GLM ps_ctl ps_tx /WSFACTOR = group 2 /EMMEANS = TABLES(group) /PRINT = DESCRIPTIVE /WSDESIGN = group . | T-TEST PAIRS= ps_ctl WITH ps_tx (PAIRED). | III. Matching in Quasi-Experimental Designs: Normative Group MatchingSuppose that you have a quasi-experiment where you want to compare an experimental group (e.g., people who have suffered mild head injury) with a sample from a normative population. Suppose that there are several hundred people in the normative population. One strategy is to randomly select the same number of people from the normative population as you have in your experimental group. If the demographic characteristics of the normative group approximate those of your experimental group, then this process may be appropriate. But, what if the normative group contains equal numbers of males and females ranging in age from 6 to 102, and people in your experimental condition are all males ranging in age from 18 to 35? Then it is unlikely that the demographic characteristics of the people sampled from the normative group will match those of your experimental group. For that reason, simple random selection is rarely appropriate when sampling from a normative population. The Normative Group Matching ProcedureDetermine the relevant characteristics (e.g., age, gender, SES, etc.) of each person in your experimental group. E.g., Exp person #1 is a 27 year-old male. Then randomly select one of the 27 year-old males from the normative population as a match for Exp person #1. Exp person #2 is a 35 year-old male, then randomly select one of the 35 year-old males as a match for Exp person #2. If you have done randomize normative group matching then the matching variable should be used as a blocking factor in the ANOVA. If you have a limited number of people in the normative group then you can do caliper matching . In caliper matching you select the matching person based a range of scores, for example, you can caliper match within a range of 3 years. Exp person #1 would be randomly selected from males whose age ranged from 26 to 27 years. If you used a five year caliper for age then for exp person #1 you randomly select a males from those whose age ranged from 25 to 29 years old. You would want a narrower age caliper for children and adolescents than for adults. This procedure becomes very difficult to accomplish when you try to start matching on more than one variable. Think of the problems of finding exact matches when several variables are used, e.g., an exact match for a 27-year old, white female with an IQ score of 103 and 5 children. Analysis of a Normative Group Matching DesignThe analysis is the same as for a matched random assignment design. If the matching variable is related to the dependent variable, then you can incorporate the matching variable as a blocking variable in your analysis of variance. III. Matching in Quasi-Experimental Designs: Normative Group EquivalenceBecause of the problems in selecting people in a normative group matching design and the potential problems with the data analysis of that design, you may want to make the normative comparison group equivalent on selected demographic characteristics. You might want the same proportion of males and females, and the mean age (and SD) of the normative group should be the same as those in the experimental group. If the ages of the people in the experimental group ranged from 18 to 35, then your normative group might contain an equal number of participants randomly selected from those in the age range from 18 to 35 in the normative population. Analysis of a Normative Group Equivalence DesignIn the case of normative group equivalence there is no special ANOVA procedure as there is in Normative Group Matching. In general, demographic characteristics themselves rarely predict the d.v., so you haven’t lost anything by using the group equivalence method. A Semantic CautionThe term "matching" implies a one-to-one matching and it implies that you have incorporated that matched variable into your ANOVA design. Please don’t use the term "matching" when you mean mere "equivalence." Click through the PLOS taxonomy to find articles in your field. For more information about PLOS Subject Areas, click here . Loading metrics Open Access Peer-reviewed Research Article Effect of an educational intervention based on self-efficacy theory and health literacy skills on preventive behaviors of urinary tract infection in pregnant women: A quasi-experimental studyRoles Writing – original draft, Writing – review & editing Affiliations Social Determinants of Health Research Center, Mashhad University of Medical Sciences, Mashhad, Iran, Faculty of Health, Department of Health, Safety, and Environment, Mashhad University of Medical Sciences, Mashhad, Iran Roles Data curation Affiliation Faculty of Health, Department of Health Education and Health Promotion, Mashhad University of Medical Sciences, Mashhad, Iran Roles Writing – review & editing Affiliation Department of Health Education and Health Promotion, School of Health, Mashhad University of Medical Sciences, Mashhad, Iran  Roles Methodology Affiliations Social Determinants of Health Research Center, Mashhad University of Medical Sciences, Mashhad, Iran, Faculty of Health Sciences, Department of Epidemiology and Biostatistics, Mashhad University of Medical Sciences, Mashhad, Iran * E-mail: [email protected] Affiliations Social Determinants of Health Research Center, Mashhad University of Medical Sciences, Mashhad, Iran, Department of Health Education and Health Promotion, School of Health, Mashhad University of Medical Sciences, Mashhad, Iran - Seyedeh Belin Tavakoly Sany,
- Vajieh Eslami,
- Elaheh lael-Monfared,
- Vahid Ghavami,
- Nooshin Peyman
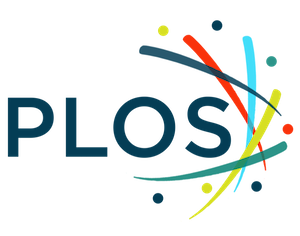 - Published: August 13, 2024
- https://doi.org/10.1371/journal.pone.0306558
- Reader Comments
 The impact of self-efficacy and health literacy skills on pregnant women’s adherence to urinary tract infection (UTI) preventive behaviors is inadequately investigated. Thus, the present study explored whether an educational intervention based on self-efficacy and health literacy skills managed to improve UTI preventive behaviors among pregnant women. A quasi-experimental study was conducted from January to July 2021 among pregnant women residing in Mashhad, Iran. To this aim, 110 pregnant women at a gestational age of 12–18 weeks were randomly assigned to a control (n = 55) and an intervention group (n = 55) and completed all questionnaires during the intervention and the 3-month follow-up. The intervention group received the full training program, comprising six 2-hourly training sessions. Most women were from low-income families (69.1%), were housewives (74.5%) with high school education or lower (63.6%). The theory-based intervention had a significant effect (P < 0·05) on UTI preventive behavior outcomes (i.e., clothing habits, nutrition, urination, health, and sexual behaviors) in the intervention group compared with the control group after intervention, and in their variation from baseline to follow-up in all scores. ConclusionsAn educational intervention based on health literacy skills and self-efficacy could be an effective theory-based intervention to improve UTI preventive behaviors and reduce recurrent UTI and complications. Citation: Tavakoly Sany SB, Eslami V, lael-Monfared E, Ghavami V, Peyman N (2024) Effect of an educational intervention based on self-efficacy theory and health literacy skills on preventive behaviors of urinary tract infection in pregnant women: A quasi-experimental study. PLoS ONE 19(8): e0306558. https://doi.org/10.1371/journal.pone.0306558 Editor: Kahsu Gebrekidan, University of Oulu: Oulun Yliopisto, FINLAND Received: December 31, 2023; Accepted: June 18, 2024; Published: August 13, 2024 Copyright: © 2024 Tavakoly Sany et al. This is an open access article distributed under the terms of the Creative Commons Attribution License , which permits unrestricted use, distribution, and reproduction in any medium, provided the original author and source are credited. Data Availability: We confirm at this time Our submission contains all raw data required to replicate the results of our study. All relevant data are within the manuscript and its Supporting Information files. Funding: This research was funded by the Mashhad University of Medical Sciences (Project number: 980582). The funders had no role in study design, data collection and analysis, decision to publish, or preparation of the manuscript. Competing interests: The authors have declared that no competing interests exist. Abbreviations: UTI, urinary tract infection; WHO, World Health Organization 1. BackgroundPregnancy is a natural physiological process in a woman’s life, accompanied by physiological and psychological changes. However, maternal comorbidities or unexpected diseases can complicate pregnancy and have adverse effects. Thus, a mother’s health before and during childbirth is very important for children’s health [ 1 , 2 ]. Urinary tract infection (UTI) is a common clinical disease marked by a continuous and active proliferation of bacteria inside the urinary tract [ 3 ], and involves the urinary tract, bladder and kidney infections. UTI may be symptomatic or asymptomatic [ 4 ] with the latter being of a particular importance due to the absence of any symptoms. Its complications account for about 150 million mortalities annually worldwide [ 5 ]. UTI is a common bacterial infection and the second main complication of pregnancy, after anemia. Anatomical and physiological changes of the urinary tracts during pregnancy increase the prevalence of UTI [ 6 ]. The prevalence of asymptomatic bacteriuria in the world is 2–15% [ 7 ]. In Iran, the prevalence of UTI in pregnant women is 8.7% [ 8 ]. In developing countries, pregnant women have a higher rate of UTI than counterparts in developed countries [ 9 ]. In a meta-analysis, the overall prevalence of UTI during pregnancy in Iran was estimated at 13%. In different parts of Iran, this rate varied greatly. For example, in Tehran and Arak, it is 2–13%, in Hamadan 10%, and in Torbat Heydarieh, it is reported to be 10% [ 10 ]. UTI is among the most widespread and costly medical complications in pregnancy which accounts for 10% of all hospitalizations during pregnancy [ 11 ]. As the existing literature shows, UTI in pregnant women begins at the 6 th week of pregnancy and reaches its peak in the 22 nd - 24 th week [ 2 , 8 ]. Besides the high cost of treatment and hospitalization, UTI during pregnancy has many lifelong maternal and fetal complications, including pyelonephritis, preeclampsia, shock, septicemia, anemia, and endometritis. Fetal complications of UTI during pregnancy include birth weight loss, premature birth, respiratory failure, fetal death, mental retardation, and lower intelligence quotient (IQ) [ 4 , 9 ]. The report of the World Health Organization (WHO) on premature birth shows that every year a million infants die due to premature birth [ 12 ] and that the probability of preeclampsia in pregnant women with UTI is 1.22 times as high as pregnant women without UTI [ 13 ]. Antibiotics are essential to fight UTIs during pregnancy [ 12 ], but an excessive use is a global health threat as it can develop antimicrobial resistance and increase the risk of spontaneous abortion and birth defects [ 14 , 15 ]. The consumption of safe antibiotics during pregnancy is limited due to their teratogenic potential [ 16 ]. In light of the aforementioned issues, several measures can be taken to prevent UTI during pregnancy, such as adherence to healthy behaviors in sexual activity, the clothing style, eating habits, urinary habits and cleaning, which are all among the predisposing factors for UTI [ 17 , 18 ]. Inadequate knowledge and skills can decrease the motivation to adopt preventive behaviors and can hinder a full prevention [ 17 ]. Health literacy skills and self-efficacy are effective factors to prevent infectious diseases [ 19 – 21 ]. In the related literature, health literacy is “a set of reading, listening, analysis and decision-making skills, and the ability to apply these skills in health-related conditions” [ 22 , 23 ]. American Center for Health Care Strategies reported that people with higher health literacy have more chances of using spoken and written information provided by professionals; therefore, they have a better state of health. Health literacy skills improve the acquisition of knowledge about health issues, correct decisions about health, and benefits of healthcare services [ 24 , 25 ]. Problems related to lifestyle changes require a high level of self-confidence. Achieving high self-efficacy, and improving self-efficacy and health literacy is possible through active education [ 26 ]. Choosing a behavior change model for health education is the first step to a planning process [ 18 , 27 ]. A prominent educational theory used to predict and describe behavior is the self-efficacy theory, commonly used in behavior changing programs[ 28 ]. According to Bandura, there are four main sources of self-efficacy including mastery experiences, vicarious experiences, verbal persuasion, and physiological and affective states” [ 29 ]. Self-efficacy is a major prerequisite for behavior change [ 30 ]. Individuals with inadequate self-efficacy are less likely to make efforts to show a new healthy behavior or to change the former unhealthy behavior [ 31 ]. There is research evidence that self-efficacy is an important psychological construct directly and indirectly affecting disease-controlling health behaviors. Self-efficacy can transform knowledge and information related to health promotion and educational interventions in behavioral performance [ 32 ]. Health literacy has been included as a predictor of self-efficacy [ 32 , 33 ]. Although a body of research in Iran shows that women’s awareness of UTI prevention is at a satisfactory level, the prevalence of UTI in pregnant women is still increasing [ 8 , 17 , 34 ]. It seems that only raising the level of knowledge cannot lead to the prevention of UTI [ 35 – 37 ], and there is a need for recognition of other factors affecting on UTI preventive behaviors [ 38 ]. Research evidence shows that self-efficacy and health literacy skills are effective in improving health behaviors. Yet, the relationship between UTI preventive behaviors and health literacy and self-efficacy in pregnant women has not been investigated. Considering the high prevalence of UTI during pregnancy and the serious risks that threaten the mother’s and fetus’ health, the present study aimed to investigate the effect of a health education intervention based on the self-efficacy theory and health literacy skills on pregnant women’s UTI preventive behaviors. The present findings can help decision-makers develop a comprehensive educational program to promote UTI preventive behaviors. Preventive behaviors against UTI helps reduce the excessive use of antibiotics, especially in pregnant women. 2. Materials and methods2.1. participants and sampling.  λ : ratio of sample size in group 2 to group 1 v : number of measures before intervention w : number of measures after intervention p t : correlation coefficient of repeated measures Δ plan : standardized expected effect size Due to the lack of data required to estimate the sample size in the existing literature (e.g., the absence of standard deviation of scores after the intervention in the intervention group), the information about control group in the study by Tehrani et al. [ 26 ] was used. The equality of variance of two groups was assumed. Cohen’s standard effect size was 0.56. The first type error was 0.05 and the test power was 80%. λ , v, w and p t. were, respectively, 1, 1, 2 and 0. 5. The estimated sample size, with an attrition rate of 10%, for each group, was 55. The participants were randomly assigned to the intervention and control groups. In the present study, the data collection was done based on a test of functional health literacy in adults [ 28 , 30 ], and the general self-efficacy questionnaire [ 39 ]. Also, a researcher-made questionnaire was developed to measure UTI preventive behaviors. This questionnaire included demographic information (occupation, age, education, husband’s education and occupation, body mass index (BMI), vomiting during pregnancy, and income) as well as the five domains of UTI prevention behaviors. The questionnaires were completed before, immediately after and three months after the educational intervention in the health centers. All participants were informed about the purpose of study and their demographic information was recorded. Having signed an informed letter of consent, the participants completed the questionnaire of UTI prevention behaviors, test of functional health literacy in adults (TOFHLA) and Schwarzer’s self-efficacy scale. General self-efficacy questionnaire (GSE).Schwarzer’s general self-efficacy questionnaire was used to measure the participants’ self-efficacy. This scale contained 17 questions rated on a 4-point Likert scale ranging from strongly disagree to strongly agree. It was scored from 17 to 85, and a high score showed stronger self-efficacy. Three aspects of behavior, including the desire to initiate the behavior, resistance to barriers, and efforts to complete the task were measured using this test (e.g., “I am a self-reliant person.”, and “I avoid facing difficulties.”) ( S1 Table ). The reliability of scale was estimated at 0.84 in the study by Woodruff and Kashman, and 0.83 in the study by Asgharanjad, and Ahmadi Qutb Al-Dini [ 27 , 39 , 40 ]. Test of functional health literacy in adults (TOFHLA).This questionnaire consisted of two sections, calculations and reading comprehension. The calculations section assessed one’s ability to understand the doctor’s and health educators’ advice. This section required certain calculations, and the score could range between 0 and 50. The reading comprehension section assessed one’s ability to read and comprehend three passages entitled as instructions on preparing for imaging of the upper gastrointestinal tract, the patient responsibilities and rights about the standard hospital consent form and insurance forms. This score ranged from 0 to 50. Thus, the overall health literacy score obtained from these two sections could range between 0 and 100. There were three levels of interpretation of scores: insufficient (0–59), borderline (60–74) and sufficient (75–100) [ 31 ]. The validity and reliability of this questionnaire in Iran were measured by Raisi et al. The reliability was estimated at 0.79 for the calculations section and 0.88 for the reading comprehension section. Its content validity ratio (CVR) was higher than 0.56. and the content validity index (CVI) was estimated at 0.79 [ 28 – 30 ]. Urinary tract infection preventive behaviors questionnaire.In this study, a researcher-made questionnaire was used to measure UTI preventive behaviors in pregnant women. This questionnaire includes demographic information and five dimensions of UTI preventive behaviors, including 25 questions on clothing style (4 questions), eating habits (6 questions), urinary habits (2 questions), cleanliness (7 questions) and sexual behavioral habits (6 questions) ( S2 Table ). In this instrument, the questions were rated based on a Likert scale ranging from never (0) to always (4), with a minimum score of 25 and a maximum score of 100. To check the content validity of the researcher-made questionnaire, it was provided to six eminent professors of health promotion, two distinguished professors of reproductive health, two gynecologists and five midwifery experts. Thus, the content validity (CVR) was measured and substantiated. For the overall instrument, the CVR was estimated at 0.94. Having made the suggested revisions, the content validity index (CVI) for all scales was increased to 0.94. To check internal consistency, Cronbach’s alpha test was used, and the estimated value was 0.72. Also, to check the reliability, a test-retest method was used for 20 pregnant mothers at a time interval of two weeks, based on which the intra-cluster correlation coefficient (ICC) was estimated at 0.97, indicating an acceptable reliability [ 41 ]. 2.2. InterventionThe present quasi-experimental study involved two groups, an intervention, and a control. The intervention was made from January 2021 to July 2021 based on a consort checklist and the Template for Intervention Description and Replication checklist (TIDieR) ( Table 1 ) [ 42 ]. Four health centers were selected randomly from a list of centers, and were assigned to the intervention group (n = 2) and the control (n = 2). Then, a list was made of pregnant women’s names based on their demographic information and health history, and a number was assigned to each using a table of random numbers. Two hundred pregnant women were randomly selected, of whom 84 women were not included in the intervention because they did not meet the inclusion criteria. Six women failed to attend the training or follow-up because of travelling, COVID-19 lock-down, and work-related problems. Finally, 110 women completed all stages of study (before intervention, immediately after intervention, and three months after intervention) ( Fig 1 ).  - PPT PowerPoint slide
- PNG larger image
- TIFF original image
https://doi.org/10.1371/journal.pone.0306558.g001  https://doi.org/10.1371/journal.pone.0306558.t001 The educational intervention was conducted for the intervention group. All women underwent a training program of six two-hour sessions every 7 days. From two centers of the control group, 55 pregnant women with similar conditions were randomly selected and considered as the control group, and the educational content was provided to them after the completion of the intervention. Due to the COVID-19 pandemic, the sensitivity of pregnant mothers’ condition and the health protocols against face-to-face group meetings, four training sessions were held face to face, and the remaining sessions were held online on WhatsApp as the mothers requested. In this study, different oral and combined methods (e.g., lectures with Q&As, brainstorming, group discussions, poster presentation and pamphlets) were used along with online sources (e.g., telephone and social networks to share videos, photos and group discussions in real-time class held in audio-only mode) ( Table 1 ). The intervention training program was designed based on Bandura’s self-efficacy theory [ 27 ] and health literacy skills (spoken communication, promotion and written communication, empowerment, improvement of support systems) [ 40 ] ( Table 2 ).  https://doi.org/10.1371/journal.pone.0306558.t002 In this educational program, according to the participants’ age and literacy level and the objectives of the educational program, there were three cognitive, attitudinal, and functional domains to address, for which visual and auditory media were used such as educational slides, overhead projectors, whiteboards, and pamphlets in this program. Face-to-face training and phone-mediated training were used along with video, photo and voice records in non-face-to-face training. Trainings were conducted by a health education specialist and a gynecologist. During the study, the control group did not receive any special training from the researcher, and after the completion of the intervention, the training was provided as e-learning to the control group. Questionnaires in both groups were completed once before the intervention, and twice more immediately after and three months after the educational intervention. This was done face to face in the first session and online via sharing the questionnaire link in the group to complete. 2.3. Data statistical analysisHaving collected the data, to analyze the descriptive data, the questionnaires were coded and punched into SPSS21. After a careful checking and ensuring of the accuracy of data entry, descriptive statistics of the central tendency and variability indices, such as the mean and standard deviation of values related to the interval variables, and the distribution of frequency and percentage of non-parametric variables were used. To check the normality of distribution of interval variables in the treatment and control groups, Kolmogorov-Smirnov test was used. As the results showed, appropriate parametric tests were used for interval variables and appropriate non-parametric tests were used for non-interval variables. To test the relationship between interval variables, Spearman and Pearson correlation coefficients were used according to the abnormal distribution of data. Mann-Whitney U-test, and Kruskal-Wallis tests were used to test the relationship between interval and non-interval variables according to the number of classes of qualitative variables. Chi-square test was used to explore the relationship between non-interval variables. To compare the two groups before, immediately after and 3 months after intervention in terms of interval variables, repeated measure analysis of variance was used. Friedman’s test was used for non-interval variables. The significance level in all tests was 0.05 and SPSS 21 was used to describe and analyze the data. Ethics approval and consent to participate.The study protocol was approved by the Ethics Committee of Mashhad University of Medical Sciences (#IR.MUMS.REC.1398.268) after obtaining the required permit for the research. The participants provided a written informed consent and were assured of confidentiality of data. All procedures performed in studies involving human participants were in accordance with the ethical standards of the institutional research committee with the 1964 Helsinki declaration. Before intervention, there were no significant differences (P>0.05) between the intervention and control groups in terms of demographic characteristics (i.e., age, gestational age, education, income, employment status, BMI, history of pre-pregnancy UTI, and vomiting during pregnancy). In this sense, the variables were homogeneous in both groups. The mean (±SD) of age, gestational age, and BMI were 24.80 (±4.92), 13.69 (± 3.82) and 24.93 (±3.18), respectively. Most eligible women were housekeepers (74.5%), low-income families (69.1%) with high school diploma or below (63.6%) ( Table 3 ).  https://doi.org/10.1371/journal.pone.0306558.t003 At the baseline, all UTI preventive behavioral constructs, total preventive behaviors, and self-efficacy were homogeneous in both groups. The results related to UTI preventive behaviors showed a significant improvement (P < 0.05) in all constructs (clothing habits, nutrition, urination, health, and sexual behaviors) in the intervention group at the follow-up, and in all scores changing from baseline to the follow-up. The results of testing self-efficacy showed a significant change (P < 0.05) in the intervention group compared to the control group in the follow-up, and in changes from the baseline to follow-up ( Table 4 ).  https://doi.org/10.1371/journal.pone.0306558.t004 The mean health literacy score immediately after the intervention and three months later was significantly different in the intervention group. The mean score of health literacy was significantly different within the intervention group (p < 0.001). There was no significant difference (P > 0.05) in the change of UTI preventive behavior constructs, total preventive behaviors, self-efficacy, and self-efficacy in the control group at the follow-up ( Table 4 ). The results presented in S3 Table showed that the incidence of UTI three months after the intervention in the control group was 25.4%. The control group significantly had more cases with UTI than the intervention group. In this section, a generalized estimating equation ( GEE ) model was used to simultaneously measure the effect of intervention, time, self-efficacy, and health literacy on UTI preventive behaviors. The results of the GEE model were in line with the bivariate analysis that showed significant interactions between groups and time. S4 and S5 Tables showed the impact of intervention based on health literacy and self-efficacy on improving UTI preventive behaviors in different groups and times. Changes in UTI preventive behavior score within the intervention group were significantly higher than the control (P = 0 <0.001), and UTI preventive behaviors were increased considerably across time in the baseline through follow-up among participants in the intervention group compared with the control (P = 0 <0.001). As the results showed, changes in self-efficacy (p = 0.043) and health literacy (p = 0.042) were significantly associated with UTI preventive behaviors. 4. DiscussionDue to the prevalence of UTI in pregnant women, UTI is considered a major concern in public health public health [ 41 , 43 , 44 ]. The present finding suggests that conducting an educational intervention based on the self-efficacy theory and health literacy skills among pregnant women is an effective intervention to control and prevent UTI because women in the intervention group represented a lower risk of UTI and better preventive behaviors compared with participants in the control group. The present findings showed a significant increase in the level of preventive behaviors in the intervention group. Before the intervention, there was no significant difference between the intervention and control groups. However, after the educational intervention, this difference was statistically significant. The present study showed that the educational intervention based self-efficacy and health literacy skills and the use of educational strategies and programs such as the mastery of alternative behavior and verbal persuasion, educational methods such as goal-setting and role-play were effective in improving preventive behaviors. As the present findings showed, the use of the self-efficacy theory can be effective in improving perceived self-efficacy in individuals. It seems that women with adequate self-efficacy and health literacy may well find and use health information and engage in their care [ 18 , 45 ]. In this study, pregnant women in the intervention group showed a significant change in the mean score of self-efficacy after the intervention. All women learned how to break complex tasks into smaller and simpler activities and set realistic goals to modify their action and commitment to conduct UTI preventive behaviors despite conflicting conditions. Likewise, the present researchers tried to improve mother’s self-confidence and self-monitoring to perform certain behaviors. The existing literature shows that individuals with low self-efficacy use fewer resources of health information and health literacy to improve their health or change the habitual behaviors [ 33 ]. The findings reported by Osborn et al., 2011 are consistent with the present findings, as individuals with higher perceived self-efficacy had a better understanding of their health state and used health information and health literacy to improve their health and show self-care behaviors [ 34 , 46 ]. The results of the present study are in line with a body of research by Hejazi et al. [ 47 ], Abdullahi et al. [ 48 ], which showed a significant effect of self-efficacy on adopting, initiating, and maintaining healthy behavior. They found that self-efficacy acted as a moderator to link healthy behaviors with motivation and knowledge [ 49 , 50 ]. The results of the present study showed statistically significant differences in the change of health literacy skills in the intervention group at 3-months follow-up, and in changes from baseline to follow-up in all scores. Health literacy is the main skill to influence one’s ability to use health information, make well-informed decisions, and maintain good health [ 38 , 40 , 42 , 43 ]. Before the intervention, women had difficulty finding and comprehending health information and healthcare services to make well-health decisions. Likewise, a significant improvement in the health literacy score was found in the intervention group in post-intervention and follow-up. This could be due to the improved women’s willingness and ability to involve in behaviors and care that improve their health. In the present study, a supportive and reliable environment was created to address health information and measures that contribute to a higher stage of well-health decisions and commitments among pregnant women to modify their UTI preventive behaviors [ 4 , 6 ]. Therefore, it is essential to promote health literacy skills in community, as high health literacy is associated with better health outcomes among patients. Therefore, it is necessary to plan and implement model-based educational programs based on the self-efficacy theory and health literacy skills to increase pregnant women’s self-efficacy and health literacy. The results of the present study are in line with a body of research. In a descriptive study conducted on 140 pregnant women in Zahedan based on the Health Belief Model (HBM), Rahimi et al. showed that self-efficacy was the strongest predictor of preventive behaviors against UTI. It seems that the reasons for the greater effect of self-efficacy are women’s self-confidence and awareness of the effect of simple behaviors and measures to control UTI [ 45 , 46 ]. In a quasi-experimental study conducted on 60 mothers to children under 6 years of age, Hashemiparast et al. showed the mean self-efficacy score was increased in the intervention group after the intervention. In this study, self-efficacy implied confidence in one’s ability to perform UTI preventive behaviors [ 47 ]. Eshghi Mutlaq et al. (2016) found that their educational intervention had a significant effect on improving self-care behaviors in mothers with prediabetes during pregnancy, who felt more self-efficacious and capable of understanding their positive state of health. They also showed showed diabetes self-care behaviors in their daily life [ 48 ]. In line with the present study, Ebrahimipour et al. (1994) conducted some research on the effect of an educational intervention based on the self-efficacy theory on the adoption of HIV-AIDS preventive behaviors in high-risk women. This study showed that the educational intervention based on self-efficacy strategies could significantly increase the adoption of self-care behaviors in the intervention group (P<0.001) [ 49 ]. Ha et al. also showed that the educational intervention and the use of educational strategies and programs such as mastery of alternative behavior and verbal persuasion, educational methods such as goal-setting and role-play were effective in improving self-care [ 45 ]. As the present study showed, the use of this theory proved effective in improving perceived self-efficacy in individuals. The findings emphasized the importance of self-efficacy in preventive behaviors as a suitable educational alternative for UTI self-care and prevention in pregnant women. Therefore, it is necessary to plan and implement model-based educational programs to increase pregnant women’s self-efficacy. It seems that women with adequate self-efficacy and health literacy may well find and use health information and well engage in their care [ 18 , 46 , 50 ]. Limited studies exist, investigating the role of self-efficacy and health literacy pandemic conditions influencing awareness and health behaviors among pregnant women. Therefore, further studies need to be conducted on enhancing women’s capability to improve health prevention behaviors toward the different diseases, and focusing on health literacy skills and self-efficacy strategies cause persistent and long-term health behaviors. The strengths of our findings lie in determining the role of self-efficacy and health literacy using valid instrument among the pregnant women as the groups at risk. Our findings highlighted self-efficacy and health literacy skills as the main modifiable determinants to control and manage unborn child’s health and mother’s health because an adequate level of health literacy and self-efficacy improved individual’s healthy behaviors and health outcomes. Future research on intervention-based health literacy and self-efficacy skills will continue in Iran because this type of training for individuals empowers communities to engage in their self-care, improve the healthy behavior, and can increase valuable health outcome in strengthening healthcare delivery. Therefore, it would be worthwhile to study the modifying health literacy and self-efficacy as a long-term measure. In this study, the data collection instrument was self-reporting, which can cause problems such as recall and distraction. Due to the COVID-19 pandemic, the questionnaires were not filled face to face. Instead, the questionnaire hyperlink was shared with the pregnant women to fill out the questionnaires in their convenience. In this type of questionnaire completion, errors occur more often, and the researcher has no control over the respondents, which reduces the number of visits by pregnant women to the health centers, as well as attendance to face-to-face training sessions. Finally, the results of the present study showed that the educational intervention based on the self-efficacy theory and health literacy skills can be effective in improving UTI preventive behaviors. The promotion of UTI preventive behaviors in pregnant women after the intervention showed that holding training sessions based on the self-efficacy theory and health literacy was useful. Such a training can improve preventive behaviors. The results of this research can be used to increase UTI preventive behaviors in all sex and age groups and can reduce recurrent UTI complications. Also, the present findings can help health system managers formulate intervention programs specifically for employees to prevent office infection and increase health indices while maintaining the health of the mother and the fetus. Development of educational programs by managers for health workers aiming to raise the awareness of women visiting health centers can reduce economic and psychological costs imposed on society. Supporting informationS1 table. scherer general self-efficacy questionnaire.. https://doi.org/10.1371/journal.pone.0306558.s001 S2 Table. Distribution of urinary tract infection prevention behaviors.https://doi.org/10.1371/journal.pone.0306558.s002 S3 Table. UTI ratio in control and intervention groups at follow-up.https://doi.org/10.1371/journal.pone.0306558.s003 S4 Table. Effectiveness of the intervention on improving the UTI preventive behaviors via self-efficacy in different group and time period.https://doi.org/10.1371/journal.pone.0306558.s004 S5 Table. Effectiveness of the intervention on improving the UTI preventive behaviors via Health literacy in different group and time period.https://doi.org/10.1371/journal.pone.0306558.s005 AcknowledgmentsThe authors wish to express their gratitude towards the vice president of research in Mashhad University of Medical Sciences, the chiefs and staffs of the health centers and the esteemed participants. - View Article
- Google Scholar
- PubMed/NCBI
- 13. Ratzan S. and Parker R., Health literacy. National library of medicine current bibliographies in medicine. Bethesda: National Institutes of Health, US Department of Health and Human Services, 2000.
 Want to create or adapt books like this? Learn more about how Pressbooks supports open publishing practices. Book Title: Graduate research methods in social workSubtitle: A project-based approach Authors: Matthew DeCarlo; Cory Cummings; and Kate Agnelli 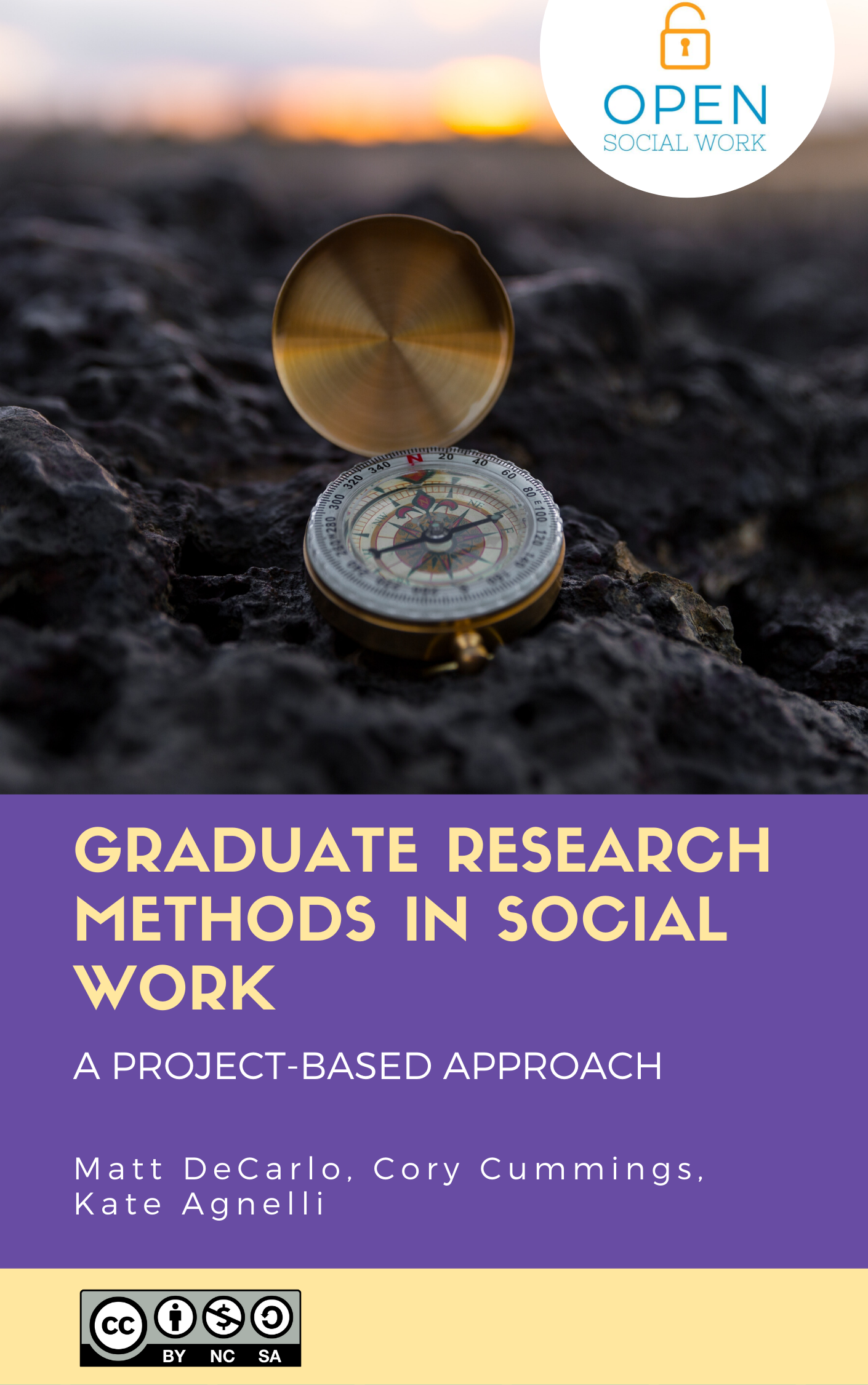 Book Description: Our textbook guides graduate social work students step by step through the research process from conceptualization to dissemination. We center cultural humility, information literacy, pragmatism, and ethics and values as core components of social work research. Book InformationGraduate research methods in social work Copyright © 2021 by Matthew DeCarlo, Cory Cummings, Kate Agnelli is licensed under a Creative Commons Attribution-NonCommercial-ShareAlike 4.0 International License , except where otherwise noted. Social work - Skip to main content
- Skip to FDA Search
- Skip to in this section menu
- Skip to footer links
 The .gov means it’s official. Federal government websites often end in .gov or .mil. Before sharing sensitive information, make sure you're on a federal government site. The site is secure. The https:// ensures that you are connecting to the official website and that any information you provide is encrypted and transmitted securely. U.S. Food and Drug Administration- Search
- Menu
- Science and Research | Drugs
How computational analysis of a 3D mucociliary clearance model can help predict drug uptake and lead to more generic nasal drug productsIn 2020, CDER’s Office of Generic Drugs (OGD) and several partner researchers quantified the effect of drug solubility and partition coefficient on the dissolution and subsequent uptake of drugs in a realistic nasal cavity model. The results provided insight into the possible effects of formulation variables such as solubility, partition coefficient, and particle size on systemic exposure inside the nasal cavity. Complex locally-acting generic drug products, such as some orally inhaled or nasal drug products, can be more challenging for generic drug developers to copy, often leading to a lack of generic competition even after patents and exclusivities no longer block generic drug approval. Accurate and realistic predictions from computer simulations about deposition and absorption of nasally inhaled drugs can provide a deeper understanding of complex fluid-particle dynamics in the nasal cavity which may help OGD clarify regulatory expectations early in the drug development process and during application assessment. While most nasal drug products target local drug delivery to nasal tissues, there is an interest within industry for developing products that target blood-brain barrier (BBB) for rapid delivery to the central nervous system (Pardeshi et al., 2013). As nasal drug products with BBB targeting enter the market, there will be a need for understanding how to assess bioequivalence for proposed generic versions of these products. Currently available models for predicting drug deposition and absorption of nasal drug products such as the model developed by Rygg et al. (2016) have shown promise but are incapable of accurately predicting local absorption. To facilitate accurate local nasal deposition predictions, a three-dimensional (3D) model using computational fluid dynamics (CFD) was developed for this study that includes a paired mucus layer model. Determining How to Enhance Drug Uptake through Clearance, Dissolution, and AbsorptionThe noninvasive nature of intranasal drug administration makes it a widely adopted technique for local and systemic delivery of therapeutic agents. The nasal mucosa, unlike other mucosae, is easily accessible. Intranasal application circumvents the issues of gastrointestinal degradation and hepatic first pass metabolism of the drug (Bitter et al., 2011). However, drugs intended to hit a target site within the nasal cavity are also trapped in the highly viscous gel layer reducing the efficacy of the drug. Soluble drugs, however, dissolve in the mucus layer, diffuse across the gel and sol layers, and are eventually absorbed by the richly vascularized nasal epithelium. This enables a drug to enter the systemic regions through the blood stream without losing efficacy. A computational 3D mucociliary clearance (MCC) model was developed for this study, with the goal of realistically quantifying the effects of drug solubility and partition coefficient on the dissolution and subsequent uptake of drugs in the nasal cavity to achieve a desired therapeutic effect. The results of the study provide insight into the effects of formulation variables like solubility and partition coefficient on systemic exposure inside the nasal cavity. The goal is to eventually enhance drug uptake through a combination of clearance, dissolution, and absorption, as well as drug targeting, to maximize drug uptake. The open-source CFD flow solver toolbox, OpenFOAM version 1706 ( www.openfoam.com ), was employed for the development of the computer simulation model. As part of the design, a novel 3D meshing technique allows the model to smoothly capture the relatively large flow domain as well as the micron-size mucus layer. This efficient meshing strategy drastically reduces the overall meshing time from hours to a matter of minutes. Segmental concentration contours as a visualization tool explain regional trends in cumulative drug uptake. The effects of pharmacokinetic characteristics of hypothetical drugs on the dissolution, subsequent uptake, and clearance were analyzed. A method to impose boundary-driven flow velocity that mimics the beating of the cilia was introduced. Rather than selecting specific drugs, the model was supplied with ranges of parameters that provide a general understanding of how drugs are absorbed in the nasal cavity. Several drug specific parameters, such as solubility, partition coefficient, and particle size, were considered. The effects of particle distribution on MCC and uptake were simulated as well. Velocity ProfilesTo validate the accuracy of the velocity field obtained from the proposed 3D mucus model, inert particle clearance data from the nose obtained from simulations was compared with in vivo data reported by Shah et al. (2015). In this in vivo study, radiolabel tracers were injected as nasal sprays, which deposited on the walls of the nasal cavities. After 15 minutes, they found that 60% of the tracers were removed, consistent with other studies (Naclerio et al., 2003; Bacon et al., 2010) that reported a similar removal percentage. Since the administered radiolabel tracers were not absorbed, they concluded that this removal must be from MCC, confirming deposition to the ciliated posterior regions beyond the nasal valve. The initial positions of the tracers reported by Shah et al. (2015) were used to define the initial positions of inert particles for simulations conducted in this study. About 60% of the inert particles were injected from the posterior, ciliated region and 40% were introduced from the non-ciliated NV region. A transient simulation tracking the trajectory of these inert particles was then run for a duration of 6 hours, consistent with the in vivo study. The mass remaining in the computational domain was calculated and compared with the value reported in the in vivo study. The computational values compare well with those observed in the experimental study. Based on this evidence, it can be concluded that the velocity field obtained from the CFD model is expected to accurately represent MCC, including the effects of local changes in the velocity profile required to maintain a constant ASL thickness. Velocity magnitude is computed at several slices by dividing each slice into the major nasal segments (inferior meatus (IM), inferior turbinate (IT), middle meatus (MM), middle turbinate (MT), olfactory region (OLF)). Each segment is then divided into several evenly spaced subdivisions and the average velocity is calculated at each segment of the slice (see Figure 1). 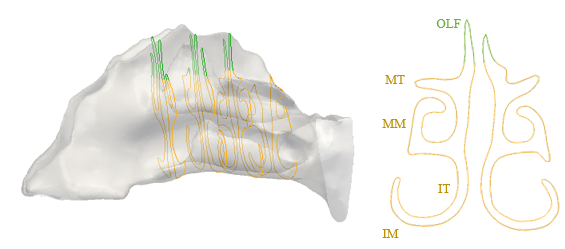 Figure 1: Mucus layer is divided into several slices. Left - Each slice is subdivided into a number of segments: inferior meatus (IM), inferior turbinate (IT), middle meatus (MM), middle turbinate (MT) & olfactory region (OLF). For visualization of the simulation results, the nasal cavity model was divided into three main regions: the anterior nasal vestibule (NV) which is unciliated, the middle passages (MPs), and the posterior nasopharynx. The MPs are further subdivided into different anatomical regions viz. IM, IT, MM, MT, OLF, and the septum (see Figure 2). 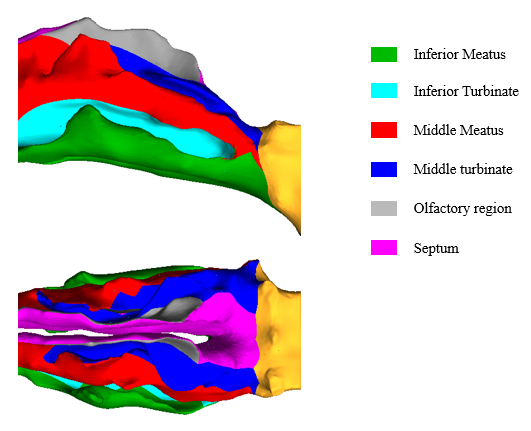 Figure 2: Middle passages (MP) divided into seven segmental regions The drug concentration and uptake in the subsequent sections were analyzed at a slice taken at approximately 50 mm from the nostril (Figure 3). This slice has a large cross section area which make it relatively easier to study trends in the concentration and uptake profiles across the thickness of the mucus layer. In addition, this slice is positioned in such a way that all seven sections of the MP detailed above are encapsulated. Its location closer to the nasopharynx helps in accounting for particles that might escape or get swallowed which otherwise would be difficult to estimate on a slice closer to NV.  Figure 3: Position of the slice (50mm from the nostrils) Findings HighlightsIncreasing the oil-in-water partition coefficient (Ko/w) from 5e-3 to 2 resulted in faster uptake of the dissolved drug in the epithelium. This relatively quicker uptake suggests that drugs with a higher value of Ko/w are more effective when targeting proximal regions in the nasal cavity. On the other hand, decreasing the partition coefficient of a drug leads to increased absorption in the posterior regions of the nasal cavity. However, reducing the partition coefficient drastically can lead to a large amount of drug being swallowed by the patient, which may then be absorbed through the gastrointestinal tract. Thus, based on the intended target site in the mucus layer, drugs with either high or low partition coefficients may be preferred. Particle size is another aspect that influences drug uptake in the epithelium. We compared two different particle sizes (3 µm and 5 µm) and studied their effect on drug uptake in the epithelium. The 3 µm particles dissolved faster than the 5 µm particles and consequently were more readily available for uptake. Also, the larger particles deposited more in the anterior third of the nasal cavity, which is predominantly lined with squamous epithelium, due to inertial impaction. For these particles to be absorbed, the dissolved drug must reach the posterior part of the nasal cavity which is ciliated and lined with columnar epithelial cells. Drug solubility also plays an important role on a drug’s subsequent uptake. As solubility increases, the rate of dissolution and subsequent uptake of the drug also increase. Two different drug solubility values (0.02 mg/ml and 0.2 mg/ml) were considered as model inputs. It was observed that the cumulative uptake for the 0.2 mg/ml drug was appreciably higher than the drug with a 0.02 mg/ml solubility. Typically, drugs with higher solubility tend to dissolve completely. This ensures a higher uptake in the epithelium, but it also makes it difficult for the drug to reach the distal regions of the nasal cavity. Finally, the effect of initial particle deposition location on cumulative drug uptake was studied. Particle deposition trends in the nasal cavity depend on the initial particle size. Larger particles tend to deposit more in the anterior third of the nasal cavity owing to inertial impaction whereas smaller particles (< 5 µm) show a more distributed spread in their initial deposition. Thus, if the goal is to achieve a high uptake rate, smaller sized particles must be selected at the drug formulation stage. Based on these observations, a number of drug formulation parameters can be tweaked to attain the desired rate of uptake. The present results indicate that drug deposition in the unciliated anterior third of the nose precludes active translocation of these drugs to the posterior, distal regions via MCC. Thus, present nasal drug delivery mechanisms may benefit from modification. One potential modification may be to combine current bio-adhesive approaches with targeted drug delivery to pre-determined sites in the nasal cavities. Although the current CFD model lays a solid foundation for drug uptake predictions through the intranasal route, it has certain limitations. The airway surface layer (ASL) in this study consists of a gel layer and a sol layer. The gel layer is modeled as a Newtonian fluid with a high viscosity compared to the sol layer whose viscosity is 10,000 times lower than that in the gel layer. Although the gel layer may exhibit rheological complexities, a Newtonian approximation can be assumed as the local shear rates are relatively small. Future WorkThe model in its current form calculates the cumulative mass of the dissolved drug that is absorbed at the epithelium but does not calculate the plasma concentration profiles. This makes it difficult to fully assess the efficacy of the drug due to MCC in the different systemic regions. To address this limitation, a physiologically-based pharmacokinetics (PBPK) model is planned to simulate the amount of drug that enters both the epithelial cells and the systemic circulation. It will allow for a one-to-one comparison of nasal deposition profiles and blood concentration of the inhaled drugs. This Impact Story is based on the work of Sriram Chari, Karthik Sridhar, Ross Walenga, Clement Kleinstreuer, “Computational analysis of a 3D mucociliary clearance model predicting nasal drug uptake,” in the Journal of Aerosol Science, Volume 155, 2021, https://doi.org/10.1016/j.jaerosci.2021.105757 How does this work advance drug development?The mucociliary clearance model developed by CDER and its research partners can help us to rationally predict the effects of changes in the properties of nasally delivered drug candidates on drug exposures in patients. This capability can aid in the development of new safe and effective nasally inhaled drugs as well as generics of these complex products. - Bacon R, Newman S, Rankin L, Pitcairn G, Whiting R. 2012. Pulmonary and nasal deposition of ketorolac tromethamine solution (SPRIX) following intranasal administration. International journal of pharmaceutics 431(1-2):39-44.
- Bitter C, Suter-Zimmermann K, Surber C. 2011. Nasal drug delivery in humans. Karger Publishers, Topical Applications and the Mucosa; p. 20-35.
- Blake J. 1972. A model for the micro-structure in ciliated organisms. Journal of Fluid Mechanics 55(1):1-23.
- Fulford GR, Blake JR. 1986. Muco-ciliary transport in the lung. Journal of theoretical biology 121(4):381-402.
- Funk JR, Hall GW, Crandall JR, Pilkey WD. 2000. Linear and quasi-linear viscoelastic characterization of ankle ligaments. Journal of Biomechanical Engineering 122(1):15-22.
- King M, Agarwal M, Shukla JB. 1993. A planar model for mucociliary transport: effect of mucus viscoelasticity. Biorheology 30(1):49-61.
- Naclerio RM, Baroody FM, Bidani N, Marcy De T, Penney BC. 2003. A comparison of nasal clearance after treatment of perennial allergic rhinitis with budesonide and mometasone. Otolaryngology Head and Neck Surgery 128(2):220-227.
- Pardeshi CV, Belgamwar VS. 2013. Direct nose to brain drug delivery via integrated nerve pathways bypassing the blood–brain barrier: an excellent platform for brain targeting. Expert opinion on drug delivery 10(7):957-72.
- Sedaghat MH, Shahmardan MM, Norouzi M, Nazari M, Jayathilake PG. 2016. On the effect of mucus rheology on the muco-ciliary transport. Mathematical biosciences 272:44-53.
- Shah SA, Berger RL, McDermott J, Gupta P, Monteith D, Connor A, Lin W, editors. 2015. Regional deposition of mometasone furoate nasal spray suspension in humans. Allergy & Asthma Proceedings.
- Sleigh MA, Blake JR, Liron N. 1988. The propulsion of mucus by cilia. American Review of Respiratory Disease 137(3):726-741.
- Smith DJ, Gaffney EA, Blake JR. 2008. Modelling mucociliary clearance. Respiratory physiology & neurobiology 163(1-3):178-188.
Iowa Reading Research Center Research Article of the Month: August 2024This blog post is part of our Research Article of the Month series. For this month, we highlight “ Teacher Professional Development and Student Reading Achievement: A Meta-Analytic Review of the Effects ,” an article published in Journal of Research on Educational Effectiveness in 2019. Important words related to research are bolded, and definitions of these terms are included at the end of the article in the “Terms to Know” section. Why Did We Pick This Paper?Research suggests that high-quality teachers play a significant role in student achievement—higher than any other school factor (Hattie, 2009). One way to build teacher capacity is through professional development (PD)—training on current, effective, evidence-based instructional methods. PD can take different forms, including workshops, professional learning communities, coaching, online training, or conference attendance. High-quality PD can improve teachers’ knowledge and skills and change their attitudes and beliefs. This, in turn, can affect their instruction and practices, which is likely to positively impact student learning (Desimone, 2009). This study measures the effects of teacher PD on student reading outcomes. Researchers also examine moderators that may influence these outcomes, including characteristics of the study design (e.g., experimental design, student outcomes measured), PD opportunities (e.g., intensity, delivery method, level of collaboration, format), teachers (e.g., years of experience, certifications and degrees), and students (e.g., disability status, grade level). Findings from this study may help districts identify and provide high-quality PD that builds teacher knowledge and supports student reading achievement. What Are the Research Questions or Purpose?The researchers examined the impact of teacher PD on student reading outcomes by addressing the following research questions: - What are the effects of PD on reading achievement for students in Grades K–8?
What elements of study design are potential moderators of effects?What characteristics of professional development are potential moderators of effects, what characteristics of participants, both teacher and student, are potential moderators of effects, what methodology do the authors employ. The authors conducted a meta-analysis of 28 quantitative research studies that examined the impacts of teacher PD on student reading outcomes. To be included in the analysis, the studies needed to: - Be conducted in a K–8 setting
- Examine teacher PD as the independent variable
- Examine student reading achievement (e.g., phonological awareness, decoding, word identification, fluency, vocabulary, or comprehension) as the dependent variable
- Utilize an experimental or quasi-experimental design
- Include effect sizes or the ability to calculate them
- Be published in a peer-reviewed journal in English between 1975 and 2017
For each of the included studies, researchers examined the students’ performance on a reading outcome. These outcomes were classified as either code-focused (e.g., phonological awareness, decoding, word identification, fluency), meaning-focused (e.g., comprehension, vocabulary), or general reading ability. Researchers also took into account other variables in the studies that could affect the outcomes of PD. These variables included: - Experimental design ( randomized control trials , quasi-experimental design)
- Student outcomes measured (code-focused or meaning-focused)
- Intensity (number of hours of PD)
- Delivery method (district staff, researchers, online)
- Level of collaboration and active participation
- Format (whole group, summer workshop, professional learning community, coaching)
- Average years of teaching experience
- Percentage of teachers with advanced degrees
- Disability status
- Grade level
The researchers estimated effect sizes of teacher PD on student reading outcomes using a random effects model . They also examined the relationships between potential moderators (i.e., study, PD, teacher, and student characteristics) and student outcomes. What Are the Key Findings?What are the effects of pd on reading achievement for students in grades k – 8. Overall, the analysis results of the study indicate that teacher PD positively impacted student reading outcomes in reading (g = 0.18). However, this is the average effect size, and there is notable variation in effect sizes reported from the primary studies included in this meta-analysis. This indicates that some kinds of PD were more effective than others. For example, Teacher Study Group (TSG), a PD model, had a medium to large positive effect on student reading outcomes (Gersten et al., 2010) whereas other PD models had small or no effect. - For randomized controlled trials (g = 0.18) and quasi-experimental design studies (g = 0.19), teacher PD significantly improved student outcomes in reading.
- Teacher PD significantly improved both code-focused student outcomes (g = 0.22) and meaning-focused student outcomes (g = 0.17).
The PD characteristics examined did not significantly moderate the effect between PD and reading outcomes. The teacher and student characteristics examined did not significantly moderate the effect between teacher PD and reading outcomes. What Are the Practical Applications of Key Findings?Findings suggest that teacher PD generally has a positive effect on student reading achievement in Grades K–8. It is worth noting that the average total length of teacher PD was around 52 hours, with a range of 4 to 295 hours across studies, and these PD opportunities were associated with varying levels of impact on student outcomes. This wide range indicates that a certain level of intensity and duration may be necessary for teacher PD to have a significant effect on student outcomes, although there is no agreement among researchers on the level of intensity required to effectively enhance teacher knowledge and practices and produce improved student outcomes. When providing reading and literacy PD for teachers, it is important to ensure that the content is both meaningful and relevant to the teacher’s instructional needs. Additionally, allocating sufficient time to PD can help maximize its benefits for teachers and students. What Are the Limitations of This Paper?When examining the relationships between the moderators and the student reading outcomes, teacher characteristics such as teaching experience and advanced degrees were included in the moderator analysis. However, teacher knowledge and skills, another important part of teacher characteristics, was not considered or included in the analysis. Teacher knowledge and skills has been shown to potentially influence student learning and, ultimately, their reading outcomes (Soodla, Jogi & Kikas, 2017; Porter et al., 2023). Future research should incorporate this characteristic to provide a more comprehensive understanding of the factors that contribute to student outcomes, as the primary goal of teacher PD programs is to enhance both teacher knowledge and skills. In addition, there is a lack of data on students with disabilities and secondary students across studies. Only one study included in the meta-analysis examined reading outcomes for students with reading difficulties, and there were few studies at the secondary level. It remains unclear whether teacher PD could effectively address the needs of students with disabilities, who require high-quality, specialized instructional strategies to support their reading. Future studies should explore the impact of PD on teacher’s ability to support these students. Terms to Know- Moderator: Moderators are variables that affect the relationship between two other variables. For example, the relationship between the length of a reading intervention and reading comprehension may be stronger for students who are at risk for reading disabilities versus students who are not at risk. In this case, at-risk status would be a moderator.
- Independent variable: An independent variable is a factor that influences dependent variables in experimental studies. For example, the length of a reading intervention in total minutes (independent variable) may affect a student’s composite reading score (dependent variable). They are called “independent” because they are manipulated by the experimenter and therefore independent of other influences.
- Dependent variable: Dependent variables are factors that may change in response to an independent variable. For example, a student’s composite reading score (dependent variable) may change in response to the length of reading intervention they receive in total minutes (independent variable).
- Experimental: Experimental research aims to determine whether a certain treatment influences a measurable outcome—for example, whether a certain instructional method influences students’ reading comprehension scores. To do this, participants are divided into two groups: an experimental group, which receives the treatment, and a control group, which does not receive the treatment. In an experimental study, these groups are randomly assigned, meaning each participant has equal probability of being in either the treatment or the control group. Both groups are tested before and after the treatment, and their results are compared. Because participants are randomly assigned to a control group, this kind of study is also known as a randomized control trial .
- Quasi-experimental: A quasi-experimental study is similar to an experimental study except that participants are not randomly assigned to groups. In educational research, groups often are assigned by classroom rather than through random assignment, making this kind of research quasi-experimental.
- Effect size: In statistics, effect size is a measure of the strength of the relationship between two variables in statistical analyses. A commonly used interpretation is to refer to effect size as small (g = 0.2), medium (g = 0.5), and large (g = 0.8) based on the benchmarks suggested by Cohen (1988), where “g” refers to Hedge’s g, a statistical measure of effect size.
- Peer-reviewed journal: When an author submits an article to a peer-reviewed journal , the article is reviewed by scholars in the field. They make sure that the article is accurate, relevant, high quality, and well written.
- Randomized control trial: See experimental.
- Random effects model: A random effects model is a type of statistical model that measures how an independent variable affects a dependent variable across a number of different samples or studies. Unlike a fixed effects model, a random effects model accounts for variability between different groups in a dataset.
Cohen, J. (1988). Statistical power analysis for the behavioral sciences . Routledge. Desimone, L. M. (2009). Improving impact studies of teachers’ professional development: Toward better conceptualizations and measures. Educational Researcher , 38 (3), 181–199. https://doi.org/10.3102/0013189X08331140 Didion, L., Toste, J. R., & Filderman, M. J. (2019). Teacher professional development and student reading achievement: A meta-analytic review of the effects. Journal of Research on Educational Effectiveness , 13 (1), 29–66. https://doi.org/10.1080/19345747.2019.1670884 Hattie, J. A. (2009). Visible learning: A synthesis of 800+ meta-analyses on achievement . Routledge. Gersten, R., Dimino, J., Jayanthi, M., Kim, J. S., & Santoro, L. E. (2010). Teacher study group: Impact of the professional development model on reading instruction and student outcomes in first grade classrooms. American Educational Research Journal , 47 (3), 694-739. https://doi.org/10.3102/0002831209361208 Porter, S. B., Odegard, T. N., Farris, E. A., & Oslund, E. L. (2023). Effects of teacher knowledge of early reading on students’ gains in reading foundational skills and comprehension. Reading and Writing , 1-17. https://doi.org/10.1007/s11145-023-10448-w Soodla, P., Jõgi, A. L., & Kikas, E. (2017). Relationships between teachers’ metacognitive knowledge and students’ metacognitive knowledge and reading achievement. European Journal of Psychology of Education , 32 , 201-218. - professional development
- professional learning communities
- Research Article of the Month
 Research Article of the Month: June 2024 Research Article of the Month: May 2024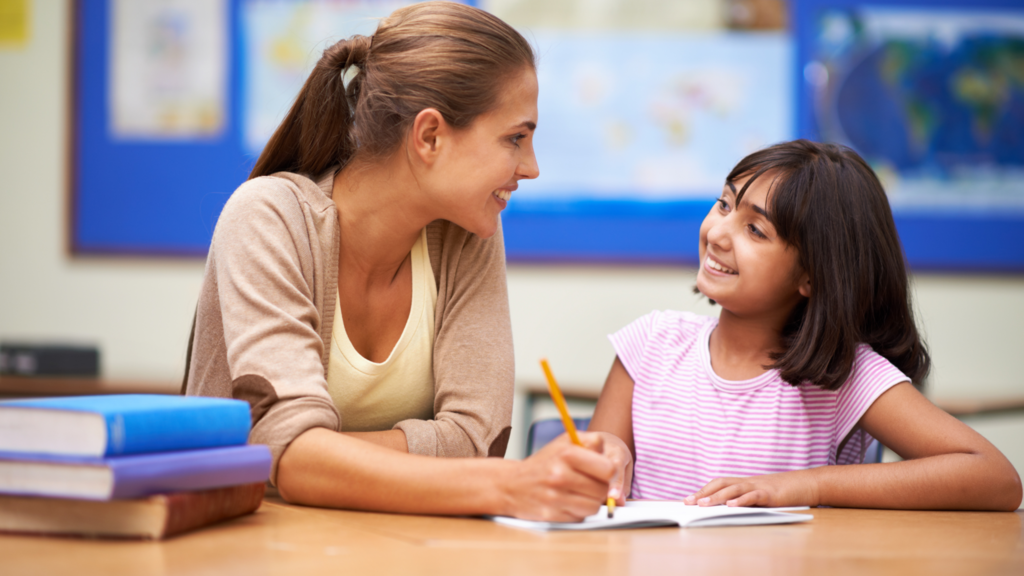 Research Article of the Month: April 2024 2024 Externally Published Research- Center for Financial Research
- Researchers
- Research Fellows
- Senior Advisor
- Special Advisor
- 2023 Fellows
- 2022 Fellows
- Visiting Scholar
- Working Papers
- 23rd Bank Research Conference
- Conference Videos
- Speaker Information
- Poster Videos
- Poster Session Videos
- Previous Conference Programs
- Other Conferences
- 2021-2022 FDIC Academic Challenge
- 2020-2021 FDIC Academic Challenge
- Prior Years
- Research Assistants
- Internships
- Visiting Scholars
Published 2024 |
---|
By George F. Shoukry (Federal Deposit Insurance Corporation) , March 2024, Volume 59, Issue 2, 896-932 | By Levent Güntay (Özyeğin University), Stefan Jacewitz (Federal Reserve Bank of Kansas City), and Jonathan Pogach (Federal Deposit Insurance Corporation) , March-April 2024, Volume 56, Issue 2-3, 537-538 | Ryan M. Goodstein (Federal Deposit Insurance Corporation) and Mark J. Kutzbach (Federal Deposit Insurance Corporation) , Forthcoming | Sumit Agarwal (National University of Singapore), Souphala Chomisisengphet (Office of the Comptroller of the Currency), Hua Kiefer (Federal Deposit Insurance Corporation), Leonard C. Kiefer (Freddie Mac), and Paolina C. Medina (Texas A&M University) , Forthcoming | Christopher Martin (Federal Deposit Insurance Corporation), Manju Puri (Duke University, Federal Deposit Insurance Corporation, National Bureau of Economic Research), and Alexander Ufier (Federal Deposit Insurance Corporation) , Forthcoming |
Last Updated: August 4, 2024 InformationInitiativesYou are accessing a machine-readable page. In order to be human-readable, please install an RSS reader. All articles published by MDPI are made immediately available worldwide under an open access license. No special permission is required to reuse all or part of the article published by MDPI, including figures and tables. For articles published under an open access Creative Common CC BY license, any part of the article may be reused without permission provided that the original article is clearly cited. For more information, please refer to https://www.mdpi.com/openaccess . Feature papers represent the most advanced research with significant potential for high impact in the field. A Feature Paper should be a substantial original Article that involves several techniques or approaches, provides an outlook for future research directions and describes possible research applications. Feature papers are submitted upon individual invitation or recommendation by the scientific editors and must receive positive feedback from the reviewers. Editor’s Choice articles are based on recommendations by the scientific editors of MDPI journals from around the world. Editors select a small number of articles recently published in the journal that they believe will be particularly interesting to readers, or important in the respective research area. The aim is to provide a snapshot of some of the most exciting work published in the various research areas of the journal. Original Submission Date Received: . - Active Journals
- Find a Journal
- Proceedings Series
- For Authors
- For Reviewers
- For Editors
- For Librarians
- For Publishers
- For Societies
- For Conference Organizers
- Open Access Policy
- Institutional Open Access Program
- Special Issues Guidelines
- Editorial Process
- Research and Publication Ethics
- Article Processing Charges
- Testimonials
- Preprints.org
- SciProfiles
- Encyclopedia
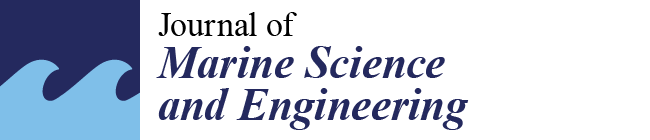 Article Menu - Subscribe SciFeed
- Recommended Articles
- Google Scholar
- on Google Scholar
- Table of Contents
Find support for a specific problem in the support section of our website. Please let us know what you think of our products and services. Visit our dedicated information section to learn more about MDPI. JSmol ViewerExperimental research on the low-cycle fatigue crack growth rate for a stiffened plate of eh36 steel for use in ship structures. 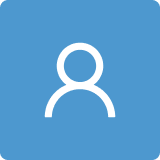 1. Introduction2. low cycle fatigue crack growth experiment for stiffened plate, 3. result and discussion, 3.1. experimental results of stiffened plates with single-edge crack, 3.2. experimental results of stiffened plates with central crack, 4. conclusions, author contributions, institutional review board statement, informed consent statement, data availability statement, conflicts of interest. - Dong, Q.; Xu, G.; Zhao, J.; Hu, Y. Experimental and numerical study on crack propagation of cracked plates under low cycle fatigue loads. J. Mar. Sci. Eng. 2023 , 11 , 1436. [ Google Scholar ] [ CrossRef ]
- Dong, Q.; Lu, X.; Xu, G. Experimental study on low-cycle fatigue characteristics of marine structural steel. J. Mar. Sci. Eng. 2024 , 12 , 651. [ Google Scholar ] [ CrossRef ]
- Gan, J.; Sun, D.; Deng, H.; Wang, Z.; Wang, X.; Yao, L.; Wu, W. Fatigue characteristics of designed T-type specimen under two-step repeating variable amplitude load with low-amplitude load below the fatigue limit. J. Mar. Sci. Eng. 2021 , 9 , 107. [ Google Scholar ] [ CrossRef ]
- Wang, Q.; Huber, N.; Liu, X.; Kashaev, N. On the analysis of plasticity induced crack closure in welded specimens: A mechanism controlled by the stress intensity factor resulting from residual stresses. Int. J. Fatigue 2022 , 162 , 106940. [ Google Scholar ] [ CrossRef ]
- Sistaninia, M.; Kolednik, O. A novel approach for determining the stress intensity factor for cracks in multilayered cantilevers. Eng. Fract. Mech. 2022 , 266 , 108386. [ Google Scholar ] [ CrossRef ]
- Nassiraei, H.; Rezadoost, P. Stress concentration factors in tubular T-joints reinforced with external ring under in-plane bending moment. Ocean. Eng. 2022 , 266 , 112551. [ Google Scholar ] [ CrossRef ]
- Nassiraei, H.; Rezadoost, P. Probabilistic analysis of the SCFs in tubular T/Y-joints reinforced with FRP under axial, in-plane bending, and out-of-plane bending loads. Structures 2022 , 35 , 1078–1097. [ Google Scholar ] [ CrossRef ]
- Nassiraei, H.; Rezadoost, P. Stress concentration factors in tubular T/Y-connections reinforced with FRP under in-plane bending load. Mar. Struct. 2021 , 76 , 102871. [ Google Scholar ] [ CrossRef ]
- Nassiraei, H.; Rezadoost, P. Static capacity of tubular X-joints reinforced with fiber reinforced polymer subjected to compressive load. Eng. Struct. 2021 , 236 , 112041. [ Google Scholar ] [ CrossRef ]
- Nassiraei, H.; Rezadoost, P. Stress concentration factors in tubular T/Y-joints strengthened with FRP subjected to compressive load in offshore structures. Int. J. Fatigue 2020 , 140 , 105719. [ Google Scholar ] [ CrossRef ]
- Dong, Q.; Yang, P.; Xu, G. Low cycle fatigue crack growth analysis by CTOD under variable amplitude loading for AH32 steel. Mar. Struct. 2019 , 63 , 257–268. [ Google Scholar ] [ CrossRef ]
- Dowling, N.E. Geometry effects and the J-integral approach to elastic-plastic fatigue crack growth. In Cracks and Fracture ; Swedlow, J., Williams, M., Eds.; ASTM STP 601; American Society for Testing and Materials: Philadelphia, PA, USA, 1976; pp. 19–32. [ Google Scholar ]
- Gonzales, G.L.G.; González, J.A.O.; Antunes, F.V.; Neto, D.M.; Díaz, F.A. Experimental determination of the reversed plastic zone around fatigue crack using digital image correlation. Theor. Appl. Fract. Mech. 2023 , 125 , 103901. [ Google Scholar ] [ CrossRef ]
- Dunham, F.W. Fatigue Testing of Large-Scale Models of Submarine Structural Details. Mar. Technol. SNAME News 1965 , 2 , 299–307. [ Google Scholar ] [ CrossRef ]
- Chen, L.; Chen, X. The low cycle fatigue tests on submarine structures. Ship Sci. Technol. 1991 , 2 , 19–20. [ Google Scholar ]
- Li, C. Research on low cycle fatigue properties of several types of steel for submarine pressure shell. Dev. Appl. Mater. 1986 , 12 , 28–37. [ Google Scholar ]
- Liu, Y.; Zhu, X.; Huang, X. Experimental research on low frequency fatigue crack propagation rate of 921A hull steel structure. J. Nav. Univ. Eng. 2008 , 20 , 69–74. [ Google Scholar ]
- Jandejsek, I.; Gajdoš, L.; Šperl, M.; Vavřík, D. Analysis of standard fracture toughness test based on digital image correlation data. Eng. Fract. Mech. 2017 , 182 , 607–620. [ Google Scholar ] [ CrossRef ]
- Zhang, W.; Liu, Y. Plastic zone size estimation under cyclic loadings using in situ optical microscopy fatigue testing. Fatigue Fract. Eng. Mater. Struct. 2011 , 34 , 717–727. [ Google Scholar ] [ CrossRef ]
- Vasco-Olmo, J.M.; Díaz, F.A.; Antunes, F.V.; James, M.N. Characterization of fatigue crack growth using digital image correlation measurements of plastic CTOD. Theor. Appl. Fract. Mech. 2019 , 101 , 332–341. [ Google Scholar ] [ CrossRef ]
- Belytschko, T.; Black, T. Elastic crack growth in finite elements with minimal remeshing. Int. J. Numer. Methods Eng. 1999 , 45 , 601–620. [ Google Scholar ] [ CrossRef ]
- Melenk, J.M.; Babuška, I. The partition of unity finite element method: Basic theory and applications. Comput. Methods Appl. Mech. 1996 , 139 , 289–314. [ Google Scholar ] [ CrossRef ]
- He, L.; Liu, Z.; Gu, J.; Wang, J.; Men, K. Fatigue crack propagation path and life prediction based on XFEM. J. Northwestern Polytech. Univ. 2019 , 37 , 737–743. [ Google Scholar ] [ CrossRef ]
- Tu, W.; Yue, J.; Xie, H.; Tang, W. Fatigue crack propagation behavior of high-strength steel under variable amplitude loading. Eng. Fract. Mech. 2021 , 247 , 107642. [ Google Scholar ] [ CrossRef ]
- Huang, X.; Zhang, X.; Bai, G.; Xu, W.; Wang, H. Residual strength analysis of thin-walled structures with multiple site damage based on crack tip opening angle method. J. Shanghai Jiao Tong Univ. 2013 , 47 , 519–524+531. [ Google Scholar ]
- Hwang, J.H.; Kim, H.T.; Kim, Y.J.; Nam, H.S.; Kim, J.W. Crack tip fields at crack initiation and growth under monotonic and large amplitude cyclic loading: Experimental and FE analyses. Int. J. Fatigue 2020 , 141 , 105889. [ Google Scholar ] [ CrossRef ]
- Xiong, K.; Deng, J.; Pei, Z.; Yang, P.; Dong, Q. Analysis of accumulative plasticity and fracture behavior of hull cracked plates subjected to biaxial low-cycle fatigue loading. J. Ship Mech. 2022 , 26 , 113–124. [ Google Scholar ]
- Wei, X. Fatigue Reliability Analysis of Ship Stiffened Panel Structure Subjected to Multiple Cracks. Master’s Thesis, Harbin Engineering University, Harbin, China, 2017. [ Google Scholar ]
- Soares, C.G.; Garbatov, Y.; Safety, S. Fatigue reliability of the ship hull girder accounting for inspection and repair. Reliab. Eng. 1996 , 51 , 341–351. [ Google Scholar ] [ CrossRef ]
- Duncheva, G.; Maximov, J.; Ganev, N.; Ivanova, M. Fatigue life enhancement of welded stiffened S355 steel plates with noncircular openings. J. Constr. Steel Res. 2015 , 112 , 93–107. [ Google Scholar ] [ CrossRef ]
- Lei, J.; Yue, J.; Xu, Z.; Fang, X.; Liu, H. Theoretical and Experimental Analysis on Low-Cycle Fatigue Crack Initiation for High Strength Steel Stiffened Plates. In Proceedings of the ASME 2022 41st International Conference on Ocean, Offshore and Arctic Engineering, Hamburg, Germany, 5–10 June 2022. [ Google Scholar ]
- Dexter, R.J.; Pilarski, P.J. Crack propagation in welded stiffened panels. J. Constr. Steel Res. 2002 , 58 , 1081–1102. [ Google Scholar ] [ CrossRef ]
- Jiang, W.; Yang, P. Experimental studies on crack propagation and accumulative mean strain of cracked stiffened plates under low-cycle fatigue loads. Ocean. Eng. 2020 , 214 , 107744. [ Google Scholar ] [ CrossRef ]
- Song, Y.; Yang, P.; Hu, K.; Jiang, W.; Zhang, G. Study of low-cycle fatigue crack growth behavior of central-cracked stiffened plates. Ocean. Eng. 2021 , 241 , 110083. [ Google Scholar ] [ CrossRef ]
- Dong, Q.; Yang, P.; Deng, J.L.; Wang, D. The theoretical and numerical research on CTOD for ship plate under cyclic loading considering accumulative plastic strain. J. Ship Mech. 2015 , 19 , 1507–1516. [ Google Scholar ]
- Deng, J.; Yang, P.; Dong, Q.; Wang, D. Research on CTOD for low cycle fatigue analysis of central through cracked plates considering accumulative plastic strain. Eng. Fract. Mech. 2016 , 154 , 128–139. [ Google Scholar ] [ CrossRef ]
- Dong, Q.; Yang, P.; Xu, G.; Deng, J.L. Mechanisms and modeling of low cycle fatigue crack propagation in a pressure vessel steel Q345. Int. J. Fatigue 2016 , 89 , 2–10. [ Google Scholar ] [ CrossRef ]
Click here to enlarge figure Elastic Modulus/GPa | Poisson’s Ratio | Yield Stress/MPa | Ultimate Tensile Strength/MPa |
---|
206 | 0.3 | 434.94 | 548.91 | Specimen Number | P /kN | R = P /P | Nominal Stress/MPa | Crack Location | Stiffener Height |
---|
P1 | 84.24 | −1 | 120 | single-edge crack | 30 mm | P2 | 90.72 | −1 | 130 | single-edge crack | 30 mm | P3 | 97.20 | −1 | 140 | single-edge crack | 30 mm | P4 | 384.00 | 0.031 | 280 | central crack | 30 mm | P5 | 420.00 | 0.2 | 300 | central crack | 30 mm | P6 | 420.00 | 0.2 | 300 | central crack | 0 mm | | The statements, opinions and data contained in all publications are solely those of the individual author(s) and contributor(s) and not of MDPI and/or the editor(s). MDPI and/or the editor(s) disclaim responsibility for any injury to people or property resulting from any ideas, methods, instructions or products referred to in the content. |
Share and CiteDong, Q.; Xu, G.; Chen, W. Experimental Research on the Low-Cycle Fatigue Crack Growth Rate for a Stiffened Plate of EH36 Steel for Use in Ship Structures. J. Mar. Sci. Eng. 2024 , 12 , 1365. https://doi.org/10.3390/jmse12081365 Dong Q, Xu G, Chen W. Experimental Research on the Low-Cycle Fatigue Crack Growth Rate for a Stiffened Plate of EH36 Steel for Use in Ship Structures. Journal of Marine Science and Engineering . 2024; 12(8):1365. https://doi.org/10.3390/jmse12081365 Dong, Qin, Geng Xu, and Wei Chen. 2024. "Experimental Research on the Low-Cycle Fatigue Crack Growth Rate for a Stiffened Plate of EH36 Steel for Use in Ship Structures" Journal of Marine Science and Engineering 12, no. 8: 1365. https://doi.org/10.3390/jmse12081365 Article MetricsArticle access statistics, further information, mdpi initiatives, follow mdpi. 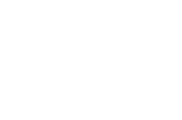 Subscribe to receive issue release notifications and newsletters from MDPI journals 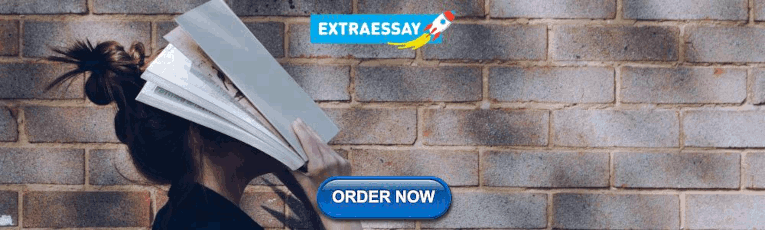 |
IMAGES
COMMENTS
Revised on January 22, 2024. Like a true experiment, a quasi-experimental design aims to establish a cause-and-effect relationship between an independent and dependent variable. However, unlike a true experiment, a quasi-experiment does not rely on random assignment. Instead, subjects are assigned to groups based on non-random criteria.
In summary, 2-group tests, regression analysis, and time-series analysis can accommodate interrupted time-series quasi-experimental data. However, statistical validity depends on using appropriate methods for the study question, meeting data requirements, and verifying modeling assumptions.
A significant advantage of quasi-experimental research over purely observational studies and correlational research is that it addresses the issue of directionality, determining which variable is the cause and which is the effect. In quasi-experiments, an intervention typically occurs during the investigation, and the researchers record outcomes before and after it, increasing the confidence ...
Quasi-experimental design is a research method that seeks to evaluate the causal relationships between variables, but without the full control over the independent variable (s) that is available in a true experimental design. In a quasi-experimental design, the researcher uses an existing group of participants that is not randomly assigned to ...
Quasi-experimental research is a quantitative researchmethod. It involves numerical data collection and statistical analysis. Quasi-experimental research compares groups with different circumstances or treatments to find cause-and-effect links. It draws statistical conclusions from quantitative data.
A quasi-experimental study (also known as a non-randomized pre-post intervention) is a research design in which the independent variable is manipulated, but participants are not randomly assigned to conditions. Commonly used in medical informatics (a field that uses digital information to ensure better patient care), researchers generally use ...
Describe three different types of quasi-experimental research designs (nonequivalent groups, pretest-posttest, and interrupted time series) and identify examples of each one. The prefix quasi means "resembling.". Thus quasi-experimental research is research that resembles experimental research but is not true experimental research.
In medical informatics, the quasi-experimental, sometimes called the pre-post intervention, design often is used to evaluate the benefits of specific interventions. The increasing capacity of health care institutions to collect routine clinical data has led to the growing use of quasi-experimental study designs in the field of medical ...
The prefix quasi means "resembling." Thus quasi-experimental research is research that resembles experimental research but is not true experimental research. Although the independent variable is manipulated, participants are not randomly assigned to conditions or orders of conditions (Cook et al., 1979).Because the independent variable is manipulated before the dependent variable is ...
First, it can allow for data analysis strategies that can incorporate cyclical temporal trends (such as seasonality-mediated risk for the outcome, such as with flu or malaria) or other underlying temporal trends. ... "Experimental and Quasi-Experimental Designs for Research on Teaching." In Gage NL (ed.), Handbook of Research on Teaching ...
Event History and Survival Analysis; Age-Period-Cohort Analysis; Qualitative Longitudinal Research; Attrition; Time Series Analysis; Dynamic Panel Data Models; Conditional Logit Model; Causal Analysis With Panel Data; Sequence Analysis; Latent Transition Analysis; Quasi-Experimental Designs; Yule, George Udny; Lazarsfeld, Paul F. Panel Data ...
Quasi-experimental research designs are the most widely used research approach employed to evaluate the outcomes of social work programs and policies. This new volume describes the logic, design ...
A quasi-experiment is an empirical interventional study used to estimate the causal impact of an intervention on target population without random assignment. Quasi-experimental research shares similarities with the traditional experimental design or randomized controlled trial, but it specifically lacks the element of random assignment to ...
Citation. Reichardt, C. S. (2019). Quasi-experimentation: A guide to design and analysis. The Guilford Press. Abstract. This volume explains the logic of both the design of quasi-experiments and the analysis of the data they produce to provide estimates of treatment effects that are as credible as can be obtained given the demanding constraints of research practice.
All said and done, the QE research design is best avoided because it is flawed and because even the best statistical approaches to data analysis would be imperfect. The QE design should be considered only when no other options are available. Readers are referred to Harris et al. 5 for a further discussion on QE studies.
The cardiometabolic risk factors that were measured included blood pressure, insulin resistance, triglyercides, and HDL. Additional data was collected such as BMI and blood levels of vitamin D as serum 25(OH)D. Baseline measurements were taken, as well as measurements at 6 months and. 12 months (end of study).
23 Quasi-experimental. 23. Quasi-experimental. In most cases, it means that you have pre- and post-intervention data. Great resources for causal inference include Causal Inference Mixtape and Recent Advances in Micro, especially if you like to read about the history of causal inference as a field as well (codes for Stata, R, and Python ...
Keywords: Quasi-experimental study, research design, univariable analysis, multivariable regression, confounding variables If we wish to study how antidepressant drug treatment affects outcomes in pregnancy, we should ideally randomize depressed pregnant women to receive an antidepressant drug or placebo; this is a randomized controlled trial ...
The prefix quasi means "resembling." Thus quasi-experimental research is research that resembles experimental research but is not true experimental research. Although the independent variable is manipulated, participants are not randomly assigned to conditions or orders of conditions (Cook & Campbell, 1979). [1] Because the independent variable is manipulated before the dependent variable ...
An experimental design is a randomized study design used to evaluate the effect of an intervention. In its simplest form, the participants will be randomly divided into 2 groups: A treatment group: where participants receive the new intervention which effect we want to study. A control or comparison group: where participants do not receive any ...
This course surveys quantitative methods to make causal inferences in the absence of randomized experiment including the use of natural and quasi-experiments, instrumental variables, regression discontinuity, fixed effects estimators, and difference-in-differences. We emphasize the proper interpretation of these research designs and critical engagement with their key assumptions for applied ...
III. Matching in Quasi-Experimental Designs: Normative Group Equivalence. Because of the problems in selecting people in a normative group matching design and the potential problems with the data analysis of that design, you may want to make the normative comparison group equivalent on selected demographic characteristics. You might want the same proportion of males and females, and the mean ...
A quasi-experimental study was conducted from January to July 2021 among pregnant women residing in Mashhad, Iran. To this aim, 110 pregnant women at a gestational age of 12-18 weeks were randomly assigned to a control (n = 55) and an intervention group (n = 55) and completed all questionnaires during the intervention and the 3-month follow-up.
13.4 Quasi-experimental designs; 13.5 Non-experimental designs; 13.6 Critical, ethical, and cultural considerations; ... 17.3 Finding the right qualitative data to answer my research question; 17.4 How to gather a qualitative sample; 17.5 What should my sample look like? ... A survey of approaches to qualitative data analysis. 19.1 Ethical ...
To validate the accuracy of the velocity field obtained from the proposed 3D mucus model, inert particle clearance data from the nose obtained from simulations was compared with in vivo data ...
To be included in the analysis, the studies needed to: Be conducted in a K-8 setting; Examine teacher PD as the independent variable; Examine student reading achievement (e.g., phonological awareness, decoding, word identification, fluency, vocabulary, or comprehension) as the dependent variable ; Utilize an experimental or quasi-experimental ...
Insurance Pricing, Distortions, and Moral Hazard: Quasi-Experimental Evidence from Deposit Insurance By George F. Shoukry (Federal Deposit Insurance Corporation) Journal of Financial and Quantitative Analysis , March 2024, Volume 59, Issue 2, 896-932
To ensure the validity of the experimental data, three replicate ... Xu, Z.; Fang, X.; Liu, H. Theoretical and Experimental Analysis on Low-Cycle Fatigue Crack Initiation for High Strength Steel Stiffened Plates. In Proceedings of the ASME 2022 41st International Conference on Ocean, Offshore and Arctic Engineering, Hamburg, Germany, 5-10 ...
6 Students, parents, HTs, and schools' data are matched to obtain the final data. 7 There is at least one parent with a master's degree or above. 8 The questionnaire allows students to self-report their degree of difficulty learning Chinese, Math, and English in the sixth grade, which can be used to measure students' previous academic ...