- En español – ExME
- Em português – EME
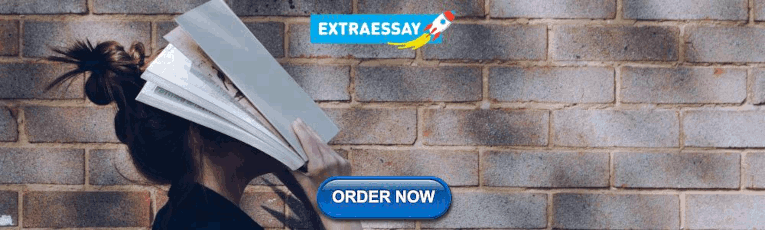
Case-control and Cohort studies: A brief overview
Posted on 6th December 2017 by Saul Crandon
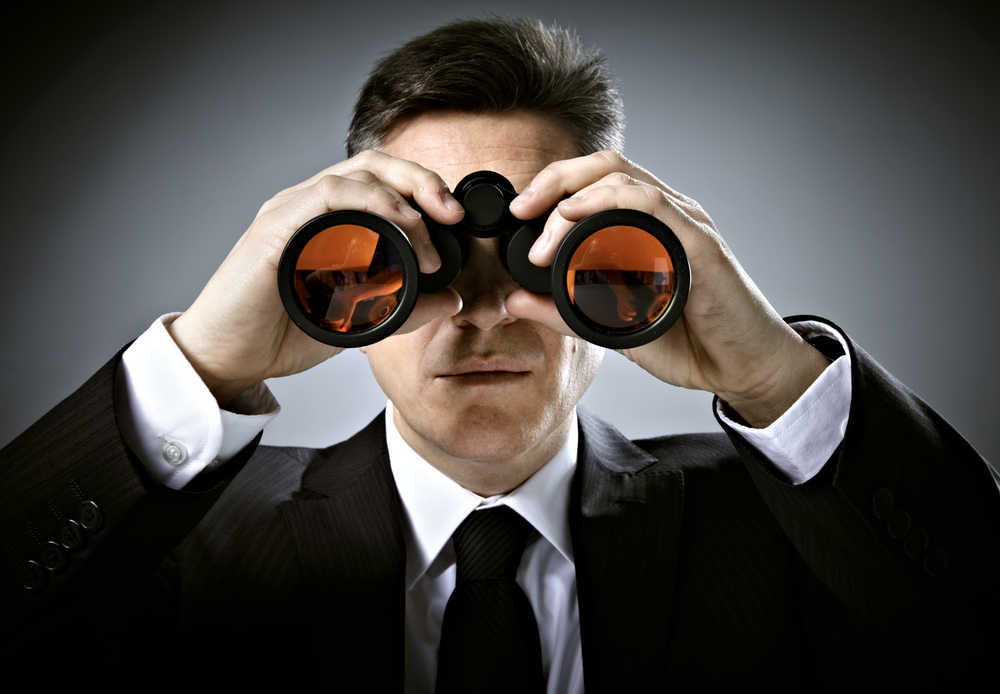
Introduction
Case-control and cohort studies are observational studies that lie near the middle of the hierarchy of evidence . These types of studies, along with randomised controlled trials, constitute analytical studies, whereas case reports and case series define descriptive studies (1). Although these studies are not ranked as highly as randomised controlled trials, they can provide strong evidence if designed appropriately.
Case-control studies
Case-control studies are retrospective. They clearly define two groups at the start: one with the outcome/disease and one without the outcome/disease. They look back to assess whether there is a statistically significant difference in the rates of exposure to a defined risk factor between the groups. See Figure 1 for a pictorial representation of a case-control study design. This can suggest associations between the risk factor and development of the disease in question, although no definitive causality can be drawn. The main outcome measure in case-control studies is odds ratio (OR) .
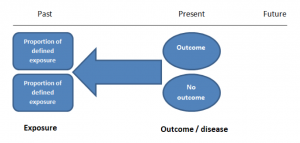
Figure 1. Case-control study design.
Cases should be selected based on objective inclusion and exclusion criteria from a reliable source such as a disease registry. An inherent issue with selecting cases is that a certain proportion of those with the disease would not have a formal diagnosis, may not present for medical care, may be misdiagnosed or may have died before getting a diagnosis. Regardless of how the cases are selected, they should be representative of the broader disease population that you are investigating to ensure generalisability.
Case-control studies should include two groups that are identical EXCEPT for their outcome / disease status.
As such, controls should also be selected carefully. It is possible to match controls to the cases selected on the basis of various factors (e.g. age, sex) to ensure these do not confound the study results. It may even increase statistical power and study precision by choosing up to three or four controls per case (2).
Case-controls can provide fast results and they are cheaper to perform than most other studies. The fact that the analysis is retrospective, allows rare diseases or diseases with long latency periods to be investigated. Furthermore, you can assess multiple exposures to get a better understanding of possible risk factors for the defined outcome / disease.
Nevertheless, as case-controls are retrospective, they are more prone to bias. One of the main examples is recall bias. Often case-control studies require the participants to self-report their exposure to a certain factor. Recall bias is the systematic difference in how the two groups may recall past events e.g. in a study investigating stillbirth, a mother who experienced this may recall the possible contributing factors a lot more vividly than a mother who had a healthy birth.
A summary of the pros and cons of case-control studies are provided in Table 1.
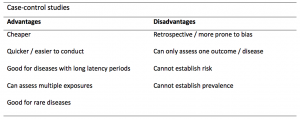
Table 1. Advantages and disadvantages of case-control studies.
Cohort studies
Cohort studies can be retrospective or prospective. Retrospective cohort studies are NOT the same as case-control studies.
In retrospective cohort studies, the exposure and outcomes have already happened. They are usually conducted on data that already exists (from prospective studies) and the exposures are defined before looking at the existing outcome data to see whether exposure to a risk factor is associated with a statistically significant difference in the outcome development rate.
Prospective cohort studies are more common. People are recruited into cohort studies regardless of their exposure or outcome status. This is one of their important strengths. People are often recruited because of their geographical area or occupation, for example, and researchers can then measure and analyse a range of exposures and outcomes.
The study then follows these participants for a defined period to assess the proportion that develop the outcome/disease of interest. See Figure 2 for a pictorial representation of a cohort study design. Therefore, cohort studies are good for assessing prognosis, risk factors and harm. The outcome measure in cohort studies is usually a risk ratio / relative risk (RR).
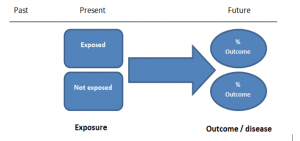
Figure 2. Cohort study design.
Cohort studies should include two groups that are identical EXCEPT for their exposure status.
As a result, both exposed and unexposed groups should be recruited from the same source population. Another important consideration is attrition. If a significant number of participants are not followed up (lost, death, dropped out) then this may impact the validity of the study. Not only does it decrease the study’s power, but there may be attrition bias – a significant difference between the groups of those that did not complete the study.
Cohort studies can assess a range of outcomes allowing an exposure to be rigorously assessed for its impact in developing disease. Additionally, they are good for rare exposures, e.g. contact with a chemical radiation blast.
Whilst cohort studies are useful, they can be expensive and time-consuming, especially if a long follow-up period is chosen or the disease itself is rare or has a long latency.
A summary of the pros and cons of cohort studies are provided in Table 2.
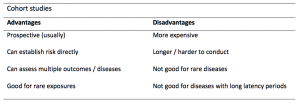
The Strengthening of Reporting of Observational Studies in Epidemiology Statement (STROBE)
STROBE provides a checklist of important steps for conducting these types of studies, as well as acting as best-practice reporting guidelines (3). Both case-control and cohort studies are observational, with varying advantages and disadvantages. However, the most important factor to the quality of evidence these studies provide, is their methodological quality.
- Song, J. and Chung, K. Observational Studies: Cohort and Case-Control Studies . Plastic and Reconstructive Surgery.  2010 Dec;126(6):2234-2242.
- Ury HK. Efficiency of case-control studies with multiple controls per case: Continuous or dichotomous data . Biometrics . 1975 Sep;31(3):643–649.
- von Elm E, Altman DG, Egger M, Pocock SJ, Gøtzsche PC, Vandenbroucke JP; STROBE Initiative. The Strengthening the Reporting of Observational Studies in Epidemiology (STROBE) statement: guidelines for reporting observational studies.  Lancet 2007 Oct;370(9596):1453-14577. PMID: 18064739.
Saul Crandon
Leave a reply cancel reply.
Your email address will not be published. Required fields are marked *
Save my name, email, and website in this browser for the next time I comment.
No Comments on Case-control and Cohort studies: A brief overview
Very well presented, excellent clarifications. Has put me right back into class, literally!
Very clear and informative! Thank you.
very informative article.
Thank you for the easy to understand blog in cohort studies. I want to follow a group of people with and without a disease to see what health outcomes occurs to them in future such as hospitalisations, diagnoses, procedures etc, as I have many health outcomes to consider, my questions is how to make sure these outcomes has not occurred before the “exposure disease”. As, in cohort studies we are looking at incidence (new) cases, so if an outcome have occurred before the exposure, I can leave them out of the analysis. But because I am not looking at a single outcome which can be checked easily and if happened before exposure can be left out. I have EHR data, so all the exposure and outcome have occurred. my aim is to check the rates of different health outcomes between the exposed)dementia) and unexposed(non-dementia) individuals.
Very helpful information
Thanks for making this subject student friendly and easier to understand. A great help.
Thanks a lot. It really helped me to understand the topic. I am taking epidemiology class this winter, and your paper really saved me.
Happy new year.
Wow its amazing n simple way of briefing ,which i was enjoyed to learn this.its very easy n quick to pick ideas .. Thanks n stay connected
Saul you absolute melt! Really good work man
am a student of public health. This information is simple and well presented to the point. Thank you so much.
very helpful information provided here
really thanks for wonderful information because i doing my bachelor degree research by survival model
Quite informative thank you so much for the info please continue posting. An mph student with Africa university Zimbabwe.
Thank you this was so helpful amazing
Apreciated the information provided above.
So clear and perfect. The language is simple and superb.I am recommending this to all budding epidemiology students. Thanks a lot.
Great to hear, thank you AJ!
I have recently completed an investigational study where evidence of phlebitis was determined in a control cohort by data mining from electronic medical records. We then introduced an intervention in an attempt to reduce incidence of phlebitis in a second cohort. Again, results were determined by data mining. This was an expedited study, so there subjects were enrolled in a specific cohort based on date(s) of the drug infused. How do I define this study? Thanks so much.
thanks for the information and knowledge about observational studies. am a masters student in public health/epidemilogy of the faculty of medicines and pharmaceutical sciences , University of Dschang. this information is very explicit and straight to the point
Very much helpful
Subscribe to our newsletter
You will receive our monthly newsletter and free access to Trip Premium.
Related Articles
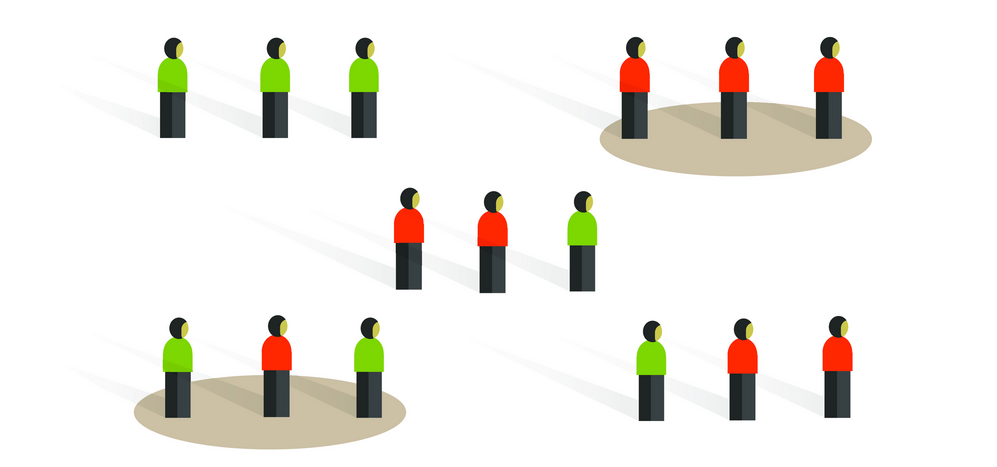
Cluster Randomized Trials: Concepts
This blog summarizes the concepts of cluster randomization, and the logistical and statistical considerations while designing a cluster randomized controlled trial.
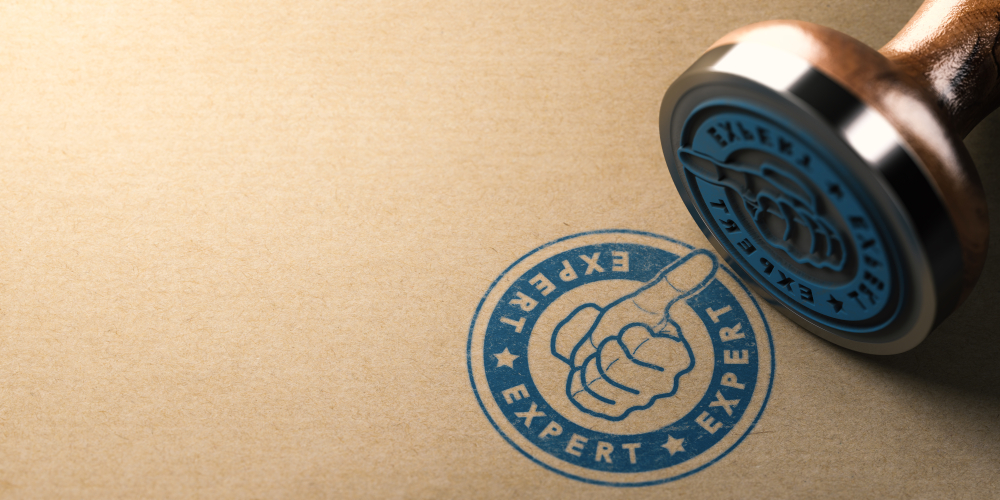
Expertise-based Randomized Controlled Trials
This blog summarizes the concepts of Expertise-based randomized controlled trials with a focus on the advantages and challenges associated with this type of study.
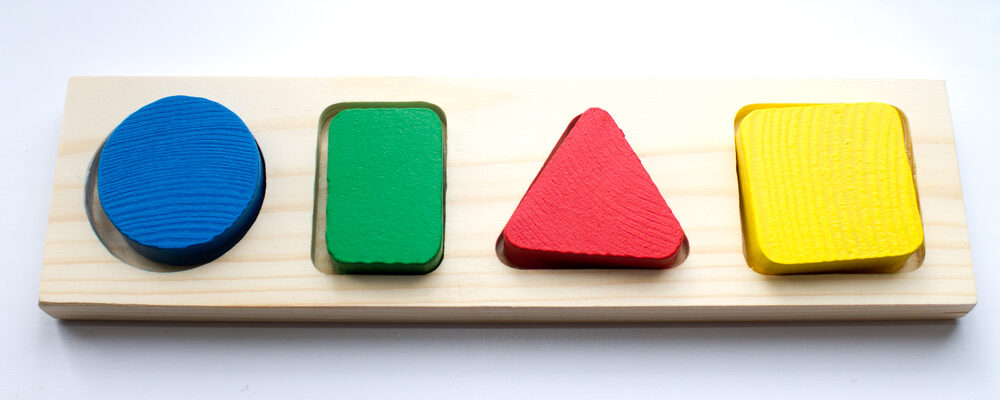
An introduction to different types of study design
Conducting successful research requires choosing the appropriate study design. This article describes the most common types of designs conducted by researchers.
- Open access
- Published: 13 December 2023
Analysing cluster randomised controlled trials using GLMM, GEE1, GEE2, and QIF: results from four case studies
- Bright C. Offorha 1 ,
- Stephen J. Walters 1 &
- Richard M. Jacques 1
BMC Medical Research Methodology volume 23 , Article number: 293 ( 2023 ) Cite this article
1082 Accesses
4 Altmetric
Metrics details
Using four case studies, we aim to provide practical guidance and recommendations for the analysis of cluster randomised controlled trials.
Four modelling approaches (Generalized Linear Mixed Models with parameters estimated by maximum likelihood/restricted maximum likelihood; Generalized Linear Models with parameters estimated by Generalized Estimating Equations (1st order or second order) and Quadratic Inference Function, for analysing correlated individual participant level outcomes in cluster randomised controlled trials were identified after we reviewed the literature. We systematically searched the online bibliography databases of MEDLINE, EMBASE, PsycINFO (via OVID), CINAHL (via EBSCO), and SCOPUS. We identified the above-mentioned four statistical analytical approaches and applied them to four case studies of cluster randomised controlled trials with the number of clusters ranging from 10 to 100, and individual participants ranging from 748 to 9,207. Results were obtained for both continuous and binary outcomes using R and SAS statistical packages.
The intracluster correlation coefficient (ICC) estimates for the case studies were less than 0.05 and are consistent with the observed ICC values commonly reported in primary care and community-based cluster randomised controlled trials. In most cases, the four methods produced similar results. However, in a few analyses, quadratic inference function produced different results compared to the generalized linear mixed model, first-order generalized estimating equations, and second-order generalized estimating equations, especially in trials with small to moderate numbers of clusters.
This paper demonstrates the analysis of cluster randomised controlled trials with four modelling approaches. The results obtained were similar in most cases, however, for trials with few clusters we do recommend that the quadratic inference function should be used with caution, and where possible a small sample correction should be used. The generalisability of our results is limited to studies with similar features to our case studies, for example, studies with a similar-sized ICC. It is important to conduct simulation studies to comprehensively evaluate the performance of the four modelling approaches.
Peer Review reports
Randomisation is used in clinical trials to achieve balance between treatment arms in variations caused by both known and unknown prognostic factors, eliminate selection bias, and improve the external validity of the study. If done properly, it should minimise the effect of the prognostic factors so that researchers can controllably study the effect of the intervention(s) of interest [ 1 ]. Instead of randomising individuals to the treatment arms as done in individually randomised controlled trials (IRCTs), groups/clusters of individuals are randomised in cluster randomised controlled trials (CRCTs). In CRCT there are two levels; the distinctive cluster level and the individual level (with correlated outcomes) which are nested within the clusters. An appropriate statistical method for analysing CRCTs will be any method that considers this hierarchical nature of the CRCT design. Ignoring the correlated outcomes within a cluster and using standard statistical methods that treat the outcomes as being independent, might lead to underestimating the standard errors of the parameters and consequently obtaining narrower confidence intervals, false small P-values, and incorrectly overstating the effect of the intervention.
Some of the common issues in CRCT design and analysis are (a) Ignoring clustering [ 2 ], (b) inadequate handling of missing data [ 3 ], (c) and poor reporting of results [ 2 , 4 ]. Newer analytical methods for handling clustering have been proposed in the literature of other study designs with clustered data, such as longitudinal study designs. Notable ones are targeted maximum likelihood estimation (TMLE) [ 5 ], quadratic inference function (QIF) [ 6 ], and alternating logistic regression (ALR) [ 7 ]. Furthermore, QIF is acclaimed to be a promising alternative to GEE1, especially when the correlation structure is misspecified [ 6 , 8 , 9 ], however, it is worth noting that these recent alternatives have not been comprehensively compared to the existing methods used in CRCTs like the GEE1, which might account for their slow uptake. This study aims to contribute to the literature (in the context of CRCTs) on the performance of the newer methods compared to the existing methods, to promote their use in CRCTs (if necessary).
This paper reviews and describes the selected statistical methods for analysing both continuous and binary outcomes in CRCTs. We focus on statistical methods for analysing individual-level outcomes which are correlated within clusters. The paper explores the performance of all the analytical methods given the settings of our case studies. The objectives of this study are to demonstrate the practical application of these selected modelling approaches for analysing CRCTs, to compare and discuss their methodological differences, and to make general comments based on our findings.
Literature review
Search strategy.
This review provides an overview of the appropriate and available statistical methods for analysing outcome data from CRCTs by mapping the evidence in the published literature on the development, refinement, and comparison of the statistical methods. This was a methodological review focusing on the appropriate, and available methods for analysing CRCTs with clustering in treatment arms. We reviewed the literature from 1 st January 2003 to 19 th December 2020. This was a year before the publication of the CONSORT statement 2004 extension for cluster randomised controlled trials.
We used a developed search strategy (see, Additional file 1 ) to search the online bibliography databases of MEDLINE, EMBASE, PsycINFO (via OVID); and CINAHL (via EBSCO), and SCOPUS. In addition to searching published literature databases, OpenGrey, web-of-science, and Scopus databases for conference proceedings were also searched to identify difficult-to-locate (grey) literature. A standardised pre-piloted data collection tool was used to extract information on the study and methodological characteristics from the included articles. One reviewer, BCO, carried out the search and extraction of the relevant information; two other independent reviewers, SJW and RMJ, supervised and validated the process. We discussed extensively to reach a consensus on issues presented during the review process.
Literature search results
The literature search identified 1573 articles and after removing duplicates 1073 articles remained. After screening the title and abstract of each of the identified articles, 116 were shortlisted and 55 articles (including 12 from pearl growing) were finally chosen, while other 73 articles were excluded for various reasons (see, Fig. 1 ). These articles are methodological and application papers and are referenced throughout. The search and selection process of the included articles is presented in Fig. 1 . Among the included 55 included articles; 34 (62%) compared already existing methods, 25% proposed new statistical methods, and 13% refined already existing ones. There was no clear pattern in the development, advancement, or comparison of statistical methods for analysing outcome data from CRCTs in the last two decades (see, Additional file 2 ).
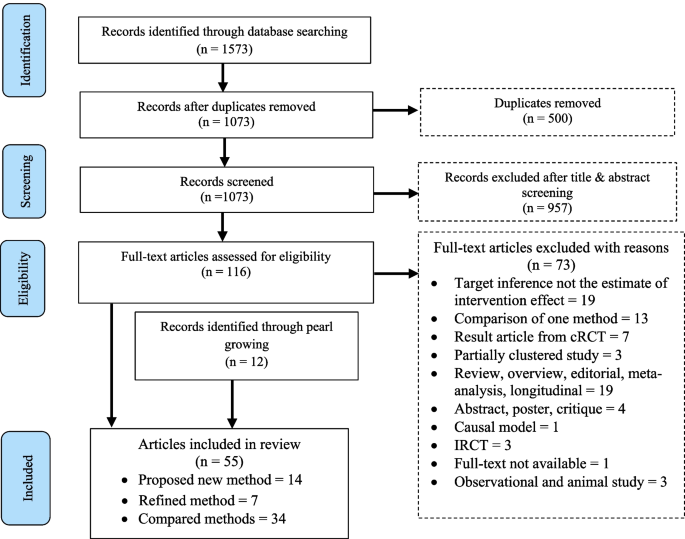
Flow chart of the search and selection process of the included articles
The number of times each method was studied in the 55 articles and their references are summarised in Table S 1 (see, Additional file 3 ). This review identified 27 unique statistical methods for analysing CRCTs which were studied 112 times in total. Regression models with parameters estimated by first-order generalized estimating equations (GEE1) was the most studied method (23/112, 21%) followed by maximum likelihood estimation (MLE) (16%). Among the newer methods, QIF was the most studied method (5%). Hence, four statistical regression models for the analysis of correlated individual participant-level outcomes in cluster randomised controlled trials were selected. They are:
1. Generalized Linear Mixed Models (GLMM) with parameters/coefficients estimated by Maximum likelihood (MLE) or restricted MLE denoted as GLMM henceforth.
2. Marginal Generalized Linear Models (mGLM) with parameters/coefficients estimated by 1st order Generalized Estimating Equations denoted as GEE1 henceforth.
3. Marginal Generalized Linear Models (mGLM) with parameters/coefficients estimated by 2nd-order Generalized Estimating Equations denoted as GEE2 henceforth.
4. Marginal Generalized Linear Models (mGLM) with parameters/coefficients estimated by Quadratic Inference Function denoted as QIF henceforth.
Specifically, GLMM and GEE1 were selected based on their popularity in the literature of CRCTs, they are the two most studied regression methods (see, Table S 1 ), while GEE2 and QIF were selected based on findings that suggested them to be the two most promising improvements on the GEE1 [ 10 , 11 , 12 , 13 ]. GEE2 and QIF are not commonly used for analysing CRCTs, however, QIF has been extensively studied and applied in the context of longitudinal studies where outcomes measured repeatedly over time from a particular individual are likely to be correlated. For example, Odueyungbo et al., [ 9 ] and Song et al., [ 8 ] compared QIF to GEE1 using real-world data from longitudinal studies. Several other papers have compared QIF to GEE1 using both real-world and computer-simulated data, both in the context of longitudinal and CRCT designs [ 6 , 14 , 15 , 16 , 17 ]. Similarly, several studies have compared GLMM to GEE1 to assess their relative performance [ 18 , 19 , 20 , 21 ]. To the best of our knowledge, no study has compared these four selected methods – GLMM, GEE1, GEE2, and QIF at the time of writing this report.
A boldface letter denotes either a vector or a matrix or as otherwise stated. The general notation is established as; let \({y}_{ij}\) denote an outcome for the \(j\) th subject in the \(i\) th cluster ( \(i=1,\dots ,N; j=1, \dots , {n}_{i})\) ; \(N\) is the number of independent clusters in the study and \({n}_{i}\) denotes the different number of subjects in each cluster (i.e., the \(i\) th cluster size), \({y}_{ij}\) has a corresponding set of \(p\) -dimensional vector covariates \({{\varvec{X}}}_{pij}^{T}= ({ x}_{1i},\cdots , {x}_{\mathrm{p}ij})\) where \({x}_{1i}\) denotes an indicator variable for the treatment group to which a cluster belongs \(( {x}_{1i}=0\) indicates the control group and \({x}_{1i}=1\) the intervention group) and \({{\varvec{Y}}}_{i}= {({y}_{i1},\cdots , {y}_{{in}_{i}})}^{T}\) is a \({n}_{i}\times 1\) vector of the collection of the individual level outcomes for the \(i\) th cluster. Also, \({{\varvec{\beta}}}_{p}= ({\beta }_{0},{\beta }_{1},\cdots , {\beta }_{p})\) is the unknown \(p\) -dimensional vector of regression parameters and \({{\varvec{\mu}}}_{i}= {({\mu }_{i1},\cdots , {\mu }_{i{n}_{i}})}^{T}\) is an \({n}_{i}\times 1\) vector of true means with \({\mu }_{ij}=E({y}_{ij }|{{\varvec{X}}}_{pij}^{T})\) being the conditional expectation for the \(j\) th subject in the \(i\) th cluster with covariates \({{\varvec{X}}}_{pij}^{T}\) .
Individual Level Analysis (ILA)
All the analytical methods considered in this study are based on individual-level analysis, meaning that outcomes from all the participating individual subjects in a trial are used as response values. This approach is further categorised according to how the regression model adjusts for clustering of the response values of subjects within a cluster. The different regression models and statistical methods used for estimating the regression coefficients in the models are explained in the subsequent subsections.
Cluster-Specific Model (CSM)
The models classed under this category adjust for clustering by using the outcome of each of the subjects and conditionally relating it to the fixed effects and random effects components of the model. The parameter estimates of the fixed effects and random effects components are obtained simultaneously. The estimate of the intervention effect from this analytical approach is interpreted as what will happen to individuals in a cluster if they receive the intervention treatment compared to them receiving the control treatment. The linear mixed model (LMM) is a common example of this approach.
Generalized Linear Mixed Model (GLMM) with coefficients estimated by MLE/REML
The GLMM is also called a random (or mixed) effects model and is the most used conditional/cluster-specific model for analysing CRCTs [ 2 , 3 ]. The LMM, with a continuous outcome and identity link function is a special case of a GLMM. In a GLMM, a single model equation is specified to assess the impact of the fixed effects of some covariates of interest and the random effects of the randomly selected clusters on the outcome of interest. MLE is commonly used to estimate the parameters of the fixed effects and random effects components of a GLMM, simultaneously.
However, technically, the MLE algorithm estimates the fixed effects component initially (ignoring the random effects component), then plugs the estimates into the algorithm to estimate the random effects component. This process is repeated until optimal estimates are obtained. However, ignoring the random effects component in the first step causes the MLE to produce negatively biased variance components, because, it means ignoring the variations present in the estimates of the fixed effects, which could be substantial when the sample size is small [ 22 , 23 , 24 ]. Also, the MLE does not adjust for the degrees of freedom (DoF) lost in estimating the parameters of the fixed effects component [ 24 ]. Hence, the MLE is likely to produce SEs that are too small, resulting in smaller P-values, and inflated Type I error rates, especially when there are few clusters.
An alternative likelihood-based estimation method is the restricted maximum likelihood estimation (REML) which can be utilised to circumvent these problems. For large sample sizes, these problems are not noticeable, and the estimates from MLE and REML are approximately the same. However, for cRCTs with small samples, the problems are more pronounced [ 21 , 23 ]. The REML first transforms the outcome data to remove the fixed effects, before estimating the random effects component. Then, it applies generalized least squares estimator to obtain the estimates of the fixed effects component within its algorithm. Put differently, REML obtains the estimates of the fixed effects and random effects components separately, starting with the random effects component [ 24 ]. To appropriately adjust for the loss in the DoF, we applied the Satterthwaite correction on the DoF, which resulted in adjusted P-values and CIs [ 21 ].
Let \({y}_{ij}\) denote a continuous outcome from a \(j\) th individual in an \(i\) th cluster. A specific example of the LMM called the random intercept LMM (because it adjusts for the random cluster effects using a random intercept term in the mixed model) is given as
where \({\beta }_{1}\) is the intervention effect, \({x}_{1i}\) and \({x}_{pij}\) are the indicator and p th variables respectively for the \(j\) th individual in the \(i\) th cluster, \({\tau }_{i}\) is the random effects term which causes variability in the cluster means and \({\varepsilon }_{ij}\) is the residual for each individual. When \({y}_{ij}\) is a non-Normally distributed outcome, such as a binary or count outcome, model Eq. ( 1 ) can be generalized. This explains the “generalized” in GLMM, the GLMM could be expressed as
where \({y}_{ij}\) is a non-normal outcome, \(\eta (.)\) is a link function that linearly relates the expected response values to the fixed effects and the random effects components of the model. For example, if \({y}_{ij}\sim Bi\left(n,\mathrm{Pr}\left({y}_{ij}=1\right)\right)\) then Eq. ( 2 ) is specified using a logit link function as
where \(\mathrm{Pr}\left({y}_{ij}=1\right)\) is the probability of a success, that is, \({y}_{ij}=1\) and \(logit \left(\mathrm{Pr}\left({y}_{ij}=1\right)\right)=\frac{\mathrm{Pr}\left({y}_{ij}=1\right)}{\left(1 -\mathrm{Pr}\left({y}_{ij}=1\right)\right)}\) . MLE is a common choice for estimating the parameters of the GLMM. The general full likelihood of Eqs. ( 1 ), ( 2 ) and ( 3 ) is given as [ 25 ]
where \(l\) (.) is the likelihood function for \({y}_{ij},\psi (.)\) is the probability function for \({y}_{ij}, {\tau }_{i}\) is often assumed to follow a Normal probability function \(g\) (.) and \({\varvec{\theta}}=( {\beta }_{0},{{\beta }_{1},\beta }_{p} )\) . Maximum likelihood estimates are obtained by taking the first derivatives of the log of \(l\) (.) for each parameter, while the second derivative produces the standard errors. It is difficult to analytically obtain a closed-form solution for Eq. ( 4 ) due to the high dimension of the integral involved, a numerical likelihood approximation method is often used to circumvent this problem. We used the Adaptive Gauss-Hermite Quadrature (AGHQ) to perform the numerical approximation [ 26 ]. The GLMM models were implemented using the SAS 9.4 procedure; PROC GLIMMIX .
Population Average Model (PAM)
The regression models under this class are appropriate for assessing the population average intervention effect. Here, inferences are made regarding the population of clusters rather than the individual subjects, and the target of the conclusions reached in the study is the population from where the clusters were drawn. Here, the intervention effect estimate is interpreted as the comparison of the average change in the population means between the intervention and control groups. PAMs are based on the marginal likelihoods of the correlated response values from the i th cluster, \({{\varvec{Y}}}_{i}\) , hence are considered to be semi-parametric models. The correlation of outcomes within clusters are accounted for using a separate working covariance matrix characterised by a working correlation matrix. In general, a PAM could be expressed as
where \(\upmu_{i}\) is the mean for the ith cluster. The marginal variance of a univariate response value \({y}_{ij}\) is often specified as \(\phi \nu ({\mu }_{ij})\) , where \(\nu (.)\) is a known variance function and \(\phi\) is a scale parameter that equals 1 for a binary outcome and \({\sigma }^{2}\) for a continuous outcome (and needs to be estimated). Equation ( 5 ) is similar to [ 2 ], but different in that corr \(({\varepsilon }_{ij},{\varepsilon }_{{ij}{\prime}})\ne 0\) but rather corr \(\left({\varepsilon }_{ij},{\varepsilon }_{{ij}{\prime}}\right)= \rho \left({x}_{ij},{x}_{i{j}{\prime}};{\varvec{P}}\right)\forall j\ne {j}{\prime}, {\varvec{P}}\) is the true correlation matrix to be approximated by a “working” correlation matrix, \({\varvec{R}}\) , which is characterised by the intracluster correlation coefficient (ICC), \(\rho\) .
The intracluster correlation coefficient
The ICC quantifies the correlation between the outcomes of any pair of subjects within a cluster. When the ICC is zero it indicates that any randomly paired outcome values from any randomly paired subjects in a cluster are independent, which gives rise to the “independence” working correlation structure. It is more common in cRCT to assume that the ICC is the same and nonzero across clusters which gives rise to the “exchangeable” working correlation structure. The independence and the exchangeable working correlation structures are the two most assumed in CRCTs. Common estimators of the ICC for continuous and binary outcomes are given as
where \({\upsigma }_{b}^{2}\) is the intracluster variation, \({\upsigma }_{w}^{2}\) is individual subject variation and \(\pi =3.141593\) [ 27 ]. These two parameters, \({\upsigma }_{b}^{2}\) and \({\upsigma }_{w}^{2}\) , can be estimated using the extracts from the output of a one-way analysis of variance (ANOVA). According to Donner [ 28 ] the following equations hold true
where \(MSB\) is the between-cluster mean squared error, \(MSW\) is the within-cluster mean square error, both \(MSB,\) and \(MSW\) are the extracts from ANOVA, \(\overline{n }\) is the average cluster size calculated with the formula below
where N is the total number of clusters, n is the total sample size, and \({n}_{i}\) is the i th cluster size. If Eq. ( 8 ) is substituted into Eq. ( 7 ) the ICC estimator becomes [ 29 ]
Obtaining either a positive or negative ICC estimate depends on which estimator is used, while the ICC estimator of Eq. ( 6 ) is positive definite because its components are variances, the other estimator, Eq. ( 9 ), can produce a negative ICC estimate because of the subtraction in its numerator, and this occurs when \(MSB<MSW\) .
mGLM with coefficients estimated by GEE1
The first-order generalized estimating equations (GEE1) is the most common multilevel statistical method used for obtaining the parameter estimates of an mGLM (aka, PAM) specified in Eq. ( 5 ). The GEE1 estimator treats the correlations of outcomes within clusters as a nuisance, such that, it does not explicitly model the effect of the correlations. However, GEE1 accounts for the correlations using a separate “working” covariance matrix characterised by the working correlation matrix.
The GEE1 draws its strength from the linear exponential family distribution [ 30 ]. Liang and Zeger [ 31 ] proposed a class of estimating equations that uses a working correlation matrix (with fewer nuisance parameters) to obtain the parameter estimates of Eq. ( 5 ) given as
where \({{\varvec{V}}}_{i}\) is the \({n}_{i}\times {n}_{i}\) covariance matrix for \({{\varvec{Y}}}_{i}\) (i.e., \({{\varvec{V}}}_{i}=Cov{({\varvec{Y}}}_{i})\) ) specified by the working correlation matrix \({\varvec{R}}(\alpha )\) and defined as
where \({{\varvec{G}}}_{i}=diag\{\nu ({\mu }_{i1}), \cdots , \nu ({\mu }_{i{n}_{i}}) \}\) is a diagonal matrix with the diagonal elements \(\nu ({\mu }_{ij})\) that is, the variance function for each response \({y}_{ij}\) , and \({{\varvec{R}}}_{i}(\alpha )\) is an \({n}_{i}\times {n}_{i}\) working correlation matrix specified by the ICC, \(\alpha\) . Estimates from a GEE1 with an exchangeable correlation structure are equal to that of a random intercept model of Eq. ( 1 ) for linear models, but it is not necessarily the case for nonlinear models [ 32 ].The GEE1 estimator computes asymptotically consistent estimates \(\widehat{{\varvec{\beta}}}\) , regardless of the choice of \({{\varvec{R}}}_{i}(\alpha )\) but provided that the mean structure is correct. However, it may suffer some loss in efficiency if the choice of \({{\varvec{R}}}_{i}(\alpha )\) is not correct [ 6 ]. The parameter estimates \(\widehat{{\varvec{\beta}}}\) are iteratively obtained by alternating between a modified Fisher scoring algorithm for \({\varvec{\beta}}\) and the moment estimation of \(\alpha\) and \(\phi\) , and its residual \({N}^\frac{1}{2}(\widehat{{\varvec{\beta}}} -{\varvec{\beta}})\) is a multivariate Normally distributed residual with mean zero and a robust sandwich variance–covariance matrix \({{\varvec{\xi}}}_{i}\) . The GEE1 models were fitted using the SAS 9.4 procedure, PROC GENMOD .
mGLM with coefficients estimated by GEE2
This class of regression models attempts to leverage the major drawback of the GEE1 – possible loss in efficiency when the correlation structure is misspecified, especially when the correlation among outcomes is substantial [ 12 , 13 ]. Statistical efficiency is a desirable property of a good estimator after unbiasedness has been established. Among all unbiased competing estimators, an efficient estimator is the one that produces the smallest standard error estimate, which is indicative of a lesser variability and a higher degree of precision.
The GEE2 model estimates the correlation parameter (i.e., the nuisance parameter in GEE1) and mean parameter simultaneously in its algorithm [ 11 , 12 , 13 , 33 , 34 ]. Hence, if modelling the correlation among subjects within a cluster is of primary interest, then GEE2 should be considered. For example, in a family study to assess the impact of the genetic relatedness of the family members on their alcohol dependence, GEE2 was highly recommended cause it may improve the efficiency of the mean parameters [ 13 ].
The models under the GEE2 analytical approach draw their strength from the quadratic exponential family distribution [ 30 ]. If the marginal density of \({{\varvec{Y}}}_{i}\) conditioned on the mean vector \({{\varvec{\mu}}}_{i}\) and the covariance matrix \({{\varvec{V}}}_{i}\) , can be expressed as belonging to the quadratic exponential family distribution, then this allows for the mean and the covariance of \({{\varvec{Y}}}_{i}\) to be obtained simultaneously. Several GEE2 estimators have been proposed for estimating the mean and correlation parameters simultaneously [ 11 , 12 , 33 , 34 ], however, Yan and Fine [ 13 ] used separate link functions to model the mean, the scale, and the correlation parameters and generated their corresponding sets of estimating equations to be solved simultaneously. This is known as the three-estimating Eqs. (3EE) GEE2, and it is applied in this paper.
To establish the model specification, let \({{\varvec{X}}}_{1i} , {{\varvec{X}}}_{2i}\ \mathrm{and}\ {{{\varvec{X}}}_{3i}}\) be the \({n}_{i}\times p, {n}_{i}\times r\) and \(\frac{n(n+1)}{2}\times q\) design matrices for the mean, the scale, and the correlation parameters of the vector of outcomes \({{\varvec{Y}}}_{i}\) , respectively. The specific link function for the mean, the scale, and correlation parameters to \({{\varvec{X}}}_{1i} , {{\varvec{X}}}_{2i}\ \mathrm{and}\ {{\varvec{X}}}_{3i}\) , respectively, is given as
where \({{\varvec{\mu}}}_{i}\) is a \({n}_{i}\times 1\) mean vector specified by \({\varvec{\beta}}\) , \({{\varvec{\phi}}}_{i}\) is a \({n}_{i}\times 1\) scale vector specified by \(\boldsymbol{\varphi }\) and \({{\varvec{\rho}}}_{i}\) is a \(\frac{{n}_{i}({n}_{i}+1)}{2}\times 1\) pairwise correlation vector specified by \(\boldsymbol{\alpha }\) . The unified corresponding set of estimating equations for Eq. ( 12 ) to be solved simultaneously is given as
where \({{\varvec{Y}}}_{i}\) and \({{\varvec{V}}}_{1i}\) is as defined in the GEE1 mean model of Eqs. ( 10 ) and ( 11 ), \({{\varvec{Z}}}_{i}\) is the \({n}_{i}\times 1\) vector of the scales, \({{\varvec{S}}}_{i}\) is the \(\frac{{n}_{i}({n}_{i}+1)}{2}\times 1\) vector of the pairwise correlations, \({{\varvec{V}}}_{1i}\) and \({{\varvec{V}}}_{2i}\) are the working covariance matrices of \({{\varvec{Z}}}_{i}\) and \({{\varvec{S}}}_{i}\) respectively.
The GEE2 (Eq. ( 13 )) requires the specification of the first four central moments of the outcome vector (mean response, variance, skewness, kurtosis). Yan and Fine [ 13 ] suggested a way around it to avoid the problem of convergence and it is implemented using the geese [ 35 ] function in the R package geepack [ 36 ]. In general, the third and fourth moments can be specified as functions of the first and second moments, thereby avoiding the direct estimation of higher-order moments [ 12 ]. The GEE2 estimator consistently estimates the mean parameters \({\varvec{\beta}}\) regardless of whether the scale and correlation structures are wrong, the estimates for scales \(\boldsymbol{\varphi }\) are consistent regardless of whether the working correlation is mis-specified, but provided that the mean and scale structures are correct.
The major merit of the 3EE GEE2 estimator is that it allows for separate covariates to be included in the mean, scale, and correlation models. This is important when investigating heterogeneous correlation across clusters or treatment arms, such as modelling multiple forms of clustering. Where each cluster or treatment arm presents a different degree of correlation \({\alpha }_{i}\) among subjects, possibly due to cluster sizes and covariates imbalance. Taking this heterogeneity into account may improve efficiency, instead of assuming a constant correlation across clusters or treatment arms [ 10 ]. The solutions of Eq. ( 13 ) are obtained iteratively by alternating between a modified Fisher scoring algorithm and the moment estimation method. The GEE2 models were fitted using the R’s geese function in the geepack package.
mGLM with coefficients estimated by QIF
Similar to GEE2, the quadratic inference function (QIF) was proposed to circumvent a major issue with GEE1, that is, the loss in efficiency due to the misspecification of the correlation structure. But compared to GEE2, QIF does not require the specification of the third and fourth moments (as it imposes additional constraints). The QIF estimator avoids the direct use of the working correlation matrix in its algorithm. Instead, it uses a linear combination of basis matrices and some constants to replace the inverse of the working correlation matrix. Hence, the QIF is more robust to misspecification of the working correlation matrix compared to GEE1, providing better protection against incorrect correlation structure. With this, the QIF produces more efficient parameter estimates compared to GEE1 [ 6 ]. However, if the working correlation structure is not misspecified, the efficiency of the parameter estimates from GEE1 and QIF are equivalent [ 6 , 8 ].
Let \({{\varvec{Y}}}_{i}, {{\varvec{X}}}_{i}, {{\varvec{\mu}}}_{i}\) , and \({{\varvec{V}}}_{i}\) be the same as defined in Eqs. ( 10 ) and ( 11 ). In the QIF equation, the inverse of \({\varvec{R}}\) specified in Eqs. ( 10 ) and ( 11 ) is approximated using a linear combination of a set of several basis matrices \({{\varvec{R}}}_{h}^{-1}\approx {k}_{h}{{\varvec{M}}}_{h}+\dots +{k}_{m}{{\varvec{M}}}_{m}; \left(h=1,\dots ,m\right); {{\varvec{M}}}_{h}\) is the \(h\) th known basis matrix with its unknown coefficient/constant, \({{\varvec{k}}}_{h}\) , that needs to be estimated. For the exchangeable and autoregressive working covariance matrix, \(h=1\) and 2 should suffice, respectively [ 6 , 17 ]. Using this new information, we can rewrite the estimating Eq. ( 10 ) of the GEE1 as extended score equations given as
where \({g}_{i}({\varvec{\beta}})\) is the score vector of each cluster, the constants \({{\varvec{k}}}_{m}\) are considered a nuisance and are not included. The QIF estimator uses the generalized method of moments (GMM) [ 37 ] to optimally combine the multiple estimating equations in [ 13 ]. Hence, the estimate \(\widehat{{\varvec{\beta}}}\) is obtained by minimising the weighted length of \({\overline{{\varvec{g}}} }_{N}\) using the GMM, which could be express as
where \(arg {min}_{{\varvec{\beta}}}\) is the argument of the minimum of \({\varvec{\beta}}\) that minimises \({\overline{{\varvec{g}}} }_{N}^{T}{{\varvec{\Sigma}}}_{N}^{-1} {\overline{{\varvec{g}}} }_{N}\) . As expected, the true covariance matrix \({{\varvec{\Sigma}}}_{N}\) is replaced by the estimated covariance matrix \({{\varvec{C}}}_{N}\) in Eq. ( 15 ), with its inverse \({{\varvec{C}}}_{N}^{-1}\) representing a weighting function. Thus, the QIF estimator becomes
where \({{\varvec{C}}}_{N}=\left(1/{N}^{2}\right){\sum }_{i}^{N}{g}_{i}\left({\varvec{\beta}}\right){g}_{i}^{T}\left({\varvec{\beta}}\right), {{\varvec{C}}}_{N}^{-1}\) is the main reason behind QIF’s efficiency advantage, because it weights the information each \(i\) th cluster contributes to the estimating equation, clusters with large variation are given less weight than the ones with small variation. The estimates \(\widehat{{\varvec{\beta}}}\) are obtained iteratively using the Newton–Raphson algorithm [ 6 ] to evaluate Eq. ( 16 ). The QIF models were fitted using the SAS 9.4 macro: qif.
Comparison between the methods
Table 1 compares the methodological properties of the four modelling approaches, and some of these properties are discussed below. For ILA there are situations where the parameter estimates from CSM and PAM are equivalent in interpretation. A random intercept LMM typifying a CSM is equivalent to a PAM with an exchangeable working correlation structure and collapsible link function, however, both methods produce inconsistent estimates (i.e., biased estimates) when the cluster sizes are informative [ 32 , 38 , 39 ]. Theoretically, the random intercept LMM and PAMs with an exchangeable working correlation structure produce different parameter estimates in the case of noncollapsible link functions, and also if the cluster sizes are informative.
In terms of efficiency (concerning the size of the SE of the estimated treatment effect), the GEE1 considers the correlation among outcomes within clusters, this improves its efficiency (see, Table 1 , row 6). However, GEE1 produces a consistent intervention effect estimate (and its SE) if the mean model is correct and outcome data are missing completely at random regardless whether the correlation structure is misspecified [ 31 ]. However, GEE1 suffers some loss in efficiency if the working correlation structure is not close to the true correlation structure, especially when the true correlation is large and/or the sample size is small. When the sample size is small (which is a recipe for imbalance) the robust SE estimator of GEE1 does not provide full protection over incorrect working correlation structure, causing GEE1 to have reduced efficiency in regards to the size of the SE of the estimated intervention effect [ 23 , 40 , 42 ].
This disadvantage of the GEE1 is the reason why GEE2 and QIF were developed to improve GEE1’s efficiency. GEE2 achieves this by explicitly modelling the mean and correlation parameters simultaneously, using separate sets of estimating equations. Also, if mean and correlation are of interest, GEE2 is more likely to produce efficient inferences for the mean and correlation parameters than GEE1, especially if the correlation within clusters is substantial and the sample size is small [ 10 , 11 , 12 , 13 ]. QIF is another alternative to GEE1 that uses a different strategy to estimate the working correlation parameter, thereby minimising the impact of its misspecification. Studies have proved this advantage of the QIF in the context of a longitudinal study [ 6 , 8 , 9 ]. Their results showed that QIF is more efficient than GEE1 when the true correlation is large and misspecified. Several authors have shown that this claim might not necessarily hold when there are few clusters and/or there is cluster and covariate imbalance between treatment arms [ 15 , 16 , 17 ].
The MLE as an estimator of GLMM is known to be consistent and efficient when the distributional assumptions made are correct. One such assumption is that the random cluster effects are Normally distributed. Previous studies had overstated the impact of misspecifying the distribution of the random effects on MLE [ 43 , 44 ]. However, a recent study has shown that the MLE is quite robust to the impact of misspecifying the distribution of the random effects in most situations considered previously [ 45 ], even when the cluster size is informative [ 46 ].
The goodness-of-fit of a statistical model is a crucial part of building an optimal regression model for practical uses. Appropriate goodness-of-fit methods for CSMs have been extensively studied in the literature whereas goodness-of-fit methods for PAMs are few. The early goodness-of-fit methods for GEE-based models involve partitioning the covariates space into separate groups and then calculating their score statistics which are approximately Chi-square distributed [ 47 , 48 ]. This strategy is an extension to that of Tsiatis [ 49 ] and Hosmer and Lemeshow [ 50 ] for uncorrelated outcomes. This strategy was found to produce different results in different statistical software because the partitioning is subjective to the software used [ 51 ], and this problem may likely extend to population average models for analysing correlated outcomes [ 41 ].
Pan (2001) [ 41 ] proposed a goodness-of-fit method for PAMs that mimics Akaike’s Information Criterion (AIC) known as the Quasi-likelihood information criterion (QIC). While AIC is based on maximum likelihood, QIC is based on quasi-likelihood under an independence working correlation structure in GEE1. The results of the simulation study conducted in the paper showed that the AIC was more efficient than the proposed QIC, however, the performance of the QIC was remarkable. The author did not clearly state if this criterion applies to GEE2 but noted that using the GEE2 approach to estimate the scale parameter included in their criterion is difficult. A goodness-of-fit method exists for GEE2 in McCullagh and Nelder (1989) [ 52 ]. To the best of our knowledge, the method is not available in standard statistical packages at the time of authoring this current paper.
Pan (2002) [ 53 ] further proposed two other tests for a logistic population average model; the Pearson chi-square G and the unweighted sum of squares U tests which are based on the Normal distribution with means and variances (using unstructured working correlation). When analysing a correlated binary outcome if the model has at least one continuous covariate, it becomes difficult to apply goodness-of-tests that are based on Chi-square distribution, because the partitioning of the continuous covariate would result in a situation where the total number of the distinct groups is bigger than the sample size. Hence, the Pan (2002) developed these two tests (Pearson chi-square G and the unweighted sum of squares U) to circumvent this problem.
QIF’s goodness-of-fit method is based on an objective function that is approximately chi-square distributed with appropriate DoF. It shares similar asymptotic properties to that of the likelihood ratio test, which is negative twice the log-likelihood [ \(-2\times (\mathrm{log}(l\left(.\right))\) ] [ 6 ]. This is one of the advantages QIF has over GEE1 [ 6 , 8 , 9 ]. The QIF’s objective function can be constructed from models with a working correlation structure different from the independence, unlike the GEE1’s QIC which is only based on an independence working correlation structure [ 41 ].
Description of the four CRCT datasets
Ponder trial [ 54 ].
The PoNDER CRCT aimed to assess the effect of two psychologically informed interventions by health visitors on postnatal depression in postnatal women who have recently given birth. One hundred and one general practices (clusters) in the Trent region of England were included in the trial. The general practices were randomised in a 2:1 ratio to the Intervention group ( n = 63 clusters) or the control group ( n = 38 clusters). Health visitors in the intervention clusters were trained to identify depressive symptoms at six to eight weeks postnatally using the Edinburgh postnatal depression scale (EPDS) and were also trained in providing psychologically informed sessions based on cognitive behavioural or person-centred principles for an hour a week for eight weeks. Health visitors in the control group provided usual care.
The primary outcome was the score on the EPDS at six months follow-up. The EPDS consists of 10 questions and generates a score on a 0 to 30 scale with higher scores indicating a great risk of depression. For the PoNDER trial, this outcome was dichotomised into a binary outcome of EPDS score < 12 vs \(\ge\) 12 with women with a score of 12 or more classified as “at risk” of postnatal depression. One hundred ( n = 63 intervention, n = 37 control) clusters and n = 2659 new mothers (1745 Intervention: 913 Control) provided valid primary outcome data at 6 months. Also, one of the secondary outcomes in the PoNDER trial “the mean EPDS score at six months” was used as a continuous outcome in this study. In the original study, both outcomes were analysed using GEE1 and an exchangeable correlation structure with robust standard errors. The descriptive statistics of the trial size are presented in Table 2 below.
Informed choice trial [ 55 ]
This study was aimed at investigating the impact of a set of 10 pairs of evidence-based leaflets – The Midwives’ Information and Resource Service (MIDIRS) and NHS Centre for Reviews and Dissemination informed choice leaflets through a survey. The study was designed to cover 8 of the 10 MIDIRS decision points in everyday maternity care. Conducted in 12 large maternity units in Wales, the maternity units were grouped into 10 clusters. Pairs of clusters were randomly assigned to the intervention arm and control arm based on their annual numbers of deliveries to achieve balance, and undertook an unmatched analysis.
The primary objective was to improve the management of women during pregnancy and childbirth, by assessing the effect of an intervention that promotes informed choice. The primary binary outcome was the change in the proportion of women who reported exercising informed choice (yes or no). For illustration, one of the secondary outcomes "the average of the women's levels of knowledge” on the 10 topics covered in the survey was used as a continuous outcome in this current study. Knowledge of the topics was assessed on a 1 (poor) to 10 (good) scale. Two samples of different women were surveyed: the antenatal and postnatal samples. The antenatal sample is made up of all women who reached 28 weeks’ gestation within six weeks and were receiving antenatal care in any setting. The questionnaire used for the cohort covered three decision points that the women may have encountered. The postnatal sample was made up of all women who delivered live babies during a six-week period.
A questionnaire that covered the remaining five decision points was used to survey the women postnatally. The postnatal sample had a total of 3,288 women, who were cross-sectionally surveyed before ( n = 1,741) and after the intervention was administered ( n = 1,547). However, to demonstrate the fitting of the statistical methods in this study only the follow-up (i.e., after the intervention) postnatal sample was used and reported. Only women who delivered in all settings and above the age of 16 years were included. Random effects models (i.e., GLMM) were used to analyse the outcomes in the original study. A summary of the trial size is presented in Table 2 .
Bridging the age gap trial [ 56 ]
Bridging the Age Gap CRCT investigated the effects of two decision support interventions (DESIs) to support treatment choices in older women (aged \(\ge\) 70 years) with operable breast cancer [ 56 ]. Forty-six breast cancer units (clusters) in England and Wales were included in the trial. The breast cancer units were randomised to have access to the DESI (Intervention group n = 21 clusters) or to continue with usual care (Control group n = 25 clusters). The DESI comprised an online algorithm, booklet, and brief decision aid to inform choices between surgery plus adjuvant endocrine therapy versus primary endocrine therapy, and adjuvant chemotherapy versus no chemotherapy.
The primary outcome was the global health status/quality of life (QoL) score (questions 29 and 30) on the cancer-specific patient-reported outcome of the European Organisation for the Research and Treatment of Cancer (EORTC) QoL questionnaire (QLQ)-C30 at 6 months post-baseline. The EORTC QLC-C30 global health status/QoL scale is scored on a 0 to 100 scale with a higher score representing a better QoL. Forty-three clusters ( n = 19 intervention, n = 24 control), and n = 748 patients (359 Intervention: 389 Control) provided valid primary outcome data at 6 months.
The primary endpoint was a continuous outcome “Global health status quality of life score” measured 6 months after diagnosis and was analysed using GEE1 with sandwich (robust) standard errors and an exchangeable working correlation matrix. The total number of participants included in the trial is 748 distributed across 43 clusters and the cluster size ranged from size 1 to 73. A summary of the trial size is provided in Table 2 .
The Nourishing Start for Health (NOSH) trial [ 57 ]
The NOSH CRCT assessed the effect of an area-level financial incentive (shopping vouchers) on breastfeeding among new mothers (and their baby(ies)) in areas with low breastfeeding prevalence [ 57 ]. Ninety-two electoral ward areas (clusters) in England were included in the trial with baseline breastfeeding prevalence at 6 to 8 weeks postnatally of less than 40%. The areas were randomised to the financial incentive plus usual care ( n = 46 clusters) or usual care alone ( n = 46 clusters). All 92 clusters provided breastfeeding outcome data on 9,207 mother-infant pairs (4,973 in the NOSH group, 4324 in the control group) (Table 2 ).
The primary outcome was the electoral ward area-level 6 to 8 weeks breastfeeding prevalence, as assessed by clinicians at the routine 6 to 8 weeks postnatal check. This was derived from the number of new mothers who were breastfeeding or not at 6 weeks in each local authority area/cluster . A cluster-level approach was used to analyse the primary outcome after obtaining a summary measure for each cluster. Specifically, a weighted multiple linear regression model was used in the original study.
The sample size characteristics of our case studies are summarised using frequencies and percentages, and all the models were fitted using complete cases. Across the case studies, the range of the missing data was from 0 to 7% which is negligible, hence no sensitivity analysis was conducted. In clinical trials, it is not uncommon to fit both unadjusted and adjusted regression models [ 58 ]. We fitted both unadjusted and adjusted models with the four analytical approaches – GLMM (with MLE and REML), GEE1, GEE2, and QIF. The unadjusted models contained only the indicator variable \({x}_{1i}\) for the randomised treatment arms as a covariate. While the adjusted models included other known prognostic covariates \({{\varvec{X}}}_{pij}^{T}\) (with the treatment arm indicator inclusive), such as baseline outcome values, age, and sex. There are several known benefits from adjusting for prognostic covariates in an adjusted analysis, such as protection against imbalance in baseline participant prognostic covariates among groups [ 59 ], increased power and precision for linear models [ 1 , 59 , 60 ], to obtain an estimate of the intervention effect that has a closer individual level interpretation, and to account for special features of the study design like stratification and subgroup consideration [ 61 ]. A study used simulations to show that adjusting for prognostic and non-prognostic covariates led to increased and reduced power, respectively [ 59 ].
To analyse the outcome data from the trials with few clusters we fitted a GLMM (with REML). Most small sample corrections are not compatible with MLE, hence REML was used with Satterthwaite (SAT) correction [ 62 ] applied to correct its DoF of the GLMM. Corrections on the DoF of a parameter estimate only affect the P -value and CI, but the point estimate of the intervention effect remains the same as that of the uncorrected version [ 21 ]. For GEE1, Fay and Graubard (FG) correction [ 63 ] was applied to correct the robust SE of the estimate of the intervention effect, which consequently affected its P -value and CI. All the corrections used are available in R and SAS. Although small sample corrections exist for GEE2 [ 16 ] and QIF [ 64 ], they are not readily available or easy to implement in standard statistical packages, respectively, as at the time of authoring this paper.
SAS (version 9.4) and R (version 1.4.1717) statistical software packages were used for this study. GLMM and QIF models were fitted using SAS while GEE1 and GEE2 models were fitted using R. The SAS syntax and R codes for fitting all the statistical models applied to one case study (the PoNDER trial) are provided (see, Additional file 4 ).
The GLMM models were fitted using the GLIMMIX procedure in SAS and we set the quadrature points (nodes) to 10 for the AGHQ algorithm. Higher nodes increase the complexity of the AGHQ procedure but produce more reliable results than lower nodes [ 26 ]. SAS PROC GLIMMIX does not produce a value for the ICC, so we calculated it using the estimates of the between cluster variation and individual variation from the PROC GLIMMIX GLMM output.
The QIF models were fitted using the qif macro in SAS. In the GEE2 models, no covariate was adjusted for the working correlation and scale parameters. The link function for the mean structure was either identity for a continuous or logit for a binary outcome, for the scale structure it was the identity, and for the correlation structure modified Fisher’s z transformation was used. GEE1 models were fitted using the geeglm function of R’s geepack package with an exchangeable correlation structure, and so was GEE2 using the geese function.
We assumed an exchangeable working correlation structure for all PAMs in this study, which is reasonable for a CRCT design, and it is the most assumed working correlation structure in CRCTs [ 31 , 65 ]. Although the LMM was used to analyse all continuous outcomes, we labelled its results as GLMM for simplicity. In each analysis, we consider a P -value \(<0.05\) to mean that the result is statistically significant. The results for each of the four CRCTs are presented below.
PoNDER trial
It is worth noting the key features of the PoNDER trial [ 54 ]. The PoNDER trial had many clusters (~ 100) with an average cluster size of twenty-seven. Two outcomes were analysed, the mean EPDS score at six months (continuous) and EPDS score < or \(\ge\) 12 at six months (binary), multiple covariates were adjusted for in the adjusted modelling including the baseline outcome covariate. The focus is to investigate and discuss (see, Discussion Section for more) the impact of these features on the parameter estimates from the different statistical methods.
The mean age of all the women in the control and intervention groups was the same (32 \(\pm\) 5yrs, respectively), and the maximum age for all women was 46 years. The proportion of women with EPDS score \(\ge\) 12 at 6 months was 16% (150/914) in the control arm and 12% (205/1745) in the intervention arm. For the continuous outcome “the mean EPDS score at six months”, was 6.4(SD = 5.0) vs. 5.5(SD = 4.9) for the control vs the intervention arms, respectively. It is worth noting that for both outcomes, smaller is better. The estimates of the unadjusted intervention effect from the analysis of the continuous primary outcome are the same across the models (mean difference = -1.00), except for QIF (-0.94). After adjustments were made for the baseline EPDS 6 weeks score, living alone, previous history of major life events, and previous history of postnatal depression, the estimates of the intervention effect became the same across the models (mean difference = -0.8, 1 d.p).
The SEs of the intervention effect estimates were the same across the models, 0.3, for the unadjusted models and 0.2 for the adjusted models. The intervention effect estimates across the models were significant as evidenced by the small P -values (< 0.05) and the confidence intervals which excluded zero. Similar results were obtained from the binary outcome analysis, the odds ratio was approximately 0.7 across unadjusted and adjusted models, except for the adjusted QIF model (Odds ratio = 0.6). All the results were statistically significant, suggested by their small P -values and CIs that excluded one (Table 3 ). Adjusting covariates in the logistic models did not affect the magnitude of the estimates of the intervention effect from the different models, except QIF (though slightly). These results are graphically compared using forest plots and shown in Fig. 2 (a, b) and Fig. 3 (a, b). Looking at the plots all the point estimates for the intervention effect and the associated 95% confidence intervals (CIs) are to the left-hand side of zero favouring the intervention arm. The width of the whiskers that represent the 95% CIs is approximately the same for all the models.
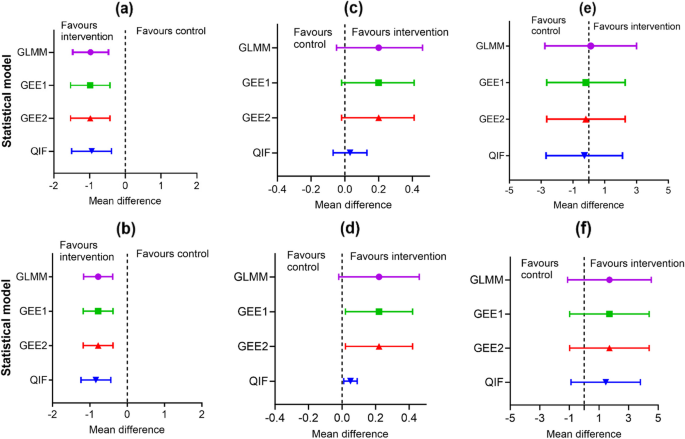
Forest plots showing the intervention effect estimate and its associated 95% CI for the four statistical models fitted using the continuous outcomes of three of the four CRCTs, where plots ( a ) & ( b ) are the unadjusted and the adjusted models fitted on the outcome data from PoNDER trial respectively, ( c ) and ( d ) is that of Informed choice and ( e ) & ( f ) is that of Bridging the Age Gap trial. The electronic version is in colour
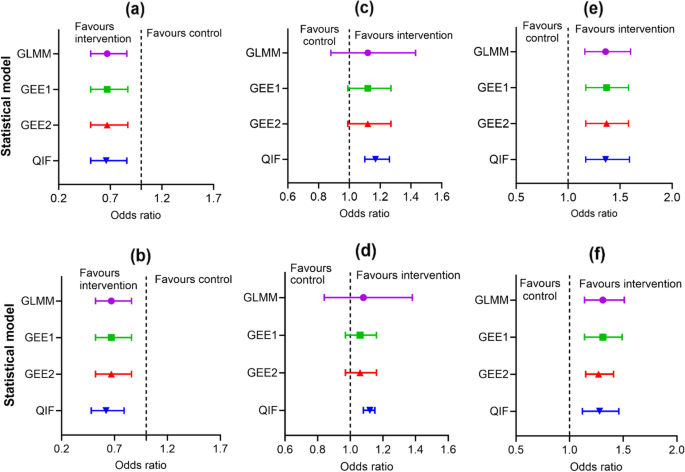
Forest plots showing the intervention effect estimate and its associated 95% CI for each of the statistical model fitted on the binary outcomes of three cluster trials datasets where plots ( a ) & ( b ) are the unadjusted and the adjusted models fitted to the outcome data from PoNDER trial respectively, ( c ) and ( d ) is that of the Informed Choice trial, and ( e ) & ( f ) is for the NOSH trial. Electronic version is in colour
Informed choice trial
The Informed Choice trial had a few clusters (ten clusters) with a large average cluster size (cluster mean = 155). The analysed outcomes were “proportion of those who answered yes about making an informed choice (binary)” and “the averaged level of a woman’s knowledge about informed choice (continuous)”, and several covariates were adjusted for but none was the baseline outcome variable as this was not measured [ 55 ]. Here the interest is the impact of a small number of clusters on the estimates from the different models. In the intervention arm, 59% (477/816) of the women reported having exercised informed choice while using the maternity service compared to 57% (346/612) in the control arm. The mean knowledge of the 10 topics covered in the survey was 3.6 (SD = 1.62) for the intervention arm compared to 3.3 (SD = 1.60) for the control arm.
The results of the unadjusted and adjusted models from the analysis of the continuous and binary outcomes are presented in Table 4 and visualised in Fig. 2 (c,d) and Fig. 3 (c,d), respectively. For the continuous outcome, the unadjusted intervention effect estimates were the same for the three models (mean difference = 0.20, SE = 0.11), except for QIF (0.03, SE = 0.05). Similarly, the adjusted intervention effect estimates were the same 0.22 (SE = 0.1) for all the models except for QIF 0.05 (SE = 0.02). The intervention effect estimate from the QIF model is far more inconsistent with the observed data (difference in mean score = 0.3). The unadjusted intervention effects were not significant (i.e., P > 0.05), but the adjusted intervention effects were somewhat significant (i.e., P \(<0.05\) ) except for GLMM.
Similarly, for the binary outcome, the unadjusted odds ratio of women who reported exercising informed choice in the intervention arm compared to the control arm was the same for all the models (odds ratio = 1.12, SE = 0.10 to 0.11) except for QIF (1.17, SE = 0.04). The adjusted odds ratios from all the models are the same (odds ratio = 1.1, SE = 0.10 to 0.11). The unadjusted and adjusted odds ratio were not significant for all the models except that of QIF which was highly significant ( P < 0.0001) (see, Table 4 ).
The results of applying small sample corrections are summarised in Table 5 . When compared to the results from the uncorrected version in Table 4 , the differences lie in the P -values and 95% CIs of the treatment effect estimates, for both the continuous and binary outcomes. The corrected P -values are bigger, and the CIs are wider (Table 5 ).
Bridging the age gap trial
The key features of Bridging the Age Gap trial are, a moderate number of clusters (forty-three clusters) with an average size of eighteen, the continuous outcome measured was global health status/quality of life at six months (measured at baseline and follow-up periods) [ 56 ]. The focus is on how the moderate number of clusters (and moderate average cluster size) and baseline outcome values affected the estimates from the four different statistical methods.
Table 6 presents the results from the analysis of the continuous outcome data, which is graphically shown in Fig. 2 (e,f). The mean global health status/quality of life (QoL) score at the 6-month follow-up was 68.9 (SD 19.6) for the control arm against 69.0 (SD 19.5) for the intervention arm. The unadjusted models produced different estimates of the intervention effect ranging from a mean difference of -0.28 to 0.12 but became stable and changed direction after the baseline QoL variable ( ql scale ) was adjusted for; the mean difference became 1.71 for all the models except QIF (mean difference = 1.46). However, the SEs of the treatment effect estimates from GEE1 and GEE2 increased while that of the GLMM and QIF reduced after the baseline outcome covariate adjustment. The SEs are approximately the same for the adjusted models (1.40) except for QIF (1.20). All the SE estimates from QIF were lesser compared to the other three models, lesser SE is indicative of better precision provided that the method is not biased towards the null [ 66 ]. Hence, the results from QIF should be interpreted with caution, because QIF produced different estimates of the intervention effect compared to the other three models which could be indicative of biasedness. Nonetheless, none of the intervention effect estimates was significant (i.e., P > 0.05).
The NOSH trial
In this study, only binary outcome was measured (i.e., the prevalence of breastfeeding in the electoral ward assessed during the routine 6–8 week postnatal check), and the number of clusters randomised was large (Ninety-two clusters) [ 57 ]. The adjusted models included cluster-level baseline outcomes and local government areas as covariates. The unique feature of this trial is that only cluster-level covariates were adjusted for.
The results from the unadjusted and adjusted models are presented in Table 7 and are graphically presented in Fig. 3 (e, f). Overall, 36% (1869/4973) of mothers in the 46 clusters of the NOSH group were breastfeeding at 6 weeks compared to 30% (1299/4324) in the 46 clusters of the control group. The odds ratios that the mothers were breastfeeding at the end of the trial were approximately the same for all the unadjusted (1.40) and adjusted (1.30) models and were statistically significant. However, it is only in this trial that the intervention effects of GEE1 and GEE2 were different, in the other trials presented previously they were the same. The SEs of the unadjusted intervention effect estimate (SEs, 0.08) and the adjusted version (SEs, 0.07) were the same for all the models, except for the adjusted GEE2 (0.05).
In this paper, four different approaches for analysing CRCTs with clustering in the treatment arms have been described. The four approaches GLMM, GEE1, GEE2, and QIF have been applied to four case studies with different features to demonstrate their implementation and evaluate their use in practice. To the best of our knowledge, this is the first study to comparatively evaluate these four methods in the context of CRCTs.
The initial plan was to fit all the models using free and open software such as R, but we observed that the qif command in the R’s qif package (CRAN—Package qif (r-project.org)) could not fit the QIF model to data with clusters size of one. The PoNDER and Bridging Age Gap trials have clusters of size one, the error message suggests that it is a problem of the incompatibility of the matrices in the matrix multiplication procedure. So, we switched to using SAS which was able to overcome the problem. We communicated our observation to one of the developers of the two QIF’s functions of both software packages (i.e., R and SAS), Peter X.K. Song, through email correspondence and Song promised to investigate it. Also, the lmer command for fitting linear mixed effects model to continuous outcomes in the lme4 package in R does not have AGHQ as an option but glmer for generalized linear mixed modelling does. The SAS procedure, GLIMMIX, has AGHQ as an option for mixed effects models for both continuous and binary outcomes.
There are previous reviews that are similar to our current methodological review, but some differences still exist. A good example is the review by Murray et al., [ 67 ] where they discussed recent methodological advances in the design and analysis of group randomised trials [ 67 ]. They looked at a five years span starting from 1999 to 2004, and they identified and discussed advances in analytical methods such as the mixed effects models with parameters estimated by MLE/REML, GEE1, Bayesian mixed effects models, survival models based on MLE and Cox methods (with robust SE), and randomisation tests. Their paper was updated in 2017 by Turner et al., of which additional methods such as augmented GEE1 (AU-GEE1), QIF, TMLE, and permutation tests were identified [ 68 ].
Our current review is more consistent with the findings of Turner et al., [ 68 ] than that of Murray. Our review was a scoping methodological review making it more comprehensive, we also employed systematic searching techniques which resulted in more methods for analysing outcome data from CRCTs being identified (27 unique methods), such as quantile GEE1 [ 69 ], generalized least squares [ 70 ], AUGEE1—inverse probability weighted (AUGEE-IPW) [ 71 ], weighted jack-knife [ 70 ]. Under methods used to analyse time to event outcome, we found a quantile estimator [ 72 ], hierarchical likelihood [ 73 ], hierarchical likelihood Laplace [ 73 ], and two-stage estimator [ 74 ] (Table S 1 , see Additional file 3 ).
Another review focused on methods used in the analysis of outcome data from stepped wedge CRCT design [ 75 ]. Similarly, Arnup et al. [ 76 ] review was focused on crossover CRCT design and was a practice review [ 76 ], whereas, own current review was a methodological review encompassing all the different types of CRCT designs with a focus on all the available and appropriate methods. A recent methodological review by Caille et al., [ 77 ] considered only methods for analysing time-to-event outcome data in CRCTs. Hence the authors identified more survival methods than our current review, such as the log-rank test, Kaplan–Meier plots, Gray’s model, competing risk model, and Fine & Gray’s cumulative incidence curve model adjusted for clustering [ 77 ]. The case studies considered have small estimates for the ICC which are consistent with those reported in primary care [ 78 ] and community-based trials [ 29 ]. The observed ICCs were less than 0.05 and three out of the four studies had an ICC less than 0.02. This indicates that there was a low clustering of outcomes as expected from primary care and community-based CRCT [ 29 , 78 ]. Three studies had negative estimates for the ICC, from GEE1, GEE2, and QIF methods (i.e., from all PAMs).
Upon reading the documentation of the functions for fitting the population average models, geeglm (for GEE1), geese (for GEE2) functions in R, and the qif macro in SAS we could not ascertain which of the estimators (i.e., Eq. ( 6 ) or [ 7 ]) that is being used in computing their ICC estimates. However, it is more likely that the population average models are using Eq. ( 7 ) or a method similar to [ 7 ], which could be the reason why negative ICC estimates were obtained. From a sample survey perspective, sampling error due to finite sample cluster size compared to the population cluster size which is assumed to be infinite could be the cause of the negative ICC estimates [ 79 ]. Another reason is when there are large discrepancies in the allotment of trial resources within the clusters, this would cause large variations in the observed outcomes [ 32 ], in other words, there is competition among the experimental units for the limited available resources resulting in the large variations observed within clusters.
Our results showed that estimates for the intervention effect, SE, P-value, and 95% CI were the same for GEE1 and GEE2 models in almost all cases, they only differ in their estimates for the ICC. This means that both methods fit the same models regardless of whether the correlation parameter is estimated or considered as a nuisance within the methods formulations, however, in GEE2 models the ICC parameter is explicitly modelled which could be recourse to producing a more consistent ICC estimate (i.e., adequately accounting for clustering) compared to GEE1 [ 10 , 13 ], especially if the correlation is substantial.
If the observed ICC is anticipated to be large or varies by cluster sizes, it is recommended that models that allow for heterogenous correlation structure should be considered, such as GEE2, because it is likely to improve inference [ 10 ]. This happens to be the major merit of Yan & Fines’ 3EE GEE2 model [ 13 ] over GEE1. Hence, it would be worth investigating to know which of the two methods is adequately modelling the correlation within clusters, since if the correlation is large and misspecified it could cause some loss in efficiency of the intervention effect estimate (i.e., having treatment effect estimates within bigger SEs). This can be achieved through simulation studies, where the true ICC value is known. Accurate estimates of the ICC are needed for planning future cluster trials [ 61 , 80 ]. Our four case studies exhibited some common features of CRCT design that are unique to primary care and community-based CRCTs. The impact of these key features on the estimates from the four statistical models is evident in the results obtained.
For example, the PoNDER trial was conducted in a primary care setting and hence had a large sample size (both in the number of clusters and cluster sizes, 100 clusters with an average cluster size of 26). Hence, the unadjusted and adjusted intervention effect estimates from the different methods were the same for the continuous and binary outcomes analyses, that of QIF were slightly different. The odds ratios obtained possibly showed the noncollapsible feature of the logistic regression model (with a logit link) – where including a baseline covariate changes the size of the intervention effect estimate, if the covariate is a strong predictor of the outcome, even if it is not related to the treatment conditions [ 81 ]. Since in this particular case the estimated intervention effect did not change upon inclusion of the baseline covariates in the adjusted analysis, except for QIF, possibly indicating that the covariates are not strong predictors of the outcome.
On the aspect of hypothesis testing, the conclusions reached were the same regardless of the statistical models used and it is consistent with findings of the original analysis by Morrell et al., [ 54 ]; a significant benefit of training health visitors to adequately manage women with postnatal depressive symptoms (i.e., favouring the intervention arm). The ICC estimates were small as expected [ 29 , 78 ], and that of the population average logistic models were negative (i.e., GEE1, GEE2, and QIF). These results are consistent with the findings of Adam et al. [ 78 ], they reanalyse thirty-one CRCTs conducted within primary care settings and provided ICC estimates for several common variables. Their median unadjusted ICC was 0.01 while the adjusted was 0.005. Similarly, our results are consistent with previous simulation studies, the studies found that both cluster-specific models (typified by GLMM) and population average models (typified by GEE1) produced similar results for CRCTs that have many clusters and small ICC with binary [ 18 ] or continuous outcomes analysed [ 21 ]. Hence, for large trials with low correlation within clusters, any of the four modelling approaches (GLMM, GEE1, GEE2, and QIF) could be used. Therefore, the choice of which model to use would be based on other factors like the aim of the research.
Informed Choice trial had a few clusters (10 clusters) with a large average cluster size (median cluster size = 145). In the original study, a cross-sectional repeated measurement approach was used, so the estimate for the intervention effect was the interaction effect term between the treatment group ( group ) and time of measurement ( time ). However, for demonstration, we used only the “after intervention” postnatal sample. Both cluster and individual-level covariates were included in the adjusted models. Three of the methods produced the same estimates which differed from that of QIF, for both continuous and binary outcomes. The most obvious difference occurred in the P -values, CIs, and SEs (continuous outcome analysis only). For the continuous outcome, the adjusted P -value of GEE1 (including GEE2, and QIF) was significant whereas that of the GLMM was not (Table 4 ). This could indicate that the few clusters had more impact on the population average models compared to the cluster-specific model (typified by GLMM).
For binary outcome, the unadjusted and adjusted P -values of QIF were significant but that of the other three methods were not. This could be indicative of a possible inflated test size, and bias in the estimated intervention effect. This result is consistent with the findings of previous studies [ 15 , 16 , 17 ]. The QIF’s 95% CI of the intervention effect estimates were narrower compared to the other methods. Westgate and Braun [ 15 ] found that the impact of the interplay between the small number of clusters, covariates, and cluster size imbalance was more severe on QIF than GEE1. A correction was proposed to improve the empirically estimated covariance matrix that causes the QIF to be poorly behaved [ 17 ]. Also, GLMM was found to perform better than GEE1 in maintaining the nominal Type I error and power in trials with few clusters ( \(\le 20)\) for both continuous [ 21 ] and binary outcomes [ 23 ]. The results from this present study are consistent with these previous findings; however, it is more likely that the differing results from the QIF are due to the impact of the small number of clusters (which is a recipe for large cluster variations). Given these findings, it is likely that the QIF is severely affected by few to moderate numbers of clusters, followed by GEE1 then GLMM. Although, no simulation study has been carried out to compare these three methods in this regard, to reach a definite conclusion.
Informed Choice trial had a small number of clusters – ten clusters. Studies with small numbers of clusters have a higher risk of imbalance in covariates and outcomes across treatment arms/clusters [ 1 , 15 , 21 ]. Hence, for a study with a continuous outcome and clusters \(\le\) 20, small sample corrections are required to maintain the nominal 5% Type I error and a reasonable power [ 21 ]. Similarly, if the study measured a binary outcome and the number of clusters randomised is \(\le\) 30, a small sample correction should be applied to the DoF of GLMM, which is the number of clusters minus cluster-level parameters estimated [ 23 ]. We only applied small sample corrections in conjunction with GLMM and GEE1. Although there are recommended corrections for GEE2 [ 64 ] and QIF [ 16 ], however, they are not readily available or easy to implement in standard statistical packages, respectively. The employed small sample corrections resulted in bigger P -values and wider CIs of the intervention effect estimates. Our small sample correction findings are consistent with those of other studies [ 21 , 23 , 24 ].
Bridging the Age Gap trial had a moderate sample size (43 clusters with an average cluster size of 18 individual subjects), and small ICC estimates. Negative ICC estimates were associated with negative treatment effect estimates from the three PAMs. Theoretically, the ICC is bounded between 0 and 1. But in practice, negative ICCs can be realised from real-world data with finite samples. The GLMM model truncates the ICC to zero instead of producing a negative ICC, effectively fitting a generalized linear model (GLM) [ 82 ], but that is not the same for the other three population average models – GEE1, GEE2, and QIF [ 79 ]. Our results confirmed this, only the PAMs produced negative ICC estimates, this occurred in trials with a small to moderate number of clusters (Table 4 and Table 6 ). Regardless of the size of the ICC, it is ideal to use an analytical method that accounts for clustering in a CRCT. Across the four statistical models, the unadjusted intervention effect estimates were unstable ranging from -0.28 to 0.12 but became stable after the baseline outcome covariate was adjusted for (mean difference = 1.78), except for QIF (mean difference = 1.46) which also had the smallest SE estimates. This elucidates the importance of accounting for relevant prognostic factors in clinical trials, especially the baseline outcome covariate [ 1 ]. However, for linear models, covariate adjustment does not change the intervention effect estimate, although it does increase its precision (i.e., reduce the SE of the intervention effect estimate) [ 1 ]. In the case of a nonlinear model, covariates adjustment does affect the estimate of the intervention effect and also leads to reduced precision [ 60 ]. In general, for a balanced trial with a continuous outcome, the unadjusted and adjusted analyses would produce equivalent estimates, but the adjusted analysis will be more precise, especially when the covariates are strongly correlated with the outcome [ 1 ]. Hence, in most cases, for both linear and nonlinear models, adjusted analysis is mostly encouraged, however, the two are often reported [ 1 , 60 ].
This was similar for the SEs and the 95% CIs of the treatment effect estimate. QIF appeared to be slightly more precise than the other methods (i.e., had smaller SEs). However, this result should be interpreted with caution since the estimate of its intervention effect could be biased – methods that are biased toward the null hypothesis often tend to have smaller SEs [ 66 ]. Studies by Westgate confirmed this possibility of QIF being negatively biased for trials with small to moderate clusters [ 16 ]. Similarly, studies have found that the GLMM with parameters estimated by REML performs better than GEE1 in maintaining the nominal Type I error rate and power, for continuous [ 21 ] and binary outcomes [ 23 ] when the number of clusters is moderate or small. Nonetheless, all four statistical models resulted in the same inference and are consistent with that of the original analysis which was “no significant difference in the Global QoL between the control and the intervention arms” [ 56 ].
Lastly, for the NOSH trial with only binary outcome measured, and a large sample size (92 clusters with an average cluster size of 100 individual subjects). The parameter estimates from the four statistical approaches are the same in almost all cases, hence, their performance was equivalent. A unique finding here is that it is only in this case study that GEE2 produced a different adjusted intervention effect estimate compared to GEE1 (1.27 vs. 1.31) with SEs of 0.05 vs. 0.07, consequently, their 95% CIs were different. The key feature of the NOSH trial which is different from other case studies is that in NOSH, only cluster-level covariates were adjusted for, maybe this feature had a differing impact on the GEE1 and GEE2. Further studies are needed to confirm this.
Our results revealed some insight into the possible simulation studies that should be conducted to investigate the operating characteristics of these four analytical approaches. Simulation studies involve generating pseudo-random numbers from computer-designed experiments that mimic different settings of CRCT design [ 66 ]. For example, two of the trials had small and moderate numbers of clusters. This feature affected QIF differently – QIF had smaller estimates for the intervention effect and its SE. A simulation study where the true parameters are known and varied to cover a reasonable parameter range should be conducted. The parameters that could be varied include the number of clusters, levels of ICC, effect sizes (i.e., the true intervention effect), cluster sizes, types of outcomes, and distribution of the cluster random. This will help create different scenarios that are needed to investigate the independent and combined impact of the varied parameters on the performance of the methods. Another possible simulation study that is similar to the one stated above, but with a focus on the impact of small numbers of clusters ( \(\le\) 30 clusters), and the methods would include both the uncorrected and corrected versions (corrected of the effect of small sample) of the four methods. This study will determine how well the corrected versions of the methods perform both absolutely and relatively.
Limitations
This study employed a formal systematic search of relevant literature to capture most of the related work conducted. However, this was not an exhaustive review of all work in this area.
We have used four case studies that have arisen from our work as applied medical statisticians in clinical trial research. The results and inferences made apply to data from CRCTs with similar properties to our case studies. For example, our investigation focused on binary and continuous endpoints, studies with observed ICCs similar to trials conducted within primary care and community-based settings, used complete cases, and some having few clusters. However, this data limitation (i.e., missing data) might not result in adverse consequences since the proportions that were missing were small. Although, the other data limitations (i.e., a small number of clusters) might be.
While a small number of clusters, and incomplete data are issues in many real-world data sets, to increase the generalisability of our results to trials with different characteristics to our case studies, we hope to conduct a simulation study soon. The study will explore how our findings might change when the following parameters: cluster sizes, ICC, and number of clusters are varied.
In summary, we analysed outcome data from four CRCTs to demonstrate the applications of four statistical methods that are appropriate for analysing CRCTs. The characteristics of the four case studies covered some common settings in CRCTs; however, the generalizability of our findings should be limited to studies with similar characteristics as our case studies. In most cases, the modelling approaches produced similar results which are consistent with the original analyses. This is not uncommon, because our case studies typified primary care and community based with low clustering and common sample sizes (i.e., small, moderate, and large).
In some cases, QIF produced differing estimates compared to the other three approaches. These differences are noticeable for studies with a small to moderate number of clusters (i.e., \(\le\) 43). Although the four statistical methods were compared to each other, we cannot determine a superior method using only this example data analysis. Nonetheless, we recommend that for trials with a small to moderate number of clusters, caution should be exercised when QIF is used without small sample correction. It is necessary to conduct further research based on simulation studies to comprehensively evaluate the performances of the analytical approaches.
Availability of data and materials
Data are available upon reasonable request from BCO at [email protected].
Abbreviations
Individual randomised controlled trial
- Cluster randomised controlled trial
Targeted maximum likelihood estimator
Alternating logistic regression
Generalized estimating equations
Quadratic inference function
Maximum likelihood estimator
Linear mixed model
Generalized linear mixed model
Marginal generalized linear model
Generalized linear model
Restricted maximum likelihood
Cluster level analysis
Individual level analysis
Adaptive Gauss-Hermit quadrature
- Intracluster correlation coefficient
Moment generating function
Three estimating equations
Generalized method of moments
Samsa G, Neely M. Two questions about the analysis and interpretation of randomised trials. Int J Hyperthermia. 2018;34(8):1396–9.
Article PubMed Google Scholar
Offorha BC, Walters SJ, Jacques RM. Statistical analysis of publicly funded cluster randomised controlled trials: a review of the National Institute for Health Research Journals Library. Trials. 2022;23(1):115.
Article PubMed PubMed Central Google Scholar
Twardella D, Bruckner T, Blettner M. Statistical analysis of community-based studies – presentation and comparison of possible solutions with reference to statistical meta-analytic methods. Gesundheitswesen Bundesverb Arzte Offentlichen Gesundheitsdienstes Ger. 2005;67(1):48–55.
CAS Google Scholar
Ivers NM, Taljaard M, Dixon S, Bennett C, McRae A, Taleban J, et al. Impact of CONSORT extension for cluster randomised trials on quality of reporting and study methodology: review of random sample of 300 trials, 2000–8. BMJ. 2011;343(26 1):d5886–d5886.
Article CAS PubMed PubMed Central Google Scholar
Balzer LB, Zheng W, van der Laan MJ, Petersen ML. A new approach to hierarchical data analysis: Targeted maximum likelihood estimation for the causal effect of a cluster-level exposure. Stat Methods Med Res. 2019;28(6):1761–80. https://doi.org/10.1177/0962280218774936 .
Qu A, Lindsay BG, Bing LI. Improving generalised estimating equations using quadratic inference functions. Biometrika. 2000;87(4):823–36.
Article Google Scholar
Carey V, Zeger S, Diggle P. Modelling Multivariate Binary Data with Alternating Logistic Regressions Author ( s ): Vincent Carey , Scott L . Zeger and Peter Diggle Published by : Oxford University Press on behalf of Biometrika Trust Stable URL : https://www.jstor.org/stable/2337173 . Biometrika. 1993;80(3):517–26.
Song PXK, Jiang Z, Park E, Qu A. Quadratic inference functions in marginal models for longitudinal data. Stat Med. 2009;28(29):3683–96.
Odueyungbo A, Browne D, Akhtar-danesh N, Thabane L. Comparison of generalized estimating equations and quadratic inference functions using data from the National Longitudinal Survey of Children and Youth ( NLSCY ) database. BMC Med Res Methodol. 2008;8(28):1–10.
Google Scholar
Crespi CM, Wong WK, Mishra SI. Using second-order generalized estimating equations to model heterogeneous intraclass correlation in cluster-randomized trials. Stat Med. 2009;28(5):814–27.
Prentice RL. Correlated Binary Regression with Covariates Specific to Each Binary Observation. Biometrics. 1988;44(4):1033.
Article CAS PubMed Google Scholar
Prentice RL, Zhao LP. Estimating Equations for Parameters in Means and Covariances of Multivariate Discrete and Continuous Responses. Biometrics. 1991;47(3):825.
Yan J, Fine J. Estimating equations for association structures: estimating equations for association structures. Stat Med. 2004;23(6):859–74.
Yu H, Li F, Turner EL. An evaluation of quadratic inference functions for estimating intervention effects in cluster randomized trials. Contemp Clin Trials Commun. 2020;19:100605–100605.
Westgate PM, Braun TM. The effect of cluster size imbalance and covariates on the estimation performance of quadratic inference functions. Stat Med. 2012;31(20):2209–22.
Westgate PM. A bias-corrected covariance estimate for improved inference with quadratic inference functions. Stat Med. 2012;31(29):4003–22.
Westgate PM, Braun TM. An improved quadratic inference function for parameter estimation in the analysis of correlated data. Stat Med. 2013;32(19):3260–73.
Heo M, Leon AC. Comparison of statistical methods for analysis of clustered binary observations. Stat Med. 2005;24(6):911–23.
Ma J, Raina P, Beyene J, et al. Comparison of population-averaged and cluster-specific models for the analysis of cluster randomized trials with missing binary outcomes: a simulation study. BMC Med Res Methodol. 2013;13(9). https://doi.org/10.1186/1471-2288-13-9 .
Omar RZ, Thompson SG. Analysis of a cluster randomized trial with binary outcome data using a multi-level model. Stat Med. 2000;19(19):2675–88. https://doi.org/10.1002/1097-0258(20001015)19:193.0.co;2-a .
Leyrat C, Morgan KE, Leurent B, Kahan BC. Cluster randomized trials with a small number of clusters: Which analyses should be used? Int J Epidemiol. 2018;47(1):321–31.
Zhang X. A Tutorial on Restricted Maximum Likelihood Estimation in Linear Regression and Linear Mixed-Effects Model. A*STAR-NUS Clinical Imaging Research Center. 2015.
Thompson JA, Leyrat C, Fielding KL, Hayes RJ. Cluster randomised trials with a binary outcome and a small number of clusters: comparison of individual and cluster level analysis method. BMC Med Res Method. 2022;22(1):222.
Mcneish D, Stapleton LM, Mcneish D, Stapleton LM. Modeling Clustered Data with Very Few Clusters Modeling Clustered Data with Very Few Clusters. Multivar Behav Res. 2016;51(4):495–518.
McCulloch CE. Maximum Likelihood Algorithms for Generalized Linear Mixed Models. J Am Stat Assoc. 1997;92(437):162–70.
Handayani D, Notodiputro KA, Sadik K, Kurnia A. A comparative study of approximation methods for maximum likelihood estimation in generalized linear mixed models (GLMM). In Jawa Barat, Indonesia; 2017 [cited 2022 Apr 16]. p. 020033. Available from: http://aip.scitation.org/doi/abs/ https://doi.org/10.1063/1.4979449 .
Rodríguez G, Elo I. Intra-class correlation in random-effects models for binary data. Stata J. 2003;3(1):32–46.
Donner A. A Review of Inference Procedures for the Intraclass Correlation Coefficient in the One-Way Random Effects Model. Int Stat Rev Rev Int Stat. 1986;54(1):67.
Ukoumunne OC, Gulliford MC, Chinn S, Sterne JAC, Burney PGJ. Methods for evaluating area-wide and organisation-based interventions in health and health care: A systematic review. Health Technol Assess. 1999;3(5):x–92.
Ziegler A. Generalized estimating equations. New York: Springer; 2011. p. 144. (Lecture notes in statistics 204).
Liang KY, Zeger SL. Longitudinal data analysis using generalized linear models. Biometrika. 1986;73(1):13–22. https://doi.org/10.1093/biomet/73.1.13 .
Campbell MJ, Walters SJ. How to Design, Analyse and Report Cluster Randomised Trials in Medicine and Health Related Research [Internet]. New York, UNITED KINGDOM: John Wiley & Sons, Incorporated; 2014. Available from: http://ebookcentral.proquest.com/lib/sheffield/detail.action?docID=1662762 .
Hall DB, Severini TA. Extended generalized estimating equations for clustered data. J Am Stat Assoc. 1998;93(444):1365–75.
Ziegler A, Kastner C, Brunner D, Blettner M. Familial associations of lipid profiles: a generalized estimating equations approach. Stat Med. 2000;19(24):3345–57.
Yan J. geepack: Yet Another Package for Generalized Estimating Equations. R-News. 2002;1(2):12–4.
Højsgaard S, Halekoh U, Yan J. The R Package geepack for Generalized Estimating Equations. J Stat Softw. 2005;15(2):1–11.
Hansen LP. Generalized method of moments estimation. In: Durlauf SN, Blume LE, editors. Macroeconometrics and Time Series Analysis. London: Palgrave Macmillan UK; 2010. p. 105–18. https://doi.org/10.1057/9780230280830_13 Available from Cited 2022 Apr 24.
Ritz J, Spiegelman D. Equivalence of conditional and marginal regression models for clustered and longitudinal data. Stat Methods Med Res. 2004;13(4):309–23.
Hubbard AE, Ahern J, Fleischer NL, der Laan MV, Lippman SA, Jewell N, et al. To GEE or Not to GEE. Epidemiology. 2010;21(4):467–74.
Liang K, Zeger S. Longitudinal Data Analysis Using GLM. Biometrika. 1986;73(1):13–22.
Pan W. Akaike’s Information Criterion in Generalized Estimating Equations. Biometrics. 2001;57(1):120–5.
Agresti A, Caffo B, Ohman-Strickland P. Examples in which misspecification of a random effects distribution reduces efficiency, and possible remedies. Comput Stat Data Anal. 2004;47(3):639–53.
Litière S, Alonso A, Molenberghs G. The impact of a misspecified random-effects distribution on the estimation and the performance of inferential procedures in generalized linear mixed models: IMPACT OF A MISSPECIFIED RANDOM-EFFECTS DISTRIBUTION IN GLMM. Stat Med. 2008;27(16):3125–44.
McCulloch CE, Neuhaus JM. Misspecifying the Shape of a Random Effects Distribution: Why Getting It Wrong May Not Matter. Stat Sci [Internet]. 2011 Aug 1 [cited 2023 Apr 5];26(3). Available from: https://projecteuclid.org/journals/statistical-science/volume-26/issue-3/Misspecifying-the-Shape-of-a-Random-Effects-Distribution--Why/ https://doi.org/10.1214/11-STS361.full .
Neuhaus JM, McCulloch CE. Estimation of covariate effects in generalized linear mixed models with informative cluster sizes. Biometrika. 2011;98(1):147–62.
Barnhart HX, Williamson JM. Goodness-of-Fit Tests for GEE Modeling with Binary Responses. Biometrics. 1998;54(2):720.
Horton NJ, Bebchuk JD, Jones CL, Lipsitz SR, Catalano PJ, Zahner GEP, et al. Goodness-of-fit for GEE: an example with mental health service utilization. Stat Med. 1999;18(2):213–22.
Tsiatis AA. A note on a goodness-of-fit test for the logistic regression model. Biometrika. 1980;67(1):250–1.
Hosmer DW, Lemesbow S. Goodness of fit tests for the multiple logistic regression model. Commun Stat - Theory Methods. 1980;9(10):1043–69.
Hosmer DW, Hosmer T, Le Cessie S, Lemeshow S. A comparison of goodness-of-fit tests for the logistic regression model. Stat Med. 1997;16(9):965-80. https://doi.org/10.1002/(sici)1097-0258(19970515)16:93.0.co;2-o .
McCullagh P, Nelder JA. Generalized Linear Models [Internet]. 2nd ed. Routledge; 1989 [cited 2023 Apr 6]. Available from: https://www.taylorfrancis.com/books/9781351445856 .
Pan W. Goodness-of-Fit Tests for GEE with Correlated Binary Data. 2002.
Morrell CJ, Warner R, Slade P, Dixon S, Walters S, Paley G, Brugha T. Psychological interventions for postnatal depression: cluster randomised trial and economic evaluation. The PoNDER trial. Health Technol Assess. 2009;13(30):iii-iv, xi-xiii, 1–153. https://doi.org/10.3310/hta13300 .
O’Cathain A, Walters SJ, Nicholl JP, Thomas KJ, Kirkham M. Use of evidence based leaflets to promote informed choice in maternity care: Randomised controlled trial in everyday practice. Br Med J. 2002;324(7338):643–6.
Wyld L, Reed MWR, Collins K, Burton M, Lifford K, Edwards A, et al. Bridging the age gap in breast cancer: cluster randomized trial of two decision support interventions for older women with operable breast cancer on quality of life, survival, decision quality, and treatment choices. Br J Surg. 2021;108(5):499–510.
Relton C, Strong M, Thomas KJ, Whelan B, Walters SJ, Burrows J, et al. Effect of financial incentives on breastfeeding a cluster randomized clinical trial. JAMA - J Am Med Assoc. 2018;172(2):1–7.
Yu LM, Chan AW, Hopewell S, Deeks JJ, Altman DG. Reporting on covariate adjustment in randomised controlled trials before and after revision of the 2001 CONSORT statement: a literature review. Trials. 2010;11(1):59.
Kahan BC, Jairath V, Doré CJ, Morris TP. The risks and rewards of covariate adjustment in randomized trials: an assessment of 12 outcomes from 8 studies. Trials. 2014;15(1):139.
Hauck WW, Anderson S, Marcus SM. Should we adjust for covariates in nonlinear regression analyses of randomized trials? Control Clin Trials. 1998;19(3):249–56.
Campbell MK, Piaggio G, Elbourne DR, Altman DG. Consort 2010 statement: Extension to cluster randomised trials. BMJ Online. 2012;345(7881):1–21.
Satterthwaite FE. An Approximate Distribution of Estimates of Variance Components. Biom Bull. 1946;2(6):110.
Article CAS Google Scholar
Fay MP, Graubard BI. Small-Sample Adjustments for Wald-Type Tests Using Sandwich Estimators. Biometrics. 2001;57(4):1198–206.
Zhang Y, Preisser JS, Li F, Turner EL, Toles M, Rathouz PJ. GEEMAEE: A SAS macro for the analysis of correlated outcomes based on GEE and finite-sample adjustments with application to cluster randomized trials. Comput Methods Programs Biomed. 2023;230:107362.
Walters SJ, Morrell CJ, Slade P. Analysing data from a cluster randomized trial (cRCT) in primary care: A case study. J Appl Stat. 2011;38(10):2253–69.
Morris TP, White IR, Crowther MJ. Using simulation studies to evaluate statistical methods. Stat Med. 2019;38(11):2074–102.
Murray DM, Varnell SP, Blitstein JL. Design and analysis of group-randomized trials: a review of recent methodological developments. Am J Public Health. 2004;94(3):423–32. https://doi.org/10.2105/ajph.94.3.423 .
Turner EL. Group-randomized trials : part 2 - analysis. Am J Public Health. 2017;107(7):1078–86.
Bossoli D, Bottai M. Marginal quantile regression for dependent data with a working odds-ratio matrix. Biostatistics. 2018;19(4):529–45.
Du R, Lee JH. A weighted Jackknife method for clustered data. Commun Stat - Theory Methods. 2019;48(8):1963–80.
Prague M, Wang R, Stephens A, Tchetgen Tchetgen E, DeGruttola V, Tchetgen ET, et al. Accounting for interactions and complex inter-subject dependency in estimating treatment effect in cluster-randomized trials with missing outcomes. Biometrics. 2016;72(4):1066–77.
Cai J, Kim J. Nonparametric quantile estimation with correlated failure time data. Lifetime Data Anal. 2003;9(4):357–71.
Christian NJ, Ha ID, Jeong JH. Hierarchical likelihood inference on clustered competing risks data. Stat Med. 2016;35(2):251–67.
Chen CM, Yu CY. A two-stage estimation in the Clayton-Oakes model with marginal linear transformation models for multivariate failure time data. Lifetime Data Anal. 2012;18(1):94–115.
Barker D, McElduff P, D’Este C, Campbell MJ. Stepped wedge cluster randomised trials: A review of the statistical methodology used and available. BMC Med Res Methodol. 2016;16(1). Available from: https://www.scopus.com/inward/record.uri?eid=2-s2.0-85007523837&doi=10.1186%2Fs12874-016-0176-5&partnerID=40&md5=0dce9ce6aee4e9cada454f2b5ba73b49 .
Arnup SJ, Forbes AB, Kahan BC, Morgan KE, McKenzie JE. Appropriate statistical methods were infrequently used in cluster-randomized crossover trials. J Clin Epidemiol. 2016;74:40–50.
Caille A, Tavernier E, Taljaard M, Desmée S. Methodological review showed that time-to-event outcomes are often inadequately handled in cluster randomized trials. J Clin Epidemiol. 2021;134:125–37.
Adams G, Gulliford MC, Ukoumunne OC, Eldridge S, Chinn S, Campbell MJ. Patterns of intra-cluster correlation from primary care research to inform study design and analysis. J Clin Epidemiol. 2004;57(8):785-94. https://doi.org/10.1016/j.jclinepi.2003.12.013 .
Eldridge SM, Ukoumunne OC, Carlin JB. The intra-cluster correlation coefficient in cluster randomized trials: a review of definitions. Int Stat Rev. 2009;77(3):378–94.
Campbell MK, Elbourne DR, Altman DG. CONSORT statement: extension to cluster randomised trials. BMJ. 2004;328(7441):702LP – 708.
Daniel R, Zhang J, Farewell D. Making apples from oranges: comparing noncollapsible effect estimators and their standard errors after adjustment for different covariate sets. Biom J. 2021;63(3):528–57.
Nelder JA, Wedderburn RWM. Generalized Linear Models. J R Stat Soc Ser Gen. 1972;135(3):370.
Download references
Acknowledgements
We wish to acknowledge all trial staff, participants, and members of the trial review boards—the Data Monitoring and Ethics Committee and Trial Steering Committee. We wish to thank the Trial Chief Investigator for the Bridging the Age Gap (Age Gap study) in breast cancer trial Professor Lynda Wyld, Department of Oncology and Metabolism, University of Sheffield Medical School, Sheffield, UK, for the use of the Bridging the Age Gap trial data.
Patient and public involvement
Patients and/or the public were not involved in the design, conduct, reporting, or dissemination plans of this research.
Provenance and peer review
Not commissioned, externally peer-reviewed.
BCO’s Ph.D. is financially sponsored by the Nigerian Tertiary Education Trust Fund (TETFund) (Grant No. TETF/ES/UNIV/UTURU/TSA/2019). SJW and RMJ received funding across various projects from NIHR. SJW was a National Institute for Health Research (NIHR) Senior Investigator (NF-SI-0617–10012) supported by the NIHR for this research project. The views expressed in this publication are those of the author(s) and not necessarily those of the TETFund, NIHR, NHS, or the UK Department of Health and Social Care. These organisations had no role in the study design; in the collection, analysis, and interpretation of the data; in the writing of the report; or in the decision to submit the paper for publication.
Author information
Authors and affiliations.
Division of Population Health, School of Medicine & Population Health, University of Sheffield, Sheffield, UK
Bright C. Offorha, Stephen J. Walters & Richard M. Jacques
You can also search for this author in PubMed Google Scholar
Contributions
All authors contributed to the study concept and design. Literature reviews underpinning the work were conducted by BCO. BCO conducted the data analysis and drafted the manuscript. The first draft of the manuscript was written by BCO with contributions from SJW, and RMJ. All authors critically revised the manuscript and approved the final manuscript.
Corresponding author
Correspondence to Bright C. Offorha .
Ethics declarations
Ethics approval and consent to participate.
The need for informed consent from each participant was waived by the University of Sheffield Research Ethics Committee (Reference number 038285). The data analysed in this paper is based on published trials where ethics approvals were obtained by the original trial teams. This paper does not involve recruiting new participants and the original trial participants cannot be identified from this analysis. Additionally, all methods were done in accordance with the relevant guidelines and regulations.
Consent for publication
Not applicable.
Competing interests
The authors declare that they have no competing interests.
Additional information
Publisher’s note.
Springer Nature remains neutral with regard to jurisdictional claims in published maps and institutional affiliations.
Supplementary Information
Additional file 1..
Search strategy.
Additional file 2:
Figure S1. Trend of published papers on statistical methods for analysing outcome data from cRCTs, from January 2003 to December 2020.
Additional file 3:
Table S1. The frequency of study of each statistical method for analysing outcome data from cRCTs ( N = 112).
Additional file 4.
SAS syntax and R code for fitting the models on PoNDER trial data set only SAS syntax.
Rights and permissions
Open Access This article is licensed under a Creative Commons Attribution 4.0 International License, which permits use, sharing, adaptation, distribution and reproduction in any medium or format, as long as you give appropriate credit to the original author(s) and the source, provide a link to the Creative Commons licence, and indicate if changes were made. The images or other third party material in this article are included in the article's Creative Commons licence, unless indicated otherwise in a credit line to the material. If material is not included in the article's Creative Commons licence and your intended use is not permitted by statutory regulation or exceeds the permitted use, you will need to obtain permission directly from the copyright holder. To view a copy of this licence, visit http://creativecommons.org/licenses/by/4.0/ . The Creative Commons Public Domain Dedication waiver ( http://creativecommons.org/publicdomain/zero/1.0/ ) applies to the data made available in this article, unless otherwise stated in a credit line to the data.
Reprints and permissions
About this article
Cite this article.
Offorha, B.C., Walters, S.J. & Jacques, R.M. Analysing cluster randomised controlled trials using GLMM, GEE1, GEE2, and QIF: results from four case studies. BMC Med Res Methodol 23 , 293 (2023). https://doi.org/10.1186/s12874-023-02107-z
Download citation
Received : 30 August 2022
Accepted : 17 November 2023
Published : 13 December 2023
DOI : https://doi.org/10.1186/s12874-023-02107-z
Share this article
Anyone you share the following link with will be able to read this content:
Sorry, a shareable link is not currently available for this article.
Provided by the Springer Nature SharedIt content-sharing initiative
- Statistical models
- Statistical methods
BMC Medical Research Methodology
ISSN: 1471-2288
- General enquiries: [email protected]
Thank you for visiting nature.com. You are using a browser version with limited support for CSS. To obtain the best experience, we recommend you use a more up to date browser (or turn off compatibility mode in Internet Explorer). In the meantime, to ensure continued support, we are displaying the site without styles and JavaScript.
- View all journals
- Explore content
- About the journal
- Publish with us
- Sign up for alerts
- Open access
- Published: 25 June 2024
Topical application of simvastatin acid sodium salt and atorvastatin calcium salt in vitiligo patients. Results of the randomized, double-blind EVRAAS pilot study
- Anna Niezgoda ORCID: orcid.org/0000-0002-8189-7520 1 ,
- Andrzej Winnicki ORCID: orcid.org/0000-0002-5295-5664 2 ,
- Jerzy Krysiński ORCID: orcid.org/0000-0002-6240-4444 2 ,
- Piotr Niezgoda ORCID: orcid.org/0000-0002-9912-9730 3 ,
- Laura Nowowiejska ORCID: orcid.org/0000-0002-2530-5797 4 &
- Rafał Czajkowski ORCID: orcid.org/0000-0003-3418-1252 5
Scientific Reports volume 14 , Article number: 14612 ( 2024 ) Cite this article
Metrics details
- Skin manifestations
Contemporary treatment of vitiligo remains a great challenge to practitioners. The vast majority of currently conducted clinical trials of modern therapeutic methods are focused on systemic medications, while there is only a very limited number of reports on new topical treatment in vitiligo. With their pleiotropic activities statins turned out to be efficient in the treatment of various autoimmune/autoinflammatory disorders. The randomized, double-blind placebo-controlled study of topical administration of the active forms of simvastatin and atorvastatin has been designed to evaluate their efficacy in patients with vitiligo. The study was registered in clinicaltrials.gov (registration number NCT03247400, date of registration: 11th August 2017). A total of 24 patients with the active form of non-segmental vitiligo were enrolled in the study. The change of absolute area of skin lesions, body surface area and vitiligo area scoring index were evaluated throughout the 12 week application of ointments containing simvastatin and atorvastatin. Measurements were performed with planimetry and processed using digital software. Use of active forms of simvastatin and atorvastatin did not result in a significant repigmentation of the skin lesions throughout the study period. Within the limbs treated with topical simvastatin, inhibition of disease progression was significantly more frequent than in the case of placebo ( p = 0.004), while the difference was not statistically significant for atorvastatin ( p = 0.082). Further studies of topical simvastatin in vitiligo patients should be considered.
Introduction
Vitiligo is a chronic, autoimmune/autoinflammatory skin disease characterized by the presence of markedly separated depigmented skin areas 1 , 2 Skin depigmentation derives from initial dysfunction followed by further destruction of melanocytes located in the basal layer of epidermis and hair follicles 3 , 4 .
The incidence of vitiligo accounts for 0.4–2.0% of general population 5 . No predilection for sex or ethnicity was observed. The disease may develop at any age, however it is estimated that nearly half of cases occur in adolescents younger than 20 years of age and around 70–80% of presentations before 30 years of age 6 . Rates of other autoimmune disorders are higher in vitiligo patients than in general population 7 . Nowadays, vitiligo is still a serious esthetic problem, negatively influencing patients’ quality of life, their public relations, self-confidence, which in turn may result in the development of depression and anxiety disorders 3 .
Contemporary clinical classification of vitiligo includes two major types: segmental vitiligo (SV) and non-segmental vitiligo (NSV), as well as mixed and unclassified forms 8 .
The loss of functional melanocytes, which is typical for vitiligo, has a multifactorial mechanism. It is believed that vitiligo develops in genetically predisposed individuals affected by unfavorable external (environmental) and internal factors inducing cellular stress within melanocytes, which leads to the activation of autoimmune and autoinflammatory responses 5 , 9 .
Vitiligo patients’ melanocytes have no or decreased distribution of E-cadherin mediating in adhesion of melanocytes and keratinocytes, thus they are more susceptible to oxidative stress 10 . Patients with vitiligo have an impaired function of mitochondria which are major inducers of reactive oxygen species (ROS). Formation and accumulation of ROS may in turn cause damage to DNA, oxidation, and fragmentation of proteins as well as lipid peroxidation, thus resulting in the impairment of cellular processes. Melanogenesis itself is an energy-consuming process, which generates pro-oxidative state 2 , 11 . Activation of innate immunity processes through reading of exogenously and endogenously induced stress signals released from melanocytes and likely keratinocytes occurs in the early phase of the disease. Due to oxidative stress, melanocytes induce the non-specific immune response via excretion of exosomes containing antigens specific for melanocytes such as micro-RNA (miRNA), Heat Shock Protein 70 (Hsp70) and other proteins acting as damage-associated molecular patterns (DAMPs). Exosomes provide target antigens associated with vitiligo to nearby dendritic cells, stimulating their maturation and antigen presentation to T-lymphocytes, thus combining cellular stress and acquired immunity. Cells of non-specific immunity may also locally excrete cytokines, which recruit and activate autoreactive T-lymphocytes, that actively destroy melanocytes. (Fig. 1 a) 5 , 11 , 12 .
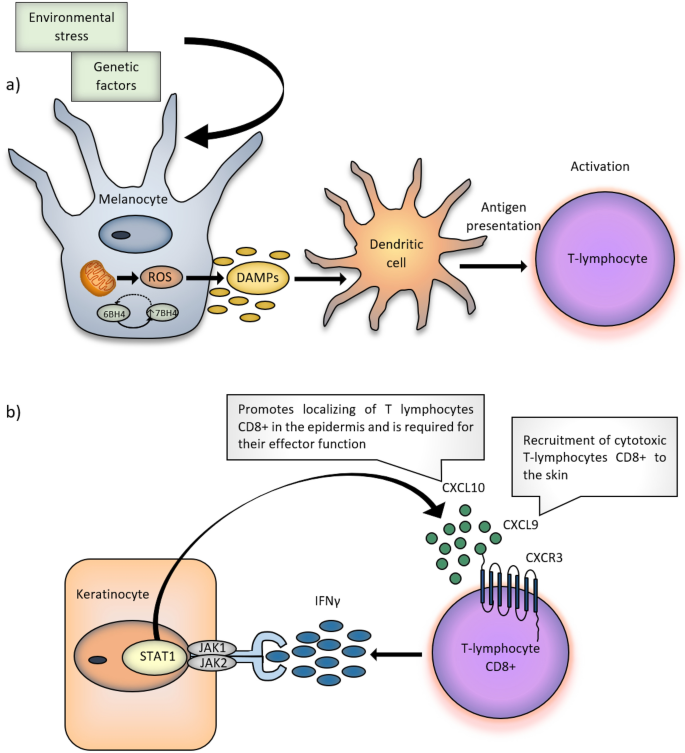
Pathogenesis of vitiligo. ( a ) In vitiligo patients, melanocytes are more susceptible to oxidative stress. The function of lipid membranes and cell proteins is altered. The abnormal function of mitochondria, the major ROS inductors, is also observed. As a result of the cellular stress, melanocytes secrete exosomes containing DAMPs. The activation of T-lymphocytes occurs after exosomes provide the dendritic cells with the antigens. ( b ) Melanocyte-specific cytotoxic CD8 + T-lymphocytes in vitiligo lesions produce cytokines, such as IFN-γ. Binding of IFN-γ to its receptor activates JAK-STAT pathway and leads to the secretion of CXCL9 and CXCL10 chemokines. By the interaction with the chemokine receptor CXCR3, CXCL9 promotes recruitment of melanocyte-specific cytotoxic CD8 + T-lymphocytes to the skin, whereas CXCL10 promotes their localization in the epidermis and their effector function, which enhances the process of destruction of the melanocytes via the positive feedback loop. 6BH4 6-tetrahydrobiopterin, 7BH4 7-tetrahydrobiopterin, CXCL9 CXC chemokine ligand 9, CXCL10 CXC chemokine ligand 10, CXCR3 chemokine receptor type 3, DAMPs damage-associated molecular patterns, IFN-γ interferon-γ, JAK 1, 2 Janus kinase 1 and 2, ROS reactive oxygen species, STAT1 signal transducer and activator of transcription 1.
Cytotoxic T CD8 + lymphocytes, are both essential and sufficient for destruction of melanocytes in patients with vitiligo. T CD8 + lymphocytes in vitiligo patients produce numerous cytokines such as interferon-γ (IFN-γ), which plays an essential role in the pathogenesis of the disease 13 . Binding of IFN-γ to its receptor activates JAK-STAT pathway and leads to secretion of CXCL9 and CXCL10 chemokines by keratinocytes. Both CXCL9 and CXCL10 have a common receptor, CXCR3. CXCL9 promotes massive recruitment of melanocyte-specific cytotoxic T CD8 + lymphocytes to the skin, whereas CXCL10 promotes their accumulation in the epidermis and their effector function, which enhances inflammation through a positive feedback loop (Fig. 1 b) 5 , 13 , 14 , 15 , 16 , 17 .
Among mechanisms inhibiting immune response, T CD4 + regulatory lymphocytes (Treg) play an important safety role. Treg deficiency within the skin of vitiligo patients is likely crucial for continuous anti-melanocyte reactivity in progressing disease 18 .
Treatment of vitiligo remains to be a serious challenge in contemporary dermatology. Current guidelines present numerous therapeutic methods comprising topical agents (corticosteroids and calcineurin inhibitors), phototherapy (NB-UVB 311 nm, PUVA), laser or 308 nm lamp, systemic glucocorticoids, transplantation of epidermis, combined methods or camouflage and depigmentation 19 . Despite this multiplicity, their efficacy is still limited, the methods are often cost-prohibitive and time-consuming. In the face of that, vitiligo is still subject of numerous ongoing clinical trials aimed at better understanding the etiopathogenesis of the disease, its connection to other systemic disorders and focused on establishing of more efficient therapeutic methods. Noteworthy, the vast majority of currently conducted clinical trials of modern therapeutic methods are focused on systemic medications, while there is only a very limited number of reports on new topical treatment in vitiligo. It needs to be underlined however, that topical treatment is associated with a noticeably lower risk of adverse events, which could be important especially in patients with a little area of vitiligous lesions or with contraindications to systemic therapy.
Statins, inhibitors of 3-hydroxy-3methylglutaryl-coenzyme A (HMG-CoA), are commonly used substances in the treatment of hypercholesterolemia. Based on available data, the positive effect on primary and secondary prevention of cardiovascular events may strongly correlate with activity which lies beyond cholesterol lowering 20 .
Statins inhibit one of the initial stages of cholesterol synthesis pathway, transformation of HMG-CoA into mevalonic acid, a primary substrate for all further reactions leading to the formation of the cholesterol molecule 21 . Through inhibiting of the aforementioned stage, HMG-CoA inhibitors lower not only the serum concentration of cholesterol, but also all intermediates in its synthesis pathway 20 . Numerous reports underline the important role of intermediate metabolites in cholesterol biosynthesis pathway, especially farnesyl pyrophosphate and geranylgeranyl pyrophosphate in the modulation of immune response. These isoprenoid pyrophosphates participate in post-translational prenylation of multiple important signal proteins, which are responsible for physiological processes such as cell growth and differentiation, endocytic and exocytic transport, intercellular signaling and apoptosis 22 , 23 . Prenylation occurs on around 100 various proteins and compounds. Among them, isoprenylation of 40 signal proteins including cell division cycle 42 (CDC42), RAC and RAS (RHO) proteins, belonging to small GTPases, which act as “molecular switches”, plays an important role. In case of all the identified prenylated proteins, which constitute around 2% of all cellular proteins, lipophilic prenyl group allows them to anchor in cell membranes, which is a primary factor determining their biological function. Apart from promotion of membranous interaction prenylation seems to play an important role in key protein–protein interactions. By inhibiting HMG-CoA reductase statins decrease also concentrations of intermediate metabolites and consequently, the activity of key signaling molecules, thus modifying the immune response independently of the lipid-lowering action 24 .
Pleiotropic effects of statins include also their antioxidative properties, which lead to the restoration of redox balance, resulting in anti-atherosclerotic and cardioprotective activity, which was confirmed in cardiovascular system 25 . Immunomodulating properties of HMG-CoA inhibitors consist of anergy of T-lymphocytes via inhibition of their clonal expansion, blocking of co-stimulatory signals and reduction of migration and influx of T-lymphocytes to the inflammatory site. Statins modify cytokine profile by switching the Th1-type response (associated with production of IFN-γ) to Th2-type. As a result of pleiotropic activity of statins, a decrease in autoreactive T-lymphocytes population and an increase of Treg population was observed. Consequently, anti-inflammatory activity exerted by statins allows to obtain a mild immunosuppression, which may be used in the treatment of multiple autoimmune diseases 26 , 27 , 28 , 29 , 30 , 31 , 32 , 33 , 34 , 35 , 36 , 37 . Selected mechanisms of statins are presented in Fig. 2 . Considering the pathophysiological aspects of vitiligo and pleiotropic activity of statins, the use of these agents may be expected to be beneficial in the therapy of vitiligo.
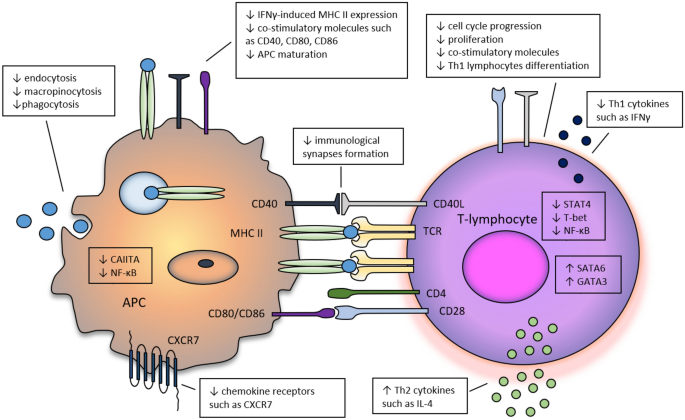
The influence of statins on the function of T-lymphocytes and antigen presenting cells. The cytokine-induced expression of MHC II and co-stimulatory molecules on antigen presenting cells (APC) and consequently, antigen presentation to T-lymphocytes are inhibited by statins. T-lymphocytes proliferation is blocked by the impact of small GTPases on the regulation of the cell cycle. Also, the organization of cytoskeleton and formation of immunological synapsis is affected by statins due to the impairment of intracellular signaling proteins’ prenylation they exert. Statins change the cytokine profile by inhibiting of the secretion of pro-inflammatory Th1 cytokines secretion and increasing of the secretion of anti-inflammatory Th2 cytokines secretion. CCR7 CC-chemokine receptor 7, CD40L CD40 ligand, CIITA class II transactivator, GATA3 GATA-binding protein 3, IFNγ interferon-γ, IL-4 interleukin 4, NF-κB nuclear factor-κB, STAT4 signal transducer and activator of transcription 4, TCR T-cell receptor.
To date, potentially positive effects of the use of statins in vitiligo were presented in literature in a case report by Noel et. al. and in an animal-model trial 17 , 38 . In both cases systemic simvastatin was used at maximum daily doses. Due to a high risk of drug intolerance and potential adverse events, especially myopathy and rhabdomyolysis, in case of systemic administration of statins in high daily doses 39 , as well as taking into account physical properties of substances which allow permeation into the skin, a study of the efficacy of topical statins applied directly onto skin lesions was designed 40 . Anti-inflammatory properties of statins applied topically were confirmed in animal models 41 , 42 , 43 , 44 .
Materials and methods
The EVRAAS pilot study was designed as a single-center, randomized, double-blind, placebo-controlled trial. Its design was approved by the Nicolaus Copernicus University (NCU) Bioethics Committee (approval no. 597/2016). The study was registered in clinicaltrials.gov (registration number NCT03247400, date of registration 11/08/2017). All study-related procedures were conducted in accordance with the rules described in The Declaration of Helsinki and guidelines of Good Clinical Practice. A written informed consent was obtained from all participants prior to any study-related procedures. The active phase of the study was carried out during the autumn–winter season (October 2016-March 2017) to minimize a potentially positive influence of sunlight on the repigmentation of skin lesions. Overall, 24 patients with an active acrofacial NSV with involvement of both upper and lower extremities were enrolled. The investigational products included ointments containing 1% simvastatin acid sodium salt and 1% atorvastatin calcium salt, whereas vehicle ointments were used as negative controls. Each study participant applied the appropriate substance onto a preselected upper and lower extremity and the vehicle ointment onto an opposite extremity. (Supplementary Material 1 ). Such a scheme enabled a direct comparison of an active substance and vehicle due to the identical biological model and environmental factors, similar localization and skin area affected by the disease. The possible combinations of application of the investigated substances were presented in the study protocol 40 .
Ointments containing active substances as well as vehicle ointments were manufactured in The Department of Pharmaceutical Technology, NCU, Collegium Medicum in Bydgoszcz. The active form of simvastatin for topical use (1% simvastatin acid sodium salt) was obtained according to the protocol described by Lin et al. with several modifications 45 . The ointments with active substances contained: atorvastatin calcium salt or simvastatin-acid sodium salt, diethylene glycol monoethyl ether and ointment absorption base – cholesterol ointment. Based on the studies conducted in The Department of Pharmaceutical Technology, NCU, substances containing 1% simvastatin acid sodium salt and 1% atorvastatin calcium salt may permeate through stratum corneum and reach stratum basale of the epidermis. Further details regarding the production of tested substances were described in the previously published study protocol 40 .
The randomization and blinding process was conducted with Random Allocation Software v.1.0 in The Department of Pharmaceutical Technology. Substances of identical organoleptic properties including color, smell, consistency, tenacity were placed in identical containers labelled with the participant number and the appropriate target extremity. The containers were then delivered to the Department of Dermatology, Sexually Transmitted Diseases and Immunodermatology, where the clinical phase of the study was conducted.
Inclusion and exclusion criteria
Adult patients, aged 18–80, with an active form of NSV, defined as the appearance of new areas of depigmentation or progression in size of previously observed lesions within a 3-month period preceding the screening visit, and the involvement of upper and lower extremities, were enrolled in the study. The main exclusion criteria included pregnancy or breast-feeding, diagnosis of segmental form of vitiligo, systemic therapy with any statin within 8 weeks before screening, hypersensitivity or allergy to simvastatin or atorvastatin, systemic therapy with immunosuppressive agents, phototherapy or surgical treatment of vitiligo within a predefined period preceding screening. A complete list of inclusion and exclusion criteria were presented in the study protocol 40 .
Study design
All participants attended study visits as follows: baseline visit – week 0 followed by visits at 4,8 and 12 weeks as previously described 40 . During each visit the areas of skin depigmentation were assessed with regard to absolute area (cm 2 ), body surface area (BSA) and vitiligo area scoring index (VASI) scales were calculated. The photographic data were analyzed with the planimetric method, using Nikon NIS Elements digital software (Fig. 3 ). Patients had to return used containers at follow-up visits and were provided with new ones with study substances which were weighed before distribution.
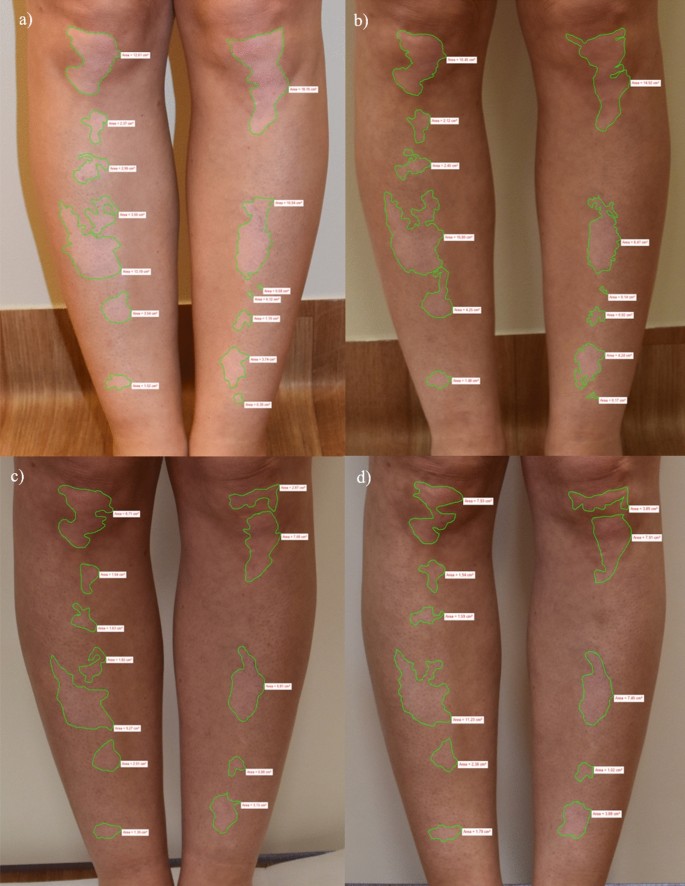
Measurements of the vitiligous lesions at predefined study time points ( a – d ) performed using Nikon D5500 photo camera assessed with Nikon NIS Elements software.
Study endpoints
The primary endpoint of the study was defined as repigmentation of vitiligous lesions assessed with the change in absolute area, BSA and VASI scales after a 12-week topical therapy with 1% simvastatin acid sodium salt and 1% atorvastatin calcium salt. Secondary endpoints included the assessment of adverse events related to the treatment, distribution of patients who achieved no improvement, poor, moderate, good and excellent improvement in absolute area, BSA, VASI on treatment. A complete list of study endpoints was presented in the study protocol 40 .
Statistical analysis
Statistical analysis was performed using Statistica v. 13.3 software (Statsoft). The sample size calculation was done with an assumed 80% power of the test used at a significance level of α = 0.05. The p value < 0.05 was considered statistically significant for each analyzed parameter. For each parameter descriptive statistics was done. Qualitative variables were presented using percentage structural indicators, while quantitative variables were presented using measures of position (mean, median, quartiles Q1 and Q3, and dispersion—standard deviation, quartile range). Due to non-normally distributed variables, Friedmann test with post hoc Holm-Bonferroni correction and Mann–Whitney test were employed to compare the differences in the absolute area of vitiligous lesions, BSA and VASI between the study groups.
A total of 24 patients (8 males and 16 females) with a mean age of 41.67 (18–69) years completed the study scheme. The mean time from the first diagnosis of NSV was 12.27 (0.5–50) years. The vast majority had undergone topical treatment (N = 19, 79.17%) or phototherapy (N = 16, 66.67%) of vitiligo in the past, however only 2 patients (8.33%) and 6 patients (25%) had achieved an improvement on topical treatment and phototherapy respectively (Table 1 ). The primary endpoint of the study showed no significant differences in the change of the analyzed parameters, absolute area of the lesions, BSA and VASI throughout the study period (Table 2 , Fig. 4 ). Also, a direct comparison of simvastatin vs. placebo and atorvastatin vs. placebo revealed no significant differences in the change of the aforementioned parameters (Table 3 ).
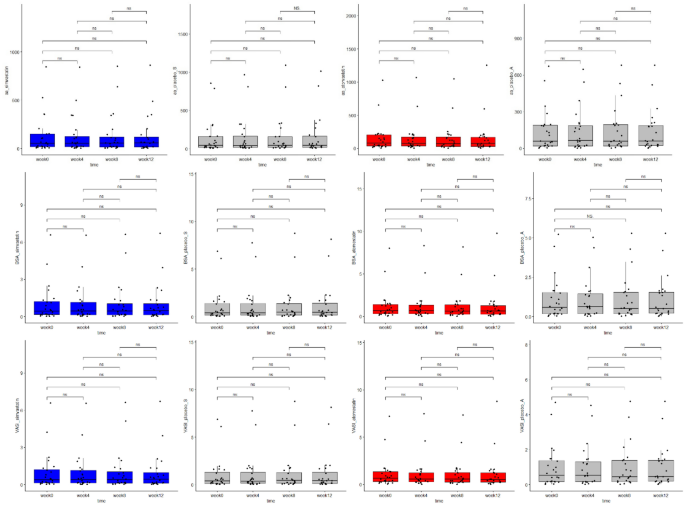
Primary endpoint of the EVRAAS study – the change of absolute area, BSA and VASI throughout the 12-week study period. BSA body surface area, VASI vitiligo area scoring index, ns non-significant.
The safety analysis of the study showed good tolerance of the tested therapeutic strategies. Only two cases of contact dermatitis were reported in the simvastatin arm. Significant differences were found in the percentage of patients who achieved no improvement on simvastatin vs. placebo in terms of the absolute area, BSA and VASI throughout the 12-week study period (10 patients, 41.7% vs. 17 patients, 70.8%; p = 0.008 for absolute area and p = 0.041 for BSA and VASI). Similarly, the percentage of patients who achieved no improvement was lower in atorvastatin vs. placebo group (8 patients, 33.3% vs. 15 patients, 62.5% respectively, p = 0.043). The percentages of patients with poor, moderate, good and excellent improvement did not differ significantly (Table 4 ). The analysis of progression of the disease (defined as at least 1% increase in the absolute area, BSA or VASI from baseline throughout the study period) showed significantly lower rates of progression vs. no progression in the simvastatin arm than in the placebo arm (29.2 vs. 70.8% and 70.8 vs. 29.2%, p = 0.004 respectively). The difference between atorvastatin and placebo in terms of rates of progression and no progression was insignificant (33.3 vs. 58.3% and 66.7 vs. 41.7%, p = 0.082 respectively) – Fig. 5 . The analysis of correlations between the time from first diagnosis of NSV and repigmentation measured in change of the absolute area, BSA and VASI showed no significant differences neither for simvastatin (correlation coefficient: 0.218, 0.183 and 0.183 respectively) nor for atorvastatin (correlation coefficient: 0.213, 0.210, and 0.222 respectively). Moreover, no significance was observed in the correlation between daily ointment use (g/cm 2 ) and repigmentation for simvastatin or atorvastatin ( p = 0.057, p = 0.056, p = 0.063 for change in the absolute area, BSA and VASI respectively in both simvastatin and atorvastatin groups).
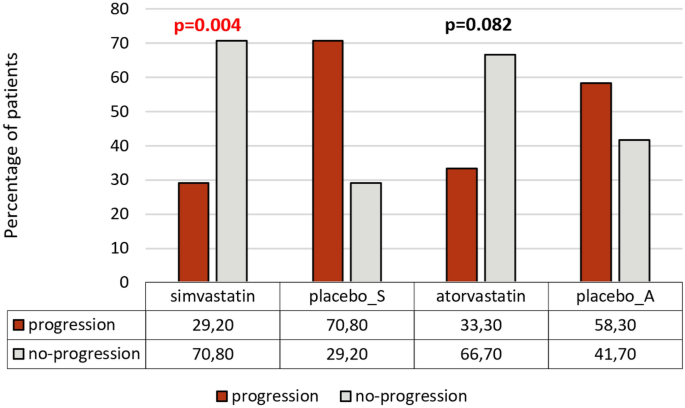
Percentages of patients with progression vs. no progression of the skin lesions assessed with the absolute area/BSA/VASI between the study arms.
Currently, most data suggest that the most important aspect of therapeutic activity of statins is their ability to modulate a wide range of proinflammatory immune mechanisms, mainly via inhibiting of small GTPases and other prenylated proteins, which leads to among others, attenuating of oxidative stress, blocking of leukocytes chemotaxis, antigen presentation, activation and proliferation of lymphocytes and switching of the cytokine profile and co-stimulatory molecules expression 24 . As a result of attenuation of protein prenylation, HMG-CoA inhibitors modulate multiple proinflammatory pathways without undesired effects on other key pathways, which are essential for the survival of the cell. Pleiotropic effects of statins are very wide. Data on their efficacy in animal models of autoimmune diseases including vitiligo 17 , experimental encephalitis and myelitis 32 , experimental myocarditis 46 , experimental arthritis 47 provide convincing premises to conduct a clinical trial in humans.
The first report of the beneficial effect of systemic simvastatin on repigmentation of vitiligous lesions is a publication by Noel et al, where a case of a 55 year-old patient who improved on oral simvastatin at a daily dose of 80 mg after unsuccessful previous therapies was presented. A noticeable repigmentation was observed on such treatment, which was documented with sequential photographs 38 . The effects of systemic statins were evaluated in several clinical studies in both humans and animal models of vitiligo. One of these studies is a trial conducted by Agarwal et. al. aiming to study the impact of intraperitoneal administration of three doses of simvastatin (0.2 mg, 0.4 mg, 0.8 mg) on repigmentation in mice with experimental vitiligo. The study showed that a 5-week treatment with simvastatin administered 3 times a day reduced depigmentation in comparison with the control group, where only placebo was used. Interestingly, a strong correlation between a clinical response and a daily dose of simvastatin was observed, with the most beneficial effect of a dose 0.8 mg. These findings justified the conduction of human clinical trials evaluating the administration of simvastatin as a potential method of treatment of vitiligo 17 . Another study aiming to evaluate the influence of simvastatin on skin repigmentation in patients with vitiligo is a small, phase 2, double-blind, randomized trial conducted by Vanderweil et al. 48 . In the active group receiving simvastatin 40 mg daily for a month followed by a dose of 80 mg daily for the next 5 months (n = 8) a mean progression of the disease assessed with VASI scale was 26% (95% confidence interval (CI), -45–97%), whereas in the control group (n = 7) the progression was 0% (95% CI − 5–5%), but the difference did not reach significance ( p = 0.094). In simvastatin group 3 participants withdrew from the study. Moreover, adverse effects including myalgia (n = 4), diarrhea (n = 3), increase in the serum concentration of aminotransferases (n = 3) and phosphocreatine kinase (n = 4) and vertigo (n = 1) were reported in the simvastatin arm. The results of this study do not justify the use of simvastatin as a potential method of treatment of vitiligo. The unfavorable results were driven by the severe progression in one patient in simvastatin arm, who developed inflammatory vitiligo and doubled the area of lesioned skin. Apart from the above, noticeable differences in efficacy of such an approach may be related to the necessity to use limited daily doses of systemically administered simvastatin due to its potential toxicity, which was not applicable in a mouse model in the study by Agarwal. The therapy might have been unsuccessful due to the long period of time since first diagnosis of vitiligo as the improvement is most pronounced in relatively new cases. The study was also biased by the low number of participants as well as by the visual assessment of lesions. However, the authors conclude that topical administration of statins may prove beneficial because it would allow to achieve much higher concentrations of the medications in the treated area without the risk of adverse effects associated with their systemic use. A randomized, double-blind study by Iraji et. al. was designed to assess the effect of 0.1% betamethasone valerate cream with (n = 27) or without oral simvastatin (80 mg daily, n = 19) on repigmentation in patients with NSV and skin involvement below 20% according to BSA. After the 12 week treatment period no significant differences in VASI scale were observed, however a trend toward better improvement could be seen in case of simvastatin co-administration 49 . Zhang et al. published the results of the study of the safety and efficacy of systemic simvastatin in vitiligo 50 . In this study, five patients with vitiligo were treated with the combination of topical tacrolimus and oral simvastatin. Three participants initially received a dose of 40 mg daily and two participants a dose of 20 mg daily and after 5 weeks the dose of simvastatin was reduced to 20 mg daily for two patients. The outcomes were evaluated with Vitiligo European Task Force (VETF) scale at baseline and after 4 and 8 weeks of the therapy. Three patients achieved noticeable clinical improvement, while two remaining participants did not benefit from the administered treatment. The authors conclude that oral simvastatin is safe in the treatment of vitiligo, but it may be inefficient. It needs to be pointed out that the trial has limitations such as a small study population. In addition, both tested doses of 20 mg and 40 mg daily are widely used in the treatment of hyperlipidemia or cardiovascular disorders and the safety profile of such therapy has already been thoroughly evaluated. In a study by Shaker et al., aiming to evaluate the correlation between the concentration of lipid fractions and the severity of vitiligous lesions, overall 79 individuals diagnosed with NSV were administered oral simvastatin at a dose of 80 mg daily 51 . The severity of vitiligous lesions was assessed using VASI and vitiligo disease activity (VIDA) scales. Simvastatin was used until the normalization of the lipid profile or for 4 months, whichever occurred first. There was no significant reduction in in the VASI scale ( p = 0.098), however a significant change was observed in the VIDA scale ( p < 0.011). A high rate of the adverse events resulting in the premature withdrawal from the study, does not support oral simvastatin in a high daily dose in vitiligo patients without hyperlipidemia.
Statins can be differentiated by their hydrophilic/lipophilic properties. Simvastatin has a molecular weight of 418.6 Da and it is characterized with relatively strong lipophilic properties 52 . Despite being relatively strong, the lipophilic character of atorvastatin is weaker than in case of simvastatin. Its molecular weight is 558.6 Da 53 . Taking into account the lipophilic character of both statins a hypothesis to use them topically on lesioned skin has been put forward. Based on the rule of 500 Da presented by Bos and Meinardi, only molecules below 500 Da can permeate through the skin. Stratum corneum was believed to be the major barrier in terms of permeability of substances through the skin. The authors suggested the limitation of further research on topical agents to only ones with molecular weight below 500 Da. However, exceptions to the rule have been found, such as tacrolimus and pimecrolimus with molecular weights of 822.03 Da and 811 Da respectively 54 .
In the presented EVRAAS study, it was assumed to use topical preparations containing active forms of simvastatin and atorvastatin, namely simvastatin acid sodium salt (458.6 Da) and atorvastatin calcium salt (1209.4 Da) 55 , 56 . After dissolving in diethylene glycol monoethyl ether atorvastatin calcium salt is present in molecular dispersion in the form of atorvastatin with the molecular weight of 558.6 Da. As mentioned above, active substances used in the present study permeate through stratum corneum and reach stratum basale of the skin. The use of 1% concentration of both study preparations (simvastatin acid sodium salt and atorvastatin calcium salt) was based on data from literature available at the time of designing the study protocol in 2015 57 , 58 , 59 .
Clinical efficacy of topical use of statins has been evaluated in numerous clinical studies throughout the last years. Data available in literature present case reports or results of trials conducted in patients with several diseases including prokeratosis 60 , 61 , chronic hand eczema 62 , acne vulgaris 63 , Child syndrome 64 , 65 , chronic vascular cutaneous ulcers 66 , pressure ulcers 59 , dry eye syndrome 67 , wound healing post hemorrhoidectomy 68 , post-radiation skin toxicity 69 or seborrheic dermatitis 70 . To date, the only evidence presenting the effects of topical simvastatin in vitiligo is a case report of a 34 year-old Chinese female with no significant improvement after an 8-month period of NB-UVB therapy published by Hu et al. 71 . A preparation of 0.11% topical simvastatin solution was obtained by dissolving of simvastatin powder in glycerol. It was applied onto the skin twice daily in combination with NB-UVB used twice weekly at increasing doses (400–1200 mJ/cm 2 ). The authors report significant repigmentation reaching 95% after 4 months of the presented therapy. Having completed the phototherapy, the patient was advised to apply topical 0.1% tacrolimus twice weekly, which allowed to achieve long-lasting remission. However, in this report, the authors presented a topical form of simvastatin which is a pro-drug without an active hydroxy-acid structure, which determines its pleiotropic activity. Due to the fact there are no further data regarding the efficacy of topical statins in vitiligo patients a more detailed comparison of the EVRAAS study results is impossible.
Study limitations
Based on the power analysis of the study, considering the obtained results of changes in the absolute area of vitiligous lesions, changes in BSA and VASI, it should be concluded that the power of the EVRAAS study is low and ranges from 0.0531 to 0.2532, depending on the assessed variable. Obtainment of a study power of 0.8 would require enrollment of 150 patients in case of simvastatin and 6500 participants for atorvastatin. Therefore, further studies only on topical form of simvastatin are reasonable. Moreover, as the efficacy of topical agents used for vitiligo treatment may vary depending on the skin area involved, it would be valuable to perform further studies evaluating the repigmentation on the trunk and the head.
Conclusions
In the proposed EVRAAS study, topical administration of active forms of simvastatin and atorvastatin did not allow to achieve significant repigmentation in vitiligo patients in comparison with vehicle ointments. Interestingly, inhibition of disease progression was more common in the simvastatin arm than in the placebo arm ( p = 0.004). The rates of no improvement were significantly higher in placebo arm than in active substances arms (0.041 and 0.043 for simvastatin and atorvastatin respectively in terms of BSA and VASI scales).
Data availability
The data that support the findings of this study are available on request from the corresponding author, AN. The data are not publicly available due to the character of the obtained materials, which might compromise the privacy of research participants.
Abbreviations
Body surface area
Cell division cycle 42
Chemokine ligand
Chemokine receptor type 3
Damage-associated molecular patterns
Guanosine triphosphate hydrolases
3-Hydroxy-3-methylglutaryl coenzyme A
Heat shock protein 70
Low-density lipoprotein
Narrowband UVB
Non-segmental vitiligo
Psoralen UVA
Reactive oxygen species
Signal transducers and activators of transcription
Segmental vitiligo
T-CD4 + regulatory lymphocytes
Vitiligo area scoring index
Vitiligo European Task Force
Vitiligo disease activity
Czajkowski, R. P. W. et al. Vitiligo. Diagnostic and therapeutic recommendations of the polish dermatological society. Dermatol. Rev. Przegląd Dermatol. 106 (1), 1–15. https://doi.org/10.5114/dr.2019.83440 (2019).
Article CAS Google Scholar
Rodrigues, M. et al. New discoveries in the pathogenesis and classification of vitiligo. J. Am. Acad. Dermatol. 77 (1), 1–13. https://doi.org/10.1016/j.jaad.2016.10.048 (2017).
Article CAS PubMed Google Scholar
Fraczek, A., Owczarczyk-Saczonek, A. & Placek, W. The role of TRM cells in the pathogenesis of vitiligo-a review of the current state-of-the-art. Int. J. Mol. Sci. https://doi.org/10.3390/ijms21103552 (2020).
Article PubMed PubMed Central Google Scholar
Burgdorf, W. H. C., Plewing, G., Wolff, H. H., Landthaler, M. Braun-Falco, O. Braun-Falco Dermatologia: Czelej. 981–1007 (2010).
Bergqvist, C. & Ezzedine, K. Vitiligo: A review. Dermatology 236 (6), 571–592. https://doi.org/10.1159/000506103 (2020).
Article PubMed Google Scholar
Sehgal, V. N. & Srivastava, G. Vitiligo: compendium of clinico-epidemiological features. Indian J. Dermatol. Venereol. Leprol. 73 (3), 149–156. https://doi.org/10.4103/0378-6323.32708 (2007).
Seneschal, J., Morice-Picard, F. & Taieb, A. Vitiligo, Associated Disorders and Comorbidities (Autoimmune-Inflammatory Disorders, Immunodeficiencies, Rare Monogenic Diseases). In Vitiligo (eds Morice-Picard, F. & Taieb, A.) 125–139 (Springer, Berlin, 2019).
Chapter Google Scholar
Ezzedine, K. et al. Revised classification/nomenclature of vitiligo and related issues: The vitiligo global issues consensus conference. Pigment Cell Melanoma Res. 25 (3), E1-13. https://doi.org/10.1111/j.1755-148X.2012.00997.x (2012).
Article CAS PubMed PubMed Central Google Scholar
Sandoval-Cruz, M. et al. Immunopathogenesis of vitiligo. Autoimmun. Rev. 10 (12), 762–765. https://doi.org/10.1016/j.autrev.2011.02.004 (2011).
Wagner, R. Y. et al. Altered E-cadherin levels and distribution in melanocytes precede clinical manifestations of vitiligo. J. Invest. Dermatol. 135 (7), 1810–1819. https://doi.org/10.1038/jid.2015.25 (2015).
Ongenae, K., Van Geel, N. & Naeyaert, J. M. Evidence for an autoimmune pathogenesis of vitiligo. Pigment Cell Res. 16 (2), 90–100. https://doi.org/10.1034/j.1600-0749.2003.00023.x (2003).
Abdallah, M. et al. CXCL-10 and Interleukin-6 are reliable serum markers for vitiligo activity: A multicenter cross-sectional study. Pigment Cell Melanoma Res. 31 (2), 330–336. https://doi.org/10.1111/pcmr.12667 (2018).
Harris, J. E. et al. A mouse model of vitiligo with focused epidermal depigmentation requires IFN-γ for autoreactive CD8⁺ T-cell accumulation in the skin. J. Invest. Dermatol. 132 (7), 1869–1876. https://doi.org/10.1038/jid.2011.463 (2012).
Rashighi, M. et al. CXCL10 is critical for the progression and maintenance of depigmentation in a mouse model of vitiligo. Sci. Transl. Med. https://doi.org/10.1126/scitranslmed.3007811 (2014).
Wang, X. X. et al. Increased expression of CXCR3 and its ligands in patients with vitiligo and CXCL10 as a potential clinical marker for vitiligo. Br. J. Dermatol. 174 (6), 1318–1326. https://doi.org/10.1111/bjd.14416 (2016).
Harris, J. E. Cellular stress and innate inflammation in organ-specific autoimmunity: Lessons learned from vitiligo. Immunol. Rev. 269 (1), 11–25. https://doi.org/10.1111/imr.12369 (2016).
Agarwal, P. et al. Simvastatin prevents and reverses depigmentation in a mouse model of vitiligo. J. Invest. Dermatol. 135 (4), 1080–1088. https://doi.org/10.1038/jid.2014.529 (2015).
Klarquist, J. et al. Reduced skin homing by functional Treg in vitiligo. Pigment Cell Melanoma Res. 23 (2), 276–286. https://doi.org/10.1111/j.1755-148X.2010.00688.x (2010).
Taieb, A. et al. Guidelines for the management of vitiligo: The European dermatology Forum consensus. Br. J. Dermatol. 168 (1), 5–19. https://doi.org/10.1111/j.1365-2133.2012.11197.x (2013).
Takemoto, M. & Liao, J. K. Pleiotropic effects of 3-hydroxy-3-methylglutaryl coenzyme a reductase inhibitors. Arterioscler. Thromb. Vasc. Biol. 21 (11), 1712–1719. https://doi.org/10.1161/hq1101.098486 (2001).
Liscum, L. Chapter 14 - Cholesterol biosynthesis. Biochemistry of Lipids, Lipoproteins and Membranes. Fifth Edition 399–421 (Elsevier, Amsterdam, 2008).
Book Google Scholar
Chan, K. K., Oza, A. M. & Siu, L. L. The statins as anticancer agents. Clin. Cancer Res. 9 (1), 10–19 (2003).
CAS PubMed Google Scholar
Weber, M. S. et al. Statins in the treatment of central nervous system autoimmune disease. J. Neuroimmunol. 178 (1–2), 140–148. https://doi.org/10.1016/j.jneuroim.2006.06.006 (2006).
Greenwood, J., Steinman, L. & Zamvil, S. S. Statin therapy and autoimmune disease: From protein prenylation to immunomodulation. Nat. Rev. Immunol. 6 (5), 358–370. https://doi.org/10.1038/nri1839 (2006).
Margaritis, M., Sanna, F. & Antoniades, C. Statins and oxidative stress in the cardiovascular system. Curr. Pharm. Des. https://doi.org/10.2174/1381612823666170926130338 (2017).
Ghittoni, R. et al. Simvastatin inhibits T-cell activation by selectively impairing the function of Ras superfamily GTPases. FASEB J. 19 (6), 605–607. https://doi.org/10.1096/fj.04-2702fje (2005).
Vicente-Manzanares, M. & Sánchez-Madrid, F. Role of the cytoskeleton during leukocyte responses. Nat. Rev. Immunol. 4 (2), 110–122. https://doi.org/10.1038/nri1268 (2004).
Kotyla, P. J. Pleiotropic activity of 3-hydroxy-3-methyl-glutharyl-coenzyme a inhibitors (statins) therapeutic potential in connective tissue diseases. Ann. Acad. Med. Stetin. 60 (1), 39–45 (2014).
PubMed Google Scholar
Grip, O., Janciauskiene, S. & Lindgren, S. Pravastatin down-regulates inflammatory mediators in human monocytes in vitro. Eur. J. Pharmacol. 410 (1), 83–92. https://doi.org/10.1016/s0014-2999(00)00870-0 (2000).
Rosenson, R. S., Tangney, C. C. & Casey, L. C. Inhibition of proinflammatory cytokine production by pravastatin. Lancet 353 (9157), 983–984. https://doi.org/10.1016/S0140-6736(98)05917-0 (1999).
Dunn, S. E. et al. Isoprenoids determine Th1/Th2 fate in pathogenic T cells, providing a mechanism of modulation of autoimmunity by atorvastatin. J. Exp. Med. 203 (2), 401–412. https://doi.org/10.1084/jem.20051129 (2006).
Youssef, S. et al. The HMG-CoA reductase inhibitor, atorvastatin, promotes a Th2 bias and reverses paralysis in central nervous system autoimmune disease. Nature 420 (6911), 78–84. https://doi.org/10.1038/nature01158 (2002).
Article ADS CAS PubMed Google Scholar
Robinson, D. S. & O’Garra, A. Further checkpoints in Th1 development. Immunity 16 (6), 755–758. https://doi.org/10.1016/s1074-7613(02)00331-x (2002).
Pawlik, A. et al. Therapy with infliximab decreases the CD4+CD28- T cell compartment in peripheral blood in patients with rheumatoid arthritis. Rheumatol. Int. 24 (6), 351–354. https://doi.org/10.1007/s00296-003-0374-4 (2004).
Tang, T. T. et al. Atorvastatin upregulates regulatory T cells and reduces clinical disease activity in patients with rheumatoid arthritis. J. Lipid. Res. 52 (5), 1023–1032. https://doi.org/10.1194/jlr.M010876 (2011).
Zhang, X. & Markovic-Plese, S. Statins’ immunomodulatory potential against Th17 cell-mediated autoimmune response. Immunol. Res. 41 (3), 165–174. https://doi.org/10.1007/s12026-008-8019-z (2008).
Hot, A. & Miossec, P. Effects of interleukin (IL)-17A and IL-17F in human rheumatoid arthritis synoviocytes. Ann. Rheum. Dis. 70 (5), 727–732. https://doi.org/10.1136/ard.2010.143768 (2011).
Noel, M., Gagne, C., Bergeron, J., Jobin, J. & Poirier, P. Positive pleiotropic effects of HMG-CoA reductase inhibitor on vitiligo. Lipids Health Dis. 3 , 7. https://doi.org/10.1186/1476-511X-3-7 (2004).
Pedersen, T. R. & Tobert, J. A. Simvastatin: A review. Expert Opin. Pharmacother. 5 (12), 2583–2596. https://doi.org/10.1517/14656566.5.12.2583 (2004).
Niezgoda, A. et al. The evaluation of vitiligous lesions repigmentation after the administration of atorvastatin calcium salt and simvastatin-acid sodium salt in patients with active vitiligo (EVRAAS), a pilot study: study protocol for a randomized controlled trial. Trials 20 (1), 78. https://doi.org/10.1186/s13063-018-3168-4 (2019).
Bracht, L. et al. Topical anti-inflammatory effect of hypocholesterolaemic drugs. J. Pharm. Pharmacol. 63 (7), 971–975. https://doi.org/10.1111/j.2042-7158.2011.01302.x (2011).
Otuki, M. F., Pietrovski, E. F. & Cabrini, D. A. Topical simvastatin: Preclinical evidence for a treatment of skin inflammatory conditions. J. Dermatol. Sci. 44 (1), 45–47. https://doi.org/10.1016/j.jdermsci.2006.04.006 (2006).
Suzuki-Banhesse, V. F. et al. Effect of atorvastatin on wound healing in rats. Biol. Res. Nurs. 17 (2), 159–168. https://doi.org/10.1177/1099800414537348 (2015).
Kulkarni, N. M. et al. Topical atorvastatin ameliorates 12-O-tetradecanoylphorbol-13-acetate induced skin inflammation by reducing cutaneous cytokine levels and NF-kappaB activation. Arch. Pharm. Res. 38 (6), 1238–1247. https://doi.org/10.1007/s12272-014-0496-0 (2015).
Lin, S. K. et al. Simvastatin as a novel strategy to alleviate periapical lesions. J. Endod. 35 (5), 657–662. https://doi.org/10.1016/j.joen.2009.02.004 (2009).
Liu, W., Li, W. M., Gao, C. & Sun, N. L. Effects of atorvastatin on the Th1/Th2 polarization of ongoing experimental autoimmune myocarditis in Lewis rats. J. Autoimmun. 25 (4), 258–263. https://doi.org/10.1016/j.jaut.2005.06.005 (2005).
Palmer, G. et al. Assessment of the efficacy of different statins in murine collagen-induced arthritis. Arthritis. Rheum. 50 (12), 4051–4059. https://doi.org/10.1002/art.20673 (2004).
Vanderweil, S. G. et al. A double-blind, placebo-controlled, phase-II clinical trial to evaluate oral simvastatin as a treatment for vitiligo. J. Am. Acad. Dermatol. 76 (1), 150–1.e3. https://doi.org/10.1016/j.jaad.2016.06.015 (2017).
Iraji, F. et al. A comparison of betamethasone valerate 0.1% cream twice daily plus oral simvastatin versus betamethasone valerate 0.1% cream alone in the treatment of vitiligo patients. Adv. Biomed. Res. 6 , 34. https://doi.org/10.4103/2277-9175.203159 (2017).
Zhang, S., Zdravković, T. P., Wang, T., Liu, Y. & Jin, H. Efficacy and safety of oral simvastatin in the treatment of patients with vitiligo. J. Investig. Med. 69 (2), 393–396. https://doi.org/10.1136/jim-2020-001390 (2021).
Shaker, E. S. E., Allam, S. H., Mabrouk, M. M., Elgharbawy, N. M. & Salaam, S. F. A. Simvastatin and non-segmental vitiligo: A new potential treatment option?. Dermatol. Ther. 35 (12), e15969. https://doi.org/10.1111/dth.15969 (2022).
PubChem. Compound Summary for CID 54454, Simvastatin; Internet: Bethesda (MD): National Library of Medicine (US), National Center for Biotechnology Information; [Available from: https://pubchem.ncbi.nlm.nih.gov/compound/Simvastatin . (2021)
PubChem. Compound Summary for CID 60823, Atorvastatin Internet: Bethesda (MD): National Library of Medicine (US), National Center for Biotechnology Information; [Available from: https://pubchem.ncbi.nlm.nih.gov/compound/Atorvastatin . (2021)
Bos, J. D. & Meinardi, M. M. The 500 Dalton rule for the skin penetration of chemical compounds and drugs. Exp. Dermatol. 9 (3), 165–169. https://doi.org/10.1034/j.1600-0625.2000.009003165.x (2000).
PubChem. Compound Summary for CID 23710209, Simvastatin (sodium) Internet: Bethesda (MD): National Library of Medicine (US), National Center for Biotechnology Information; [Available from: https://pubchem.ncbi.nlm.nih.gov/compound/Simvastatin-_sodium . (2023)
PubChem. Compound Summary for CID 656846, Atorvastatin calcium trihydrate Internet: Bethesda (MD): National Library of Medicine (US), National Center for Biotechnology Information; [Available from: https://pubchem.ncbi.nlm.nih.gov/compound/Atorvastatin-calcium-trihydrate . (2023)
Adami, M. et al. Simvastatin ointment, a new treatment for skin inflammatory conditions. J. Dermatol. Sci. 66 (2), 127–135. https://doi.org/10.1016/j.jdermsci.2012.02.015 (2012).
Rego, A. C. et al. Simvastatin improves the healing of infected skin wounds of rats. Acta. Cir. Bras. 22 (Suppl 1), 57–63. https://doi.org/10.1590/s0102-86502007000700012 (2007).
Farsaei, S., Khalili, H., Farboud, E. S., Karimzadeh, I. & Beigmohammadi, M. T. Efficacy of topical atorvastatin for the treatment of pressure ulcers: A randomized clinical trial. Pharmacotherapy. 34 (1), 19–27. https://doi.org/10.1002/phar.1339 (2014).
Byth, L. A. & Byth, J. Topical simvastatin-cholesterol for disseminated superficial actinic porokeratosis: An open-label, split-body clinical trial. Australas. J. Dermatol. 62 (3), 310–313. https://doi.org/10.1111/ajd.13601 (2021).
Blue, E., Abbott, J., Bowen, A. & Cipriano, S. D. Linear porokeratosis with bone abnormalities treated with compounded topical 2% cholesterol/2% lovastatin ointment. Pediatr. Dermatol. 38 (1), 242–245. https://doi.org/10.1111/pde.14447 (2021).
Mehrpooya, M., Ghaed-Amini, F., Firozian, F., Mohammadi, Y. & Alirezaei, P. Beneficial effects of adding topical atorvastatin 5% cream to topical betamethasone 1% ointment on chronic hand eczema. Arch. Iran Med. 23 (9), 605–613. https://doi.org/10.34172/aim.2020.71 (2020).
Ahmadvand, A. et al. Evaluating the effects of oral and topical simvastatin in the treatment of acne vulgaris: A double-blind, randomized, Placebo-controlled clinical trial. Curr. Clin. Pharmacol. 13 (4), 279–283. https://doi.org/10.2174/1574884713666180821143545 (2018).
Yu, X. et al. CHILD syndrome mimicking verrucous nevus in a Chinese patient responded well to the topical therapy of compound of simvastatin and cholesterol. J. Eur. Acad. Dermatol. Venereol. 32 (7), 1209–1213. https://doi.org/10.1111/jdv.14788 (2018).
Bajawi, S. M., Jafarri, S. A., Buraik, M. A., Al Attas, K. M. & Hannani, H. Y. Pathogenesis-based therapy: Cutaneous abnormalities of CHILD syndrome successfully treated with topical simvastatin monotherapy. JAAD Case. Rep. 4 (3), 232–234. https://doi.org/10.1016/j.jdcr.2017.11.019 (2018).
Raposio, E., Libondi, G., Bertozzi, N., Grignaffini, E. & Grieco, M. P. Effects of topic simvastatin for the treatment of chronic vascular cutaneous ulcers: A pilot study. J. Am. Coll. Clin. Wound. Spec. 7 (1–3), 13–18. https://doi.org/10.1016/j.jccw.2016.06.001 (2015).
Ooi, K. G., Wakefield, D., Billson, F. A. & Watson, S. L. Efficacy and safety of topical atorvastatin for the treatment of dry eye associated with blepharitis: A pilot study. Ophthalmic Res. 54 (1), 26–33. https://doi.org/10.1159/000367851 (2015).
Ala, S. et al. Effects of topical atorvastatin (2 %) on posthemorrhoidectomy pain and wound healing: A randomized double-blind placebo-controlled clinical trial. World J. Surg. 41 (2), 596–602. https://doi.org/10.1007/s00268-016-3749-x (2017).
Ghasemi, A. et al. Topical atorvastatin 1% for prevention of skin toxicity in patients receiving radiation therapy for breast cancer: a randomized, double-blind, placebo-controlled trial. Eur. J. Clin. Pharmacol. 75 (2), 171–178. https://doi.org/10.1007/s00228-018-2570-x (2019).
Sobhan, M., Gholampoor, G., Firozian, F., Mohammadi, Y. & Mehrpooya, M. Comparison of efficacy and safety of atorvastatin 5% lotion and betamethasone 0.1% lotion in the treatment of scalp seborrheic dermatitis. Clin. Cosmet. Investig. Dermatol. 12 , 267–275. https://doi.org/10.2147/CCID.S196412 (2019).
Hu, W., Ma, Y., Lin, F., Zhou, M. & Xu, A. E. Narrowband ultraviolet B combined with topical simvastatin solution in the treatment of vitiligo: A case report. Photobiomodul. Photomed. Laser Surg. 40 (5), 362–364. https://doi.org/10.1089/photob.2021.0090 (2022).
Download references
Acknowledgements
The authors would like to thank all the employees of the The Department of Pharmaceutical Technology and The Department of Dermatology, Sexually Transmitted Diseases and Immunodermatology of the Nicolaus Copernicus University, Collegium Medicum in Bydgoszcz for their contribution to the conduction of the study.
The study was entirely financed by The Nicolaus Copernicus University in Toruń, Collegium Medicum in Bydgoszcz and did not receive any external funding.
Author information
Authors and affiliations.
T. Browicz Provincial Observation and Infectious Diseases Hospital Anna Niezgoda, Gajowa 78/17, 85-087, Bydgoszcz, Poland
Anna Niezgoda
Department of Pharmaceutical Technology, Faculty of Pharmacy, Nicolaus Copernicus University, Ludwik Rydygier Collegium Medicum in Bydgoszcz, Bydgoszcz, Poland
Andrzej Winnicki & Jerzy Krysiński
Department of Cardiology and Internal Medicine, Faculty of Medicine, Nicolaus Copernicus University, Ludwik Rydygier Collegium Medicum in Bydgoszcz, Bydgoszcz, Poland
Piotr Niezgoda
Department of Cosmetology and Aesthetic Dermatology, Faculty of Pharmacy, Nicolaus Copernicus University, Ludwik Rydygier Collegium Medicum in Bydgoszcz, Bydgoszcz, Poland
Laura Nowowiejska
Department of Dermatology and Venerology, Faculty of Medicine, Nicolaus Copernicus University, Ludwik Rydygier Collegium Medicum in Bydgoszcz, Bydgoszcz, Cuiavian-Pomeranian, Poland
Rafał Czajkowski
You can also search for this author in PubMed Google Scholar
Contributions
A.N., A.W., J.K., R.C. created the design of the study; A.W., J.K. performed the pre-clinical phase of the study with the randomization of study participants; A.N., R.C. performed the clinical phase of the study; A.N. processed the obtained data; A.N. prepared the draft of the manuscript, P.N., L.N. performed language correction; A.N., A.W., J.K., P.N., R.C. reviewed and approved the manuscript; R.C. supervised the conduction of the trial.
Corresponding author
Correspondence to Anna Niezgoda .
Ethics declarations
Competing interests.
The authors declare no conflict of interest regarding the publication of this manuscript.
Additional information
Publisher's note.
Springer Nature remains neutral with regard to jurisdictional claims in published maps and institutional affiliations.
Supplementary Information
Supplementary information 1., supplementary information 2., rights and permissions.
Open Access This article is licensed under a Creative Commons Attribution 4.0 International License, which permits use, sharing, adaptation, distribution and reproduction in any medium or format, as long as you give appropriate credit to the original author(s) and the source, provide a link to the Creative Commons licence, and indicate if changes were made. The images or other third party material in this article are included in the article's Creative Commons licence, unless indicated otherwise in a credit line to the material. If material is not included in the article's Creative Commons licence and your intended use is not permitted by statutory regulation or exceeds the permitted use, you will need to obtain permission directly from the copyright holder. To view a copy of this licence, visit http://creativecommons.org/licenses/by/4.0/ .
Reprints and permissions
About this article
Cite this article.
Niezgoda, A., Winnicki, A., Krysiński, J. et al. Topical application of simvastatin acid sodium salt and atorvastatin calcium salt in vitiligo patients. Results of the randomized, double-blind EVRAAS pilot study. Sci Rep 14 , 14612 (2024). https://doi.org/10.1038/s41598-024-65722-w
Download citation
Received : 03 May 2024
Accepted : 24 June 2024
Published : 25 June 2024
DOI : https://doi.org/10.1038/s41598-024-65722-w
Share this article
Anyone you share the following link with will be able to read this content:
Sorry, a shareable link is not currently available for this article.
Provided by the Springer Nature SharedIt content-sharing initiative
By submitting a comment you agree to abide by our Terms and Community Guidelines . If you find something abusive or that does not comply with our terms or guidelines please flag it as inappropriate.
Quick links
- Explore articles by subject
- Guide to authors
- Editorial policies
Sign up for the Nature Briefing newsletter — what matters in science, free to your inbox daily.

- Published: 31 May 2001
Bridging case-control studies and randomized trials
- Frits R Rosendaal 1
Trials volume 2 , Article number: 109 ( 2001 ) Cite this article
5435 Accesses
5 Citations
1 Altmetric
Metrics details
Randomized trials and observational studies, such as case-control studies, are often seen as opposing approaches. However, in many instances results obtained by different designs may complement each other. For instance, case-control studies on aetiology of disease may help to give the direction of future trials. In this commentary, the author discusses the purpose of randomization and observation, and under which conditions one design may be preferred to another. Randomization is useful to combat 'confounding by indication', and is therefore the design of choice for most therapeutic trials. When this confounding is not an issue, as in studies of genetic risk factors or side-effects, then case-control studies are preferred.
In this issue of Current Controlled Trials in Cardiovascular Medicine , Ray et al [ 1 ] report the results of a study on genetic and acquired risk factors for venous thrombosis in women. This paper is remarkable, not only because it focuses on women, but also because it is an observational, case-control study rather than a randomized trial.
In their editorial in the first issue of the journal, editors-in-chief Curt Furberg and Bertram Pitt did not explicitly mention randomized trials - they spoke of a journal for 'clinical trials' [ 2 ]. This suggests experimental rather than observational studies, but does not necessarily imply randomization. Nevertheless, by encouraging prospective authors to report trial results according to the Consolidated Standards of Reporting Trials guidelines [ 3 ], they implicitly made it clear that the journal was aimed at reporting randomized clinical trials.
Does this publication therefore represent a major change in policy? Did it take only a handful of issues before the editors decided to 'lower' their standards? I think not. Sir Austin Bradford Hill is credited with performing the first properly randomized trial in 1948 [ 4 ], although studies with some form of random treatment allocation antedated it by at least 50 years [ 5 ]. When we read his Principles of Medical Statistics , from the first edition in 1937 [ 6 ] to the last posthumous edition of 1984 [ 7 ], we see an increasing emphasis on randomization, the use of placebo controls and double blinding. However, even as a strong advocate for experimentation, he defined a clinical trial as a study in which we learn from a patient; up to the 12th edition he continued to quote the 1949 Presidential Address to the Royal Society of Medicine by Sir George Pickering, who argued that all that happened to a patient should be recorded.
Randomization is a tool, not a goal in and of itself. The goal of clinical research is to obtain an answer that is valid and precise, and the ultimate goal is to prevent and treat disease in the best way. Each study design has indications and contraindications. The main threats to validity in treatment studies are regression to the mean (ie improvement due to the natural course of a disorder) and 'confounding by indication' (ie incomparability of groups when the risk profile affects the choice of drug). Control groups are included to address regression to the mean, whereas randomization is aimed at creating groups with similar prognosis to combat confounding by indication. In clinical practice, physicians tailor treatment to a patient's prognosis, and so a simple comparison of patients treated with different regimens will often be biased. Because of the need to counter this confounding by indication, randomization has become nearly synonymous with good research into medical therapies. Many have broadened this to the belief that randomization is synonymous with good research, and have created a hierarchy of study designs. This is a mistake. First, randomized trials do have drawbacks. Secondly, they are not always possible, or, for that matter, ethical.
One important drawback of randomized trials is that they typically involve patients who were considered fit to enter, were likely to finish the trial, and believed, or even shown during a run-in phase, to comply with the medications. This population is quite different from the patients in the waiting room. Another important drawback is that, because the precision of an estimate is dependent on the number of patients experiencing an event, randomized trials, unless they are very large, will seldom be precise. A third drawback is that in all prospective studies, including randomized trials, it is seldom possible to relate the outcome of interest to determinants that occurred immediately before that outcome, and that might even have interacted in producing it (for instance lifestyle factors, intercurrent disease). In some cases, randomization is simply not possible, as in aetiological studies of genetic variants. Also, even for nongenetic risk factors, randomization would often lead to ethical problems (for instance, studies on the effects of alcohol).
Case-control studies, such as the one on venous thrombosis published in the present issue [ 1 ], have other indications and contraindications. In this type of study, patients with the outcome of interest are contrasted to those without, and therefore the precision of the estimate is much greater. Ideally, all patients in a certain geographical region are included, so generalizibility is better. Finally, in contrast to randomized trials and other cohort studies, patients can be seen shortly after the event and recent risk factors can be recorded.
Case-control studies also have drawbacks; if the disease changes the risk factor measurement, then inference becomes difficult (for instance, varicose veins are often seen after a deep vein thrombosis, but are probably not a cause of venous thrombosis). In studies of treatments, case-control studies, like all observational studies, may be subject to bias through confounding by indication. It is important to make a distinction between expected or intended effects (efficacy), and unintended or unexpected effects (side effects). Although in the case of efficacy confounding by indication is a likely source of bias, this is not so in the case of side effects. If physicians or patients neither intend nor expect a certain effect of a drug, then the presence of risk factors for that effect is unlikely to affect prescription, and therefore groups using and not using the drug will be comparable, and estimates will be unbiased. This can be illustrated with the effects of hormone replacement therapy. A large observational study (the Nurses' Health study) showed a strong protective effect on coronary heart disease [ 8 ] that was not confirmed in a randomized trial [ 9 ]. Both studies found very similar relative risks of venous thrombosis, which was an unexpected side effect [ 10 , 11 ].
Genetic studies on the aetiology of disease and side effects of drugs are needed to direct or complement randomized trials of therapies. For both such study types the case-control design is the best choice. It is therefore appropriate that case-control studies and randomized controlled trials are published side by side, in order to serve our ultimate goal of improving patient care.
Ray JG, Langman L, Vermeulen MJ, Evrovski J, Yeo E, Cole DEC: Genetics University of Toronto Thrombophilia Study in Women (GUTTSI): genetic and other risk factors for venous thromboembolism in women. Curr Control Clin Trials Cardiovasc Med. 2001, 2: 141-149. 10.1186/CVM-2-3-141.
Article CAS Google Scholar
Furberg C, Pitt B: Current Controlled Trials in Cardiovascular Medicine : a new journal for a new age (http://cvm.controlled-trials.com). Current Controlled Trials in Cardiovascular Medicine. 2000, 1: 1-2. 10.1186/CVM-1-1-001.
Article PubMed Central PubMed Google Scholar
Begg C, Cho M, Eastwood ELS, Horton R, Moher D, Olkin I, Pitkin R, Rennie D, Schulz K, Simel D, Stoup D: Improving the quality of reporting of randomised controlled trials. The CONSORT statement. JAMA. 1996, 276: 637-639. 10.1001/jama.276.8.637.
Article CAS PubMed Google Scholar
Medical Research Council : Streptomycin treatment of pulmonary tuberculosis. Br Med J. 1948, ii: 769-782.
Google Scholar
Fibiger J: On treatment of diptheria with serum [in Danish]. Hospitalstidende. 1898, 6: 309-325.
Hill AB: Principles of Medical Statistics, 1st ed. London: Lancet;. 1937
Hill AB, Hill ID: Principles of Medical Statistics. 12th ed. London: Edward Arnold;. 1984
Stampfer MJ, Willett WC, Colditz GA, Rosner B, Speizer FE, Hennekens CH: A prospective study of postmenopausal estrogen therapy and coronary heart disease. N Engl J Med. 1985, 313: 1044-1049.
Hulley S, Grady D, Bush T, Furberg C, Herrington D, Riggs B, Vit-tinghoff E, for the Heart and estrogen/progestin Replacement Study (HERS) Research Group: Randomized trial of estrogen plus progestin for secondary prevention of coronary heart disease in postmenopausal women. JAMA. 1998, 280: 605-613. 10.1001/jama.280.7.605.
Grodstein F, Stampfer MJ, Goldhaber SZ, Manson JE, Colditz GA, Speizer FE, Willett WC, Hennekens CH: Prospective study of exogenous hormones and risk of pulmonary embolism in women. Lancet. 1996, 348: 983-987. 10.1016/S0140-6736(96)07308-4.
Grady D, Wenger NK, Herrington D, Khan S, Furberg C, Hunninghoke D, Vittinghoff E, Hulley S: Postmenopausal hormone therapy increases risk for venous thromboembolic disease. Ann Intern Med. 2000, 132: 689-696.
Download references
Author information
Authors and affiliations.
Department of Clinical Epidemiology, Leiden University Medical Center (LUMC), C0-P, PO Box 9600, 2300 RC, Leiden, The Netherlands
Frits R Rosendaal
You can also search for this author in PubMed Google Scholar
Corresponding author
Correspondence to Frits R Rosendaal .
Rights and permissions
Reprints and permissions
About this article
Cite this article.
Rosendaal, F.R. Bridging case-control studies and randomized trials. Trials 2 , 109 (2001). https://doi.org/10.1186/cvm-2-3-109
Download citation
Published : 31 May 2001
DOI : https://doi.org/10.1186/cvm-2-3-109
Share this article
Anyone you share the following link with will be able to read this content:
Sorry, a shareable link is not currently available for this article.
Provided by the Springer Nature SharedIt content-sharing initiative
- case-control studies
- randomization
- side-effects
- therapeutics
ISSN: 1745-6215
- Submission enquiries: Access here and click Contact Us
- General enquiries: [email protected]
Study Design 101
- Helpful formulas
- Finding specific study types
- Randomized Controlled Trial
- Meta- Analysis
- Systematic Review
- Practice Guideline
- Cohort Study
- Case Control Study
- Case Reports
A study design that randomly assigns participants into an experimental group or a control group. As the study is conducted, the only expected difference between the control and experimental groups in a randomized controlled trial (RCT) is the outcome variable being studied.
- Good randomization will "wash out" any population bias
- Easier to blind/mask than observational studies
- Results can be analyzed with well known statistical tools
- Populations of participating individuals are clearly identified
Disadvantages
- Expensive in terms of time and money
- Volunteer biases: the population that participates may not be representative of the whole
- Loss to follow-up attributed to treatment
Design pitfalls to look out for
An RCT should be a study of one population only.
Was the randomization actually "random", or are there really two populations being studied?
The variables being studied should be the only variables between the experimental group and the control group.
Are there any confounding variables between the groups?
Fictitious Example
To determine how a new type of short wave UVA-blocking sunscreen affects the general health of skin in comparison to a regular long wave UVA-blocking sunscreen, 40 trial participants were randomly separated into equal groups of 20: an experimental group and a control group. All participants' skin health was then initially evaluated. The experimental group wore the short wave UVA-blocking sunscreen daily, and the control group wore the long wave UVA-blocking sunscreen daily.
After one year, the general health of the skin was measured in both groups and statistically analyzed. In the control group, wearing long wave UVA-blocking sunscreen daily led to improvements in general skin health for 60% of the participants. In the experimental group, wearing short wave UVA-blocking sunscreen daily led to improvements in general skin health for 75% of the participants.
Real-life Examples
van Der Horst, N., Smits, D., Petersen, J., Goedhart, E., & Backx, F. (2015). The preventive effect of the nordic hamstring exercise on hamstring injuries in amateur soccer players: a randomized controlled trial. The American Journal of Sports Medicine, 43 (6), 1316-1323. https://doi.org/10.1177/0363546515574057
This article reports on the research investigating whether the Nordic Hamstring Exercise is effective in preventing both the incidence and severity of hamstring injuries in male amateur soccer players. Over the course of a year, there was a statistically significant reduction in the incidence of hamstring injuries in players performing the NHE, but for those injured, there was no difference in severity of injury. There was also a high level of compliance in performing the NHE in that group of players.
Natour, J., Cazotti, L., Ribeiro, L., Baptista, A., & Jones, A. (2015). Pilates improves pain, function and quality of life in patients with chronic low back pain: a randomized controlled trial. Clinical Rehabilitation, 29 (1), 59-68. https://doi.org/10.1177/0269215514538981
This study assessed the effect of adding pilates to a treatment regimen of NSAID use for individuals with chronic low back pain. Individuals who included the pilates method in their therapy took fewer NSAIDs and experienced statistically significant improvements in pain, function, and quality of life.
Related Formulas
- Relative Risk
Related Terms
Blinding/Masking
When the groups that have been randomly selected from a population do not know whether they are in the control group or the experimental group.
Being able to show that an independent variable directly causes the dependent variable. This is generally very difficult to demonstrate in most study designs.
Confounding Variables
Variables that cause/prevent an outcome from occurring outside of or along with the variable being studied. These variables render it difficult or impossible to distinguish the relationship between the variable and outcome being studied).
Correlation
A relationship between two variables, but not necessarily a causation relationship.
Double Blinding/Masking
When the researchers conducting a blinded study do not know which participants are in the control group of the experimental group.
Null Hypothesis
That the relationship between the independent and dependent variables the researchers believe they will prove through conducting a study does not exist. To "reject the null hypothesis" is to say that there is a relationship between the variables.
Population/Cohort
A group that shares the same characteristics among its members (population).
Population Bias/Volunteer Bias
A sample may be skewed by those who are selected or self-selected into a study. If only certain portions of a population are considered in the selection process, the results of a study may have poor validity.
Randomization
Any of a number of mechanisms used to assign participants into different groups with the expectation that these groups will not differ in any significant way other than treatment and outcome.
Research (alternative) Hypothesis
The relationship between the independent and dependent variables that researchers believe they will prove through conducting a study.
Sensitivity
The relationship between what is considered a symptom of an outcome and the outcome itself; or the percent chance of not getting a false positive (see formulas).
Specificity
The relationship between not having a symptom of an outcome and not having the outcome itself; or the percent chance of not getting a false negative (see formulas).
Type 1 error
Rejecting a null hypothesis when it is in fact true. This is also known as an error of commission.
Type 2 error
The failure to reject a null hypothesis when it is in fact false. This is also known as an error of omission.
Now test yourself!
1. Having a volunteer bias in the population group is a good thing because it means the study participants are eager and make the study even stronger.
a) True b) False
2. Why is randomization important to assignment in an RCT?
a) It enables blinding/masking b) So causation may be extrapolated from results c) It balances out individual characteristics between groups. d) a and c e) b and c
← Previous Next →
© 2011-2019, The Himmelfarb Health Sciences Library Questions? Ask us .

- Himmelfarb Intranet
- Privacy Notice
- Terms of Use
- GW is committed to digital accessibility. If you experience a barrier that affects your ability to access content on this page, let us know via the Accessibility Feedback Form .
Volume 18 Supplement 2
Rethinking the pros and cons of randomiszed controlled trials and observational studies in the era of big data and advanced methods: A panel discussion
- Meeting report
- Open access
- Published: 18 January 2024
Rethinking the pros and cons of randomized controlled trials and observational studies in the era of big data and advanced methods: a panel discussion
- Pamela Fernainy 1 , 2 ,
- Alan A. Cohen 3 , 4 , 5 , 6 , 7 ,
- Eleanor Murray 8 ,
- Elena Losina 9 ,
- Francois Lamontagne 4 , 10 &
- Nadia Sourial 1 , 2
BMC Proceedings volume 18 , Article number: 1 ( 2024 ) Cite this article
5941 Accesses
2 Citations
4 Altmetric
Metrics details
Randomized controlled trials (RCTs) have traditionally been considered the gold standard for medical evidence. However, in light of emerging methodologies in data science, many experts question the role of RCTs. Within this context, experts in the USA and Canada came together to debate whether the primacy of RCTs as the gold standard for medical evidence, still holds in light of recent methodological advances in data science and in the era of big data. The purpose of this manuscript, aims to raise awareness of the pros and cons of RCTs and observational studies in order to help guide clinicians, researchers, students, and decision-makers in making informed decisions on the quality of medical evidence to support their work. In particular, new and underappreciated advantages and disadvantages of both designs are contrasted. Innovations taking place in both of these research methodologies, which can blur the lines between the two, are also discussed. Finally, practical guidance for clinicians and future directions in assessing the quality of evidence is offered.
Randomized controlled trials (RCTs) have traditionally been considered the gold standard for medical evidence because of their ability to eliminate bias due to confounding and to thereby ensure internal validity [ 1 ]. However, the primacy of RCTs is far from universally accepted by methodological experts. This is particularly true in the era of big data and in light of emerging methodologies in data science, machine learning, causal inference methods, and other research methods, which may shift how researchers view the relative quality of evidence from observational studies compared to RCTs. In this context, on February 24, 2022, a debate took place to discuss the pros and cons of randomized control trials and observational studies. This debate was intended to reach a wide audience at all levels of training and expertise, and welcomed clinicians, researchers, students, and decision-makers seeking to better navigate the complex landscape of health evidence in a fast-changing world. The webinar announcement was shared through multiple research centers and the social networks of the panelists. A broad range of attendees participated (total of 267 attendees: 35% researchers, 28% students, 16% clinicians, 5% managers and 15% other), with varying levels of methodological expertise (26% minimal, 56% moderate, and 18% advanced). The panel was composed of clinicians and researchers with methodological expertise in experimental and observational studies from the USA and Canada (authors AAC, EM, EL, FL, and NS). This article seeks to summarize areas of agreement and disagreement among discussion panelists, highlight methodological innovations, and guide researchers, students, decision-makers, and clinicians in making informed decisions on the quality of medical evidence. The debate can be viewed at https://www.youtube.com/watch?v=VNc30fab9nM&t=17s . A lay infographic of the key points of the debate is also available (Appendix A ).
In general, RCTs are studies where investigators randomly assign subjects to different treatment groups (intervention or control group) to examine the effect of an intervention on relevant outcomes [ 2 ]. In large samples, random assignment generally results in balance between both observed (measured) and unobserved (unmeasured) group characteristics [ 1 ]. In observational studies, investigators observe the effects of exposures on outcomes using either existing data such as electronic health records (EHRs) [ 3 ], health administrative data, or collected data such as through population-based surveys [ 4 ]. Thus, in observational studies, the investigator does not play a role in the assignment of an exposure to the study subjects [ 5 ].
Pros and cons of RCTs and observational studies
By and large, RCTs are well suited to establish the efficacy of interventions involving medical interventions, and can accordingly advance knowledge that is important to the work of clinicians and the subsequent improvement of patients’ well-being. Besides being prescriptive and intuitive, the key feature of RCTs is the control for confounding due to the random assignment of the exposure of interest. Under ideal conditions, this design ensures high internal validity and can provide an unbiased causal effect of the exposure on the outcome [ 6 ]. Consequently, RCTs are helpful to physicians who prescribe medications, and studies that deal with medications as interventions lend themselves to such studies. Conversely, the lack of random assignment in observational studies is a key disadvantage, opening up the possibility of bias due to confounding and requiring researchers to employ more sophisticated methods when attempting to control for this important source of bias [ 7 ]. For instance, when considering the effect of alcohol consumption on lung cancer, factors such as smoking should be considered, as smoking has been linked to both alcohol consumption and lung cancer and can therefore confound the effect of interest if not controlled. Yet, in reality, generalizability of RCTs may also be threatened due to selection bias [ 8 ] or particularities of the study population. Furthermore, randomization of the exposure only protects against confounding at baseline [ 9 ]. Confounding might occur during the course of the study, due to loss to follow up, non-compliance, and missing data [ 10 , 11 ]. These post-randomization biases are often overlooked and the benefits of randomization at baseline may give researchers and clinicians a false sense of security.
Conversely, in observational studies, researchers are keenly aware of the threat to validity due to bias and must often consider and implement methods at the design, analysis and interpretation stage to account for it [ 12 ]. An advantage of observational studies is that they allow researchers to examine the effect of natural experiments including the effect of interventions under real-world conditions [ 13 , 14 ]. This is particularly relevant when the study system is formally complex, such as for physiological and biochemical regulatory networks, healthcare systems, infectious diseases, and social networks. In this case, results may be highly contingent on many factors, for example, when assessing COVID-19 public health measures during the pandemic, determining the impact of lifestyle, or a patient belonging to an interprofessional primary care team. In these contexts, observational studies may provide better external validity than RCTs, which typically occur under well-controlled and, by the same token, often less realistic conditions. Observational studies are also preferred when RCTs are too costly, not feasible, time-intensive, or unethical to conduct [ 13 ]. For example, a RCT studying the development of melanoma would require a long follow-up period and may not be feasible. Among researchers, there is overall agreement that low-quality RCTs might not be generally superior to observational studies, but disagreement remains as to whether high-quality RCTs, as a rule, provide a higher standard of evidence [ 13 ]. For panelists, this disagreement stemmed partly from the relative weights they accorded to internal versus external validity. While no panelist felt that observational studies were systematically better than RCTs, there was disagreement as to whether the notion that RCTs are a gold standard is helpful or harmful. Still, despite this disaccord, methodological advances are opening the door to promising opportunities. Table 1 provides a succinct summary of several pros and cons of RCTs and observational studies.
Innovations and opportunities in RCTs and observational studies
Recent innovations in RCTs have facilitated or improved the results of this research method and can result in trials that are more flexible, efficient, or ethical [ 15 ]. New designs being considered in RCTs include, but are not limited to, adaptive trials, sequential trials, and platform trials. Adaptive trials, for instance, include scheduled interim looks at the data during the trial. This leads to predetermined changes based on the analyses of accumulating data, all the while maintaining trial validity and integrity [ 15 ]. Sequential trials are an approach to clinical trials during which subjects are serially recruited and study results are continuously analyzed [ 16 ]. Once enough data enabling a decision regarding treatment effectiveness is collected, the trial is stopped [ 17 ]. Platform trials focus on an entire disease or syndrome to compare multiple interventions and add or drop interventions over time [ 18 ]. Also, the development of EHRs and an expanded access to routinely-collected clinical data has resulted in RCTs being conducted within the context of EHR-based clinical trials. EHRs have the potential to advance clinical health research by facilitating RCTs in real-world settings. Many RCTs have leveraged EHRs to recruit patients or assess clinical outcomes with minimal patient contact [ 19 ]. Such approaches are considered a particularly innovative convergence of observational and experimental data, which blurs the line between these two methodologies going forward.
As well as innovations in RCTs, innovations are taking place in observational studies. The last two decades have seen the use of novel methods such as causal inference to analyze observational data as hypothetical RCTs, which have generated similar results to those of randomized trials [ 13 ]. Causal inference in observational studies refers to an intellectual discipline which allows researchers to draw causal conclusions based on data by considering the assumptions, study design, and estimation strategies [ 20 ]. Causal inference methods, through their well-defined frameworks and assumptions, have the advantage of requiring researchers to be explicit in defining the design intervention, exposure, and confounders, for example through the use of DAGs (Directed Acyclic Graphs) [ 21 ], and have helped to overcome concerns about bias in the analysis of observational studies [ 10 ]. Moreover, recently, large observational studies have become more popular in the era of big data because of their ability to leverage and analyze multiple sources of observational data [ 22 ] such as from population databases, social media, and digital health tools [ 23 ]. Another innovation is the E-value, “the minimum strength of association, on the risk ratio scale, that an unmeasured confounder would need to have with both the treatment and the outcome to fully explain away a specific treatment-outcome association, conditional on the measured covariates” [ 24 ]. The E-value is an intuitive metric to help determine how robust the results of a study are to unmeasured confounding. A summary of the methods and their application can be seen in Table 2 .
Despite the salient advances taking place, challenges and future considerations exist for both observational and experimental research methodologies (see Appendix A ). One concern is how to apply innovations to new contexts, different topics, and novel areas of research. For example, causal inference methods are widely used in pharmacoepidemiology, but have so far rarely been used in other fields such as primary care [ 44 ]. One solution could be to encourage the use of these novel techniques by developing guidelines, sensitizing medical students to these methods by including them in the curriculum, or inclusion of more impartial and open-minded journal review boards. Such measures could facilitate cross-fertilization of methods across disciplines and foster their use in more studies.
When considering RCTs and observational studies, several key take-home messages can be drawn:
No study is designed to answer all questions, and consequently, neither RCTs nor observational studies can answer all research questions at all times. Rather, the research question and context should drive the choice of method to be used.
Both observational studies and RCTs face methodological challenges and are subject to bias. While any single study is flawed, it is the hope that the body of evidence together will show consistency in the effect of the exposure. Furthermore, triangulation of evidence from observational and experimental approaches can furnish a stronger basis for causal inference to better understand the phenomenon studied by the researcher [ 10 ].
Recent methodological innovations in health research represent a paradigm shift in how studies should be planned and conducted [ 44 ]. More knowledge translation is needed to disseminate these innovations across the different health research fields.
Finally, RCTs and observational studies can result in evidence that can subsequently improve the health and clinical care for patients, the desired effect and general aim for all researchers, decision-makers, and physicians using these study methods. However, the necessity of RCTs for establishing the highest level of evidence, remains an area of substantial disagreement, and it will be important to continue discussions around these issues going forward.
Availability of data and materials
Not applicable.
Abbreviations
- Randomized controlled trial
Alan A Cohen
Ellie Murray
Elena Losina
Francois Lamontagne
Nadia Sourial
Electronic health records
Directed Acyclic Graph
Suresh K. An overview of randomization techniques: an unbiased assessment of outcome in clinical research. J Hum Reprod Sci. 2011;4(1):8–11 (PubMed PMID: 21772732. PMCID: PMC3136079. Epub 2011/07/21. eng).
Article PubMed PubMed Central Google Scholar
Bhide A, Shah PS, Acharya G. A simplified guide to randomized controlled trials. Acta Obstet Gynecol Scand. 2018;97(4):380–7 (PubMed PMID: 29377058. Epub 2018/01/30. eng).
Article PubMed Google Scholar
Tu K, Mitiku TF, Ivers NM, Guo H, Lu H, Jaakkimainen L, et al. Evaluation of Electronic Medical Record Administrative data Linked Database (EMRALD). Am J Manag Care. 2014;20(1):e15-21 (PubMed PMID: 24669409. Epub 2014/03/29. eng).
PubMed Google Scholar
Benchimol EI, Smeeth L, Guttmann A, Harron K, Moher D, Petersen I, et al. The REporting of studies Conducted using Observational Routinely-collected health Data (RECORD) statement. PLoS Med. 2015;12(10):e1001885 (PubMed PMID: 26440803. PMCID: PMC4595218 conflicts of interest to declare. Epub 2015/10/07. eng).
Jepsen P, Johnsen SP, Gillman MW, Sørensen HT. Interpretation of observational studies. Heart. 2004;90(8):956–60 (PubMed PMID: 15253985. PMCID: PMC1768356. Epub 2004/07/16. eng).
Article CAS PubMed Central Google Scholar
Akobeng AK. Understanding randomised controlled trials. Arch Dis Child. 2005;90(8):840.
Article CAS PubMed PubMed Central Google Scholar
Hammer GP, du Prel JB, Blettner M. Avoiding bias in observational studies: part 8 in a series of articles on evaluation of scientific publications. Dtsch Arztebl Int. 2009;106(41):664–8 (PubMed PMID: 19946431. PMCID: PMC2780010. Epub 2009/12/01. eng).
PubMed PubMed Central Google Scholar
Kahan BC, Rehal S, Cro S. Risk of selection bias in randomised trials. Trials. 2015;16:405 (PubMed PMID: 26357929. PMCID: PMC4566301. Epub 2015/09/12. eng).
Peng YG, Nie XL, Feng JJ, Peng XX. Postrandomization confounding challenges the applicability of randomized clinical trials in comparative effectiveness research. Chin Med J (Engl). 2017;130(8):993–6 (PubMed PMID: 28397731. PMCID: PMC5407048. Epub 2017/04/12. eng).
Hammerton G, Munafò MR. Causal inference with observational data: the need for triangulation of evidence. Psychol Med. 2021;51(4):563–78 (Epub 2021/03/08).
Mansournia MA, Higgins JP, Sterne JA, Hernán MA. Biases in randomized trials: a conversation between trialists and epidemiologists. Epidemiology. 2017;28(1):54–9 (PubMed PMID: 27748683. PMCID: PMC5130591. Epub 2016/10/18. eng).
Nguyen VT, Engleton M, Davison M, Ravaud P, Porcher R, Boutron I. Risk of bias in observational studies using routinely collected data of comparative effectiveness research: a meta-research study. BMC Med. 2021;19(1):279 (PubMed PMID: 34809637. PMCID: PMC8608432. Epub 2021/11/24. eng).
Faraoni D, Schaefer ST. Randomized controlled trials vs. observational studies: why not just live together? BMC Anesthesiol. 2016;16(1):102 (PubMed PMID: 27769172. PMCID: PMC5073487. Epub 2016/10/23. eng).
Ross JS. Randomized clinical trials and observational studies are more often alike than unlike. JAMA Intern Med. 2014;174(10):1557 (PubMed PMID: 25111371. Epub 2014/08/12. eng).
Pallmann P, Bedding AW, Choodari-Oskooei B, Dimairo M, Flight L, Hampson LV, et al. Adaptive designs in clinical trials: why use them, and how to run and report them. BMC Med. 2018;16(1):29 (PubMed PMID: 29490655. PMCID: PMC5830330. Epub 2018/03/02. eng).
Article PubMed Central Google Scholar
Lewis RJ, Bessen HA. Sequential clinical trials in emergency medicine. Ann Emerg Med. 1990;19(9):1047–53 (PubMed PMID: 2393170. Epub 1990/09/01. eng).
Article CAS PubMed Google Scholar
Tooth L. Use of sequential medical trials in rehabilitation research. Am J Phys Med Rehabil. 1999;78(1):87–97 (PubMed PMID: 9923437. Epub 1999/01/29. eng).
Berry SM, Connor JT, Lewis RJ. The platform trial: an efficient strategy for evaluating multiple treatments. Jama. 2015;313(16):1619–20 (PubMed PMID: 25799162. Epub 2015/03/24. eng).
Article Google Scholar
Mc Cord KA, Hemkens LG. Using electronic health records for clinical trials: Where do we stand and where can we go? Cmaj. 2019;191(5):E128-e33 (PubMed PMID: 30718337. PMCID: PMC6351244 Collected Data for Randomized Controlled Trials Initiative (RCD for RCT initiative), which aims to explore the use of routinely collected data for clinical trials. They are members of The Making Randomized Trials Affordable (MARTA) Group. No other competing interests were declared. Epub 2019/02/06. eng).
Hill J, Stuart EA. Causal Inference: Overview. In: Wright JD, editor. International Encyclopedia of the Social & Behavioral Sciences. 2nd ed. Oxford: Elsevier; 2015. p. 255–60.
Chapter Google Scholar
Tennant PWG, Murray EJ, Arnold KF, Berrie L, Fox MP, Gadd SC, et al. Use of directed acyclic graphs (DAGs) to identify confounders in applied health research: review and recommendations. Int J Epidemiol. 2020;50(2):620–32.
Gill J, Prasad V. Improving observational studies in the era of big data. Lancet. 2018;392(10149):716–7 (PubMed PMID: 30191816. Epub 2018/09/08. eng).
Lee CH, Yoon HJ. Medical big data: promise and challenges. Kidney Res Clin Pract. 2017;36(1):3–11 (PubMed PMID: 28392994. PMCID: PMC5331970. Epub 2017/04/11. eng).
VanderWeele TJ, Ding P. Sensitivity analysis in observational research: introducing the E-value. Ann Intern Med. 2017;167(4):268–74 (PubMed PMID: 28693043. Epub 2017/07/12. eng).
Jardine MJ, Kotwal SS, Bassi A, Hockham C, Jones M, Wilcox A, et al. Angiotensin receptor blockers for the treatment of covid-19: pragmatic, adaptive, multicentre, phase 3, randomised controlled trial. BMJ. 2022;379:e072175.
Wang SJ, Peng H, Hung HJ. Evaluation of the extent of adaptation to sample size in clinical trials for cardiovascular and CNS diseases. Contemp Clin Trials. 2018;67:31–6 (PubMed PMID: 29427757. Epub 2018/02/11. eng).
Gu WJ, Zhang Z, Bakker J. Early lactate clearance-guided therapy in patients with sepsis: a meta-analysis with trial sequential analysis of randomized controlled trials. Intensive Care Med. 2015;41(10):1862–3 (PubMed PMID: 26154408. Epub 2015/07/15. eng).
Park JJH, Harari O, Dron L, Lester RT, Thorlund K, Mills EJ. An overview of platform trials with a checklist for clinical readers. Journal of Clinical Epidemiology. 2020 2020/09/01/;125:1–8.
Roustit M, Demarcq O, Laporte S, Barthélémy P, Chassany O, Cucherat M, et al. Platform trials. Therapie. 2023;78(1):29–38 (PubMed PMID: 36529559. PMCID: PMC9756081. Epub 2022/12/19. eng).
Parker CC, James ND, Brawley CD, Clarke NW, Hoyle AP, Ali A, et al. Radiotherapy to the primary tumour for newly diagnosed, metastatic prostate cancer (STAMPEDE): a randomised controlled phase 3 trial. Lancet. 2018;392(10162):2353–66 (PubMed PMID: 30355464. PMCID: PMC6269599. Epub 2018/10/26. eng).
Yee D, Shatsky RA, Yau C, Wolf DM, Nanda R, van ‘t Veer L, et al. Improved pathologic complete response rates for triple-negative breast cancer in the I-SPY2 Trial. J Clin Oncol. 2022;40(16_suppl):591.
Thadani SR, Weng C, Bigger JT, Ennever JF, Wajngurt D. Electronic screening improves efficiency in clinical trial recruitment. J Am Med Inform Assoc. 2009;16(6):869–73 (PubMed PMID: 19717797. PMCID: PMC3002129. Epub 2009/09/01. eng).
Price M, Davies I, Rusk R, Lesperance M, Weber J. Applying STOPP guidelines in primary care through electronic medical record decision support: randomized control trial highlighting the importance of data quality. JMIR Med Inform. 2017;5(2):e15 (PubMed PMID: 28619704. PMCID: PMC5491896. Epub 2017/06/18. eng).
Bereznicki BJ, Peterson GM, Jackson SL, Walters EH, Fitzmaurice KD, Gee PR. Data-mining of medication records to improve asthma management. Med J Aust. 2008;189(1):21–5 (PubMed PMID: 18601636. Epub 2008/07/08. eng).
Eklind-Cervenka M, Benson L, Dahlström U, Edner M, Rosenqvist M, Lund LH. Association of candesartan vs losartan with all-cause mortality in patients with heart failure. Jama. 2011;305(2):175–82 (PubMed PMID: 21224459. Epub 2011/01/13. eng).
Skerritt L, de Pokomandy A, O’Brien N, Sourial N, Burchell AN, Bartlett G, et al. Discussing reproductive goals with healthcare providers among women living with HIV in Canada: the role of provider gender and patient comfort. Sex Reprod Health Matters. 2021;29(1):1932702 PubMed PMID: 34165395. PMCID: PMC8231384. Epub 2021/06/25. eng.
Suttorp MM, Siegerink B, Jager KJ, Zoccali C, Dekker FW. Graphical presentation of confounding in directed acyclic graphs. Nephrol Dial Transplant. 2015;30(9):1418–23.
Pakzad R, Nedjat S, Salehiniya H, Mansournia N, Etminan M, Nazemipour M, et al. Effect of alcohol consumption on breast cancer: probabilistic bias analysis for adjustment of exposure misclassification bias and confounders. BMC Med Res Methodol. 2023;23(1):157.
Byrne AL, Marais BJ, Mitnick CD, Garden FL, Lecca L, Contreras C, et al. Asthma and atopy prevalence are not reduced among former tuberculosis patients compared with controls in Lima, Peru. BMC Pulmonary Med. 2019;19(1):40.
Bender Ignacio RA, Madison AT, Moshiri A, Weiss NS, Mueller BA. A population-based study of perinatal infection risk in women with and without systemic lupus erythematosus and their infants. Paediatr Perinat Epidemiol. 2018;32(1):81–9 (PMCID: PMC5771993. Epub 2017/12/02. eng).
Eastwood B, Peacock A, Millar T, Jones A, Knight J, Horgan P, et al. Effectiveness of inpatient withdrawal and residential rehabilitation interventions for alcohol use disorder: a national observational, cohort study in England. J Subst Abuse Treat. 2018;88:1–8 (PubMed PMID: 29606222. Epub 2018/04/03. eng).
Khera AV, Chaffin M, Aragam KG, Haas ME, Roselli C, Choi SH, et al. Genome-wide polygenic scores for common diseases identify individuals with risk equivalent to monogenic mutations. Nat Genet. 2018;50(9):1219–24 (Epub 2018/08/15. eng).
Ahmed W, Das R, Vidal-Alaball J, Hardey M, Fuster-Casanovas A. Twitter’s role in combating the magnetic vaccine conspiracy theory: social network analysis of tweets. J Med Internet Res. 2023;25:e43497 PMCID: PMC10131940. Epub 2023/03/18. eng.
Sourial N, Longo C, Vedel I, Schuster T. Daring to draw causal claims from non-randomized studies of primary care interventions. Fam Pract. 2018;35(5):639–43 (PubMed PMID: 29912314. PMCID: PMC6142715. Epub 2018/06/19. eng).
Download references
Acknowledgements
Lise Gauvin, Department of Social and Preventive medicine, School of Public Health, University of Montreal, Research Centre of the Centre Hospitalier de l’Université de Montréal (CRCHUM).
Hosting research centres: CRCHUM, Research Centre of the University of Sherbrooke and the University of Sherbrooke Research Center on Aging.
This work was funded by a Canadian Institutes of Health Research grant (#178264).
Author information
Authors and affiliations.
Department of Health Management, Evaluation and Policy, School of Public Health, University of Montreal, Montreal, QC, Canada
Pamela Fernainy & Nadia Sourial
Research Centre of the Centre Hospitalier de L’Université de Montréal (CHUM), Montreal, QC, Canada
Department of Family and Emergency Medicine, Faculty of Medicine and Health Sciences, University of Sherbrooke, Montreal, QC, Canada
Alan A. Cohen
CHUS Research Centre, Montreal, QC, Canada
Alan A. Cohen & Francois Lamontagne
Centre de Recherche Sur Le Vieillissement, Montreal, QC, Canada
Butler Columbia Aging Center, New York, NY, USA
Department of Environmental Health Sciences, Mailman School of Public Health, Columbia University New York, New York, USA
School of Public Health, Boston University, Boston, MA, USA
Eleanor Murray
Harvard Medical School Department of Orthopedic Surgery, Cambridge, MA, USA
Departement de Medicine, University of Sherbrooke, Montreal, QC, Canada
You can also search for this author in PubMed Google Scholar
Contributions
PF contributed to the conception of the paper and drafted the work. AAC contributed to conception and revision of the manuscript. EM contributed to conception and revision of the manuscript. EL contributed to conception and revision of the manuscript. FL contributed to conception and revision of the manuscript. NS was responsible for conception and revision of the manuscript and substantially revised the work. All authors read and approved the submitted manuscript.
Corresponding author
Correspondence to Pamela Fernainy .
Ethics declarations
Ethics approval and consent to participate, consent for publication, competing interests.
The authors declare that they have no competing interests.
Additional information
Publisher’s note.
Springer Nature remains neutral with regard to jurisdictional claims in published maps and institutional affiliations.
Supplementary Information
Additional file 1., rights and permissions.
Open Access This article is licensed under a Creative Commons Attribution 4.0 International License, which permits use, sharing, adaptation, distribution and reproduction in any medium or format, as long as you give appropriate credit to the original author(s) and the source, provide a link to the Creative Commons licence, and indicate if changes were made. The images or other third party material in this article are included in the article's Creative Commons licence, unless indicated otherwise in a credit line to the material. If material is not included in the article's Creative Commons licence and your intended use is not permitted by statutory regulation or exceeds the permitted use, you will need to obtain permission directly from the copyright holder. To view a copy of this licence, visit http://creativecommons.org/licenses/by/4.0/ . The Creative Commons Public Domain Dedication waiver ( http://creativecommons.org/publicdomain/zero/1.0/ ) applies to the data made available in this article, unless otherwise stated in a credit line to the data.
Reprints and permissions
About this article
Cite this article.
Fernainy, P., Cohen, A.A., Murray, E. et al. Rethinking the pros and cons of randomized controlled trials and observational studies in the era of big data and advanced methods: a panel discussion. BMC Proc 18 (Suppl 2), 1 (2024). https://doi.org/10.1186/s12919-023-00285-8
Download citation
Published : 18 January 2024
DOI : https://doi.org/10.1186/s12919-023-00285-8
Share this article
Anyone you share the following link with will be able to read this content:
Sorry, a shareable link is not currently available for this article.
Provided by the Springer Nature SharedIt content-sharing initiative
- Randomized control trial
- Observational study
- Medical evidence
- Research method
- Research methodologies
- Study design
- Quality of evidence
BMC Proceedings
ISSN: 1753-6561
- Submission enquiries: [email protected]
- General enquiries: [email protected]
- - Google Chrome
Intended for healthcare professionals
- My email alerts
- BMA member login
- Username * Password * Forgot your log in details? Need to activate BMA Member Log In Log in via OpenAthens Log in via your institution

Search form
- Advanced search
- Search responses
- Search blogs
- Randomised controlled...
Randomised controlled trials in primary care: case study
- Related content
- Peer review
- Sue Wilson , senior research fellow ( s.wilson{at}bham.ac.uk ) ,
- Brendan C Delaney , senior lecturer ,
- Andrea Roalfe , medical statistician ,
- Lesley Roberts , research associate ,
- Val Redman , project officer ,
- Andy M Wearn , lecturer ,
- F D Richard Hobbs , professor of primary care and general practice
- Department of Primary Care and General Practice, Division of Primary Care, Public and Occupational Health, University of Birmingham, Medical School, Birmingham B15 2TT
- Correspondence to: S Wilson
- Accepted 25 April 2000
Editorial by Thomas
Although over 90% of patient contacts within the NHS occur in primary care, many of the interventions used in this setting remain unproved. 1 The relevance of research undertaken in secondary or tertiary care to general practice is questionable, and more research based in primary care is needed. 2 Increasing research in primary care will inevitably increase demand for randomised controlled trials in this setting. Some of the trials will be of health service interventions (pragmatic trials), 3 where the focus lies in assessing the cost effectiveness of an intervention rather than efficacy or safety. The difficulties experienced in doing randomised controlled trials in primary care have been reported 4 – 6 and are not restricted to this setting. 7 8 We discuss some of the issues that must be considered when conducting and interpreting the results of trials in primary care using examples generated during a trial of the management of dyspepsia (box).
Birmingham open access endoscopy study
The study aimed to evaluate the effectiveness of two management strategies for patients presenting in primary care with symptoms of dyspepsia. Two randomised controlled trials were conducted concurrently, with eligibility being determined by the patient's age at presentation. Randomisation was done at the individual patient level by using sealed opaque, sequentially numbered envelopes during a primary care consultation for dyspepsia.
Initial endoscopy trial
Eligible patients— 50 years of age or older.
Intervention— Referred for open access endoscopy.
Test and endoscopy trial
Eligible patients— Under 50 years.
Intervention —Tested for Helicobacter pylori antibodies with Helisal near patient test. Patients with positive results referred for open access endoscopy; those with negative results received symptomatic treatment only.
Control arms (both trials)— Managed according to “usual practice” excluding open access endoscopy. This included antacids, H 2 receptor antagonists, proton pump inhibitors, outpatient gastroenterology referral, facilitated or direct access endoscopy (for example, vetted by consultant), and testing for H pylori.
Outcomes— Primary outcomes were change in symptom score and cost effectiveness. Secondary outcomes included quality of life and acceptability.
Data collection— At recruitment, general practitioners completed a case report form providing patient identifiers and a limited amount of baseline data. Patients completed the dyspepsia symptom questionnaire and the quality of life questionnaire at recruitment and at six and 18 months after randomisation. A patient satisfaction questionnaire was also completed at 18 months. Data on use of health services were collected from general practice records and endoscopy units at 12 months after recruitment.
Summary points
All trials require a compromise between including sufficient practitioners to recruit a representative cohort of patients and the time and cost of recruiting and maintaining the motivation of these practitioners
Prior beliefs relating to the efficacy and direct or side effects of an intervention affect both doctor and patient participation
Trials in any setting are rarely fully representative with respect to both patient and disease related characteristics
Modelling, sensitivity analysis, and statistical estimates of uncertainty are necessary to determine the generalisability of trials and to particularise results to a given clinical setting
Trials in primary care should give more representative results and are preferable to applying results obtained in secondary care
Dyspepsia is a common clinical problem. About 2% of the population consult their general practitioner each year with dyspeptic symptoms, 9 and it costs the NHS more than £1bn a year, with a large proportion of these costs relating to drug prescription. 10 The evidence base has largely consisted of cohort studies of patients referred to secondary care for investigation 11 12 and economic models. 13 In the absence of evidence from primary care, several conflicting consensus guidelines have been generated. 14 15 Dyspepsia represents a good example of a chronic disease that is largely managed in primary care and that requires high quality evidence from randomised controlled trials in primary care.
Why do research in…
The natural course of any disease can be described as progression from the first occurrence of disease to the first episode of symptoms, which may lead to a primary care consultation and subsequent treatment. For some conditions patients will be referred to secondary care. The population available to the researcher at each of these stages differs in terms of severity of symptoms, stage of disease, patient attitudes, and response to treatment. Research undertaken in secondary care is subject to biases of case selection and referral and may underestimate the prevalence of disease and overestimate the impact on quality of life compared with observations in primary care. Interventions shown to be effective in secondary care may therefore have limited value in the community.
Recruitment of practices into Birmingham endoscopy study
- View inline
Important differences also occur in the outcomes of similar interventions in different healthcare settings. For example, most patients seen in primary care have earlier or milder disease than those referred to hospital. Therefore, the positive predictive value of diagnostic tests in primary care is lower than in secondary care, and invasive investigations may be less justified and less acceptable to patients. Management decisions taken by primary and secondary care doctors may also differ systematically, reflecting different experience and priorities. 16
Unit of randomisation
The conduct of therapeutic trials in which selected groups of patients are randomised to two or more treatments is well established, and the unit of randomisation is invariably the patient. However, randomisation by patient may be inappropriate when evaluating some health services. For example, we may wish to evaluate the effect of issuing a new set of guidelines and therefore wish to randomise general practitioners into those who did or did not receive the guidelines. As practitioners may discuss guidelines within the practice and patients do not necessarily have continuity of care with individual practitioners, randomisation by practice may be necessary. Similarly, within the community neighbours talk to each other, and if a practitioner becomes known to have a particular interest in a condition then patients in a group practice may select to see him or her. This “contamination” of the study group may also necessitate randomisation by practice. Cluster randomisation brings statistical complexities and requires a larger sample size. 17
Recruitment bias
Participating practices.
All trials have to make a compromise between including sufficient practitioners to recruit a representative cohort of patients and the time and cost involved in recruiting and maintaining the motivation of these practitioners. These problems are more acute within primary care where, even for common conditions, the number of patients that practitioners see with the disease of interest represents only a small proportion of their total consultations.
Inevitably, not all practices within the defined catchment area will agree to participate in a trial. The self selected group of practitioners who agree to recruit patients can affect the representativeness of the study population. Not all groups of patients may be adequately represented. Practitioners with a particular interest in the condition under investigation may be more likely to participate and may manage their patients more effectively than the average practitioner, decreasing the effect size observed. 18 19
Only a quarter of general practices contacted for our dyspepsia trial actively participated (that is, recruiting ≥5 patients) (table 1 ). Participating practices had more partners and were located in less deprived areas (table 2 ).
Characteristics of participating and non-participating practices
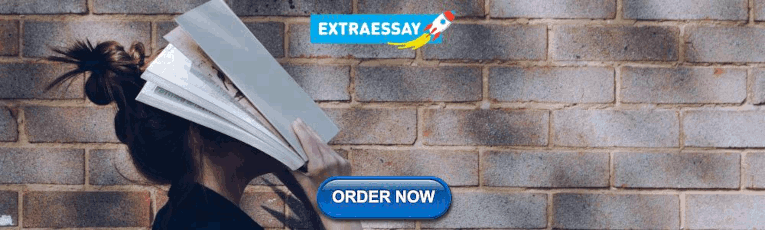
Participating patients
Trials may fail to recruit a representative sample of patients, either because all eligible patients are not prepared to enter the study or because all eligible patients are not asked to enter the study. The Birmingham endoscopy trial had practice recruitment rates ranging from 0.1 to 15.6/10 000 population per month (see fig 1 ).
The variability in recruitment rates may be due to differences in the prevalence of disease or presentation rates but is inevitably also due to differences in the proportion of eligible patients who were recruited. Interpretation of these differences requires access to the records of all patients within participating practices who have the relevant disease. However, it is rarely possible to obtain consent to access records from all patients not entering trials. The study denominator and representativeness of the sample can be determined by comparing the patient and disease related characteristics of participants with those of the total eligible population using anonymised data. However, not all practices routinely record all consultations on computer and manual searches of paper records are costly.
Factors affecting recruitment rates
Incident or prevalent cases.
Figure 1 shows that the recruitment rate fell sharply over the first year to reach a relatively steady state in the second year. The fall in recruitment observed during the first year could be attributable to waning enthusiasm for the trial. However, an alternative explanation is the recruitment of existing (prevalent) cases. Once the pool of prevalent patients has been recruited eligible cases are restricted to those with incident disease. The inevitable mixture of incident and prevalent cases emphasises a further difficulty in analysing and interpreting data on chronic diseases irrespective of whether trials are conducted in primary or secondary care.
Relation between recruitment rate and length of time in study. Recruitment rate is calculated as a 3 month moving average for each period after entry into the study. To adjust for differing practice populations, monthly recruitment rates have been directly standardised by practice list size.
- Download figure
- Open in new tab
- Download powerpoint
The primary care consultation rate for dyspepsia in the United Kingdom is 20/1000 population. 9 Assuming the recruitment rate after 12 months of the study can be taken as a proxy for incidence, the observed incidence rate in the Birmingham dyspepsia trial was 1.67/1000 practice population. Even after practices with poor recruitment rates were excluded (<5 patients) the observed recruitment rate was only 1.97/1000. Thus, less than 10% of the eligible population seems to be have been recruited to the trial.
Although it is technically more appealing to restrict a trial to incident cases, definition of new disease is often difficult, and the findings will rarely be of relevance to general practitioners, whose caseloads comprise people with both new and existing disease.
Ethical issues
Patients may participate in trials to “please” their practitioner or because they are “afraid” to refuse. The need for reassurance that future management will not be compromised by non-participation may be greater in primary care, where the patient may see the same practitioner over many years. The appropriateness of general practitioners recruiting their own patients is further complicated if they receive financial rewards for recruiting cases, even if payment only covers costs.
Ethical trials require both participating clinicians and patients to be in equipoise. 20 Although robust evidence for the cost effectiveness of a particular intervention may not yet exist, if practitioners have a prior belief that one form of management is beneficial they may choose not to randomise patients to treatment.
When attempting to recruit practices we found that many general practitioners were enthusiastic about particular management strategies and were unwilling to randomise patients to different management options. Beliefs relating to the efficacy, direct effects, or side effects of an intervention affect both doctor and patient participation. Patients who are not prepared to accept randomisation are not eligible to be entered into a trial. However, the exclusion of patients who refuse endoscopy because they think it is an uncomfortable or painful procedure could affect estimates of the impact of the trial results. An intervention may be cost effective but unacceptable to most patients. Trials of management strategies should aim to determine the proportion of patients who refuse randomisation because of treatment preferences. Complex interventions may require the use of additional research tools to assess any barriers to accepting the intervention. 21
Selective recruitment of patients
Lack of concealment of allocation can increase the effect size observed in randomised controlled trials. 22 The most secure method requires the clinician to contact an external randomisation service after obtaining informed consent. When recruitment occurs within the routine consultation, any complexities in the randomisation process may deter general practitioners from recruiting patients. Assessing eligibility, explaining the trial, addressing patients' questions and obtaining consent, randomising, and collecting baseline data are time consuming for participating practitioners. 23
The additional workload led to some practices suspending recruitment at busy times (Monday mornings, holidays, flu season, etc). Furthermore, although a practice agreed to participate in the trial this did not necessarily mean that all partners were equally committed to recruiting patients. The effect of such variation in recruitment is not easy to quantify. Recruitment rates may be more predictable for trials conducted in the community, when independent research staff perform all the research activity.
Incomplete ascertainment of eligible patients may also be due to the selective recruitment of patients with particular sets of symptoms. Figure 2 shows that the practices with lower rates of recruitment tended to recruit patients with more severe symptoms. The reasons for this apparent selection bias are unknown, although possible explanations include practices that had suspended recruitment being reminded about the study when a patient with severe disease presents or practitioners applying differing definitions of disease.
Trials in primary care are no different from those in secondary or tertiary care in terms of their lack of success in recruiting all patients with the disease of interest. 24 Similarly, trials in any setting are rarely fully representative with respect to both patient and disease related characteristics. Such limitations do not invalidate the use of the randomised controlled trial. They merely require additional work to be undertaken to establish the effect of the intervention on the total population.
Trials in primary care should recruit participants that are more representative of patients seen in the community than are participants in trials in secondary care. However, if the processes that operate to determine whether a patient is included in a trial are not random, trial participants may be skewed with respect to disease severity or other factors such as age or social class. Although this will not bias the trial result (internal validity), it may misrepresent the effect of the intervention in non-trial settings (external validity). Modelling, sensitivity analysis, and statistical estimates of uncertainty are necessary to determine the generalisability of the trial and to particularise results to a given clinical setting. 25
Primary care provides many opportunities but is not an easy place to conduct research. Trials must be designed and undertaken by multidisciplinary teams with expertise in both the context of clinical practice and research methods.
Acknowledgments
We acknowledge the support of the Dyspepsia Trials Collaborators Group, particularly Robbie Foy, Anne Duggan, and Jayne Parry. This manuscript was stimulated by our participation in the Birmingham open access endoscopy study. The success of this project has been dependent on the enthusiasm and cooperation of the general practices that participated.
Contributors: BCD and SW were responsible for the idea and the initial drafts of this paper. BCD was lead investigator of the Birmingham open access endoscopy study, which was designed by BCD, FDRH, and SW. LR and VR were responsible for practice recruitment, data collection, and validation. AR undertook the analyses. All authors were members of the trial management group and took part in redrafting the paper. SW and BCD are joint guarantors.
Funding The Birmingham open access endoscopy study received financial support from the NHS Research and Development Primary/Secondary Care Interface Programme, West Midlands NHS Research and Development, and the Astra Foundation. BCD holds an NHS Research and Development primary care career scientist award and LR holds a New Blood Fellowship awarded by the NHS Executive (West Midlands).
Competing interests None declared.
- Medical Research Council
- Department of Health
- Fairhurst K ,
- Tognoni G ,
- Avanzini F ,
- Bettelli G ,
- Colombo F ,
- MacIntyre I
- McCormick A ,
- Fleming D ,
- Mendall M ,
- Northfield T
- Sobala GM ,
- Crabtree JE ,
- Pentith JA ,
- Rathbone BJ ,
- Shallcross TM ,
- Mendall MA ,
- Jazrawi RP ,
- Marrero JM ,
- Molineaux N ,
- Maxwell JD ,
- Briggs AH ,
- Sculpher RPH ,
- Logan RPH ,
- Ramsay ME ,
- European Helicobacter Pylori Study Group
- Fendrick AM ,
- Kinmonth AL ,
- Woodcock A ,
- Griffin S ,
- Spiegal N ,
- Campbell MJ
- UK Prospective Diabetes Study (UKPDS) Group
- Lilford RJ ,
- Bradley F ,
- Schulz KF ,
- Chalmers I ,
- Delaney B ,
- Coulter A ,
- Lilford R ,
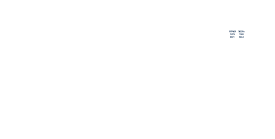
Why randomized controlled trials matter and the procedures that strengthen them
Randomized controlled trials are a key tool to study cause and effect. why do they matter and how do they work.
At Our World in Data, we bring attention to the world's largest problems. We explore what these problems are, why they matter and how large they are. Whenever possible, we try to explain why these problems exist, and how we might solve them.
To make progress, we need to be able to identify real solutions and evaluate them carefully. But doing this well is not simple. It's difficult for scientists to collect good evidence on the costs and benefits of a new idea, policy, or treatment. And it's challenging for decision-makers to scrutinize the evidence that exists, to make better decisions.
What we need are reliable ways to distinguish between ideas that work and those that don't.
In this post, I will explain a crucial tool that helps us do this – randomized controlled trials (RCTs). We will see that RCTs matter for three reasons: when we don't know about the effects of interventions, when we don't know how to study them, and when scientific research is affected by biases.
What are randomized controlled trials?
To begin with, what are RCTs? These are experiments where people are given, at random, either an intervention (such as a drug) or a control and then followed up to see how they fare on various outcomes.
RCTs are conducted by researchers around the world. In the map, you can see how many RCTs have ever been published in high-ranked medical journals, by the country where the first author was based. Over 18,000 of these were from the United States, but most countries have had fewer than a hundred. 1 RCTs have also become more common over time. 2
It's easy to take RCTs for granted, but these trials have transformed our understanding of cause and effect. They are a powerful tool to illuminate what is unknown or uncertain; to discern whether something works and how well it works.
But it's also important to recognize that these trials are not always perfect, and understand why.
The strengths of RCTs are subtle: they are powerful because of the set of procedures that they are expected to follow. This includes the use of controls, placebos, experimentation, randomization, concealment, blinding, intention-to-treat analysis, and pre-registration.
In this post, we will explore why these procedures matter – how each one adds a layer of protection against complications that scientists face when they do research.
The fundamental problem of causal inference
We make decisions based on our understanding of how things work – we try to predict the consequences of our actions.
But understanding cause and effect is not just crucial for personal decisions: our knowledge of what works can have large consequences for people around us.
An example is antiretroviral therapy (ART), which is used to treat HIV/AIDS . The benefits of these drugs were surprising. One of the first ART drugs discovered was azidothymidine, which had previously been abandoned as an ineffective treatment for cancer. 3 The discovery that azidothymidine and other antiretroviral therapies worked, and their use worldwide, has prevented millions of deaths from HIV/AIDS, as the chart shows.
Discovering what works can save lives. But discovering what doesn’t work can do the same. It means we can redirect our time and resources away from things that don't work, towards things that do.
Even when we already know that something works, understanding how well it works can help us make better decisions.
An example for this is the BCG vaccine , which reduces the risk of tuberculosis. The efficacy of this vaccine is different for different people around the world, and the reasons for this are unclear. 4 Still, knowing this is informative, because it tells us that more effort is needed to protect people against the disease in places where the benefit of the vaccine is low.
If there was a reliable way to know about the effects of the measures we took, we could prioritize solutions that are most effective.
So, how would we understand cause and effect without trials?
One way is through observation: we can observe what different people did and track their outcomes afterwards. But it's possible that the events that followed their actions were simply a coincidence, or that they would have happened anyway.
The biggest challenge in trying to understand causes and effects is that we are only able to see one version of history.
When someone makes a particular decision, we are able to see what follows, but we cannot see what would have happened if they had made a different decision. This is known as “the fundamental problem of causal inference." 5
What this means is that it is impossible to predict the effects that an action will have for an individual person, but we can try to predict the effects it would have on average.
Why randomized controlled trials matter
Sometimes we do not need an RCT to identify causes and effects. The effect of smoking on lung cancer is one example, where scientists could be confident as early as the 1960s that the large increase in lung cancer rates could not have been caused by other factors.
This was because there was already knowledge from many different lines of evidence. 6 Experiments, biopsies and population studies all showed that cigarette smoke was associated with specific changes in lung tissue and with the incidence of lung cancer. The association was so large and consistent that it could not be explained by other factors. 7
Even now, smoking is estimated to cause a large proportion of cancer deaths in many countries, as you can see in the chart.
But in other situations, RCTs have made a huge difference to our understanding of the world. Prioritizing the drugs that were shown to be effective in trials saved lives, as we saw with the example of antiretroviral therapy to treat HIV/AIDS.
People sometimes refer to RCTs as the "gold standard of evidence", but it's more useful to recognize that their strengths emerge from a set of procedures that they aim to follow.
Why do RCTs matter?
In my opinion, even though we do have lots of knowledge about various topics, these trials matter for three reasons. They matter because we may not know enough, because we can be wrong, and because we might see what we want to see.
First, they matter when we don't know .
Randomized controlled trials can illuminate our understanding of effects, especially when there is uncertainty around them. They can help resolve disagreements between experts.
In some cases, scientists can use other methods to investigate these topics. But when there is insufficient knowledge, other methods may not be enough.
This is because, in a typical study, scientists need to be able to account for the possibility that other factors are causing the outcome.
They need to have knowledge about why an effect usually occurs and whether it will happen anyway. They need to know about which other risk factors can cause the outcome and what makes it likely that someone will receive the treatment. They also need to know how to measure these factors properly, and how to account for them.
The second reason they matter is when we are wrong .
Even when scientists think they know which risk factors affect the outcome, they might be incorrect. They might not account for the right risk factors or they might account for the wrong ones.
In an RCT where scientists use randomization, blinding and concealment, they can minimize both of these problems. Despite people's risks before the trial, the reason that someone will receive the treatment is the random number they are given. The reason that participants in each group differ at the end of the study is the group they were randomized to.
Even if we don't know about other risk factors, or even if we are wrong about them, these procedures mean that we can still find out whether a treatment has an effect and how large the effect is.
The third reason is when we see what we want to see .
When participants expect to feel different after a treatment, they might report that they feel different, even if they didn't receive the treatment at all.
When scientists want to see people improve after a treatment, they might decide to allocate the treatment to healthier participants. They might measure their data differently or re-analyze their data until they can find an improvement. They might even decide against publishing their findings when they don't find a benefit.
If scientists use concealment, blinding, and pre-registration, they can reduce these biases. They can protect research against their own prejudices and the expectations of participants. They can also defend scientific knowledge from poor incentives, such as the desires of scientists to hype up their findings and the incentives for pharmaceutical companies to claim that their drugs work.
The layers of protection against bias
Previously, I described how the strengths of RCTs emerge from the set of procedures that they aim to follow.
In medicine, this commonly includes the use of controls, placebos, experimentation, randomization, concealment, blinding, intention-to-treat analysis, and pre-registration. 8 Each of these helps to protect the evidence against distortions, which can come from many sources. But they are not always enforced. Below, we will explore them in more detail.
The control group gives us a comparison to see what would have happened otherwise
The most crucial element to understand causes and effects is a control group.
Let's use antidepressants as an example to see why we need them.
If no one in the world received antidepressants, we wouldn’t know what their effects were. But the opposite is also true. If everyone received antidepressants, we wouldn't know how things would be without them.
In order to understand the effect of an antidepressant, we need to see which other outcomes are possible. The most important thing we need to understand effects is a comparison – we need some people who receive the treatment and some who don’t. The people who don’t could be our control group.
An ideal control group should allow us to control the biases and measurement errors in a study completely.
For example, different doctors may diagnose depression differently. If someone had symptoms of depression, then the chances they get diagnosed should be equal in the antidepressant group and the control group. If they weren't equal, we could mistake differences between the groups for an effect of antidepressants, even if they didn't have any effect.
In fact, an ideal control group should allow us to control the total effects of all of the other factors that could affect people's mood, not just control for the biases and errors in studies.
As an example, we know that the symptoms of depression tend to change over time, as I explained in this earlier post .
You can see this in the chart. This shows that the symptoms of depression tend to decline over time, among people who are diagnosed with depression but not treated for it. This is measured by seeing how many patients would still meet the threshold for a diagnosis of depression later on.
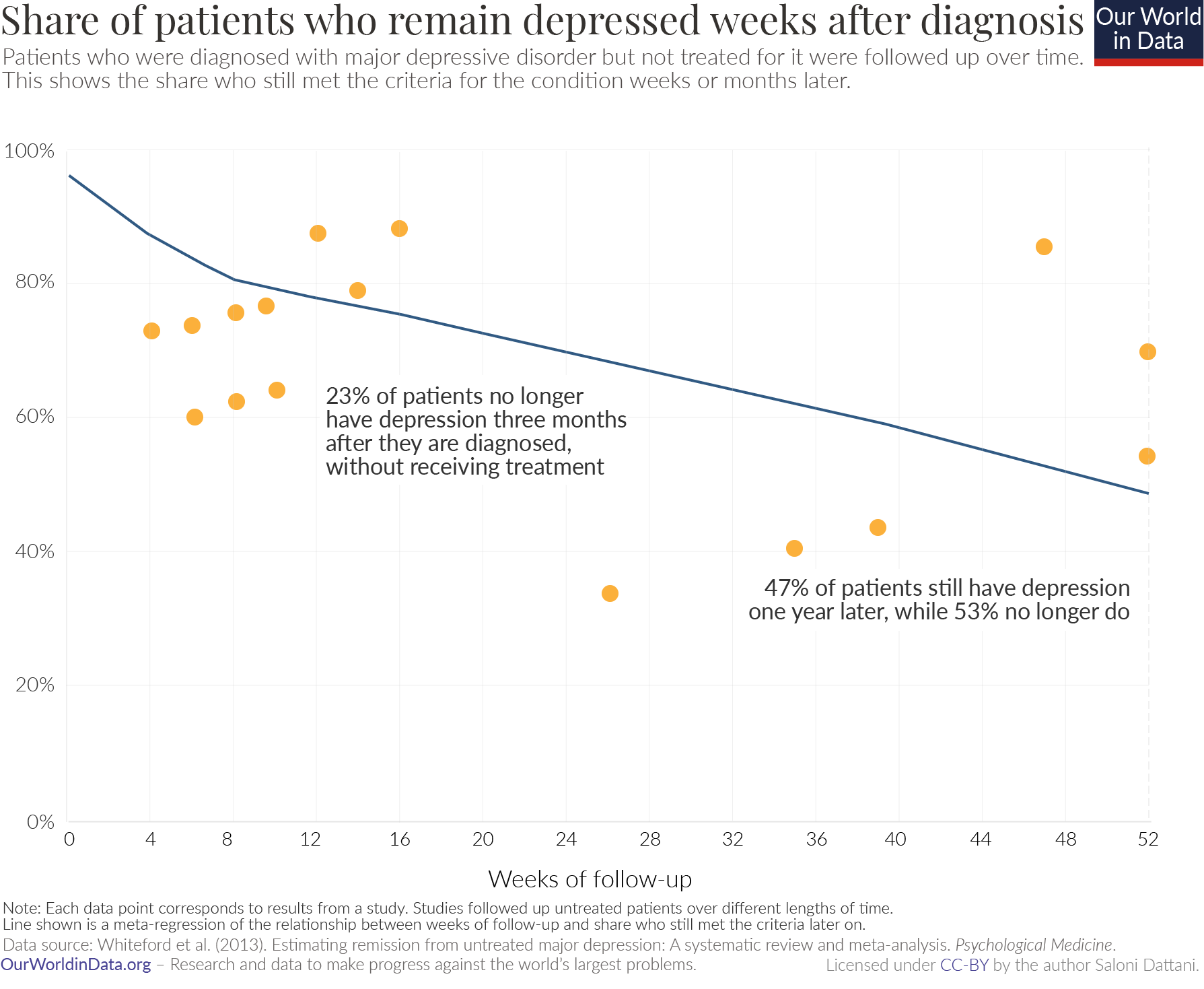
Almost a quarter of patients with depression (23%) would no longer meet the threshold for depression after three months, despite receiving no treatment. Just over half (53%) would no longer meet the threshold after one year. 9 This change is known as "regression to the mean."
If we didn’t have a control group in our study, we might misattribute such an improvement to the antidepressant. We need a control group to know how much their symptoms would improve anyway.
Placebos allow us to account for placebo effects
Some types of controls are special because they resemble the treatment without actually being it – these controls are called placebos. An ideal placebo has all the qualities above and also allows us to account for "placebo effects." In this case, the placebo effect refers to when people's moods improve from the mere procedure of receiving the antidepressant.
For example, taking a pill might improve people's mood because they believe that taking a pill will give them some benefit, even if the pill does not actually contain any active ingredient.
How large is the placebo effect?
Some clinical trials have tried to estimate this by comparing patients who received a placebo to patients who received no treatment at all. Overall, these studies have found that placebo effects are small in clinical trials for many conditions, but are larger for physical treatments, such as acupuncture to treat pain. 10
Placebo effects tend to be larger when scientists are recording symptoms reported by patients, rather than when they are measuring something that can be verified by others, such as results from a blood test or death.
For depression, the placebo effect is non-significant. Studies do not detect a difference in the moods of patients who receive a placebo and those who do not receive any treatment at all. 11 Instead, the placebo group serves as a typical control group, to show what would happen to these patients even if they did not receive treatment.
Randomization ensures that there are no differences between control and treatment group apart from whether they received the treatment
Before participants are enrolled in a trial, they might already have different levels of risk of developing the outcomes.
Let's look at an example to illustrate this point.
Statins are a type of drug commonly used to prevent stroke. But strokes are more common among people who use statins. Does that mean that statins caused an increase in the rates of stroke?
No – people who are prescribed statins are more likely to have cardiovascular problems to begin with, which increases the chances of having a stroke later on. When this is accounted for, researchers find that people who take statins are actually less likely to develop a stroke. 12
If researchers simply compared the rates of stroke in those who used statins with those who did not, they would miss the fact that there were other differences between the two groups, which could have caused differences in their rates of stroke.
Important differences such as these – which affect people's likelihood of receiving the treatment (using statins) and also affect the outcome (the risk of a stroke) – are called ‘confounders’.
But it can be difficult to know what all of these confounders might be. It can also be difficult to measure and account for them properly. In fact, scientists can actually worsen a study by accounting for the wrong factors. 13
What happens when participants are randomized in a trial?
Randomization is the procedure of allocating them into one of two groups at random.
With randomization, the problems above are minimized: everyone has the possibility of receiving the treatment. Whether people receive the treatment is not determined by the risks they have, but whether they are randomly selected to receive the treatment.
So, the overall risks of developing the outcome in one group become comparable to the risks in the other group.
Randomization means that it is not a problem when there are confounders that are not known or not measured. Researchers don't have to know about why or how the outcome usually occurs. 14
Concealment and blinding limit the biases of researchers and expectations of participants
In a clinical trial, participants or scientists might realize which groups they are assigned to. For example, the drug might smell, taste or look different from the placebo. Or it might have obvious benefits or different side effects compared to the placebo. 15
Concealment is a first step in preventing this: this is the procedure of preventing scientists from knowing which treatment people will be assigned to.
Blinding is a second step: this is the procedure of preventing participants and scientists from finding out which treatment group people have been assigned to. 16
When blinding is incomplete, it can partly reverse the benefits of randomization. For example, in clinical trials for oral health, the benefits of treatments appear larger when patients and the scientists who assess their health are not blinded sufficiently. 17
If randomization was maintained, the only reason that groups would differ on their outcomes was the treatment they received. However, if the treatments were not hidden from scientists and participants, other factors could cause differences between them.
Sometimes, blinding is not possible – there might not be something that is safe and closely resembles the treatment, which could be used as a placebo.
Fortunately, this does not necessarily mean that these trials cannot be useful. Researchers can measure verifiable outcomes (such as changes in blood levels or even deaths) to avoid some placebo effects.
But even when blinding occurs, participants and researchers might still make different decisions, because of the effects of the treatment or placebo.
For example, some participants might decide to withdraw from the trial or not follow the protocols of the trial closely. Similarly, scientists might guess which groups people are in and therefore treat them differently or measure their outcomes in a biased way.
It's difficult to predict how this might affect the results of a trial. It could cause us to overestimate or underestimate the benefit of a treatment. For example, in the clinical trials for Covid-19 vaccines , some participants may have guessed that they received the vaccine because they experienced side effects.
So, they may have believed that they were more protected and took fewer precautions. This means they may have increased their risk of catching Covid-19. This would make it appear as if the vaccines gave less protection than they actually did: it would result in an underestimate of their efficacy.
Preregistration allows us to hold researchers accountable to their original study plans
Some of the procedures we've explored so far are used to safeguard research against errors and biases that scientists can have. Pre-registration is another procedure that contributes to the same goal.
After the data in a study is analyzed, scientists have some choice in which results they present to their colleagues and the wider community. This opens up research to the possibility of cherry-picking.
This problem unfortunately often arises in studies that are sponsored by industry. If a pharmaceutical company is testing their new drugs in trials, disappointing results can lead to financial losses. So, they may decide not to publish them.
But this problem is not limited to trials conducted by pharmaceutical companies.
For many reasons, scientists may decide not to publish some of their studies. Or they might re-analyze their data in a different way to find more positive results. Even if scientists want to publish their findings, journals may decide not to publish them because they may be seen as disappointing, controversial or uninteresting.
To counter these incentives, scientists can follow the practice of "pre-registration." This is when they publicly declare which analyses they plan to do in advance of collecting the data.
In 2000, the United States Food and Drug Administration (FDA) established an online registry for the details of clinical trials. The FDA required that scientists who were studying therapies for serious diseases needed to provide some details of their study designs and the outcomes that they planned to investigate. 18
This requirement reduced the bias towards positive findings in published research. We see evidence of this in the chart, showing clinical trials funded by the National Heart, Lung and Blood Institute (NHLBI), which adopted this requirement.
Before 2000 – when pre-registration of studies was not required – most candidate drugs to treat or prevent cardiovascular disease showed large benefits. But most trials published after 2000 showed no benefit. 19
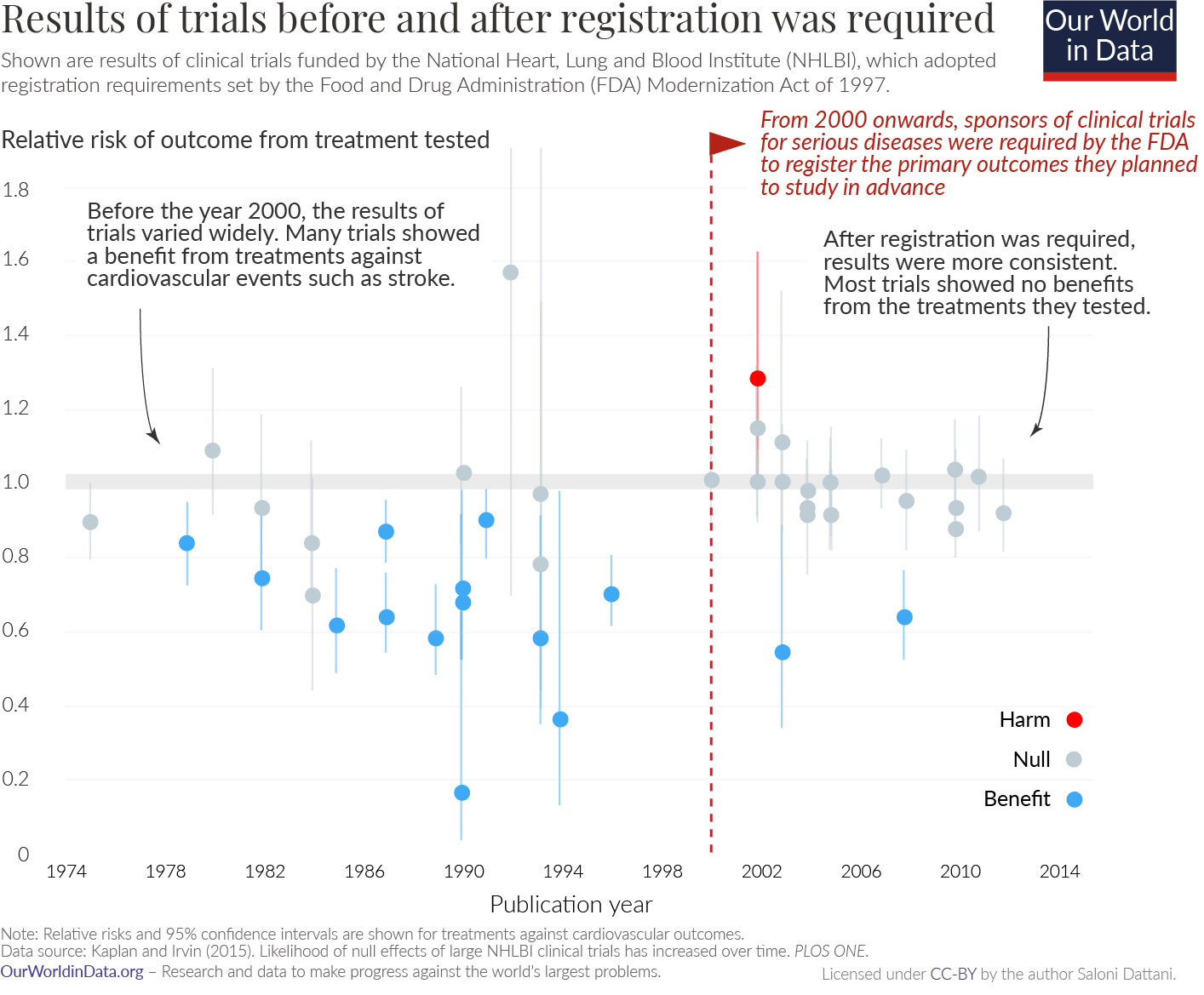
Over the last two decades, this practice was strengthened and expanded. In 2007, the FDA required that most approved trials must be registered when people are enrolled into the study. They introduced notices and civil penalties for not doing so. Now, similar requirements are in place in Europe, Canada and some other parts of the world. 20
Importantly, sponsors of clinical trials are required to share the results online after the trial is completed.
But unfortunately many still violate these requirements. For example, less than half (40%) of trials that were conducted in the US since 2017 actually reported their results on time. 21
Here, you can see patterns in reporting results in Europe. According to EU regulations, all clinical trials in the European Economic Area are required to report their results to the European Clinical Trials Register (EU-CTR) within a year of completing the trial. But reporting rates vary a lot by country. By January 2021, most clinical trials by non-commercial sponsors in the UK (94%) reported their results in time, while nearly none (4%) in France had. 22
Although a large share of clinical trials fail to report their results when they are due, this has improved recently: only half (50%) of clinical trials by non-commercial sponsors reported their results in time in 2018, while more than two-thirds (69%) did in 2021. 23
Pulling the layers together
Together, these procedures give us a far more reliable way to distinguish between what works and what doesn't – even when we don't know enough, when we're wrong and when we see what we want to see.
Unfortunately, we have seen that many clinical trials do not follow them. They tend not to report the details of their methods or test whether their participants were blinded to what they received. These standards cannot just be expected from scientists – they require cooperation from funders and journals, and they need to be actively enforced with penalties and incentives.
We've also seen that trials can still suffer from remaining problems: that participants may drop out of the study and not adhere to the treatment. And clinical trials tend to not share their data or the code that they used to analyze it.
So, despite all the benefits they provide, we shouldn't see these layers as a fixed checklist. Just as some of them were introduced recently, they may still evolve in the future – open data sharing, registered reports and procedures to reduce dropouts may be next on the list. 24
The procedures used in these trials are not a silver bullet. But when they are upheld, they can make these trials a more powerful source of evidence for understanding cause and effect.
To make progress, we need to be able to understand the problems that affect us, their causes and solutions. Randomized controlled trials can give scientists a reliable way to collect evidence on these important questions, and give us the ability to make better decisions. At Our World in Data, this is why they matter most of all.
Acknowledgements
I would like to thank Darren Dahly, Nathaniel Bechhofer, Hannah Ritchie and Max Roser for reading drafts of this post and their very helpful guidance and suggestions to improve it.
Additional information
Trials are useful to test new ideas or treatments, when people do not have enough knowledge to recommend them on a larger scale.
But people also have ethical concerns surrounding trials of treatments that are already used by doctors. For one, they might believe that it isn't justified to give patients a placebo when a drug is available, even if it is unclear how well the drug works.
How can we balance these concerns with the benefits of trials? When are trials important?
A leading view is that they are justified when there is disagreement or uncertainty about the benefits of an intervention. 25
Outside of a trial, some doctors might prescribe a particular treatment, while other doctors would not, because they believe that same treatment is ineffective. In this case, the options in a trial – an intervention or control – would not be so different from what people could already encounter.
When experts disagree on a topic, an RCT is useful because it can resolve these conflicts and inform us about which option is better.
So, to make the most of trials, they should be planned in line with the questions that people have. For example, if we wanted to understand whether vaccines gave less protection to people who were immunocompromised, then we should plan a trial with enough participants with these conditions.
Catalá-López, F., Aleixandre-Benavent, R., Caulley, L., Hutton, B., Tabarés-Seisdedos, R., Moher, D., & Alonso-Arroyo, A. (2020). Global mapping of randomised trials related articles published in high-impact-factor medical journals: a cross-sectional analysis. Trials , 21 (1), 1-24.
Vinkers, C. H., Lamberink, H. J., Tijdink, J. K., Heus, P., Bouter, L., Glasziou, P., Moher, D., Damen, J. A., Hooft, L., & Otte, W. M. (2021). The methodological quality of 176,620 randomized controlled trials published between 1966 and 2018 reveals a positive trend but also an urgent need for improvement. PLOS Biology , 19 (4), e3001162. https://doi.org/10.1371/journal.pbio.3001162
National Research Council (U.S.) (1993). The social impact of AIDS in the United States. 4. Clinical Research and Drug Regulation. National Academy Press.
Dockrell, H. M., & Smith, S. G. (2017). What Have We Learnt about BCG Vaccination in the Last 20 Years? Frontiers in Immunology , 8 , 1134. https://doi.org/10.3389/fimmu.2017.01134
Mangtani, P., Abubakar, I., Ariti, C., Beynon, R., Pimpin, L., Fine, P. E. M., Rodrigues, L. C., Smith, P. G., Lipman, M., Whiting, P. F., & Sterne, J. A. (2014). Protection by BCG Vaccine Against Tuberculosis: A Systematic Review of Randomized Controlled Trials. Clinical Infectious Diseases , 58 (4), 470–480. https://doi.org/10.1093/cid/cit790
Holland, P. W. (1986). Statistics and Causal Inference. Journal of the American Statistical Association , 81 (396), 945–960. https://doi.org/10.1080/01621459.1986.10478354
Imbens, G. W., & Rubin, D. B. (2010). Rubin causal model. In Microeconometrics (pp. 229–241). Springer.
Rubin, D. B. (1977). Assignment to Treatment Group on the Basis of a Covariate. Journal of Educational Statistics , 2 (1), 1–26. https://doi.org/10.3102/10769986002001001
Hill, G., Millar, W., & Connelly, J. (2003). “The Great Debate”: Smoking, Lung Cancer, and Cancer Epidemiology. Canadian Bulletin of Medical History , 20 (2), 367-386.
Cornfield, J., Haenszel, W., Hammond, E. C., Lilienfeld, A. M., Shimkin, M. B., & Wynder, E. L. (1959). Smoking and Lung Cancer: Recent Evidence and a Discussion of Some Questions. JNCI: Journal of the National Cancer Institute . https://doi.org/10.1093/jnci/22.1.173
Although these procedures are also used outside of medicine, it can be difficult to apply them elsewhere. For example, in a trial that tests the effectiveness of talking therapy, it would be known to the participants that they are receiving it; it may not be possible to find a placebo control version to disguise the procedure. Due to constraints in length and focus, I will not detail the advantages of intention-to-treat analysis or experimentation.
Whiteford, H. A., Harris, M. G., McKeon, G., Baxter, A., Pennell, C., Barendregt, J. J., & Wang, J. (2013). Estimating remission from untreated major depression: A systematic review and meta-analysis. Psychological Medicine , 43 (8), 1569–1585. https://doi.org/10.1017/S0033291712001717
Hróbjartsson, A., & Gøtzsche, P. C. (2010). Placebo interventions for all clinical conditions. Cochrane Database of Systematic Reviews . https://doi.org/10.1002/14651858.CD003974.pub3
Hróbjartsson, A., & Gøtzsche, P. C. (2001). Is the Placebo Powerless?: An Analysis of Clinical Trials Comparing Placebo with No Treatment. New England Journal of Medicine , 344 (21), 1594–1602. https://doi.org/10.1056/NEJM200105243442106
Orkaby, A. R., Gaziano, J. M., Djousse, L., & Driver, J. A. (2017). Statins for Primary Prevention of Cardiovascular Events and Mortality in Older Men. Journal of the American Geriatrics Society , 65 (11), 2362–2368. https://doi.org/10.1111/jgs.14993
Makihara, N., Kamouchi, M., Hata, J., Matsuo, R., Ago, T., Kuroda, J., Kuwashiro, T., Sugimori, H., & Kitazono, T. (2013). Statins and the risks of stroke recurrence and death after ischemic stroke: The Fukuoka Stroke Registry. Atherosclerosis , 231 (2), 211–215. https://doi.org/10.1016/j.atherosclerosis.2013.09.017
Ní Chróinín, D., Asplund, K., Åsberg, S., Callaly, E., Cuadrado-Godia, E., Díez-Tejedor, E., Di Napoli, M., Engelter, S. T., Furie, K. L., Giannopoulos, S., Gotto, A. M., Hannon, N., Jonsson, F., Kapral, M. K., Martí-Fàbregas, J., Martínez-Sánchez, P., Milionis, H. J., Montaner, J., Muscari, A., … Kelly, P. J. (2013). Statin Therapy and Outcome After Ischemic Stroke: Systematic Review and Meta-Analysis of Observational Studies and Randomized Trials. Stroke , 44 (2), 448–456. https://doi.org/10.1161/STROKEAHA.112.668277
Cinelli, C., Forney, A., & Pearl, J. (2020). A crash course in good and bad controls. Available at SSRN, 3689437: http://dx.doi.org/10.2139/ssrn.3689437
Aronow, P., Robins, J. M., Saarinen, T., Sävje, F., & Sekhon, J. (2021). Nonparametric identification is not enough, but randomized controlled trials are. ArXiv Preprint ArXiv:2108.11342 .
Higgins, J. P. T., Altman, D. G., Gotzsche, P. C., Juni, P., Moher, D., Oxman, A. D., Savovic, J., Schulz, K. F., Weeks, L., Sterne, J. A. C., Cochrane Bias Methods Group, & Cochrane Statistical Methods Group. (2011). The Cochrane Collaboration’s tool for assessing risk of bias in randomised trials. BMJ , 343 (oct18 2), d5928–d5928. https://doi.org/10.1136/bmj.d5928
Schulz, K. F., Chalmers, I., & Altman, D. G. (2002). The Landscape and Lexicon of Blinding in Randomized Trials. Annals of Internal Medicine , 136 (3), 254. https://doi.org/10.7326/0003-4819-136-3-200202050-00022
Saltaji, H. et al. Influence of blinding on treatment effect size estimate in randomized controlled trials of oral health interventions. BMC Med Res Methodol 18 , 42 (2018).
Dickersin, K., & Rennie, D. (2012). The evolution of trial registries and their use to assess the clinical trial enterprise. Jama , 307 (17), 1861–1864.
Kaplan, R. M., & Irvin, V. L. (2015). Likelihood of Null Effects of Large NHLBI Clinical Trials Has Increased over Time. PLOS ONE , 10 (8), e0132382. https://doi.org/10.1371/journal.pone.0132382
Dickersin, K., & Rennie, D. (2012). The evolution of trial registries and their use to assess the clinical trial enterprise. Jama , 307 (17), 1861–1864. https://doi.org/10.1001/jama.2012.4230
DeVito, N. J., & Goldacre, B. (2021). Evaluation of Compliance With Legal Requirements Under the FDA Amendments Act of 2007 for Timely Registration of Clinical Trials, Data Verification, Delayed Reporting, and Trial Document Submission. JAMA Internal Medicine, 181(8), 1128. https://doi.org/10.1001/jamainternmed.2021.2036
Note: Data is shown until January 2021 for trials. After the UK left the European Union in January 2021, clinical trials in the UK were then no longer required to report their results to the EU-CTR. Only data from trials by non-commercial sponsors is shown. This includes trials sponsored by institutions such as universities, hospitals, research foundations and so on.
Dal-Ré, R., Goldacre, B., Mahillo-Fernández, I., & DeVito, N. J. (2021). European non-commercial sponsors showed substantial variation in results reporting to the EU trial registry. Journal of Clinical Epidemiology , S0895435621003577. https://doi.org/10.1016/j.jclinepi.2021.11.005
Chambers, C., & Tzavella, L. (2020). The past, present, and future of Registered Reports.
Wendler, D. The Ethics of Clinical Research. in The Stanford Encyclopedia of Philosophy (ed. Zalta, E. N.) (Metaphysics Research Lab, Stanford University, 2021).
London, A. J. Equipoise in Research: Integrating Ethics and Science in Human Research. JAMA 317, 525 (2017).
Cite this work
Our articles and data visualizations rely on work from many different people and organizations. When citing this article, please also cite the underlying data sources. This article can be cited as:
BibTeX citation
Reuse this work freely
All visualizations, data, and code produced by Our World in Data are completely open access under the Creative Commons BY license . You have the permission to use, distribute, and reproduce these in any medium, provided the source and authors are credited.
The data produced by third parties and made available by Our World in Data is subject to the license terms from the original third-party authors. We will always indicate the original source of the data in our documentation, so you should always check the license of any such third-party data before use and redistribution.
All of our charts can be embedded in any site.
Our World in Data is free and accessible for everyone.
Help us do this work by making a donation.
Log in using your username and password
- Search More Search for this keyword Advanced search
- Latest content
- Current issue
- BMJ Journals
You are here
- Online First
- Simplified Helicobacter pylori therapy for patients with penicillin allergy: a randomised controlled trial of vonoprazan-tetracycline dual therapy
- Article Text
- Article info
- Citation Tools
- Rapid Responses
- Article metrics

- http://orcid.org/0000-0003-3549-6846 Wen Gao 1 ,
- Jianxiang Liu 1 ,
- Xiaolei Wang 1 ,
- Jingwen Li 2 ,
- Xuezhi Zhang 3 ,
- Jiang Li 1 ,
- Xinhong Dong 1 ,
- Binbin Liu 1 ,
- Chi Wang 1 ,
- Ying Xu 1 ,
- http://orcid.org/0000-0001-5535-4752 Guigen Teng 1 ,
- Yuling Tian 1 ,
- http://orcid.org/0000-0002-9885-261X Jinpei Dong 1 ,
- Chaoyi Ge 1 ,
- Hong Cheng 1
- 1 GI Department , Peking University First Hospital , Beijing , Beijing , China
- 2 Tsinghua University School of Medicine , Beijing , Beijing , China
- 3 TCM and Integrative Medicine Department , Peking University First Hospital , Beijing , Beijing , China
- Correspondence to Dr Hong Cheng, GI Department, Peking University First Hospital, Beijing, Beijing 100034, China; chenghong1969{at}163.com
Background and aims This study aimed to evaluate the efficacy and safety of vonoprazan and tetracycline (VT) dual therapy as first-line treatment for Helicobacter pylori infection in patients with penicillin allergy.
Methods In this randomised controlled trial, treatment-naïve adults with H. pylori infection and penicillin allergy were randomised 1:1 to receive either open-label VT dual therapy (vonoprazan 20 mg two times per day+tetracycline 500 mg three times a day) or bismuth quadruple therapy (BQT; lansoprazole 30 mg two times per day+colloidal bismuth 150 mg three times a day+tetracycline 500 mg three times a day+metronidazole 400 mg three times a day) for 14 days. The primary outcome was non-inferiority in eradication rates in the VT dual group compared with the BQT group. Secondary outcomes included assessing adverse effects.
Results 300 patients were randomised. The eradication rates in the VT group and the BQT group were: 92.0% (138/150, 95% CI 86.1% to 95.6%) and 89.3% (134/150, 95% CI 83.0% to 93.6%) in intention-to-treat analysis (difference 2.7%; 95% CI −4.6% to 10.0%; non-inferiority p=0.000); 94.5% (138/146, 95% CI 89.1% to 97.4%) and 93.1% (134/144, 95% CI 87.3% to 96.4%) in modified intention-to-treat analysis (difference 1.5%; 95% CI −4.9% to 8.0%; non-inferiority p=0.001); 95.1% (135/142, 95% CI 89.7% to 97.8%) and 97.7% (128/131, 95% CI 92.9% to 99.4%) in per-protocol analysis (difference 2.6%; 95% CI −2.9% to 8.3%; non-inferiority p=0.000). The treatment-emergent adverse events (TEAEs) were significantly lower in the VT group (14.0% vs 48.0%, p=0.000), with fewer treatment discontinuations due to TEAEs (2.0% vs 8.7%, p=0.010).
Conclusions VT dual therapy demonstrated efficacy and safety as a first-line treatment for H. pylori infection in the penicillin-allergic population, with comparable efficacy and a lower incidence of TEAEs compared with traditional BQT.
Trial registration number ChiCTR2300074693.
- Helicobacter pylori - treatment
Data availability statement
Data are available on reasonable request.
This is an open access article distributed in accordance with the Creative Commons Attribution Non Commercial (CC BY-NC 4.0) license, which permits others to distribute, remix, adapt, build upon this work non-commercially, and license their derivative works on different terms, provided the original work is properly cited, appropriate credit is given, any changes made indicated, and the use is non-commercial. See: http://creativecommons.org/licenses/by-nc/4.0/ .
https://doi.org/10.1136/gutjnl-2024-332640
Statistics from Altmetric.com
Request permissions.
If you wish to reuse any or all of this article please use the link below which will take you to the Copyright Clearance Center’s RightsLink service. You will be able to get a quick price and instant permission to reuse the content in many different ways.
WHAT IS ALREADY KNOWN ON THIS TOPIC
High-dose amoxicillin dual therapy is specifically effective for treating Helicobacter pylori infection.
So far, no dual therapy with a single antibiotic other than amoxicillin has been reported.
This therapy is unsuitable for individuals allergic to penicillin.
WHAT THIS STUDY ADDS
This study is the first randomised controlled trial using tetracycline-containing dual therapy for H. pylori treatment.
The results show that vonoprazan-tetracycline (VT) dual therapy is not inferior to classic bismuth quadruple therapy in efficacy and offers better safety and adherence.
HOW THIS STUDY MIGHT AFFECT RESEARCH, PRACTICE OR POLICY
The VT dual regimen has been proven effective, indicating that combining a single sensitive antibiotic with potent acid suppression can potentially eradicate H. pylori and offers more possibilities for expanding dual therapy.
Introduction
Helicobacter pylori eradication treatment is essential for decreasing the risk of gastric cancer. 1 High-dose proton pump inhibitor (PPI) or standard-dose vonoprazan (a novel potassium-competitive acid blocker) plus amoxicillin dual therapy has been proven to be effective and safe for H. pylori treatment in Asia. 2 3 However, amoxicillin dual therapy may not be suitable for individuals allergic to penicillin or infected with H. pylori resistant to amoxicillin. Tetracycline is one of the most effective antimicrobial agents against H. pylori , with resistance generally <1.2%~3.3%. 4 Traditionally, tetracycline was used as a component of bismuth quadruple therapy (BQT: PPI, bismuth, metronidazole and tetracycline). In our previous retrospective study, 14-day vonoprazan and tetracycline (VT) dual therapy achieved eradication rates of 100% (18/18) as first-line treatment and 90.9% (40/44, 95% CI 78.8%~96.4%) as rescue treatment. 5
This study aimed to prospectively evaluate the efficacy and tolerability of 14-day VT dual therapy compared with BQT as first-line treatment.
Materials and methods
Study design and participants.
This study was a prospective, single-centre, open-label, randomised controlled, non-inferiority trial conducted between August 2023 and March 2024 at Peking University First Hospital in China. Reporting adhered to the Consolidated Standards of Reporting Trials statement guidelines for randomised controlled trials. This trial was registered at chictr.org.cn (ChiCTR2300074693). The coauthors had access to the study data and have reviewed and approved the final manuscript.
Eligible patients were aged between 18 and 70 years, were treatment-naïve for H. pylori , and met the following criteria: they had not undergone prior treatment for H. pylori and were allergic to penicillin (due to an allergy to penicillin or a history of a positive penicillin skin test). Exclusion criteria included subjects who had previously received H. pylori treatment, pregnant or lactating women, individuals with severe systemic diseases or malignancy, those receiving antibiotics, bismuth, antisecretory drugs or with contraindications to the study drugs, or individuals deemed unsuitable for participation in the study by the researcher.
On enrolment, all subjects underwent confirmation of H. pylori infection with a positive 13 C-urea breath test (UBT, 75 mg 13 C-urea, Shenzhen Zhonghe Headway Bio-Sci & Tech).
Randomisation and interventions
At the start of the trial, patients were randomly assigned in a 1:1 ratio to receive either open-label VT dual therapy, consisting of vonoprazan 20 mg two times per day (20 mg/tablet, Takeda Pharmaceutical) and tetracycline 500 mg three times a day (250 mg/tablet, Guilin Pharmaceutical), or BQT therapy, consisting of lansoprazole 30 mg two times per day (30 mg/tablet, Takeda Pharmaceutical), colloidal bismuth 150 mg three times a day (North China Pharmaceutical), tetracycline 500 mg three times a day (250 mg/tablet, Guilin Pharmaceutical) and metronidazole 400 mg three times a day (Sichuan Kelun Pharmaceutical Research Institute), each administration for a duration of 14 days. Patients were instructed to take vonoprazan, lansoprazole and bismuth 30 min before meals, while tetracycline and metronidazole were to be taken immediately after meals.
Randomisation was conducted using a computer-generated random number sequence with a block size of four. The sequence was sealed in an envelope and kept by an independent research assistant until the intervention was assigned. This study was open-labelled, and both physicians and patients were aware of the treatment received. The technician responsible for performing 13 C-UBT was unaware of the treatment allocation. All patients were informed of the medication administration schedule, potential adverse events and the procedure for reporting them. Throughout and following the treatment period, patients were monitored for any discomforts. Patient education during consultations, combined with offline and online follow-up, was performed to enhance adherence.
Trial assessments
Demographic characteristics and relevant medical history were obtained during the screening visit. Treatment-emergent adverse events (TEAEs) and concomitant medication use were recorded. TEAEs were collected from patients who received at least one dose of medicine.
The primary end point was the eradication rate, assessed by H. pylori status via 13 C-UBT at week 8 (6 weeks after the last dose of study drugs). The secondary end point included the incidence and severity of adverse events and adherence. The severity of adverse events was graded as follows: ‘none’; ‘mild’ (transient and well-tolerated); ‘moderate’ (discomfort noticeably interfering with daily activities) or ‘severe’ (considerable interference with daily activities, or requiring hospitalisation, or resulting in a study-related death). Adherence was considered ‘poor’, if the patient had taken <80% of the prescribed medications.
Sample size calculation and statistical analysis
As a positive drug parallel control with a non-inferiority hypothesis test, the sample size was determined based on the primary end point. The sample ratio of the VT dual group to the BQT group was set at 1:1, employing a unilateral test with a one-sided α error of 0.025, a power of 80% (equivalent to a β error of 0.20) and a non-inferiority threshold of 10%. Assuming an eradication rate of 90% for the control group, 6 PASS 2021 software calculated that 142 patients per treatment group would provide over 90% power to establish non-inferiority. Accounting for potential dropouts (estimated at approximately 5% of subjects), a sample size of at least 300 subjects (with 150 subjects in each group) was targeted for recruitment in this trial.
The effectiveness of treatment was assessed in three patient cohorts: (1) intention-to-treat (ITT) analysis, which encompassed all patients collected from 2023 to 2024 with a follow-up of at least 2 months. Cases lost to follow-up were considered treatment failures; (2) modified intention-to treat (mITT) analysis, which comprised patients who received at least one dose of medication and underwent reexamination of 13 C-UBT at least 6 weeks after the end of treatment, regardless of adherence. This analysis was designed to reflect results closest to those observed in clinical practice; (3) per-protocol (PP) analysis, which included patients who had taken at least 80% of the study drugs and completed follow-up.
Continuous variables were presented as the mean±SD, while categorical variables were expressed as numbers and percentages (%). Data analysis employed Student’s t-test for continuous variables and either the χ 2 test or Fisher’s exact test for categorical variables, as appropriate. All p values were two-tailed except for the test of non-inferiority, where the level of statistical significance was specified as p<0.05. Statistical analysis was conducted using SPSS statistical software (V.26).
Patients enrolled and baseline characteristics
Enrolment occurred between August 2023 and March 2024 at Peking University First Hospital. Out of 315 patients screened for eligibility, a total of 300 were randomised, with 150 subjects allocated to the VT dual therapy group and 150 subjects to the BQT group ( figure 1 ). Final follow-up was completed in March 2024.
- Download figure
- Open in new tab
- Download powerpoint
Flow chart of screening and recruitment of study subjects. LBTM group: (or bismuth quadruple therapy group) quadruple therapy group including lansoprazole 30 mg two times per day+colloidal bismuth 150 mg three times a day+tetracycline 500 mg three times a day+metronidazole 400 mg three times a day) for 14 days. VT group: dual therapy group including vonoprazan 20 mg two times per day+tetracycline 500 mg three times a day for 14 days. Data are n (%), or mean (SD). Cigarette smoking was defined by consumed >5 cigarettes a day or >1 cigarette pack/week consumed in the past 6 months. Alcohol drinking was defined by > 50 g of alcohol/day consumed in the past 6 months. Family history of gastric cancer was defined as a family history of gastric cancer in a first-degree relative (such as parents, siblings or children), which is associated with double to triple the risk of gastric cancer. 6 The severity of AE was graded as: mild: transient and well-tolerated; moderate: discomfort noticeably interfering with daily activities; severe: considerable interference with daily activities, or requiring hospitalisation, or resulting in a study-related death. AEs, adverse effects; ITT, intention-to-treat analysis; mITT, modified intention-to-treat analysis; PP, per-protocol analysis.
The demographic and clinical characteristics of the enrolled population are summarised in table 1 . All patients were deemed penicillin allergy, either due to previous penicillin allergy or a positive history in previous penicillin skin test. There was no significant difference between the two groups in terms of gender, age, body weight, body mass index (BMI), smoking and drinking habits, family history of gastric cancer, diagnosis, accompanying diseases and medicines. Among the enrolled subjects, four cases in the VT dual therapy group and six cases in the BQT group were lost to follow-up without 13 C-UBT results. These 10 cases were considered treatment failures in the ITT analysis and were excluded in the mITT analysis. Additionally, 4 subjects in the VT dual therapy group and 13 subjects in the BQT group discontinued treatment (taken <80% of tablets) but underwent 13 C-UBT follow-up. In total, 27 subjects (8 in the VT dual therapy group and 19 in the BQT group) were excluded from the PP analysis ( figure 1 ).
- View inline
Demographic and clinical data of all patients who were involved
Eradication of H. pylori infection
The eradication rates in the VT dual therapy group and the BQT group were 92.0% (138/150, 95% CI 86.1% to 95.6%) and 89.3% (134/150, 95% CI 83.0% to 93.6%) in the ITT analysis (difference 2.7%; 95% CI −4.6% to 10.0%; non-inferiority p=0.000), respectively; 95.1% (135/142, 95% CI 89.7% to 97.8%) and 97.7% (128/131, 95% CI 92.9% to 99.4%) in the PP analysis (difference 2.6%; 95% CI −2.9% to 8.3%; non-inferiority p=0.000), 94.5% (138/146, 95% CI 89.1% to 97.4%) and 93.1% (134/144, 95% CI 87.3% to 96.4%) in the modified mITT analysis (difference 1.5%; 95% CI −4.9% to 8.0%; non-inferiority p=0.001), respectively.
There was no significant difference in the overall eradication rates between the two groups (p=0.56, 0.78 and 0.25 in the ITT, mITT and PP analysis, respectively). VT dual therapy met the primary end point of non-inferiority to BQT (lansoprazole, bismuth, tetracycline and metronidazole (LBTM) group) as first-line treatment, as the eradication rates did not significantly differ between two groups in the ITT, mITT and PP analyses ( table 2 ).
Eradication rate of each group
A total of 17 patients (13 in the LBTM group and 4 in the VT group) discontinued the medication prematurely due to TEAEs but completed the follow-up examination. The basic information and eradication status of these patients are summarised in table 3 . Although some patients did not complete the full 14-day treatment course, they still achieved successful eradication. It appears that the longer the duration of medication, the greater the likelihood of eradication.
Cure rates of those patients who stopped prematurely (with poor adherence) in each group
Adverse events and adherence
The adverse event analysis set comprised 300 patients, including 10 randomised patients who were lost to follow-up. TEAEs were reported in 48.0% (72/150) of the BQT group compared with 14.0% (21/150) in the VT dual therapy group (p=0.000) ( table 4 ). The incidence of TEAEs was higher in the BQT group than in the VT dual therapy group, whether classified as mild (p=0.000), moderate (p=0.016) or severe (p=0.005) ( table 4 ). TEAE-related discontinuations occurred in 8.7% (13/150) of the BQT group vs 2.0% (3/150) of the VT dual therapy group (p=0.010) ( table 4 ).
Adverse events in each group
All TEAEs are listed in table 4 , with nausea, dizziness, bitter taste, abdominal discomfort, vomiting and diarrhoea being the most common adverse events. No medical invention or hospitalisation was required, and no death occurred during treatment. Additionally, all adverse events disappeared after cessation of treatment. Adherence, defined as taking at least 80% of the prescribed drugs, was 87.3% (131/150) in the BQT group compared with 94.7% (142/150) in the VT dual therapy group (p=0.027).
In addition, three patients who initially failed treatment in our study underwent repeat penicillin skin test, two of them obtained negative results. Subsequently, they were administrated VA dual therapy (vonoprazan+amoxicillin) as rescue treatment, achieving successful eradication without allergic reactions ( table 5 ).
Information of three patients who received rescue therapy following initial treatment failure in the study
In our study, the vonoprazan and tetracycline dual regimen demonstrated non-inferiority to BQT for the eradication of H. pylori infection as first-line treatment among patients with penicillin allergy. This randomised clinical study involving 300 patients provided the initial assessment of VT dual therapy, supporting the efficacy of tetracycline-containing dual therapy for H. pylori infection treatment as first-line option.
Antibiotic resistance is a significant factor contributing to the failure of H. pylori eradication. It has been reported that the overall prevalence of primary antibiotic resistance of H. pylori in the Asia-Pacific region between 1990 and 2022 was 22% (95% CI 20% to 23%) for clarithromycin, 52% (95% CI 49% to 55%) for metronidazole, 26% (95% CI 24% to 29%) for levofloxacin, 4% (95% CI 3% to 5%) for tetracycline and 4% (95% CI 3% to 5%) for amoxicillin. 7 Given that infectious disease studies are often susceptibility-based, 8 tetracycline and amoxicillin emerge as primary option for antibiotic candidates. Amoxicillin, characterised by its low resistance rate, high efficacy, safety and availability, is an essential component of H. pylori eradication regimens. Recently, dual therapy comprising high-dose amoxicillin has been validated as effective. 9 10 However, amoxicillin may not be the preferred choice, as approximately 10% of the population in the USA and 4%–5.6% in the Asia-Pacific region (including China) report penicillin allergy. 11 According to the Maastricht VI/Florence consensus report, BQT (PPI, bismuth, metronidazole and tetracycline) is the preferred treatment option for this population. 1 Tetracycline, at the outset of H. pylori treatment, has shown efficacy as a single-antibiotic regimen. 12 The efficacy of tetracycline-containing dual therapy was initially explored by Al-Assi et al . 13 A dual therapy comprising omeprazole 20 mg three times a day+tetracycline 500 mg four times a day and omeprazole 40 mg once daily plus tetracycline 500 mg four times a day, alongside bismuth subsalicylate 2 tablets four times a day for 14 days, was administrated to 19 and 20 patients with H. pylori -positive peptic ulcer disease, respectively. Successful eradication was achieved in 5/19 (26%) and 12/25 (48%) patients in tetracycline dual therapy group and the bismuth-adding group, respectively. However, the efficacy of tetracycline dual therapy was deemed inadequate for routine treatment, with low intragastric pH being considered a major barrier to effective antimicrobial therapy.
When the environmental pH increased from 5.0 to 6.0, the Minimum Inhibitory Concentration 90% (MIC90) for tetracycline decreased from 0.5 mg/L to 0.125 mg/L. 14 However, the evolution of the medicine has facilitated the updating of treatment regimens and rejuvenated the efficacy of tetracycline-containing dual therapy. Vonoprazan, a potassium-competitive acid blocker, swiftly and potently elevates intragastric pH levels and sustains them to a greater extent than PPIs. It has been associated with higher H. pylori eradication rates in amoxicillin-containing dual therapy. 9 Inspired by the enhanced efficacy observed in AMX-containing dual therapy, it was hypothesised that tetracycline’s antimicrobial effect might be more stable and exhibit better bioavailability in the gastric cavity under the higher intragastric pH value produced by vonoprazan. The results of this study demonstrated that the efficacy of VT dual therapy was non-inferior to that of classical BQT, as determined by ITT, mITT and PP analyses.
Gastric acid can reduce the absorption rate of tetracycline antibiotics, particularly in environments with higher acidity levels. Additionally, gastric acid may undergo chemical reactions with tetracycline, leading to partial degradation or inactivation. This discrepancy might explain why VT dual therapy yielded different results compared with omeprazole and tetracycline dual therapy in previous study.
Adherence and adverse events play a significant role in successful eradication. In the BQT group, 48.0% of patients reported TEAEs, with 8.7% discontinuing treatment as a result. In fact, TEAEs are frequently encountered during BQT treatment. 15 16 Gisbert 17 reported that the overall incidence of adverse events was 43% (95% CI 35% to 50%, 24 studies), leading to nearly 3% of the treatment being interrupted. 6 In our study, VT dual therapy demonstrated fewer TEAEs compared with BQT (14.0% vs 48.0%, p=0.000), while maintaining similar efficacy. This suggested that it could be a promising candidate for optimisation of regimens.
The occurrence of TEAEs was significantly lower in the VT dual therapy group compared with the BQT group (14.0% vs 48.0%, p=0.000), across all grades of severity. In theory, the use of fewer drugs leads to fewer side effects. VT dual therapy represents an optimised approach to classical BQT, offering high efficacy with low TEAE rates.
This is the first randomised trial reporting the efficacy of VT dual therapy in first-line therapy and in patients with penicillin allergy. In comparison with amoxicillin, tetracycline presents a convenient option for prescription as there is no requirement for allergy testing. Restrictions on amoxicillin use are often influenced by concerns regarding ‘penicillin-allergic’ status or positive penicillin skin test results. However, it is worth noting that while 10% of the population has reported penicillin allergies, only 5% of these cases were considered true allergies, meaning that 95% of reported allergies may be categorised as ‘false penicillin allergy’. 18 In our study, it is likely that at least two individuals with ‘false allergy’ were included who were administrated VA dual therapy (vonoprazan+amoxicillin) as rescue treatment, achieving successful eradication without allergy reactions ( table 5 ).
The VT dual therapy may also need to be optimised since the vonoprazan trial and amoxicillin dual therapy shows better effectiveness in Asian regions, while the eradication rate is relatively low in European and American countries. 19 Possible factors related to differences in eradication effectiveness include 20 : the timing of antibiotic administration, emergence of resistance, failure to achieve the intragastric pH required for effectiveness (including different prevalence of rapid metabolisers) and host factors such as body wight, for instance, the average BMI in the US/European study was 28.7 and 29.1, respectively, 19 whereas the population in our study has an average BMI of 23.2 ( table 1 ). Optimisation could be approached through the following aspects: (1) to improve the effectiveness of vonoprazan by increasing the dosage, frequency of administration or both, or alternatively adding an H 2 receptor antagonist concurrently 20 ; (2) to increase the dose of tetracycline from 1.5 g/day to 2.25 g/day, which has been confirmed to be safe in a large population study in China (750 mg three times a day) 21 ; (3) to perform antibiotic resistance testing before treatment 1 8 ; (4) to adjust the timing of medication of antibiotic from before meal to after meal. 20
This study simultaneously conducted patient education when prescribing medications, including medication timing, precautions and reminded patients to contact the researchers at any time if they had questions or discomfort during medication. The medication process included maintaining telephone or online follow-ups. Therefore, there were fewer lost to follow-ups in this study, and there was not much difference in the results between ITT, mITT and PP. Previous studies have shown that adequate patient education and close follow-up during the medication process can effectively improve adherence, thereby increasing the success rate of eradication. 22
Limitations
There were several limitations in our study. First, this trail was open-label and VT dual regimen is distinguishable from BQT, which might have influenced the incidence of adverse events or introduced other potential biases. Second, the study was conducted in a single medical centre with a limited population of patients with penicillin allergy. It needs to be verified whether the combination is applicable to a broader population. Third, there was no data available regarding H. pylori isolation and antimicrobial susceptibility in this study. Fourth, using vonoprazan-based BQT as comparator appears to be more favourable than using PPI. Fifth, vonoprazan is not available in many Western countries (it became available in the USA in 2022), and tetracycline may be difficult to obtain in many countries, including most tertiary hospital in China, thus limiting the use of this therapy. In the future, we could explore dual therapy regimens involving minocycline or doxycycline. Nevertheless, despite these limitations, this study still contributed to the promotion of tetracycline-containing dual therapy, especially for patients who are not suitable for amoxicillin use. 23
VT dual therapy, consisting of vonoprazan 20 mg two times per day and tetracycline 500 mg three times a day for 14 days, proved to be effective and safe as first-line treatment for H. pylori infection in a population with penicillin allergy. VT dual therapy could serve as an optimised alternative to classical BQT, offering similar efficacy, fewer TEAEs and good adherence. Further studies are needed to evaluate the efficacy of VT dual therapy in the general population, both as a primary and as a rescue option.
Ethics statements
Patient consent for publication.
Not applicable.
Ethics approval
The study received approval from the Ethics Committee Ethics Committee of Peking University First Hospital, in accordance with the principles of the Declaration of Helsinki. Written informed consent was obtained from all patients prior to their participation in the study.
- Malfertheiner P ,
- Megraud F ,
- Rokkas T , et al
- Zhang T , et al
- Wang C , et al
- Wang J , et al
- Liu J , et al
- Choi IJ , et al
- El-Omar EM ,
- Kuo Y-T , et al
- Ouyang Y , et al
- Tai W-C , et al
- Yeung MHY ,
- Wong JCY , et al
- Al-Assi MT ,
- Sheng W-H ,
- Liou J-M , et al
- Ding Z , et al
- Alsamman MA ,
- Vecchio EC ,
- Shawwa K , et al
- Nyssen OP ,
- McNicholl AG ,
- Brigham TJ , et al
- Mégraud F ,
- Laine L , et al
- Gerhard M , et al
- Qu J-Y , et al
Contributors Study concept and design: HC, WG. Case collection and data acquisition: HC, WG, JL (Jianxiang Liu), XW, XZ, HY, XD, BL, CW, YX, GT, YT, JD, CG. Performance of 13 C-UBT: JL(Jiang Li). Analysis and interpretation of data, statistical analysis, drafting of the manuscript: WG, HC, JL (Jingwen Li). Critical revision of the manuscript for important intellectual content: HC. Drafting of the manuscript revision: HC, WG, JL (Jiangwen Li). HC is the guarantor of this work and accepts full responsibility for the content, the conduct of the study, access to the data and the decision to publish.
Funding National High Level Hospital Clinical Research Funding (Youth Clinical Research Project of Peking University First Hospital) 2023YC27.
Competing interests None declared.
Patient and public involvement Patients and/or the public were not involved in the design, or conduct, or reporting, or dissemination plans of this research.
Provenance and peer review Not commissioned; externally peer reviewed.
Read the full text or download the PDF:

An official website of the United States government
The .gov means it’s official. Federal government websites often end in .gov or .mil. Before sharing sensitive information, make sure you’re on a federal government site.
The site is secure. The https:// ensures that you are connecting to the official website and that any information you provide is encrypted and transmitted securely.
- Publications
- Account settings
- My Bibliography
- Collections
- Citation manager
Save citation to file
Email citation, add to collections.
- Create a new collection
- Add to an existing collection
Add to My Bibliography
Your saved search, create a file for external citation management software, your rss feed.
- Search in PubMed
- Search in NLM Catalog
- Add to Search
A simplified guide to randomized controlled trials
Affiliations.
- 1 Fetal Medicine Unit, St. Georges University Hospital, London, UK.
- 2 Division of Neonatology, Department of Pediatrics, Mount Sinai Hospital, Toronto, ON, Canada.
- 3 Institute of Health Policy, Management and Evaluation, University of Toronto, Toronto, ON, Canada.
- 4 Department of Clinical Science, Intervention and Technology, Karolinska Institute and Center for Fetal Medicine, Karolinska University Hospital, Stockholm, Sweden.
- 5 Women's Health and Perinatology Research Group, Department of Clinical Medicine, UiT-The Arctic University of Norway, Tromsø, Norway.
- 6 Department of Obstetrics and Gynecology, University Hospital of North Norway, Tromsø, Norway.
- PMID: 29377058
- DOI: 10.1111/aogs.13309
A randomized controlled trial is a prospective, comparative, quantitative study/experiment performed under controlled conditions with random allocation of interventions to comparison groups. The randomized controlled trial is the most rigorous and robust research method of determining whether a cause-effect relation exists between an intervention and an outcome. High-quality evidence can be generated by performing an randomized controlled trial when evaluating the effectiveness and safety of an intervention. Furthermore, randomized controlled trials yield themselves well to systematic review and meta-analysis providing a solid base for synthesizing evidence generated by such studies. Evidence-based clinical practice improves patient outcomes and safety, and is generally cost-effective. Therefore, randomized controlled trials are becoming increasingly popular in all areas of clinical medicine including perinatology. However, designing and conducting an randomized controlled trial, analyzing data, interpreting findings and disseminating results can be challenging as there are several practicalities to be considered. In this review, we provide simple descriptive guidance on planning, conducting, analyzing and reporting randomized controlled trials.
Keywords: Clinical trial; good clinical practice; random allocation; randomized controlled trial; research methods; study design.
© 2018 Nordic Federation of Societies of Obstetrics and Gynecology.
PubMed Disclaimer
Similar articles
- Ethical pitfalls in neonatal comparative effectiveness trials. Modi N. Modi N. Neonatology. 2014;105(4):350-1. doi: 10.1159/000360650. Epub 2014 May 30. Neonatology. 2014. PMID: 24931328
- Evidence-based medicine, systematic reviews, and guidelines in interventional pain management: Part 2: Randomized controlled trials. Manchikanti L, Hirsch JA, Smith HS. Manchikanti L, et al. Pain Physician. 2008 Nov-Dec;11(6):717-73. Pain Physician. 2008. PMID: 19057624 Review.
- Clinical research in interventional pain management techniques: the clinician's point of view. Van Zundert J. Van Zundert J. Pain Pract. 2007 Sep;7(3):221-9. doi: 10.1111/j.1533-2500.2007.00139.x. Pain Pract. 2007. PMID: 17714100 Review.
- Methodology citations and the quality of randomized controlled trials in obstetrics and gynecology. Grimes DA, Schulz KF. Grimes DA, et al. Am J Obstet Gynecol. 1996 Apr;174(4):1312-5. doi: 10.1016/s0002-9378(96)70677-4. Am J Obstet Gynecol. 1996. PMID: 8623862
- Assessing the quality of randomization from reports of controlled trials published in obstetrics and gynecology journals. Schulz KF, Chalmers I, Grimes DA, Altman DG. Schulz KF, et al. JAMA. 1994 Jul 13;272(2):125-8. JAMA. 1994. PMID: 8015122
- Influence of Cavity Lining on the 3-Year Clinical Outcome of Posterior Composite Restorations: A Randomized Controlled Clinical Trial. Nguyen AD, Pütz N, Michaelis M, Bitter K, Gernhardt CR. Nguyen AD, et al. Dent J (Basel). 2024 May 7;12(5):128. doi: 10.3390/dj12050128. Dent J (Basel). 2024. PMID: 38786526 Free PMC article.
- Denosumab vs. bisphosphonates in primary osteoporosis: a meta-analysis of comparative safety in randomized controlled trials. Kobayashi T, Morimoto T, Ito K, Mawatari M, Shimazaki T. Kobayashi T, et al. Osteoporos Int. 2024 May 11. doi: 10.1007/s00198-024-07118-0. Online ahead of print. Osteoporos Int. 2024. PMID: 38733394 Review.
- Portable robots for upper-limb rehabilitation after stroke: a systematic review and meta-analysis. Tseng KC, Wang L, Hsieh C, Wong AM. Tseng KC, et al. Ann Med. 2024 Dec;56(1):2337735. doi: 10.1080/07853890.2024.2337735. Epub 2024 Apr 19. Ann Med. 2024. PMID: 38640459 Free PMC article.
- Research hotspots and trends on acupuncture treatment for headache: a bibliometric analysis from 2003 to 2023. Zhao S, Hu S, Luo Y, Li W, Zhao F, Wang C, Meng F, He X. Zhao S, et al. Front Neurosci. 2024 Mar 21;18:1338323. doi: 10.3389/fnins.2024.1338323. eCollection 2024. Front Neurosci. 2024. PMID: 38591064 Free PMC article.
- Effect of lipid emulsion on neuropsychiatric drug-induced toxicity: A narrative review. Hwang Y, Sohn JT. Hwang Y, et al. Medicine (Baltimore). 2024 Mar 15;103(11):e37612. doi: 10.1097/MD.0000000000037612. Medicine (Baltimore). 2024. PMID: 38489675 Free PMC article. Review.
Publication types
- Search in MeSH
Related information
- Cited in Books
LinkOut - more resources
Full text sources.
- Ovid Technologies, Inc.
Other Literature Sources
- scite Smart Citations
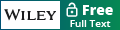
- Citation Manager
NCBI Literature Resources
MeSH PMC Bookshelf Disclaimer
The PubMed wordmark and PubMed logo are registered trademarks of the U.S. Department of Health and Human Services (HHS). Unauthorized use of these marks is strictly prohibited.

An official website of the United States government
The .gov means it’s official. Federal government websites often end in .gov or .mil. Before sharing sensitive information, make sure you’re on a federal government site.
The site is secure. The https:// ensures that you are connecting to the official website and that any information you provide is encrypted and transmitted securely.
- Publications
- Account settings
Preview improvements coming to the PMC website in October 2024. Learn More or Try it out now .
- Advanced Search
- Journal List
- Curr Control Trials Cardiovasc Med
- v.2(3); 2001
Bridging case-control studies and randomized trials
Frits r rosendaal.
1 Leiden University Medical Center (LUMC), Department of Clinical Epidemiology, C0-P, PO Box 9600, 2300 RC Leiden, The Netherlands
Randomized trials and observational studies, such as case-control studies, are often seen as opposing approaches. However, in many instances results obtained by different designs may complement each other. For instance, case-control studies on aetiology of disease may help to give the direction of future trials. In this commentary, the author discusses the purpose of randomization and observation, and under which conditions one design may be preferred to another. Randomization is useful to combat 'confounding by indication', and is therefore the design of choice for most therapeutic trials. When this confounding is not an issue, as in studies of genetic risk factors or side-effects, then case-control studies are preferred.
In this issue of Current Controlled Trials in Cardiovascular Medicine , Ray et al [ 1 ] report the results of a study on genetic and acquired risk factors for venous thrombosis in women. This paper is remarkable, not only because it focuses on women, but also because it is an observational, case-control study rather than a randomized trial.
In their editorial in the first issue of the journal, editors-in-chief Curt Furberg and Bertram Pitt did not explicitly mention randomized trials - they spoke of a journal for 'clinical trials' [ 2 ]. This suggests experimental rather than observational studies, but does not necessarily imply randomization. Nevertheless, by encouraging prospective authors to report trial results according to the Consolidated Standards of Reporting Trials guidelines [ 3 ], they implicitly made it clear that the journal was aimed at reporting randomized clinical trials.
Does this publication therefore represent a major change in policy? Did it take only a handful of issues before the editors decided to 'lower' their standards? I think not. Sir Austin Bradford Hill is credited with performing the first properly randomized trial in 1948 [ 4 ], although studies with some form of random treatment allocation antedated it by at least 50 years [ 5 ]. When we read his Principles of Medical Statistics , from the first edition in 1937 [ 6 ] to the last posthumous edition of 1984 [ 7 ], we see an increasing emphasis on randomization, the use of placebo controls and double blinding. However, even as a strong advocate for experimentation, he defined a clinical trial as a study in which we learn from a patient; up to the 12th edition he continued to quote the 1949 Presidential Address to the Royal Society of Medicine by Sir George Pickering, who argued that all that happened to a patient should be recorded.
Randomization is a tool, not a goal in and of itself. The goal of clinical research is to obtain an answer that is valid and precise, and the ultimate goal is to prevent and treat disease in the best way. Each study design has indications and contraindications. The main threats to validity in treatment studies are regression to the mean (ie improvement due to the natural course of a disorder) and 'confounding by indication' (ie incomparability of groups when the risk profile affects the choice of drug). Control groups are included to address regression to the mean, whereas randomization is aimed at creating groups with similar prognosis to combat confounding by indication. In clinical practice, physicians tailor treatment to a patient's prognosis, and so a simple comparison of patients treated with different regimens will often be biased. Because of the need to counter this confounding by indication, randomization has become nearly synonymous with good research into medical therapies. Many have broadened this to the belief that randomization is synonymous with good research, and have created a hierarchy of study designs. This is a mistake. First, randomized trials do have drawbacks. Secondly, they are not always possible, or, for that matter, ethical.
One important drawback of randomized trials is that they typically involve patients who were considered fit to enter, were likely to finish the trial, and believed, or even shown during a run-in phase, to comply with the medications. This population is quite different from the patients in the waiting room. Another important drawback is that, because the precision of an estimate is dependent on the number of patients experiencing an event, randomized trials, unless they are very large, will seldom be precise. A third drawback is that in all prospective studies, including randomized trials, it is seldom possible to relate the outcome of interest to determinants that occurred immediately before that outcome, and that might even have interacted in producing it (for instance lifestyle factors, intercurrent disease). In some cases, randomization is simply not possible, as in aetiological studies of genetic variants. Also, even for nongenetic risk factors, randomization would often lead to ethical problems (for instance, studies on the effects of alcohol).
Case-control studies, such as the one on venous thrombosis published in the present issue [ 1 ], have other indications and contraindications. In this type of study, patients with the outcome of interest are contrasted to those without, and therefore the precision of the estimate is much greater. Ideally, all patients in a certain geographical region are included, so generalizibility is better. Finally, in contrast to randomized trials and other cohort studies, patients can be seen shortly after the event and recent risk factors can be recorded.
Case-control studies also have drawbacks; if the disease changes the risk factor measurement, then inference becomes difficult (for instance, varicose veins are often seen after a deep vein thrombosis, but are probably not a cause of venous thrombosis). In studies of treatments, case-control studies, like all observational studies, may be subject to bias through confounding by indication. It is important to make a distinction between expected or intended effects (efficacy), and unintended or unexpected effects (side effects). Although in the case of efficacy confounding by indication is a likely source of bias, this is not so in the case of side effects. If physicians or patients neither intend nor expect a certain effect of a drug, then the presence of risk factors for that effect is unlikely to affect prescription, and therefore groups using and not using the drug will be comparable, and estimates will be unbiased. This can be illustrated with the effects of hormone replacement therapy. A large observational study (the Nurses' Health study) showed a strong protective effect on coronary heart disease [ 8 ] that was not confirmed in a randomized trial [ 9 ]. Both studies found very similar relative risks of venous thrombosis, which was an unexpected side effect [ 10 , 11 ].
Genetic studies on the aetiology of disease and side effects of drugs are needed to direct or complement randomized trials of therapies. For both such study types the case-control design is the best choice. It is therefore appropriate that case-control studies and randomized controlled trials are published side by side, in order to serve our ultimate goal of improving patient care.
- Ray JG, Langman L, Vermeulen MJ, Evrovski J, Yeo E, Cole DEC. Genetics University of Toronto Thrombophilia Study in Women (GUTTSI): genetic and other risk factors for venous thromboembolism in women. Curr Control Clin Trials Cardiovasc Med. 2001; 2 :141–149. doi: 10.1186/CVM-2-3-141. [ PMC free article ] [ PubMed ] [ CrossRef ] [ Google Scholar ]
- Furberg C, Pitt B. Current Controlled Trials in Cardiovascular Medicine : a new journal for a new age (http://cvm.controlled-trials.com). Current Controlled Trials in Cardiovascular Medicine. 2000; 1 :1–2. doi: 10.1186/CVM-1-1-001. [ PMC free article ] [ PubMed ] [ CrossRef ] [ Google Scholar ]
- Begg C, Cho M, Eastwood ELS, Horton R, Moher D, Olkin I, Pitkin R, Rennie D, Schulz K, Simel D, Stoup D. Improving the quality of reporting of randomised controlled trials. The CONSORT statement. JAMA. 1996; 276 :637–639. doi: 10.1001/jama.276.8.637. [ PubMed ] [ CrossRef ] [ Google Scholar ]
- Medical Research Council Streptomycin treatment of pulmonary tuberculosis. Br Med J. 1948; ii :769–782. [ PMC free article ] [ PubMed ] [ Google Scholar ]
- Fibiger J. On treatment of diptheria with serum [in Danish]. Hospitalstidende. 1898; 6 :309–325. [ Google Scholar ]
- Hill AB. Principles of Medical Statistics, 1st ed London: Lancet; 1937.
- Hill AB, Hill ID. Principles of Medical Statistics 12th ed London: Edward Arnold; 1984.
- Stampfer MJ, Willett WC, Colditz GA, Rosner B, Speizer FE, Hennekens CH. A prospective study of postmenopausal estrogen therapy and coronary heart disease. N Engl J Med. 1985; 313 :1044–1049. [ PubMed ] [ Google Scholar ]
- Hulley S, Grady D, Bush T, Furberg C, Herrington D, Riggs B, Vit-tinghoff E, for the Heart and estrogen/progestin Replacement Study (HERS) Research Group Randomized trial of estrogen plus progestin for secondary prevention of coronary heart disease in postmenopausal women. JAMA. 1998; 280 :605–613. doi: 10.1001/jama.280.7.605. [ PubMed ] [ CrossRef ] [ Google Scholar ]
- Grodstein F, Stampfer MJ, Goldhaber SZ, Manson JE, Colditz GA, Speizer FE, Willett WC, Hennekens CH. Prospective study of exogenous hormones and risk of pulmonary embolism in women. Lancet. 1996; 348 :983–987. doi: 10.1016/S0140-6736(96)07308-4. [ PubMed ] [ CrossRef ] [ Google Scholar ]
- Grady D, Wenger NK, Herrington D, Khan S, Furberg C, Hunninghoke D, Vittinghoff E, Hulley S. Postmenopausal hormone therapy increases risk for venous thromboembolic disease. Ann Intern Med. 2000; 132 :689–696. [ PubMed ] [ Google Scholar ]
- Introduction
- Conclusions
- Article Information
Participants continued to live in their home environment without any prescribed diet or physical activity during the 28 consecutive days of the study. Error bars are SEs of the mean. The vertical dashed line separates the two 2-week sleep periods.
A-D, Data are in ascending order of change in sleep duration for the control group and sleep extension group. E, Data were from 74 participants. All available data were used. The line represents the line of best fit from the linear regression model. One participant in the control group and 3 participants in the sleep extension group had missing data in change in sleep duration (ie, missing mean data in at least 1 of 2 study periods). One participant in the control group and 4 participants in the sleep extension group had missing data in change in energy intake. Overall, 1 participant in the control group and 5 participants in the sleep extension group had missing data in either change in sleep duration or change in energy intake.
Trial Protocol
eMethods. Participants, Inclusion and Exclusion Criteria
eReferences
eTable 1. Effect of Treatment on Actigraphy-Based Time in Bed and Sleep Duration on All Days, Workdays and Free Days
eTable 2. Effect of Treatment on Actigraphy-Based Outcomes
eTable 3. Baseline Characteristics of Participants With Complete vs Incomplete Data
eTable 4. Self-Reported Outcomes by Visual Analog Scales
Data Sharing Statement
- Good Sleep, Better Life—Enhancing Health and Safety With Optimal Sleep JAMA Internal Medicine Invited Commentary April 1, 2022 Mark R. Rosekind, PhD; Rafael Pelayo, MD; Debra A. Babcock, MD
See More About
Select your interests.
Customize your JAMA Network experience by selecting one or more topics from the list below.
- Academic Medicine
- Acid Base, Electrolytes, Fluids
- Allergy and Clinical Immunology
- American Indian or Alaska Natives
- Anesthesiology
- Anticoagulation
- Art and Images in Psychiatry
- Artificial Intelligence
- Assisted Reproduction
- Bleeding and Transfusion
- Caring for the Critically Ill Patient
- Challenges in Clinical Electrocardiography
- Climate and Health
- Climate Change
- Clinical Challenge
- Clinical Decision Support
- Clinical Implications of Basic Neuroscience
- Clinical Pharmacy and Pharmacology
- Complementary and Alternative Medicine
- Consensus Statements
- Coronavirus (COVID-19)
- Critical Care Medicine
- Cultural Competency
- Dental Medicine
- Dermatology
- Diabetes and Endocrinology
- Diagnostic Test Interpretation
- Drug Development
- Electronic Health Records
- Emergency Medicine
- End of Life, Hospice, Palliative Care
- Environmental Health
- Equity, Diversity, and Inclusion
- Facial Plastic Surgery
- Gastroenterology and Hepatology
- Genetics and Genomics
- Genomics and Precision Health
- Global Health
- Guide to Statistics and Methods
- Hair Disorders
- Health Care Delivery Models
- Health Care Economics, Insurance, Payment
- Health Care Quality
- Health Care Reform
- Health Care Safety
- Health Care Workforce
- Health Disparities
- Health Inequities
- Health Policy
- Health Systems Science
- History of Medicine
- Hypertension
- Images in Neurology
- Implementation Science
- Infectious Diseases
- Innovations in Health Care Delivery
- JAMA Infographic
- Law and Medicine
- Leading Change
- Less is More
- LGBTQIA Medicine
- Lifestyle Behaviors
- Medical Coding
- Medical Devices and Equipment
- Medical Education
- Medical Education and Training
- Medical Journals and Publishing
- Mobile Health and Telemedicine
- Narrative Medicine
- Neuroscience and Psychiatry
- Notable Notes
- Nutrition, Obesity, Exercise
- Obstetrics and Gynecology
- Occupational Health
- Ophthalmology
- Orthopedics
- Otolaryngology
- Pain Medicine
- Palliative Care
- Pathology and Laboratory Medicine
- Patient Care
- Patient Information
- Performance Improvement
- Performance Measures
- Perioperative Care and Consultation
- Pharmacoeconomics
- Pharmacoepidemiology
- Pharmacogenetics
- Pharmacy and Clinical Pharmacology
- Physical Medicine and Rehabilitation
- Physical Therapy
- Physician Leadership
- Population Health
- Primary Care
- Professional Well-being
- Professionalism
- Psychiatry and Behavioral Health
- Public Health
- Pulmonary Medicine
- Regulatory Agencies
- Reproductive Health
- Research, Methods, Statistics
- Resuscitation
- Rheumatology
- Risk Management
- Scientific Discovery and the Future of Medicine
- Shared Decision Making and Communication
- Sleep Medicine
- Sports Medicine
- Stem Cell Transplantation
- Substance Use and Addiction Medicine
- Surgical Innovation
- Surgical Pearls
- Teachable Moment
- Technology and Finance
- The Art of JAMA
- The Arts and Medicine
- The Rational Clinical Examination
- Tobacco and e-Cigarettes
- Translational Medicine
- Trauma and Injury
- Treatment Adherence
- Ultrasonography
- Users' Guide to the Medical Literature
- Vaccination
- Venous Thromboembolism
- Veterans Health
- Women's Health
- Workflow and Process
- Wound Care, Infection, Healing
Others Also Liked
- Download PDF
- X Facebook More LinkedIn
Tasali E , Wroblewski K , Kahn E , Kilkus J , Schoeller DA. Effect of Sleep Extension on Objectively Assessed Energy Intake Among Adults With Overweight in Real-life Settings : A Randomized Clinical Trial . JAMA Intern Med. 2022;182(4):365–374. doi:10.1001/jamainternmed.2021.8098
Manage citations:
© 2024
- Permissions
Effect of Sleep Extension on Objectively Assessed Energy Intake Among Adults With Overweight in Real-life Settings : A Randomized Clinical Trial
- 1 Department of Medicine, The University of Chicago, Chicago, Illinois
- 2 Department of Public Health Sciences, The University of Chicago, Chicago, Illinois
- 3 Biotechnology Center, Department of Nutritional Sciences, University of Wisconsin–Madison, Madison
- Invited Commentary Good Sleep, Better Life—Enhancing Health and Safety With Optimal Sleep Mark R. Rosekind, PhD; Rafael Pelayo, MD; Debra A. Babcock, MD JAMA Internal Medicine
Question What is the effect of sleep extension on objectively assessed energy intake in adults with overweight in their usual home environment?
Findings In this randomized clinical trial of 80 adults with overweight and habitual sleep less than 6.5 hours per night, those randomized to a 2-week sleep extension intervention significantly reduced their daily energy intake by approximately 270 kcal compared with the control group. Total energy expenditure did not significantly differ between the sleep extension and control groups, resulting in a negative energy balance with sleep extension.
Meaning The findings suggest that improving and maintaining adequate sleep duration could reduce weight and be a viable intervention for obesity prevention and weight loss programs.
Importance Short sleep duration has been recognized as a risk factor for obesity. Whether extending sleep duration may mitigate this risk remains unknown.
Objective To determine the effects of a sleep extension intervention on objectively assessed energy intake, energy expenditure, and body weight in real-life settings among adults with overweight who habitually curtailed their sleep duration.
Design, Setting, and Participants This single-center, randomized clinical trial was conducted from November 1, 2014, to October 30, 2020. Participants were adults aged 21 to 40 years with a body mass index (calculated as weight in kilograms divided by height in meters squared) between 25.0 and 29.9 and had habitual sleep duration of less than 6.5 hours per night. Data were analyzed according to the intention-to-treat principle.
Interventions After a 2-week habitual sleep period at baseline, participants were randomized to either an individualized sleep hygiene counseling session that was intended to extend their bedtime to 8.5 hours (sleep extension group) or to continue their habitual sleep (control group). All participants were instructed to continue daily routine activities at home without any prescribed diet or physical activity.
Main Outcomes and Measures The primary outcome was change in energy intake from baseline, which was objectively assessed as the sum of total energy expenditure and change in body energy stores. Total energy expenditure was measured by the doubly labeled water method. Change in body energy stores was computed using regression of daily home weights and body composition changes from dual-energy x-ray absorptiometry. Sleep duration was monitored by actigraphy. Changes from baseline were compared between the 2 groups using intention-to-treat analysis.
Results Data from 80 randomized participants (mean [SD] age, 29.8 [5.1] years; 41 men [51.3%]) were analyzed. Sleep duration was increased by approximately 1.2 hours per night (95% CI, 1.0 to 1.4 hours; P < .001) in the sleep extension group vs the control group. The sleep extension group had a significant decrease in energy intake compared with the control group (−270 kcal/d; 95% CI, −393 to −147 kcal/d; P < .001). The change in sleep duration was inversely correlated with the change in energy intake ( r = −0.41; 95% CI, −0.59 to −0.20; P < .001). No significant treatment effect in total energy expenditure was found, resulting in weight reduction in the sleep extension group vs the control group.
Conclusions and Relevance This trial found that sleep extension reduced energy intake and resulted in a negative energy balance in real-life settings among adults with overweight who habitually curtailed their sleep duration. Improving and maintaining healthy sleep duration over longer periods could be part of obesity prevention and weight loss programs.
Trial Registration ClinicalTrials.gov Identifier: NCT02253368
Obesity is a major public health concern. 1 The obesity epidemic appears to coincide with a pattern of sleeping less that has been observed in society over the past several decades. For example, one-third of the US population reported not getting the recommended 7 to 9 hours of sleep per night. 2 - 4 Substantial evidence suggests that sleeping less than 7 hours per night on a regular basis is associated with adverse health consequences. 5 Particularly, insufficient sleep duration has been increasingly recognized as an important risk factor for obesity. 6 , 7 Prospective epidemiologic studies suggest that short sleep duration is an important risk factor for weight gain. 8 - 10 However, it remains unknown whether extending sleep duration can be an effective strategy for preventing or reversing obesity. Although sleep hygiene education is encouraged by obesity experts, 11 most health professionals and patients do not implement obtaining adequate sleep duration as part of the strategies to combat the obesity epidemic. 12
At the population level, the association between energy flux and body weight implicates that increased energy intake is the main factor in higher body weights in modern society. 13 According to dynamic prediction models, a sustained increase in energy intake of even 100 kcal/d would result in a weight gain of about 4.5 kg over 3 years. 14 , 15 Factors that underlie the observed persistent increase in energy intake and mean weight gain at the population level need to be better understood. One such factor is insufficient sleep duration. Short-term experimental laboratory studies have found that sleep restriction in healthy individuals is associated with an increased mean energy intake of about 250 to 350 kcal/d with minimal to no change in energy expenditure. 16 - 19 However, these laboratory studies do not represent real life. The magnitude of sleep restriction was extreme in most cases, and energy intake was ascertained from a single or a few meals. In a real-life setting in which participants continue their normal daily activities, multiple interacting factors (eg, social interactions and free-living physical activity) can influence energy intake or expenditure and weight.
To date, it remains unknown whether and to what extent an intervention that is intended to increase sleep duration in a real-life setting affects energy balance and body weight. We conducted a randomized clinical trial (RCT) to determine the effects of a sleep extension intervention on objectively assessed energy intake, energy expenditure, and body weight in real-life settings among adults with overweight who habitually curtailed their sleep duration.
This single-center, parallel-group RCT was conducted from November 1, 2014, to October 30, 2020. The protocol was approved by The University of Chicago Institutional Review Board, and participants provided written informed consent. The study protocol is available in Supplement 1 . We followed the Consolidated Standards of Reporting Trials ( CONSORT ) reporting guideline.
Adult men and women aged 21 to 40 years with a body mass index (calculated as weight in kilograms divided by height in meters squared) between 25.0 and 29.9 and a mean habitual sleep duration of less than 6.5 hours per night were eligible. Individuals were required to have stable self-reported sleep habits for the past 6 months. They were recruited from the community and completed an initial online survey followed by a face-to-face interview. Race and ethnicity data were self-reported at this time and included the following race and ethnicity categories: Asian, Black or African American, Hispanic, and White. Those who met the inclusion criteria underwent laboratory screening (polysomnography, oral glucose tolerance test, and blood tests) to determine eligibility. Habitual sleep duration was confirmed by a 1-week screening wrist actigraphy at home. Those who had obstructive sleep apnea confirmed by laboratory polysomnography (apnea-hypopnea index >5), insomnia or history of any other sleep disorder, or night shift and rotating shift work (current or in the past 2 years) were excluded. Detailed eligibility criteria are provided in the eMethods in Supplement 2 .
After a 2-week habitual sleep period at baseline, participants were randomized to either 2-week sleep extension (sleep extension group) or 2-week continued habitual sleep (control group) ( Figure 1 ). Participants continued their daily routine activities at home without any prescribed diet or physical activity.
To blind participants to the sleep extension intervention, we described the study in the recruitment materials as follows: “we will collect information about sleep habits and metabolism.” The sleep extension group was blinded to randomization until after the 2-week baseline assessments, and the control group was blinded until the end of the 4-week study. This approach allowed us to capture habitual sleep-wake patterns without influencing participants' usual behavior or creating selection bias with only participants interested in improving sleep habits. After study completion, all participants were provided with information about the health benefits of optimal sleep duration. Block randomization, stratified by sex, was performed using computer-generated random numbers. Before the trial, randomization assignments were prepared by a biostatistician (K.W.) using opaque, sealed, and numbered envelopes and were given to the research coordinator (E.K.).
Sleep-wake patterns were continuously monitored at home by wrist actigraphy throughout the 4-week study. Participants were asked to wear an accelerometer (motion)-based monitor (Actiwatch Spectrum Plus; Philips) and to press a built-in event marker button when they went to bed to sleep each night and when they got out of bed each morning. Sleep was automatically scored (Actiware, version 6.0.9; Philips) using validated algorithms as the sum of all epochs that were scored as sleep during the total time spent in bed. 20 , 21
During the 2-week baseline, all participants were instructed to continue their habitual sleep patterns at home. On the morning of day 15, participants met with study investigators (E.T. and E.K.) in the research center. Those who were randomized to the sleep extension group received individualized sleep hygiene counseling through a structured interview (E.T.) (eMethods in Supplement 2 ). 22 At the end of the interview, participants were provided with individualized recommendations to follow at home for 2 weeks, with the aim of extending their bedtime duration to 8.5 hours. On day 22, participants returned for a brief follow-up visit. Actigraphy data from the first intervention week were reviewed, and further sleep counseling was provided as needed.
To minimize any imbalance in contact with the investigators between the 2 groups, we asked participants in the control group to meet with the study investigators on days 15 and 22. Actigraphy data of these participants were downloaded, but the participants did not receive any specific sleep recommendations and were instructed to continue their daily routine and habitual sleep behaviors until the end of the study.
For each 2-week period, the energy intake was calculated from the sum of total energy expenditure and change in body energy stores using the principle of energy balance. 14 , 23 , 24 Total energy expenditure was measured by the doubly labeled water method. 25 - 29 For each 2-week period, the change in body energy stores was computed from the regression (slope, grams per day) of daily home weights and change in body composition (ie, fat mass and fat-free mass) using dual-energy x-ray absorptiometry. Participants were provided a cellular-enabled weight scale (BodyTrace; BodyTrace Inc) and instructed to take their nude weights twice every morning after awakening before eating or drinking. Weight values were hidden from the participants to minimize potential influence on behavior. Changes in body composition were converted to changes in energy stores using 9.5 kcal/g as the energy coefficient of fat mass and 1.0 kcal/g as the energy coefficient of fat-free mass. 30 Resting metabolic rate was measured by indirect calorimetry for 30 minutes after fasting and for 4 hours after eating a standardized breakfast. Thermic effect of the meal was calculated, which was previously described elsewhere. 31 Activity energy expenditure was calculated by subtracting the resting metabolic rate and thermic effect of the meal from the total energy expenditure. 31 , 32 Additional details are provided in the eMethods in Supplement 2 .
The primary outcome was change in energy intake from baseline. A total final sample size of 80 participants (40 per group) was originally planned and provided 80% power to detect a true difference in energy intake between groups of 207 kcal/d using a 2-sided α = .05 significance threshold (trial protocol in Supplement 1 ). An intention-to-treat analysis was conducted in Stata, version 16 (StataCorp LLC) using 2-tailed tests with statistical significance set at P < .05. Categorical data are presented as counts and percentages. Continuous data are presented as means and SDs. Linear mixed-effects models were fit to determine the treatment differences between the groups. 33 Models included the randomization group, 2-week baseline period (period 1) vs 2-week intervention (period 2) and their interaction, and random effects for each participant. The treatment effect (95% CI) was estimated by the treatment group and period interaction, which is equivalent to testing the difference in change from baseline (period 2 minus period 1) in the sleep extension group vs the control group. To confirm the robustness of primary findings, we fit additional models using the analysis of covariance approach with the period 2 value as the dependent variable, treatment group as the independent variable, and period 1 value as covariates.
In secondary analyses, mixed models that adjusted for sex or menstrual cycle were also fit; these covariates were chosen because of the known influence of menstrual cycle on short-term changes in weight. A Pearson correlation coefficient was calculated to assess the relationships between the changes from baseline in sleep duration and the changes from baseline in energy intake. No adjustments were made to P values or CIs for multiple comparisons. Baseline characteristics of participants with complete data were compared with those of participants with incomplete data using unpaired, 2-tailed t tests and Fisher exact tests. No imputation for missing values was performed.
Of the 210 adults who provided consent and were assessed for eligibility, 81 were randomized (41 to the control group and 40 to the sleep extension group) initially ( Figure 1 ). One participant in the control group revealed adhering to a weight loss regimen and thus did not meet the study inclusion criteria and was deemed ineligible after randomization. 34 The 80 participants had a mean (SD) age of 29.8 (5.1) years and consisted of 41 men (51.3%) and 39 women (48.7%). Baseline characteristics of participants were similar between randomization groups ( Table 1 ). None of the participants were using any antihypertensive or lipid-lowering agents or any prescription medication that can affect sleep or metabolism.
Figure 2 illustrates the mean nightly sleep duration by actigraphy in each group throughout the 4-week study. Participants in the sleep extension group had a significant increase from baseline in mean sleep duration by actigraphy compared with those in the control group (1.2 hours; 95% CI, 1.0-1.4 hours; P < .001). The findings were similar with regard to change in sleep duration when only participants' workdays (1.3 hours; 95% CI, 1.0-1.5 hours; P < .001) or free days (1.1 hours; 95% CI, 0.7-1.5 hours; P < .001) were considered (eTable 1 in Supplement 2 ). No difference was found in change in sleep efficiency (percentage of time spent asleep during time in bed) between the 2 groups (–0.6 hours; 95% CI, –2.1 to 1.0 hours; P = .48), confirming the success of the intervention (eTable 2 in Supplement 2 ).
Energy intake was statistically significantly decreased in the sleep extension group compared with the control group (−270.4 kcal/d; 95% CI, −393.4 to −147.4 kcal/d; P < .001). Figure 3 A through D illustrates the changes from baseline in energy intake and the changes from baseline in sleep duration in individual participants. There was a significant increase in energy intake from baseline in the control group (114.9 kcal/d; 95% CI, 29.6 to 200.2 kcal/d) and a significant decrease in energy intake from baseline in the sleep extension group (−155.5 kcal/d; 95% CI, −244.1 to −66.9 kcal/d) ( Table 2 ). Considering all participants, the change in sleep duration was inversely correlated with the change in energy intake ( r = −0.41; 95% CI, −0.59 to −0.20; P < .001) ( Figure 3 E). Each 1-hour increase in sleep duration was associated with a decrease in energy intake of approximately 162 kcal/d (−162.3 kcal/d; 95% CI, −246.8 to −77.7 kcal/d; P < .001).
No statistically significant treatment effect was found in total energy expenditure or other measures of energy expenditure ( Table 2 ). Participants in the sleep extension group had a statistically significant reduction in weight compared with those in the control group (−0.87 kg; 95% CI, −1.39 to −0.35 kg; P = .001). There was weight gain from baseline in the control group (0.39 kg; 95% CI, 0.02 to 0.76 kg) and weight reduction from baseline in the sleep extension group (−0.48 kg; 95% CI, −0.85 to −0.11 kg) ( Table 2 ).
The findings on energy intake, energy expenditure, and weight were similar after adjustment for the effects of sex or menstrual cycle. No statistically significant differences in baseline characteristics were found between the 75 participants (93.8%) who had complete data on energy intake (primary outcome) vs participants with missing data on energy intake. The proportion of participants with complete data on energy intake was not significantly different between the sleep extension and control groups (90.0% vs 97.5%; P = .36). When all reported outcomes were considered, no significant differences (except for depressive symptoms) in baseline characteristics were found between participants with complete data and participants with incomplete or missing data (eTable 3 in Supplement 2 ). The proportion of participants with complete data on all reported outcomes was similar between the sleep extension and control groups (82.5% vs 85.0%; P > .99).
In this RCT of adults with overweight who habitually curtailed their sleep duration, sleep extension reduced energy intake and resulted in a negative energy balance (ie, energy intake that is less than energy expenditure) in real-life settings. To our knowledge, this study provides the first evidence of the beneficial effects of extending sleep to a healthy duration on objectively assessed energy intake and body weight in participants who continued to live in their home environment. Modest lifestyle changes in energy intake or expenditure are increasingly promoted as viable interventions to reverse obesity.
According to the Hall dynamic prediction model, a decrease in energy intake of approximately 270 kcal/d, which we observed after short-term sleep extension, would predict an approximately 12-kg weight loss over 3 years if the effects were sustained over a long term. 14 , 15 However, this study cannot infer how long healthy sleep habits may be sustained. Nevertheless, these modeling predictions on weight change suggest that continued adequate sleep duration and beneficial effect on energy intake could translate into clinically meaningful weight loss and help reverse or prevent obesity. Thus, the findings of this study may have important public health implications for weight management and policy recommendations.
The findings of decreased energy intake, negative energy balance, and weight reduction resulting from sleep extension are in agreement with the findings of short-term laboratory sleep-restriction studies showing increased energy intake and weight gain 17 as well as the findings of prospective epidemiologic studies linking sleep restriction to obesity risk. 8 A recent meta-analysis of randomized controlled laboratory studies found that short-term sleep restriction over 1 to 14 days of duration in healthy individuals was associated with increases of mean energy intake by approximately 253 kcal/d, as assessed during a single meal. 17 Another meta-analysis of prospective cohort studies found that the risk of obesity increased by 9% for each 1-hour decrease in sleep duration. 8 We did not observe a statistically significant change in total energy expenditure by doubly labeled water method or mean daytime activity counts by actigraphy (eTable 2 in Supplement 2 ). Although some laboratory sleep-restriction studies reported an increase in total energy expenditure of approximately 92 to 111 kcal/d, using a whole-room calorimeter, 35 , 36 other studies observed no change. 16 , 37 We found a modest reduction in weight after sleep extension, and the composition of weight change was primarily in fat-free mass, which is consistent with the short-term changes in body composition. 38 , 39 If sleep is extended over longer periods, weight loss in the form of fat mass would likely increase over time. A few observations suggest that sleeping 7 to 8 hours per night is associated with greater success in weight loss interventions. 40 - 43
In this RCT, we found an overall increase in objective sleep duration of approximately 1.2 hours in participants who habitually slept less than 6.5 hours per night. The change in sleep duration from baseline varied between participants and from night to night in the real-life setting. Overall, the sleep extension group compared with the control group had significantly higher subjective scores in obtaining sufficient sleep, with more daytime energy and alertness and better mood (eTable 4 in Supplement 2 ). Similar to a previous study of sleep extension, 22 the present RCT used an individualized counseling approach. Another study used bedtime extension in habitual short sleepers in real-life conditions but obtained variable benefits on sleep, likely because of a lack of an individualized approach or appropriate blinding. 44 None of these previous studies objectively measured energy intake.
Future similarly rigorous intervention studies of longer duration and using objective assessments of energy balance under real-life conditions are warranted to elucidate the underlying mechanisms and to investigate whether sleep extension could be an effective, scalable strategy for reversing obesity in diverse populations. Along with a healthy diet and regular physical activity, healthy sleep habits should be integrated into public messages to help reduce the risk of obesity and related comorbidities.
This study has several strengths. The major strengths are the randomized design and the objective tracking of energy intake and sleep in real-life settings. Most epidemiologic studies linking short sleep duration to body weight relied on self-reported dietary intake. 45 We did not collect self-reported dietary data because this method is subject to bias and has been shown to be inaccurate compared with the doubly labeled water method. 46 , 47 Most experimental studies that measured energy intake used a single meal under unnatural laboratory conditions. We used a validated method to objectively track energy intake by the doubly labeled water method and change in energy stores. 23 , 48 , 49 In this trial, we objectively quantified energy intake after sleep extension while individuals continued their daily routine in their usual environment. Participant blinding and use of actigraphy allowed us to capture true habitual sleep patterns at baseline. 22 , 50 In addition, we excluded insomnia and sleep apnea.
This study also has several limitations. We enrolled adults with overweight and used selective eligibility criteria, which may limit generalizability to more diverse populations. The increase in energy intake and weight from baseline that we observed in the control group may have contributed to the significant treatment effects. However, in RCTs, performing a between-group comparison, rather than separate tests against baseline within the groups, is strongly recommended. 51 The study did not provide information on how long healthy sleep habits could be maintained over longer periods. 44 We did not systematically assess the factors that may have influenced sleep behavior, but limiting the use of electronic devices appeared to be a key intervention among the participants (eTable 4 in Supplement 2 ). The doubly labeled water method has a precision of 5%, which may translate into some degree of uncertainty in the energy intake calculations. Although whole-room calorimeters can measure energy expenditure with a higher precision of approximately 1% to 2%, they do not represent real-life measurement and are not feasible over longer periods. We did not assess the underlying biological mechanisms of food frequency and the circadian timing of food intake. Multiple interrelated factors could contribute to the finding of decreased energy intake after sleep extension. 6 , 52 Evidence from laboratory sleep restriction studies suggests that increased hunger, alterations in appetite-regulating hormones, and changes in brain regions related to reward-seeking behavior are potential mechanisms that promote overeating after sleep restriction. 6 , 45
This RCT found that short-term sleep extension reduced objectively measured energy intake and resulted in a negative energy balance in real-life settings in adults with overweight who habitually curtailed their sleep duration. The findings highlighted the importance of improving and maintaining adequate sleep duration as a public health target for obesity prevention and increasing awareness about the benefits of adequate sleep duration for healthy weight maintenance.
Accepted for Publication: November 14, 2021.
Published Online: February 7, 2022. doi:10.1001/jamainternmed.2021.8098
Open Access: This is an open access article distributed under the terms of the CC-BY License . © 2022 Tasali E et al. JAMA Internal Medicine .
Corresponding Author: Esra Tasali, MD, Department of Medicine, The University of Chicago, 5841 S Maryland Ave, Chicago, IL 60637 ( [email protected] ).
Author Contributions: Author Dr Tasali and Ms Wroblewski had full access to all of the data in the study and take responsibility for the integrity of the data and the accuracy of the data analysis.
Concept and design: Tasali, Schoeller.
Acquisition, analysis, or interpretation of data: All authors.
Drafting of the manuscript: Tasali, Schoeller.
Critical revision of the manuscript for important intellectual content: All authors.
Statistical analysis: Tasali, Wroblewski.
Obtained funding: Tasali.
Administrative, technical, or material support: Tasali, Kahn, Kilkus, Schoeller.
Supervision: Tasali.
Other - research coordination duties: Kahn.
Conflict of Interest Disclosures: None reported.
Funding/Support: This study was funded by grants R01DK100426, CTSA-UL1 TR0002389, and UL1TR002389 from the National Institutes of Health and by the Diabetes Research and Training Center at The University of Chicago.
Role of the Funder/Sponsor: The funders had no role in the design and conduct of the study; collection, management, analysis, and interpretation of the data; preparation, review, or approval of the manuscript; and decision to submit the manuscript for publication.
Data Sharing Statement : See Supplement 3 .
Additional Contributions: Timothy Shriver, MS, University of Wisconsin–Madison, assisted with doubly labeled water measurements. Maureen Costello, MS, The University of Chicago, assisted with dual-energy x-ray absorptiometry scans. Becky Tucker, BA, Harry Whitmore, RPSGT, and Kristin Hoddy, PhD, RD, The University of Chicago, assisted with data collection. We thank the nurses, dieticians, and technicians at the Clinical Research Center at The University of Chicago for their expert assistance in data collection. We also thank the staff of the Sleep Research Center at The University of Chicago for their support. These individuals received no additional compensation, outside of their usual salary, for their contributions. We thank the volunteers for participating in this study.
- Register for email alerts with links to free full-text articles
- Access PDFs of free articles
- Manage your interests
- Save searches and receive search alerts
What is a Randomized Control Trial (RCT)?
Julia Simkus
Editor at Simply Psychology
BA (Hons) Psychology, Princeton University
Julia Simkus is a graduate of Princeton University with a Bachelor of Arts in Psychology. She is currently studying for a Master's Degree in Counseling for Mental Health and Wellness in September 2023. Julia's research has been published in peer reviewed journals.
Learn about our Editorial Process
Saul Mcleod, PhD
Editor-in-Chief for Simply Psychology
BSc (Hons) Psychology, MRes, PhD, University of Manchester
Saul Mcleod, PhD., is a qualified psychology teacher with over 18 years of experience in further and higher education. He has been published in peer-reviewed journals, including the Journal of Clinical Psychology.
Olivia Guy-Evans, MSc
Associate Editor for Simply Psychology
BSc (Hons) Psychology, MSc Psychology of Education
Olivia Guy-Evans is a writer and associate editor for Simply Psychology. She has previously worked in healthcare and educational sectors.
On This Page:
A randomized control trial (RCT) is a type of study design that involves randomly assigning participants to either an experimental group or a control group to measure the effectiveness of an intervention or treatment.
Randomized Controlled Trials (RCTs) are considered the “gold standard” in medical and health research due to their rigorous design.
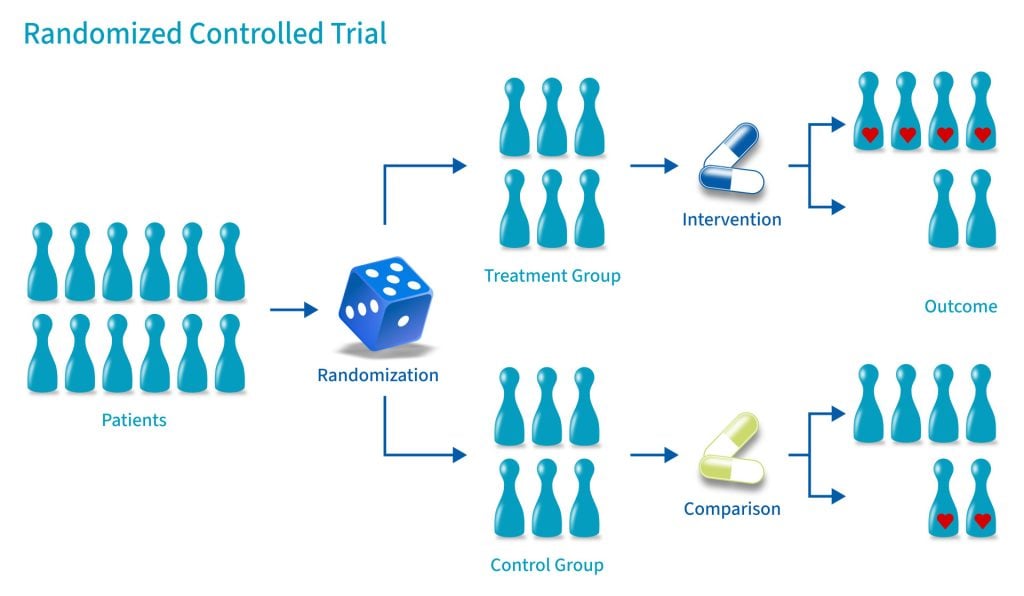
Control Group
A control group consists of participants who do not receive any treatment or intervention but a placebo or reference treatment. The control participants serve as a comparison group.
The control group is matched as closely as possible to the experimental group, including age, gender, social class, ethnicity, etc.
Because the participants are randomly assigned, the characteristics between the two groups should be balanced, enabling researchers to attribute any differences in outcome to the study intervention.
Since researchers can be confident that any differences between the control and treatment groups are due solely to the effects of the treatments, scientists view RCTs as the gold standard for clinical trials.
Random Allocation
Random allocation and random assignment are terms used interchangeably in the context of a randomized controlled trial (RCT).
Both refer to assigning participants to different groups in a study (such as a treatment group or a control group) in a way that is completely determined by chance.
The process of random assignment controls for confounding variables , ensuring differences between groups are due to chance alone.
Without randomization, researchers might consciously or subconsciously assign patients to a particular group for various reasons.
Several methods can be used for randomization in a Randomized Control Trial (RCT). Here are a few examples:
- Simple Randomization: This is the simplest method, like flipping a coin. Each participant has an equal chance of being assigned to any group. This can be achieved using random number tables, computerized random number generators, or drawing lots or envelopes.
- Block Randomization: In this method, participants are randomized within blocks, ensuring that each block has an equal number of participants in each group. This helps to balance the number of participants in each group at any given time during the study.
- Stratified Randomization: This method is used when researchers want to ensure that certain subgroups of participants are equally represented in each group. Participants are divided into strata, or subgroups, based on characteristics like age or disease severity, and then randomized within these strata.
- Cluster Randomization: In this method, groups of participants (like families or entire communities), rather than individuals, are randomized.
- Adaptive Randomization: In this method, the probability of being assigned to each group changes based on the participants already assigned to each group. For example, if more participants have been assigned to the control group, new participants will have a higher probability of being assigned to the experimental group.
Computer software can generate random numbers or sequences that can be used to assign participants to groups in a simple randomization process.
For more complex methods like block, stratified, or adaptive randomization, computer algorithms can be used to consider the additional parameters and ensure that participants are assigned to groups appropriately.
Using a computerized system can also help to maintain the integrity of the randomization process by preventing researchers from knowing in advance which group a participant will be assigned to (a principle known as allocation concealment). This can help to prevent selection bias and ensure the validity of the study results .
Allocation Concealment
Allocation concealment is a technique to ensure the random allocation process is truly random and unbiased.
RCTs use allocation concealment to decide which patients get the real medicine and which get a placebo (a fake medicine)
It involves keeping the sequence of group assignments (i.e., who gets assigned to the treatment group and who gets assigned to the control group next) hidden from the researchers before a participant has enrolled in the study.
This helps to prevent the researchers from consciously or unconsciously selecting certain participants for one group or the other based on their knowledge of which group is next in the sequence.
Allocation concealment ensures that the investigator does not know in advance which treatment the next person will get, thus maintaining the integrity of the randomization process.
Blinding (Masking)
Binding, or masking, refers to withholding information regarding the group assignments (who is in the treatment group and who is in the control group) from the participants, the researchers, or both during the study .
A blinded study prevents the participants from knowing about their treatment to avoid bias in the research. Any information that can influence the subjects is withheld until the completion of the research.
Blinding can be imposed on any participant in an experiment, including researchers, data collectors, evaluators, technicians, and data analysts.
Good blinding can eliminate experimental biases arising from the subjects’ expectations, observer bias, confirmation bias, researcher bias, observer’s effect on the participants, and other biases that may occur in a research test.
In a double-blind study , neither the participants nor the researchers know who is receiving the drug or the placebo. When a participant is enrolled, they are randomly assigned to one of the two groups. The medication they receive looks identical whether it’s the drug or the placebo.
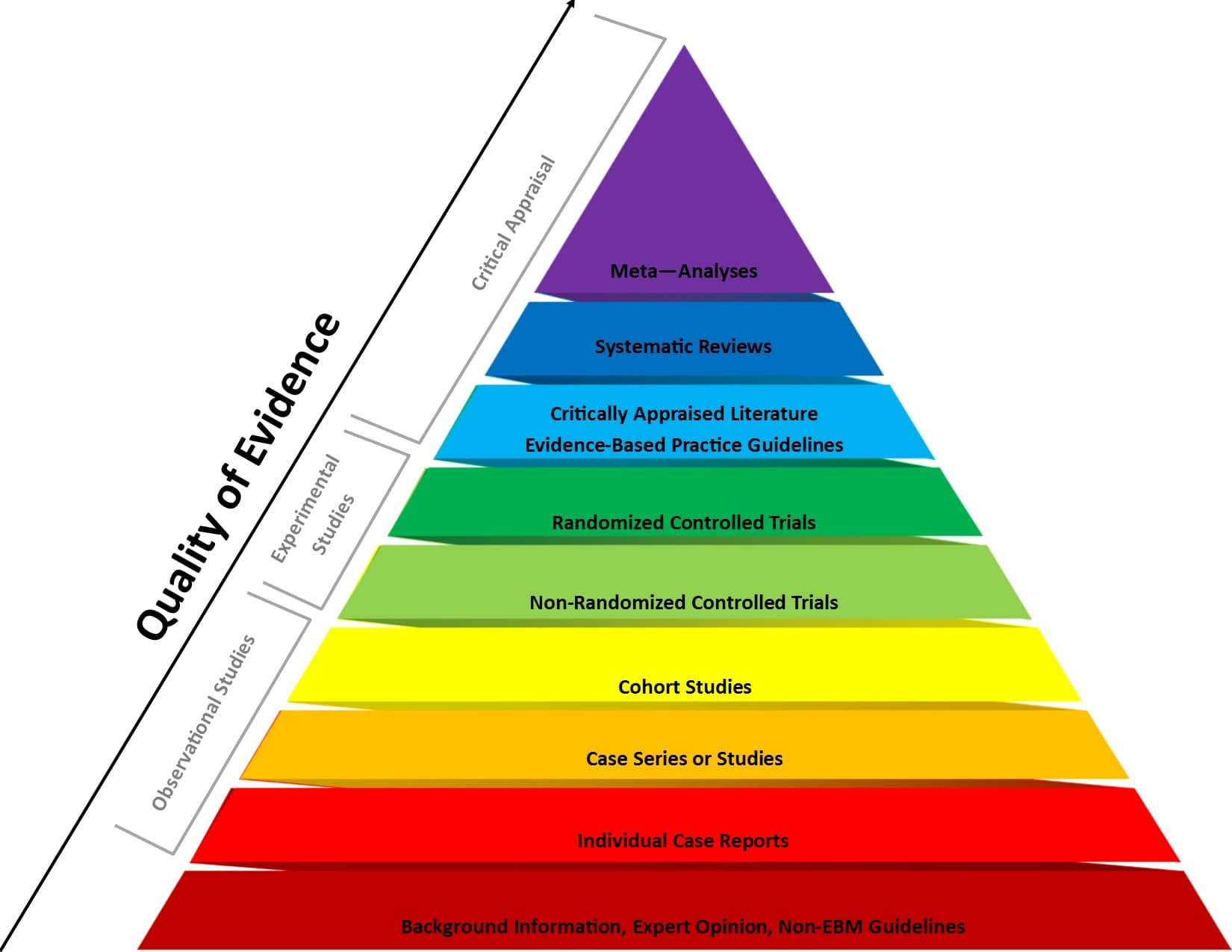
Figure 1 . Evidence-based medicine pyramid. The levels of evidence are appropriately represented by a pyramid as each level, from bottom to top, reflects the quality of research designs (increasing) and quantity (decreasing) of each study design in the body of published literature. For example, randomized control trials are higher quality and more labor intensive to conduct, so there is a lower quantity published.
Prevents bias
In randomized control trials, participants must be randomly assigned to either the intervention group or the control group, such that each individual has an equal chance of being placed in either group.
This is meant to prevent selection bias and allocation bias and achieve control over any confounding variables to provide an accurate comparison of the treatment being studied.
Because the distribution of characteristics of patients that could influence the outcome is randomly assigned between groups, any differences in outcome can be explained only by the treatment.
High statistical power
Because the participants are randomized and the characteristics between the two groups are balanced, researchers can assume that if there are significant differences in the primary outcome between the two groups, the differences are likely to be due to the intervention.
This warrants researchers to be confident that randomized control trials will have high statistical power compared to other types of study designs.
Since the focus of conducting a randomized control trial is eliminating bias, blinded RCTs can help minimize any unconscious information bias.
In a blinded RCT, the participants do not know which group they are assigned to or which intervention is received. This blinding procedure should also apply to researchers, health care professionals, assessors, and investigators when possible.
“Single-blind” refers to an RCT where participants do not know the details of the treatment, but the researchers do.
“ Double-blind ” refers to an RCT where both participants and data collectors are masked of the assigned treatment.
Limitations
Costly and timely.
Some interventions require years or even decades to evaluate, rendering them expensive and time-consuming.
It might take an extended period of time before researchers can identify a drug’s effects or discover significant results.
Requires large sample size
There must be enough participants in each group of a randomized control trial so researchers can detect any true differences or effects in outcomes between the groups.
Researchers cannot detect clinically important results if the sample size is too small.
Change in population over time
Because randomized control trials are longitudinal in nature, it is almost inevitable that some participants will not complete the study, whether due to death, migration, non-compliance, or loss of interest in the study.
This tendency is known as selective attrition and can threaten the statistical power of an experiment.
Randomized control trials are not always practical or ethical, and such limitations can prevent researchers from conducting their studies.
For example, a treatment could be too invasive, or administering a placebo instead of an actual drug during a trial for treating a serious illness could deny a participant’s normal course of treatment. Without ethical approval, a randomized control trial cannot proceed.
Fictitious Example
An example of an RCT would be a clinical trial comparing a drug’s effect or a new treatment on a select population.
The researchers would randomly assign participants to either the experimental group or the control group and compare the differences in outcomes between those who receive the drug or treatment and those who do not.
Real-life Examples
- Preventing illicit drug use in adolescents: Long-term follow-up data from a randomized control trial of a school population (Botvin et al., 2000).
- A prospective randomized control trial comparing medical and surgical treatment for early pregnancy failure (Demetroulis et al., 2001).
- A randomized control trial to evaluate a paging system for people with traumatic brain injury (Wilson et al., 2009).
- Prehabilitation versus Rehabilitation: A Randomized Control Trial in Patients Undergoing Colorectal Resection for Cancer (Gillis et al., 2014).
- A Randomized Control Trial of Right-Heart Catheterization in Critically Ill Patients (Guyatt, 1991).
- Berry, R. B., Kryger, M. H., & Massie, C. A. (2011). A novel nasal excitatory positive airway pressure (EPAP) device for the treatment of obstructive sleep apnea: A randomized controlled trial. Sleep , 34, 479–485.
- Gloy, V. L., Briel, M., Bhatt, D. L., Kashyap, S. R., Schauer, P. R., Mingrone, G., . . . Nordmann, A. J. (2013, October 22). Bariatric surgery versus non-surgical treatment for obesity: A systematic review and meta-analysis of randomized controlled trials. BMJ , 347.
- Streeton, C., & Whelan, G. (2001). Naltrexone, a relapse prevention maintenance treatment of alcohol dependence: A meta-analysis of randomized controlled trials. Alcohol and Alcoholism, 36 (6), 544–552.
How Should an RCT be Reported?
Reporting of a Randomized Controlled Trial (RCT) should be done in a clear, transparent, and comprehensive manner to allow readers to understand the design, conduct, analysis, and interpretation of the trial.
The Consolidated Standards of Reporting Trials ( CONSORT ) statement is a widely accepted guideline for reporting RCTs.
Further Information
- Cocks, K., & Torgerson, D. J. (2013). Sample size calculations for pilot randomized trials: a confidence interval approach. Journal of clinical epidemiology, 66(2), 197-201.
- Kendall, J. (2003). Designing a research project: randomised controlled trials and their principles. Emergency medicine journal: EMJ, 20(2), 164.
Akobeng, A.K., Understanding randomized controlled trials. Archives of Disease in Childhood , 2005; 90: 840-844.
Bell, C. C., Gibbons, R., & McKay, M. M. (2008). Building protective factors to offset sexually risky behaviors among black youths: a randomized control trial. Journal of the National Medical Association, 100 (8), 936-944.
Bhide, A., Shah, P. S., & Acharya, G. (2018). A simplified guide to randomized controlled trials. Acta obstetricia et gynecologica Scandinavica, 97 (4), 380-387.
Botvin, G. J., Griffin, K. W., Diaz, T., Scheier, L. M., Williams, C., & Epstein, J. A. (2000). Preventing illicit drug use in adolescents: Long-term follow-up data from a randomized control trial of a school population. Addictive Behaviors, 25 (5), 769-774.
Demetroulis, C., Saridogan, E., Kunde, D., & Naftalin, A. A. (2001). A prospective randomized control trial comparing medical and surgical treatment for early pregnancy failure. Human Reproduction, 16 (2), 365-369.
Gillis, C., Li, C., Lee, L., Awasthi, R., Augustin, B., Gamsa, A., … & Carli, F. (2014). Prehabilitation versus rehabilitation: a randomized control trial in patients undergoing colorectal resection for cancer. Anesthesiology, 121 (5), 937-947.
Globas, C., Becker, C., Cerny, J., Lam, J. M., Lindemann, U., Forrester, L. W., … & Luft, A. R. (2012). Chronic stroke survivors benefit from high-intensity aerobic treadmill exercise: a randomized control trial. Neurorehabilitation and Neural Repair, 26 (1), 85-95.
Guyatt, G. (1991). A randomized control trial of right-heart catheterization in critically ill patients. Journal of Intensive Care Medicine, 6 (2), 91-95.
MediLexicon International. (n.d.). Randomized controlled trials: Overview, benefits, and limitations. Medical News Today. Retrieved from https://www.medicalnewstoday.com/articles/280574#what-is-a-randomized-controlled-trial
Wilson, B. A., Emslie, H., Quirk, K., Evans, J., & Watson, P. (2005). A randomized control trial to evaluate a paging system for people with traumatic brain injury. Brain Injury, 19 (11), 891-894.

A multicenter randomized controlled trial comparing short- and medium-term outcomes of novel biologics and lightweight synthetic mesh for laparoscopic inguinal hernia repair
- Original Article
- Published: 20 June 2024
Cite this article
- P. Xue 1 na1 ,
- F. Yue 1 na1 ,
- S. Li 2 na1 ,
- W. Cheng 3 ,
- H. Zhou 3 ,
- Y. Zhou 3 ,
- J. Tang 2 ,
- J. Li 1 &
- J. Zhang 3
22 Accesses
Explore all metrics
Introduction
The use of biological graft in laparoscopic inguinal hernia repair (LIHR) has been controversial, and there is a lack of high-level evidence to confirm the value of biological graft in LIHR. The purpose of this study is to evaluate the effectiveness of a novel composite biologics in LIHR.
A multicenter, single-blinded, randomized controlled clinical trial was designed. Fifty patients with unilateral primary inguinal hernia were randomly assigned to the experimental and control group (1:1). The experimental group was repaired with a non-crosslinked composite extracellular matrix from porcine urinary bladder matrix and small intestinal submucosa (UBM/SIS). The control group was repaired with a lightweight, large-pore, synthetic mesh. The primary endpoint was the effectiveness rate of hernia repair.
The patients were followed up for four years. No significant difference was found between the experimental group and the control group in the effective rate of hernia repair (24/24[100%] vs 21/22[95.45%], RR, 0.4667; 95%CI, 0.3294–2.304; P = 0.4783). There was no fever, seroma, infection, groin pain, foreign body discomfort or recurrence in the experimental group during the follow-up. In the control group, there were 2 cases of seroma 14 days after operation, 1 case of groin discomfort 60 days after operation and one case of recurrence 410 days after surgery.
Compared with the lightweight synthetic mesh, the novel UBM/SIS graft has comparable short-term and medium-term effectiveness in LIHR, and the incidence of postoperative complications such as seroma groin discomfort is lower.
Trial registration Clinical Trials Registry: ChiCTR1800020173.
This is a preview of subscription content, log in via an institution to check access.
Access this article
Price includes VAT (Russian Federation)
Instant access to the full article PDF.
Rent this article via DeepDyve
Institutional subscriptions
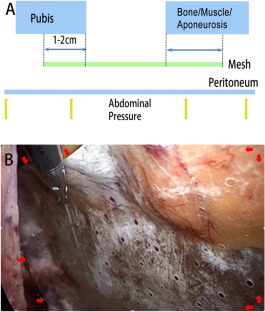
Similar content being viewed by others
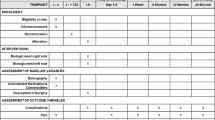
BIOLAP: biological versus synthetic mesh in laparo-endoscopic inguinal hernia repair: study protocol for a randomized, multicenter, self-controlled clinical trial
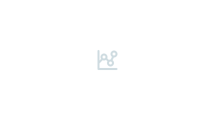
What is the evidence for the use of biologic or biosynthetic meshes in abdominal wall reconstruction?
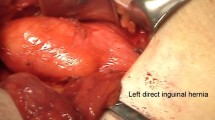
Pure tissue inguinal hernia repair with the use of biological mesh: a 10-year follows up. A prospective study
Data availability.
Available from the corresponding author on reasonable request.
Nahabedian MY, Sosin M, Bhanot P (2018) A current review of biologic meshes in abdominal wall reconstruction. Plast Reconstr Surg 142:74S-81S. https://doi.org/10.1097/PRS.0000000000004866
Article CAS PubMed Google Scholar
Fang Z, Ren F, Zhou J, Tian J (2015) Biologic mesh versus synthetic mesh in open inguinal hernia repair: system review and meta-analysis. ANZ J Surg 85:910–916. https://doi.org/10.1111/ans.13234
Article PubMed Google Scholar
van Hanswijck de Jonge P, Lloyd A, Horsfall L, Tan R, O'Dwyer PJ (2008) The measurement of chronic pain and health-related quality of life following inguinal hernia repair: a review of the literature. Hernia 12:561–569. https://doi.org/10.1007/s10029-008-0412-y
Trippoli S, Caccese E, Tulli G, Ipponi P, Marinai C, Messori A (2018) Biological meshes for abdominal hernia: lack of evidence-based recommendations for clinical use. Int J Surg 52:278–284. https://doi.org/10.1016/j.ijsu.2018.02.046
Clapp ML, Hicks SC, Awad SS, Liang MK (2013) Trans-cutaneous closure of central defects (TCCD) in laparoscopic ventral hernia repairs (LVHR). World J Surg 37:42–51. https://doi.org/10.1007/s00268-012-1810-y
Cobb WS, Burns JM, Kercher KW, Matthews BD, James Norton H, Todd Heniford B (2005) Normal intraabdominal pressure in healthy adults. J Surg Res 129:231–235. https://doi.org/10.1016/j.jss.2005.06.015
Klinge U, Klosterhalfen B, Conze J, Limberg W, Obolenski B, Ottinger AP, Schumpelick V (1998) Modified mesh for hernia repair that is adapted to the physiology of the abdominal wall. Eur J Surg 164:951–960. https://doi.org/10.1080/110241598750005138
Bittner R, Arregui ME, Bisgaard T, Dudai M, Ferzli GS, Fitzgibbons RJ, Fortelny RH, Klinge U, Kockerling F, Kuhry E, Kukleta J, Lomanto D, Misra MC, Montgomery A, Morales-Conde S, Reinpold W, Rosenberg J, Sauerland S, Schug-Pass C, Singh K, Timoney M, Weyhe D, Chowbey P (2011) Guidelines for laparoscopic (TAPP) and endoscopic (TEP) treatment of inguinal hernia [international endohernia society (IEHS)]. Surg Endosc 25:2773–2843. https://doi.org/10.1007/s00464-011-1799-6
Article CAS PubMed Central PubMed Google Scholar
Carver DA, Kirkpatrick AW, Eberle TL, Ball CG (2019) Performance of biological mesh materials in abdominal wall reconstruction: study protocol for a randomised controlled trial. BMJ Open 9:e024091. https://doi.org/10.1136/bmjopen-2018-024091
Article PubMed Central PubMed Google Scholar
Ravo B, Falasco G (2020) Pure tissue inguinal hernia repair with the use of biological mesh: a 10-year follows up: A prospective study. Hernia 24:121–126. https://doi.org/10.1007/s10029-019-01976-y
Li B, Zhang X, Man Y, Xie J, Hu W, Huang H, Wang Y, Ma H (2021) Lichtenstein inguinal hernia repairs with porcine small intestine submucosa: a 5- year follow-up. A prospective randomized controlled study. Regen Biomater 8(1):rbaa055. https://doi.org/10.1093/rb/rbaa055
Ho CH, Liao PW, Yang SS, Jaw FS, Tsai YC (2015) The use of porcine small intestine submucosa implants might be associated with a high recurrence rate following laparoscopic herniorrhaphy. J Formos Med Assoc 114:216–220. https://doi.org/10.1016/j.jfma.2013.03.0071
Vrijland WW, van den Tol MP, Luijendijk RW, Hop WC, Busschbach JJ, de Lange DC, van Geldere D, Rottier AB, Vegt PA, IJzermans JN, Jeekel J (2002) Randomized clinical trial of non-mesh versus mesh repair of primary inguinal hernia. Br J Surg 89:293–297. https://doi.org/10.1046/j.0007-1323.2001.02030.x
Wang Y, Zhang K, Yang J, Yao Y, Guan Y, Cheng W, Zhang J, Han J (2023) Outcome of a novel porcine-derived UBM/SIS composite biological mesh in a rabbit vaginal defect model. Int Urogynecol J 34(7):1501–1511. https://doi.org/10.1007/s00192-022-05400-5
Nie X, Xiao D, Wang W, Song Z, Yang Z, Chen Y, Gu Y (2015) Comparison of porcine small intestinal submucosa versus polypropylene in open inguinal hernia repair: a systematic review and meta-analysis. PLoS ONE 10(8):e0135073. https://doi.org/10.1371/journal.pone.0135073
Ng TP, Loo BYK, Chia CLK (2023) Seroma-prevention strategies in minimally invasive inguinal hernia repair: a systematic review and meta-analysis. Int J Abdom Wall Hernia Surg 6(1):14–22. https://doi.org/10.4103/ijawhs.ijawhs_5_23
Article Google Scholar
Gupta A, Zahriya K, Mullens PL, Salmassi S, Keshishian A (2006) Ventral herniorrhaphy: experience with two different biosynthetic mesh materials, Surgisis and Alloderm. Hernia 10:419–425. https://doi.org/10.1007/s10029-006-0130-2
Brown BN, Londono R, Tottey S, Zhang L, Kukla KA, Wolf MT, Daly KA, Reing JE, Badylak SF (2012) Macrophage phenotype as a predictor of constructive remodeling following the implantation of biologically derived surgical mesh materials. Acta Biomater 8(3):978–987. https://doi.org/10.1016/j.actbio.2011.11.031
Jacobs HN, Rathod S, Wolf MT, Elisseeff JH (2017) Intra-articular injection of urinary bladder matrix reduces osteoarthritis development. AAPS J 19(1):141–149. https://doi.org/10.1208/s12248-016-9999-6
Sadtler K, Sommerfeld SD, Wolf MT, Wang X, Majumdar S, Chung L, Kelkar DS, Pandey A, Elisseeff JH (2017) Proteomic composition and immunomodulatory properties of urinary bladder matrix scaffolds in homeostasis and injury. Semin Immunol 29:14–23. https://doi.org/10.1016/j.smim.2017.05.002
Haladu N, Alabi A, Brazzelli M, Imamura M, Ahmed I, Ramsay G, Scott NW (2022) Open versus laparoscopic repair of inguinal hernia: an overview of systematic reviews of randomised controlled trials. Surg Endosc 36(7):4685–4700. https://doi.org/10.1007/s00464-022-09161-6
Download references
Funding was provided by National Defense Science and Technology Excellent Youth Science Fund, 2019-JCJQ-ZQ-002, Jian Zhang, National Defense Science and Technology Foundation Strengthening Plan, 2019-JCJQ-JQ-069, Jian Zhang
Author information
P. Xue, F. Yue and S. Li equal contribution as first authors.
Authors and Affiliations
Department of Surgery, Ruijin Hospital, Shanghai Jiaotong University School of Medicine, 197 Ruijin Road (No.2), Shanghai, 200025, China
P. Xue, F. Yue & J. Li
Department of Surgery, Huadong Hospital, Fudan University, Shanghai, China
S. Li & J. Tang
Department of Colorectal Surgery, Shanghai Changzheng Hospital, Naval Medical University, 415 Fengyang Road, Shanghai, 200003, China
W. Cheng, H. Zhou, W. Yan, Y. Zhou & J. Zhang
You can also search for this author in PubMed Google Scholar
Corresponding authors
Correspondence to J. Li or J. Zhang .
Ethics declarations
Conflict of interest.
The authors declare no conflict of interest or financial ties.
Ethical approval
The study was approved by Ruijin Hospital Ehics Committee (2019/62), Huadong Hospital Ehics Committee (20180037) and Changzheng Hospital Ehics Committee (2018-10).
Informed consent
All individuals who took part in the study gave their informed consent.
Human and animal rights
This article does not contain any studies with animals performed by any of the authors.
Additional information
Publisher's note.
Springer Nature remains neutral with regard to jurisdictional claims in published maps and institutional affiliations.
Rights and permissions
Springer Nature or its licensor (e.g. a society or other partner) holds exclusive rights to this article under a publishing agreement with the author(s) or other rightsholder(s); author self-archiving of the accepted manuscript version of this article is solely governed by the terms of such publishing agreement and applicable law.
Reprints and permissions
About this article
Xue, P., Yue, F., Li, S. et al. A multicenter randomized controlled trial comparing short- and medium-term outcomes of novel biologics and lightweight synthetic mesh for laparoscopic inguinal hernia repair. Hernia (2024). https://doi.org/10.1007/s10029-024-03046-4
Download citation
Received : 07 February 2024
Accepted : 13 April 2024
Published : 20 June 2024
DOI : https://doi.org/10.1007/s10029-024-03046-4
Share this article
Anyone you share the following link with will be able to read this content:
Sorry, a shareable link is not currently available for this article.
Provided by the Springer Nature SharedIt content-sharing initiative
- Laparoscopic inguinal hernia repair
- UBM/SIS composite graft
- Find a journal
- Publish with us
- Track your research
- Open access
- Published: 17 June 2024
The effects of simulation-based education on undergraduate nursing students' competences: a multicenter randomized controlled trial
- Lai Kun Tong 1 ,
- Yue Yi Li 1 ,
- Mio Leng Au 1 ,
- Wai I. Ng 1 ,
- Si Chen Wang 1 ,
- Yongbing Liu 2 ,
- Yi Shen 3 ,
- Liqiang Zhong 4 &
- Xichenhui Qiu 5
BMC Nursing volume 23 , Article number: 400 ( 2024 ) Cite this article
191 Accesses
Metrics details
Education in nursing has noticed a positive effect of simulation-based education. There are many studies available on the effects of simulation-based education, but most of those involve a single institution, nonrandomized controlled trials, small sample sizes and subjective evaluations of the effects. The purpose of this multicenter randomized controlled trial was to evaluate the effects of high-fidelity simulation, computer-based simulation, high-fidelity simulation combined with computer-based simulation, and case study on undergraduate nursing students.
A total of 270 nursing students were recruited from five universities in China. Participants were randomly divided into four groups at each institution: the high-fidelity simulation group, the computer-based simulation group, the high-fidelity simulation combined with computer-based simulation group, and the case study group. Finally, 239 participants completed the intervention and evaluation, with 58, 67, 57, and 57 participants in each group. The data were collected at three stages: before the intervention, immediately after the intervention, and three months after the intervention.
The demographic data and baseline evaluation indices did not significantly differ among the four groups. A statistically significant difference was not observed between the four methods for improving knowledge, interprofessional collaboration, critical thinking, caring, or interest in learning. While skill improvement differed significantly among the different groups after the intervention ( p = 0.020), after three months, no difference was observed ( p = 0.139). The improvement in skill in the computer-based simulation group was significantly lower at the end of the intervention than that in the high-fidelity simulation group ( p = 0.048) or the high-fidelity simulation combined with computer-based simulation group ( p = 0.020).
Conclusions
Nursing students benefit equally from four methods in cultivating their knowledge, interprofessional collaboration, critical thinking, caring, and interest in learning both immediately and over time. High-fidelity simulation and high-fidelity simulation combined with computer-based simulation improve skill more effectively than computer-based simulation in the short term. Nursing educators can select the most suitable teaching method to achieve the intended learning outcomes depending on the specific circumstances.
Trial registration
This clinical trial was registered at the Chinese Clinical Trial Registry (clinical trial number: ChiCTR2400084880, date of the registration: 27/05/2024).
Peer Review reports
Introduction
There are many challenges nursing students face in the clinical setting because of the gap between theory and practice, the lack of resources, and unfamiliarity with the medical environment [ 1 ]. Nursing education needs an innovative teaching method that is more closely related to the clinical environment. Simulation-based education is an effective teaching method for nursing students [ 2 ]. It provides students with an immersive clinical environment for practicing skills and gaining experience in a safe, controlled setting [ 3 ]. This educational approach not only supports the development of various competencies [ 2 , 4 ], including knowledge, skill, interprofessional collaboration, critical thinking, caring, and interest in learning, but also enables students to apply learned concepts to complex and challenging situations [ 5 ].
Manikin-based and computer-based simulations are commonly employed simulators in nursing education. Manikin-based simulation involves the use of a manikin to mimic a patient’s characteristics, such as heart and lung sounds [ 6 ]. Computer-based simulation involves the modeling of real-life processes solely using computers, usually with a keyboard and monitor as inputs and outputs [ 6 ]. According to a recent meta-analysis, manikin-based simulation improves nursing students' knowledge acquisition more than computer-based simulation does, but there are no significant differences in confidence or satisfaction with learning [ 4 ].
Based on the level of fidelity, manikin-based simulation can be categorized as low, medium, or high fidelity [ 7 ]. High-fidelity simulation has become increasingly popular since it replaces part of clinical placement without compromising nursing student quality [ 8 ]. Compared to other teaching methods, high-fidelity simulation is associated with elevated equipment and labor costs [ 9 ]. To enhance cost-effectiveness, it is imperative to maximize the impact of high-fidelity simulation. To improve learning outcomes, mixed learning has gained popularity across higher education in recent years [ 10 ]. The most widely used mixed learning method for simulation education in the nursing field is high-fidelity simulation combined with computer-based simulation. There have been only a few studies on the effect of high-fidelity simulation combined with computer-based simulation on nursing students, and these are either pre-post comparison studies without control groups [ 11 ] or quasi-experimental studies without randomization [ 12 ]. To obtain a better grasp of the effects of combining high-fidelity simulation and computer-based simulation, a randomized controlled trial is needed.
In addition to enhancing effectiveness, optimizing cost-effectiveness can be achieved by implementing cost reduction measures. Case study, which eliminates the need for additional equipment, offers a relatively low-cost alternative. A traditional case study provides all pertinent information, whereas an unfolding case study purposefully leaves out information [ 13 ]. It has been shown that unfolding case study fosters critical thinking in students more effectively than traditional case studies [ 14 ]. Despite being regarded as an innovative and inexpensive teaching method, there is little research comparing unfolding case study with other simulation-based teaching methods. To address this knowledge gap, further study is necessary.
An umbrella review highlights that the existing literature on the learning outcomes of simulation-based education predominantly emphasizes knowledge and skills, while conferring limited focus on other core competencies, such as interprofessional collaboration and caring [ 15 ]. Therefore, future research should evaluate various learning outcome indicators.
This multicenter randomized controlled trial aimed to assess the effectiveness of high-fidelity simulation, computer-based simulation, high-fidelity simulation combined with computer-based simulation, and case study on nursing students’ knowledge, skill, interprofessional collaboration, critical thinking, caring, and interest in learning.
Study design
A multicenter randomized controlled trial was conducted between March 2022 and May 2023 in China. The study conforms to the CONSORT guidelines. This clinical trial was registered at the Chinese Clinical Trial Registry (clinical trial number: ChiCTR2400084880, date of the registration: 27/05/2024).
Participants and setting
Participants were recruited from five universities in China, two of which were private and three of which were public. Among the five universities, four were equipped with two high-fidelity simulation laboratories. Specifically, three universities had laboratories simulating intensive care unit wards and delivery rooms, while the remaining university had two laboratories simulating general wards. Additionally, one university possessed a high-fidelity simulation laboratory specifically designed to simulate a general ward setting. Three universities utilized Laerdal patient simulators in their laboratories, while the other two universities employed Gaumard patient simulators.
A recruitment poster with the time and location of the project promotion was posted on the school bulletin board. The research team provided a briefing to students at the designated time and location indicated on the poster, affording them the opportunity to inquire about and enhance their understanding of the project.
The study mandated that participants fulfill the following criteria: 1) enroll in a nursing undergraduate program; 2) have full-time student status; 3) complete courses in Anatomy and Physiology, Pathophysiology, Pharmacology, Health Assessment, Basic Nursing, and Medical and Surgical Nursing (Respiratory System); 4) have proficiency in reading and writing Chinese; and 5) participate voluntarily. Those who met the following criteria were excluded: 1) had a degree or diploma and 2) took the course again.
The sample size was calculated through the use of G*Power 3.1, which was based on F tests (ANOVA: Repeated measures, between factors). Several assumptions were taken into consideration, including a 5% level of significance, 80% power, four groups, three measurements, and a 0.50 correlation between pre- and postintervention time points. Compared to other teaching methods, high-fidelity simulation exhibited a medium effect size (d = 0.49 for knowledge, d = 0.50 for performance) [ 16 ]. The calculation employed a conservative approach, accommodating a small yet clinically significant effect size (0.25), thereby bolstering the reliability and validity of the findings. Based on these assumptions, the total sample size required was determined to be 124, with each group requiring 31 participants.
Randomization and blinding
Due to inconsistent teaching schedules at the five universities involved in the study, the participants were divided into four groups at each institution: the high-fidelity simulation group, the computer-based simulation group, the high-fidelity simulation combined with computer-based simulation group, and the case study group. Participant grouping was carried out by study team members who were not involved in the intervention or evaluation. The participants were each assigned a random nonduplicate number between zero and 100 using Microsoft Excel. The random numbers/participants were divided into four groups based on quartiles: the lower quarter, the lower quarter to a half, the half to three-fourths, and the upper quarter were assigned to the high-fidelity simulation group, the computer-based simulation group, the high-fidelity simulation combined with computer-based simulation group, and the case study group, respectively. It was not possible to implement participant blinding because the four teaching methods differed significantly, while effect evaluation and data analysis were conducted in a blinded manner. Each participant was assigned a unique identifier to maintain anonymity throughout the study.
Baseline test
Baseline testing started after participant recruitment had ended, so the timing of the study varied between universities. The baseline test items were the same for all participants and included general characteristics, knowledge, skills, interprofessional collaboration, critical thinking, caring, and interest in learning. The evaluation of skills was conducted by trained assessors, whereas a non-face-to-face online survey was utilized for the assessment of others.
Intervention
The four groups were taught with three scenarios covering the three different cases, in the following order: asthma worsening, drug allergy, and ventricular fibrillation. These three cases represent commonly encountered scenarios necessitating emergency treatment. It is anticipated that by means of training, students can enhance their aptitude to effectively handle emergency situations within clinical settings. It is vital that the case used in simulation-based education is valid so that its effectiveness can be enhanced [ 17 ]. The cases used in this study were from vSim® for Nursing | Lippincott Nursing Education, which was developed by Wolters Kluwer Health (Lippincott), Laerdal Medical, and the National League for Nursing. Hence, the validity of the cases can be assured. Participants received all the materials, including learning outcomes, theoretical learning materials, and case materials (medical history and nursing document), at least one day before teaching. All the teachers in charge of teaching participated in the meeting to discuss the lesson plans to reach a consensus on the lesson plans. The lesson plans were written by three members of the research team and revised according to the feedback. Table 1 shows the teaching experience of each case in the different intervention groups. The instructors involved had at least five years of teaching experience and a master's degree or higher.
Posttest and follow-up test
The posttest was conducted within one week of the intervention using the same items as those used in the baseline test. The follow-up test was administered after three months of the intervention.
General characteristics
The general characteristics of the participants included gender, age, and previous semester grade.
This was measured by five multiple-choice items developed for this study. The items were derived from the National Nurse Licensing Examination [ 18 ]. The maximum score was five, with one awarded for each correct answer. The questionnaire exhibited high content validity (CVI = 1.00) and good reliability (Kuder-Richardson 20 = 0.746).
The Creighton Competency Evaluation Instrument (CCEI) is designed to assess clinical skills in a simulated environment by measuring 23 general nursing behaviors. This tool was originally developed by Todd et al. [ 19 ] and subsequently modified by Hayden et al. [ 20 ]. The Chinese version of the CCEI has good reliability (Cronbach’s α = 0.94) and validity (CVI = 0.98) [ 21 ]. The CCEI was scored by nurses with master’s degrees who were trained by the research team and blinded to the intervention information. A dedicated person was assigned to handle the rating for each university, and the raters did not rotate among the participants. The Kendall's W coefficient for the raters' measures was calculated to be 0.832, indicating a high level of interrater agreement and reliability. All participants were tested using a high-fidelity simulator, with each test lasting ten minutes. The skills test without debriefing employed a single-person format, and the nursing procedures did not rely on laboratory results, so the items "Delegates Appropriately," "Reflects on Clinical Experience," "Interprets Lab Results," and "Reflects on Potential Hazards and Errors" were excluded from the assessment. The total score ranged from 0–19 and a higher score indicated a higher level of skill.
- Interprofessional collaboration
The Assessment of the Interprofessional Team Collaboration Scale for Students (AITCS-II Student) was used to assess interprofessional collaboration. It consists of 17 items rated on a 5-point Likert scale (1 = never, 5 = always), for a total score ranging from 17 to 85 [ 22 ]. The Chinese version of the AITCS-II has good reliability (Cronbach’s α = 0.961) and validity [ 23 ].
- Critical thinking
Critical thinking was measured by Yoon's Critical Thinking Disposition Scale (YCTD). It is a five-point Likert scale with values ranging from 1 to 5, resulting in a total score ranging from 27 to 135 [ 24 ]. Higher scores on this scale indicate greater critical thinking ability. The YCTD has good reliability (Cronbach’s α = 0.948) and validity when applied to Chinese nursing students [ 25 ].
Caring was assessed using the Caring Dimensions Inventory (CDI), which employs a five-point Likert scale ranging from 25 to 125 [ 26 ]. Higher scores on the CDI indicate a greater level of caring. The Chinese version of the CDI exhibited good reliability (Cronbach’s α = 0.97) and validity [ 27 ].
- Interest in learning
The Study Interest Questionnaire (SIQ) was used to assess interest in learning. The SIQ is a four-point Likert scale ranging from 18 to 72, where a higher total score indicates a greater degree of interest in the field of study [ 28 ]. The SIQ has good reliability (Cronbach’s α = 0.90) and validity when applied to Chinese nursing students [ 29 ].
Ethical considerations
The institution of the first author granted ethical approval (ethical approval number: REC-2021.801). Written informed consent was obtained from all participants. Participants were permitted to withdraw for any reason at any time without penalty. Guidelines emphasizing safety measures and precautions during the intervention were provided to participants, and study coordinators closely monitored laboratory and simulation sessions to address concerns or potential harm promptly.
Data analysis
Descriptive statistics were used to describe the participant characteristics and baseline characteristics. Continuous variables are presented as the mean and standard deviation, while categorical variables are presented as frequencies and percentages. According to the Quantile–Quantile Plot, the data exhibited an approximately normal distribution. Furthermore, Levene's test indicated equal variances for the variables of knowledge, skill, interprofessional collaboration, critical thinking, caring, and interest in learning, with p-values of 0.171, 0.249, 0.986, 0.634, 0.992, and 0.407, respectively. The baseline characteristics of the four groups were compared using one-way analysis of variance. The indicators of knowledge, skill, interprofessional collaboration, critical thinking, caring, and interest in learning were assessed at baseline, immediately after the intervention, and three months postintervention. Changes in these indicators from baseline were calculated for both the postintervention and three-month follow-up periods. The changes among the four groups were compared using one-way analysis of variance. Cohen's d effect sizes were computed for the between-group comparisons (small effect size = 0.2; medium effect size = 0.5; large effect size = 0.8). Missing data were treated as missing without imputation. The data analysis was conducted using jamovi 2.3.28 ( https://www.jamovi.org/ ). Jamovi was developed on the foundation of the R programming language, and is recognized for its user-friendly interface. The threshold for statistical significance was established at a two-sided p < 0.05.
Participants
A total of 270 participants were initially recruited from five universities for this study. However, an attrition rate of 11.5% was observed, resulting in 31 participants discontinuing their involvement. Consequently, the final analysis included data from 239 participants who successfully completed the intervention and remained in the study. Specifically, there were 58 participants in the high-fidelity simulation group, 67 in the computer-based simulation group, 57 in the high-fidelity simulation combined with computer-based simulation group, and 57 in the case study group (Fig. 1 ). The participant demographics and baseline characteristics are displayed in Table 2 , and no significant differences were observed in these variables.
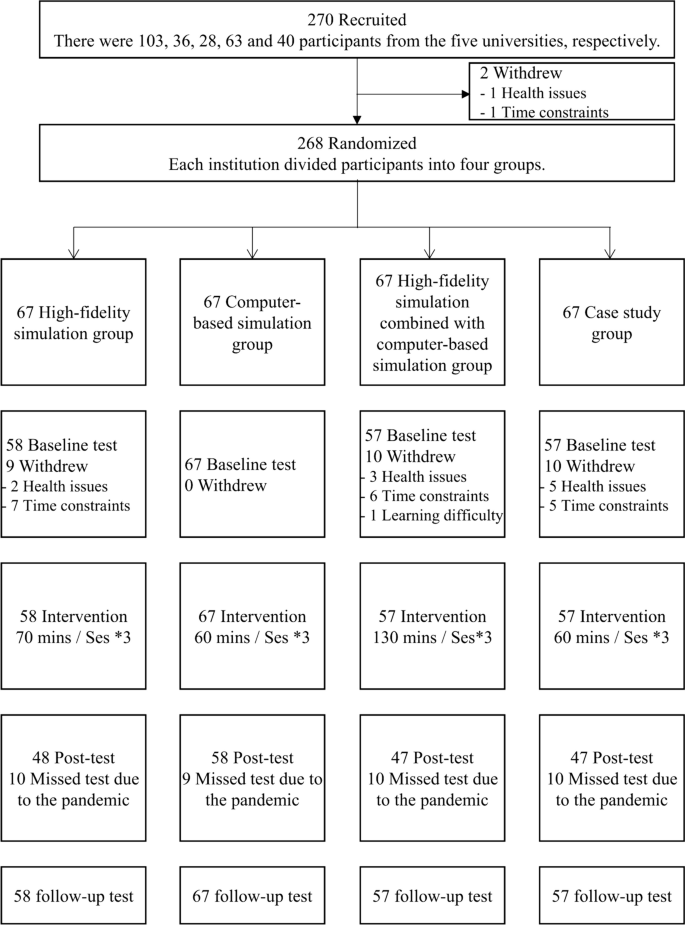
Study subject disposition flow chart
Efficacy outcomes
All the intervention groups showed improvements in knowledge after the intervention, with the high-fidelity simulation group showing the greatest improvement (Fig. 2 ). However, there were no significant differences in knowledge improvement among the groups (p = 0.856). The computer-based simulation group and case study group experienced a decrease in knowledge compared to baseline three months after the intervention, while the other groups showed an increase in knowledge. The high-fidelity simulation combined with computer-based simulation group performed best (Fig. 3 ), but no significant differences were observed (p = 0.872). The effect sizes between groups were found to be small, both immediately after the intervention and at the three-month follow-up (Table 3 ).
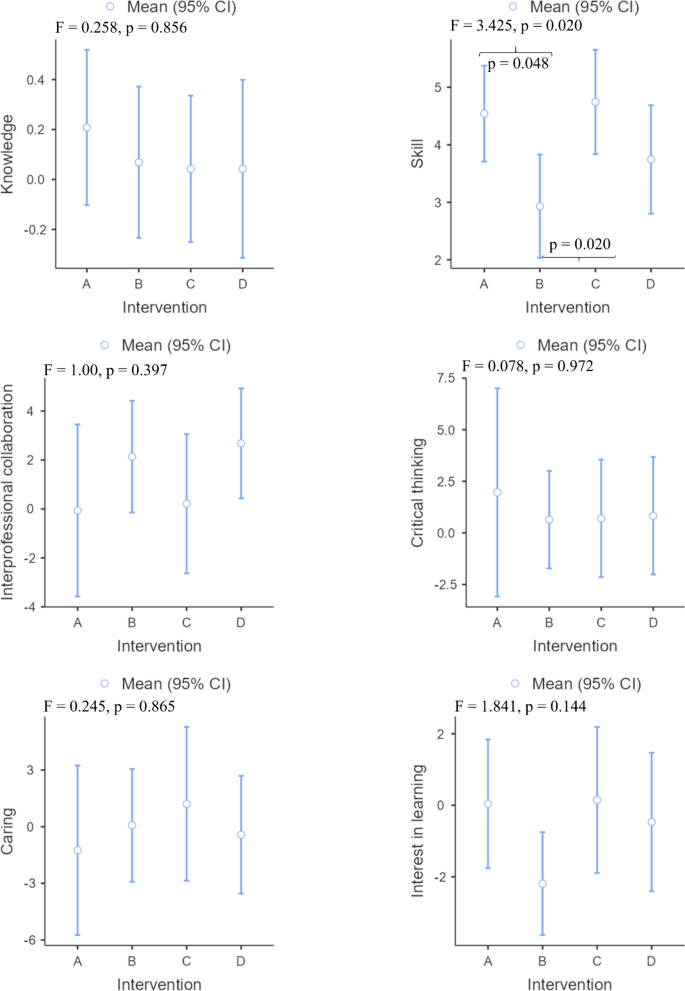
Changes in all effectiveness outcomes at post intervention. Note: A High-fidelity simulation group; B Computer-based simulation group; C High-fidelity simulation combined with computer-based simulation group; D Case study group
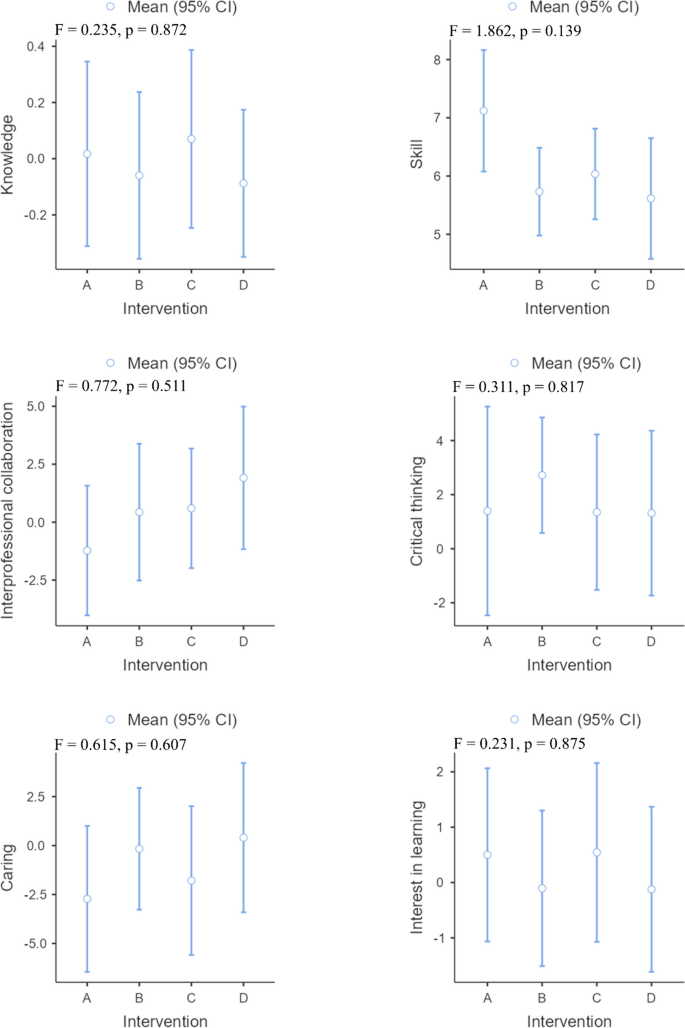
Changes in all effectiveness outcomes at three months of intervention. Note: A High-fidelity simulation group; B Computer-based simulation group; C High-fidelity simulation combined with computer-based simulation group; D Case study group
The different intervention groups showed improvements in skills after the intervention and three months after the intervention. The high-fidelity simulation combined with computer-based simulation group showed the greatest improvement after the intervention (Fig. 2 ), while the greatest improvement was observed in the high-fidelity simulation group three months after the intervention (Fig. 3 ). There was a significant difference in the improvement in skills among the different groups after the intervention ( p = 0.020). Specifically, the improvement observed in the computer-based simulation group was significantly lower than that in both the high-fidelity simulation group ( p = 0.048) and the high-fidelity simulation combined with computer-based simulation group ( p = 0.020). However, three months after the intervention, there was no statistically significant difference in skill improvement among the groups ( p = 0.139). Except for the between-group effect sizes of the high-fidelity simulation group compared to the computer-based simulation group (Cohen d = 0.51) and the computer-based simulation group compared to the high-fidelity simulation combined with computer-based simulation group (Cohen d = 0.56), the effects were found to be medium after the intervention, while the other between-group effect sizes were small both after the intervention and three months after the intervention (Table 3 ).
In all intervention groups except for the high-fidelity simulation group, interprofessional collaboration improved after the intervention and three months after the intervention, with the case study group (Figs. 2 and 3 ) demonstrating the greatest improvement. No significant difference was found between the intervention groups after or three months after the intervention in terms of changes in interprofessional collaboration. Both immediately following the intervention and three months later, the effect sizes between groups were small (Table 3 ).
After the intervention and three months after the intervention, the critical thinking of all the intervention groups improved. Among them, the high-fidelity simulation group improved the most after the intervention (Fig. 2 ), while the computer-based simulation group improved the most three months after the intervention (Fig. 3 ). However, no statistically significant differences were observed in the improvement of critical thinking across the different groups. The between-group effect sizes of each group were small both after the intervention and three months after the intervention (Table 3 ).
Caring improved following the intervention in all intervention groups, with the exception of the high-fidelity simulation group and case study group (Fig. 2 ). However, no significant difference was observed between the intervention groups in terms of changes ( p = 0.865). A decrease in caring was observed three months after the intervention in all intervention groups, except for the case study group (Fig. 3 ). Nevertheless, no statistically significant difference was detected between the intervention groups in terms of changes (p = 0.607). Both immediately following the intervention and three months later, the effect sizes between groups were small (Table 3 ).
In terms of interest in learning, both the high-fidelity simulation group and the high-fidelity simulation combined with computer-based simulation group improved after the intervention or three months later. Among the groups, the high-fidelity simulation combined with computer-based simulation group improved the most after both the intervention and three months after the intervention (Figs. 2 and 3 ). However, no statistically significant difference was detected between the intervention groups in terms of changes either after the intervention (p = 0.144) or three months after the intervention (p = 0.875). Both immediately following the intervention and three months later, the effect sizes between groups were small (Table 3 ).
To our knowledge, this study is the first multicenter randomized controlled trial to explore the effects of different simulation teaching methods on nursing students' competence and the first study in which multiple different indicators were evaluated simultaneously. The indicators included both objectively assessed indicators of knowledge and skills and subjectively assessed indicators of interprofessional collaboration, critical thinking, caring, and interest in learning. This study assessed the immediate and long-term effects of the intervention by examining its immediate impact as well as its effects three months postintervention.
The results obtained from this study indicate that high-fidelity simulation, computer-based simulation, high-fidelity simulation combined with computer-based simulation, and case study could improve nursing students’ knowledge immediately after intervention. Furthermore, these four teaching methods exhibited comparable effectiveness in improving knowledge. The findings of this study contradict previous meta-analyses that showed that high-fidelity simulation improved nursing students' knowledge over other teaching techniques [ 2 ]. This discrepancy may be attributed to the inclusion of simulation teaching in the previous study alongside theoretical teaching [ 12 ], whereas the current study solely employed simulation teaching without incorporating theoretical instruction. Notably, three months following the intervention, computer-based simulation and case study did not result in knowledge retention. Conversely, high-fidelity simulation, particularly when combined with computer-based simulation, demonstrated knowledge retention, with the latter exhibiting superior performance in this regard. The realistic nature of the simulation provided students with a context in which to apply their knowledge, enhancing their understanding of key concepts [ 30 ]. High-fidelity simulation surpasses computer-based simulation and case study in terms of realism. When combined with computer-based simulation, this approach affords students the opportunity to practice their knowledge in a safe environment while also providing them with access to additional resources and learning opportunities [ 31 ]. Therefore, in this study, high-fidelity simulation combined with computer-based simulation proved to be the most effective at retaining knowledge.
Four simulation-based education strategies were found to be effective at acquiring and retaining skills by the students in this study. High-fidelity simulation combined with computer-based simulation was found to be more effective at acquiring skill than was using either method alone. This method combines the benefits of both teaching methods, providing students with a comprehensive learning experience that combines physical realism and virtual interactivity [ 32 ]. Hybrid simulation creates a seamless learning experience in which individuals can practice their skills in a simulated environment, receive immediate feedback, and then transfer those skills to real-world situations. This integration provides a seamless transition from theoretical knowledge to practical skills, making it easier for individuals to apply what they have learned and enhance their overall performance [ 33 ]. Hybrid simulation may seem to be an attractive option [ 34 ]; however, this study found that hybrid simulation had no advantage in terms of skill retention; rather, high-fidelity simulation performed best. More research is needed in the future to confirm the results of this study and the underlying reasons since previous studies have not compared hybrid simulation with high-fidelity simulation on skill retention.
The findings of this study reveal a noteworthy observation: interprofessional collaboration improved across all interventions, except for high-fidelity simulation. This finding diverges from prior studies that indicated high-fidelity simulation as a more effective method for enhancing students' interprofessional collaboration compared to traditional case study [ 35 ]. This discrepancy may be attributed to the use of an unfolding case study in the current study, wherein patient scenarios evolve unpredictably, thereby prompting students and team members to engage in heightened collaborative efforts to address evolving patient care challenges [ 36 ]. Interprofessional collaboration plays a crucial role in improving healthcare outcomes. Studies have shown that when healthcare professionals collaborate effectively, patients experience better outcomes, fewer errors, and shorter hospital stays [ 37 ]. While high-fidelity simulation has gained popularity as a training tool, according to the results of this study, its impact on interprofessional collaboration remains limited. There may be two reasons for this. First, high-fidelity simulation scenarios are often time constrained [ 38 ], which can hinder effective interprofessional collaboration. Each team member may prioritize their individual goals or tasks, making it difficult to achieve optimal teamwork and coordination. Second, interprofessional team members may not have worked together extensively, which can hinder their ability to collaborate effectively in a high-fidelity simulation setting. It takes time to build trust and rapport, which may not be readily available in a simulated environment [ 39 ]. Despite being assigned the roles of senior nurse or junior nurse, participants in the high-fidelity simulation group were provided with the opportunity to engage with peers at various levels and individuals from different professions, such as instructors assuming the role of doctors. However, the duration of the simulation section for this group was limited to only 10 min. In contrast, participants in the computer-based simulation group and case study group were allocated 30 min and 35 min, respectively. It is crucial for healthcare institutions and educators to critically evaluate their simulation-based training programs and incorporate key components that promote interprofessional collaboration [ 40 ].
This study revealed that four interventions effectively promoted students' critical thinking, and these effects lasted for three months after the interventions. Furthermore, high-fidelity simulation was most effective at improving critical thinking in the short term, whereas computer-based simulation was most effective at fostering long-term improvements. High-fidelity simulation involves creating a realistic and immersive environment that closely resembles a real-world scenario [ 41 ]. This approach affords individuals the opportunity to actively participate and immerse themselves in the simulated scenario, thereby enhancing their experiential understanding [ 3 ]. Computer-based simulation does not provide the same immediate and tangible experience as high-fidelity simulation. High-fidelity simulation commonly incorporates the utilization of medical devices and mannequins that closely resemble clinical scenarios, thereby affording students a more authentic and immersive learning encounter. Only 5% of students perceive computer-based simulation as a viable substitute for mannequin-based simulation within the curriculum [ 42 ]. As a result, high-fidelity simulation is highly effective in the short term, and a previous meta-analysis reported similar results [ 43 ]. However, computer-based simulation provides advantages for data collection and analysis that contribute to the long-term development of critical thinking skills. In the simulation, participants can record their actions, decisions, and results [ 3 ]. These data can be used to compare different strategies and approaches, allowing participants to reflect on their own critical thinking skills and identify areas for improvement. Furthermore, it is noteworthy that the four simulation teaching methods demonstrated the ability to enhance students' critical thinking. However, it is important to consider the substantial disparity in costs among these methods. Therefore, educators should carefully evaluate their available resources and opt for the most cost-effective approach to foster students' critical thinking.
This study found limited evidence that all four simulation teaching methods contribute to improve caring among students. High-fidelity simulation often focuses on technical skills rather than patient interaction or emotional sensitivity [ 44 , 45 ]. Moreover, research has demonstrated that using mannequins in high-fidelity simulation leads some students to perceive them as separate from real-life patients [ 45 ]. This perception reduces students' concern for the consequences of their actions during the simulation [ 45 ], hindering empathy development and limiting the cultivation of their caring abilities [ 46 ]. Unlike high-fidelity simulation, which provides tactile experiences and simulates real-life interactions, computer-based simulation is characterized by the absence of human connections. This lack of physical proximity can hinder the development of caring behaviors such as nonverbal communication, empathy, and sympathy [ 47 , 48 ]. Similarly, the absence of direct patient interaction is a notable drawback of case study. Although case study simulates complex patient care scenarios, they do not allow students to practice hands-on or experience caregiving emotions. Similarly, the absence of direct patient interactions in case study is a notable limitation. This lack of personal connection and guided practice may hinder the development of caring behaviors. By recognizing these limitations and seeking alternative instructional methods, educational institutions can strive to enhance students' caring skills and equip them with the qualities and behaviors necessary for providing compassionate and patient-centered care.
The findings of this study revealed that neither computer-based simulation nor case study improved students' interest in learning, whereas high-fidelity simulation combined with computer-based simulation was most effective. One possible explanation for the ineffectiveness of computer-based simulation and case study in promoting students' interest is that they may lack the authenticity and immersive nature of real-world experiences [ 47 , 48 ]. High-fidelity simulation, on the other hand, provides a more lifelike and interactive learning environment, which may enhance students' engagement, interest, and retention [ 49 ]. High-fidelity simulation combined with computer-based simulation allows students to interact with the simulation in a hands-on manner while also having access to additional resources and information through computer-based simulation [ 50 ]. This combination provides a well-rounded learning experience that can captivate students' attention and keep them engaged. Notably, these findings are exploratory and should be further explored and validated in future studies. Further research should aim to identify the reasons behind the lack of improvement in students' interest in learning when using computer-based simulation and case study alone. Additionally, the impact of different combinations of simulation techniques on students' interest in learning should be investigated to further refine instructional practices.
Limitations
This study provides valuable insights into the effectiveness of simulation-based education in improving nursing students' competences. However, it is essential to acknowledge and address the study's limitations. One of the limitations is the possible selection bias introduced by the recruiting process. It is possible that students who were more motivated or had a greater interest in simulation-based education may have been more likely to participate in the study. This bias may have influenced the outcomes and interpretation of the results. Additionally, the participants were primarily from one cultural background, which may limit the generalizability of the findings. Future studies should include participants from diverse backgrounds to enhance generalizability. Third, participants assigned to different intervention groups may engage in communication and information sharing, potentially leading to contamination effects. To mitigate this issue, future studies could employ cluster randomized controlled trials, which can effectively minimize the risk of contamination among participants. Finally, the follow-up period was relatively short, which limits the understanding of the long-term impact of simulation-based education on competence. Long-term follow-up studies are needed to evaluate the sustained effect of simulation-based education on competence. Future research should aim to address these limitations to further our understanding of the effects of simulation-based education on undergraduate nursing students' competences.
The four methods are effective at improving skills and critical thinking both immediately and over time. In addition to high-fidelity simulation, the other three methods promote interprofessional collaboration both immediately and long term. High-fidelity simulation combined with computer-based simulation is the most effective approach for enhancing interest in learning both immediately and long term. Undergraduate nursing students benefit equally from four methods in cultivating their knowledge, interprofessional collaboration, critical thinking, caring, and interest in learning both immediately and over time. High-fidelity simulation and high-fidelity simulation combined with computer-based simulation improve skill more effectively than computer-based simulation in the short term. Nursing educators can select the most suitable teaching method to achieve the intended learning outcomes depending on the specific circumstances.
Availability of data and materials
The data that support the findings of this study are available from the corresponding author, upon reasonable request.
Panda S, Dash M, John J, Rath K, Debata A, Swain D, et al. Challenges faced by student nurses and midwives in clinical learning environment – A systematic review and meta-synthesis. Nurse Educ Today. 2021;101: 104875. https://doi.org/10.1016/j.nedt.2021.104875 .
Article PubMed Google Scholar
Li YY, Au ML, Tong LK, Ng WI, Wang SC. High-fidelity simulation in undergraduate nursing education: A meta-analysis. Nurse Educ Today. 2022;111: 105291. https://doi.org/10.1016/j.nedt.2022.105291 .
Tamilselvan C, Chua SM, Chew HSJ, Devi MK. Experiences of simulation-based learning among undergraduate nursing students: A systematic review and meta-synthesis. Nurse Educ Today. 2023;121: 105711. https://doi.org/10.1016/j.nedt.2023.105711 .
Mulyadi M, Tonapa SI, Rompas SSJ, Wang R-H, Lee B-O. Effects of simulation technology-based learning on nursing students’ learning outcomes: A systematic review and meta-analysis of experimental studies. Nurse Educ Today. 2021;107: 105127. https://doi.org/10.1016/j.nedt.2021.105127 .
Chernikova O, Heitzmann N, Stadler M, Holzberger D, Seidel T, Fischer F. Simulation-Based Learning in Higher Education: A Meta-Analysis. Rev Educ Res. 2020;90(4):499–541. https://doi.org/10.3102/0034654320933544 .
Article Google Scholar
Lioce L. Healthcare Simulation Dictionary. 2nd ed. Rockville: Agency for Healthcare Research and Quality; 2020.
Book Google Scholar
Kim J, Park J-H, Shin S. Effectiveness of simulation-based nursing education depending on fidelity: a meta-analysis. BMC Med Educ. 2016;16(1):152. https://doi.org/10.1186/s12909-016-0672-7 .
Article PubMed PubMed Central Google Scholar
Roberts E, Kaak V, Rolley J. Simulation to Replace Clinical Hours in Nursing: A Meta-narrative Review. Clin Simul Nurs. 2019;37:5–13. https://doi.org/10.1016/j.ecns.2019.07.003 .
Lapkin S, Levett-Jones T. A cost–utility analysis of medium vs. high-fidelity human patient simulation manikins in nursing education. J Clin Nurs. 2011;20(23–24):3543–52. https://doi.org/10.1111/j.1365-2702.2011.03843.x .
Dziuban C, Graham CR, Moskal PD, Norberg A, Sicilia N. Blended learning: the new normal and emerging technologies. Int J Educ Technol High Educ. 2018;15(1):3. https://doi.org/10.1186/s41239-017-0087-5 .
Goldsworthy S, Ferreira C, Shajani Z, Snell D, Perez G. Combining Virtual and High-fidelity Simulation to Foster Confidence and Competency in Postpartum Assessment Complications among Undergraduate Nursing Students. Clin Simul Nurs. 2022;66:18–24. https://doi.org/10.1016/j.ecns.2022.02.001 .
Kang KA, Kim SJ, Lee MN, Kim M, Kim S. Comparison of Learning Effects of Virtual Reality Simulation on Nursing Students Caring for Children with Asthma. Int J Enviro Res Public Health. 2020;17(22):8417. https://doi.org/10.3390/ijerph17228417 .
Ellis M, Hampton D, Makowski A, Falls C, Tovar E, Scott L, et al. Using unfolding case scenarios to promote clinical reasoning for nurse practitioner students. J Am Assoc Nurse Pract. 2023;35(1):55–62. https://doi.org/10.1097/jxx.0000000000000806 .
Englund H. Using unfolding case studies to develop critical thinking skills in baccalaureate nursing students: A pilot study. Nurse Educ Today. 2020;93: 104542. https://doi.org/10.1016/j.nedt.2020.104542 .
Wang X, Yang L, Hu S. Teaching nursing students: As an umbrella review of the effectiveness of using high-fidelity simulation. Nurse Educ Pract. 2024;77: 103969. https://doi.org/10.1016/j.nepr.2024.103969 .
La Carmen C, Angelo D, Valeria C, Ilaria F, Elona G, Cristina P, et al. Effects of high-fidelity simulation based on life-threatening clinical condition scenarios on learning outcomes of undergraduate and postgraduate nursing students: a systematic review and meta-analysis. BMJ Open. 2019;9(2): e025306. https://doi.org/10.1136/bmjopen-2018-025306 .
Au ML, Tong LK, Li YY, Ng WI, Wang SC. Impact of scenario validity and group size on learning outcomes in high-fidelity simulation: A systematics review and meta-analysis. Nurse Educ Today. 2023;121: 105705. https://doi.org/10.1016/j.nedt.2022.105705 .
Book ECfAtNNLE. 2022 National Nurse Licensing Examination Guided Simultaneous Practice Question Set. Beijing: People's Medical Publishing House Co. LTD; 2022.
Todd M, Manz JA, Hawkins KS, Parsons ME, Hercinger M. The Development of a Quantitative Evaluation Tool for Simulations in Nursing Education. Int J Nurs Educ Scholarsh. 2008;5(1). https://doi.org/10.2202/1548-923X.1705
Hayden J, Keegan M, Kardong-Edgren S, Smiley RA. Reliability and Validity Testing of the Creighton Competency Evaluation Instrument for Use in the NCSBN National Simulation Study. Nurs Educ Perspect. 2014;35(4):244–52. https://doi.org/10.5480/13-1130.1 .
Song X, Jin R. Chinese revised CCEI cross-cultural debugging and measurement features evaluation. Int J Nurs. 2018;37(19):2622–7. https://doi.org/10.3760/cma.j.issn.1637-4351.2019.19.009 .
Orchard C, Mahler C, Khalili H. Assessment of the Interprofessional Team Collaboration Scale for Students-AITCS-II (Student): Development and Testing. J Allied Health. 2021;50(1):E1–7.
PubMed Google Scholar
Shi Y, Zhu Z, Hu Y. The reliability and validity of the Chinese version of the Assessment of Interprofessional Team Collaboration in Student Learning Scale. Chinese J Nurs Educ. 2020;17(5):435–8. https://doi.org/10.3761/j.issn.1672-9234.2020.05.011 .
Shin H, Park CG, Kim H. Validation of Yoon’s Critical Thinking Disposition Instrument. Asian Nurs Res. 2015;9(4):342–8. https://doi.org/10.1016/j.anr.2015.10.004 .
Au ML, Li YY, Tong LK, Wang SC, Ng WI. Chinese version of Yoon Critical Thinking Disposition Instrument: validation using classical test theory and Rasch analysis. BMC Nurs. 2023;22(1):362. https://doi.org/10.1186/s12912-023-01519-y .
Watson R, Lea A. The caring dimensions inventory (CDI): content validity, reliability and scaling. J Adv Nurs. 1997;25(1):87–94. https://doi.org/10.1046/j.1365-2648.1997.1997025087.x .
Article CAS PubMed Google Scholar
Tong LK, Zhu MX, Wang SC, Cheong PL, Van IK. A Chinese Version of the Caring Dimensions Inventory: Reliability and Validity Assessment. Int J Environ Res Public Health. 2021;18(13):6834. https://doi.org/10.3390/ijerph18136834 .
Schiefele U, Krapp A, Wild KP, Winteler A. Der Fragebogen zum Studieninteresse (FSI). [The Study Interest Questionnaire (SIQ)]. Diagnostica. 1993;39(4):335–51.
Google Scholar
Tong LK, Au ML, Li YY, Ng WI, Wang SC. The mediating effect of critical thinking between interest in learning and caring among nursing students: a cross-sectional study. BMC Nurs. 2023;22(1):30. https://doi.org/10.1186/s12912-023-01181-4 .
Graham AC, McAleer S. An overview of realist evaluation for simulation-based education. Adv Simul. 2018;3(1):13. https://doi.org/10.1186/s41077-018-0073-6 .
Sharoff L. Faculty’s Perception on Student Performance using vSim for Nursing® as a Teaching Strategy. Clin Simul Nurs. 2022;65:1–6. https://doi.org/10.1016/j.ecns.2021.12.007 .
Cole R, Flenady T, Heaton L. High Fidelity Simulation Modalities in Preregistration Nurse Education Programs: A Scoping Review. Clin Simul Nurs. 2023;80:64–86. https://doi.org/10.1016/j.ecns.2023.04.007 .
Park S, Hur HK, Chung C. Learning effects of virtual versus high-fidelity simulations in nursing students: a crossover comparison. BMC Nurs. 2022;21(1):100. https://doi.org/10.1186/s12912-022-00878-2 .
Goldsworthy S, Patterson JD, Dobbs M, Afzal A, Deboer S. How Does Simulation Impact Building Competency and Confidence in Recognition and Response to the Adult and Paediatric Deteriorating Patient Among Undergraduate Nursing Students? Clin Simul Nurs. 2019;28:25–32. https://doi.org/10.1016/j.ecns.2018.12.001 .
Tosterud R, Hedelin B, Hall-Lord ML. Nursing students’ perceptions of high- and low-fidelity simulation used as learning methods. Nurse Educ Pract. 2013;13(4):262–70. https://doi.org/10.1016/j.nepr.2013.02.002 .
Cheng C-Y, Hung C-C, Chen Y-J, Liou S-R, Chu T-P. Effects of an unfolding case study on clinical reasoning, self-directed learning, and team collaboration of undergraduate nursing students: A mixed methods study. Nurse Educ Today. 2024;137: 106168. https://doi.org/10.1016/j.nedt.2024.106168 .
Kaiser L, Conrad S, Neugebauer EAM, Pietsch B, Pieper D. Interprofessional collaboration and patient-reported outcomes in inpatient care: a systematic review. Syst Rev. 2022;11(1):169. https://doi.org/10.1186/s13643-022-02027-x .
Tong LK, Li YY, Au ML, Wang SC, Ng WI. High-fidelity simulation duration and learning outcomes among undergraduate nursing students: A systematic review and meta-analysis. Nurse Educ Today. 2022;116: 105435. https://doi.org/10.1016/j.nedt.2022.105435 .
Livne N. High-fidelity simulations offer a paradigm to develop personal and interprofessional competencies of health students: A review article. Int J Allied Health Sci Pract. 2019;17(2). https://doi.org/10.46743/1540-580X/2019.1835
Marion-Martins AD, Pinho DLM. Interprofessional simulation effects for healthcare students: A systematic review and meta-analysis. Nurse Educ Today. 2020;94: 104568. https://doi.org/10.1016/j.nedt.2020.104568 .
Macnamara AF, Bird K, Rigby A, Sathyapalan T, Hepburn D. High-fidelity simulation and virtual reality: an evaluation of medical students’ experiences. BMJ simulation & technology enhanced learning. 2021;7(6):528–35. https://doi.org/10.1136/bmjstel-2020-000625 .
Foronda CL, Swoboda SM, Henry MN, Kamau E, Sullivan N, Hudson KW. Student preferences and perceptions of learning from vSIM for Nursing™. Nurse Educ Pract. 2018;33:27–32. https://doi.org/10.1016/j.nepr.2018.08.003 .
Lei Y-Y, Zhu L, Sa YTR, Cui X-S. Effects of high-fidelity simulation teaching on nursing students’ knowledge, professional skills and clinical ability: A meta-analysis and systematic review. Nurse Educ Pract. 2022;60: 103306. https://doi.org/10.1016/j.nepr.2022.103306 .
Najjar RH, Lyman B, Miehl N. Nursing Students’ Experiences with High-Fidelity Simulation. Int J Nurs Educ Scholarsh. 2015;12(1):27–35. https://doi.org/10.1515/ijnes-2015-0010 .
Au ML, Lo MS, Cheong W, Wang SC, Van IK. Nursing students’ perception of high-fidelity simulation activity instead of clinical placement: A qualitative study. Nurse Educ Today. 2016;39:16–21. https://doi.org/10.1016/j.nedt.2016.01.015 .
Dean S, Williams C, Balnaves M. Practising on plastic people: Can I really care? Contemp Nurse. 2015;51(2–3):257–71. https://doi.org/10.1080/10376178.2016.1163231 .
Chang YM, Lai CL. Exploring the experiences of nursing students in using immersive virtual reality to learn nursing skills. Nurse Educ Today. 2021;97: 104670. https://doi.org/10.1016/j.nedt.2020.104670 .
Jeon J, Kim JH, Choi EH. Needs Assessment for a VR-Based Adult Nursing Simulation Training Program for Korean Nursing Students: A Qualitative Study Using Focus Group Interviews. Int J Environ Res Public Health. 2020;17(23):8880. https://doi.org/10.3390/ijerph17238880 .
Davis R. Nursing Student Experiences with High-Fidelity Simulation Education [Ed.D.]. Arizona: Grand Canyon University; 2021.
Saab MM, Landers M, Murphy D, O’Mahony B, Cooke E, O’Driscoll M, et al. Nursing students’ views of using virtual reality in healthcare: A qualitative study. J Clin Nurs. 2022;31(9–10):1228–42. https://doi.org/10.1111/jocn.15978 .
Download references
Acknowledgements
Not applicable.
This work was supported by a research grant from Higher Education Fund of Macao SAR Government (project number: HSS-KWNC-2021–01). This funding source had no role in the design of this study and will not have any role during its execution, analyses, interpretation of the data, or decision to submit results.
Author information
Authors and affiliations.
Kiang Wu Nursing College of Macau, Edifício do Instituto de Enfermagem Kiang Wu de Macau, Avenida do Hospital das Ilhas no.447, Coloane, RAEM, Macau SAR, China
Lai Kun Tong, Yue Yi Li, Mio Leng Au, Wai I. Ng & Si Chen Wang
School of Nursing, Yangzhou University, No.136, Jiangyang Middle Road, Hanjiang District, Yangzhou, Jiangsu Province, China
Yongbing Liu
School of Nursing, Guangzhou Xinhua University, 19 Huamei Road, Tianhe District, Guangzhou, Guangdong Province, China
School of Nursing, Guangzhou Medical University, Dongfeng West Road, Yuexiu District, Guangzhou, Guangdong Province, China
Liqiang Zhong
School of Nursing, Shenzhen University, No. 3688, Nanhai Road, Nanshan District, Shenzhen, Guangdong Province, China
Xichenhui Qiu
You can also search for this author in PubMed Google Scholar
Contributions
Study conceptualization and planning were organized and performed by LKT, YYL, MLA, WIN, SCW, YBL, YS, LQZ, and XCHQ. Data collection, data analysis and data interpretation were performed by LKT, YYL, MLA, WIN, SCW, YBL, YS, LQZ, and XCHQ. LKT drafted the initial version of the manuscript. YYL, MLA, WIN, SCW, YBL, YS, LQZ, and XCHQ revised the manuscript for important intellectual content. All authors had full access to the data and have reviewed and approved the submitted version of the manuscript. All authors agree to be accountable for all aspects of the work in ensuring that questions related to the accuracy or integrity of any part of the work are appropriately investigated and resolved.
Corresponding author
Correspondence to Mio Leng Au .
Ethics declarations
Ethics approval and consent to participate.
This research was approved by the Research Management and Development Department of Kiang Wu Nursing College of Macau (No. REC-2021.801) and conducted according to the Declaration of Helsinki. It was a completely voluntary, anonymous, and unrewarded study. Written consent was obtained from all participants.
Consent for publication
Competing interests.
The authors declare no competing interests.
Additional information
Publisher's note.
Springer Nature remains neutral with regard to jurisdictional claims in published maps and institutional affiliations.
Rights and permissions
Open Access This article is licensed under a Creative Commons Attribution 4.0 International License, which permits use, sharing, adaptation, distribution and reproduction in any medium or format, as long as you give appropriate credit to the original author(s) and the source, provide a link to the Creative Commons licence, and indicate if changes were made. The images or other third party material in this article are included in the article's Creative Commons licence, unless indicated otherwise in a credit line to the material. If material is not included in the article's Creative Commons licence and your intended use is not permitted by statutory regulation or exceeds the permitted use, you will need to obtain permission directly from the copyright holder. To view a copy of this licence, visit http://creativecommons.org/licenses/by/4.0/ . The Creative Commons Public Domain Dedication waiver ( http://creativecommons.org/publicdomain/zero/1.0/ ) applies to the data made available in this article, unless otherwise stated in a credit line to the data.
Reprints and permissions
About this article
Cite this article.
Tong, L.K., Li, Y.Y., Au, M.L. et al. The effects of simulation-based education on undergraduate nursing students' competences: a multicenter randomized controlled trial. BMC Nurs 23 , 400 (2024). https://doi.org/10.1186/s12912-024-02069-7
Download citation
Received : 21 March 2024
Accepted : 05 June 2024
Published : 17 June 2024
DOI : https://doi.org/10.1186/s12912-024-02069-7
Share this article
Anyone you share the following link with will be able to read this content:
Sorry, a shareable link is not currently available for this article.
Provided by the Springer Nature SharedIt content-sharing initiative
- High-fidelity simulation
- Computer-based simulation
- High-fidelity simulation combined with computer-based simulation
BMC Nursing
ISSN: 1472-6955
- General enquiries: [email protected]
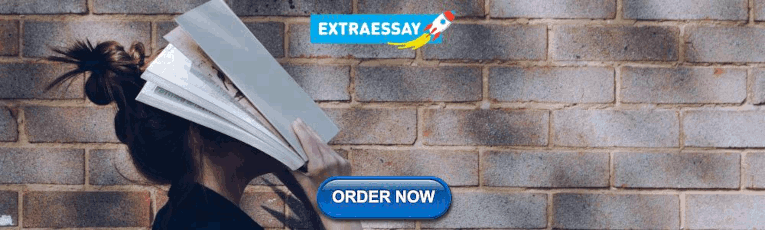
IMAGES
VIDEO
COMMENTS
Randomized controlled trials (RCT) are prospective studies that measure the effectiveness of a new intervention or treatment. Although no study is likely on its own to prove causality, randomization reduces bias and provides a rigorous tool to examine cause-effect relationships between an intervention and outcome. This is because the act of ...
Introduction. Case-control and cohort studies are observational studies that lie near the middle of the hierarchy of evidence. These types of studies, along with randomised controlled trials, constitute analytical studies, whereas case reports and case series define descriptive studies (1). Although these studies are not ranked as highly as ...
Randomized controlled trials (RCTs) have traditionally been viewed as the gold standard of clinical trial design, residing at the top of the hierarchy of levels of evidence in clinical study; this is because the process of randomization can minimize differences in characteristics of the groups that may influence the outcome, thus providing the ...
The highest grade is reserved for research involving "at least one properly randomized controlled trial," and the lowest grade is applied to descriptive studies (e.g., case series) and expert ...
Randomized controlled trials (RCTs) are the hallmark of evidence-based medicine and form the basis for translating research data into clinical practice. ... Nonexperimental research include case reports, case series, cross-sectional, and prospective observational studies, such as case-control and cohort studies. These types of research ...
Background While randomised controlled trials (RCTs) provide high-quality evidence to guide practice, much routine care is not based upon available RCTs. This disconnect between evidence and practice is not sufficiently well understood. This case study explores this relationship using a novel approach. Better understanding may improve trial design, conduct, reporting and implementation ...
Randomized, controlled trials are key in advancing medical understanding, but some questions are not amenable to this approach. ... AR, Viscidi, R, et al. Case-control study of human ...
WalkBack was a two-armed, randomised controlled trial, which recruited adults (aged 18 years or older) from across Australia who had recently recovered from an episode of non-specific low back pain that was not attributed to a specific diagnosis, and which lasted for at least 24 h.
Using four case studies, we aim to provide practical guidance and recommendations for the analysis of cluster randomised controlled trials. Four modelling approaches (Generalized Linear Mixed Models with parameters estimated by maximum likelihood/restricted maximum likelihood; Generalized Linear Models with parameters estimated by Generalized Estimating Equations (1st order or second ...
Randomized controlled trials (RCTs) are considered the highest level of evidence to establish causal associations in clinical research. There are many RCT designs and features that can be selected to address a research hypothesis. Designs of RCTs have become increasingly diverse as new methods have been proposed to evaluate increasingly complex ...
The EVRAAS pilot study was designed as a single-center, randomized, double-blind, placebo-controlled trial. Its design was approved by the Nicolaus Copernicus University (NCU) Bioethics Committee ...
Study protocol; Open access; Published: 18 June 2024 The effect of an online acceptance and commitment intervention on the meaning-making process in cancer patients following hematopoietic cell transplantation: study protocol for a randomized controlled trial enhanced with single-case experimental design
Randomized trials and observational studies, such as case-control studies, are often seen as opposing approaches. However, in many instances results obtained by different designs may complement each other. For instance, case-control studies on aetiology of disease may help to give the direction of future trials. In this commentary, the author discusses the purpose of randomization and ...
Randomized controlled trials (RCTs) are considered the highest level of evidence to establish causal associations in clinical research. There are many RCT designs and features that can be selected to address a research hypothesis. Designs of RCTs have become increasingly diverse as new methods have been proposed to evaluate increasingly complex scientific hypotheses. This article reviews the ...
Definition. A study design that randomly assigns participants into an experimental group or a control group. As the study is conducted, the only expected difference between the control and experimental groups in a randomized controlled trial (RCT) is the outcome variable being studied.
Design, Setting, and Participants The Safety and Efficacy of Adalimumab for Hidradenitis Suppurativa Peri-Surgically (SHARPS) trial was a phase 4, randomized, double-blind, placebo-controlled study of adalimumab in conjunction with surgery. Patients were enrolled in 45 sites across 20 countries from July 18, 2016, to February 2, 2019, with the ...
Randomized controlled trials (RCTs) have traditionally been considered the gold standard for medical evidence. However, in light of emerging methodologies in data science, many experts question the role of RCTs. Within this context, experts in the USA and Canada came together to debate whether the primacy of RCTs as the gold standard for medical evidence, still holds in light of recent ...
Abstract. In the hierarchy of research designs, the results of randomized controlled trials are considered the highest level of evidence. Randomization is the only method for controlling for known and unknown prognostic factors between two comparison groups. Lack of randomization predisposes a study to potentially important imbalances in ...
A recent investigation to compare observational studies and randomized, controlled trials was performed by the United Kingdom Health Technology Assessment Group. 13 They found eight treatments ...
A single-center, prospective, randomized, clinical trial was conducted on patients undergoing elective MVR surgery. The study was approved by the ethics committee of the Affiliated Hospital of Xuzhou Medical University, Xuzhou, Jiangsu Province, China (XYFY2016-KL035-01). It was performed in compliance with the Declaration of Helsinki.
Randomised controlled trials in primary care: case study. Although over 90% of patient contacts within the NHS occur in primary care, many of the interventions used in this setting remain unproved. 1 The relevance of research undertaken in secondary or tertiary care to general practice is questionable, and more research based in primary care is ...
In this post, I will explain a crucial tool that helps us do this - randomized controlled trials (RCTs). We will see that RCTs matter for three reasons: when we don't know about the effects of interventions, when we don't know how to study them, and when scientific research is affected by biases.
Background and aims This study aimed to evaluate the efficacy and safety of vonoprazan and tetracycline (VT) dual therapy as first-line treatment for Helicobacter pylori infection in patients with penicillin allergy. Methods In this randomised controlled trial, treatment-naïve adults with H. pylori infection and penicillin allergy were randomised 1:1 to receive either open-label VT dual ...
Abstract. A randomized controlled trial is a prospective, comparative, quantitative study/experiment performed under controlled conditions with random allocation of interventions to comparison groups. The randomized controlled trial is the most rigorous and robust research method of determining whether a cause-effect relation exists between an ...
Abstract. Randomized trials and observational studies, such as case-control studies, are often seen as opposing approaches. However, in many instances results obtained by different designs may complement each other. For instance, case-control studies on aetiology of disease may help to give the direction of future trials.
General Principles of Randomized Controlled Trials. The randomized controlled trial is one of the simplest but most powerful tools of research. In essence, the randomized controlled trial is a study in which people are allocated at random to receive one of several clinical interventions [ 2 ].
Findings In this randomized clinical trial of 80 adults with overweight and habitual sleep less than 6.5 hours per night, those randomized to a 2-week sleep extension intervention significantly reduced their daily energy intake by approximately 270 kcal compared with the control group. Total energy expenditure did not significantly differ ...
A randomized control trial (RCT) is a type of study design that involves randomly assigning participants to either an experimental group or a control group to measure the effectiveness of an intervention or treatment. Randomized Controlled Trials (RCTs) are considered the "gold standard" in medical and health research due to their rigorous ...
The purpose of this study is to evaluate the effectiveness of a novel composite biologics in LIHR. A multicenter, single-blinded, randomized controlled clinical trial was designed. Fifty patients with unilateral primary inguinal hernia were randomly assigned to the experimental and control group (1:1).
The purpose of this multicenter randomized controlled trial was to evaluate the effects of high-fidelity simulation, computer-based simulation, high-fidelity simulation combined with computer-based simulation, and case study on undergraduate nursing students. A total of 270 nursing students were recruited from five universities in China.