Revisiting Cu-based shape memory alloys: Recent developments and new perspectives
- Invited Feature Paper-Review
- Annual Issue: Early Career Scholars in Materials Science 2022
- Open access
- Published: 01 January 2022
- Volume 37 , pages 162–182, ( 2022 )
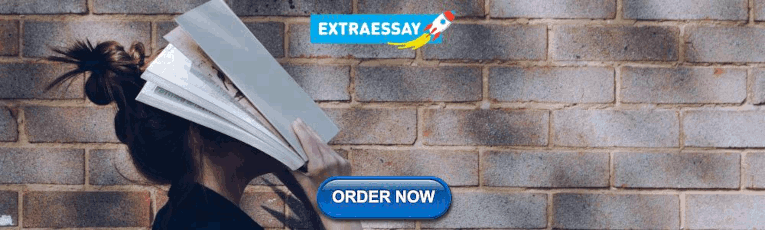
Cite this article
You have full access to this open access article
- E. M. Mazzer ORCID: orcid.org/0000-0001-9208-2047 1 ,
- M. R. da Silva 2 &
- P. Gargarella 2 , 3
7689 Accesses
49 Citations
Explore all metrics
Cu-based shape memory alloys belong to one important class of functional alloys, presenting shape memory effect and superelasticity due to their reversible martensitic transformation. Although they have been extensively studied since the middle of the last century, there are still many challenges to be solved. In the last decades, these alloys were extensively studied regarding new compositions, processing routes, phase transformation, mechanical and functional properties. Aspects of the thermoelastic phase transformation have been described using thermodynamic and thermo-mechanical studies, while the role of metallurgical features (such as grain size and morphology, ordering, precipitates and second phases) have been described mainly by phenomenological approach. In this sense this review discusses the advances in the general fundamentals of Cu-based shape memory alloys, the recent developments in processing routes, compositions, and applications in the last years.
Graphical abstract
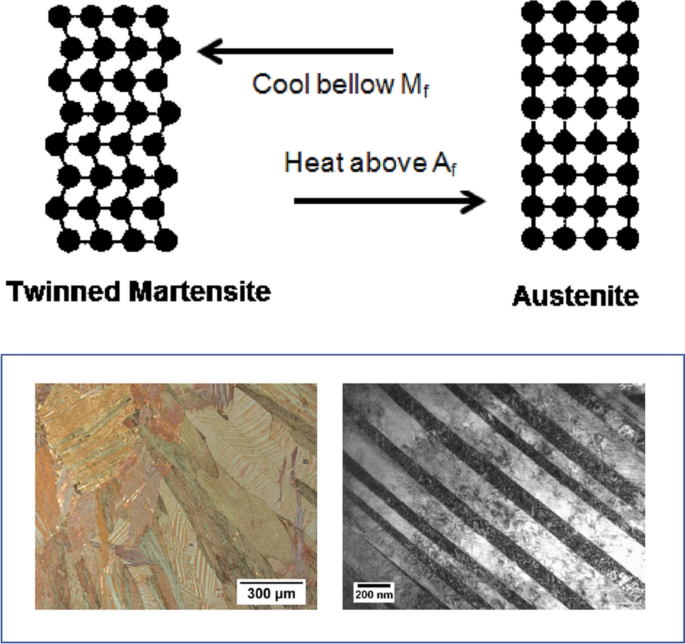
Similar content being viewed by others
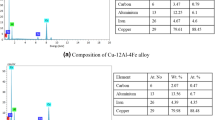
Experimental and theoretical analyses of transformation temperatures of Cu-based shape memory alloys
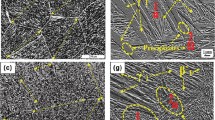
Effect of a fourth alloying element on the microstructure and mechanical properties of Cu–Al–Ni shape memory alloys
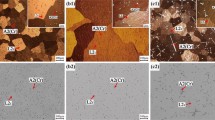
Microstructure characterization, stress–strain behavior, superelasticity and shape memory effect of Cu–Al–Mn–Cr shape memory alloys
Avoid common mistakes on your manuscript.
Introduction
The development of smart materials, which react with an external stimulus changing some properties or shape,has been boosted with the advancement of technology in several areas of knowledge. Among these areas, one of great importance is that regarding new and advanced materials development. Shape memory alloys (SMA) can be framed between the new functional materials, which can be used mainly in actuators, microcontrollers and sensors [ 1 ]. They present two unique functional properties that make them different from other common alloys: (i) shape memory effect (SME), which is the ability to recover the original shape after deformation; and (ii) superelasticity (SE) or (pseudoelasticity), which is the ability to recover a large non-linear strain upon loading–unloading [ 2 , 3 ]. Both properties occur due to a special class of non-diffusional solid state phase transformation, called thermoelastic martensitic transformation, which is a reversible transition with small volume change and low hysteresis. Within the most important SMA systems, Cu-based SMA plays an important role due to their large range of transformation temperatures and their flexibility, which enable the composition and processing routes tailoring, envisioning the optimization of their properties. Cu-based SMA must present one phase at high temperature called β , that transforms into a martensite by a thermoelastic transformation, with small volume change.
These Cu-based SMA are traditionally fabricated by casting or powder metallurgy with rigorous control of composition because a small shift from stoichiometry greatly affects their properties. Subsequent heat treatments are also commonly carried out: solution-treatments followed by quenching are used to form the martensitic phase and aging at lower temperatures is used to stabilize the martensite and memorize the shape. Because the Cu-based SMA are mostly applied in sheet or wire form, they may also undergo hot- or cold-working through forging, rolling, and extrusion. More recently, innovative processing routes have been proposed to fabricate different types of Cu-based SMA with improved functional and/or mechanical properties. New cyclic heat treatments with low cost and less time consuming have been proposed to obtain bulk single crystals of Cu–Al–Mn, which shows superior superelastic properties than in the polycrystalline form due to its polycrystal brittleness [ 4 ]. Moreover, additive manufacturing methods have also been proposed to fabricate complex shape Cu-based SMA and the high cooling rates applied during this processing usually result in parts with higher strength and ductility [ 5 ].
Currently, Cu-based shape memory alloys are classified into several alloy systems. Traditional systems such as Cu–Al–Zn and Cu–Al–Ni SMA, widely studied in recent years, continue to be reported in the literature [ 6 , 7 ]; however, other systems have been drawing attention, such as Cu–Al–Mn and Cu–Al–Be SMA [ 8 , 9 ]. Furthermore, a new SMA system was recently reported in the literature, based on Cu–Al–Fe–Mn [ 10 ], which shows shape memory mechanisms different from those conventionally observed. This different mechanism is related to the stress-induced martensite that can be unusually retained in the microstructure during the pre-strain condition in the superelastic regime. During heating, this retained martensitic phase rapidly transforms into austenite, and consequently, an instantaneous shape memory effect takes place. In addition to all these systems, the practice of alloying is widely used, which creates various SMA compositions. Several alloying elements can be added for the purpose of modify the properties of SMA aiming at certain specific applications. In some systems, such as for the Cu–Al–Fe–Mn SMA, there are not many reports in the literature regarding the alloying process, and thus just a small number of alloy compositions were investigated until now. In this case, the alloy composition space for these systems is still mostly unexplored and new compositions may be discovered in the future. In contrast, for the Cu–Al–Ni, Cu–Al–Mn, and Cu–Al–Be SMA, several studies were carried out [ 11 , 12 ], investigating the influence of different types of alloy elements on the properties of SMA. Cu–Al–Mn have currently highlighted among the Cu-based SMA since they may present shape memory properties similar to those of NiTi alloys, but with the advantage of a lower manufacturing cost [ 9 ]. These improved properties of Cu–Al–Mn SMA are directly related to the recent advancement in manufacturing processes, which allowed a more controlled alloy preparation.
Based on emerging compositions and processing routes, novel applications have been visioned for Cu-based SMA. Elastocaloric SMA and high temperature shape memory alloys (HTSMA) are in the spotlight of Cu-based SMA studies. In this review, the authors first present the general aspects of Cu-based SMA, following by the recent developments, where we discuss the novel processing route, new compositions, and recent applications. Lastly, we present the future perspectives and conclusions remarks.
General aspects of Cu-based shape memory alloys
General features.
Cu-based SMA are considered as an alternative to be used in high temperature applications and to other commercially available SMA in terms of their final cost, due to a lower cost of the elements, as well as a lower cost of production [ 1 , 3 ]. However, polycrystalline Cu-based SMA are susceptible to brittle intergranular fracture, which limits their commercial application. It occurs mainly due to the high elastic anisotropy, which generates stress concentration in the grain boundaries and triple junctions due to the incompatibility of plastic and elastic deformations between the grains [ 3 , 13 , 14 ].
In order to improve the mechanical properties of the polycrystalline Cu-based SMA, several efforts involving different types of processing [ 15 , 16 , 17 , 18 , 19 ] and modification in the compositions with the addition of alloying elements for grain refinement or precipitates formation have been carried out [ 20 , 21 , 22 , 23 ]. Nevertheless, the improvement in polycrystals SMA’s properties to the present days have been below the researcher’s expectations for them to be applied in engineering systems. The excellent shape memory properties of the Cu-based SMA single-crystals s were only attained by the so-called oligocrystalline alloys, in which the free surface area is larger than the grain boundary areas, allowing the transformation to occur with fewer constraints [ 13 , 14 , 24 , 25 , 26 ]. As a disadvantage, the shape of the oligocrystalline alloys is generally limited to small wires or ribbons whose grains must be in a bamboo-like structure to fill the entire cross-section of the material, which limits their processing and possible applications. Another issue involving SMAs is the stabilization of martensitic-austenitic phases. It affects the shape memory properties such as transforming temperatures, hysteresis, strain recovery, stresses during shape recovery, among others [ 3 ]. Stabilization of the phases is thermally activated and can occur both at room temperature and higher temperatures. Both problems, intergranular fracture and stabilization of phases are highly dependent on the material processing history and the specific composition of the alloy and are related to the alloy microstructure (mainly grain size, present phases, defects, martensitic types, and variants).
Shape memory effect and superelasticity in Cu-based SMA
The shape memory behavior occurs due to a phase transformation from a high temperature phase, called austenite (A—usually cubic) into a lower temperature phase with lower symmetry, called martensite (M—with tetragonal, orthorhombic, or monoclinic structure). In general, the austenite–martensite transformation occurs without diffusion of atoms, but rather by a shear of the crystalline lattice. This type of transformation is called "martensitic transformation" and can be irreversible, as observed in quenched mild steels, or reversible, as in SMA [ 27 , 28 ]. Regarding irreversible transformations, as occur in carbon steel and CoNi alloys, the transformation takes place during a fast cooling, forming martensites in the form of plates, laths or needles, which have immobile interfaces when applied temperature or mechanical loading [ 28 ]. In Cu-based SMA the transformation is almost completely reversible, generally called thermoelastic transformation. The main key factors for the transformation reversibility are their low hysteresis, low volume change and absence of plastic accommodation during the transformation from the austenite (also called the "parent phase"). Therefore, the martensitic microstructure is formed in a self-accommodation process, in which the variants (different orientations of martensites) are elastically accommodated inside a single preferred variant and, consequently, no macroscopic shape change is visible. This microstructure is easily modified with loading application. Thus, it is completely reversible when heated up to the austenitic field, generating a microstructure with little or no dislocations [ 28 ].
A necessary and sufficient condition for a thermoelastic martensitic transformation is that the variants are formed without any plastic deformation by dislocation slip [ 29 , 30 ]. Instead of this, they must self-accommodate [ 29 , 30 ], normally by twinning, forming twin-related variants. When mechanically loaded, the variants detwin and orient themselves in preferential directions, forming specific variants [ 27 ]. Thus, this reversible phase transformation between austenite–martensite forms the basis for the shape memory effect and superelasticity. Some important features of thermoelastic transformation are the four temperatures at which phase transformations occur. In cooling, the direct transformation takes place, which consists in the transformation from austenite to martensite. The start transformation temperature is called M s and the end of the reaction, where 100% of the austenite has been transformed, is called M f . The reverse transformation occurs upon heating, from martensite to austenite, and the start and end temperatures of this transformation are called A s and A f , respectively. Between the start and end temperature range, both phases are present in the microstructure. Figure 1 illustrates the transformations and their respective temperatures. There is usually a hysteresis observed between the beginning of reverse and direct transformation (| M s – A f | or | A s – M f |) and between the start and end interval of each transformation ( A f – A s or M s – M f ) [ 31 ].
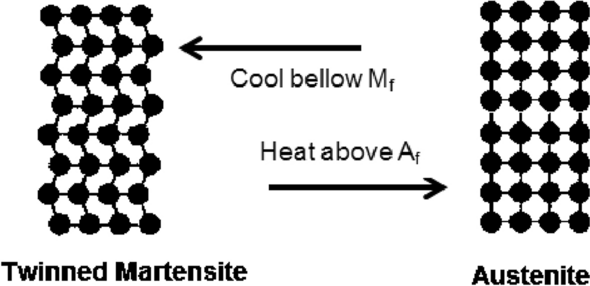
Scheme of the direct and reverse transformation and their final transformation temperatures.
When stress is applied to a SMA microstructure with twinned martensite, the variants are reoriented, and part of the microstructure becomes detwinned. This process results in a macroscopic shape change of the material, which is retained when the loading is released. In this case, the mechanism of deformation is the variants reorientation in a preferred direction, which is kept after unloading. When the metal is heated above A f , the reverse transformation takes place and the martensite transforms into austenite, recovering the original shape. When cooling from this phase, the twinned martensites are formed again, without an associated change of shape, closing the SME, as shown in Fig. 2 . In the detwinning process there is a minimum stress to start the process ( σ s ) and a stress at which it finishes completely ( σ f ). If the material is cooled from the austenitic region at a stress above σ s , the martensite formed will already be detwinned and there will be a change of shape with the phase transformation. With heating above A f the shape will be recovered. It is also known that the transformation temperatures are strongly dependent on the stress applied to the material and that the higher its magnitude, the higher the transformation temperatures [ 1 , 3 , 27 , 32 ].
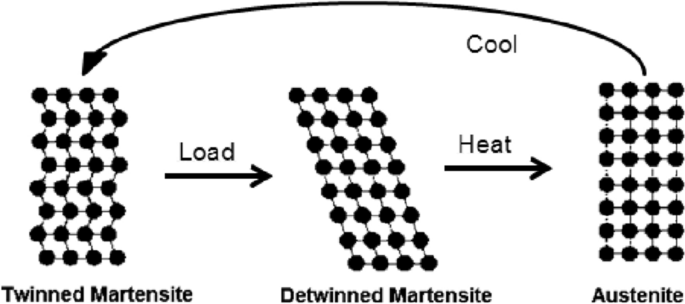
Illustration of the Shape Memory Effect cycle and the type of phase in each step.
Following the same principles, superelasticity is a phenomenon that happens due to the stress induced martensitic transformation within the austenitic field of the material. When the external loading is released, the martensite turns back into austenite and the deformation is recovered. Typically, a superelastcity thermomechanical path begins at a sufficiently higher temperature, i. e. above A f , at which the austenitic phase is stable. Thus, when stresses are applied, a state in which the detwinned martensite forms is reached and, when stresses are removed, the martensite is back transformed into the austenitic phase [ 1 , 3 , 16 , 27 ]. It is worth noticing that stress stabilizes the martensitic phase, increasing the transformation temperatures when applied. Figure 3 summarizes the stress–strain-temperature relationship of the SME and superelasticity in SMA and the critical stresses for plastic deformation by dislocation slip.
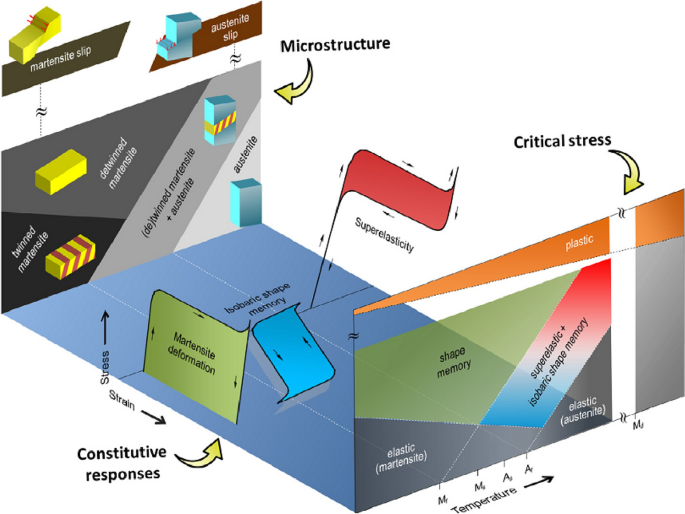
Reprinted from [ 33 ]. Copyright (2017) by permission from Elsevier.
Scheme showing the stress–strain and temperature relationship for SMA in austenitic and martensitic phases. SME, superelasticity and dislocation slip are represented in the stress-temperature maps.
Within the systems of alloys that present SME and superelasticity, there are three major groups in which most shape memory alloys fit in: Cu-based SMA (Cu–Al–Ni, Cu–Zn–Al, Cu–Al–Mn, etc.), those containing NiTi intermetallic and Fe-based SMA (Fe–Mn–Si, Fi–Ni–Co–Ti, etc.). The first two groups are more commonly used in engineering applications than the latter because of their better functional properties [ 32 ]. NiTi alloys have been used for military, medical, safety and robotic applications. In the military area, they are applied in aircraft components [ 1 , 3 ]. In the field of medicine, they are applied in tweezers to remove objects through small incisions; anchors in the form of hooks to attach the tendons to the bone were used for shoulder surgery; orthodontic wires, glasses frames; guide for catheters [ 32 , 34 , 35 ]. In safety, they are applied as anti-scorching devices and sprinklers against fire and other types of sensors [ 1 , 3 ]. Future applications foresee the use of these alloys in car engines and airplanes and electric generators that use the mechanical energy resulting from the shape transformations; springs for engine cooling systems, carburetor and engine lubrication controls [ 1 , 32 ]. NiTi SMA have an excellent shape memory effect, allowing a wide range of applications from the aerospace industry to medical applications. However, they are more expensive than Cu-based SMA, both because of the high price of the alloying elements and because of their high reactivity with oxygen at higher temperatures, which makes their processing costly. In contrast, Cu-based SMA, besides being cheaper, have the advantage of being good thermal and electrical conductors. Besides that, Cu-based SMA are the most attractive because of the higher thermal stability against aging phenomena and stabilization [ 3 , 36 ].
One issue related to the applicability of the Cu-based SMA is the reduced deformation recovery of the and the brittle behavior at room temperature due to intergranular fracture [ 3 ]. The main reason for this is an abnormal and high elastic anisotropy, which leads to stress concentration within the grain boundary due to elastic and plastic incompatibilities between neighboring grains, particularly when large grains are present [ 3 , 13 , 14 , 37 ]. On the other hand, Cu-based SMA thermomechanically processed showed a significant reduction of grain size to 5 μm, resulting in a high fracture stress and a considerable ductility in tension of 1200 MPa and 10%, respectively [ 38 ]. Additions of Mn and Ti are also known to improve ductility and control grain size in Cu-based SMA [ 39 ] and the substitution of Al for Mn improves ductility without significant changes in the transformation temperature. In Cu–Al–Ni systems, the addition of small amounts of Ti, however, reduces grain size as a result of a decrease in the diffusion rate of the constituent atoms [ 3 ] and due to the hindering effect of Ti–rich nanosized precipitates formed during solidification that suppresses the grain growth [ 39 ]. Elements such as Zr and Si also have the effect of reducing grain size [ 40 ]. The mechanical properties of these multicomponent copper-based alloys have shown promise for future applications. The Cu-13.4Al-3.1Ni-0.06Si-0.58Zr (wt%) SMA, for example, shows tensile and deformation of fracture at around 830 MPa and 8%, respectively [ 40 ]. Cu-13.4Al-3.05Ni-0.24Ti-0.63Zr (wt%) alloys also showed high fracture stress of 903 MPa and a considerable fracture deformation of 8.6% in tension and the fracture mechanism changes from intergranular to transgranular [ 20 ]. Others Cu-based systems, such as Cu–Al–Mn were developed and they have shown better ductility [ 41 , 42 ] than others Cu-based SMA.
In Cu-based SMA the high temperature phase (austenite) is called β (normally cubic at high temperatures), sometimes referred to as the mother or parent phase in martensitic transformations [ 43 ]. Different types of martensites can be formed, depending on the composition and also on the stress level of the material [ 3 ]. Generally, the martensites are named as γ ʹ, β ʹʹ and β ʹ. In Cu-Al–Ni and Cu–Al–Zn the phase β becomes β ʹ (monoclinic), γ ʹ (orthorhombic) or a mixture β ʹ + γ ʹ depending on the amount of the alloying element [ 37 , 43 , 44 ]. The typical morphology of the β ʹ martensite is shown in Fig. 4 . The equilibrium phases that can be formed are called α and γ . These usually precipitate in the matrix after heat treatments, or even thermal cycles of austenite–martensite transformation. Thus, the knowledge of the precipitation temperature of these phases is important, since their presence can modify the functional properties of the alloy.
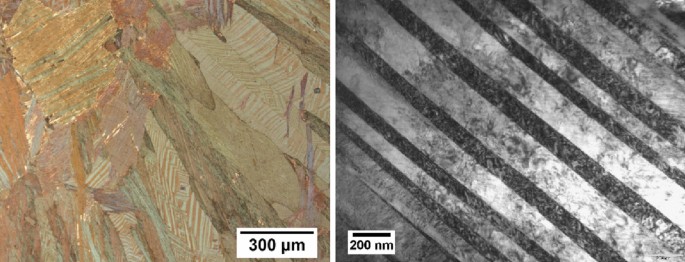
Typical β ʹ martensitic microstructure of a Cu–Al–Ni alloy.
Generally, for Cu-based SMA, before the martensitic transformation, the austenitic phase β undergoes two types of atomic ordering during cooling: the first arrangement, B2, is among the closest neighbors and is considered unavoidable even at extremely high cooling rates [ 43 ]. The other arrangement is called L2 1 or D0 3 , which refers to the neighboring atoms of the nearest neighbors. This can be avoided depending on the cooling rate adopted during processing. Thereby, the L2 1 arrangement becomes a stabilization mechanism of the austenite and martensite phases when subjected to thermal treatments [ 45 , 46 ]. Typically, the order B2 is in the first neighbors of the central atom. When the temperature is reached for the L2 1 arrangement, the outer atoms, are also ordered. There is usually a temperature range in which the ordinates B2 and L2 1 occur. Below the ordering temperatures, the ordering phenomena take place. However, it happens since there is enough driving force for the diffusion of the atoms, considering that this is a thermally activated phenomenon. The ordering of the alloys becomes a question of great importance in the martensitic transformations, since the change of the local composition and the structure of the material influences significantly the temperature range in which the phase transformation occurs [ 47 ]. Regarding the Cu–Al–Mn SMA system, similar sorting phenomena occur, since both alloying elements form solid solutions with Cu in the fractions used, and there is no influence of other phases [ 46 , 48 , 49 , 50 , 51 ]
The mechanical performance of the SMA depends heavily on the microstructure of the alloy, such as the phases present and their distribution, grain size and stability of the austenite and martensite phases [ 47 , 48 , 49 , 52 , 53 , 54 ]. These characteristics are derived from the history of thermomechanical processing history carried out on the material. Thus, factors linked to the functional properties of shape memory and mechanical properties will be influenced by the characteristics of the material, which will depend on the different processing parameters and treatments. Consequently, it is seen in the literature many different Cu-based SMA(with or without the addition of a fourth alloying element) obtained by various processes reported in the review by Dasgupta [ 55 ]. One can observe a great variation in the values of fracture stress and strains, as well as grain sizes.
The deformation mechanisms for SMA, which strongly influence the mechanical properties, will depend greatly on the temperature of the material and, consequently, on the predominant phases. Thus, the mechanical properties are fully tied to the functional properties. As one of the main requirements for the reversibility of a thermoelastic martensitic transformation is the absence of slip mediated plastic deformation [ 33 ], the stress required to detwinning or reorientation ( \({\sigma }_{\mathrm{dt}})\) should be lower than the yield stress. This is important in order to lower the possibility of plastic deformation or plastic residual stresses in the material, which are deleterious to the SME. In view of this, along with the mechanical properties, the factors related to the functional properties such as hysteresis, transformation temperatures, damping capacity, recovery stress will be fully tied to the thermomechanical state of the material and its microstructure/composition. The thermomechanical condition affects those functional properties, by helping or impairing the phase transformation to occur. Consequently, there is a great variation in the value of these properties due to the combination of all these factors. Thus, the transformation temperatures in Cu–Al–Ni SMA can range from − 200 to 200 °C and their thermal hysteresis from 20 to 40 °C. The specific damping capacity, which takes into account the energy dissipated by the supplied energy, is between 10 and 20% [ 32 ].
Recent developments of Cu-based SMA
New processing routes of cu-based shape memory alloys (sma).
Some innovative processing routes have been recently proposed to process Cu-based SMA with improved functional and/or mechanical properties, exploring advanced casting methods [ 56 , 57 ], powder metallurgy [ 57 ], new heat treatments[ 4 ], additive manufacturing[ 5 ], severe plastic deformation [ 58 ] or methods to obtain functionally graded materials[ 59 ].
Advanced casting methods have long been used to obtain Cu-based SMA single crystals, which show better fracture strain and larger superelasticity than polycrystalline alloys as a result of the absence of high angle grain boundaries [ 24 ]. Superelasticity up to 10% was obtained by Otsuka et al. [ 60 ] for a Cu–Al–Ni SMA fabricated by the Bridgeman method. A new and simpler method was recently proposed to obtain large single-crystal bars using cyclic heat treatment (CHT) [ 4 ]. CHT between 500 and 900 °C was applied to accelerate abnormal grain growth (AGG) in a Cu–Al–Mn SMA. Subgrains formed in association with α -phase precipitation during the cyclic heat treatment promotes AGG, which gives rise to a single grain structure after an annealing step. As a result, excellent superelasticity up to 5% was obtained in a 15.4 mm diameter and 682 mm length Cu–Al–Mn single-crystal bar [ 61 ]. SMA single crystals of small size have also shown astonish damping behavior, with the capacity to reduce the amplitude of mechanical oscillations by the dissipation of energy. Juan et al. [ 62 ] fabricated nano- and micropillars of single crystals Cu–Al–Ni SMA by Focused Ion Beam (FIB) and they demonstrated that these pillars exhibit a damping behavior significantly better than other bulk SMA, which make them very attractive to be used in Micro-Electro-Mechanical Systems (MEMS) and other nano and microscale devices. They measured the damping capacity through multiple-cycle nano-compression tests, with the dissipated energy per cycle being represented by the hysteresis loop during the stress–strain cycle [ 62 ].
The grain boundary of Cu-based SMA has been also engineered by Ueland et al.[ 24 ]. They demonstrated that the formation of a bamboo-like oligocrystalline structure in a Cu–Al–Ni wire also shows significant improvement in fracture strain and larger superelastic strain, with intermediate values between poly- and single-crystalline structures (Fig. 5 ). A new direct continuous casting method was proposed to develop microfibers of Cu-based SMA with this oligocrystalline structure[ 56 ]. It was produced over meter-long microfibers, which exhibit a large superelastic strain above 8% [ 56 ].
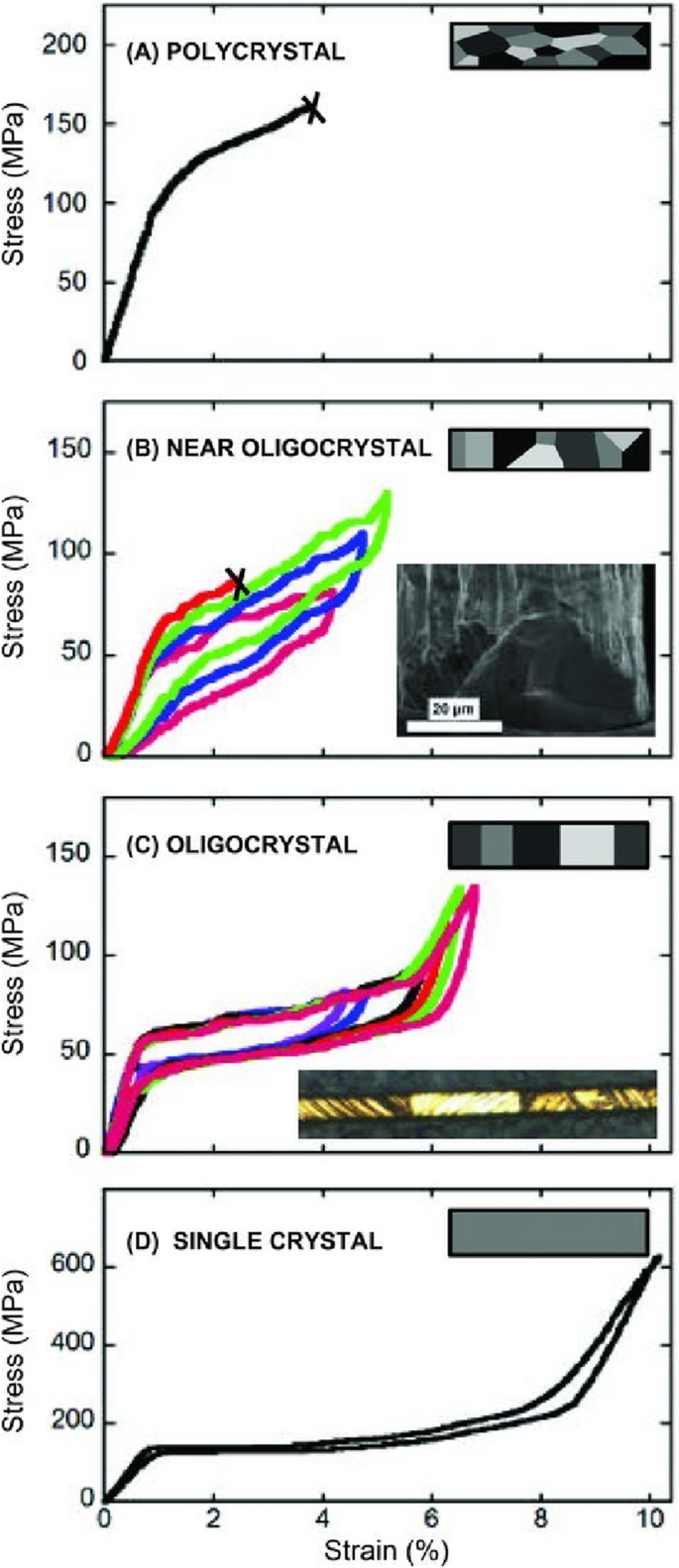
Reprinted from Ueland et al. [ 24 ]. Copyright (2012) by permission from Wiley.
Stress–strain curves of a polycrystalline Cu-14.1Al-4.2Ni, b near oligocrystalline Cu-13.7Al-5Ni, c oligocrystalline Cu-13.7Al-5Ni, and d single-crystalline Cu-14Al-4Ni.7. The insets show the grain structure of each sample.
A recently proposed method to fabricate Cu–Al–Ni–Mn and Cu–Al–Ni–Mn–Zr SMA is spray forming [ 19 , 63 ]. The big advantage of this method is to obtain a homogeneous equiaxial microstructure, without segregation, which allows improving the mechanical properties. Near-net-shape parts with simple shapes as plates and tubes can be obtained by this method. Cava et al.[ 63 ] have shown that small addition of 0.5 at% Zr changes significantly the grain size of the spray-formed Cu–Al–Ni-MnSMA, decreasing the grain size from 135 to 29 µm. Additionally, precipitation of secondary phases during heat treatment allows tuning the transformation temperature, with the martensitic start transformation temperature varying from − 32 to 139 °C for isothermal heat treatments with temperature and time of 300 °C/1440 min and 850 °C/30 min, respectively.
Rapid solidification techniques as melt spinning [ 17 ], Cu-mold casting [ 64 ], and gas atomization [ 5 , 51 , 65 ] have also been used to produce Cu-based SMA. These methods apply a high cooling rate, which promotes microstructural refinement and improves the mechanical properties. Nevertheless, they have a limitation on the final shape and geometry that can be obtained. Laser-based additive manufacturing techniques as the Powder Bed Fusion (PBF) are also rapid solidification techniques with cooling rates varying from 10 2 to 10 5 K/s, depending on process parameters [ 66 ], which allows producing parts with complex shapes and geometries (Fig. 6 ). The Cu–Al–Ni–Mn [ 65 , 67 ], Cu–Al–Ni–Mn–Zr [ 64 ], and Cu–Al–Mn [ 68 ] SMA have been successfully fabricated by this technique. Parts with relative density higher than 99% and with a refined columnar microstructure were obtained, with grains aligned to the build direction[ 67 ]. This promotes the formation of highly anisotropic materials, with better mechanical properties (measured uniaxially at the build direction) than samples obtained using conventional processing methods. Yield strengths comparable to cast alloys have been obtained for Cu–Al–Ni–Mn and Cu–Al–Ni–Mn–Zr (≈ 200 MPa) but with a larger ductility in tension, as a result of the greater microstructural refinement[ 64 ]. Also using atomized powders, porous scaffolds of a Cu–Al–Ni–Mn–Nb alloy were developed by Láper et al. [ 69 ]. The structures were produced through freeze-drying and sintering. The samples presented a porous structure of around 58% in volume, with porous size around 20 μm. A good damping capacity was achieved, of around tg δ = 0.05. This parameter is commonly used to measure the damping capacity of materials. It is the ratio between the loss and storage modulus and was obtained using a dynamic mechanical analyzer (DMA).
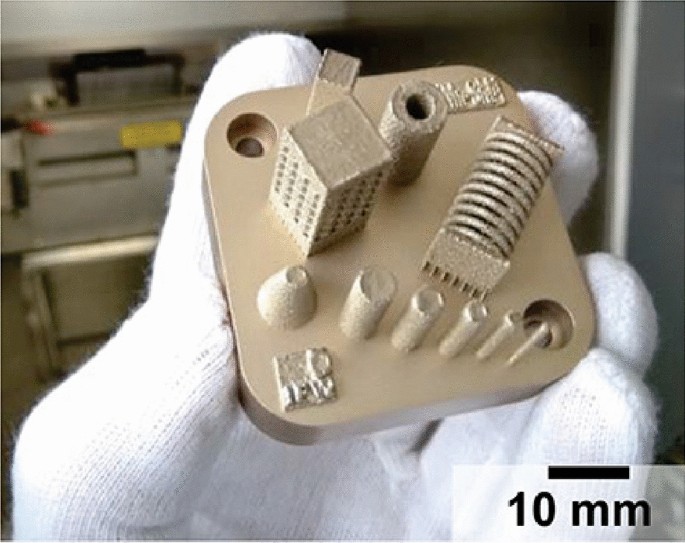
Reprinted from [ 68 ]. Copyright (2021) by permission from Elsevier.
Image of Cu–Al–Mn samples fabricated by the additive manufacturing method of Powder Bed Fusion.
Gustmann [ 70 ] showed that the use of a remelting step after the layers deposition during PBF can significantly improve the mechanical properties of these alloys. He also showed that a large superelasticity of up to 5% was obtained for a Cu–Al–Ni–Mn using this procedure. Additionally, Gustmann et al.[ 71 ] showed that the remelting step can increase the relative density of Cu-11.85Al-3.2Ni-3Mn shape-memory parts and control the grain size and transformation temperature in a broad range, depending on processing parameters. Laser surface remelting treatments have been also applied to improve the mechanical properties of a Cu-based SMA [ 72 ]. Da Silva et al. [ 72 ] applied this treatment to Cu–Al–Mn–Ni SMA plates and demonstrated an increase of up to 162 MPa in fracture stress, 2.2% in ductility, and 20.9 HV in microhardness when compared with the as-cast sample.
Many recent studies have been carried out applying severe plastic deformation (SPD) to modify the microstructure/phase formation, decrease grain size and improve the functional and mechanical properties of Cu-based shape memory alloys. López et al. [ 58 ] and Straumal et al. [ 73 ] have applied high-pressure torsion (HPT) in two different Cu–Al–Mn SMA. They observed that this treatment led to diffusive transformations (precipitation of α1-and γ1-phases) and promoted significant grain refinement, with grain sizes in the order of 100 nm. These changes modified the characteristics of these alloys with a clear shift of the martensitic transformation temperatures towards higher values together with a strong transformation peak broadening. Silva Junior and Mazzer [ 74 ] showed that using appropriate parameters during HPT of a CuAlNiMn SMA, an increase in strength can be obtained without sacrifice the shape recovery ratio. Another SPD method, equal channel angular pressing (ECAP), has been applied to a Cu–Al–Be–B SMA [ 75 ]. After ECAP, ultra-fine grains (~ 2 μm) were obtained but with a small fraction of martensite because of the stabilization of the austenitic phase. After heat treatment at 873 K and oil quenching, the grains coarsened to size up to 50 μm but the fraction of martensite increases, which improved the shape memory effect.
Very recently, several processing routes have been proposed to produce functionally graded (FG) shape memory alloys[ 59 ]. Differential heat treatments, additive manufacturing methods, multi-layer thin films, powder metallurgy, and surface diffusion have been used to create FG materials. An FG SMA may transform or deform over a wide range of stress or temperature, which can be interesting especially in actuating and sensing applications to improve the actuators and sensors controllability. The FG SMA can be obtained by microstructural, compositional, or geometrical gradients (Fig. 7 ) but these concepts have been applied only to TiNi-based SMA so far[ 59 ].
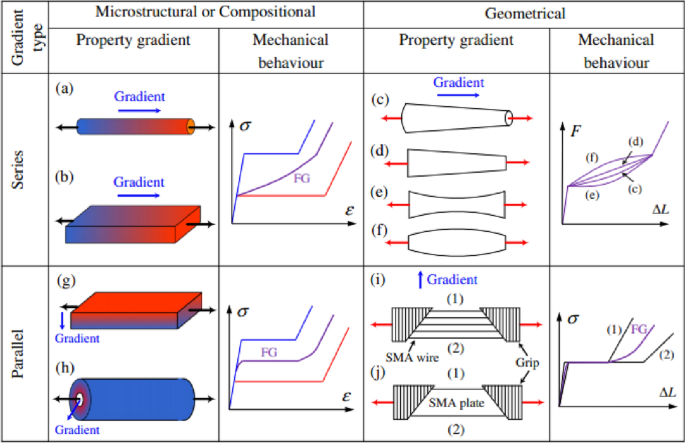
Reprinted from Shariat et al. [ 59 ]. Copyright (2017) by permission from Elsevier.
Designs of functionally graded (FG) NiTi-based SMA by microstructure or composition and geometrical gradient type and their corresponding mechanical behaviors.
Novel Cu-based SMA compositions
Until recently, most of the studied Cu-based SMA were classified in two main systems: Cu–Al–Ni and Cu–Zn–Al [ 55 ]. The former system has better thermal and microstructure stability and greater potential for high-temperature applications, while the second has a lower production cost and lower transformation temperatures. These Cu-SMA systems exhibit low ductility due to the strong tendency to intergranular fracture, which is attributed to the formation of a highly ordered structure and elastic anisotropy. This brittle behavior hinders the mechanical processing of these alloys, making it difficult even to shape them into sheets and wire, which is important for stents and guidewires applications [ 3 , 39 ].
In this context, new alloys have been developed aiming to overcome these issues. New compositions are suggested in the literature based on the systems previously presented, but not limited to them. Most of these new compositions are obtained based on ternary or quaternary alloying, which corresponds to one of the most employed methods to improve the properties of the Cu-based SMA. Several alloying elements are recommended in the literature and the influence of each specific alloying element will be addressed in the sequence for different Cu-based SMA systems.
The addition of Mn to Cu–Al–Ni SMA for example has substantially improved the ductility of these alloys without significantly modifying the martensitic transformation temperatures. The replacement of about 2% of Al by Mn lead to a suppression of the eutectoid reaction \({\beta }_{1} \to \alpha + {\gamma }_{2}\) [ 76 ], favoring the stabilization of the martensitic phase and decreasing the effect of intergranular embrittlement. Furthermore, for Cu–Al–Ni–Mn SMA, additions of a fifth alloying element have been proposed, such as Nb [ 7 , 77 ]. Small additions of Nb, of about 0.5wt%, in the Cu-11.45Al-3.2Ni-3Mn (wt%) SMA, resulted in a maximum shape recovery of 1% when subjected to stresses of 690 MPa, thus presenting a recovery ratio of 73%. In addition, the fracture stress for this SMA with Nb additions was about 1560 MPa. Another alloying element reported in the Cu–Al–Ni–Mn quaternary system is Zr [ 78 , 79 ]. An addition of 0.5 wt% of Zr was proposed, which leads to an increase in the martensitic transformation temperatures. Upon annealing, precipitation of the Y-phase ( \({\mathrm{Cu}}_{2}\mathrm{AlZr}\) ) in the grain boundaries was observed. These precipitates hamper grain growth and seem to produce an irregular phase transformation on heating [ 78 ]. Cu-11.35Al-3.2Ni-3Mn-0.5Zr (wt%) SMA processed by different routes presented a maximum tensile strength between 500 and 600 MPa and a fracture deformation between 5.4 and 7.2%. Under compression, fracture stress and strain of up 1200 MPa and 19.7% were obtained.
Low solubility quaternary elements play an important role in inhibiting grain growth and improving mechanical properties for Cu-based SMA. For instance, Boron is recognized as a refiner element that produces a significant improvement in mechanical properties [ 80 , 81 ]. A more recent study investigated the doping of B in the Cu-12Al-4Ni (wt%) SMA [ 41 ]. It was reported that the addition of boron in small amounts resulted in an increase in ductility of more than four times compared to the base alloy. Besides that, shape recovery was considerably increased with the addition of 0.3wt%B, from 75% (base alloy) to 95%. An increase in martensitic transformation temperatures was also reported with the doping of B.
Saud et al. [ 76 ] reported the addition of Co in the Cu-11.9Al-4Ni (wt%) SMA and observed the formation of a new \({\gamma }_{2}\) -phase. The size and volume fraction of \({\gamma }_{2}\) -phase increases as more Co is added to the alloy. The presence of the \({\gamma }_{2}\) -phase resulted in an increase in the transformation temperatures compared to the unmodified alloy. Furthermore, the addition of 1wt.%Co resulted in a maximum ductility of 7% and an increase in recovered deformation by the shape memory effect. Titanium addition in the Cu–Al–Ni SMA system has also been reported [ 82 , 83 ]. Similarly, as observed for Co, a new phase has been indexed with Ti additions, which is denoted as X-phase \({\left(\mathrm{Cu},\mathrm{Ni}\right)}_{2}\mathrm{AlTi})\) . With Ti additions, a sharp refinement of the grain size of about 70%, an increase in ductility from 1.65 to 3.2%, and an increase in the recovered deformation by the shape memory effect were obtained. Optimized properties, i.e. better mechanical properties and higher transformation temperatures were obtained for additions of 0.7 wt% Ti.
The addition of Ag nanoparticles has also been suggested in the literature [ 84 ]. The addition of 0.25 wt% Ag nanoparticles results in a microstructural modification with the formation of precipitates enriched in Ag. The volume fraction of the \({\gamma }_{1}^{^{\prime}}\) -phase is reduced and the morphology of the \({\beta }_{1}^{^{\prime}}\) -phase is refined with the addition of Ag nanoparticles. In addition, fracture strength and ductility were improved with the addition of Ag nanoparticles: values up to 420 MPa and 2.35% wereobtained respectively. Furthermore, the presence of Ag in these alloys increased the shape recovery ratio from 50 to 80%. The presence of this noble element also provided an improvement in corrosion resistance due to the formation of a surface protective layer of complex stoichiometry.
The addition of rare earth to Cu–Al–Ni alloys has also been reported recently [ 85 ]. The addition of Nd was performed to the Cu-13Al-4Ni (wt%) SMA and the grain size was reduced from the millimeter scale to a few hundred microns. The addition of Nd did not alter the phase formation for this SMA, which for this composition is composed of the β ʹ and γ ʹ martensitic phases. However, a second phase \(\left(\mathrm{Al},\mathrm{Ni}\right){\mathrm{Cu}}_{4}\mathrm{Nd}\) , with a hexagonal structure, can be formed if the Nd content is increased. For Nd contents of 0.5 wt%, an increase in fracture stress and ductility was obtained, from 580 MPa and 10.5% (base alloy) to 940 MPa and 18.3%, respectively. The presence of Nd also culminated in an increase in the superelasticity, with a reversible strain value of 6.8% being obtained for an Nd content of 0.2 wt% under the condition of pre-strain of 10% after heating. Additions of other rare earth elements were also reported in the literature, such as Gd, in which there was also high grain refining and improved mechanical properties [ 86 ].
Other several additions of elements were reported in the literature, such as Cu–Al–Ni -Hf [ 6 ], Cu–Al–Ni–Ta[ 87 ], Cu–Al–Ni–Fe[ 88 , 89 ], Cu–Al–Ni -Zn [ 11 ], Cu–Al–Ni–Cr [ 90 ], Cu–Al–Ni–Ti-Cr [ 91 ], Cu–Al–Ni–V[ 92 ] and Cu–Al–Ni–Sn [ 93 ].
More recently, the Cu–Al–Mn SMA system, which was first reported in 1995 by Kainuma et al. [ 9 , 94 ], has been receiving great attention in the literature. This is attributed to the recent development of metal alloy manufacturing processes that allow the production of these SMAs with adequate control of texture and grain size, enabling the achievement of superior superelastic properties than those of traditional Cu–Al–Ni and Cu–Al–Zn SMA systems [ 9 , 61 , 95 ]. Cu–Al–Mn SMA generally have an Al content of less than 18 at% and show excellent ductility and good shape memory effect mainly due to the low degree of ordering of their \(L{2}_{1}\) parent phase [ 9 , 68 , 94 ]. The low-temperature martensitic phases that can be found in these alloys are: \({\alpha }_{1}^{^{\prime}}\) (3R) observed for low Al contents, \({\beta }_{1}^{^{\prime}}\) (18R) for intermediate Al contents, and \({\gamma }_{1}^{^{\prime}}\) (2H) that is predominant in alloys with high Al contents [ 96 ]. Alloys of this system with Al content lower than 14 at% show two distinct austenitic phases: β (A2, disordered) and \({\upbeta }_{1}\) ( \({\mathrm{L}2}_{1}\) , ordered). Alloys with Al content between 16 and 17 at% Al only have the \({\upbeta }_{1}\) -phase, which results in improved shape memory properties [ 96 ]. This Al compositional range is generally used for the manufacture of the Cu–Al–Mn SMA.
The degree of ordering of Cu–Al–Mn SMA tends to decrease with the reduction of Al content, and because of this disorder, there is an increase in both ductility and cold workability for these alloys. As an example, it is possible to obtain tensile elongation values greater than 10% and cold workability greater than 60%, which corresponds to values higher than that found for the alloys of the Cu–Al–Zn and Cu–Al–Ni systems. Combined with the improved mechanical properties, Cu–Al–Mn can present shape memory properties similar to those reported for NiTi alloys. Superelasticity values of 7.5% have been reported for these alloys, which are slightly below those obtained for NiTi-based alloys (~ 8%) [ 97 ]. Superelasticity can be improved both by controlling grain size, where the increase in grain size generates an increase in superelasticity, as well as by texture introduction. Texture development is mainly achieved by thermomechanical deformation processes. Since the alloys of the Cu–Al–Ni and Cu–Al–Zn systems have low cold workability, the texture introduction during processing is quite difficult. In contrast, Cu–Al–Mn SMA, with higher ductility can be mechanically cold processed, and thus texture may be implemented in these alloys. The possibility of processing by cold deformation allows the development of low-cost SMA with shapes that would only be possible through special processing such as additive manufacturing [ 97 ]. These alloys can be cold deformed by several processes that include rolling, punching, and cutting. Applications of the Cu–Al–Mn SMA have been reported, as in the biomedical field where a small device in the shape of a plate of few centimeters long is used to relieve ingrown nails using the shape memory effect [ 97 , 98 ].
Researches have also been directed towards the development of alloys of the Cu–Al–Mn system with a smaller volumetric fraction of grain boundaries since the superelasticity is drastically increased with their reduction [ 97 ]. Great effort has been made to manufacture large-scale single-crystalline parts of Cu–Al–Mn SMA, which can be implemented in the civil engineering and architecture field.
Cu–Al–Mn has also been studied aiming improvement of other typical properties of SMA, such as its high damping capacity. This property is very important for applications where vibration and noise attenuation of engineering structures is required. Due to their thermoelastic properties, SMA are excellent candidates for this type of application as they have a high damping capacity due to the movement of interfaces during the martensitic transformation [ 99 ]. The damping capacity of structures can be usually improved by introducing pores during processing. Recent studies [ 100 ] have reported the fabrication of Cu-11.9Al-2.5Mn (wt%) SMA with an interconnected pore structure to obtain a high damping capacity since porosity acts as energy scattering centers.
Also aiming to improve the damping properties, Cu–Al–Mn/polystyrene composites were manufactured via sintering-dissolution and sol–gel methods. Porous alloys, which already had excellent compressive energy absorption and damping properties, had their properties improved when prepared in a composite form. This is attributed to the impediment effect on the pore collapse by polystyrene and the overlapping of multiple sources of damping.
Alloying can also be used to improve the properties of the Cu–Al–Mn SMA. Several distinct elements have been investigated in the literature. Sampath et al. [ 12 ] reported the addition of boron and observed an effective grain refining of about 80%, with a consequent increase in phase transformation temperatures. Enhanced grain refinement was also obtained using CuZr inoculants in an amount of 0.9 wt% [ 101 ]. The use of these inoculants resulted in a reduction in grain size to 37 μm from an initial grain size of 1050 μm. This grain size reduction increased mechanical strength and elongation, which were attributed to the higher grain boundaries volumetric fraction that hinder dislocations movement. Recent studies show that inoculation using rare earth increases the ductility of the Cu–Al–Mn SMA and may avoid intergranular failure. Lu et al. [ 102 ] studied Ce doping and obtained an increase in both ductility, mechanical strength, and damping capacity. Other compositions that can be found in the literature for the Cu–Al–Mn system are those with the addition of Ag and Nb [ 103 ].
Another recently reported SMA system is the one based on the Cu-Al–Fe–Mn, whose composition range is Cu-(12.2–12.9)Al-(3.8–4.3)Fe-(5.5–6.6)Mn (wt%) [ 10 ]. These alloys show an instantaneous shape memory effect during heating, which results from a recovery mechanism slightly different from that usually reported for conventional SMA. This mechanism consists of the recovery of the 2H martensitic phase ( \({\gamma }_{1}^{{^{\prime}}{^{\prime}}}\) ) that was previously stabilized during the pre-strain condition. During the deformation of the sample in the superelastic regime, above the temperature \({A}_{\mathrm{f}}\) (austenitic transformation final temperature), the martensitic phase is stress-induced from the parent phase ( \(L{2}_{1}\) ). With load removal, the martensitic phase is stabilized in a metastable condition since the temperatures are significantly above \({M}_{\mathrm{s}}\) (martensitic transformation start temperature). During sample heating, this retained martensitic phase instantly transforms into the parent phase, and consequently, the sample immediately recovers its shape. These alloys are considered to be highly temperature-sensitive, with a low hysteresis during shape recovery (~ 25 °C), which results in an instantaneous recovery speed. In this way, the shape recovery process can be completed instantly, accompanied by an unusual “jump” of the sample. This high-temperature sensitivity can be used for high-performance sensor applications. Furthermore, these new SMA present a shape recovery of about 9% that corresponds to a relatively high value for Cu-based SMA.
New Cu–Al–Fe–Mn SMA has been recently suggested in the literature with the addition of a fifth element with low solubility in Cu, such as Nb and Zr [ 104 ]. Due to their low solubility, these alloying elements, when present, tend to form second phases that can assist the martensite stabilization after unloading. The addition of these elements produces a microstructure at room temperature consisting of the parent phase \(\mathrm{L}{2}_{1}-{\mathrm{Cu}}_{2}\mathrm{AlMn}\) , small fractions of the 2H martensitic phase, and fine second β -phases ((Fe, Al, Zr) or (Fe, Al, Nb)). The fraction of the β phase increases with the addition of Zr or Nb. Again, 2H martensite is stabilized during the pre-strain condition, in this case even for deformations smaller than 6%. Stabilization of the martensitic phase is attributed to the inhibition of the movement of the habit planes during unloading due to the presence of precipitates and dislocations in the microstructure. This stabilization can be reversed on heating through the shape memory effect (SME). The maximum SME value obtained was 4.4% for Nb and Zr contents of 1% and 2%, respectively. More recent studies related to the Cu–Al–Fe–Mn system can be found in the literature [ 105 , 106 , 107 ].
Another system of Cu-based SMA is Cu–Al–Be, which was discovered in 1982. Despite not being a recent Cu-based SMA system, there has been a growing number of recent works about new compositions [ 8 ]. Cu–Al–Be consists of a family of Cu-based SMA with compositions close to the eutectoid point which has good thermal stability. These SMA present, in addition to shape memory and superelasticity effects, other interesting properties, such as good corrosion resistance and good mechanical strength. There is a particular technological interest for these SMA for applications at low and intermediate temperatures. The addition of small amounts of Be drastically reduces the phase transformation temperatures, thus allowing superelasticity to occur at very low temperatures. Some empirical equations have been proposed to determine the martensitic transformation temperatures in Cu–Al–Be alloys based on their composition [ 10 , 108 ]. Hence, these alloys can be used in a wide range of temperatures and can even be considered for cryogenic applications.
Recently studies have been carried out to modify the properties and characteristics of these SMA by alloying. Narasimha et al. [ 109 ] studied the addition of Zr in the Cu-11.42Al-0.45Be alloy (wt%) and observed a reduction in grain size of 89%, with a consequent increase in ductility and mechanical strength. Furthermore, the addition of up to 0.3 wt% Zr did not change the shape recovery ratio, despite an increase in martensitic transformation temperatures. Melo et al. [ 110 , 111 ] produced Cu–Al–Be SMA with additions of Ni and Nb and observed a large reduction in grain size, as well as an increase in mechanical strength and ductility. Candido et al. [ 112 ] studied the addition of Cr in the Cu–Al–Be system and also observed a marked reduction in grain size. Additions of 0.2 wt% Cr led to higher mechanical strengths and to lower martensitic transformation temperatures, allowing the application of these alloys at even lower temperatures Rare earth elements such as gadolinium have also been added to these SMA at low levels between 0.05 and 0.15 wt%. Again, large grain refinement was obtained, from 463 µm in the Gd-free condition to 81 µm, which corresponds to a reduction in grain size of about 82%. Mechanical strength and ductility were increased with the addition of 0.08 wt% Gd. However, a reduction of the shape recovery ratio from 97 to 65% was also observed.
More recently, boron doping in Cu–Al–Be SMA has been proposed [ 113 ]. Boron doping is also used for the refining of Cu–Al–Mn SMA, as previously discussed. The addition of 0.15 wt% B in the Cu-11.5Al-0.57Be SMA produced an increase in the ultimate tensile strength to 744 MPa and an increase in ductility to 29%. Besides that, fracture morphology changed from intergranular to transgranular with B doping. A maximum superelasticity of 4% was obtained by B doping, which is well suited to use as dampers in seismic applications.
The addition of alloying elements to the Cu–Al–Be SMA must be carried out with caution since large concentrations of added elements can exceed the solubility limit and generate the precipitation of second phases, which modify the shape memory effects [ 114 ]. With the formation of precipitates, the fraction of Be and Al in the β-phase matrix is probably reduced, which alters the martensitic transformation temperatures that are strongly dependent on the chemical composition, mainly on Be content [ 114 ]. Variations of about 0.1 wt% Be can result in a modification of about 90 °C [ 115 ].
The most important properties (transformation temperatures range, recovery strain, superelasticity, fracture strain, and stress, structure/grain size) and processing routes for the alloys discussed in this manuscript are summarized in Table 1
Recent applications
Besides the well known SMA applications, Cu-based shape memory alloys have been attracting attentions due to the possibility of applying them at higher temperatures than others SMA systems. Moreover, the use of their phase transformation energies makes possible the application in energy/heat harvesting materials, such as elastocaloric alloys. The next sections will be dedicated to discussing these topics.
Elastocaloric effect (eC): The promising Cu–Zn-Al and Cu–Al–Mn systems
One recent important application of Cu-based SMA is related to solid-state cooling by the elastocaloric effect (eC), exploiting the latent heat (∆H) absorbed from the superelastic phase transformation. As well known for first order transformation, the martensitic reverse transformation in thermoelastic SMA releases heat during the direct transformation (exothermic phase transformation from austenite to martensite) and absorbs heat during the reverse transformation (endothermic phase transformation from martensite to austenite). The elastocaloric cycle consists of loading and unloading a SMA in the superelastic region, as shown in Fig. 8 . As a first-order phase transformation from a high-symmetry phase (austenite) to a low-symmetry phase (martensite), the entropy change involves the release or absorption of heat. This change in entropy could result in vaiation of temperature, which allows cooling around 10 K for Cu-based alloys [ 116 ], as will be further detailed in the next sections. The coefficient of performance (COP) is ratio between the absorbed energy ∆H and the energy spent in the elastocaloric cycle and is considered an indicator for eC in SMA. For certain alloys, the eC efficiency is comparable to the Carnot process in the same thermal operation [ 117 ].
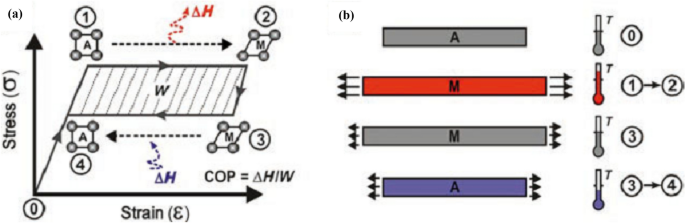
Reprinted from Frenzel et al. [ 118 ]. Copyright (2018) by permission from Springer Nature.
Scheme representing the elastocaloric cycle in a SMA. (a) Four steps of loading and unloading of a superelastic alloy. The latent heat of transformation is released from 1 to 2 and absorbed from 3 to 4. (b) Heat effect of each elastocaloric step.
Generally, the four steps elastocaloric cycle consists firstly in the mechanical loading of the alloy, which induces the direct martensitic transformation. Heat is released by the material while the transformation occurs, which represents the second step. This release heat is exchanged with a heat sink by solid or liquid conduction.. The third step is the adiabatic unloading of the alloy, which allows the material to undergo the reverse transformation and absorbs heat from the environment, which corresponds to the last step [ 116 , 119 , 120 , 121 , 122 , 123 , 124 ]. Ideally, the fourth step must comprise an adiabatic transformation, so the system can be cooled by the absorption of heat by the material. Many different prototypes have been developed with the intention to obtain the best efficiency with the cooling capacity using the eC superelastic SMA [ 118 , 119 , 120 , 122 , 125 ]. Parallel to the prototypes studies, many research groups have been developing and studying new elastocaloric alloys. The main target features of these materials is to improve the latent heat of the martensitic transformation without compromise the functionality of the alloy, such as the structural and functional fatigue resistance, which is related to hysteresis and plastic deformation mediated by slip [ 125 , 126 , 127 ].
Aiming the improvement of eC in SMA, the change in the transformation entropy (∆ S t ) under adiabatic conditions should be maximized, so the latent heat of martensitic phase transformation (∆ H t ) would also be maximized, since ∆ S t = ∆ H t / T 0 or ∆ S t = −(d σ t /d T ) ε t , where T 0 is the equilibrium transformation temperature, d σ t /d T is the Clausius–Clapeyron slope and ε t is the theoretical transformation strain. Thus, the theoretical temperature change is ∆ T th = −∆ S t ( T / C p ), where C p is the specific heat capacity and T is the working temperature [ 126 , 127 ]. Based on this approach, an alloy with higher entropy change will perform a better cooling capacity. This fact does not strictly happen in practice because during the thermoelastic martensite transformation, hysteretic losses and plastic deformation impairs the full reversibility of the phase transformation, which makes ∆ T th practically unachievable [ 33 ]. In order to access the eC parameters in practice, direct and indirect experimental methods are applied. Normally, the indirect experimental methodology for the temperature changes predictions can be carried out indirectly by DSC (Differential Scanning Calorimetry) through the computation of ∆ H t , and/or by the Clausius–Clapeyron slope through the use of uniaxial tests and the calculation of the start stress for stress-induced martensite at various temperatures. Direct measurements of the temperatures changes have been performed by the combination of Digital Image Correlation (DIC) and infrared imaging (IR) in uniaxial superelastic tests [ 126 , 127 , 128 ], as can be seen in Fig. 9 .
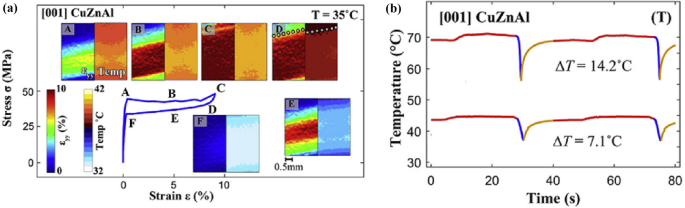
Adapted from Wu et al. [ 126 ]. Copyright (2017) by permission from Elsevier.
(a) Stress–strain diagram of a Cu–Al–Zn superelastic alloy at 35 °C. The inset figures from A to E show the strain measured by the DIC and the temperature measured by infrared camera. (b) Temperature variation versus time during the loading and adiabatic unloading of the same alloy.
The first significant elastocaloric effect was reported on a Cu–Zn–Al single crystal by Bonnot et al. [ 129 ] with a transition entropy-change of ∆ S t = 1.37 ± 0.10 J/mol K with a maximum temperature change of 14 K under a adiabatic condition. Figure 10 shows the stress and strain-induced entropy-change for this alloy at distinct temperatures in a different range of stress (a) and strain (b).This behavior shows that the maximum entropy change ∆ S generally remains unchanged over a broad range of temperatures, representing a promising elastocaloric effect.
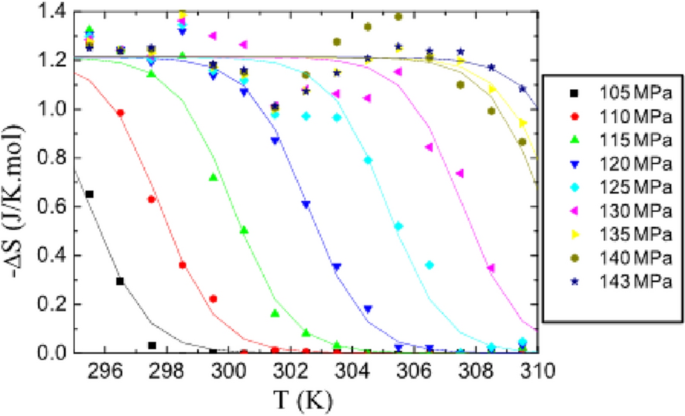
Reprinted from Bonnot et al. [ 129 ]. Copyright (2008) by permission from American Physical Society.
Entropy variation DS during the phase transformation as a function of the temperature or various stress levels of a Cu–Zn–Al SMA.
In the last decade, many other authors also reported a good elastocaloric effect in the Cu–Al–Zn system [ 120 , 126 , 130 , 131 , 132 , 133 , 134 ]. The main findings are related to the adiabatic change temperature ∆ T , which ranges around 2 and 20 K, depending on the applied stress, temperature, composition, and grain structures. Also, the temperature span where the eC takes place in Cu–Al–Zn is generally higher than in other types of caloric materials. One of the most important discovery in this regard was done by Mañosa et al. [ 132 ] in a polycrystalline Cu 68 Zn 16 Al l6 (at%) SMA, which has a working temperature span of 130 K. This large temperature span implicates that the entropy change ∆ S and the temperature change ∆T remain over a broad range of temperature, which is a pre-requisite for a good elastocaloric material. Another important results were reported by Gràcia-Condal et al. [ 131 ] in a Cu 68.3 Zn 14.2 Al 17.5 (at%) single-crystal. They found that the isothermal stress-driven ∆ S saturates at 22 J K −1 kg −1 for an applied compression change of 25 MPa and that the adiabatic temperature change ∆ T increases linearly with compression and is expected to saturate at 15 K at a compression change of 50 Mpa, representing reasonable results for the elastocaloric effects at feasible conditions.
Other promising Cu-based SMA for elastocaloric applications are Cu–Al–Mn SMA [ 135 , 136 , 137 , 138 , 139 , 140 , 141 ]. In a study published by Xu et al. [ 138 ] it was reported an adiabatic temperature change ∆ T of about 13 K at a temperature range of 100 K in a columnar-grained Cu 71.5 Al 17.5 Mn 11 (at%) SMA. A bamboo-grained Cu 71.1 Al 17.2 Mn 11.7 (at%) SMA microwires with ∆ T = 11.9 K under a maximum stress of 400 MPa was reported by Yuan et al. [ 135 ]. Also, the same research group studied elastocaloric properties of a heterogenous grained structure Cu 71.1 Al 17.2 Mn 11.7 (at%) wire [ 139 ]. They reported that the local temperature along the wire’s axes varied depending on the grains structure during the elastocaloric cycle. Single-crystals and oligocrystals presented a higher temperature change ∆ T due to larger transformation strain for a given applied stress. Polycrystals and single-crystals presented more stable attenuation of ∆ T after many elastocaloric cycles in comparison with the other grains structures. These results represent an important step in the understanding of polycrystalline elastocaloric properties.
During the last decade, the development of Cu-based SMA with larger ductility and with transformation temperatures over a large range of temperature have opened an opportunity to develop new caloric materials. The key motivations for researchers are the innumerous challenges to be solved, involving the thermoelastic transformation in Cu-based SMA, the energies involved in the transformation, the role of microstructure, the influence of plastic deformation and the relation between the theoretical temperature change and the real temperature change in an elastocaloric cycle. In the meantime, researchers are also using computational methods using the known thermodynamics properties of these alloys in order to design new and optimized composition for elastocaloric applications [ 142 ]. Details of the thermodynamics related to elastocaloric effects can be found in [ 120 , 133 , 134 , 143 ]. One specific review about other elastocaloric systems can be found in [ 144 ].
High temperature shape memory alloys (HTSMA) – Cu-Al-X systems
Another promising application of Cu–Al SMA englobes the high temperature shape memory alloys (HTSMA), which operates above 373 K. Cu–Al SMA have higher transformation temperatures than other Cu-based systems [ 7 , 77 , 145 , 146 ]. Some Cu–Al–Ni single-crystals presents recoverable strain of about 17% and shape memory effect above 473 K, which is an outstanding behavior at high temperatures. Normally, Cu–Al alloys are more likely to be applied as HTSMA due to their higher transformation temperatures and higher microstructure stability than Cu–Zn alloys. On the other hand, the main disadvantage of Cu–Al alloys for high temperature applications is the possibility of structural ordering and equilibrium phases’ precipitation. As pointed out by Ma et al. [ 147 ], although equilibrium phases can be avoided by quenching, this process generates vacancies and prevents the ordering of the alloy. When operating above 373 K, the ordering process would take place and change the transformation temperatures after thermal cycles [ 7 , 51 ]. One possible solution to mitigate the effect of transformation temperatures shifting due to ordering process is to age the alloy until complete ordering at a temperature that does not activate the precipitation of the equilibrium phase. Depending on the composition, both temperatures are overlapped so this solution becomes impossible. This is a challenge that motivates researchers in the survey for new compositions of Cu-based SMA. Also, the mechanisms that act at high temperatures, i.e. above 373 K are subjects of interest.
Many other compositions that operates with good superelasticity and shape memory effect above 373 K have been developed during the past decades. Cu–Al–Nb [ 146 ], Cu–Al–Ni [ 147 ], Cu–Al–Ni–V [ 92 ], Cu–Al–Ni–Mn [ 19 , 47 ], Cu–Al–Ni–Mn–Nb [ 7 ] are exemples of such systems.
Future perspectives and conclusion remarks
The recent revival of interest in Cu-based SMA has been motivated by the emerging of novel processing routes, compositions, as well as new methods of characterization and technological applications that demand new SMA.
Considering the processing methods, there are still many possibilities of studies about additive manufacturing in Cu-based SMA. Different additive manufacturing methods as direct metal laser sintering, direct energy deposition, metal binder jetting, were still not explored to fabricate these alloys. Different microstructure and phase formation could be obtained, which can promote different functional and mechanical properties. A systematic investigation of the effect of different scanning strategies and remelting processes during additive manufacturing on the mechanical and functional properties of these alloys as superelasticity, shape recovery, and transformation temperatures, still needs to be carried out. Severe plastic deformation could also be applied to produced layered Cu-based SMA with advanced properties, tunning the transformation temperatures and shape recovery according to application. Functionally graded (FG) Cu-based SMA were also not investigated so far. None of the methods used to produce FG materials as differential heat treatments, additive manufacturing methods, multi-layer thin films, powder metallurgy, and surface diffusion have been used for Cu-based SMA so far. These FG materials are very promising to be applied as new sensors and actuators with higher controllability.
In the regarding of SMA compositions covered in this review, it is expected three main approaches to the development of new compositions in a future trend.The first approach is based on the widely used alloying method, which was already described in the present review. This method is performed with the aim to modify and adjust the microstructure and properties of SMA. One example of this approach is the recently reported Cu–Al–Fe–Mn system, in which a systematic study of the implementation of a fifth alloying element is expected. This procedure has already been started, as discussed previously; however, several alloying elements may be used to modify the microstructure and properties of the SMA of this system. Since this SMA system has a slightly different shape memory effect mechanism, new behaviors can be found by adding another alloying element. The second approach is related to the recent progress and development in manufacturing processes for metallic materials. The improvement of the various fabrication methods that were covered in this review, as well as the creation of new methods, will allow the development of novel SMA with improved properties. This trend of development of novel compositions related to technological advances in manufacturing is already observed for the Cu–Al–Mn system as discussed in this review. This approach can also be extrapolated to the well know SMA compositions. In addition, for Cu–Al–Fe–Mn SMA, the use of different (and new) manufacturing processes may allow different compositions to present the same SME mechanism. The third approach to the development of novel compositions is based on the recent advances in machine learning methods. New compositions can be obtained from a database of different properties of SMA, which, combined with mathematical and thermodynamic formulations, can lead to obtaining not only new SMA compositions but also new SMA systems with optimized microstructures and properties.
One important demand for advanced alloys, including SMA, is for energy applications. Followed by this trend, Cu-based SMA have been considered as high potential materials to be explored in elastocaloric applications. Towards the development of devices with a higher refrigeration capacity, the study of the phase transformation in Cu-based SMA and the mechanisms that affect their latent heat of transformation play an important role in eC. In this context, the key challenge for Cu-based SMA is to find ideal composition and microstructure, aiming to explore the maximum of the transformation energies without implicating in their functionality. Considering that a higher latent heat of transformation generally implies in a higher hysteresis, functional damage after the thermal or stress cycles still limits their application.
In the view of the present article, the authors conclude that very interesting and innovative researches have been performed in Cu-based SMA. With the development of new technologies demanding new materials and functionalities, this class of alloys become important candidates and many researches should still address this topic in the near future.
Data availability
All data generated or analysed during this study are included in this published article.
J.M. Jani, M. Leary, A. Subic, M.A. Gibson, Mater. Des. 56 , 1078–1113 (2014)
Article Google Scholar
K. Otsuka, X. Ren, Intermetallics 41 , 511–528 (1999)
Google Scholar
K. Otsuka, C.M. Wayman, Shape Memory Materials (Cambridge University Press, Cambridge, 1998)
T. Omori, T. Kusama, S. Kawata, I. Ohnuma, Y. Sutou, Y. Araki, K. Ishida, R. Kainuma, Science 341 , 1500 (2013)
CAS Google Scholar
E.M. Mazzer, C.S. Kiminami, P. Gargarella, R.D. Cava, L.A. Basilio, C. Bolfarini, W.J. Botta, J. Eckert, T. Gustmann, S. Pauly, Mater. Sci. Forum 802 , 343–348 (2014)
I.N. Qader, M. Kök, F. Dağdelen, Physica B 553 , 1–5 (2019)
F.R. Milhorato, E.M. Mazzer, Mater. Sci. Eng. A 753 , 232–237 (2019)
J.P. Oliveira, Z. Zeng, S. Berveiller, D. Bouscaud, F.M.B. Fernandes, R.M. Miranda, N. Zhou, Mater. Des. 148 , 145–152 (2018)
R. Kainuma, S. Takahashi, K. Ishida, J. Phys. 5 , 8–961 (2014)
S. Yang, T. Omori, C. Wang, Y. Liu, M. Nagasako, J. Ruan, R. Kainuma, K. Ishida, X. Liu, Sci. Rep. 6 , 21754 (2016)
R. Dasgupta, A.K. Jain, P. Kumar, S. Hussein, A. Pandey, J. Market. Res. 3 , 264–273 (2014)
V. Sampath, U.S. Mallik, European Symposium on Martensitic Transformations (ESOMAT), 2009.
Y. Chen, C.A. Schuh, Acta Mater. 59 , 537–553 (2011)
S.M. Ueland, C.A. Schuh, Acta Mater. 60 , 282–292 (2012)
S.K. Vajpai, R.K. Dube, S. Sangal, Mater. Sci. Eng. A 570 , 32–42 (2013)
S.K. Vajpai, R.K. Dube, S. Sangal, Mater. Sci. Eng. A 529 , 378–387 (2011)
G. Lojen, I. Anžel, A. Kneissl, A. Križman, E. Unterweger, B. Kosec, M. Bizjak, J. Mater. Process. Technol. 162–163 , 220–229 (2005)
V. Sampath, Smart Mater. Struct. 14 , S253–S260 (2005)
R.D. Cava, C. Bolfarini, C.S. Kiminami, E.M. Mazzer, W.J.B. Filho, P. Gargarella, J. Eckert, J. Alloys Compd. 615 , S602–S606 (2014)
J. Dutkiewicz, T. Czeppe, J. Morgiel, Mater. Sci. Eng. A 273 , 703–707 (1999)
J. Lelatko, H. Morawiec, Mater. Sci. Eng. A 481–482 , 684–687 (2008)
J. Lelatko, H. Morawiec, Mater. Chem. Phys. 81 , 472–475 (2003)
M.A. Morris, Acta Metall. 40 , 1573–1586 (1992)
S.M. Ueland, Y. Chen, C.A. Schuh, Adv. Func. Mater. 22 , 2094–2099 (2012)
S.M. Ueland, Departement of materials science and engineering, Massachusetts institute os technology 2013.
S.M. Ueland, C.A. Schuh, Acta Mater. 61 , 5618–5625 (2013)
D.C. Lagoudas, Shape Memory Alloys - Modeling and Engineering Applications (Springer, New York, 2008)
K. Bhattacharya, S. Conti, G. Zanzotto, J. Zimmer, Nature 40 , 55–59 (2004)
G.B. Olson, M. Cohen, Scr. Metall. 9 , 1247–1254 (1975)
R.J. Salzbrenner, M. Cohen, Acta Metall. 27 , 739–748 (1979)
H. Kato, Y. Yasuda, K. Sasaki, Acta Mater. 59 , 3955–3964 (2011)
L. Sun, W.M. Huang, Z. Ding, Y. Zhao, C.C. Wang, H. Purnawali, C. Tang, Mater. Des. 33 , 577–640 (2012)
P. Chowdhury, H. Sehitoglu, Prog. Mater Sci. 85 , 1–42 (2017)
A. Biesiekierski, J. Wang, M.A. Gepreel, C. Wen, Acta Biomater. 8 , 1661–1669 (2012)
W. Huang, Mater. Des. 23 , 11–19 (2002)
A. Aydogdu, Y. Aydogdu, O. Adiguzel, J. Mater. Process. Technol. 153–154 , 164–169 (2004)
V. Recarte, R.B. Pérez-Sáez, E.H. Bocanegra, M.L. Nó, J. San-Juan, Mater. Sci. Eng. A 273 , 380–384 (1999)
V. Novák, P. Šittner, N. Zárubová, Mater. Sci. Eng. A A234–236 , 414–417 (1997)
K. Otsuka, H. Sakamoto, K. Shimizu, Acta Metall. 27 , 585–601 (1979)
T. Waitz, T. Antretter, F.D. Fischer, H.P. Karnthaler, Mater. Sci. Technol. 24 , 934–940 (2008)
S. Hussain, A. Pandey, R. Dasgupta, Mater. Lett. 240 , 157–160 (2019)
Y. Sutou, N. Koeda, T. Omori, R. Kainuma, K. Ishida, Acta Mater. 57 , 5759–5770 (2009)
V. Recarte, R.B. Pérez-Sáez, E.H. Bocanegra, M.L. Nó, J. San-Juan, Metall. Mater. Trans. A. 33A , 2581–2591 (2002)
J.I. Pérez-Landazábal, V. Recarte, V. Sánchez-Alarcos, J. Phys. 78 , 4223–4236 (2005)
J.I. Pérez-Landazábal, V. Recarte, R.B. Pérez-Sáez, M.L. Nó, J. Campo, J.S. Juan, A.P. Letters, Appl. Phys. Lett. 81 , 1794 (2002)
S. Hussain, P. Kumar, A.K. Jain, A. Pandey, R. Dasgupta, Mater. Perform. Charact. 4 , 17 (2015)
E.M. Mazzer, P. Gargarella, R.D. Cava, C. Bolfarini, M. Galano, C.S. Kiminami, J. Alloys. Compd. 723 , 841–849 (2017)
T. Omori, N. Koeda, Y. Sutou, R. Kainuma, K. Ishida, Mater. Trans. 48 , 2914–2918 (2007)
Y. Sutou, N. Koeda, T. Omori, R. Kainuma, K. Ishida, Acta Mater. 57 , 5748–5758 (2009)
J. Fornell, N. Tuncer, C.A. Schuh, J. Alloys. Compd. 693 , 1205–1213 (2017)
E.M. Mazzer, C.S. Kiminami, C. Bolfarini, R.D. Cava, W.J. Botta, P. Gargarella, Thermochim. Acta 608 , 1–6 (2015)
J. Rodríguez-Aseguinolaza, I. Ruiz-Larrea, M.L. Nó, A. López-Echarri, E.H. Bocanegra, J.S. Juan, Intermetallics 18 , 2183–2190 (2010)
Y. Sutou, T. Omori, R. Kainuma, K. Ishida, Acta Mater. 61 , 3842–3850 (2013)
G.-S. Yang, J.-K. Lee, W.-Y. Jang, Trans. Nonferrous Metals Soc. China 19 , 979–983 (2009)
R. Dasgupta, J. Mater. Res. 29 , 1681–1698 (2014)
N. Tuncer, C.A. Schuh, Scr. Mater. 117 , 46–50 (2016)
A. Agrawal, R.K. Dube, J. Alloys. Compd. 750 , 235–247 (2018)
G.A. López, I. López-Ferreño, A.R. Kilmametov, T. Breczewski, B.B. Straumal, B. Baretzky, M.L. Nó, J.S. Juan, Mater. Today 2 , S747–S750 (2015)
B.S. Shariat, Q. Meng, A.S. Mahmud, Z. Wu, R. Bakhtiari, J. Zhang, F. Motazedian, H. Yang, G. Rio, T.-H. Nam, Y. Liu, Mater. Des. 124 , 225–237 (2017)
K. Otsuka, C.M. Wayman, K. Nakai, H. Sakamoto, K. Shimizu, Acta Metall. 24 , 207–226 (1976)
T. Kusama, T. Omori, T. Saito, S. Kise, T. Tanaka, Y. Araki, R. Kainuma, Nat. Commun. 8 , 354 (2017)
J.S. Juan, M.L. Nó, C.A. Schuh, Nat. Nanotechnol. 4 , 415–419 (2009)
R.D. Cava, C. Bolfarini, C.S. Kiminami, E.M. Mazzer, V.M. Pedrosa, W.J. Botta, P. Gargarella, 22º CBECiMat - Congresso Brasileiro de Engenharia e Ciência dos Materiais, Natal/Brazil, 2016.
T. Gustmann, J.M. dos Santos, P. Gargarella, U. Kühn, J. Van Humbeeck, S. Pauly, Shape Memory Superelasticity 3 , 24–36 (2017)
P. Gargarella, C.S. Kiminami, E.M. Mazzer, R.D. Cava, L.A. Basilio, C. Bolfarini, W.J. Botta, J. Eckert, T. Gustmann, S. Pauly, Mater. Res. 18 , 35–38 (2015)
S. Pauly, P. Wang, U. Kühn, K. Kosiba, Addit. Manuf. 22 , 753–757 (2018)
T. Gustmann, A. Neves, U. Kühn, P. Gargarella, C.S. Kiminami, C. Bolfarini, J. Eckert, S. Pauly, Addit. Manuf. 11 , 23–31 (2016)
N. Babacan, S. Pauly, T. Gustmann, Mater. Design 203 , 109625 (2021)
M.L. Lapér, R. Guimarães, B.R. Barrioni, P.A.P. Silva, M. Houmard, E.M. Mazzer, E.H.M. Nunes, J. Market. Res. 9 , 3676–3685 (2020)
T. Gustmann, Fakultät Maschinenwesen (Technischen Universität Dresden, Dresden, 2018)
T. Gustmann, H. Schwab, U. Kühn, S. Pauly, Mater. Des. 153 , 129–138 (2018)
M.R. da Silva, P. Gargarella, T. Gustmann, W.J.B. Filho, C.S. Kiminami, J. Eckert, S. Pauly, C. Bolfarini, Mater. Sci. Eng. A 661 , 61–67 (2016)
B.B. Straumal, A.R. Kilmametov, G.A. López, I. López-Ferreño, M.L. Nó, J.S. Juan, H. Hahn, B. Baretzky, Acta Mater. 125 , 274–285 (2017)
H.E. da Silva, E.M. Mazzer, Materialia 12 , 100712 (2020)
P. Zhang, A. Ma, S. Lu, P. Lin, J. Jiang, H. Ma, C. Chu, Mater. Lett. 63 , 2676–2679 (2009)
S.N. Saud, T.A.A. Bakar, E. Hamzah, M.K. Ibrahim, A. Bahador, Metall. Mater. Trans. A 46 , 3528–3542 (2015)
E.M. Mazzer, C.S. Kiminami, C. Bolfarini, R.D. Cava, W.J. Botta, P. Gargarella, F. Audebert, M. Galano, Mater. Sci. Eng. A 663 , 64–68 (2016)
T. Gustmann, J.M. dos Santos, P. Gargarella, U. Kühn, J. Van Humbeeck, S. Pauly, Shape Memory Superelasticity 3 , 24–36 (2016)
D.B. Gera, J. Soyama, R.D. Cava, J.E. Spinelli, C.S. Kiminami, Adv. Eng. Mater. 20 , 1800372 (2018)
M.A. Morris, Acta Metall. Mater. 40 (7), 1573–1586 (1992)
J.W. Kim, D.W. Roh, E.S. Lee, Y.G. Kim, Metall. Trans. A 21 , 741–744 (1990)
S.N. Saud, E. Hamzah, T. Abubakar, M. Zamri, M. Tanemura, J. Therm. Anal. Calorim. 118 , 111–122 (2014)
Z. Deng, H. Yin, C. Zhang, W. Li, Mater. Sci. Eng. A 803 , 140472 (2021)
S.N. Saud, E. Hamzah, T. Abubakar, H.R. Bakhsheshi-Rad, S. Farahany, A. Abdolahi, M.M. Taheri, J. Alloys. Compd. 612 , 471–478 (2014)
X. Zhang, T. Cui, X. Zhang, Q. Liu, Z. Dong, C. Man, J. Alloys Compd. 858 , 157685 (2021)
X. Zhang, J. Sui, Q. Liu, W. Cai, Mater. Lett. 180 , 223–227 (2016)
S.N. Saud, E. Hamzah, H.R. Bakhsheshi-Rad, T. Abubakar, Scanning 2017 , 1789454 (2017)
S.N. Saud, E. Hamzah, T. Abubakar, S. Farahany, J. Mater. Eng. Perform. 23 , 255–261 (2013)
M. Kök, K. Yildiz, Appl. Phys. A 116 , 2045–2050 (2014)
M. Zare, M. Ketabchi, J. Therm. Anal. Calorim. 127 , 2113–2123 (2016)
P. Ochin, A. Dezellus, P. Plaindoux, J. Pons, P. Vermaut, R. Portier, E. Cesari, Acta Mater. 54 , 1877–1885 (2006)
X. Zhang, Q. Liu, Intermetallics 92 , 108–112 (2018)
S.N. Saud, E. Hamzah, T. Abubakar, H.R. Bakhsheshi-Rad, M.N. Mohammed, Metall. Mater. Trans. A 47 , 5242–5255 (2016)
R. Kainuma, S. Takahashi, K. Ishida, Metall. Mater. Trans. A 27 , 2187–2195 (1996)
S. Xu, T. Kusama, X. Xu, H. Huang, T. Omori, J. Xie, R. Kainuma, Materialia 6 , 100336 (2019)
J.P. Oliveira, B. Panton, Z. Zeng, T. Omori, Y. Zhou, R.M. Miranda, F.M.B. Fernandes, Mater. Design 90 , 122–128 (2016)
R. Kainuma, Mater. Trans. 59 , 327–331 (2018)
S.K. Tanaka, T. Omori, R. Kainuma, K. Ishida, Materia (Rio J.) 51 , 108–110 (2012). ( (in Japanese) )
Q. Wang, F. Han, J. Wu, G. Hao, Mater. Lett. 61 , 2598–2600 (2007)
X. Ji, Q. Wang, F. Yin, C. Cui, P. Ji, G. Hao, Compos. A Appl. Sci. Manuf. 107 , 21–30 (2018)
J. Yang, Q.Z. Wang, F.X. Yin, C.X. Cui, P.G. Ji, B. Li, Mater. Sci. Eng. A 664 , 215–220 (2016)
X. Lu, F. Chen, W. Li, Y. Zheng, J. Alloys. Compd. 480 , 608–611 (2009)
A. Pandey, S. Hussain, P. Nair, R. Dasgupta, J. Alloys Compds 836 , 155266 (2020)
S. Yang, M. Chi, J. Zhang, K. Zhang, X. Liu, C. Wang, X. Liu, Mater. Sci. Eng. A 739 , 455–462 (2019)
C.A. Canbay, S. Gudeloglu, Z.K. Genc, Int. J. Thermophys. 36 , 783–794 (2015)
C.A. Canbay, O. Karaduman, N. Ünlü, S.A. Baiz, İ Özkul, Compos. B 174 , 106940 (2019)
C.A. Canbay, A. Tataroğlu, A. Dere, A.G. Al-Sehemi, A. Karabulut, A. Bektaş, A.A. Al-Ghamdi, F. Yakuphanoglu, Mater. Sci. Eng. B 264 , 114931 (2021)
S. Montecinos, M.O. Moroni, A. Sepúlveda, Mater. Sci. Eng. A 419 , 91–97 (2006)
G.B. Narasimha, S.M. Murigendrappa, Mater. Sci. Eng. A 755 , 211–219 (2019)
V.H.C. de Albuquerque, T.A.D.A. Melo, R.M. Gomes, S.J.G. de Lima, J.M.R.S. Tavares, Mater. Sci. Eng. A 528 , 459–466 (2010)
V.H.C. de Albuquerque, T.A.D.A. Melo, D.F. de Oliveira, R.M. Gomes, J.M.R.S. Tavares, Mater. Des. 31 , 3275–3281 (2010)
G.V.M. da Candido, T.A.A. de Melo, V.H.C. De Albuquerque, R.M. Gomes, S.J.G. de Lima, J.M.R.S. Tavares, J. Mater. Eng. Perform. 21 , 2398–2406 (2012)
T. Kalinga, S.M. Murigendrappa, S. Kattimani, J. Mater. Eng. Perform. 48 , 1–11 (2021)
B.H.D.S. Andrade, R.E. Caluête, D.F. de Oliveira, R.M. Gomes, T.A.D.A. Melo, J. Thermal Anal. Calorim. 139 , 905–911 (2019)
ŞN. Balo, M. Ceylan, J. Mater. Process. Technol. 124 (1–2), 200–208 (2002)
L. Mañosa, A. Planes, M. Acet, J. Mater. Chem. A 1 , 4925 (2013)
M. Schmidt, A. Schütze, S. Seelecke, APL Mater. 4 , 064107 (2016)
J. Frenzel, G. Eggeler, E. Quandt, S. Seelecke, M. Kohl, MRS Bull. 43 , 280–284 (2018)
H. Ossmer, M. Kohl, Nat. Energy 1 , 1–2 (2016)
J. Tušek, K. Engelbrecht, R. Millán-Solsona, L. Mañosa, E. Vives, L.P. Mikkelsen, N. Pryds, Adv. Energy Mater. 5 , 1500361 (2015)
X. Moya, S. Kar-Narayan, N.D. Mathur, Nat. Mater. 13 , 439–450 (2014)
K. Engelbrecht, J. Phys. 1 , 021001 (2019)
S. Qian, Y. Wang, L. Yuan, J. Yu, Energy 182 , 881–899 (2019)
D. Cong, W. Xiong, A. Planes, Y. Ren, L. Manosa, P. Cao, Z. Nie, X. Sun, Z. Yang, X. Hong, Y. Wang, Phys. Rev. Lett. 122 , 255703 (2019)
S. Qian, L. Yuan, J. Yu, G. Yan, Energy 141 , 744–756 (2017)
Y. Wu, E. Ertekin, H. Sehitoglu, Acta Mater. 135 , 158–176 (2017)
H. Sehitoglu, Y. Wu, E. Ertekin, Scr. Mater. 148 , 122–126 (2018)
G.J. Pataky, E. Ertekin, H. Sehitoglu, Acta Mater. 96 , 420–427 (2015)
E. Bonnot, R. Romero, L. Manosa, E. Vives, A. Planes, Phys. Rev. Lett. 100 , 125901 (2008)
S. Qian, Y. Geng, Y. Wang, T.E. Pillsbury, Y. Hada, Y. Yamaguchi, K. Fujimoto, Y. Hwang, R. Radermacher, J. Cui, Y. Yuki, K. Toyotake, I. Takeuchi, Philos. Trans. Ser. A 374 , 2–8 (2016)
A. Gràcia-Condal, E. Stern-Taulats, A. Planes, E. Vives, L. Mañosa, Phys. Status Solidi B 255 , 1700422 (2018)
L. Mañosa, S. Jarque-Farnos, E. Vives, A. Planes, Appl. Phys. Lett. 21 , 2013 (1904)
L. Manosa, A. Planes, Adv. Mater. 29 , 1603607 (2017)
J. Tušek, K. Engelbrecht, L. Mañosa, E. Vives, N. Pryds, Shape Memory Superelasticity 2 , 317–329 (2016)
B. Yuan, S. Zhong, M. Qian, X. Zhang, L. Geng, J. Alloys Compd. 850 , 156612 (2021)
B. Yuan, X. Zhu, X. Zhang, M. Qian, J. Mater. Sci. 54 , 9613–9621 (2019)
B. Yuan, M. Qian, X. Zhang, M. Imran, L. Geng, Int. J. Refrig 114 , 54–61 (2020)
S. Xu, H. Huang, J. Xie, S. Takekawa, X. Xu, T. Omori, R. Kainuma, APL Mater. 4 , 106106 (2016)
B. Yuan, M. Qian, X. Zhang, L. Geng, Scr. Mater. 178 , 356–360 (2020)
N.-H. Lu, C.-H. Chen, Mater. Sci. Eng. A 800 , 140386 (2021)
H. Wang, H.-Y. Huang, Y.-J. Su, J. Alloys Compd. 828 , 154265 (2020)
X.-P. Zhao, H.-Y. Huang, C. Wen, Y.-J. Su, P. Qian, Comput. Mater. Sci. 176 , 109521 (2020)
J. Tan, Y. Wang, S. Xu, H. Liu, S. Qian, Energy 197 , 117261 (2020)
M. Imran, X. Zhang, Mater. Des. 195 , 109030 (2020)
E.M. Mazzer, P. Gargarella, C.S. Kiminami, C. Bolfarini, R.D. Cava, M. Galano, J. Mater. Res. 32 , 3165–3174 (2017)
J. Ma, I. Karaman, R.D. Noebe, Int. Mater. Rev. 55 , 257–315 (2013)
I. López-Ferreño, J.F. Gómez-Cortés, T. Breczewski, I. Ruiz-Larrea, M.L. Nó, J.M.S. Juan, J. Mater. Res. Technol. 9 , 9972–9984 (2020)
Download references
Acknowledgments
The authors would like to acknowledge the financial support of CAPES (Coordination for the Improvement of Higher Education Personnel—Brazil) under the program BRAGECRIM (BEX 7185/13-8), FAPESP (São Paulo Research Foundation—Brazil) under the projects 2013/05987-8, and 2017/27031-4 and FAPEMIG grant number APQ-00264-17. Additionally, we would like to thank Professor Claudio Kiminami and Professor Claudemiro Bolfarini for their valuable contributions to the field.
Author information
Authors and affiliations.
Departamento de Engenharia Metalúrgica e de Materiais, Universidade Federal de Minas Gerais (UFMG), Belo Horizonte, MG, Brazil
E. M. Mazzer
Departamento de Engenharia de Materiais, Universidade Federal de São Carlos (UFSCar), São Carlos, SP, Brazil
M. R. da Silva & P. Gargarella
Centro de Caracterização e Desenvolvimento de Materiais (CCDM), Universidade Federal de São Carlos (UFSCar), São Carlos, SP, Brazil
P. Gargarella
You can also search for this author in PubMed Google Scholar
Corresponding author
Correspondence to E. M. Mazzer .
Ethics declarations
Conflict of interest.
The authors declare no conflict of interest.
Rights and permissions
Open Access This article is licensed under a Creative Commons Attribution 4.0 International License, which permits use, sharing, adaptation, distribution and reproduction in any medium or format, as long as you give appropriate credit to the original author(s) and the source, provide a link to the Creative Commons licence, and indicate if changes were made. The images or other third party material in this article are included in the article's Creative Commons licence, unless indicated otherwise in a credit line to the material. If material is not included in the article's Creative Commons licence and your intended use is not permitted by statutory regulation or exceeds the permitted use, you will need to obtain permission directly from the copyright holder. To view a copy of this licence, visit http://creativecommons.org/licenses/by/4.0/ .
Reprints and permissions
About this article
Mazzer, E.M., da Silva, M.R. & Gargarella, P. Revisiting Cu-based shape memory alloys: Recent developments and new perspectives. Journal of Materials Research 37 , 162–182 (2022). https://doi.org/10.1557/s43578-021-00444-7
Download citation
Received : 30 June 2021
Accepted : 12 November 2021
Published : 01 January 2022
Issue Date : 14 January 2022
DOI : https://doi.org/10.1557/s43578-021-00444-7
Share this article
Anyone you share the following link with will be able to read this content:
Sorry, a shareable link is not currently available for this article.
Provided by the Springer Nature SharedIt content-sharing initiative
- Find a journal
- Publish with us
- Track your research
Accessibility Links
- Skip to content
- Skip to search IOPscience
- Skip to Journals list
- Accessibility help
- Accessibility Help
Click here to close this panel.
Purpose-led Publishing is a coalition of three not-for-profit publishers in the field of physical sciences: AIP Publishing, the American Physical Society and IOP Publishing.
Together, as publishers that will always put purpose above profit, we have defined a set of industry standards that underpin high-quality, ethical scholarly communications.
We are proudly declaring that science is our only shareholder.
Application of shape memory alloys in engineering – A review
M Balasubramanian 1 , R Srimath 2 , L Vignesh 2 and S Rajesh 2
Published under licence by IOP Publishing Ltd Journal of Physics: Conference Series , Volume 2054 , International Conference on Advances in Thermal Engineering and Applications 19-20 March 2021, Tamil Nadu, India Citation M Balasubramanian et al 2021 J. Phys.: Conf. Ser. 2054 012078 DOI 10.1088/1742-6596/2054/1/012078
Article metrics
6626 Total downloads
Share this article
Author e-mails.
Author affiliations
1 Professor, Department of Mechanical Engineering
2 Undergraduate Student, Department of Mechanical Engineering, RMK College of Engineering and Technology, Tamil Nadu, India
Buy this article in print
Shape Memory Alloy is a smart alloy which retains their original shape under thermomechanical or magnetic variation. Shape Memory Alloy are widely used in different engineering field because of its superior properties and variety of application. Recent research of SMA has been applied in the field of Aerospace, Automotive, Biomedical, and Robotics. This memory effect is due to the presence of austenite and martensite crystalline structures. These alloys are bio-compatible, lightweight, and also possess a high force-to-weight ratio. Due to this SMA actuators are very much suitable for soft robotic applications. However, due to high cooling times during phase change, SMA has small bandwidth and low operating frequencies. This alloy can replace a sensor as it performs the same work done by sensors or transducer. An extensive review of history, material characterization, and opportunities of SMA in the engineering field.
Export citation and abstract BibTeX RIS
Content from this work may be used under the terms of the Creative Commons Attribution 3.0 licence . Any further distribution of this work must maintain attribution to the author(s) and the title of the work, journal citation and DOI.

An official website of the United States government
The .gov means it’s official. Federal government websites often end in .gov or .mil. Before sharing sensitive information, make sure you’re on a federal government site.
The site is secure. The https:// ensures that you are connecting to the official website and that any information you provide is encrypted and transmitted securely.
- Publications
- Account settings
Preview improvements coming to the PMC website in October 2024. Learn More or Try it out now .
- Advanced Search
- Journal List
- Materials (Basel)

Shape Memory Alloys for Aerospace, Recent Developments, and New Applications: A Short Review
Girolamo costanza.
1 Industrial Engineering Department, University of Rome Tor Vergata, Via del Politecnico 1, 00133 Rome, Italy
Maria Elisa Tata
2 Civil Engineering and Computer Science Department, University of Rome Tor Vergata, Via del Politecnico 1, 00133 Rome, Italy; [email protected]
Shape memory alloys (SMAs) show a particular behavior that is the ability to recuperate the original shape while heating above specific critical temperatures (shape memory effect) or to withstand high deformations recoverable while unloading (pseudoelasticity). In many cases the SMAs play the actuator’s role. Starting from the origin of the shape memory effect, the mechanical properties of these alloys are illustrated. This paper presents a review of SMAs applications in the aerospace field with particular emphasis on morphing wings (experimental and modeling), tailoring of the orientation and inlet geometry of many propulsion system, variable geometry chevron for thrust and noise optimization, and more in general reduction of power consumption. Space applications are described too: to isolate the micro-vibrations, for low-shock release devices and self-deployable solar sails. Novel configurations and devices are highlighted too.
1. Introduction
Shape memory alloys (SMAs) are metals able to recuperate a preset shape just while heating up to a critical transformation temperature. The physical principle they are based on is a particular martensitic transformation (thermoelastic) occurring in some metallic alloys [ 1 ]. The list includes but is not limited to NiTi, CuAlNi, CuZnAl, AuCd, and many others. Buehler et al. in 1963 [ 2 ] were the first to evidence the shape memory effect in near equiatomic Ti-Ni alloys. The shape memory effect was found earlier in Au-47.5% Cd [ 3 ] and In-Tl [ 4 , 5 ] but without attracting attention from the researchers. On the contrary, equiatomic Ni-Ti alloys became popular thanks to Naval Ordinance Laboratory, hence the name Nitinol. The real understanding of the phenomena occurring during the martensitic transformation was not immediate. The main reason may be ascribed to the complexity of the Ti-Ni phase diagram ( Figure 1 ) and to the formation of a multiplicity of precipitates under certain heat-treatments (TiNi, Ti 2 Ni, TiNi 3 , Ti 2 Ni 3 , Ti 3 Ni 4 ). Moreover, the R-phase (rhombohedral) transformation, considered to be a pre-martensitic phenomenon, appears under certain conditions while cooling. Now it is established that the R-phase itself is a martensitic transformation and contends with the successive martensitic transformation. Due to the excellent mechanical properties, corrosion, and abrasion resistance Ni-Ti shape memory alloys have been widely employed in many technological applications: stent, guide wire, antenna for mobile phones, coffee maker, orthodontic wire, flap for air-conditioner, and many others. The main aim of this review is to identify and exemplify a detailed list of novel applications of SMA in the aerospace field in the most updated manner without the claim of comprehensiveness, according to available recent literature in terms of relevance, number of articles, and novelty of the proposals. Ni-Ti alloys are the most employed ones, but the subject is not limited to them.

Binary phase diagram of Ti-Ni alloy [ 7 ]. Figure 1 Reprinted from Materials, Vol. 12 (5), Chekotu, J.C.; Groarke, R.; O’Toole, K., Brabazon, D., Advances in Selective Laser Melting of Nitinol Shape Memory Alloy Part Production, 2019.
2. Origin of the Shape Memory Effect
For the comprehension of the basic principles regarding the shape memory effect and pseudoelasticity, many contributions are useful. The crystallography of martensite, the transformation temperatures, and rate of martensite formation are discussed in details by Nishiyama [ 6 ].
The macrostructure consequences of the microstructure and the shape memory effect have been highlighted by Bhattacharya [ 8 ]. The design of some shape memory alloy actuators were focused on by Otsuka and Wayman [ 9 ]. National Aeronautics and Space Administration (NASA) report SP5111 published in 1972 provides experimental results, although the base mechanism was not fully understood at that time [ 10 ]. The phase transformation occurring on an SMA is peculiar because it coexists with great strain and is fully recoverable, so that in a uniaxial tensile test in NiTi it can reach also 10% [ 1 ]. For this reason, SMAs belong to the family of the “active materials” [ 11 ]. SMAs can also provide high forces and wide displacements when activated, if compared to other active materials. Novel application fields continue to be identified [ 12 ]. Biomedical industry is the most served one, together with the aerospace industry. SMAs can be identified also into the class of the “smart materials”: an SMA component, with structural or functional purpose, can make a system simpler in comparison with the same manufactured piece adopting conventional know-how (for instance electromechanical or hydraulic). High activation loads and displacements generates great energy density. These properties are welcome especially in the aerospace field, hence the main reason of this review. The technological requirements of the aerospace field are more important than any considerations about the technology cost and this aspect can be identified as the real reason of the success of SMAs.
3. SMA Behavior
In an extremely simplified manner, the main phases involved in an SMA are being austenite stable at higher temperature (parent phase) and martensite stable at lower temperature with tetragonal or monocline structure [ 1 , 8 , 12 ]. A lot of stress-strain curves obtained in tensile tests for the Ti-50.6Ni alloy at different and constant temperatures are reported in Figure 2 [ 13 ], highlighting the mechanical behavior from low temperature to high temperature. At lower temperatures ( Figure 2 a–i), it is evident the shape memory effect: after an initial linear stage a plateau of stress is evident, followed by residual strain while unloading. After that, the shape recovery (not shown in the graph) on heating starts at As (Austenite start temperature) and finishes at Af (Austenite finish temperature). At temperatures higher than Af ( Figure 2 j–p), austenite is the only stable phase. Tensile behavior exhibits a loading path with a plateau at higher stress than that in the martensitic phase. During unloading, the strain is fully recovered and the classic flag-type diagram is evidenced, without the necessity of further heating. The higher the temperature, the higher the level of stress for the plateau.

Stress-strain curves for a Ti-50.6Ni alloy showing shape memory effect ( a – i ) and pseudoelastic effect ( j – p ) [ 13 ]. Figure 2 Reprinted from Scripta Metallurgica, Vol. 15, Miyazaki, S.; Otsuka, K.; Suzuki, Y., Transformation pseudoelasticity and deformation behavior in a Ti-50.6Ni alloy, 1981, with permission from Elsevier.
The aerospace industry is actively looking for novel solutions and applications based on the integration of the SMAs in the actual technologies as well as the definition and development of new ones. SMA adoption allows to increase the simplicity of the systems as well as to reduce the weight and the volume of such active devices allowing it to achieve more compact structures. SMAs are attractive as a solution to complex engineering problems, along with high actuation stresses and strains due to their intrinsic great power/weight ratio. Integrating actuators and structures means it is possible to achieve high reliability and compact arrangements, reliable also for a high number of activation cycles [ 14 , 15 ]. Systems activated by SMAs have been widely examined in many studies and pieces of research in substitution of standard actuation systems, hydraulic and electric.
4. SMA Applications on Wings
Wing morphing in planes is a practical solution involving structural modifications so that the required specifications can be met in different utilization conditions. Some of the advantages are reported in the following: increased speed, reduced power consumption, roll control, and camber change. On the other hand, morphing is usually associated to drawbacks like surface discontinuity and being over-weight due to the necessary control structures. Adopting shape memory solutions and their corresponding mechanisms means some of these drawbacks can be overcome. Smart Wing Program and Smart and Aircraft and Marine Propulsion System Demonstration (SAMPSON) [ 16 , 17 ] are the most famous projects regarding fixed wings both recognized by Defense Research Projects Agency (DARPA). The first one was conceived for the utilization of smart materials like lifting devices thanks to the specific power and power density of SMA actuators in comparison with traditional electromechanical ones. The second one had a main goal of the development of new affordable smart materials and is concerned with shape-adaptive structures for aircraft. In particular, the Sampson project examined models for changing the shape in gas turbine inlets for application in supersonic airplanes. A novel application for a hydrodynamic maneuvering system to be employed in maritime propulsion has been investigated too. The shape memory effect has been employed to provide actuation by means of shape recovery [ 18 , 19 ]. The main goal was to show the possibility of adoption of SMAs in modifying the orientation and geometry in different propulsion devices. An experiment on the real F-15 inlet was performed in the configuration of an SMA cable antagonist to another one ( Figure 3 ). Some examples of morphing wings are reported in Figure 4 .

The SAMPSON F-15 inlet tested in the facility at Langley (NASA) [ 19 ]. Figure 3 Available online: https://crgis.ndc.nasa.gov/historic/File:2000-L-00475.jpg .

Various morphing devices employed on the wing airplanes can change, stretching or compressing, the aerodynamic profile [ 20 ]. Figure 4 Reprinted from Chinese Journal of Aeronautics, Vol. 32 (4), Chen, Y.; Shen, X; Li, J.; Chen, J., Nonlinear hysteresis identification and compensation based on the discrete Preisach model of an aircraft morphing wing device manipulated by an SMA actuator, 2019, open access.
The F15E Strike Eagle is a fighter jet conceived to accomplish different kinds of missions. Thanks to the two engines, the aircraft can reach speeds higher than Mach 2.5. The inlet system of this jet consists of five ramps adopted for the conditioning of the air flow for both engines. The ramps are connected to a cowl which can rotate up to 15° as a function of the different flight conditions. The SMAs are employed to rotate the inlet cowl in order to change the air flow cross section. In the tested model, many modifications have been applied from the original configuration allowing to host the shape changing elements. A force of 26,700 N and 9° rotation angle have been applied by means of a bundles of 34 wires [ 21 ]. On the aft side of the fan nozzle shape, memory cables have been disposed circumferentially around and tested too [ 22 ] so that fan nozzle area can be modified in different operative conditions during flight. For the wind tunnel tests, the configuration with a sting attached in the lower side of the inlet by means of a plate support was adopted. For the experimental validation of the F-15 inlet, the NASA Langley wind tunnel was used ( Figure 3 ). Slow SMA cooling and longer retraction time was the prime limitations of the proposed system, and even at the stage of experimental tests.
A project to decrease noise at airports generated by departing airplanes was focused on by Hartl and Lagouda [ 23 , 24 ]. It has been demonstrated that the adoption of aerodynamic devices, better known as chevrons, on the rear edge of an exhaust nozzle (both on primary and secondary exhaust flow) can reduce jet noise at take-off thanks to the appropriate streams mixing. As a consequence of the secondary exhaust nozzle, chevrons immersed in the fan flow show a reduction of thrust and an increase of drag while cruising was detected. For these reasons, the optimum solution was identified in the Variable Geometry Chevron (VGC). Based on SMA components, VGC exhibits all-out bending during take-off and landing with minimum deflection flow while cruising so that efficiency is increased and noise reduction ensured. The system contains active SMA bundles enclosed in a complex case. The activation of the SMA beams allows the requested bending force on the chevron structure so that noise can be reduced. Boeing tested in flight the proposed solution adopting active SMA elements ( Figure 5 ). At the end of the study results from experiments and modeling have been compared. Tip displacement and chevron deflection have been analyzed focusing the attention on the actuation profile. The developed model can be a useful instrument for the prediction of mechanical actuation of such a system subjected to pre-determined thermal inputs. Suitable systems for use in service are still under investigation.

Variable geometry chevron activated by SMA in the full scale test [ 23 ]. Figure 5 Reprinted from Proceedings of the Institution of the Mechanical Engineer, Part. G: Journal of Aerospace Engineering, Vol. 221, Hartl, D.J.; Lagoudas, D.C., Aerospace applications of shape memory alloys, 535–552, 2007, open access.
In addition to the propulsion system, SMAs can be usefully applied in adapting lifting bodies and morphing the wing structures. The integration of the SMA elements in aerostructure has been focused in many studies. One of the promising approaches is to insert SMA wires into an innovative composite structure. In order to exploit the one-way shape memory effect, NiTi alloy wires of 150 μm diameter have been pre-stressed and inserted into a Kevlar fiber epoxy matrix [ 25 ]. SMA composites have a great potential in adaptive uses such as progressive reinforcing of components (structure) or change of the intrinsic vibration frequencies. However, the SMA behavior is not linear and offers many options. Moreover, increased knowledge regarding the stress transfer between metal and polymer matrix is required as well the fatigue behavior of such structures. Another application concerns SMA wire actuators, which can be connected to some internal points of an airfoil and activated to change the shape of the airfoil itself. The change in shape can rise the wing’s efficiency also in different flow regimes in operative flying conditions. The main goal to identify the number and the right positioning in the wing of the SMA wire actuators within the wing through a global optimization method was achieved. For the verification of the predicted structural and aerodynamic response, a suitable wing model to be tested in a wind tunnel was manufactured. The numerical pressure data have been found to be in good agreement with experiments performed in the wind tunnel tests, according to which a higher value of lift for a fixed speed and angle of attack by activating the SMA wire actuators was detected [ 26 ].
SMA actuators to be employed for morphing wings attracted researchers’ attention. Brailowski et al. [ 27 ] modeled morphing wings by means of a coupled thermo-mechanical approach and the results have been validated in some tunnel tests. An experimental Morphing Laminar Wing (MLW) was set-up to demonstrate the practicability of airplanes’ fuel saving by improving the laminar flow regime on the wing extrados while cruising (subsonic motion). The prototype combined three main subsystems: flexible extrados, rigid extrados, and actuator positioned in the wing box ( Figure 6 ). The wing morphing capacity was based on precise buckle of the wing extrados obtained by means of SMA actuators. The evaluation of the mechanical and aerodynamic behavior of the morphing wing in various flight scenario was possible thanks to a coupled model (fluid and structure). A small-scale morphing wing prototype (chord 0.5 m, span 1 m) was tested in a tunnel wing under subsonic conditions. The integration of the coupled thermomechanical finite element model with the previously developed structure-aerodynamic one of the morphing wing can be successfully employed to achieve the optimum solution of the whole system.

( a ) Sketch of the morphing laminar wing adopting extrados with shape memory alloy (SMA) active element [ 27 ]. ( b ) Scheme of the SMA actuator’s working principle [ 27 ]. Figure 6 Reprinted from Physics Procedia, Vol. 10, Brailovski, V.; Terriault, P.; Georges, T.; Coutu, D., SMA Actuators for morphing wings, 197–203, 2010, open access.
Coutu et al. [ 28 ] identified two core design constraints: the number of layers in the composite of the flexible extrados and the number of actuators. In order to equipoise the trade-off between stiffness and elasticity of the whole active structure, mechanical (deformation energy) and aerodynamic (laminar air flow regime improvement) performance benchmarks have been taken into account at the same time. By means of the multi-objective optimization practice, the designers selected the configuration 4-ply 2-actuator for the extrados configuration activated by SMA elements.
A method based on the discontinuous Preisach modeling, able to identify the hysteretic behavior of the morphing wing, has been suggested by Chen et al. in [ 20 ]. A group of discontinuous and equidistant points was applied to the Preisach plane so that the Preisach density in the selected unit was determined. Discontinuous Preisach modeling is achieved by the discontinuous first-order reversible curve. Thanks to the discrete Preisach model, it has been possible to simulate the airplane morphing wing; the effectiveness of the adopted model has been shown by opposing the modelled results with experimental results of the main hysteresis and the wing displacement. Afterwards, a hysteresis compensation strategy was defined for the actuation and control of the airplane wing. Such a device manifested a hysteretic behavior in which the shrinking had been compensated. In this way it has been possible to achieve the extension of the morphing application also to the intrinsic hysteretic region.
Another interesting advantage of this technical solution is that morphing wings can generate smoother wing surfaces, thus increasing airplane agility and efficiency by adopting discontinuous moving surfaces. A novel solution based on a double corrugated variable camber structure with morphing units of the rear edge has been proposed by Kumar et al. [ 29 ]. Two configurations have been considered: the first one is thought on the basis of a fish bone active camber, the second one on an adjustable camber morphing wing made of single corrugated structure. The comparison between the two configurations has been performed in terms of structural and dynamic analysis. The structural investigation of all simulations was carried out by means of the finite element analysis with particular reference to the actuation mechanism. The aerodynamic analysis was performed following two different techniques: the analytical approach based on thin airfoil theory and the other one numerically using Xfoil software. The last one combines a potential-flow panel process with viscous boundary-layer solver. An assessment was performed according to the stress and strain established in different portions of the models. The results have evidenced that the configuration double-corrugated variable camber morphing arrangement was able to undertake a higher level of loads in absence of detrimental strain. On the other hand, the aerodynamic investigation has shown that the efficiency of such a morphing wing is greater in comparison with other configurations.
An interesting historical review about morphing aircraft can be found in [ 30 ]. The challenge to concept a structural element that is able not only to allow the prescribed loads but with a shape adaptable to the various operative conditions has been well illustrated. The right mixing of morphing structures and smart actuators in the perspective of an integrated approach requires multidisciplinary thinking, from the initial development at the preliminary design stage. The review presents the state-of-the-art on morphing airplanes. Attention is focused on the fundamental concepts and SMA-activated morphing wing for both configurations (fixed and rotary wings) and in particular to the active systems. Solutions based on inflatable devices and skin issues have not been considered. In general, the penalty due to the weight increase associated with the actuation systems in any successful wing morphing system must be overcome. Despite that many fascinating concepts have been combined, just a few have reached the development stage for the wind tunnel test and ever fewer have been tested in real flight conditions.
An interesting overview and a case study of morphing wing for the improvement of the control performance of airplanes have been illustrated by Hattalli and Srivatsa [ 31 ]. The case study focused on the camber change of the horizontal tail of an aircraft employing SMAs. A numerical analysis was carried out aimed at the examination of the camber change by means of shape memory actuators. A maximum deflection of 0.6 mm at the trailing edge tip ( Figure 7 ) on the horizontal tail yielded was detected. By modulating the shape memory intensity and the positioning of the strips, a novel and enhanced model for the delivery of a morphable wing was defined.

Camber change of activated and deactivated airfoil profile [ 31 ]. Figure 7 Reprinted from Materials Today Proceedings, Vol. 5, Hattalli, V.L.; Sritvasa, S.R., Wing morphing to improve control performance of an aircraft—An overview and a case study, 21442–21451, 2018, with permission from Elsevier.
The comparison of the results from wind tunnel tests and numerical modeling regarding a morphing wing fitted out with flexible upper surface actuated by aileron have been presented by Gabor et al. [ 32 ]. The experimental setup was demonstrative of a real airplane’s wing tip section. The development was performed according to a multifaceted and multidisciplinary design process. The prototype was tailored by a composite material skin on top with a morphable shape according to the various flight conditions exploiting four shape memory devices positioned inside the wing. The main aim of the optimization was to control the level of the region under laminar flow. The subsequent shapes were acquired by means of an accurate photogrammetry technique. Computational fluid dynamics analysis was conducted and a suitable model for the prediction of the transition from laminar to turbulent flow on the wing surface was developed. Three different aileron deflection angles and angles of attack in the range of five degrees were considered. The outcome of the simulation was compared with the measurements carried out by means of infrared thermography technique. In this case, during tunnel testing in subsonic conditions surface pressure measurements, balance load measurement and transition location were acquired. Good agreement was found between numerical and infrared thermography results with a 5% average deviation of the chord.
A novel genetic algorithm useful for the optimization of the shape and of the upper surface of an airfoil by means of actuators has been presented by Koreanski et al. [ 33 ]. The method was adopted for two dissimilar airfoils and the algorithm was related to two other optimization methods: gradient method and artificial bee colony. The outcomes of the optimization with each of method was schemed on response surfaces obtained with the Monte Carlo method. From the comparison it was demonstrated that they were placed in the global optimum region. Results for different wind tunnel test case (16) and objective Functions (2) were shown. The enhancement of about 17% of the chord for the former (transition interval) and about 31% of the chord for the latter (transition advancement) were identified. The outcomes of the performed optimization (displacement) were used as input data for the positioning control of the upper surface in the wind tunnel testing on the MDO 505 wing tip demonstrator. Comparisons between the experimental transition regions of the wing section (morphed and un-morphed) were carried out by means of infrared (IR) thermography. Numerical optimization was validated as documented in a successive paper [ 34 ] ( Figure 8 and Figure 9 ). The optimization was accomplished for 16 flight cases by changing the following parameters: speed, attack angles, and aileron deflections. The results of the optimization of the flow for the airfoil morphing upper-surface were validated by means of IR thermography on the wing-tip demonstrator. According to the validation results, the 2D numerical optimization of the genetic algorithm was demonstrated to be a useful tool for the improvement of various aerodynamic features of a wing’s performance.

Wing model setup in wind tunnel section [ 34 ]: Leading edge ( Left ); Trailing edge ( Right ). Figure 8 Reprinted from Chinese Journal of Aeronautics, Vol. 30 (1), Koreanski, A.; Gabor, O.S.; Acotto, J.; Brianchon, G.; Portier, G., Botez, R.M.; Mamou, M.; Mebarki, Y., Optimization and design of an aircraft’s morphing wing-tip demonstrator for drag reduction at low speed, Part. II – Experimental validation using Infra-red transition measurement from wind tunnel tests, 164–174, 2017, open access.

Example of infrared results for morphed wing demonstrator without aileron [ 34 ]. Figure 9 Reprinted from Chinese Journal of Aeronautics, Vol. 30 (1), Koreanski, A.; Gabor, O.S.; Acotto, J.; Brianchon, G.; Portier, G., Botez, R.M.; Mamou, M.; Mebarki, Y., Optimization and design of an aircraft’s morphing wing-tip demonstrator for drag reduction at low speed, Part. II – Experimental validation using Infra-red transition measurement from wind tunnel tests, 164–174, 2017, open access.
Also, flap can be morphed by means of shape memory alloys, as proposed and demonstrated by Bashir et al. [ 35 ]. The aerodynamic features of a flap have been examined by means of aerodynamic analyses and wind tunnel tests. The beams of the morphing flap have been manufactured with multiple elements joined together allowing rotations between adjacent elements and forming a flat shape of the morphing flap ( Figure 10 ). Such a morphing flap consisting of multiple elements was analyzed from the aerodynamic point of view using XLFR pro: the aerodynamic behavior was compared with that of a classical mechanical-flap and the main characteristics were measured in a wind-tunnel facility. The first comparison regards the improvements of the lift increment and drag decrease of the standard mechanical flap. On the other hand, the morphing-flap wings exhibited the highest lift/drag ratio gain in the comparison with standard flap at corresponding attack angles. The comparison has shown that the maximum lift-drag ratio gains is evidenced in correspondence of the 0° angle of attack. In conclusion, if a mechanical flap is changed with a morphing flap, at 0° incident angle, a more efficient level flight can be achieved. For what concerns the results of the lift/drag ratio gain of the six-element morphing flap, the comparison where the mechanical and morphing flaps have shown their maximum lift/drag ratios was analyzed. The maximum lift/drag ratios were detected for different attack angles for the mechanical flap and for morphing flap. Results of experiments in the wind tunnel test have shown that the maximum lift/drag ratio for the morphing-flap has been detected with a 0° incident angle, independent to the flap deflection angle. The maximum efficiency of a level flight can be obtained when incident angle is set to be angle of attack of maximum efficiency. The multi-element morphing flap adopted in the final model has shown the maximum lift/drag ratio at incident angle of 0°. The experimental results of the wind tunnel test showed an increase of the lift/drag ratio of about 83.98% achieved with a flap deflection angle of 20°, which is a highly significant gain with respect to flight efficiency. Despite many studies, research projects, prototypal wind tunnel tests, and in-flight with unmanned airplane (NASA), SMA-activated morphing wings are still not adopted on modern aircrafts.

Shape memory alloy actuator (extension) in a morphing wing [ 35 ]. Figure 10 Reprinted from Materials Today Proceedings, Vol. 5, Bashir, M.; Rajendram, P.; Sharma, C.; Smrutiranjan, D., Investigation of smart material actuators & aerodynamic optimization of morphing wing, 21069–21075, 2018, with permission of Elsevier.
5. Space Applications
Space applications of SMAs seek to solve the exceptional problems of actuation, release, and vibration attenuation in the launch of a spacecraft or subsequent operations, in conditions of microgravity and zero atmosphere. A solution to this problem has been proposed by Kwon et al. [ 36 ] with a pseudoelastic shape memory alloy mesh washer. Thanks to this device, vibration isolation is achieved in a severe launch vibration environment as well for micro-vibrations from the cryocooler on-orbit ( Figure 11 ). Undesirable microvibrations generated by a spaceborne cryocooler during the on-orbit operation are the main source of loss of the image quality taken from high-resolution satellites. For the compliance of the mission requirement, in order to acquire high-quality images, the isolation of the micro-vibrations generated by a cryocooler is required. In the proposed solution a spaceborne cryocooler micro-vibration isolator, based on a pseudoelastic mesh washer, has guaranteed adequate vibration isolation in an environment as severe as launch. Micro-vibrations from the cryocooler on orbit have been mitigated too. Static tests and free vibration tests have been performed on the assembly and the effectiveness of the concept has been demonstrated by the micro-vibration assessment tests. The working principle of such usefully employed devices is based on the wide hysteresis and strong non-linearity exhibited during the loading and unloading cycle. An overview of the pseudoelastic behavior, modeling, and applications at the same time with their limitations in seismic isolation and vibration control can be found in the paper by Saadat et al. [ 37 ]. The evaluation of the pyroshock isolation performance of a compressed mesh washer isolators has been focused on by Youn et al. [ 38 ]. Shape memory alloy compressed mesh washer isolators have been prepared and tested. The dynamic response of the compressed mesh washer isolators due to precompressive displacements has been analyzed too. The results showed that damping capacity and the natural frequency can be modified by varying the precompressive displacement of the isolator. These features can be useful for smart isolation systems based on the adaptable dynamic capacity of the isolator.

Shape memory alloys (SMA) mesh washer developed by Kwon et al. ( Left ) [ 36 ]. Pseudoelastic SMA mesh washer ( Right ) [ 36 ]. Figure 11 Reprinted from Cryogenics, Vol. 67, Kwon, S.C; Jeon, S.H.; Oh, H.U., Performance evaluation of spaceborne cryocooler micro-vibration isolation system employing pseudoelastic SMA mesh washer, 19–27, 2015, with permission of Elsevier.
Present solar array equipment delivers specific energies of about 20–40 W/kg in the case of the solar array deployment system. Higher specific energies, greater than 100 W/kg, can be achieved with Lightweight Flexible Solar Array (LFSA) technology proposed by Carpenter and Lyons [ 39 ]. Photovoltaic arrays could in some missions provide high power/weight ratios in comparison with conventional solar arrays. A higher science payload mass fraction can consequently be exploited. The solar array uses copper indium diselinide solar cells on a bendable substrate. Shape memory alloys applied on the hinge as a deployment system can be adopted. In this way, the heating of the SMA elements allows the hinges to be deployed [ 39 ]. In this application, the spacecraft 28 V bus can be employed. The shock-less deployment could increase the spacecraft dynamics while deployment is happening. SMA elements are also safer to be handled, integrated and tested in comparison with conventional ones. SMA hinge devices for solar array are at the same time less expensive, more reliable, and simpler. However, the development stage of SMA-activated flexible devices is still experimental.
One of the most successful uses of SMA in space is the solution to the problem of low-shock release devices. These mechanisms are very common in the design of spacecraft and have been conceived and developed some years ago, as shown by the Johnson’s Patent [ 40 ]. It deals with a release device based on non-explosive separation system for releasable components of equipment or apparatus that are required to be released under no-shock conditions. In such a separation device, the active element is strained to an armed configuration in low temperature condition that is below the activation temperature. The activation of the device happens while heating the shape memory element up to reach the transition temperature. In this way, the elements can recover its preset shape in a continuous manner, without shock. A prototype of the invention deals with a method for testing the integrity and the readiness of the low-shock separation device and at the same time a method for the calibration of the actuator’s release point. The relevance of the argument is ascribable to the fact that the pyrotechnic release mechanism has been often found as the root of failure and the cause of abortion of the mission [ 41 ]. As the activation of the SMAs happens slowly by continuous and controlled heating, SMA components can be suitably employed in low-shock release mechanisms also in micro-sized satellites. A new era is coming in device design thanks to the continuous request for satellites and spacecraft to be smaller, faster, and cheaper. Simple designs and applications based on novel technologies seem to be ideal for miniature mechanism design. The developed mechanisms in practical use include separation nut (micro and mini), mini rotary actuator, micro burn wire release, an SMA linear actuator, and an SMA redundant release mechanism [ 42 ]. Also, in small satellites, a great variety of pull-out systems to accomplish functions of many missions (separation from the launch vehicle, separation from each other, instruments deployment) are always more frequently required. Two effective flights have been arranged by the Air Force Research Laboratory on the topic of low-shock release mechanisms. The first experiment was called Shape Memory Alloy Release Device (SMARD) and it was performed in May 1999. The second experiment in January 2000 regarded the deployment of the Air Force Academy FalconSat spacecraft from the Orbital Sub-Orbital Program Space Launch Vehicle (OSP-I) [ 43 ]. Smaller rotary actuators were developed by Park et al. [ 44 ] through microfabrication methods employing shape memory wires with a small diameter (about 100 micron). It has been well established that rotary actuators of a maximum dimension of 5 mm may be constructed with an actuation angle of around +/−60°. On the basic principle of this bi-directional actuator, SMA ratchet micro mechanisms (about 200 micron) have been designed and manufactured for the transformation of angular motion in continuously rotating motion. Shape memory elements have been embedded into polyurethane structures with a suitable manufacturing process. Layer by layer in this way it is possible to manufacture complex 3D components by means of combination of material addition and subtraction. In order to offer an actuator of the same compactness with conventional systems, very small parts should be manufactured while SMA elements are at the same scale of the actuators housing itself.
Another important SMA possible application field is in solar sailing, still in the small-prototype laboratory test development stage. The solar sail working principle is based on the radiation pressure acting as a propulsion system. Sunlight can be employed to move space vehicles by the reflection of solar photons from a huge and light-weight material. In this way, no propellant is necessary for primary propulsion. A novel self-deploying solar sail activated by NiTi shape memory wires has been designed and manufactured in the form of a small prototype by Costanza and Tata [ 45 ]. Aluminum and Kapton have been adopted in this experiment in order to simulate the sail. Three dissimilar arrangements have been analyzed for bending the sail. For the active elements, two NiTi wires were employed so that the self-deployment of the sail happens when the active elements approach the critical transformation temperature. In the first experiments, heating was performed by infrared lamps up to the activation of the shape memory elements. Successively, Costanza and Tata [ 46 ] focused their attention in the deployment experiments performed in the laboratory, on the analysis of different heating technique, and different pressure conditions on the activation times. By employing shape memory wires, a small solar sail prototype was self-deployed in laboratory in atmospheric conditions and in low pressure conditions (0.05 bar) just from the effect of a halogen lamp ( Figure 12 ). In order to evaluate the effectiveness of the self-deploying system, the planarity degree of the sail has been evaluated by Boschetto et al. [ 47 ]. The level curves from the regression plane were highlighted and the differences between the four analyzed configurations analyzed. The vertices were identified as the most critical zones. Some small-scale solar sail prototypes have been studied, prepared, and tested by Bovesecchi et al. [ 48 ] in three different environmental conditions to simulate, as much as possible, the real working environment of solar sails ( Figure 13 ). In the same work, heat transfer mechanisms were analyzed too. Moreover, to demonstrate the feasibility of the project, the smallest distance from the sun allowing the full activation of the SMA elements and the self-deployment of the sail was also calculated. The integration of SMAs with carbon fiber composite in a solar sail was discussed for the optimization of the surface/weight ratio by Costanza et al. [ 49 ]. In a device conceived for one-time activation (i.e., micro-actuator for satellite), the training of the SMA is not required and the shape memory stability is not a problem. But if the system is designed for many activation cycles, the stability of the material, the capability to perform many cycles in a stable manner, as well fatigue behavior (mechanical, thermal, or combination or both) must be considered. The capabilities of SMA at a high number of activation cycles has been focused on and discussed in many papers. Shape memory alloys can successfully be employed to provide linear displacements. Costanza et al. [ 14 ] demonstrated that SMA springs can be subjected to up to 600,000 activation cycles with a limited and controlled loss of the shape memory recovery. The stabilization of the SMA spring’s behavior with the increase of the working cycle has occurred as evidenced in [ 14 ]. The loss of the shape recovery efficiency has been quantified in [ 15 ]. The phenomenon is ascribable to functional fatigue and the formation of the R phase upon heating has been evidenced by resistivity measurements. The effect of temperature on the tensile behavior of NiTi sheets has been discussed in [ 50 ]. Four NiTi flat elements have been analyzed in tensile conditions (load/unload cycles) at constant temperatures from room temperature up to 60 °C. The shape recovery has been measured and was found to be higher at increasing testing temperatures. The energy dissipation on each load-unload cycle has been measured and found to be greater at higher temperatures.

Step of solar sail folding sequence for two different configurations of the SMA actuators [ 47 ]: towards inside ( a – c ) and towards outside ( d – f ). Figure 12 Reprinted from Actuators, Vol. 8 (2), Boschetto, A.; Bottini, L.; Costanza, G.; Tata, M.E., Shape memory activated self-deployable solar sails: small scale prototypes manufacturing and planarity analysis by 3D laser scanner, Art. N. 38, 2019, open access.

Solar-sail self-deployment experiment under vacuum condition in the bell jar ( Left ) [ 48 ], solar sail with carbon fiber on the frame integrating the SMA ( Right ) [ 49 ]. Figure 13 Reprinted from Aerospace, Vol. 6 (7), Bovesecchi, G.; Corasaniti, S.; Costanza, G.; Tata, M.E., A novel self-deployable solar sail system activated by shape memory alloys, Art. N. 78, 2019, open access e Reprinted from Advances in materials science and engineering, Vol. 2017, Costanza, G.; Leoncini, G.; Quadrini, F.; Tata, M.E. Design and characterization of a small-scale solar sail prototype by integrating NiTi SMA and carbon fibre composite, Art. N. 8467971, 2017, open access.
A prototypal linear actuator activated by shape memory elements with modular stroke has been designed and manufactured by Costanza et al. [ 51 ]. The developed prototype has been employed for the analysis of the working principle and its response with particular reference to the positioning and the displacement. The opening and closing of the actuator is performed by the Joule effect. One of the main innovations of the proposed prototype is the possibility to modulate opening and closing just by acting on the corresponding SMA springs. As the applied voltage is changed, the opening or closing springs can be activated in order to set the desired positioning and overcome the limits of the standard configuration SMA spring—steel spring (bias).
6. Conclusions and Future Trend
In the last two decades, many applications of SMAs in the aerospace field have been identified, designed, modeled, and tested. Many others will be conceived and developed in the near future. The present review confirms that SMAs attracted researcher’s attention especially in the applications in which the added value is more important than the pure economic saving. Extensive studies, results of analytical and numerical modeling, and experimental tests have been found in literature regarding SMA. In many cases, the SMAs play the role of an actuator. Starting from the basic principle and the mechanical behavior (shape memory effect and pseudoelastic), many applications regarding the wing morphing have been presented (experimental and modeling) as well tailoring of the inlet geometry of various propulsion systems. Variable geometry chevron actuated by SMA has been illustrated for thrust, noise, and general efficiency optimization. Also, space applications have been described for isolating the micro-vibrations, for low-shock release devices and self-deployable solar sails. The development stage in many cases is limited to prototypes tested in laboratories or in wind tunnels, with only a few actually flown and even fewer in use. The main limitations may be ascribable to the higher weight, in some cases complexity of the systems, and the structural and functional fatigue phenomena in cycling applications. In the near future, many other SMA-based applications will be identified and conceived in which the actuation due to the shape memory effect or the pseudoelasticity will be based on.
Author Contributions
Conceptualization, G.C. and M.E.T.; writing—original draft preparation, G.C. and M.E.T.; writing—review and editing, G.C. and M.E.T.; supervision, G.C. and M.E.T. All authors have read and agreed to the published version of the manuscript.
This research received no external funding.
Conflicts of Interest
The authors declare no conflict of interest.
- DOI: 10.1016/J.MATDES.2013.11.084
- Corpus ID: 108440671
A review of shape memory alloy research, applications and opportunities
- J. M. Jani , M. Leary , +1 author M. Gibson
- Published 1 April 2014
- Materials Science, Engineering
- Materials & Design
Figures and Tables from this paper
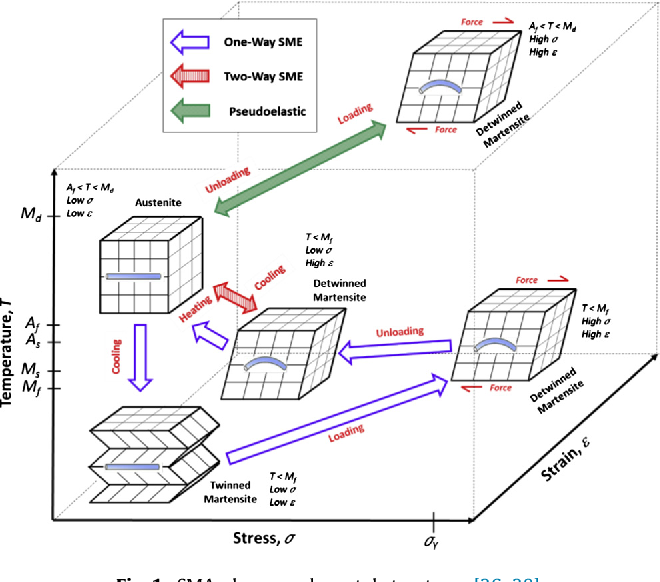
3,095 Citations
Shape memory alloy properties, modelling aspects and potential applications - a review, shape memory alloys (sma) for automotive applications and challenges, a review on shape memory alloys with applications to morphing aircraft, a half a decade timeline of shape memory alloys in modeling and applications, application of shape memory alloys in engineering – a review.
- Highly Influenced
Introduction to Shape-Memory Alloys
Shape memory alloys for space applications, a review on additive manufacturing of shape-memory materials for biomedical applications, multifunctional properties of shape memory materials in civil engineering applications: a state-of-the-art review, 519 references, shape‐memory materials: a review of technology and applications, design of space systems using shape memory alloys, applications of shape memory alloys in civil structures, a new look at biomedical ti-based shape memory alloys., development of ni-ti based shape memory alloys for actuation and control, non-medical applications of shape memory alloys, smartflex niti wires for shape memory actuators, design and application of shape memory actuators, shape memory materials : state of the art and requirements for future applications, review of mechanics of shape memory alloy structures, related papers.
Showing 1 through 3 of 0 Related Papers
Thank you for visiting nature.com. You are using a browser version with limited support for CSS. To obtain the best experience, we recommend you use a more up to date browser (or turn off compatibility mode in Internet Explorer). In the meantime, to ensure continued support, we are displaying the site without styles and JavaScript.
- View all journals
- Explore content
- About the journal
- Publish with us
- Sign up for alerts
- Open access
- Published: 15 August 2024
Self-supervised probabilistic models for exploring shape memory alloys
- Yiding Wang 1 ,
- Tianqing Li 1 ,
- Hongxiang Zong ORCID: orcid.org/0000-0002-0139-4078 1 ,
- Xiangdong Ding ORCID: orcid.org/0000-0002-1220-3097 1 ,
- Songhua Xu 2 ,
- Jun Sun ORCID: orcid.org/0000-0001-9127-088X 1 &
- Turab Lookman ORCID: orcid.org/0000-0001-8122-5671 1 , 3
npj Computational Materials volume 10 , Article number: 185 ( 2024 ) Cite this article
184 Accesses
Metrics details
- Computational methods
- Metals and alloys
- Phase transitions and critical phenomena
Recent advancements in machine learning (ML) have revolutionized the field of high-performance materials design. However, developing robust ML models to decipher intricate structure-property relationships in materials remains challenging, primarily due to the limited availability of labeled datasets with well-characterized crystal structures. This is particularly pronounced in materials where functional properties are closely intertwined with their crystallographic symmetry. We introduce a self-supervised probabilistic model (SSPM) that autonomously learns unbiased atomic representations and the likelihood of compounds with given crystal structures, utilizing solely the existing crystal structure data from materials databases. SSPM significantly enhances the performance of downstream ML models by efficient atomic representations and accurately captures the probabilistic relationships between composition and crystal structure. We showcase SSPM’s capability by discovering shape memory alloys (SMAs). Amongst the top 50 predictions, 23 have been confirmed as SMAs either experimentally or theoretically, and a previously unknown SMA candidate, MgAu, has been identified.
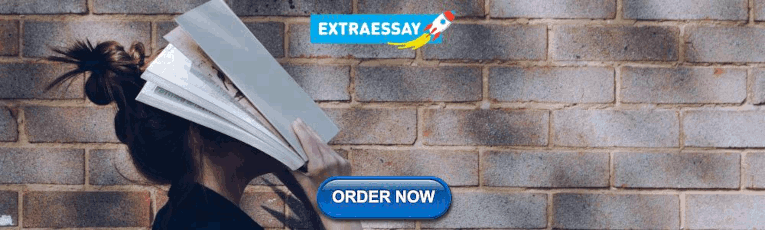
Similar content being viewed by others

MLMD: a programming-free AI platform to predict and design materials
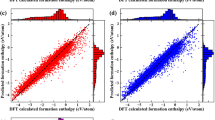
Topological representations of crystalline compounds for the machine-learning prediction of materials properties
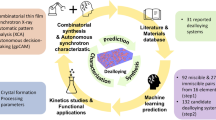
Machine-learning for designing nanoarchitectured materials by dealloying
Introduction.
Recent years have witnessed remarkable progress in the fourth paradigm of materials science through data-driven methodologies 1 . The driving force behind this is the need to incorporate the developments and success of data science and machine learning methods to rapidly design better-performing materials 2 , 3 , 4 , 5 . An important aspect is the intrinsic link between specific material functionalities and their crystal structures. A significant subset of materials display properties intricately tied to their crystal structure. For example, cuprates with layered crystal structures are more likely to show high-temperature superconductivity 6 . The functional properties of shape memory alloys (SMAs) are intricately tied to the transformation of crystal structures from high-symmetry phases (i.e., B2, D03 or L12 in most cases 7 ) to low-symmetry phases, exhibiting a group-subgroup relationship to guarantee the reversibility of the transformation 8 , 9 . Bearing this in mind, the ability to establish a structure-composition-property relationship in materials is particularly important for new materials discovery.
Utilizing representations from crystal graphs or structural descriptors, initial efforts have been to directly map the relationship between crystal structures and properties 10 , 11 , 12 , 13 , 14 . However, a common challenge in materials design is the scarcity of labeled crystal structures, limiting the application of structure-based ML models. While experimental measurement of desired properties with well characterized crystal structures are labor-intensive, computational methods like density functional theory (DFT), in combination with heuristic structure prediction tools such as CALYPSO 15 and USPEX 16 , can accurately predict stable crystal structures for given compositions by eliminating redundant experimental cycles. Nevertheless, these methods are also time-consuming when predicting properties such as the shape memory effect, requiring frequent adjustments to the crystal structure guided by phonon dispersion data 17 , 18 . It is evident that the knowledge of stable structures provides important information regarding the true interatomic interactions present 19 . In this sense, seamlessly weaving in the intricate details of crystallography with composition can bridge the gap between experimental and computational approaches.
In this study, we introduce a self-supervised probabilistic model (SSPM) framework designed to extract knowledge from unlabeled crystal structures, thereby incorporating structural information to search for new compounds. Taking advantage of the large number of unlabeled crystal structures in materials databases 20 , 21 , 22 , 23 , we devise a self-supervised learning graph neural network (Fig. 1a ) to acquire effective atomic representations (Fig. 1c ) and to determine conditional probability distributions for each element within a given crystal structure. Upon comprehensive evaluation using several composition-only benchmark datasets 24 , our method outperforms other atomic representations. Additionally, we construct a Bayesian network representing the probabilistic relationship between material compositions and crystal structures (Fig. 1d ), based on the output of the pre-trained GNN with the input of created crystal structures (Fig. 1b ). Therefore, the likelihood of compositions (C) given a crystal structure (S) of interest ( \(P({C|S})\) ) can be deduced. \(P({C|S})\) realizes predictions of the compositions with a specific crystal structure, with results validated through DFT calculations or experimental methods. By leveraging atomic representations and \(P({C|S})\) , we can design new materials more efficiently (Fig. 1e ). We showcase SSPM by applying it towards the discovery of SMA binary compounds. Of the top 50 SMA binary compounds predicted by SSPM, 23 have been confirmed by independent experimental or theoretical methods that cover almost all known SMA binary compounds. Moreover, we also discover MgAu as a new SMA compound.
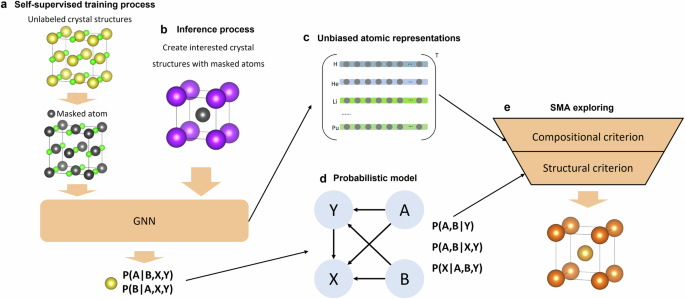
a Training process: Unlabeled crystal structure representations are learnt by self-supervised learning algorithms using GNN. b Inference process: Create different crystal structures and then feed them into the trained GNN. c Atomic representations are obtained from the trained GNN. d The probabilistic model representing the relationships between composition and crystal structure. Conditional probabilities are mathematically established in binary materials, i.e., P(A,B|Y) , P(A,B|X,Y) and P(X|A,B,Y) (where A and B are atom types at A and B sites in a binary material respectively, X is lattice parameters and Y is crystal structure). e Compositional and structural criteria are constructed based on the atomic representations and the conditional probabilities, respectively. New SMAs are explored efficiently by combining these two criteria.
Self-supervised learning graph neural network
A notable feature of our method is its ability to extract knowledge from unlabeled crystal structures. In periodic 3D crystal structures, both atomic information and bonding interactions can be effectively represented using crystal graphs. Thus, graph neural networks (GNNs) can serve as end-to-end algorithms to map the relationship between crystal structures and properties 10 , 11 , 12 , 13 , 14 . Nevertheless, these algorithms typically are not suitable for unlabeled crystal structures. However, it’s noteworthy that an atom’s intrinsic properties can be deduced from the stable crystal environments it occupies, implying that both physical and bonding information of atoms are embedded within unlabeled crystal structures. To extract this information, we can associate pseudo labels to the samples, where these pseudo labels differentiate the types of elements, thereby enabling the GNNs to be self-trained without the reliance on expensive labeling. This is self-supervised learning (SSL), which has found wide application in areas such as natural language processing and computer vision 25 , 26 , 27 , 28 .
Here we develop an SSL-GNN model to acquire atomic information from unlabeled crystal structures 29 , 30 , 31 , 32 , 33 . Firstly, as shown in Fig. 2a , we mask all atoms of a specific element for each crystal to generate pseudo-labeled crystal structure samples. As is illustrated, atoms of elements A and B are masked in the first crystal structure, whereas atoms of elements B and C are masked in the second crystal structure. The labels of these four samples are A, B and B, C.
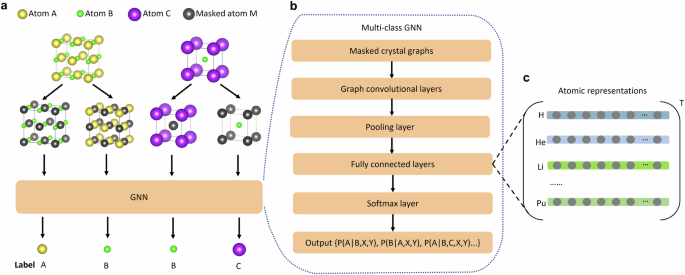
a SSL task: For a complete crystal structure, all atoms of a specific element are masked. In the first crystal structure, atoms of elements A and B are masked. In the second crystal structure, atoms of elements B and C are masked. The type of the masked element is the pseudo label of the masked crystal structure samples. b Multi-class GNN architecture: Graph convolutional, pooling, fully connected and Softmax layers are used in sequence. Output of GNN is P(A|B,X,Y) , P(A|B,C,X,Y) and P(A|B,C,D,X,Y) for binary, ternary, and quaternary materials, respectively. c Columns of the fully connected layer are encoded as a 128 dimensional atomic representation.
Next, the masked crystal structures are represented by graphs and are then input into a multi-class GNN model, which predicts the type of the masked element. The corresponding architecture of the GNN model is shown in Fig. 2b . The crystal graphs of masked crystal structures are fed into graph convolutional layers. These convolutional layers can encode information from neighboring atoms and bonds using a nonlinear graph convolution function. A pooling layer is then used for producing an overall feature vector for the crystal. Subsequent to the convolutional and pooling layers, fully connected layers are incorporated to capture the intricate mapping between crystal structure and the type of masked atom. Finally, a softmax output layer is at the end of the network to maximize the classification of the masked elements. Thus, this layer naturally estimates the probability distribution of the types of masked elements within a given crystal structure environment. For example, \(P({A|B},X,Y)\) , \(P({A|B},C,X,Y)\) and \(P({A|B},C,D,X,Y)\) for binary, ternary and quaternary materials are yielded by the SSL-GNN, respectively, where A, B, C and D represent the atom types at different sites in a crystal compound, respectively, and where X and Y denote the lattice parameters and crystal structure, respectively. The SSL-GNN is then trained on the calculated crystal structures sourced from the Materials Project (MP) 20 . For our database construction, we compiled 86,913 unlabeled crystal structures from MP, covering a range of binary, ternary, and quaternary compounds with 94 different chemical elements (Supplementary Fig. 1 ). We specifically collected structures that are stable or metastable characterized by a formation energy less than zero. As there can be any number of chemical elements in a graph, the GNN is not limited to these compounds and can be extended to systems with more elements if needed.
Atomic representation
The SSL-GNN is capable of encoding atomic representations to improve the performance of downstream property-predictive models. This diverges from typical scenarios observed with SSL models, which are commonly transferred to downstream tasks right after pre-training, as in natural language processing or computer vision. Inspired by the Word2Vec 34 , 35 approach, the weights of the output matrix in a well-pre-trained neural network encode information for each class. Therefore, we use the columns of the weights from the last fully connected layer within the pre-trained SSL-GNN to serve as atomic representations (Fig. 2c ). In SSL-GNN, graph convolutional and pooling layers extract chemical and structural environmental information, which is then encapsulated by the fully connected layer. Consequently, atoms appearing in similar environments should have similar representations, while those in different environments exhibit distinct representations. Thus, our method encodes more precise environmental information compared to Atom2Vec, which focuses solely on compositional information 36 , and SkipAtom, which is limited to nearest-neighbor atom pairs 37 . Contrasting with Mat2Vec, which tokenizes atomic vectors similar to those in natural language processing 38 , our method learns directly from crystal structures, providing a more intuitive and interpretable strategy. Moreover, our representations are learnt from unlabeled data in a self-supervised manner which is naturally prevented from being biased to one certain aspect. In addition, unlike hand-crafted materials descriptors that rely on the expertise of researchers, our method autonomously learns from data, thereby attaining a higher level of artificial intelligence.
To evaluate the effectiveness of the atomic representations, we first focus on their interpretability, i.e., the correlation between the atomic representations and the elements’ physical properties. The SSPM atomic representations are dimensionally reduced to 5D using Isomap, Locally Linear Embedding (LLE), and Principal Component Analysis (PCA). Figure 3 illustrates the Spearman correlations between these 5D vectors and commonly referenced physical properties 3 . The dimensionally-reduced atomic representation shows strong correlations with these physical properties. Notably, the atomic radius and electronegativity give the most significant correlation with the dimensionally-reduced representation, as indicated by correlations of −0.70 and 0.63 in the second dimension of LLE. This aligns with the permutation feature importance analysis for crystal structure 39 , which suggests a crucial role for atomic radius and electronegativity. It is also important to highlight that the atomic representations maintain strong correlations with physical properties even without dimensional reduction, as shown in Supplementary Fig. 2 . This highlights the reason for deriving atomic representations learnt from crystal structures, enabling human experts to gain a deeper understanding of SSPM.
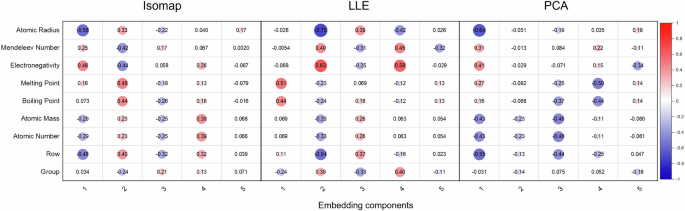
Spearman correlations between dimensionally-reduced atomic vectors and physical properties.
To evaluate their effectiveness, a range of downstream materials ML tasks are employed. Table 1 showcases a comparative analysis of the SSPM atomic representation against other material descriptors. The results reveal that, in most cases, SSPM outperforms other types of descriptors, with an average improvement over one-hot vectors, physical property features 4 , Atom2Vec 36 , SkipAtom 37 and Mat2Vec 38 of 1.27, 5.22, 2.53, 2.81, and 2.99%, respectively. The average improvement over the one-hot vectors is marginal as the one-hot vector performance for the Steels dataset is an outliner and significantly outperforms all the other representations. Nevertheless, our method fairs better than all the other approaches. Notably, SSPM atomic representations show lower errors for the Expt_gap dataset, achieving a significant reduction in Mean Absolute Error (MAE) of 9.91, 13.99, 11.11, 6.29, and 7.44%, respectively. However, for the glass dataset, our representation only outperforms physical property features. Although the SSL-GNN can be trained by non-symmetric systems using larger graph representation, it is now trained exclusively on symmetric crystal structures from the MP using primitive unit cell graph, suggesting that SSPM primarily captures periodic and long-range structural information, rather than the short-range order that is crucial for glass materials. This indicates that SSPM is suitable for crystalline functional materials. For example, a typical microstructure of SMAs involves alternating layers of periodic, long-range martensitic variants 40 , which are key to the presence of the shape memory effect and superelasticity.
Probabilistic model of composition and crystal structure
A crystal stability criterion can further improve the screening efficiency based on a composition-only predictive ML model. Notably, our SSL-GNN not only offers atomic representations but also provides conditional probabilities, such as \(P({A|B},X,Y)\) and \(P({B|A},X,Y)\) , as shown in Fig. 2b . Specifically, given a crystal structure, many different realizations with different lattice parameters can be created and input into the pre-trained GNN model. Subsequently, \(P({A|B},X,Y)\) and \(P({B|A},X,Y)\) can be obtained as the output (Fig. 1b ). From this approach, we can estimate the probability of stability for various compositions within a particular crystal structure through probabilistic graphical models (PGMs) or Bayesian networks. The top-left corner of Fig. 4 shows a PGM for binary compounds, A N B 1-N with lattice parameters X and crystal structure Y. In this PGM, A, B, X and Y are represented as nodes, while the directed edges between two nodes signify their conditional dependencies. Based on this, the conditional probability for the compositions of elements A, B given crystal structure Y and lattice parameter X, \(P(A,{B|X},Y)\) , can be inferred by solving linear Eq. ( 1 ).
where the first two equations represent the sum rule, the third equation is normalization, and the last two equations represent the product rule. We use least-squares to solve these overdetermined equations. Through a maximum likelihood estimate (MLE), we can determine the conditional probability density function for lattice parameter \(p({X|Y})\) for a given crystal structure, assuming that \(p({X|Y})\) follows a normal distribution (Supplementary Fig. 3 ). Furthermore, using the product and sum rules, we can derive \(P(A,{B|Y})\) , which is the conditional probability for the composition of elements A, B given crystal structure Y, and \(p({X|A},B,Y)\) , which is the conditional probability density of the lattice parameter X of a material with compositions of elements A, B and crystal structure Y, as shown in Fig. 4 . Computational details are given in the Methods section. Note that this methodology can be extended to multicomponent compounds with more complicated large-scale overdetermined linear equations and Gibbs sampling (Supplementary Section 3 ). From the conditional probabilistic model, three key metrics of crystal structures can be deduced as follows:
\({{ArgMax}}_{a,b}P(A,{B|X},Y)\) predicts the compositions of elements A, B given crystal structure Y and lattice parameter X;
\({{ArgMax}}_{a,b}P(A,{B|Y})\) predicts the composition of elements A, B given crystal structure Y;
\({{ArgMax}}_{x}p({X|A},B,Y)\) predicts the lattice parameter X of a material with compositions of elements A, B and crystal structure Y.
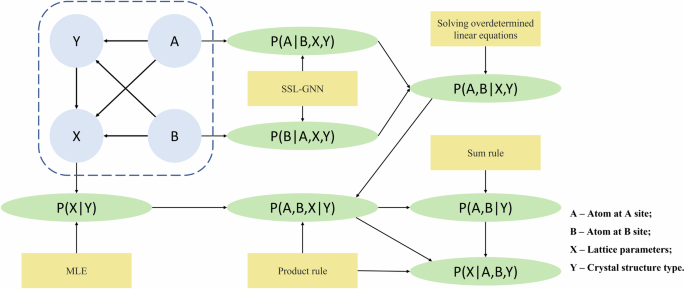
The Bayesian network is at the top-left corner of the figure. The conditional dependencies within A, B, X and Y are represented as a directed graph. P(A|B,X,Y) and P(B|A,X,Y) are obtained by SSL-GNN; P(A,B|X,Y) is calculated by solving overdetermined linear equations; P(X|Y) is determined through MLE; P(A,B,X|Y) and P(X|A,B,Y) are computed using the product rule and P(A,B|Y) is obtained via the sum rule.
We describe via an example the prediction of stability of a crystal and its lattice parameter. For a B2 structure, we first traverse and rank all possible compositions of \(P(A,{B|X}=2.99,Y=B2)\) . We performed pairwise combinations of all 69 metallic elements among the first 94 chemical elements. This resulted in a total of 69 × 68 ÷ 2 = 2346 possible combinations. Figure 5A shows the top 10 predictions, confirmed as stable via DFT calculations. Moreover, the optimized lattice parameters closely match the input X, indicated by a small MAE of 0.019 Å. Furthermore, SSPM is also good at screening the possible compositions of compounds with B2 crystal structure. This is shown by the top 10 compounds for \(P\left(A,B,|Y=B2\right)\) , all of which meet the criterion of crystal stability based on DFT calculations (Fig. 5B ). Additionally, the lattice parameters for specific compositions and crystal structure deduced from \(p({X|A},B,Y=B2)\) have a high degree of confidence. As shown in Fig. 5C , the predicted lattice parameters of TiNi, CuZr and RuNb B2 compounds have an MAE of 0.01 Å (Similalry, see Supplementary Figs. 4 , 5 and 8 for Y = D0 3 , L1 2 and Heusler from SSPM).
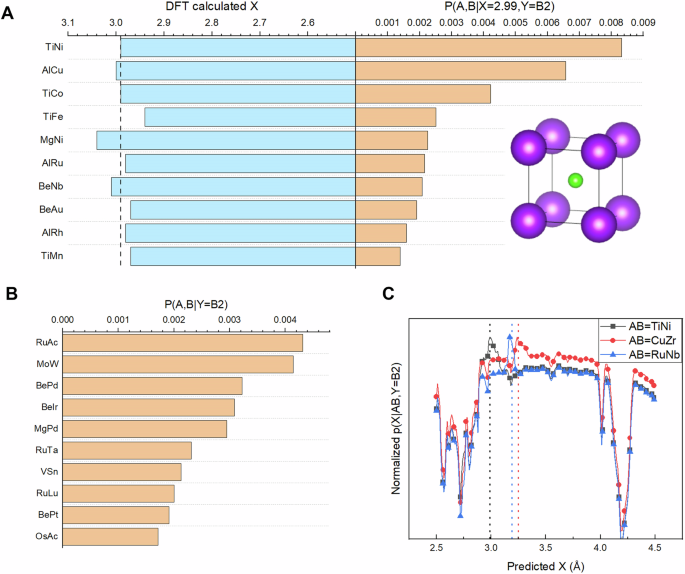
A Top 10 compositions of P(A,B|X = 2.99,Y = B2) . The right part shows the probability for each composition. The left part shows the DFT calculated X compared to the input X (dashed line). Inset is the crystal structure for B2. B Top 10 compositions for P(A,B|Y = B2) . C Predictions for p(X|A,B,Y = B2) , where AB = TiNi, CuZr and RuNb, respectively. The X-coordinate of the highest point in each curve corresponds to the predicted X and the dashed line is to the true X from ICSD 21 .
SMA exploration by SSPM
We apply SSPM to build a structure-based model for exploring possible SMA compounds. An SMA compound is a representative crystal with representative composition, without composition regulation or doping. First, we build a composition binary classifier similar to ElemNet 2 , where the input is the mole fraction weighted SSPM atomic representations for a specific alloy composition and the output is a binary label of SMA or not (hereafter we refer to this model as a composition-only SMA classifier). Our training dataset was constructed by referring to several previous reports that meticulously compiled experimental data from the published literature. The dataset consists of 658 SMAs and 213 other alloys (Supplementary Fig. 6 ). We note that the accuracy of the composition-only SMA classifier is 98.92% in this dataset, outperforming ElemNet using other materials descriptors (Supplementary Table 1 ). However, this composition-only SMA classifier still falls short in predicting “new” SMA compounds that are not included in the training dataset. The left side of Fig. 6A lists the top 30 compounds of 1:1 stoichiometry predicted by the composition-only SMA classifier, and only 10 compositions are SMAs according to existing experiments 41 , 42 , 43 , 44 , 45 , 46 , 47 , 48 , 49 , 50 (Supplementary Table 2 ). This indicates that the precision of the composition-only SMA classifier is only 33.33%. This limitation arises from the fact that although the SMA dataset contains 871 compositions, it comprises only a small number of SMA compounds. Specifically, the known SMA compounds make up merely 0.2% of the entire binary composition space, creating significant challenges in extending these findings to other compositions (Supplementary Fig. 7 ).
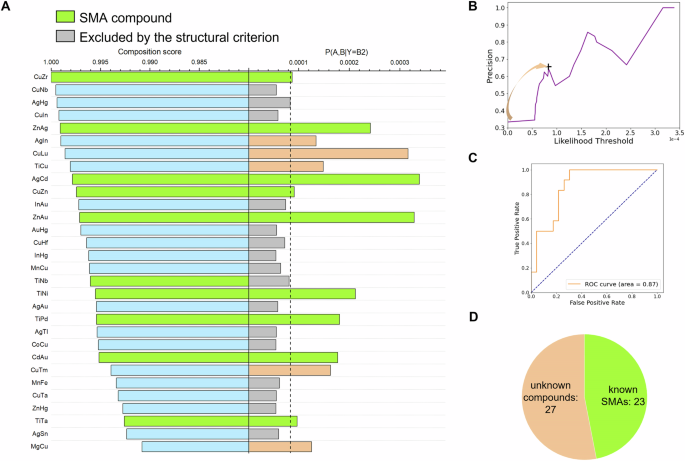
A Top 30 compounds predicted by the composition-only SMA classifier. After applying a structural criterion (dashed line), some of the compounds are excluded (gray bar). B Changes of precision with likelihood threshold. Precision = TP/(TP + FP) , where TP represents the true positive rate and FP the false positive rate. C ROC curve of the structural criterion for the top 30 compounds predicted by the composition-only SMA classifier. D Pie chart of the top 50 predictions with 1:1 or 1:3 stoichiometry.
Subsequently, an SSPM-based structural criterion is created to improve the performance of new SMA design. For binary SMAs, the crystal structures of their parent phase are B2, D0 3 and L1 2 in most cases 7 . Thus, we deduced the conditional probabilities for \(P(A,B{\rm{|}}Y=B2)\) , \(P(A,B{\rm{|}}Y={D0}_{3})\) and \(P(A,B{\rm{|}}Y={L1}_{2})\) , which provide the likelihood of specific compositions for the three different crystal structures. The right side of Fig. 6A lists the likelihood of \(P(A,B{\rm{|}}Y=B2)\) for the top 30 compounds that are predicted by the composition-only SMA classifier. We then investigated the performance of the structure-based model as a function of the likelihood threshold (LT) of \(P(A,B{\rm{|}}Y=B2)\) . Here, the likelihood threshold is a hyperparameter for optimizing the structural criterion. As shown in Fig. 6B , the precision of the structure-based model improves as a function of LT. We note that the precision rises sharply from 33.33 to 64.29% as LT increases to 8.34 × 10 −5 . Using this LT for the structural criterion, 16 candidates are excluded from the top 30 compounds predicted by the composition-only SMA classifier, which are shown by gray bars in Fig. 6A . Remarkably, amongst the 14 remaining candidates, 9 are recognized as SMA compounds, with the exception of TiNb, which does not display a B2 structure. This result suggests that the integration of a structural criterion into the composition-only ML model can efficiently improve the performance of SMA design. This is further confirmed by the areas under the receiver operating characteristic (ROC) curve (Fig. 6C ). We also employ the structure-based model to identify potential shape memory alloys (SMAs) in binary compounds with a 1:3 stoichiometry, i.e., alloys with crystal structures of D0 3 or L1 2 . Integrating both aspects together, we list the top 50 predictions, of which 23 SMAs have either experimentally or theoretically been studied, encompassing most of the common SMA binary compounds 41 , 42 , 43 , 44 , 45 , 46 , 47 , 48 , 49 , 50 , 51 , 52 , 53 , 54 , 55 , 56 , 57 , 58 , 59 , 60 (Fig. 6D , Supplementary Tables 3 and 4 ).
We further verified the unknown candidates in the top 50 binary compounds with theoretical methods. We performed DFT calculations to identify SMA compounds with energetic and dynamical stabilities for both the parent and martensitic phases, as well as their structural compatibility, which is expected to be related to the functional stability. Figure 7a shows the optimized B2 crystal structure for MgAu and its corresponding phonon dispersion. The presence of imaginary frequencies indicates that the B2-MgAu phase is dynamically unstable at 0 K. Nevertheless, the corresponding martensitic phase, whose crystal structure is achieved by distorting the B2 structure guided by the eigenvectors of its phonon dispersion, exhibits a tetragonal crystal that is dynamically stable with no imaginary modes in its phonon dispersion (Fig. 7B ). More importantly, the parent B2 and product tetragonal lattices satisfy a group-subgroup relationship. Both the determinant (1.0152) and the second eigenvalue (λ 2 = 0.9995) of the corresponding distortion matrix are close to 1, suggesting a low volume change and small interphase distortion upon phase transition. We thus predict MgAu to be an SMA.
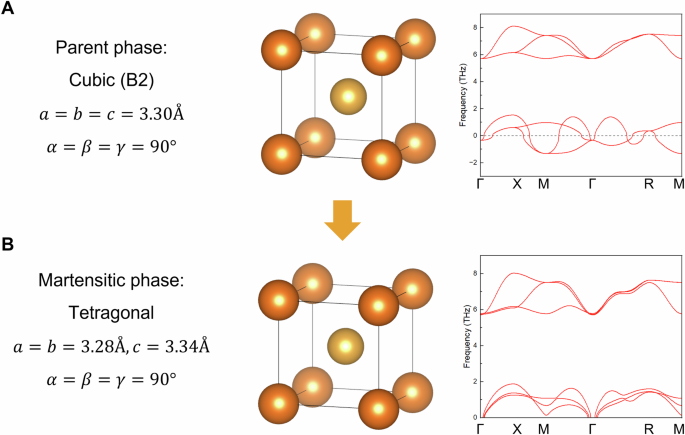
A Crystal structure of the parent phase and corresponding phonon dispersion. B Crystal structure of the martensitic phase and corresponding phonon dispersion.
We have introduced a framework (SSPM), that extracts information from unlabeled crystal structures to find possible SMAs. On one hand, SSPM utilizes the SSL-GNN architecture to create effective atomic representations, which significantly improve the performance of composition-only downstream ML models. On the other hand, it employs a Bayesian network to establish probabilistic relationships between compositions and crystal structures, offering a systematic method for integrating domain knowledge of crystal structures into materials design. Although SSPM can capture the probabilistic relationships between compositions and crystal structures of compounds, adapting this framework to solid solutions presents challenges. One could overcome such limitations by devising tailored representations for solid solutions and refining the SSL model with datasets of such materials. SSPM provides an innovative integration of deep learning and probabilistic model in the field of materials science, promising to extend its utility to a broader spectrum of functional materials besides SMAs. These include, but are not limited to, superconductors with layered crystal structures and photoelectric materials with perovskite crystal structures, highlighting the framework’s potential to improve the design of materials with intricate crystallographic dependencies.
Evaluation of the atomic representations
To evaluate the physical characteristics, the atomic representations were dimensionally-reduced to 5D vectors using scikit-learn 61 . In the dimensionality reduction processes, such as Isomap and LLE, five neighbors were considered for each data point. The spearman correlation coefficients were calculated using the formula:
where v denotes the rank of dimensionally-reduced vectors, p denotes the rank of physical properties.
To evaluate the performance, the atomic representations were mean-pooled and served as inputs to a 17-layer feed-forward neural network, as conceptualized in ElemNet 2 , 37 . This architecture included four layers of 1024 neurons each, three layers of 512 neurons, three layers of 256 neurons, three layers of 128 neurons, two layers of 64 neurons, and a final layer of 32 neurons, all activated by ReLU functions. The regression task’s output layer featured a single neuron with linear activation, whereas the classification task’s output layer had a single neuron with sigmoid activation (as only binary classification was performed). L2 regularization was employed with a regularization constant of 10 −5 . The training objective was to minimize MAE for regression tasks and cross-entropy loss for classification tasks. Training parameters included a mini-batch size of 32, a learning rate of 10 −4 , and the use of the Adam optimizer with an epsilon parameter of 10 −8 . The process involved 10-fold cross-validation.
Detailed operations and modules for SSL-GNN
The masked crystal structures are represented by using primitive unit cell graph with periodic symmetry similar to those in CGCNN 10 , where nodes represent atoms and edges represent bonds. The GNN framework comprises three graph convolutional layers with an atom feature length of 64, followed by a sum pooling layer, a fully connected layer with 128 neurons, a softplus activation layer incorporating a 50% dropout, another fully connected layer of 128 neurons, and concluding with a log softmax layer. The training objective was to reduce the negative log-likelihood loss associated with the classification of masked atoms. Training parameters included a mini-batch size of 64, a learning rate of 0.01, and the use of the stochastic gradient descent optimizer with a momentum of 0.9. For training, 80% of the unlabeled crystal data was used.
Inference of the probabilistic model
By applying Eqs. ( 3 )–( 5 ), \(P(A,{B|Y})\) and \(p({X|A},B,Y)\) can be deduced. Equations ( 3 ) and ( 5 ) are derived using the product rule, while Eq. ( 4 ) is established based on the sum rule and an approximation of normal distribution.
DFT simulation details
First-principles calculations were conducted using the projector augmented wave (PAW) method within the Vienna ab-initio simulation package (VASP). The energy cutoff was established at 500 eV, and K points were distributed using a KSPACING of 0.15. The optimization of the cells’ volume, shape, and internal atomic coordinates proceeded until the energy variation was less than 10 −8 eV. The crystal structure is stable (or metastable) if the optimized structure is the same as the expected one. Phonon assessments were executed within the harmonic approximation framework on the lattice, utilizing the PHONOPY software 62 , 63 . This study employed a combination of the supercell approach and density functional perturbation theory for phonon frequency calculations. Specifically, force constants for an alloy were determined by applying density functional perturbation theory at the Γ point within a supercell model of the alloy, from which phonon frequencies were subsequently calculated.
Data availability
The unlabeled crystal structures are collected from MP. Their material ID are available in the GitHub repository at https://github.com/Yidingwyd/SSPM .
Code availability
The source codes for the workflow of the SSPM are available in the GitHub repository at https://github.com/Yidingwyd/SSPM .
Iten, R., Metger, T., Wilming, H., Del, R. L. & Renner, R. Discovering Physical Concepts with Neural Networks. Phys. Rev. Lett. 124 , 010508 (2020).
Article CAS PubMed Google Scholar
Jha, D. et al. ElemNet: Deep Learning the Chemistry of Materials From Only Elemental Composition. Sci. Rep. 8 , 17593 (2018).
Article PubMed PubMed Central Google Scholar
Ward, L., Agrawal, A., Choudhary, A. & Wolverton, C. A general-purpose machine learning framework for predicting properties of inorganic materials. npj Comput. Mater. 2 , 10 (2016).
Article Google Scholar
Goodall, R. E. A. & Lee, A. A. Predicting materials properties without crystal structure: deep representation learning from stoichiometry. Nat. Commun. 11 , 6280 (2020).
Article CAS PubMed PubMed Central Google Scholar
Zeng, S. et al. Atom table convolutional neural networks for an accurate prediction of compounds properties. npj Comput. Mater. 5 , 84 (2019).
Habermeier, H. U. Science and technology of cuprate-based high temperature superconductor thin films, heterostructures and superlattices—the first 30 years (Review Article). Low. Temp. Phys. 42 , 840–862 (2016).
Article CAS Google Scholar
Notomi, M., Van, V. K. J. & Yip, S. Classification and Characterization of the Shape Memory Binary Alloys. MRS Proc. 980 , 0980-II0904-0907 (2006).
Otsuka, K. & Kakeshita, T. Science and Technology of Shape-Memory Alloys: New Developments. MRS Bull. 27 , 91–100 (2002).
Bhattacharya, K., Conti, S., Zanzotto, G. & Zimmer, J. Crystal symmetry and the reversibility of martensitic transformations. Nature 428 , 55–59 (2004).
Xie, T. & Grossman, J. C. Crystal Graph Convolutional Neural Networks for an Accurate and Interpretable Prediction of Material Properties. Phys. Rev. Lett. 120 , 145301 (2018).
Chen, C., Ye, W., Zuo, Y., Zheng, C. & Ong, S. P. Graph Networks as a Universal Machine Learning Framework for Molecules and Crystals. Chem. Mater. 31 , 3564–3572 (2019).
Choudhary, K. & DeCost, B. Atomistic Line Graph Neural Network for improved materials property predictions. npj Comput. Mater. 7 , 185 (2021).
Jiang, Y. et al. Topological representations of crystalline compounds for the machine-learning prediction of materials properties. npj Comput. Mater. 7 , 28 (2021).
Schütt, K. T. et al. How to represent crystal structures for machine learning: Towards fast prediction of electronic properties. Phys. Rev. B 89 , 205118 (2014).
Tong, Q., Lv, J., Gao, P. & Wang, Y. The CALYPSO methodology for structure prediction. Chin. Phys. B 28 , 106105 (2019).
Oganov, A. R. & Glass, C. W. Crystal structure prediction using ab initio evolutionary techniques: principles and applications. J. Chem. Phys. 124 , 244704 (2006).
Article PubMed Google Scholar
Huang, X., Bungaro, C., Godlevsky, V. & Rabe, K. M. Lattice instabilities of cubic NiTi from first principles. Phys. Rev. B 65 , 014108 (2001).
Huang, X., Rabe, K. M. & Ackland, G. J. First-principles study of the structural energetics of PdTi and PtTi. Phys. Rev. B 67 , 024101 (2003).
Fischer, C. C., Tibbetts, K. J., Morgan, D. & Ceder, G. Predicting crystal structure by merging data mining with quantum mechanics. Nat. Mater. 5 , 641–646 (2006).
Jain, A. et al. Commentary: The Materials Project: A materials genome approach to accelerating materials innovation. APL Mater. 1 , 011002 (2013).
Zagorac, D., Müller, H., Ruehl, S., Zagorac, J. & Rehme, S. Recent developments in the Inorganic Crystal Structure Database: theoretical crystal structure data and related features. J. Appl Crystallogr. 52 , 918–925 (2019).
Saal, J. E., Kirklin, S., Aykol, M., Meredig, B. & Wolverton, C. Materials Design and Discovery with High-Throughput Density Functional Theory: The Open Quantum Materials Database (OQMD). Jom 65 , 1501–1509 (2013).
Kirklin, S. et al. The Open Quantum Materials Database (OQMD): assessing the accuracy of DFT formation energies. npj Comput. Mater. 1 , 15010 (2015).
Dunn, A., Wang, Q., Ganose, A., Dopp, D. & Jain, A. Benchmarking materials property prediction methods: the Matbench test set and Automatminer reference algorithm. npj Comput. Mater. 6 , 138 (2020).
Devlin, J., Chang, M. W., Lee, K. & Toutanova, K. BERT: Pre-training of Deep Bidirectional Transformers for Language Understanding. Preprint at https://arxiv.org/abs/1810.04805 (2018).
Liu, X. et al. Self-supervised Learning: Generative or Contrastive. IEEE Transactions on Knowledge and Data Engineering 35 , 857–876 (2023).
Google Scholar
Jing, L. & Tian, Y. Self-supervised Visual Feature Learning with Deep Neural Networks: A Survey. IEEE Transactions on Pattern Analysis and Machine Intelligence 43 , 4037–4058 (2021).
Schmarje, L., Santarossa, M., Schröder, S. M. & Koch, R. A survey on Semi-, Self- and Unsupervised Learning for Image Classification. IEEE Access 9 , 82146–82168 (2021).
Magar, R., Wang, Y. & Barati, F. A. Crystal twins: self-supervised learning for crystalline material property prediction. npj Comput. Mater. 8 , 231 (2022).
Fu, N., Wei, L. & Hu, J. Physics-Guided Dual Self-Supervised Learning for Structure-Based Material Property Prediction. J. Phys. Chem. Lett. 15 , 2841–2850 (2024).
Aroboto, B. et al. Universal and interpretable classification of atomistic structural transitions via unsupervised graph learning. Appl. Phys. Lett. 123 , 094103 (2023).
Ishiai, S., Yasuda, I., Endo, K. & Yasuoka, K. Graph-Neural-Network-Based Unsupervised Learning of the Temporal Similarity of Structural Features Observed in Molecular Dynamics Simulations. J. Chem. Theory Comput 20 , 819–831 (2024).
Dong, Z., Feng, J., Ji, Y. & Li, Y. SLI-GNN: A Self-Learning-Input Graph Neural Network for Predicting Crystal and Molecular Properties. J. Phys. Chem. A 127 , 5921–5929 (2023).
Mikolov, T., Sutskever, I., Chen, K., Corrado, G. & Dean, J. Distributed Representations of Words and Phrases and their Compositionality. Advances in Neural Information Processing Systems (eds Burges, C. J., Bottou, L., Welling, M., Ghahramani, Z., Weinberger, K. Q.) vol 26, (Curran Associates, Inc., 2013) https://proceedings.neurips.cc/paper_files/paper/2013/file/9aa42b31882ec039965f3c4923ce901b-Paper.pdf .
Mikolov, T., Chen, K., Corrado, G. & Dean, J. Efficient Estimation of Word Representations in Vector Space. arXiv e-prints. arXiv 1301 , 3781 (2013).
Zhou, Q. et al. Learning atoms for materials discovery. Proc. Natl Acad. Sci. USA 115 , E6411–E6417 (2018).
Antunes, L. M., Grau-Crespo, R. & Butler, K. T. Distributed representations of atoms and materials for machine learning. npj Comput. Mater. 8 , 44 (2022).
Tshitoyan, V. et al. Unsupervised word embeddings capture latent knowledge from materials science literature. Nature 571 , 95–98 (2019).
Lee, K., Ayyasamy, M. V., Delsa, P., Hartnett, T. Q. & Balachandran, P. V. Phase classification of multi-principal element alloys via interpretable machine learning. npj Comput. Mater. 8 , 25 (2022).
Cui, J. et al. Combinatorial search of thermoelastic shape-memory alloys with extremely small hysteresis width. Nat. Mater. 5 , 286–290 (2006).
Song, K. K. et al. Strategy for pinpointing the formation of B2 CuZr in metastable CuZr-based shape memory alloys. Acta Mater. 59 , 6620–6630 (2011).
Cornelis, I. & Wayman, C. M. The shape memory effect in AgZn alloys. Scr. Metall. 8 , 1321–1326 (1974).
Saburi, T. & Wayman, C. M. The shape memory mechanism and related phenomena in Ag-45at.%Cd. Acta Metall. 28 , 1–14 (1980).
Schroeder, T. A. & Wayman, C. M. The formation of martensite and the mechanism of the shape memory effect in single crystals of Cu-Zn alloys. Acta Metall. 25 , 1375–1391 (1977).
Lashley, J. C. et al. The heat capacity of single-crystal AuZn near the martensitic transition. J. Chem. Thermodyn. 34 , 251–261 (2002).
Kim, H. Y., Ikehara, Y., Kim, J. I., Hosoda, H. & Miyazaki, S. Martensitic transformation, shape memory effect and superelasticity of Ti–Nb binary alloys. Acta Mater. 54 , 2419–2429 (2006).
Otsuka, K. & Ren, X. Physical metallurgy of Ti–Ni-based shape memory alloys. Prog. Mater. Sci. 50 , 511–678 (2005).
Solomon, V. & Nishida, M. Effect of Aging on Martensitic Transformation in Ti-Rich Ti–Pd Shape Memory Alloy. Mater. Trans. 43 , 897–901 (2002).
Xue, D. et al. Martensite aging effects on the dynamic properties of Au–Cd shape memory alloys: Characteristics and modeling. Acta Mater. 59 , 4999–5011 (2011).
Buenconsejo, P. J. S., Kim, H. Y., Hosoda, H. & Miyazaki, S. Shape memory behavior of Ti–Ta and its potential as a high-temperature shape memory alloy. Acta Mater. 57 , 1068–1077 (2009).
Lee, J., Ikeda, Y. & Tanaka, I. First-principles screening of structural properties of intermetallic compounds on martensitic transformation. npj Comput. Mater. 3 , 52 (2017).
Yasuda, H. Y., Fukushima, K., Aoki, M. & Umakoshi, Y. Temperature Dependence of Pseudoelasticity and Shape Memory Effect in Fe3Ga Single Crystals with D03 Structure. ISIJ Int. 48 , 1014–1019 (2008).
Oikawa, K. et al. Phase equilibria and phase transformations in new B2-type ferromagnetic shape memory alloys of Co-Ni-Ga and Co-Ni-Al systems. Mater. Trans. 42 , 014108 (2001).
Semenova, E. L. & Kudryavtsev, Y. V. Structural phase transformation and shape memory effect in ZrRh and ZrIr. J. Alloy. Compd. 203 , 165–168 (1994).
Yamabe-Mitarai, Y., Hara, T., Miura, S. & Hosoda, H. Phase transformation and shape memory effect of Ti (Pt, Ir). Metall. Mater. Trans. A 43 , 2901–2911 (2012).
Chiu, W. T., Tahara, M. & Hosoda, H. A Review of Investigations on Microstructure and Mechanical Properties of the Present Achievements of the Ti-Au-based Shape Memory Alloys. ISIJ Int. 63 , 1159–1171 (2023).
Balun, J. & Inden, G. Phase equilibria in the binary Rh–Ti system. Intermetallics 14 , 260–271 (2006).
Manzoni, A. M. et al. Shape memory deformation mechanisms of Ru–Nb and Ru–Ta shape memory alloys with transformation temperatures. Intermetallics 52 , 57–63 (2014).
Kudryavtsev, Y. V. & Semenova, O. Shape memory effect in ZrIr and Zr-Ir-Co alloys. Powder Metall. Met. Ceram. 50 , 471–478 (2011).
Xing, W. et al. First-principles studies of structural stabilities and enthalpies of formation of refractory intermetallics: TM and TM3 (T= Ti, Zr, Hf; M= Ru, Rh, Pd, Os, Ir, Pt). Intermetallics 28 , 16–24 (2012).
Pedregosa, F. et al. Scikit-learn: Machine learning in Python. J. Mach. Learn. Res. 12 , 2825–2830 (2011).
Togo, A., Chaput, L., Tadano, T. & Tanaka, I. Implementation strategies in phonopy and phono3py. J. Phys. Condens. Matter 35 , 353001 (2023).
Togo, A. First-principles Phonon Calculations with Phonopy and Phono3py. J. Phys. Soc. Jpn. 92 , 012001 (2023).
Download references
Acknowledgements
This work was supported by the National Natural Science Foundation of China (51931004, 52322103, 52350710205, and 52171011), Key Technologies R&D Program (2022YFB3707600), and the 111 project 2.0 (BP2018008). T.Q.L. acknowledges the support from the China Scholarship Council (202306280011).
Author information
Authors and affiliations.
State Key Laboratory for Mechanical Behavior of Materials, Xi’an Jiaotong University, Xi’an, 710049, China
Yiding Wang, Tianqing Li, Hongxiang Zong, Xiangdong Ding, Jun Sun & Turab Lookman
National Engineering Laboratory for Big Data Analytics, School of Mathematics and Statistics, Xi’an Jiaotong University, Xi’an, 710049, China
AiMaterials Research LLC, Santa Fe, NM, 87501, USA
Turab Lookman
You can also search for this author in PubMed Google Scholar
Contributions
H.Z. and X.D. conceived of this study. Y.W. performed the machine learning algorithms, DFT calculations and analyses. T.Q.L. assisted with coding. T.L. contributed to the shape of manuscript structure. S.X. and J.S. participated in the discussion of the main results. Y.W. and H.Z. analyzed the results and wrote the manuscript.
Corresponding authors
Correspondence to Hongxiang Zong or Xiangdong Ding .
Ethics declarations
Competing interests.
The authors declare no competing interests.
Additional information
Publisher’s note Springer Nature remains neutral with regard to jurisdictional claims in published maps and institutional affiliations.
Supplementary information
Supplementary information, rights and permissions.
Open Access This article is licensed under a Creative Commons Attribution-NonCommercial-NoDerivatives 4.0 International License, which permits any non-commercial use, sharing, distribution and reproduction in any medium or format, as long as you give appropriate credit to the original author(s) and the source, provide a link to the Creative Commons licence, and indicate if you modified the licensed material. You do not have permission under this licence to share adapted material derived from this article or parts of it. The images or other third party material in this article are included in the article’s Creative Commons licence, unless indicated otherwise in a credit line to the material. If material is not included in the article’s Creative Commons licence and your intended use is not permitted by statutory regulation or exceeds the permitted use, you will need to obtain permission directly from the copyright holder. To view a copy of this licence, visit http://creativecommons.org/licenses/by-nc-nd/4.0/ .
Reprints and permissions
About this article
Cite this article.
Wang, Y., Li, T., Zong, H. et al. Self-supervised probabilistic models for exploring shape memory alloys. npj Comput Mater 10 , 185 (2024). https://doi.org/10.1038/s41524-024-01379-3
Download citation
Received : 12 April 2024
Accepted : 04 August 2024
Published : 15 August 2024
DOI : https://doi.org/10.1038/s41524-024-01379-3
Share this article
Anyone you share the following link with will be able to read this content:
Sorry, a shareable link is not currently available for this article.
Provided by the Springer Nature SharedIt content-sharing initiative
Quick links
- Explore articles by subject
- Guide to authors
- Editorial policies
Sign up for the Nature Briefing newsletter — what matters in science, free to your inbox daily.

Information
- Author Services
Initiatives
You are accessing a machine-readable page. In order to be human-readable, please install an RSS reader.
All articles published by MDPI are made immediately available worldwide under an open access license. No special permission is required to reuse all or part of the article published by MDPI, including figures and tables. For articles published under an open access Creative Common CC BY license, any part of the article may be reused without permission provided that the original article is clearly cited. For more information, please refer to https://www.mdpi.com/openaccess .
Feature papers represent the most advanced research with significant potential for high impact in the field. A Feature Paper should be a substantial original Article that involves several techniques or approaches, provides an outlook for future research directions and describes possible research applications.
Feature papers are submitted upon individual invitation or recommendation by the scientific editors and must receive positive feedback from the reviewers.
Editor’s Choice articles are based on recommendations by the scientific editors of MDPI journals from around the world. Editors select a small number of articles recently published in the journal that they believe will be particularly interesting to readers, or important in the respective research area. The aim is to provide a snapshot of some of the most exciting work published in the various research areas of the journal.
Original Submission Date Received: .
- Active Journals
- Find a Journal
- Proceedings Series
- For Authors
- For Reviewers
- For Editors
- For Librarians
- For Publishers
- For Societies
- For Conference Organizers
- Open Access Policy
- Institutional Open Access Program
- Special Issues Guidelines
- Editorial Process
- Research and Publication Ethics
- Article Processing Charges
- Testimonials
- Preprints.org
- SciProfiles
- Encyclopedia
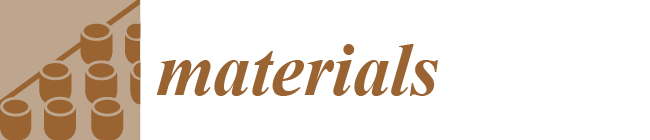
Journal Menu
- Materials Home
- Aims & Scope
- Editorial Board
- Reviewer Board
- Topical Advisory Panel
- Instructions for Authors
- Special Issues
- Sections & Collections
- Article Processing Charge
- Indexing & Archiving
- Editor’s Choice Articles
- Most Cited & Viewed
- Journal Statistics
- Journal History
- Journal Awards
- Society Collaborations
- Conferences
- Editorial Office
Journal Browser
- arrow_forward_ios Forthcoming issue arrow_forward_ios Current issue
- Vol. 17 (2024)
- Vol. 16 (2023)
- Vol. 15 (2022)
- Vol. 14 (2021)
- Vol. 13 (2020)
- Vol. 12 (2019)
- Vol. 11 (2018)
- Vol. 10 (2017)
- Vol. 9 (2016)
- Vol. 8 (2015)
- Vol. 7 (2014)
- Vol. 6 (2013)
- Vol. 5 (2012)
- Vol. 4 (2011)
- Vol. 3 (2010)
- Vol. 2 (2009)
- Vol. 1 (2008)
Find support for a specific problem in the support section of our website.
Please let us know what you think of our products and services.
Visit our dedicated information section to learn more about MDPI.
Shape Memory Alloys (SMAs) for Engineering Applications
- Print Special Issue Flyer
Special Issue Editors
Special issue information, benefits of publishing in a special issue.
- Published Papers
A special issue of Materials (ISSN 1996-1944). This special issue belongs to the section " Smart Materials ".
Deadline for manuscript submissions: closed (31 October 2020) | Viewed by 49298
Share This Special Issue

Dear Colleagues,
This Special Issue of Materials is dedicated to “Shape Memory Alloys (SMAs) for Engineering Applications”. We are expecting to receive papers dealing with cutting-edge issues on research and application of SMAs in structural engineering. The topics of the Special Issue include, but are not limited to:
- Nickel-titanium-based SMAs
- Copper-based SMAs
- Iron-based SMAs
- Aluminum-based SMAs
- Damping capacity of SMAs
- Superelasticity of SMAs
- Coupling, Fastener
- Concrete reinforcement
- Near surface mounted reinforcement
- Short fibers
- Actuator applications of SMAs in structural engineering
- Active vibration control in structural engineering using SMAs
- Hybrid composites of shape memory alloys and polymers for structural engineering
- SMAs as sensors for health monitoring of structural engineering
- Material constitutive models
- Structural behavior models
- Long term behavior models
Prof. Dr. Masoud Motavalli Dr. Christoph Czaderski Prof. Dr. Moslem Shahverdi Collection Editors
Manuscripts should be submitted online at www.mdpi.com by registering and logging in to this website . Once you are registered, click here to go to the submission form . Manuscripts can be submitted until the deadline. All submissions that pass pre-check are peer-reviewed. Accepted papers will be published continuously in the journal (as soon as accepted) and will be listed together on the special issue website. Research articles, review articles as well as short communications are invited. For planned papers, a title and short abstract (about 100 words) can be sent to the Editorial Office for announcement on this website.
Submitted manuscripts should not have been published previously, nor be under consideration for publication elsewhere (except conference proceedings papers). All manuscripts are thoroughly refereed through a single-blind peer-review process. A guide for authors and other relevant information for submission of manuscripts is available on the Instructions for Authors page. Materials is an international peer-reviewed open access semimonthly journal published by MDPI.
Please visit the Instructions for Authors page before submitting a manuscript. The Article Processing Charge (APC) for publication in this open access journal is 2600 CHF (Swiss Francs). Submitted papers should be well formatted and use good English. Authors may use MDPI's English editing service prior to publication or during author revisions.
- shape memory alloys (SMAs)
- shape memory effect (SME)
- superelasticity
- alloy design
- structural engineering
- civil engineering
- smart materials
- external strengthening
- structural rehabilitation
- constitutive models
- long term behavior
- Ease of navigation: Grouping papers by topic helps scholars navigate broad scope journals more efficiently.
- Greater discoverability: Special Issues support the reach and impact of scientific research. Articles in Special Issues are more discoverable and cited more frequently.
- Expansion of research network: Special Issues facilitate connections among authors, fostering scientific collaborations.
- External promotion: Articles in Special Issues are often promoted through the journal's social media, increasing their visibility.
- e-Book format: Special Issues with more than 10 articles can be published as dedicated e-books, ensuring wide and rapid dissemination.
Further information on MDPI's Special Issue polices can be found here .
Published Papers (15 papers)
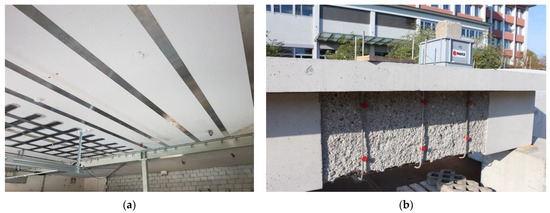
Graphical abstract
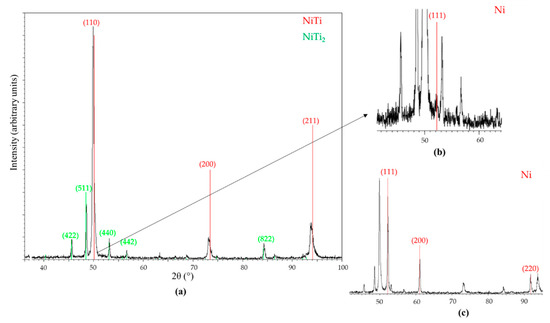
Further Information
Mdpi initiatives, follow mdpi.
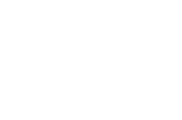
Subscribe to receive issue release notifications and newsletters from MDPI journals
Academia.edu no longer supports Internet Explorer.
To browse Academia.edu and the wider internet faster and more securely, please take a few seconds to upgrade your browser .
Enter the email address you signed up with and we'll email you a reset link.
- We're Hiring!
- Help Center
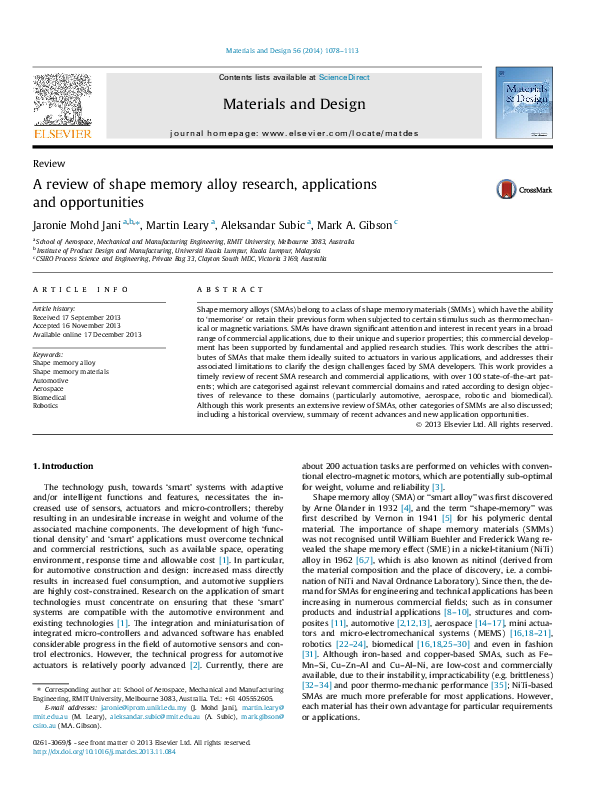
A review of shape memory alloy research, applications and opportunities

Shape memory alloys (SMAs) belong to a class of shape memory materials (SMMs), which have the ability to 'memorise' or retain their previous form when subjected to certain stimulus such as thermomechan-ical or magnetic variations. SMAs have drawn significant attention and interest in recent years in a broad range of commercial applications, due to their unique and superior properties; this commercial development has been supported by fundamental and applied research studies. This work describes the attributes of SMAs that make them ideally suited to actuators in various applications, and addresses their associated limitations to clarify the design challenges faced by SMA developers. This work provides a timely review of recent SMA research and commercial applications, with over 100 state-of-the-art patents ; which are categorised against relevant commercial domains and rated according to design objectives of relevance to these domains (particularly automotive, aerospace, robotic and biomedical). Although this work presents an extensive review of SMAs, other categories of SMMs are also discussed; including a historical overview, summary of recent advances and new application opportunities.
Related Papers
IRJET Journal
Shape memory alloys (SMAs) belong to a class of shape memory materials (SMMs), which have the ability to 'memorise' or retain their previous form when subjected to certain stimulus such as thermo mechanical or magnetic variations. SMAs have drawn significant attention and interest in recent years in a broad range of commercial applications, due to their unique and superior properties; this commercial development has been supported by fundamental and applied research studies. This work describes the attributes of SMAs that make them ideally suited to actuators in various applications, and addresses their associated limitations to clarify the design challenges faced by SMA developers. This work provides a timely review of recent SMA research and commercial applications, with over 100 state-of-the-art patents; which are categorized against relevant commercial domains and rated according to design objectives of relevance to these domains (particularly automotive, aerospace, robotic and biomedical). Although this work presents an extensive review of SMAs, other categories of SMMs are also discussed; including a historical overview, summary of recent advances and new application opportunities.
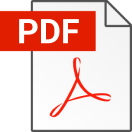
Journal of Materials Engineering and Performance
Jose M San Juan
ABSTRACT A novel shape memory alloy (SMA) has been developed as an alternative to currently available alloys. This alloy, commercially known by its proprietary brand SMARQ, shows a higher working range of temperatures with respect to the SMA materials used until now in actuators, limited to environment temperatures below 90 A degrees C. SMARQ is a high temperature SMA (HTSMA) based on a fully European material technology and production processes, which allows the manufacture of high quality products, with tuneable transformation temperatures up to 200 A degrees C. Both, material and production processes have been evaluated for its use in space applications. A full characterization test campaign has been completed in order to obtain the material properties and check its suitability to be used as active material in space actuators. In order to perform the functional characterization of the material, it has been considered as the key element of a basic SMA actuator, consisting in the SMA wire and the mechanical and electrical interfaces. The functional tests presented in this work have been focused on the actuator behavior when heated by means of an electrical current. Alloy composition has been adjusted in order to match a transition temperature (As) of +145 A degrees C, which satisfies the application requirements of operating temperatures in the range of -70 and +125 A degrees C. Details of the tests and results of the characterization test campaign, focused in the material unique properties for their use in actuators, will be presented in this work. Some application examples in the field of space mechanisms and actuators, currently under development, will be summarized as part of this work, demonstrating the technology suitability as active material for space actuators.
Proceedings of the First International Conference on Biomedical Electronics and Devices
Roberto Romano
Trans Tech Publications
Jaronie Mohd Jani
Shape memory alloy (SMA) actuators have drawn much attention and interest due to their unique and superior properties, and are expected to be equipped in many modern vehicles at competitive market prices. The key advantage is that SMA actuators do not require bulky and complicated mechanical design to function, where the active element (e.g. SMA wire or spring) can be deformed by applying minimal external force and will retain to their previous form when subjected to certain stimuli such as thermomechanical or magnetic changes. This paper describes the SMA attributes that make them ideally suited as actuators in automotive applications and to address their limitations, feasibilities and prospects.
American journal of Engineering Research (AJER)
Shape memory alloys (SMAs) are metals that "remember" their original shapes. SMAs are useful for such things as actuators which are materials that "change shape, stiffness, position, natural frequency, and other mechanical characteristics in response to temperature or electromagnetic fields" The potential uses for SMAs especially as actuators have broadened the spectrum of many scientific fields. The study of the history and development of SMAs can provide an insight into a material involved in cutting-edge technology. The diverse applications for these metals have made them increasingly important and visible to the world. This paper presents the working of shape memory alloys , the phenomenon of super-elasticity and applications of these alloys.
International Journal of Recent Technology and Engineering
Nouman Khan
SMA has drawn massive interest and hobby in today’s years in a great form of an extensive sort of commercial applications, due to their precise and superior properties, this concern improvement has been bearing with the useful resource of way of improvement and carried out research studies. SMA can heal its original shape at a certain temperature even under maximum loads applied and huge inelastic deformation. In this overview, we describe the primary functions of SMAs, their constitutive models, and their features. We also explained various properties that help to build a device/system. These devices help in cueing health issues such as heart treatment emptying urine so on. SMA has important in reducing the vibration of structures by increasing damping of the materials and this has effective in energy dissipating comparing with other materials. In the aerospace industry wing aircraft, rotorcraft, spacecraft, and micro-electromechanical systems are made up of SMA. In the automobile ...
Subash Mishra
Shape memory alloys (SMAs) impart a potential application in a wide range in micro- and nano-industrial world. Classic properties such as free recovery or pseudo-elasticity still needed further specifications in smart materials or micro-actuators applications. Nowadays, a primary goal is achieved that these materials offer a price-competitive advantage compared to other functional materials design. Among all types of functional SMAs, NiTi-based SMA attracted much attention in the rapidly growing field of micro-electro-mechanical-systems (MEMS) and bio-MEMS. This paper points out different products of Ni-Ti based SMAs. This will be illustrated by describing some device-based applications realizing why they are successful than that of other micro-instruments
Vladimir Brailovski
MRS Proceedings
Christian Boller
Loading Preview
Sorry, preview is currently unavailable. You can download the paper by clicking the button above.
RELATED PAPERS
Shape Memory Alloy Engineering
Alfonso Martone
Journal of Physics: Conference Series
Nikita Gabdullin
Science Insights
Insights Publisher
Materials Science and Engineering: A
Rudy Stalmans
Mechatronics
Eduardo Tannuri
The International Journal of Advanced Manufacturing Technology
Brahim Necib
Nicolas Chaillet , Joël Abadie
Measurement and Control
Reza Nadafi
MRS Advances
Ingrith Yuritsa Paez Pidiache
International Journal of Computational Materials Science and Surface Engineering
F. Auricchio
AIMS Materials Science
Abdelmoneim Elnaggar
Yves Bellouard
Jean-Yves Gauthier
Le Journal de Physique IV
Kęstutis Vaitasius
International Journal of Engineering Research and Technology (IJERT)
IJERT Journal
ESOMAT 1989-Ist European …
Jamal Arghavani
Ladislau augustinov , Sava Ianici
International Journal of Applied Mathematics, Electronics and Computers
Nihat Akkuş
ABIRAMI RAJAVEL PANDIAN
- We're Hiring!
- Help Center
- Find new research papers in:
- Health Sciences
- Earth Sciences
- Cognitive Science
- Mathematics
- Computer Science
- Academia ©2024
Strain Glass State in Ni50.3Ti29.7Hf20 High-Temperature Shape Memory Alloy
13 Pages Posted: 15 Aug 2024 Publication Status: Under Review
Northwestern Polytechnical University, China - State Key Laboratory of Solidification Processing
Zhongsheng Yang
Kengfeng xu.
Northwestern Polytechnical University, China - School of Materials Science and Engineering
Dingcong Cui
Northwestern Polytechnical University, China
Zhijun Wang
Jincheng wang.
NiTiHf alloy is one of the most promising candidates for high-temperature shape-memory actuators. However, the phase transition of NiTiHf is not fully understood, yet. Here, we for the first time report the strain glass state in a NiTiHf alloy. Our results demonstrate that the H-phase precursor generated during rapid cooling suppresses the normal martensitic phase transition of NiTiHf, leading to the strain glass state. Moreover, we successfully controlled the strain glass transition via changing the cooling rate. These new findings make the NiTiHf system promising for high-temperature superelastic actuators.
Keywords: Strain glass, martensitic transition, shape memory alloys, Precipitation
Suggested Citation: Suggested Citation
Northwestern Polytechnical University, China - State Key Laboratory of Solidification Processing ( email )
Northwestern polytechnical university, china - school of materials science and engineering ( email ), northwestern polytechnical university, china ( email ).
127# YouYi Load Xi'an, Shaanxi 710072 China
127 YouYi Load XiAn, Shaanxi 710072 China
Feng He (Contact Author)
Do you have a job opening that you would like to promote on ssrn, paper statistics, related ejournals, acta materialia, inc..
Subscribe to this free journal for more curated articles on this topic
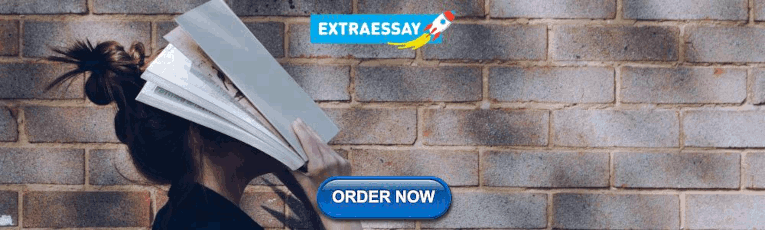
IMAGES
COMMENTS
2. Shape memory alloy overview. SMAs are a group of metallic alloys that can return to their original form (shape or size) when subjected to a memorisation process between two transformation phases, which is temperature or magnetic field dependent. This transformation phenomenon is known as the shape memory effect (SME).
This paper focuses on introducing shape memory alloy and their applications in past, present and in future, also revealed the concept and mechanism of shape memory materials for a particular requirement. Properties of SMAs, behaviour and characteristics of SMA, summary of recent advances and new application opportunities are also discussed.
Abstract Cu-based shape memory alloys belong to one important class of functional alloys, presenting shape memory effect and superelasticity due to their reversible martensitic transformation. Although they have been extensively studied since the middle of the last century, there are still many challenges to be solved. In the last decades, these alloys were extensively studied regarding new ...
A review of shape memory alloy research, applications and opportunities. April 2014; Materials & Design (1980-2015) 56:1078-1113 ... Shape memory alloys (SMAs) belong to a class of shape memory ...
Shape memory alloys (SMAs) are a class of materials that, after being deformed at a lower temperature, can recover their shape when heated above a certain temperature. Because of this ability, SMAs have been considered as alternative actuating building blocks for replacing conventional actuators, such as electric and fuel-powered motors.
Shape memory alloy (SMA) or "smart alloy" was first discovered by Arne Ölander in 1932 [4], and the term "shape-memory" was first described by Vernon in 1941 [5] for his polymeric dental material. The importance of shape memory materials (SMMs) was not recognised until William Buehler and Frederick Wang revealed the shape memory effect ...
Shape memory materials are advanced materials that are of immense interest among researchers and have vast commercial applications. Shape Memory Alloys (SMA) were initially brought to light in 1932 by Arne Ölander, whereas the title Shape Memory Alloy was first defined by vernom in 1941 [1], [2]. Shape Memory Materials tend to retain a ...
Abstract. Shape Memory Alloy is a smart alloy which retains their original shape under thermomechanical or magnetic variation. Shape Memory Alloy are widely used in different engineering field because of its superior properties and variety of application. Recent research of SMA has been applied in the field of Aerospace, Automotive, Biomedical ...
1. Introduction. Shape memory alloys (SMAs), in comparison to other materials, have the exceptional ability to change their properties, structures, and functionality, depending on the thermal, magnetic, and/or stress fields applied. As is well-known, in recent decades, the development of SMAs has allowed innovative solutions as alternatives in ...
Shape memory alloys (SMAs), as previously indicated, have bee n used in a variety of high per formance. applications requiring large recoverable deformation, high w ork densities, excellent ...
1. Introduction. Iron-based shape memory alloy (Fe-SMA, especially referring to Fe-Mn-Si class shape memory alloy) possesses shape memory effect (SME) [1,2], outstanding low-cycle fatigue (LCF) resistance [], and some other desirable characteristics by which the material has proven its potential in the field of the construction industry.Fe-SMA was traditionally regarded as an ideal material ...
Shape memory alloys (SMAs) are metals able to recuperate a preset shape just while heating up to a critical transformation temperature. The physical principle they are based on is a particular martensitic transformation (thermoelastic) occurring in some metallic alloys [ 1 ]. The list includes but is not limited to NiTi, CuAlNi, CuZnAl, AuCd ...
This article reviews the class of active materials known as shape memory alloys (SMAs), especially as used in aerospace applications. To begin, a general overview of SMAs is provided. Their useful properties and engineering effects are described and the methods in which these may be utilized are discussed. A review of past and present aerospace ...
In this paper, the progress on shape memory alloys (SMAs) for the past 10 years was reviewed and research trends in the eld were brie y introduced. Especially, basic properties in some novel alloys, such as the ductile Cu-Al-Mn SMA, ferrous superelastic alloys and Ni-Mn-based metamagnetic SMAs are focused on and the superelastic behavior at ...
DOI: 10.1016/J.MATDES.2013.11.084 Corpus ID: 108440671; A review of shape memory alloy research, applications and opportunities @article{Jani2014ARO, title={A review of shape memory alloy research, applications and opportunities}, author={Jaronie Mohd Jani and Martin Leary and Aleksandar Subic and Mark A. Gibson}, journal={Materials \& Design}, year={2014}, volume={56}, pages={1078-1113}, url ...
The functional properties of shape memory alloys (SMAs) are intricately tied to the transformation of crystal structures from high-symmetry phases (i.e., B2, D03 or L12 in most cases 7) to low ...
Shape Memory Alloys (SMAs) are a new generation of smart materials with the capability of recovering their predefined shape after experiencing a large strain. ... The increasing number of published papers in recent years shows ... A review of shape memory alloy research, applications and opportunities. Mater Des, 56 (2014), pp. 1078-1113 ...
Shape memory alloy (SMA) thin films have received great importance in the research of smart (or intelligent) materials for their utilization in micro-electromechanical systems (MEMS). It is suggested that the fabrication of SMA thin films on flexible polyimide (PI) substrates paves the way for their utility as micro-actuators and sensors in ...
Feature papers represent the most advanced research with significant potential for high impact in the field. A Feature Paper should be a substantial original Article that involves several techniques or approaches, provides an outlook for future research directions and describes possible research applications. ... Shape memory alloys (SMAs) are ...
Expanding the Repertoire of Shape Memory Alloys. An iron alloy may open up new applications for strong materials that are also capable of large reversible shape changes. The exceptional properties of many materials often come at the expense of limited performance in other areas. For example, conventional metals and their alloys are strong ...
Printed on acid-free paper 987654321 springer.com. To all of our loved ones. Preface ... These latter three coauthors were still members of the Shape Memory Alloy Research Team (SMART), or in close proximity, when we started the project ... 2.1.2 Shape Memory Alloy Specimens..... 55 2.2 Thermomechanical Material Properties of SMAs ...
Abstract. Most of shape memory alloys are functional intermetallics. They are now practically being used for couplings, actuators, medical guide wires etc., and are hopeful candidates for smart materials, which already exist. In the present paper, recent developments within nearly 10 years on shape memory alloys and martensitic: transformations ...
ABSTRACT A novel shape memory alloy (SMA) has been developed as an alternative to currently available alloys. This alloy, commercially known by its proprietary brand SMARQ, shows a higher working range of temperatures with respect to the SMA materials used until now in actuators, limited to environment temperatures below 90 A degrees C. SMARQ is a high temperature SMA (HTSMA) based on a fully ...
Keywords: Strain glass, martensitic transition, shape memory alloys, Precipitation Suggested Citation: Suggested Citation Li, Longjia and Yang, Zhongsheng and Xu, Kengfeng and Cui, Dingcong and Wang, Lei and Wang, Zhijun and Li, Junjie and Wang, Jincheng and He, Feng, Strain Glass State in Ni50.3Ti29.7Hf20 High-Temperature Shape Memory Alloy.
Leading to a deep research on Shape Memory Alloys, going through the material itself and its properties, explaining how it works and how the structure of the wheel developed by NASA works. The research provides a background on rover wheels, that lead to the understanding of Shape Memory Alloys and their usage on wheels for Mars