Y., Zhang, X. & Hou, X. Green fiscal policy and ESG performance: Evidence from the energy-saving and emission-reduction policy in China. Energies 16 , 3667 (2023).
Download references
This study is supported by the National Social Science Fund Major Project: “Research on the Policy System and Implementation Path to Accelerate the Formation of New Productive Forces,” Project Number: 23&ZD069.
Author information
Authors and affiliations.
School of Public Finance and Administration, Harbin University of Commerce, Harbin, 150028, China
Shuguang Wang & Zequn Zhang
School of Finance, Harbin University of Commerce, Harbin, 150028, China
Zhicheng Zhou & Shen Zhong
You can also search for this author in PubMed Google Scholar
Contributions
All authors contributed to the study conception and design. Material preparation, data collection and analysis were performed by ZC. Z, S. Z. The first draft of the manuscript was written by ZQ. Z and all authors commented on previous versions of the manuscript. All authors read and approved the final manuscript.
Corresponding author
Correspondence to Shen Zhong .
Ethics declarations
Competing interests.
The authors declare no competing interests.
Additional information
Publisher's note.
Springer Nature remains neutral with regard to jurisdictional claims in published maps and institutional affiliations.
Rights and permissions
Open Access This article is licensed under a Creative Commons Attribution 4.0 International License, which permits use, sharing, adaptation, distribution and reproduction in any medium or format, as long as you give appropriate credit to the original author(s) and the source, provide a link to the Creative Commons licence, and indicate if changes were made. The images or other third party material in this article are included in the article's Creative Commons licence, unless indicated otherwise in a credit line to the material. If material is not included in the article's Creative Commons licence and your intended use is not permitted by statutory regulation or exceeds the permitted use, you will need to obtain permission directly from the copyright holder. To view a copy of this licence, visit http://creativecommons.org/licenses/by/4.0/ .
Reprints and permissions
About this article
Cite this article.
Wang, S., Zhang, Z., Zhou, Z. et al. The carbon emission reduction effect of green fiscal policy: a quasi-natural experiment. Sci Rep 14 , 20317 (2024). https://doi.org/10.1038/s41598-024-71728-1
Download citation
Received : 07 March 2024
Accepted : 30 August 2024
Published : 02 September 2024
DOI : https://doi.org/10.1038/s41598-024-71728-1
Share this article
Anyone you share the following link with will be able to read this content:
Sorry, a shareable link is not currently available for this article.
Provided by the Springer Nature SharedIt content-sharing initiative
- Carbon emission reduction
- Fiscal policies for energy conservation and emission reduction
- Multi-period difference-in-differences method
- Quasi-natural experiment
By submitting a comment you agree to abide by our Terms and Community Guidelines . If you find something abusive or that does not comply with our terms or guidelines please flag it as inappropriate.
Quick links
- Explore articles by subject
- Guide to authors
- Editorial policies
Sign up for the Nature Briefing: Anthropocene newsletter — what matters in anthropocene research, free to your inbox weekly.

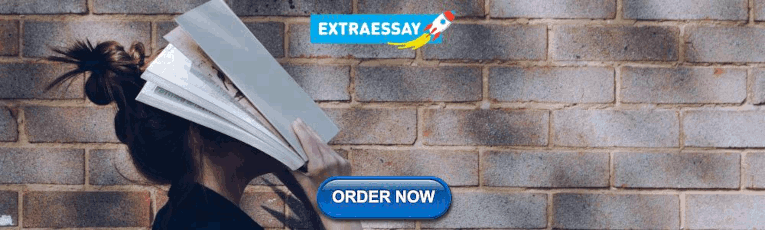
IMAGES
VIDEO
COMMENTS
Here are several examples of independent and dependent variables in experiments: In a study to determine whether how long a student sleeps affects test scores, the independent variable is the length of time spent sleeping while the dependent variable is the test score. You want to know which brand of fertilizer is best for your plants.
The two key variables in science are the independent and dependent variable, but there are other types of variables that are important. In a science experiment, a variable is any factor, attribute, or value that describes an object or situation and is subject to change. An experiment uses the scientific method to test a hypothesis and establish whether or not there is a cause and effect ...
Types of Variables. Independent Variable: The independent variable is the one condition that you change in an experiment. Example: In an experiment measuring the effect of temperature on solubility, the independent variable is temperature. Dependent Variable: The dependent variable is the variable that you measure or observe.
Some people refer to controlled variables as "constant variables." In the best experiments, the scientist must be able to measure the values for each variable. Weight or mass is an example of a variable that is very easy to measure. However, imagine trying to do an experiment where one of the variables is love. There is no such thing as a "love ...
The common types of variables in an experiment with an example. A-Z: ... top » science » experiments » experiment variables. 9 Types of Experiment Variables John Spacey, updated on June 23, 2019. Variables are factors that influence an experiment or that are of interest as a result. These include variables you change to test a hypothesis ...
Control Variable Examples. Anything you can measure or control that is not the independent variable or dependent variable has potential to be a control variable. Examples of common control variables include: Duration of the experiment. Size and composition of containers. Temperature.
The dependent variables are the things in an experiment that the scientist observes and measures. Scientists want to know if changing the independent variable has an effect on the dependent variables. In our dog example, the dependent variable is how much the dogs eat. This is what we are watching and measuring.
An example of a control in science would be cells that get no treatment in an experiment. Say there is a scientist testing how a new drug causes cells to grow. One group, the experimental group ...
This video explains independent, dependent, and controlled variables, with a special emphasis on controlling variables in experimental design. Helpful in u...
Independent and Dependent Variable Examples. In a study to determine whether the amount of time a student sleeps affects test scores, the independent variable is the amount of time spent sleeping while the dependent variable is the test score. You want to compare brands of paper towels to see which holds the most liquid.
Parts of the experiment: Independent vs dependent variables. Experiments are usually designed to find out what effect one variable has on another - in our example, the effect of salt addition on plant growth.. You manipulate the independent variable (the one you think might be the cause) and then measure the dependent variable (the one you think might be the effect) to find out what this ...
There are two parts of this hypothesis, and thus two experiments: Experiment #1: Measure the voltage of fresh AA batteries as they are used in different current drain devices. Experiment #2: Compare the rate of voltage change between devices with low, medium, and high current drain. The second experiment does not require any more data ...
1. Precision and Accuracy: By identifying and defining variables, researchers can design experiments with precision and accuracy. This clarity helps ensure that the measurements and observations made during the experiment are relevant to the research question, reducing the likelihood of errors or misinterpretations. 2.
In math, a variable is a quantity that can assume any value from a set of values. A scientific variable is a little more complicated, plus there are different types of scientific variables. Scientific variables are associated with the scientific method. Variables are things that are controlled and measured as part of a scientific experiment.
All types of variables can affect your science experiment. Get information about independent, dependent, control, intervening, and extraneous variables. ... It is dependent on your other variables. For example: In the diaper experiment, the dependent variable might be how much liquid you add to the diapers to see their liquid capacity. ...
Examples of Independent and Dependent Variables. 1. Gatorade and Improved Athletic Performance. A sports medicine researcher has been hired by Gatorade to test the effects of its sports drink on athletic performance. The company wants to claim that when an athlete drinks Gatorade, their performance will improve.
The independent variable is the cause. Its value is independent of other variables in your study. The dependent variable is the effect. Its value depends on changes in the independent variable. Example: Independent and dependent variables. You design a study to test whether changes in room temperature have an effect on math test scores.
FOCUS ON THE VARIABLES. Students can sometimes get lost in the steps of an experiment and forget what brought the results about. For this reason, I make sure that my students can communicate to each other what the variables were and, more importantly, why each variable exists.For example, in the plant growth experiment, the goal is for my students to be able to explain that:
The dependent variable is the factor that you observe or measure in an experiment. It is the variable that is affected by changes made to the independent variable. In the plant example, the dependent variable would be the plant's growth. We are. measuring the plant's growth to see how it is affected by the different amounts of water.
Here are some examples of an independent variable. A scientist is testing the effect of light and dark on the behavior of moths by turning a light on and off. The independent variable is the amount of light (cause) and the moth's reaction is the dependent variable (the effect). In a study to determine the effect of temperature on plant ...
A variable is used in a Fair Test. A variable is any one of the elements of the test which could be changed. They can change the outcome of an experiment. In a fair test, only one variable is allowed to be manipulated - all others have to be kept the same, or "controlled".
The independent variable is the variable that is controlled or changed in a scientific experiment to test its effect on the dependent variable. It doesn't depend on another variable and isn't changed by any factors an experimenter is trying to measure. The independent variable is denoted by the letter x in an experiment or graph.
Science in School 59. Promote critical thinking by adding some variables to the classic candle-mystery experiment: Ka Kit Yu S (2024) A twist on the candle mystery. Science in School 66. Explore laboratory safety with creative horror stories about lab disasters: Havaste P, Hlaj J (2024) Lab disasters: creative learning through storytelling.
Treated i is the group variable, where it takes the value 1 if city i belongs to the treatment group, and 0 if it belongs to the control group; Post it is the post-treatment period dummy variable ...