
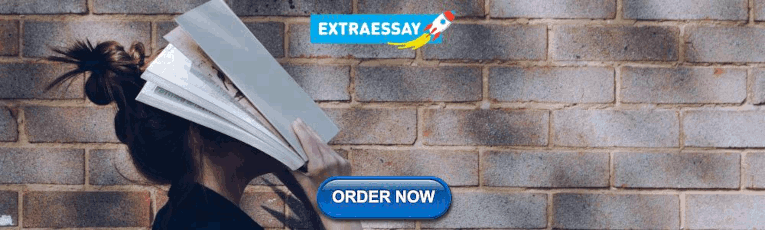
Definition:
The Problem Space refers to the set of all possible states, actions, and outcomes in a given problem or task. It encompasses the various variables, constraints, and parameters that define the problem and shape its solution space.
Components of Problem Space:
The problem space typically comprises the following key components:
- States: These are the different configurations or conditions that the problem can exist in. States may include initial states, intermediate states, and goal states.
- Actions: Actions represent the operations or steps that can be taken to transition between different states within the problem space. These actions can be predetermined or discovered during the problem-solving process.
- Constraints: Constraints define the limitations or restrictions that govern the problem-solving process. These constraints may involve factors such as available resources, time limits, legal considerations, or specific rules.
- Variables: Variables are the elements that can be adjusted or manipulated to find the optimal solution within the problem space. They can include numerical values, parameters, conditions, or settings.
- Outcomes: Outcomes refer to the potential results or consequences that can occur as a result of the problem-solving process. These outcomes can be evaluated based on predefined criteria such as efficiency, accuracy, effectiveness, or desirability.
Characteristics of Problem Space:
The problem space is characterized by the following attributes:
- Complexity: The problem space can range from simple and well-defined to complex and ambiguous, depending on the nature of the problem.
- Size: The problem space may vary in size, representing a small, specific problem or a large-scale, multidimensional challenge.
- Search Space: The search space refers to the subset of the problem space that is explored during the problem-solving process.
- Interconnectedness: States, actions, and outcomes within the problem space are often interconnected, with each element influencing and being influenced by others.
- Dynamic Nature: The problem space may evolve and change as new information is acquired or as the problem-solving process unfolds.
In summary, the problem space encompasses the range of possibilities, constraints, and variables associated with a given problem. Understanding and analyzing the problem space are crucial for defining effective problem-solving strategies and finding optimal solutions.
Cognitive Psychology
- Precursors to Cognitive Psychology
- Emergence of Cognitive Psychology
- Research Methods
- Brain and Cognition: Neurons
- Brain and Cognition: Brain Structure
- Introduction to perception
- Top-down and bottom-up theories of perception
- Problem Solving
- Problem solving and insight
- Problem solving in animals
- Welcome page
- What is a Wiki Site?
- How to edit pages?
- How to join this site?
- Site members
- Recent changes
- List all pages
- Site Manager
It seems you have no tags attached to pages. To attach a tag simply click on the tags button at the bottom of any page.
Add a new page
edit this panel
What is problem solving?
A problem arises when we need to overcome some obstacle in order to get from our current state to a desired state. Problem solving is the process that an organism implements in order to try to get from the current state to the desired state.
An historical review of approaches to problem solving
The behaviourist approach.
Behaviourist researchers argued that problem solving was a reproductive process; that is, organisms faced with a problem applied behaviour that had been successful on a previous occasion. Successful behaviour was itself believed to have been arrived at through a process of trial-and-error. In 1911 Edward Thorndike had developed his law of effect after observing cats discover how to escape from the cage into which he had placed them. This greatly influenced the behaviourist view of problem solving:
The Gestalt approach
By contrast, Gestalt psychologists argued that problem solving was a productive process. In particular, in the process of thinking about a problem individuals sometimes "restructured" their representation of the problem, leading to a flash of insight that enabled them to reach a solution. In The Mentality of Apes (1915) Wolfgang Köhler described a series of studies with apes in which the animals appeared to demonstrate insight in problem solving situations. A description of these studies, with photographs, can be found here .
The Gestalt psychologists described several aspects of thought that acted as barriers to successful problem solving. One of these was called the Einstellung effect , now more commonly referred to as mental set or entrenchment . This occurs when a problem solver becomes fixated on applying a strategy that has previously worked, but is less helpful for the current problem. Luchins (1942) reported a study in which people had to use three jugs of differing capacity (measured in cups) to measure out a required amount of water (given by the experimenter). Some people were given a series of "practice" trials prior to attempting the critical problems. These practice problems could be solved by filling Jug B, then tipping water from Jug B into Jug A until it is filled, and then twice using the remainging contents of Jug A to fill Jug C. Expressed as a formula, this is B - A - 2C. However, although this formula could be applied to the subsequent "critical" problems, these also had simpler solutions, such as A - C. People who had experienced the practice problems mostly tried to apply the more complex solution to these later problems, unlike people who had not experienced the earlier problems (who mostly found the simpler solutions).
Another barrier to problem solving is functional fixedness , whereby individuals fail to recognize that objects can be used for a purpose other than that they were designed for. Maier (1930) illustrated this with his two string problem .
For a real life example of overcoming fuctional fixedness, see: Overcoming functional fixedness: Apollo 13
Questions : What do you think of Köhler's claim that his apes had demonstrated insight? What proportion of Maier's participants spontaneously found the solution before getting any kind of hint? What did Maier do that led some people to get the correct solution? (these questions require some research)
The cognitive approach to problem solving
Problem space theory.
In 1972, Allen Newell and Herbert Simon published the book Human Problem Solving , in which they outlined their problem space theory of problem solving. In this theory, people solve problems by searching in a problem space . The problem space consists of the initial (current) state, the goal state, and all possible states in between. The actions that people take in order to move from one state to another are known as operators . Consider the eight puzzle . The problem space for the eight puzzle consists of the initial arrangement of tiles, the desired arrangement of tiles (normally 1, 2, 3….8), and all the possible arrangements that can be arrived at in between. However, problem spaces can be very large so the key issue is how people navigate their way through the possibilities, given their limited working memory capacities. In other words, how do they choose operators? For many problems we possess domain knowledge that helps us decide what to do. But for novel problems Newell and Simon proposed that operator selection is guided by cognitive short-cuts, known as heuristics . The simplest heuristic is repeat-state avoidance or backup avoidance 1 , whereby individuals prefer not to take an action that would take them back to a previous problem state. This is unhelpful when a person has taken an inappropriate action and actually needs to go back a step or more.
Another heuristic is difference reduction , or hill-climbing , whereby people take the action that leads to the biggest similarity between current state and goal state. Before reading further, see if you can solve the following problem:
In the hobbits and orcs problem the task instructions are as follows:
On one side of a river are three hobbits and three orcs. They have a boat on their side that is capable of carrying two creatures at a time across the river. The goal is to transport all six creatures across to the other side of the river. At no point on either side of the river can orcs outnumber hobbits (or the orcs would eat the outnumbered hobbits). The problem, then, is to find a method of transporting all six creatures across the river without the hobbits ever being outnumbered.
The solution to this problem, together with an explanation of how difference reduction is often applied, can be found by clicking here .
A more sophisticated heuristic is means-ends analysis . Like difference reduction, the means-ends analysis heuristic looks for the action that will lead to the greatest reduction in difference between the current state and goal state, but also specifies what to do if that action cannot be taken. Means-ends analysis can be specified as follows 2 :
- Compare the current state with the goal state. If there is no difference between them, the problem is solved.
- If there is a difference between the current state and the goal state, set a goal to solve that difference. If there is more than one difference, set a goal to solve the largest difference.
- Select an operator that will solve the difference identified in Step 2.
- If the operator can be applied, apply it. If it cannot, set a new goal to reach a state that would allow the application of the operator.
- Return to Step 1 with the new goal set in Step 4.
The application of means-ends analysis can be illustrated with the Tower of Hanoi problem .
In 1957 Newell and Simon developed the General Problem Solver , a computer program that used means-ends analysis to find solutions to a range of well-defined problems - problems that have clear paths (if not easy ones) to a goal state. In their 1972 book on problem solving they reported the verbal protocols of participants engaged in problem solving, which showed a close match between the steps that they took and those taken by the General Problem Solver.
Acquiring operators
There are three ways in which operators can be acquired:
- Trial-and-error. As noted above, this formed the basis of the behaviourist account of problem solving.
- Direct instruction.
- Analogies. Analogies are examples from one domain (the source), whose elements can be used to aid problem solving in another domain (the target). However, novices often struggle to spot analogies, as described here .
Next: Problem solving and insight
Breadcrumbs Section. Click here to navigate to respective pages.

Reasoning, Problem Solving, and Decision Processes: The Problem Space as a Fundamental Category
DOI link for Reasoning, Problem Solving, and Decision Processes: The Problem Space as a Fundamental Category
Click here to navigate to parent product.
The notion of a problem space is well known in the area of problem solving research, both in cognitive psychology and artificial intelligence. The Problem Space Hypothesis is enunciated that the scope of problem spaces is to be extended to all symbolic cognitive activity. The chapter is devoted to explaining the nature of this hypothesis and describing some of its potential implications, with no attempt at a critical marshalling of the evidence pro and con. Two examples are used, one a typical problem solving activity (the Tower of Hanoi) and the other syllogistic reasoning. The latter is an example where the search behavior typical of problem spaces is not clearly in evidence, so it provides a useful area to explore the extension of the concept. A focal issue used in the chapter is the origin of the numerous flow diagrams that serve as theories of how subjects behave in tasks in the psychological laboratory. On the Problem Space Hypothesis these flow diagrams derive from the interaction of the task environment and the problem space.
- Privacy Policy
- Terms & Conditions
- Cookie Policy
- Taylor & Francis Online
- Taylor & Francis Group
- Students/Researchers
- Librarians/Institutions
Connect with us
Registered in England & Wales No. 3099067 5 Howick Place | London | SW1P 1WG © 2024 Informa UK Limited
- Corpus ID: 7684665
Reasoning, problem solving, and decision processes: the problem space as a fundamental category
- Published 1 August 1993
- Computer Science, Philosophy
Figures from this paper
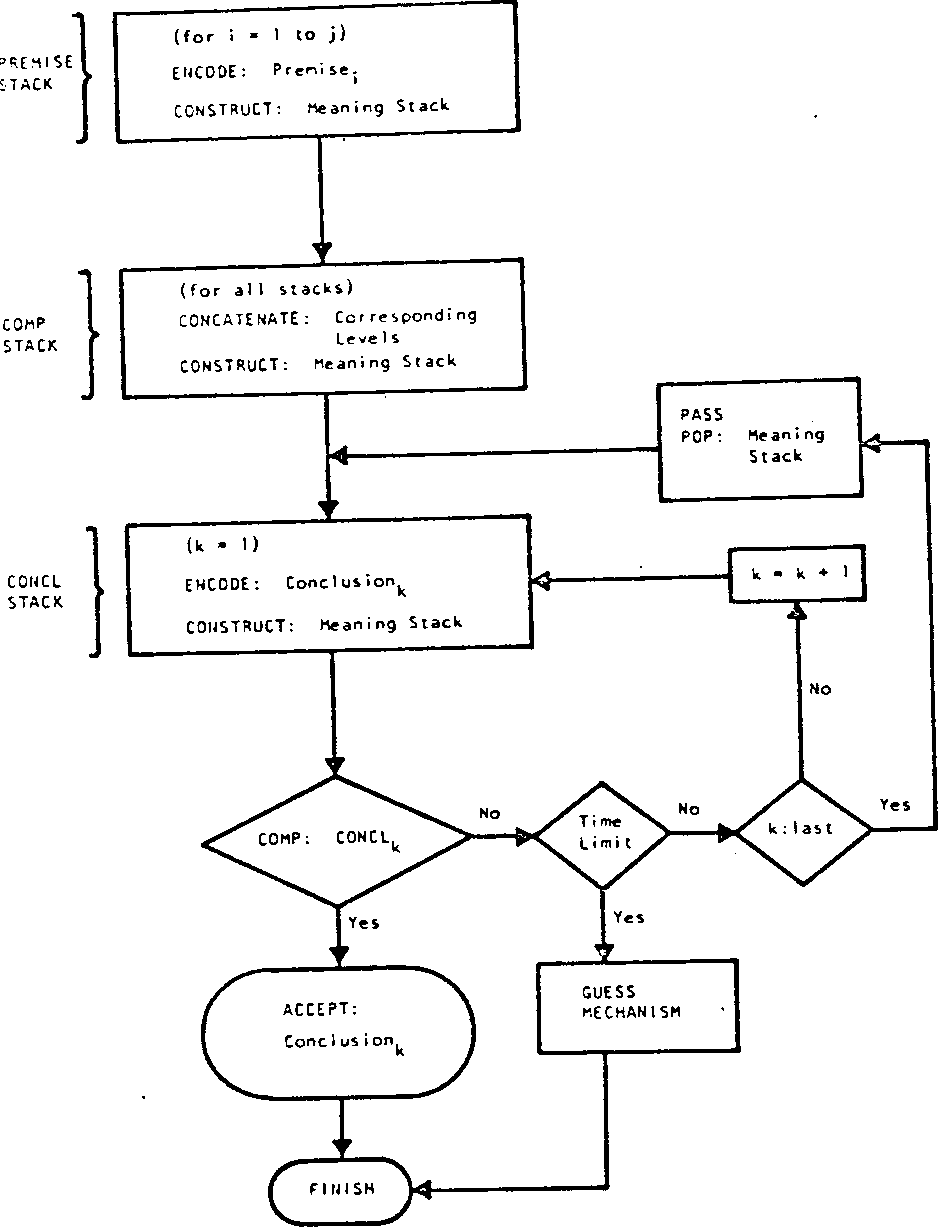
225 Citations
Complex problem-solving: a field in search of a definition, a guided tour of artificial intelligence research: volume i: knowledge representation, reasoning and learning, human reasoning: deduction rules or mental models, or both, problem solving and mathematical knowledge, planning and problem solving, science watch problem solving and learning, contemporary approaches to the study of thinking and problem solving, defining real world problems: a conceptual language, artificial intelligence and high-level cognition, reasoning about properties: a computational theory., 22 references, the psychology of syllogisms, the functional equivalence of problem solving skills, psychology of reasoning: structure and content, two models of syllogistic reasoning: feature selection and conversion, harpy, production systems and human cognition, the nature of mental abilities., production systems: models of control structures, human problem solving., human memory: a proposed system and its control processes, problem-solving methods in artificial intelligence, related papers.
Showing 1 through 3 of 0 Related Papers
- All Activity
- Ask a Question
What is the Problem Space Theory?
- problemsolving
Please log in or register to add a comment.
Please log in or register to answer this question..
The Problem Space Theory in which people try and solve problems by looking at the problem space.
The problem space consists of multiple states being:
- Initial or Current State
- End or Goal State
- All Possible States in between
Actions that we take in order to move into another state are known as Operators. As humans, we take short-cuts known as heuristics in order to solve problems that we do not have any knowledge in. Heuristics also operates within the human processing system which can be applied to solve problems in areas that are well defined. A common heuristic is "means-end analysis" where an operator is used to shorten the length between the Initial and Goal States. Sub-goals can also be defined in order to make the overall problem easier to execute which a "Divide and Conquer" method could be applied to reach the Goal State faster.
Related questions
- programming
- All categories
- Programming (38)
- Data Science (130)
- Machine Learning (77)
- Deep Learning (9)
- Artificial Intelligence (3)
- Web Development (8)
- Mobile Development (0)
- Cloud Computing (10)
- Human Computer Interaction (92)
- Python Interview Questions (18)
- Data Science Interview Questions (19)
- Machine Learning Interview Questions (17)
- Deep Learning Interview Questions (2)
423 questions
363 answers
99 comments
- Send feedback
- Privacy Policy
- Terms of Use

- IGNOU Answers
Define Problem space hypothesis
In today's rapidly changing and interconnected world, businesses, organizations, and individuals face a multitude of complex challenges. To effectively navigate these challenges, it is crucial to understand and define the problem space accurately.
The problem space hypothesis provides a framework for identifying, analyzing, and addressing complex problems systematically. This hypothesis suggests that a clear understanding of the problem space can lead to more effective problem-solving and innovative solutions.
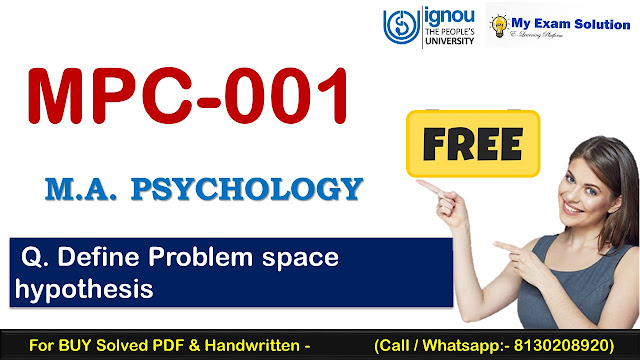
Define Problem space hypothesis- The problem space refers to the entire context and environment within which a problem exists. It encompasses all the factors and variables that influence the problem and its potential solutions. This includes internal and external elements such as stakeholders, constraints, resources, market dynamics, technological factors, social factors, and more.
Cultural Blocks To Problem Solving Definition Of Functional Fixedness Definition Symptoms And Causes Of Aphasia
The problem space hypothesis posits that by comprehensively understanding this context, one can gain insights into the problem's root causes and identify potential avenues for resolution.
Significance of the Problem Space Hypothesis:
The problem space hypothesis is essential because it helps in avoiding superficial or narrow problem definitions. By thoroughly exploring the problem space, decision-makers can avoid focusing solely on symptoms or surface-level issues. Instead, they can dig deeper, uncover underlying complexities, and develop a more holistic understanding of the problem at hand.
This broader perspective enhances the chances of finding innovative and sustainable solutions.
Key Elements of the Problem Space Hypothesis:
1. Problem Context Analysis: This involves analyzing the internal and external factors influencing the problem. It includes identifying stakeholders, understanding their motivations and interests, and assessing the larger social, economic, and environmental context in which the problem exists.
2. Systems Thinking: The problem space hypothesis emphasizes the importance of adopting a systems thinking approach. This means recognizing that problems are interconnected and understanding the relationships and feedback loops between various elements within the problem space. It helps in identifying leverage points and potential unintended consequences of interventions.
3. Problem Framing: Properly framing the problem is crucial for effective problem-solving. The problem space hypothesis encourages defining the problem in terms of outcomes and objectives rather than predefined solutions. This allows for a more flexible and creative exploration of potential alternatives.
4. Iterative Exploration: The problem space is not static but evolves over time. The hypothesis suggests an iterative approach to problem exploration, where new insights are continually gained, and assumptions are tested. This approach enables adaptive problem-solving, accommodating new information and changing circumstances.
Application of the Problem Space Hypothesis
The problem space hypothesis is applicable across various domains and scales. In business, it can help organizations identify market opportunities, understand customer needs, and design innovative products or services. In public policy, it can aid in crafting comprehensive and effective policies by considering the broader social, economic, and environmental dimensions of a problem. In technology development, it can guide research and development efforts by uncovering hidden challenges and improving the chances of success.
Challenges and Limitations: While the problem space hypothesis offers valuable insights, it is not without challenges. One of the key challenges is the complexity and ambiguity inherent in many problem spaces. It requires extensive data gathering, analysis, and expertise to fully grasp the nuances and interdependencies within the problem space. Additionally, the hypothesis relies on the availability of accurate and up-to-date information, which can be a limitation in certain contexts.
Problem Solving Strategies
Problem-solving is an essential skill in both personal and professional settings. It involves analyzing a situation, identifying obstacles or challenges, and finding appropriate solutions. However, not all problems are created equal, and each may require a tailored approach. In this article, we will explore various problem-solving strategies that can help individuals and organizations tackle complex issues effectively. These strategies encompass different techniques, methods, and frameworks that facilitate critical thinking, creativity, and innovation.
1. Define and Understand the Problem: The first step in problem-solving is to clearly define and understand the problem. This involves gathering relevant information, identifying the root causes, and analyzing the various factors contributing to the problem. It is crucial to delve beyond the surface-level symptoms and uncover the underlying complexities.
2. Divide and Conquer: For complex problems, it can be beneficial to break them down into smaller, more manageable parts. This strategy, known as "divide and conquer," involves separating the problem into sub-problems and addressing them individually. By tackling smaller components, it becomes easier to identify specific issues and develop targeted solutions. Once the sub-problems are solved, the solutions can be integrated to address the larger problem.
3. Brainstorming and Idea Generation: Brainstorming is a widely used technique for generating a wide range of ideas and potential solutions. It involves encouraging free thinking, suspending judgment, and promoting creativity. Group brainstorming sessions can leverage the collective intelligence and diverse perspectives of team members, fostering innovative thinking and uncovering unique solutions. Additionally, individual brainstorming allows for independent ideation, avoiding groupthink and encouraging individual creativity.
4. Root Cause Analysis: Identifying the root causes of a problem is critical for effective problem-solving. Root cause analysis involves systematically investigating the underlying reasons that contribute to the problem's existence.
Define Problem space hypothesis- Tools such as the "5 Whys" technique can help in progressively digging deeper into the causes behind a problem. By addressing the root causes, rather than merely treating symptoms, more sustainable and long-lasting solutions can be developed.
5. Critical Thinking and Analytical Skills: Developing strong critical thinking and analytical skills is essential for effective problem-solving. This includes the ability to evaluate information objectively, identify biases or assumptions, and draw logical conclusions. Critical thinking also involves considering alternative perspectives, weighing evidence, and making informed decisions based on available data. Cultivating these skills enables individuals to approach problems with a rational and systematic mindset.
6. Design Thinking: Design thinking is a human-centered problem-solving approach that emphasizes empathy, creativity, and iteration. It involves understanding the needs and perspectives of the end-users, ideating potential solutions, prototyping and testing those solutions, and iterating based on feedback. Design thinking encourages a holistic view of the problem space, fostering innovative and user-centric solutions.
7. Decision-Making Strategies: Problem-solving often involves making decisions in the face of uncertainty. Several decision-making strategies can aid in this process. These include the rational decision-making model, which involves a systematic evaluation of alternatives based on criteria and preferences, and intuitive decision-making, which relies on instinct, experience, and pattern recognition. Additionally, techniques such as the decision matrix and SWOT analysis can help in evaluating options and selecting the most suitable course of action.
8. Collaboration and Teamwork: Many complex problems require collaborative efforts. Teamwork enables diverse perspectives, expertise, and skills to come together, leading to more comprehensive problem analysis and innovative solutions.
Define Problem space hypothesis- Effective collaboration involves open communication, active listening, and respect for different viewpoints. Leveraging the collective intelligence of a team can foster synergy and improve the quality of problem-solving outcomes.
0 comments:
Note: Only a member of this blog may post a comment.
Popular Posts
- Why does Plato want the artists to be kept away from the ideal state? Discuss Why does Plato want the artists to be kept away from the ideal state? Discuss Ans . Plato, born around 427 BC in Athens, Greece, was an i...

- Comment on Mary Wollstonecraft’s contributions to the rights of women Q. Comment on Mary Wollstonecraft’s contributions to the rights of women Introduction: Mary Wollstonecraft’s contributions to the ...
Watch Video Now !
Labels Cloud
- BA Assignment 2022-23 9
- Biography 11
- Book Summary 13
- Competitive Exams 9
- English Literature 743
- Govt Exams 5
- IGNOU Answers 1490
- IGNOU ASSIGNMENT 502
- ignou assignment 2022-23 288
- ignou guess paper 278
- NMIMS Assignment 14
Means-Ends Planning: An Example Soar System
Cite this chapter.
- Aladin Akyürek 3
Part of the book series: Studies in Cognitive Systems ((COGS,volume 10))
164 Accesses
3 Citations
Although Soar is intended to cover the full range of weak problem-solving methods-often hypothesized in cognitive science as basic for all intelligent agents, earlier attempts to add means-ends analysis to Soar’s repertoire of methods have not been particularly successful or convincing. Considering its psychological significance, stipulated by Newell and Simon (1972), it seems essential that Soar, when taken as a general cognitive architecture, should allow the means-ends analysis to arise naturally from its own structure. This paper presents a planner program that interleaves a difference reduction process with Soar’s default mechanism for operator subgoaling that it modifies in order to map meansends analysis onto Soar. The scheme advanced is shown to produce “macro-operators” of a novel kind, called macrochunks , which may have important implications for explaining routine behavior. The approach taken and the problems that had to be dealt with in implementing this planner are treated in detail. Also, SoarL —a language used for state representations- is reviewed with respect to the frame problem.
This is a preview of subscription content, log in via an institution to check access.
Access this chapter
Subscribe and save.
- Get 10 units per month
- Download Article/Chapter or eBook
- 1 Unit = 1 Article or 1 Chapter
- Cancel anytime
- Available as PDF
- Read on any device
- Instant download
- Own it forever
- Available as EPUB and PDF
- Compact, lightweight edition
- Dispatched in 3 to 5 business days
- Free shipping worldwide - see info
Tax calculation will be finalised at checkout
Purchases are for personal use only
Institutional subscriptions
Unable to display preview. Download preview PDF.
Similar content being viewed by others
Automated Planning
Non-classical Planning with a Classical Planner: The Power of Transformations
What an algorithm is.
Akyürek, A. (1992). On a computational model of human planning. In J. A. Michon & A. Akyürek (Eds.), Soar: A cognitive architecture in perspective (pp. 81–108). Dordrecht, The Netherlands: Kluwer.
Chapter Google Scholar
Anderson, J. R. (1986). Knowledge compilation: The general learning mechanism. In R. S. Michalski. J. G. Carbonell. & T. M. Mitchell (Eds.). Machine learning: An artificial intelligence approach (Vol. II. pp. 289–310). Los Altos. CA: Morgan Kaufma
Google Scholar
Atwood, M. E. & Polson. P. G. (1976). A process model for water jug problems. Cognitive Psychology , 8 ,191–216.
Article Google Scholar
Carbonell, J. G. (1983). Learning by analogy: Formulating and generalizing plans from past experience, In R. S. Michalski. J. G. Carbonell, & T. M. Mitchell (Eds.). Machine learning: An artificial intelligence approach (pp. 137–161). Los Altos. CA: Morgan Kaufmann.
Dawson, C., & Siklóssy, L. (1977). The role of preprocessing in problem solving systems. In Proceedings of the Fifth International Joint Conference on Artificial Intelligence (pp. 465–471). San Mateo. CA: Morgan Kaufmann.
DeJong, G., & Mooney. R. (1986). Explanation-based learning: An alternative view. Machine Learning , 1 , 145–176.
Ernst, G. W. (1969). Sufficient conditions for the success of GPS. Journal of the Association for Computing Machinery , 16 , 517–533.
Fikes, R. E., Hart, P.E., & Nilsson, N. J. (1972). Learning and executing generalized robot plans. Artificial Intelligence , 3 , 251–288.
Fikes, R. E., & Nilsson, N. J. (1971). STRIPS: A new approach to the application of theorem proving to problem solving. Artificial Intelligence , 2 , 189–208.
Hayes. P. J. (1973). The frame problem and related problems in artificial intelligence. In A. Elithorn & D. Jones (Eds.). Artificial and human thinking (pp. 45–59). Amsterdam: Elsevier.
Jeffries, R., Polson, P. G., & Razran, L. (1977). A process model for missionaries-cannibals and other river-crossing problems. Cognitive Psychology , 9 ,412–440.
Korf, R. E. (1985). Learning to solve problems by searching for macro-operators . Boston, MA: Pitman.
Knoblock, C. A. (1989). Learning hierarchies of abstraction spaces. In Proceedings of the Sixth International Workshop on Machine Learning (pp. 241–245). San Mateo. CA: Morgan Kaufmann.
Laird, J. E. (1984). Universal subgoaling (Tech. Rep. CMU-CS-84-129). Pittsburgh. PA: Carnegie Mellon University, Department of Computer Science. [Also available as part of Laird, I., Rosenbloom, P., & Newell, A. (1986). Universal subgoaling and chunking: The automatic generation and learning of goal hierarchies (pp. 1–131). Boston. MA: Kluwer.)
Laird, J. E., Congdon, C. B., Altmann, E., & Swedlow, K. (1990). Soar user’s manual: Version 5.2 (Tech. Rep. CMU-CS-90-179). Pittsburgh, PA: Carnegie Mellon University, School of Computer Science.
Laird, J., & Newell, A. (1983). A universal weak method ([ech. Rep. CMU-CS-83-141). Pittsburgh, PA: Carnegie Mellon University, Department of Computer Science.
Laird, J. E., & Rosenbloom, P. S. (1990). Integrating execution, planning, and learning in Soar for external environments. In Proceedings of the Eighth National Conference on Artificial Intelligence (pp. 1022–1029). San Mateo, CA: Morgan Kaufmann.
Laird, J. E., Rosenbloom, P. S., & Newell, A. (1984). Towards chunking as a general learning mechanism. In Proceedings of the Fourth National Conference on Artificial Intelligence (pp. 188–192). San Mateo, CA: Morgan Kaufmann.
Laird, J. E., Rosenbloom, P. S., & Newell, A. (1986). Chunking in Soar: The anatomy of a general learning mechanism. Machine Learning , 1 , 11–46.
Laird, J., Swedlow, K., Altmann, E., Congdon, C. B., & Wiesmeyer, M. (1989). Soar user’s manual: Version 4.5 . Pittsburgh, PA: Carnegie Mellon University, School of Computer Science.
McDermott, D. (1987). AI, logic, and the frame problem. In F. M. Brown (Ed.), The frame problem in artificial intelligence (pp. 105–118). Los Altos, CA: Morgan Kaufmann.
McDermott, D. (1978). Planning and acting. Cognitive Science , 2 , 71–109).
Minton, S. (1988). Learning search control knowledge: An explanation-based approach . Boston, MA: Kluwer.
Book Google Scholar
Minton, S., Knoblock, C. A., Kuokka, D. R., Gil, Y., Joseph, R. L., & Carbonell, J. G. (1989). PRODIGY 2.0: The manual and tutorial (Tech. Rep. CMU-CS-89-146). Pittsburgh, PA: Carnegie-Mellon University, School of Computer Science.
Mitchell, T. M., Keller, R. M., & Kedar-Cabelli, S. T. (1986). Explanation-based generalization: A unifying view. Machine Learning , 1 ,47–80.
Newell, A. (1980). Reasoning, problem solving, and decision processes: The problem space as a fundamental category. In R. S. Nickerson (Ed.), Attention and performance (Vol. 8, pp. 693–718). Hillsdale, NJ: Erlbaum.
Newell, A. (1990). Unified theories of cognition . Cambridge, MA: Harvard University Press.
Newell, A. (1992). Unified theories of cognition and the role of Soar. In J. A. Michon & A. Akyürek (Eds.), Soar: A cognitive architecture in perspective (pp. 25–79). Dordrecht, The Netherlands: Kluwer.
Newell, A., Rosenbloom, P. S., & Laird, J. E. (1989). Symbolic architectures for cognition. In M. I. Posner (Ed.), Foundations of cognitive science (pp. 93–131). Cambridge, MA: MIT Press.
Newell, A., Shaw, J. C., & Simon, H. A. (1962). The processes of creative thinking. In H. E. Gruber, G. Terrell, & M. Wertheimer (Eds.), Contemporary approaches to creative thinking (pp. 63–119). New York: Atherton Press.
Newell, A., & Simon, H. A. (1963). GPS, a program that simulates human thought. In E. A. Feigenbaum & J. Feldman (Eds.), Computers and thought (pp. 279–293). New York: McGraw-Hill. (Original work published 1961)
Newell, A., & Simon, H. A. (1972). Human problem solving . Englewood Cliffs. NJ: PrenticeHall.
Newell, A., Yost, G., Laird, J. E., Rosenbloom, P. S., & Altmann, E. (1990). Formulating the problem space computational model . Paper presented at the 25th Anniversary Symposium. School of Computer Science, Carnegie Mellon University, Pittsburgh, PA.
Nilsson, N. J.(1980). Principles of artificial intelligence . Los Altos, CA: Morgan Kaufmann.
Russell, S. J. (1989). Execution architectures and compilation. In Proceedings of the Eleventh International Joint Conference on Artificial Intelligence (pp. 15–20). San Mateo, CA: Morgan Kaufmann.
Sacerdoti, E. D. (1974). Planning in a hierarchy of abstraction spaces. Artificial Intelligence , 5 ,115–135.
Sandewall, E. J. (1969). A planning problem solver based on look-ahead in stochastic game trees. Journal of the Association for Computing Machinery , 16 , 364–382.
Schubert, L. (1990). Monotonic solution of the frame problem in the situation calculus: An efficient method for worlds with fully specified actions. In H. E. Kyburg, R. P. Loui, & G. N. Carlson (Eds.), Knowledge representation and defeasible reasoning (pp. 23–67). Dordrecht, The Netherlands: Kluwer.
Sussman, G. J. (1975). A computer model of skill acquisition . New York: American Elsevier.
Tambe, M., Newell, A., & Rosenbloom, P. S. (1990). The problem of expensive chunks and its solution by restricting expressiveness. Machine Learning , 5 ,299–348.
Tenenberg, J. D. (1988). Abstraction in planning (Tech. Rep. No. 250). Rochester, NY: University of Rochester. Computer Science Department.
Unruh, A., & Rosenbloom, P. S. (1989). Abstraction in problem solving and learning. In Proceedings of the Eleventh International Joint Conference on Artificial Intelligence (pp. 681–687). San Mateo. CA: Morgan Kaufmann.
Unruh, A., & Rosenbloom, P. S. (1990). Two new weak method increments for abstraction . Manuscript submitted for publication.
Wilkins, D. E. (1988). Practical planning: Extending the classical AI planning paradigm . San Mateo,CA: Morgan Kaufmann.
Woods, W. A. (1975). What’s in a link: Foundations for semantic networks. In D. G. Bobrow & A. Collins (Eds.). Representation and understanding: Studies in cognitive science (pp. 35–82). Orlando, FL: Academic Press.
Download references
Author information
Authors and affiliations.
Department of Psychology, University of Groningen, Groningen, The Netherlands
Aladin Akyürek
You can also search for this author in PubMed Google Scholar
Editor information
Editors and affiliations.
John A. Michon & Aladin Akyürek &
Rights and permissions
Reprints and permissions
Copyright information
© 1992 Springer Science+Business Media Dordrecht
About this chapter
Akyürek, A. (1992). Means-Ends Planning: An Example Soar System. In: Michon, J.A., Akyürek, A. (eds) Soar: A Cognitive Architecture in Perspective. Studies in Cognitive Systems, vol 10. Springer, Dordrecht. https://doi.org/10.1007/978-94-011-2426-3_5
Download citation
DOI : https://doi.org/10.1007/978-94-011-2426-3_5
Publisher Name : Springer, Dordrecht
Print ISBN : 978-94-010-5070-8
Online ISBN : 978-94-011-2426-3
eBook Packages : Springer Book Archive
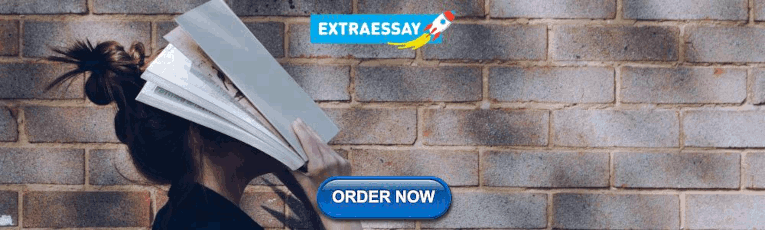
Share this chapter
Anyone you share the following link with will be able to read this content:
Sorry, a shareable link is not currently available for this article.
Provided by the Springer Nature SharedIt content-sharing initiative
- Publish with us
Policies and ethics
- Find a journal
- Track your research
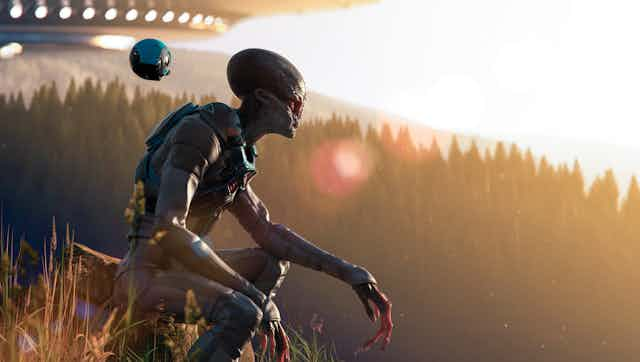
Belief in alien visits to Earth is spiralling out of control – here’s why that’s so dangerous
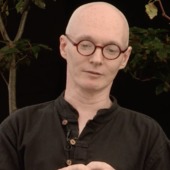
Research Fellow in the Philosophy of Ethics, King's College London
Disclosure statement
Tony Milligan receives funding from the European Research Council (ERC) under the European Union's Horizon 2020 research and innovation programme (Grant agreement No. 856543).
King's College London provides funding as a member of The Conversation UK.
View all partners
The idea that aliens may have visited the Earth is becoming increasingly popular. Around a fifth of UK citizens believe Earth has been visited by extraterrestrials , and an estimated 7% believe that they have seen a UFO.
The figures are even higher in the US – and rising. The number of people who believe UFO sightings offer likely proof of alien life increased from 20% in 1996 to 34% in 2022 . Some 24% of Americans say they’ve seen a UFO.
This belief is slightly paradoxical as we have zero evidence that aliens even exist. What’s more, given the vast distances between star systems, it seems odd we’d only learn about them from a visit. Evidence for aliens is more likely to come from signals from faraway planets.
In a paper accepted for publication in the Proceedings of the International Astronomical Union , I argue that the belief in alien visitors is no longer a quirk, but a widespread societal problem.
The belief is now rising to the extent that politicians, at least in the US, feel they have to respond. The disclosure of information about claimed Unidentified Anomalous Phenomena ( UAPs rather than UFOs) from the Pentagon has got a lot of bi-partisan attention in the country.
Much of it plays upon familiar anti-elite tropes that both parties have been ready to use, such as the idea that the military and a secretive cabal of private commercial interests are keeping the deep truth about alien visitation hidden. That truth is believed to involve sightings, abductions and reverse-engineered alien technology.
Belief in a cover-up is even higher than belief in alien visitation. In 2019, a Gallop poll found that a staggering 68% of Americans believed that “the US government knows more about UFOs than it is telling”.
This political trend has been decades in the making. Jimmy Carter promised document disclosure during his presidential campaign in 1976, several years after his own reported UFO sighting. Like so many other sightings, the simplest explanation is that he saw Venus. (That happens a lot.)
Hillary Clinton also suggested she wanted to “open [Pentagon] files as much as I can” during her presidential campaign against Donald Trump. As seen in the video below, Trump suggested he’d need to “think about” whether it was possible to declassify the so-called Roswell documentation (relating to the notorious claimed crash of a UFO and the recovery of alien bodies).
Former president Bill Clinton claimed to have sent his chief of staff, John Podesta, down to Area 51, a highly classified US Air Force facility, just in case any of the rumours about alien technology at the site were true. It is worth nothing that Podesta is a long-time enthusiast for all things to do with UFOs.
The most prominent current advocate of document disclosure is the Democratic Senate leader Chuck Schumer . His stripped back 2023 UAP disclosure bill for revealing some UAP records was co-sponsored by three Republican senators.
Pentagon disclosure finally began during the early stages of Joe Biden’s term of office, but so far there has been nothing to see. Nothing looks like an encounter. Nothing looks close.
Still, the background noise does not go away.
Problems for society
All this is ultimately encouraging conspiracy theories, which could undermine trust in democratic institutions. There have been humorous calls to storm Area 51 . And after the storming of the Capitol in 2021, this now looks like an increasingly dangerous possibility.
Too much background noise about UFOs and UAPs can also get in the way of legitimate science communication about the possibility of finding microbial extraterrestrial life. Astrobiology, the science dealing with such matters, has a far less effective publicity machine than UFOlogy.
History , a YouTube channel part owned by Disney, regularly delivers shows about “ancient aliens”. The show is now in its 20th season and the channel has 13.8 million subscribers. The Nasa astrobiology channel has a hard won 20,000 subscribers. Actual science finds itself badly outnumbered by entertainment repackaged as factual.
Alien visitation narratives have also repeatedly tried to hijack and overwrite the history and mythology of indigenous people.
The first steps in this direction go back to Alexander Kazantsev’s science fiction tale Explosion: The Story of a Hypothesis (1946). It presents the 1908 Tunguska meteorite impact event as a Nagasaki-like explosion of an alien spacecraft engine. In Kazantsev’s tale, a single giant black female survivor has been left stranded, equipped with special healing powers. This lead to her adoption as a shaman by the indigenous Evenki people.
Nasa and the space science community do support efforts such as the Native Skywatchers initiative set up by the indigenous Ojibwe and Lakota communities to ensure the survival of storytelling about the stars. There is a real and extensive network of indigenous scholarship about these matters.
But UFOlogists promise a far higher profile for indigenous history in return for the mashing together of genuine indigenous stories about life arriving from the skies with fictional tales about UFOs, repackaged as suppressed history.
The modern alien visitation narrative has not, after all, emerged out of indigenous communities. Quite the opposite. It emerged in part as a way for conspiracy-minded thinkers in a Europe torn apart by racism to “explain” how complex urban civilisations in places like South America could have existed prior to European settlement.
Squeezed through a new age filter of 1960s counterculture, the narrative was flipped to value indigenous people as having once possessed advanced technology . Once upon a time, according to this view, every indigenous civilisation was Wakanda, a fictional country appearing in American comic books published by Marvel Comics.
If all of this stayed in its own box, as entertaining fiction, then matters would be fine. But it doesn’t, and they aren’t. Visitation narratives tend to overwrite indigenous storytelling about sky and ground.
This is a problem for everyone, not just indigenous peoples struggling to continue authentic traditions. It threatens our grasp of the past. When it comes to insight into our remote ancestors, the remnants of prehistoric storytelling are few and precious, such as within indigenous storytelling about the stars.
Take the tales of the Pleiades , which date back in standard forms to at least 50,000 years ago .
This may be why these tales in particular are heavily targeted by alien visitation enthusiasts, some of whom even claim to be “Pleiadeans”. No surprises, Pleiadeans do not look like the Lakota or Ojibwe, but are strikingly blond, blue-eyed and Nordic.
It is increasingly clear that belief in alien visitation is no longer just a fun speculation, but something that has real and damaging consequences.
- Conspiracy theories
- Indigenous peoples
- Give me perspective

Admissions Officer

Director of STEM

Community member - Training Delivery and Development Committee (Volunteer part-time)

Chief Executive Officer

Head of Evidence to Action
- Open access
- Published: 30 August 2024
Intraspecific encounters can lead to reduced range overlap
- William F. Fagan ORCID: orcid.org/0000-0003-2433-9052 1 ,
- Ananke Krishnan 1 na1 ,
- Qianru Liao 1 na1 ,
- Christen H. Fleming 1 , 2 , 3 , 4 ,
- Daisy Liao 1 ,
- Clayton Lamb 5 ,
- Brent Patterson 6 ,
- Tyler Wheeldon 6 ,
- Ricardo Martinez-Garcia 2 , 7 ,
- Jorge F. S. Menezes 2 , 8 ,
- Michael J. Noonan 9 ,
- Eliezer Gurarie 10 &
- Justin M. Calabrese 1 , 2 , 11
Movement Ecology volume 12 , Article number: 58 ( 2024 ) Cite this article
Metrics details
Direct encounters, in which two or more individuals are physically close to one another, are a topic of increasing interest as more and better movement data become available. Recent progress, including the development of statistical tools for estimating robust measures of changes in animals’ space use over time, facilitates opportunities to link direct encounters between individuals with the long-term consequences of those encounters. Working with movement data for coyotes ( Canis latrans ) and grizzly bears ( Ursus arctos horribilis ), we investigate whether close intraspecific encounters were associated with spatial shifts in the animals’ range distributions, as might be expected if one or both of the individuals involved in an encounter were seeking to reduce or avoid conflict over space. We analyze the movement data of a pair of coyotes in detail, identifying how a change in home range overlap resulting from altered movement behavior was apparently a consequence of a close intraspecific encounter. With grizzly bear movement data, we approach the problem as population-level hypothesis tests of the spatial consequences of encounters. We find support for the hypotheses that (1) close intraspecific encounters between bears are, under certain circumstances, associated with subsequent changes in overlap between range distributions and (2) encounters defined at finer spatial scales are followed by greater changes in space use. Our results suggest that animals can undertake long-term, large-scale spatial changes in response to close intraspecific encounters that have the potential for conflict. Overall, we find that analyses of movement data in a pairwise context can (1) identify distances at which individuals’ proximity to one another may alter behavior and (2) facilitate testing of population-level hypotheses concerning the potential for direct encounters to alter individuals’ space use.
Introduction
Animals’ use of space can be affected by many factors [ 55 ], including physical features (waterbodies, highways), food resources [ 8 , 43 ], social systems [ 22 ], and escape cover and travel routes [ 45 ]. These factors can affect the intensity of space use within home ranges as well as the location of home range boundaries. In some cases, memory-based movement processes can lead to non-territorial spatial segregation in the absence of interactions between individuals (e.g., [ 48 ]), but in other scenarios, perhaps the most important factor determining territoriality is the presence of nearby conspecifics [ 4 , 50 ]. Species might actively defend their territories [ 46 ], avoid areas in which they have a high probability of encountering neighboring individuals [ 34 ], or be more alert when they move through possible encounter areas [ 54 ]. The importance of intraspecific interactions is well demonstrated by mechanistic home range analysis, which accurately predicts spatial conformation of individual home ranges by modelling the impact of indirect interactions, such as scent marking, on the formation, structure and maintenance of home range boundaries [ 36 , 37 ]. That these deterministic, interaction-based models can accurately predict the spatial configuration of individual home ranges highlights the importance of interactions in governing patterns of space use [ 11 ].
Studies of encounters between animals has a long history in ecology, with Doncaster [ 10 ] providing early key insights via methods for quantifying overlap and encounters from tracking data. More recently, Joo et al. [ 25 ] reviewed approaches for studying species exhibiting collective motion, scenarios in which pairwise encounters may be more common but also highly dynamic in time. Yet, for many animal species and ecological contexts, direct encounters are relatively rare and difficult to study. However, identifying encounters and quantifying their consequences have become a topic of increasing interest in spatial ecology as more and better movement data become available [ 5 , 7 , 9 , 26 , 42 , 44 , 51 ]. Direct encounters between individuals also play a central role in evolutionary theory regarding territoriality, including considerations of the influence of cost-avoidance on behavior [ 32 , 52 ]. Tests of such ideas have historically been difficult to evaluate on the spatial scales typical of the home ranges of large mammals. This difficulty stems from the joint needs to first, identify that an encounter between individuals has taken place, and second, observe individuals long enough both before and after the encounter to have a clear view of the consequences. Relatively recent developments in movement ecology, such as the widespread availability of movement tracks with high temporal resolution [ 38 ] and the development of statistical tools for estimating robust measures of changes in space use over time [ 53 ], hold promise for resolving this knowledge gap. Ecologists are now in a better position to identify encounter events, quantify the impacts of encounters on the individuals involved, and interpret those results in the context of theoretical predictions regarding space use.
Some work in this direction has already begun. For example, working in a landscape with several species of mammalian carnivores, Ruprecht et al. [ 49 ] demonstrated how scavenging at kill sites increased opportunities for interspecific encounters, resource-transfer, and mortality events. Klauder et al. [ 28 ] and Périquet et al. [ 42 ] also explored encounters between individual predators in association with carrion, with the latter study discussing how encounters between individuals of different carnivore species at carrion or waterholes may lead to local-scale displacement of one or more of the individuals involved in the encounter. Noonan et al. [ 40 ] developed methods for analyzing animal movement data to identify the locations within a set of nearby home ranges at which individuals were likely to encounter one another and further demonstrated that known encounters fell within zones of heightened encounter probability.
Several models have examined the likelihood and location of encounters taking place as a function of animal movement behaviors. For example, Gurarie and Ovaskainen [ 20 ] examined scenarios involving encounters between foragers and stationary prey, leading to a taxonomy of encounter processes. Laidre et al. [ 29 ] identified sex-based differences in the movement patterns of polar bears, finding that males’ more tortuous movements reduced the rate of male–male encounters, while having little impact on male–female encounters. Martinez-Garcia et al. [ 31 ] analyzed stochastic models of pairs of moving animals, demonstrating how range-residency and a non-local perceptual range can alter the probability of pairwise encounters.
Despite progress on both theoretical and empirical fronts, much remains to be explored concerning the relationship between direct encounters and home range usage in animals [ 40 ]. Here, we examine the connection between close intraspecific encounters and home range dynamics. Working with movement track data for two species of mammalian carnivores, we investigate whether close intraspecific encounters were associated with any changes in the spatial overlap between range distributions. To do this, we leverage recently developed methods for population-level analyses of home range distributions [ 19 ] demonstrating two different approaches to the question of whether close encounters lead to changes in range overlap. First, we analyze the movement data of one such encounter pair in detail, identifying how a change in home range overlap resulting from altered movement behavior was apparently a consequence of a close intraspecific encounter. Second, in a separate analysis, we approach the problem in the context of population-level hypothesis tests. Working with a set of encounter pairs within a population, we (1) test the hypothesis that close intraspecific encounters are, on average, associated with changes in overlap between range distributions, (2) explore how seasonality, sex, and the presence of young influence the spatial consequences of such encounters, and (3) gauge the importance of the encounter threshold distance that defines an encounter.
Materials and methods
Tracking data.
To investigate evidence for changes in the overlap between animals’ home ranges before and after direct encounters, we used available GPS tracking data from Movebank.org [ 60 ]. We considered two datasets, each for a different purpose. First, for a detailed analysis of movement and space use associated with an encounter in a dataset with high temporal resolution for an extended duration, we considered movement tracks for a pair of adult (1 ♂, 1 ♀) coyotes ( Canis latrans) from Ontario, Canada [ 58 ] (Table S1 ). This pair of coyotes was specifically chosen from among the much larger set of data collected by Wheeldon [ 58 ] because it included both a close encounter and sufficient ecological context to facilitate illustration and interpretation of the consequences of the encounter. Second, to illustrate how movement data for a large number of individuals in a population can be used to investigate whether encounters were, on average, associated with altered patterns of space use, we used a hypothesis testing framework working with a population of animal pairs, and considered data for N = 40 grizzly bears ( Ursus arctos horribilis; 22 ♀; 18 ♂) living near Fernie in southwestern Canada near the border of British Columbia and Alberta [ 30 ] (Table S2). Roughly 21 to 29% of the adult grizzly population was collared in each year of the study. Grizzly bears are generally considered non-territorial in the sense that they do not actively defend spatial boundaries between individuals and allow spatial overlap with conspecifics at times [ 33 ]. In what follows, we explore the connection between encounters and space use, without any assumptions about territorial defense.
Identification of encounters and estimation of range distributions
Working with pairs of tracks, we used the distances () function in the R package ctmm [ 6 ] to estimate distances between pairs of individuals that resided near each other in the same geographic area over an extended period of time. The function ctmm:distances () is a robust distance estimation method that yields point estimates that are relatively unbiased due to effects of mismatched sampling times between tracks, irregular sampling rates, and location error. For our core analyses, two individuals were said to “encounter” one another if they came within 100 m of each other, and the time of the encounter was defined as the time at which there was the shortest distance between the pair of animals involved in the encounter. Our 100 m threshold for defining encounters, though ultimately arbitrary, is informed by previous studies. Specifically, 100 m is a highly conservative threshold compared to previous studies of carnivore encounter dynamics (800 m in [ 26 ], 500 m in [ 5 ] and [ 9 ], and 200 m in [ 42 ]). Though we focused the bulk of our results and discussion on this 100 m threshold, we also conducted a comparative analysis in which we considered encounter thresholds ranging from 50 to 500 m.
Once encounters were identified, we fit a series of continuous-time movement models to the tracking data for each individual in each pair before, and separately, after, the encounter. The fitted models included the Independent and Identically Distributed (IID) process, which features uncorrelated positions and velocities; the Ornstein–Uhlenbeck (OU) process, which features correlated positions but uncorrelated velocities (Uhlenbeck and Ornstein 1930); and an OU-Foraging (OUF) process, which features both correlated positions and correlated velocities [ 13 , 14 ].
We followed procedures developed in Fleming et al. [ 18 ] and summarized in Silva et al. [ 53 ] for fitting these models using different maximum likelihood-based approaches depending on the amount of information that the movement tracks provided about space use. Movement tracks can be distinguished in terms of their home range effective sample size (hereafter, ESS, but not to be confused with evolutionarily stable strategy), which measures the information content in a tracking dataset with autocorrelation in comparison to the information content of a dataset with the same number of observations, but where all observations are independent. With analogies to the conceptual distinction between population size and effective population size in population genetics, the ESS of a range-resident movement track is always less (and sometimes dramatically less) than the number of observations in the movement track, with the magnitude of the decrease in ESS (relative to the same number of independent observations) being proportional to the strength of autocorrelation in the data. Thus, in the context of home range analysis, ESS is not a ‘number of datapoints’ measure, but is instead interpretable as the number of ‘home range crossing equivalents’ that occurred over the duration of the track. Roughly speaking, ESS is approximately the total duration of the tracking dataset divided by the characteristic home range crossing time. Following methods of Fleming et al. [ 18 ] and Silva et al. [ 53 ], bear encounters that resulted in one or more of the partial movement tracks (i.e., the portions of the track before or after the encounter) having ESS < 2 were excluded as data-deficient, whereas partial tracks with 2 < ESS < 5 were fit via bootstrapped perturbative hybrid residual maximum likelihood to reduce estimation biases as much as possible. We used AIC c -based model selection to identify the best model for use in delineating each individual’s home range before versus after the encounter. Individuals that had an IID selected model were rechecked and refit.
We then used autocorrelated kernel density estimation (AKDE; [ 15 , 16 , 53 ]) to estimate each individual’s home range before versus after the encounter as the corresponding range distribution (RD) and uncertainty of that distribution for the best-fit movement model. As modern high-resolution animal tracking data have become increasingly available, traditional methods of home range estimation, such as calculation of minimum convex polygons or conventional kernel density estimation, have been demonstrated to be unsuitable [ 39 ]. AKDE is a statistically efficient method for estimation of home ranges of animals whose movement data includes autocorrelation, small sample size, and missing or irregular data [ 53 ].
Having obtained four RD estimates for each encounter (i.e., RDs for each individual in each pair both before and after the encounter), we sought to characterize differences between particular pairs of RDs. Specifically, we wanted to explore if (1) a given individual’s RD changed (by comparing the before encounter and after encounter RDs for that individual) and (2) if differences in space use between individuals changed in association with the encounter (by comparing the overlap between the two individuals’ RDs before the encounter against the overlap between the two individuals’ RDs after the encounter). We calculated differences between RDs using both the Bhattacharyya Distance [ 2 , 61 ] and the proportional overlap. The Bhattacharyya Distance (BD,sometimes referred to as Bhattacharyya Affinity, which is equivalent is a unitless measure of dissimilarity between two probability distributions that takes values of zero for identical distributions and infinity for distributions with no shared support. Because of its probabilistic derivation, BD is readily incorporated into statistical tests. We also report results in terms of the Bhattacharyya Coefficient (BC) or ‘proportional overlap', which is zero for distributions with no shared support and 1 for identical distributions. In contrast to BD which has statistical utility, BC (proportional overlap) is more easily interpretable on both conceptual and visual grounds. Comparisons of individual distances, estimation of RDs, determination of BDs, and calculation of RD proportional overlaps were conducted using ctmm . Foundational code in ctmm for population-level analyses of RDs first appeared in association with Fleming et al. [ 19 ]. New code in ctmm created for this project allows for the propagation of individual-level uncertainties to calculations of population-mean BDs; these updates appear in ctmm version 1.1.0 involving the functions overlap() and meta() .
Pair-level analysis: coyotes
We identified a pair of coyotes that, on 5/26/12, demonstrated a close (66 ± 1 m) encounter, which was about 1 order of magnitude closer than the animals came to each other at any other time during the 8 months that they were simultaneously tracked. Nominal sampling frequency for both individuals ranged from 15 min to 3 h during the tracking periods. Mean (± SD) realized sampling intervals for the two animals were 98 (± 85) minutes and 97 (± 85) minutes over tracking durations of 52 and 43 weeks, respectively. For the before and after encounter periods for both coyotes, effective sample size (ESS) values far exceeded the key estimability threshold of 5 [ 53 ]. These results and other tracking details for the two animals appear in Table S1 . We then investigated aspects of the movement tracks and the spatial consequences that could be attributed to this encounter in detail.
We estimated RDs for each individual before and after the encounter, and determined the degree of spatial overlap between RDs as described above. To provide further support for a change in the spatial overlap between RDs as a consequence of the encounter, we estimated RD overlap at eight other times (12/19/11, 1/16/12, 2/13/12, 3/11/12, 4/8/12, 5/6/12, 6/3/2012, 6/30/2012, spanning the period over which both animals were simultaneously tracked) when the animals were actually far apart, which we termed “null encounters.” RDs for each individual and RD overlaps (both within and between individuals) were calculated before and after each null encounter as described above. This allowed us to verify that any change in RD overlap following the real encounter was a true signal different from any changes in RD that could be calculated for alternative time points at which the coyotes were much farther apart.
Lastly, we calculated the ballistic length scale for each individual as a running measure across 60 days of movement data. Ballistic length scale ( \(\text{denoted} \,{l}_{v})\) is a measure of linearity in movement [ 41 , 57 ]. That is, \({l}_{v}\) quantifies the average distance over which linear (directed) motion is maintained by a moving animal, and is calculated by:
where the parameters \({\tau }_{v}\) (timescale of autocorrelation in velocity; seconds), \({\tau }_{p}\) (timescale of autocorrelation in position; seconds), and \({\sigma }_{p}\) (spatial variance in movement; m 2 ) are estimated via the ctmm package from an OUF model for range-resident movement (which was the best-fit model for these two coyotes). This allowed us to quantify the directedness of each individual’s movement [ 41 ] before and after the encounter.
Population-level analysis: Grizzly Bears
We next tested the hypothesis that changes in range overlap following encounters are consistent features of a population of grizzly bears. Range distributions (RDs) and changes in overlap associated with encounters were calculated for each pair of bears that came within 100 m of each other (N = 32 pairs, which includes two bear-pairs with encounters in different years). Nominal sampling frequency for individuals ranged from 2 min to 24 h with mean (± SD) realized sampling intervals of 17 (± 27) hours and tracking durations of 73 (± 67) days. Effective sample size (ESS) values exceeded the key threshold of 5 for all encounter-involved bears, for both the before and after encounter periods (Table S2). These data did not provide the level of pairwise detail that was available with the single pair of coyotes, but because we had access to many more pairs of bears and thus more resulting encounters, the grizzly dataset lent itself to population-level analyses.
We tested the statistical significance between RD overlaps before versus after encounters on the whole population using a χ 2 inverse-Gaussian ( χ 2 - IG ) meta-analysis framework [ 19 ]. This approach employs a non-linear hierarchical model developed for estimating population-mean home-range parameters from individual home-range estimates, while propagating the individual-level uncertainties [ 19 ]. These methods are particularly useful for population-level hypothesis testing because any among-individual differences in the amount or quality of movement data available for estimating RDs is captured by the before and after uncertainty estimates of the individual pairs, which are then propagated into population-level estimates and used in making the final population-level comparison. That is, unlike simpler analyses conducted outside of a formal metanalysis framework (i.e., analyses conducted on point estimates), the uncertainty propagation employed here explicitly mitigates against problems stemming from the possibility that individuals (or the track components before versus after an encounter), differ in available data. The methods, available in the R package ctmm , model the individual parameters with an inverse-Gaussian population-level distribution and the individual parameter estimates with scaled-χ2 individual-level distributions, which are appropriate for individual-scale parameter estimates that could have large corresponding uncertainties (such as home-range size and distance). Analyses return a statistical comparison between models assuming that the before-encounter and after-encounter RDs are (or are not) distinguishable. The difference between RDs is measured via the BD, and the significance of any difference is quantified as a p -value.
Most analyses and graphics were completed on a laptop computer in the R statistical package (v.4.2.2; [ 47 ]) using the tidyverse (v. 1.3.2; [ 59 ]), ctmm (v.1.2.1; [ 17 ]) and geosphere (v. 1.5.18; [ 24 ]) packages. The only exception was the encounter overlap analyses, which were calculated on a high performance computing system using R (4.1.1 [ 47 ]) and the tidyverse (v. 1.3.0) and ctmm (v. 1.1.1) packages.
Coyote analysis
Basic data on the movement tracks for coyotes PEC068 and PEC088 appear in Table S1 . The coyotes encountered each other around 3:01 AM on 26 May 2012, coming within 66 m of each other. This is by far the closest they came to each other during an ~ 8-month window of simultaneous tracking (median distance: 7249 m, IQR: 5225–9056 m; Fig. 1 A).
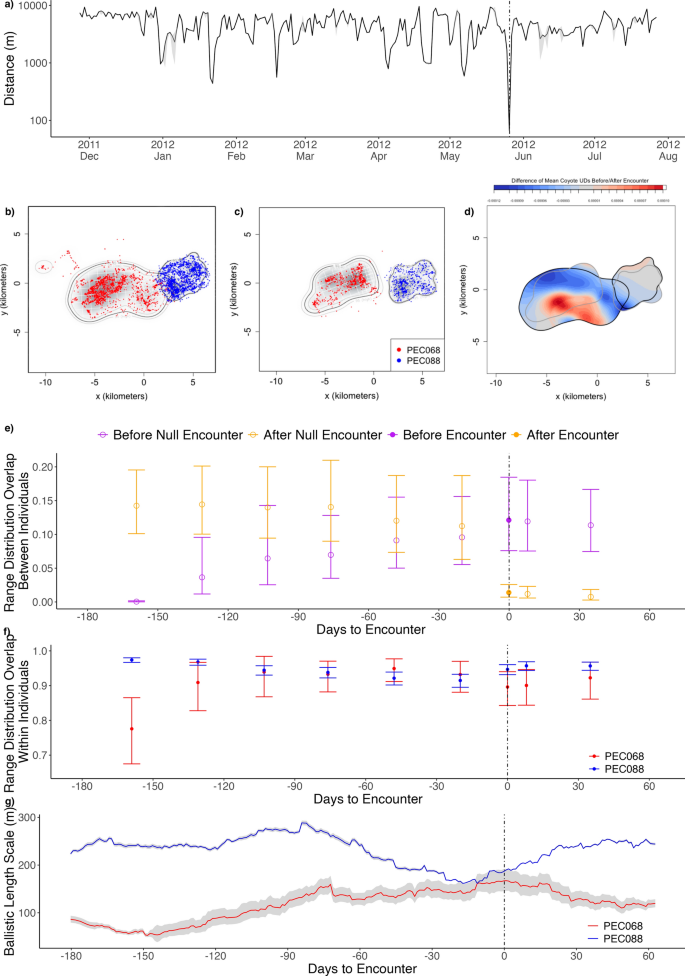
Detailed analyses of a close encounter between two coyotes. A provides inter-individual distances between coyote individuals PEC068 and PEC088 in Ontario, Canada, over an ~ 8-month period in 2011–2012. When missing data necessitated interpolation of coyote positions, estimated inter-individual distances (± 95% CI as gray shading) are plotted. GPS tracking data indicate that the coyotes’ movements brought them within 66 m of each other on 26 May 2012 (shown as day 0). B and C plot range distributions (RDs) (± 95% CI) for the coyotes before and after this encounter, respectively, calculated as range distributions in the R package ctmm . Note the spatial changes by PEC068, reducing overlap with PEC088 after versus before the encounter (ΔAICc = 24.04). D reveals that the two coyotes’ home ranges were completely disjunct after the encounter as judged by the 95% contours of their respective RD. Furthermore, a region in the western part of PEC068’s original RD had the greatest increase in utilization after the encounter, whereas a region in the eastern part of PEC068’s original RD had the greatest decrease in utilization ( D ). E provides the proportional overlap (Bhattacharyya Coefficient ± 95% CI) between individuals based on their RDs before (purple) and after (orange) the real encounter (denoted by solid symbols and the dashed vertical line) compared with similar overlaps measured, for comparative purposes, for alternative ‘null-encounter’ dates when encounters did not occur (open symbols). F provides the proportional overlap within individuals for the same real and null encounters dates as in E , revealing the RD of PEC088 was almost completely static, whereas the RD of PEC068 showed a nonsignificant 15% decrease in overlap for the real encounter date. G gives each individual’s ballistic length scale (± 95% CI) calculated on a running basis for 60-day windows. Note that the ballistic length scale of PEC068 (whose RD changed following the encounter, decreasing inter-individual RD overlap) decreased by ~ 50% in the 60 days after the encounter whereas that of PEC088 increased by ~ 60%
In the months prior to this encounter, the coyotes’ RDs overlapped by 12.1% (Fig. 1 B); following the encounter, the RD of PEC068 changed substantially, reducing the overlap with the RD of PEC088 to only 1.1% (Fig. 1 C). As measured by the 95% contours of the coyotes’ respective RDs, the reduction in overlap was even greater, with the animals’ 95% RDs after the encounter showing zero overlap (Fig. 1 D). A region in the western part of PEC068’s home range showed the greatest increase in activity after the encounter, whereas an area in the eastern part of PEC068’s home range, near its area of overlap with PEC088’s home range, showed the greatest decrease in utilization (Fig. 1 D). The 88% reduction in RD overlap following the encounter (Fig. 1 E, for the real encounter date) is significant: a model in which the RDs overlap less after an encounter versus before the encounter is better supported by 24.04 ΔAICc units versus a model in which the before versus after distributions are not distinguishable. In contrast, several alternative ‘null encounter’ dates in the four months preceding the observed encounter yielded no evidence of changes in RD overlap (Fig. 1 E), whereas the very low levels of overlap between the coyotes’ RD for two null encounter dates after the real encounter indicated that the changes in overlap occurring as a consequence of the real encounter were long-lasting. The two earliest null encounter dates actually show increases in before versus after RD overlap between individuals, which are attributable to increases in eastward foraying activity by PEC068 into the home range of PEC088 while that animal was far away from the zone of overlap between the two animals’ RDs (Fig. 1 E).
Compared to the observed changes in between-individual RD overlap, within-individual range overlap changed relatively little, based on either the real encounter date or the null encounter dates (Fig. 1 F). PEC068 exhibited less RD overlap after versus before the encounter (88%) than did PEC088 (96%), but with large confidence intervals. Given those intervals, we found no difference between the within-individual RD overlap values for the real encounter, nor for most of the null encounters (the first being the only exception). As noted above, the Bhattacharyya Coefficient values quantify overall overlap between the two probability distributions with respect to probability density. Consequently, this measure incorporates elements of both RD size (for instance, the area enclosed by the location of the 95% contours of the RDs) and the relative intensity of space use within the RDs. Both of these attributes provide perspectives on the animals’ space use. For example, comparing RDs before versus after the encounter within individuals provides context that is helpful for understanding the above-mentioned between-individual differences in RD. Specifically, the area enclosed by PEC068’s RD after the encounter was only 52.6% the size of the corresponding area before the encounter, whereas the area enclosed by PEC088’s RD effectively did not change following the encounter (increasing by only 0.74%). Thus, the observed reduction in RD overlap between individuals (Fig. 1 E) derived largely from a 47.4% (i.e., 100–52.6%) shrinkage in the size of PEC068’s RD that involved only a modest 12% (i.e., 100–88%) change in the before versus after RD overlap for that individual (Fig. 1 F). This result implies that the portion of PEC068’s RD that it used with intensity did not change much as a result of the encounter, but that the outer fringe area that it used with less intensity changed greatly, a result that was also evident in Fig. 1 D. Similarly, in the 60 days after the encounter, the ballistic length scale (which is calculated over a 60d running window, see Methods) for PEC068 decreased by ~ 50% (Fig. 1 G), implying a substantial reduction in longer distance linear movements (i.e., the aforementioned forays). In contrast, the ballistic length scale for PEC088 increased by ~ 60% after the encounter, implying more extensive linear movements (Fig. 1 G).
Grizzly bear analysis
Evaluated at the population level, pairwise analyses of encounters between grizzly bears also demonstrated changes in RD overlap following encounters, but only in certain situations. Encounter-related changes in RD overlap were not significant for the entire set of 32 pairs of bears. However, provided at least one of the bears involved in an encounter within 100 m during late fall (1 September–30 November) was accompanied by one or more cubs (n = 9 pairs in the ‘cubs involved late fall’ category), the BD between the bears’ RDs increased significantly following the encounter (i.e., the overlaps between their respective RDs decreased; Fig. 2 ).
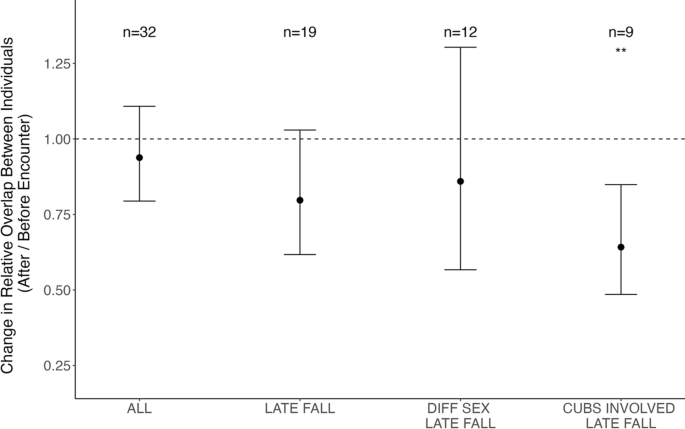
Mean (± 95% CI) relative change in RD overlap between pairs of Canadian grizzly bears that encountered each other at distances < 100 m. The y-axis plots the ratio of the RD overlap after an encounter versus RD overlap before the encounter; thus, values less than 1 indicate decreases in pairwise overlap. Results are shown for all pairs of bears exhibiting an encounter, all late fall encounters (1 September–30 November), all late fall encounters involving different-sex pairs, and all late-fall encounters in which at least one individual was accompanied by cubs. Asterisks above the error bars indicate significant (** p < 0.05) changes in overlap
Figure S1 provides plots of proportional overlap of RDs between the individuals in each pair of bears before versus after an encounter, and Figure S3 provides before versus after encounter RD maps for the 9 pairs of bears in the ‘cubs involved late fall’ category. We also visualized encounter-related changes in RD overlap within individuals in each pair before versus after an encounter to identify whether one bear involved in a given encounter altered its RD more than the other bear (Fig. S2). For the nine Late Fall encounters < 100 m involving cubs, we found no pattern as to whether individuals with cubs altered their RDs more or less than did individuals without cubs.
Repeating the above analyses using a distance threshold of < 50 m yielded a similarly strong effect for late fall encounters including cubs. However, with more liberal thresholds of 200–500 m, we found no population-level evidence for changes in RD overlap for late fall encounters including cubs (Fig. 3 ). For thresholds of 50–200 m, late fall encounters (including both those with and without cubs) showed marginal reductions in overlap (0.05 < p < 0.10). Late fall encounters between male and female bears also led to significantly reduced overlap for encounters < 200 m, but not for other encounter distances.
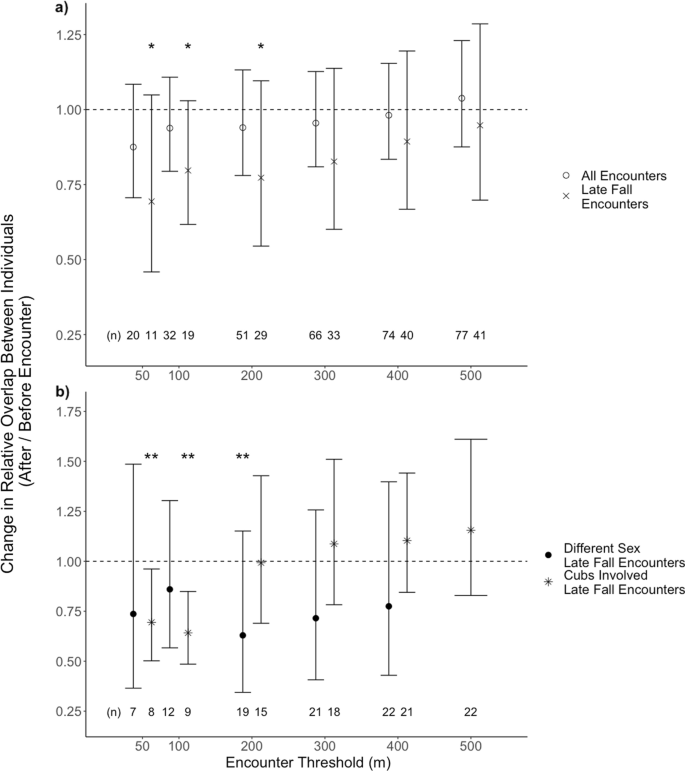
Mean (± 95% CI) relative change in RD overlap between pairs of Canadian grizzly bears for different definitions of what constitutes an encounter. The y-axis plots the ratio of the RD overlap after an encounter versus RD overlap before the encounter; thus, values less than 1 indicate decreases in pairwise overlap. Results in A are shown for all pairs exhibiting an encounter and all pairs involving an encounter in late fall (1 September – 30 November). B plots results for late fall encounters involving different-sex pairs and late fall encounters in which at least one individual was accompanied by cubs. Note that sample sizes in both panels depend on the definition of encounter distance. For late fall different sex encounters, no new encounters were identified after extending the radius from 400 to 500 m, so that duplicate result is not plotted. Asterisks above the error bars indicate marginally nonsignificant (* p < 0.1) and significant (** p < 0.05) changes in overlap
Encounters between pairs of bears, and especially encounters in late fall, occurred much closer to carcass pits (locations where road kill and hunters’ gut dumps were systematically deposited) than did generic non-encounter fixes between pairs of bears (Fig. S3).
Here we have demonstrated how encounters, defined on the basis of spatial proximity in pairwise analyses of GPS tracks for mammalian carnivores, can be associated with changes in range distributions (RDs). Through a combination of detailed pairwise analysis and population-level hypothesis testing, we characterized changes in the overlap between individuals’ ranges and explored how seasonality, sex, and the presence of young influence the spatial consequences of encounters. Overall, we found evidence suggesting that animals can sometimes undertake long-term, large-scale spatial changes in their range distributions in response to close intraspecific encounters that have the potential for conflict, corroborating the use of home range overlap to quantify encounter potential (e.g., [ 40 ]).
The coyote data suggest a scenario whereby the encounter yielded an outcome in which, for the remainder of the tracking period, one individual, PEC068, was a clear ‘loser’ with respect to occupancy of contested space. Based on the 95% contour estimated by ctmm, the range distribution of PEC068 decreased by 47% following the encounter, and that coyote no longer used the easternmost part of the range it occupied before the encounter (Fig. 1 B–D). In the context of a larger, regional study of coyote home ranges and territoriality [ 57 ], PEC068 was determined to have exhibited an ambiguous space use pattern, and herein is considered a “non-territorial resident,” exhibiting relatively weak range fidelity and undertaking multiple forays outside of its range that intruded on territories of other coyotes, including but not limited to PEC088. As such, the before versus after encounter RDs estimated for PEC068 would correspond to ‘undefended home ranges’ rather than territories per se, and the spatial changes in RD overlap observed for this animal (Fig. 1 E,F) appear to have derived from a post-encounter reduction in its foray activity rather than contraction of a defended territory (Fig. 1 D,G).
Detailed inspection of the coyotes’ tracking data revealed that the close encounter between the coyotes occurred near the intersection between two fields separated by a hedgerow, so it is almost certain that at least one of the coyotes detected the other. Indeed, accelerometer data from around the time of the coyotes’ encounter (Wheeldon, unpublished data) suggest that the encounter may have been a ‘close-call’ for PEC068 because that individual appeared to have gone undetected and thereby avoided a physical confrontation or direct aggression. More specifically, PEC068 was inactive for a period of ~ 50 min beginning shortly (~ 10 min) after the encounter, indicating that it may have bedded down to hide from PEC088, which continued to be active for ~ 35 min post-encounter and then became inactive, at which point PEC068 became active and eventually left the territory of PEC088.
The ballistic length scale results support the above interpretation in that PEC068 decreased the linearity of its movement following the encounter, presumably reflecting the reduction in its foray activity (i.e., out-and-back movements), whereas PEC088 increased the linearity of its movement following the encounter, which may partly reflect increased territorial behavior in the form of patrolling the perimeter (note the relative increase in the intensity of PEC088’s usage of the western part of its range following the encounter in Fig. 1 C). The encounter occurred during the denning season and PEC088—based on its reproductive history—may have had pups at the time, which may have heightened the intensity of its encounter and subsequent activity.
Only PEC088 continued to use the disputed area over a period of more than three months following the encounter (Fig. 1 C, D). This observation suggests that “ownership” of this area was being respected by the losing party (PEC068), reflecting a kind of low-conflict coexistence known as the “bourgeois strategy” [ 32 , 51 ]). Theory has predicted that this strategy should be selected for in most natural environments, because it reduces the overall risk of injury for all parties involved [ 32 , 51 ]. Further, theory also suggests that the “bourgeois strategy” should occur when animals have the liberty of reducing their activity while maintaining their energetic budget [ 35 ]. Considering that coyotes can substantially vary their daily activity and diet [ 27 ], PEC068’s reduction in foray activity following the encounter that resulted in decreased overlap with the territory of PEC088 (Fig. 1 D), did not necessarily involve a loss of resources. Combining this support with previous studies indicating that coyotes forage optimally [ 23 ], there is mounting evidence suggesting current theory can contribute to the successful prediction of coyote behavior.
Grizzly bears
In general, encounters between bears were only associated with significant changes in RD overlap under certain circumstances, specifically in late fall, with cubs present, and at encounter distances ≤ 100 m (Figs. 2 , 3 ). In contrast, when we broadened the definition of encounter to include proximity events occurring beyond this 100 m encounter threshold, or when we included encounters at other times of the year, we found little evidence for a population-level association between encounters and changes in RD overlap. Beyond the late-fall with cubs results, only male–female encounters within the 200 m threshold exhibited significant changes in RD overlap (Fig. 3 ). During late fall, encounters between bears occurred disproportionately close to carcass pits (Fig. S3), and may have involved heightened aggression during a period of increased resource competition in the weeks preceding hibernation.
Previous studies using animal movement data to investigate the consequences of encounters in other systems have relied upon a broad range of threshold distances, including some as great as 500–800 m. Our results suggest caution in relying upon such large distances to delineate encounters between tracked individuals. Here, very liberal definitions of encounters indicated the absence of encounter-associated changes in space use that were in fact observable with more restrictive assumptions (Figs. 2 , 3 ). Moreover, shorter distance thresholds when defining encounters were associated with stronger spatial changes, likely because those shorter encounter thresholds were more apt to correspond to actual interactions (i.e., one or both individuals detecting the presence of the other and undertaking a behavioral change).
The importance of an encounter depends not just on proximity, but also on perceptual abilities (visual, olfactory, auditory) and spatial context (e.g., local conditions such as substrate, vegetation type and density, and other factors that can influence individuals’ movements, detection abilities, and decisions to interact). For example, in the case of the bears, the encounters occurred primarily in broken timber such that proximity may not always have led to mutual detection, which could have contributed to the heterogeneity in pairwise results even for encounter thresholds of 100 m (Figs. 2 , S1 , S2 ). Experimental work involving human proximity to wolves presents similar ideas, and emphasizes that encounters can be decidedly one-sided in terms of detection and spatial response [ 56 ]. Thus, proximity and perception together determine whether an encounter translates into an interaction with a behavioral component.
Caveats and limitations
Many possible explanations exist for temporal changes in an animal’s home range. Further, because RDs can only be calculated using movement data collected over an extended period of time, there exist increased opportunities for factors beyond a specific encounter to shape animals’ use of space during a tracking period. For example, resource-related events (e.g., the phenology of vegetation green-up, (un)successful hunting experiences) are widely known to influence how animals move and where they spend their time [ 1 , 21 ]. A related issue is that, in almost all field studies, only a portion of a population will be monitored, so encounters between tracked and untracked individuals will go unobserved and remain unspecified with regard to time. When such events are unobserved, it is generally difficult to infer anything about the degree to which they shape movement. Consequently, we cannot exclude the possibility that other, unobserved factors beyond pairwise close encounters were responsible for the changes in RDs that we documented.
However, a distinct advantage of our approach is that we can evaluate potential changes in overlap that are tied specifically to temporally precise encounters between tracked individuals. Indeed, the null encounters approach that we implemented for the coyotes allowed testing whether time points other than that of the encounter event were associated with comparable changes in RDs. Thus, when detailed analyses of null encounter dates reveal no effect on overlap, they provide an extra level of confidence that any observed changes in overlap are associated with an observed encounter. Conversely, if a null encounter date were associated with a substantial change in overlap, it would suggest that some unobserved event other than a pairwise encounter between tracked individuals had an influence on their RDs. Satisfactory evaluation of the potential for long-term, gradual processes (rather than temporally precise events) to influence range overlaps seems unlikely using the breakpoint approach that we implemented here, and would necessitate alternative approaches such as those involving spatial correlates of movement.
Future applications
A variety of future directions are possible. For example, future encounter-based research could conduct sensitivity analyses to identify the encounter distances that are most strongly associated with changes in space use between tracked individuals that occur in close proximity. Such analyses could have the additional benefit of providing insight into just how big are the perceptual ranges of the animals involved [ 3 , 12 , 31 , 62 ]. Likewise, one could use the same Bhattacharyya methods that we employed here to test a hypothesis that the range distribution for a given animal, or the range overlap between a pair of animals, changed in response to the timing of a particular event in their environment (e.g., the onset of berry availability). One would simply use a particular date (or range of dates) as a specific breakpoint for the movement tracks to evaluate whether the RD (or RDs) after the event differed from that before the event. Future work could also investigate to what extent multiple behavioral shifts are consistent within individuals (indicating winner/loser dynamics in the context of territorial interactions). For example, little-investigated theoretical issues, such as transitions between ideal free and ideal despotic distributions, could be investigated by connecting encounter data with assessments of resources lost and gained as a result of spatial changes in occupied ranges.
Increasing availability of high resolution movement data (e.g., [ 38 ] facilitates identification of putative encounters between individual animals based on proximity, necessitating methods for assessing the long-term consequences of such encounters. Here, we explored how statistical methods for population-level home range analysis [ 19 ] could be repurposed to gauge whether and to what extent the spatial overlap between individuals’ range distributions changed following an encounter compared to conditions prior to the encounter. We showed how different analytical approaches are possible depending on the type and amount of movement data available. Detailed analyses of a pair of high-resolution coyote tracks demonstrated how the overlap between the animals’ range distributions was reduced following the encounter when one of the coyotes altered its movement behavior. In contrast, when movement data were available for many grizzly bears in the same landscape, we framed the problem in terms of hypothesis tests, demonstrating that significant post-encounter changes in range distribution overlap occurred when females-with-cubs were involved in the encounter in late fall. We also demonstrated that smaller spatial thresholds for the delineation of encounters were associated with more frequent and greater changes in range overlap. With caveats and limitations, we suggest that encounter-based analyses can be used to interpret changes in space use, identify distances at which individuals’ proximity to one another may alter behavior, and test population-level hypotheses concerning the potential for direct encounters to alter individuals’ space use.
Availability of data and materials
Movement data for the coyotes and grizzly bears are posted on Movebank.org as datasets 1614661371 and 1044288582, respectively. Statistical tools for estimating, manipulating, and comparing home ranges from movement data are implemented in the open-source R package ctmm . R scripts used to carry out specific analyses for this study are openly available on GitHub at https://github.com/anagkrish/encounter_homerangeshift . Upon acceptance, movement data for the coyotes and grizzly bears will be provided via Movebank.org as datasets 1614661371 and 1044288582, respectively. Statistical tools for estimating, manipulating, and comparing home ranges from movement data are available in the open-source R package ctmm . Upon acceptance, R scripts used to carry out specific analyses for this study will be provided via https://zenodo.org/records/13529988 .
Aikens EO, Mysterud A, Merkle JA, Cagnacci F, Rivrud IM, Hebblewhite M, Hurley MA, Peters W, Bergen S, De Groeve J, Dwinnell SP. Wave-like patterns of plant phenology determine ungulate movement tactics. Curr Biol. 2020;30(17):3444–9.
Article CAS PubMed Google Scholar
Bhattacharyya A. On a measure of divergence between two statistical populations defined by their probability distributions. Bull Calcutta Math Soc. 1943;35:99–109.
Google Scholar
Boonman A, Bar-On Y, Cvikel N, Yovel Y. It’s not black or white—on the range of vision and echolocation in echolocating bats. Front Physiol. 2013;4:248.
Article PubMed PubMed Central Google Scholar
Brashares JS, Werner JR, Sinclair ARE. Social ‘meltdown’ in the demise of an island endemic: Allee effects and the Vancouver Island marmot. J Anim Ecol. 2010;79(5):965–73.
Article PubMed Google Scholar
Broekhuis F, Madsen EK, Keiwua K, Macdonald DW. Using GPS collars to investigate the frequency and behavioural outcomes of intraspecific interactions among carnivores: a case study of male cheetahs in the Maasai Mara, Kenya. PLoS ONE. 2019;14:e0213910-e213916.
Article CAS PubMed PubMed Central Google Scholar
Calabrese JM, Fleming CH, Gurarie E. CTMM: an R package for analyzing animal relocation data as a continuous-time stochastic process. Methods Ecol Evol. 2016;7(9):1124–32.
Article Google Scholar
Cheraghi F, Delavar MR, Amiraslani F, Alavipanah K, Gurarie E, Hunter L, Ostrowski S, Jowkar H, Fagan WF (2019) Inter-dependent movements of Asiatic Cheetah and Persian Leopard in their environment. Zoology in the Middle East. 1–10.
Corriale MJ, Muschetto E, Herrera EA. Influence of group sizes and food resources in home-range sizes of capybaras from Argentina. J Mammal. 2013;94:19–28.
Courbin N, Loveridge AJ, Macdonald DW, Fritz H, Valeix M, Makuwe ET, Chamaillé-Jammes S. Reactive responses of zebras to lion encounters shape their predator–prey space game at large scale. Oikos. 2016;125(6):829–38.
Doncaster CP. Non-parametric estimates of interaction from radio-tracking data. J Theor Biol. 1990;143(4):431–43.
Ellison N, Hatchwell BJ, Biddiscombe SJ, Napper CJ, Potts JR. Mechanistic home range analysis reveals drivers of space use patterns for a non-territorial passerine. J Anim Ecol. 2020;89(12):2763–76.
Fagan WF, Gurarie E, Bewick S, Howard A, Cantrell S, Cosner C. Perceptual ranges, information gathering, and foraging success in dynamic landscapes. Am Nat. 2017;189:474–89.
Fleming CH, Calabrese JM, Mueller T, Olson KA, Leimgruber P, Fagan WF. From fine-scale foraging to home ranges: a semivariance approach to identifying movement modes across spatiotemporal scales. Am Nat. 2014;183(5):E154–67.
Fleming CH, Calabrese JM, Mueller T, Olson KA, Leimgruber P, Fagan WF. Non-Markovian maximum likelihood estimation of autocorrelated movement processes. Methods Ecol Evol. 2014;5(5):462–72.
Fleming CH, Fagan WF, Mueller T, Olson KA, Leimgruber P, Calabrese JM. Rigorous home range estimation with movement data: a new autocorrelated kernel density estimator. Ecology. 2015;96(5):1182–8.
Fleming CH, Calabrese JM. A new kernel density estimator for accurate home-range and species-range area estimation. Methods Ecol Evol. 2017;8(5):571–9.
Fleming CH, Calabrese JM (2023). CTMM: Continuous-time movement modeling, https://github.com/ctmm-initiative/ctmm .
Fleming CH, Noonan MJ, Medici EP, Calabrese JM. Overcoming the challenge of small effective sample sizes in home-range estimation. Methods Ecol Evol. 2019;10(10):1679–89.
Fleming CH, Deznabi I, Alavi S, Crofoot MC, Hirsch BT, Medici EP, Noonan MJ, Kays R, Fagan WF, Sheldon D, Calabrese JM. Population-level inference for home-range areas. Methods Ecol Evol. 2022;13(5):1027–41.
Gurarie E, Ovaskainen O. Towards a general formalization of encounter rates in ecology. Thyroid Res. 2013;6(2):189–202.
Gurarie E, Bracis C, Brilliantova A, Kojola I, Suutarinen J, Ovaskainen O, Potluri S, Fagan WF. Spatial memory drives foraging strategies of wolves, but in highly individual ways. Front Ecol Evol. 2022;10:768478.
Harestad AS, Bunnel FL. Home range and body weight–A reevaluation. Ecology. 1979;60:389–402.
Hernández L, Parmenter RR, Dewitt JW, Lightfoot DC, Laundré JW. Coyote diets in the Chihuahuan Desert, more evidence for optimal foraging. J Arid Environ. 2002;51(4):613–24.
Hijmans R (2022) Geosphere: Spherical Trigonometry. R package version 1.5–14, https://CRAN.R-project.org/package=geosphere .
Joo R, Etienne MP, Bez N, Mahévas S. Metrics for describing dyadic movement: a review. Mov Ecol. 2018;6:1–17.
Jordan NR, Buse C, Wilson AM, Golabek KA, Apps PJ, Lowe JC, Van der Weyde LK, Weldon McNutt J. Dynamics of direct inter-pack encounters in endangered African wild dogs. Behav Ecol Sociobiol. 2017;71(8):1–12.
Kitchen AM, Gese EM, Schauster ER. Changes in coyote activity patterns due to reduced exposure to human persecution. Can J Zool. 2000;78(5):853–7.
Klauder KJ, Borg BL, Sivy KJ, Prugh LR. Gifts of an enemy: scavenging dynamics in the presence of wolves ( Canis lupus ). J Mammal. 2021;102(2):558–73.
Laidre KL, Born EW, Gurarie E, Wiig Ø, Dietz R, Stern H. Females roam while males patrol: divergence in breeding season movements of pack-ice polar bears ( Ursus maritimus ). Proc R Soc B: Biol Sci. 2013;280(1752):20122371.
Lamb CT, Smit L, Mowat G, McLellan B, Proctor M. Unsecured attractants, collisions, and high mortality strain coexistence between grizzly bears and people in the Elk Valley, southeast British Columbia. Conserv Sci Pract. 2023;5(10):e13012.
Martinez-Garcia R, Fleming CH, Seppelt R, Fagan WF, Calabrese JM. How range residency and long-range perception change encounter rates. J Theor Biol. 2020;498:110267.
Maynard Smith J. Evolution and the theory of games. Cambridge University Press; 1982.
Book Google Scholar
Mcloughlin PD, Ferguson SH, Messier F. Intraspecific variation in home range overlap with habitat quality: a comparison among brown bear populations. Evol Ecol. 2000;14:39–60.
Mech LD, Harper EK. Differential use of a wolf, Canis lupus , pack territory edge and core. Can Field-Nat. 2002;116:315–6.
Menezes JFS, Oliveira-Santos LGR. Cautious individuals have non-invadable territories, according to an evolutionary mechanistic model. Ecol Model. 2021;449:109551.
Moorcroft PR, Lewis MA, Crabtree RL. Mechanistic home range models capture spatial patterns and dynamics of coyote territories in Yellowstone. Proc R Soc B: Biol Sci. 2006;273(1594):1651–9.
Moorcroft PR, Lewis MA. Mechanistic home range analysis. (MPB-43). In Mechanistic Home Range Analysis. (MPB-43) . Princeton University Press, 2013.
Nathan R, Monk CT, Arlinghaus R, Adam T, Alós J, Assaf M, Baktoft H, Beardsworth CE, Bertram MG, Bijleveld AI, Brodin T. Big-data approaches lead to an increased understanding of the ecology of animal movement. Science. 2022;375(6582):eabg1780.
Noonan MJ, Tucker MA, Fleming CH, Akre TS, Alberts SC, Ali AH, Altmann J, Antunes PC, Belant JL, Beyer D, Blaum N, Böhning-Gaese K, Cullen L, Paula RC, Dekker J, Drescher-Lehman J, FarwigN, Fichtel C, Fischer C, Ford AT, Goheen JR, Janssen R, Jeltsch F, Kauffman M, Kappeler PM, Koch F, LaPoint S, Markham AC, Medici EP, Morato RG, Nathan R, Oliveira-Santos LGR, Olson K, Patterson BD, Paviolo A, Ramalho EE, Rösner S, Schabo DG, Selva N, Sergiel A, Xavier da Silva M, Spiegel O, Thompson P, Ullmann W, Zieba F, Zwijacz-Kozica T, Fagan WF, Mueller T, Calabrese JM. A comprehensive analysis of autocorrelation and bias in home range estimation. Ecol Monograph. 2019;89(2):e01344.
Noonan MJ, Martinez-Garcia R, Davis GH, Crofoot MC, Kays R, Hirsh BT, Caillaud D, Payne E, Sih A, Sinn D, Spiegel O, Fagan WF, Fleming CH, Calabrese JM. Estimating encounter location distributions from animal tracking data. Methods Ecol Evol. 2021;12:1158–73.
Noonan MJ, Martinez-Garcia R, Fleming CH, De Figueiredo BG, Ali AH, Attias N, Belant JL, Beyer Jr DE, Berteaux D, Bidner LR, Boone R et al. The search behavior of terrestrial mammals. bioRxiv. 2022-12.
Périquet S, Fritz H, Revilla E, Macdonald DW, Loveridge AJ, Mtare G, Valeix M. Dynamic interactions between apex predators reveal contrasting seasonal attraction patterns. Oecologia. 2021;195(1):51–63.
Pletenev A, Kruchenkova E, Mikhnevich Y, Rozhnov V, Goltsman M. The overabundance of resources leads to small but exclusive home ranges in Arctic fox ( Vulpes lagopus ) on Bering Island. Polar Biol. 2021;44:1427–43.
Polansky L, Wittemyer G. A framework for understanding the architecture of collective movements using pairwise analyses of animal movement data. J R Soc Interface. 2011;8(56):322–33.
Powell RA. Animal home ranges and territories and home range estimators. Res Tech Anim Ecol Controvers Conseq. 2000;442:65–110.
Powell RA, Mitchell MS. What is a home range? J Mammal. 2012;93:948–58.
R Core Team (2022). R: A language and environment for statistical computing. R Foundation for Statistical Computing, Vienna, Austria, https://www.R-project.org/ .
Riotte-Lambert L, Benhamou S, Chamaillé-Jammes S. How memory-based movement leads to nonterritorial spatial segregation. Am Nat. 2015;185(4):E103–16.
Ruprecht J, Eriksson CE, Forrester TD, Spitz DB, Clark DA, Wisdom MJ, Bianco M, Rowland MM, Smith JB, Johnson BK, Levi T. Variable strategies to solve risk–reward tradeoffs in carnivore communities. Proc Natl Acad Sci. 2021;118(35):e2101614118.
Schoepf I, Schmohl G, König B, Pillay N, Schradin C. Manipulation of population density and food availability affects home range sizes of African striped mouse females. Anim Behav. 2015;99:53–60.
Schlägel UE, Signer J, Herde A, Eden S, Jeltsch F, Eccard JA, Dammhahn M. Estimating interactions between individuals from concurrent animal movements. Methods Ecol Evol. 2019;10(8):1234–45.
Sherratt TN, Mesterton-Gibbons M. The evolution of respect for property. J Evol Biol. 2015;28(6):1185–202.
Silva I, Fleming CH, Noonan MJ, Alston J, Folta C, Fagan WF, Calabrese JM. Autocorrelation-informed home range estimation: A review and practical guide. Methods Ecol Evol. 2022;13(3):534–44.
Tórrez-Herrera LL, Davis GH, Crofoot MC. Do monkeys avoid areas of home range overlap because they are dangerous? A test of the risk hypothesis in white-faced capuchin monkeys ( Cebus capucinus ). Int J Primatol. 2020;41:246–64.
Tucker MA, Ord TJ, Rogers TL. Evolutionary predictors of mammalian home range size: body mass, diet and the environment. Glob Ecol Biogeogr. 2014;23(10):1105–14.
Versluijs E, Eriksen A, Fuchs B, Wikenros C, Sand H, Wabakken P, Zimmermann B. Wolf responses to experimental human approaches using high-resolution positioning data. Front Ecol Evol. 2022;10:279.
Visser AW, Kiørboe T. Plankton motility patterns and encounter rates. Oecologia. 2006;148(3):538–46.
Wheeldon T. Population dynamics of eastern coyotes in southeastern Ontario. Ph.D. dissertation, Trent University, Peterborough, Ontario, Canada 2020.
Wickham H, Averick M, Bryan J, Chang W, McGowan LD, François R, Grolemund G, Hayes A, Henry L, Hester J, Kuhn M, Pedersen TL, Miller E, Bache SM, Müller K, Ooms J, Robinson D, Seidel DP, Spinu V, Takahashi K, Vaughan D, Wilke C, Woo K, Yutani H. Welcome to the tidyverse. J Open Source Softw. 2019;4(43):1686. https://doi.org/10.21105/joss.01686 .
Wikelski M, Kays R Movebank: archive, analysis and sharing of animal movement data (Hosted by theMax Planck Institute for Ornithology. www.movebank.org , accessed on July 15, 2022), 2022.
Winner K, Noonan MJ, Fleming CH, Olson KA, Mueller T, Sheldon D, Calabrese JM. Statistical inference for home range overlap. Methods Ecol Evol. 2018;9(7):1679–91.
Zollner PA, Lima SL. Landscape-level perceptual abilities in white-footed mice: perceptual range and the detection of forested habitat. Oikos. 1997;80:51–60.
Download references
W.F.F., C.H.F., and J.M.C. were supported by NSF IIBR 1915347. The University of Maryland provided additional financial support. R.M-G. was supported by FAPESP BIOTA Young Investigator Research Grant no. 2019/05523-8457 and the Simons Foundation through grant no. 284558FY19. This work was partially funded by the Center of Advanced Systems Understanding (CASUS), which is financed by Germany's Federal Ministry of Education and Research (BMBF) and by the Saxon Ministry for Science, Culture, and Tourism (SMWK) with tax funds on the basis of the budget approved by the Saxon State Parliament. The authors acknowledge the University of Maryland supercomputing resources ( http://hpcc.umd.edu ) made available for conducting the research reported in this paper.
Author information
Ananke Krishnan and Qianru Liao are joint second authors.
Authors and Affiliations
Department of Biology, University of Maryland, College Park, MD, USA
William F. Fagan, Ananke Krishnan, Qianru Liao, Christen H. Fleming, Daisy Liao & Justin M. Calabrese
Center for Advanced Systems Understanding (CASUS), Helmholtz-Zentrum Dresden-Rosendorf (HZDR), Görlitz, Germany
Christen H. Fleming, Ricardo Martinez-Garcia, Jorge F. S. Menezes & Justin M. Calabrese
Smithsonian Conservation Biology Institute, National Zoological Park, Front Royal, VA, USA
Christen H. Fleming
Department of Biology, University of Central Florida, Orlando, FL, USA
Department of Biology, University of British Columbia, Kelowna, BC, Canada
Clayton Lamb
Ontario Ministry of Natural Resources and Forestry, Trent University, Peterborough, ON, Canada
Brent Patterson & Tyler Wheeldon
ICTP - South American Institute for Fundamental Research and Instituto de Física Teórica, Universidade Estadual Paulista - UNESP, São Paulo, SP, Brazil
Ricardo Martinez-Garcia
Feline Research Group, Mamirauá Institute for Sustainable Development, Tefé, AM, Brazil
Jorge F. S. Menezes
Department of Biology, The University of British Columbia Okanagan, Kelowna, BC, Canada
Michael J. Noonan
Department of Environmental Biology, SUNY Environmental Science and Forestry, Syracuse, NY, USA
Eliezer Gurarie
Department of Ecological Modelling, Helmholtz Centre for Environmental Research-UFZ, Leipzig, Germany
Justin M. Calabrese
You can also search for this author in PubMed Google Scholar
Contributions
W.F.F., Q.L., and C.H.F conceptualized the study. A.K., Q.L., and D.L. conducted statistical analyses and visualization under guidance from W.F.F, with J.M.C. and C.H.F. providing additional suggestions regarding the statistical analyses. B.P., T.W, and C.L. provided data and biological insights. C.H.F. developed new computer code now included in the open-source R package ctmm. W.F.F. wrote the initial draft with input from A.K., Q.L., and J.S.F.M. All authors contributed to interpretation of results plus review and editing of later stages of the draft.
Corresponding author
Correspondence to William F. Fagan .
Ethics declarations
Ethical approval.
Coyote collars were permitted under Ontario Ministry of Natural Resources and Forestry WACC permits nos. 10-219 through 12-219 and Trent University Animal Care Committee permit no. 10016. Grizzly collars were permitted under Province of British Columbia Capture Permit #CB17-119264200 and University of Alberta Animal Ethics Permit #AUP00002181.
Competing interests
The authors declare no conflicts of interest.
Additional information
Publisher's note.
Springer Nature remains neutral with regard to jurisdictional claims in published maps and institutional affiliations.
Supplementary Information
Additional file 1., rights and permissions.
Open Access This article is licensed under a Creative Commons Attribution-NonCommercial-NoDerivatives 4.0 International License, which permits any non-commercial use, sharing, distribution and reproduction in any medium or format, as long as you give appropriate credit to the original author(s) and the source, provide a link to the Creative Commons licence, and indicate if you modified the licensed material. You do not have permission under this licence to share adapted material derived from this article or parts of it. The images or other third party material in this article are included in the article’s Creative Commons licence, unless indicated otherwise in a credit line to the material. If material is not included in the article’s Creative Commons licence and your intended use is not permitted by statutory regulation or exceeds the permitted use, you will need to obtain permission directly from the copyright holder. To view a copy of this licence, visit http://creativecommons.org/licenses/by-nc-nd/4.0/ .
Reprints and permissions
About this article
Cite this article.
Fagan, W.F., Krishnan, A., Liao, Q. et al. Intraspecific encounters can lead to reduced range overlap. Mov Ecol 12 , 58 (2024). https://doi.org/10.1186/s40462-024-00501-w
Download citation
Received : 04 January 2024
Accepted : 20 August 2024
Published : 30 August 2024
DOI : https://doi.org/10.1186/s40462-024-00501-w
Share this article
Anyone you share the following link with will be able to read this content:
Sorry, a shareable link is not currently available for this article.
Provided by the Springer Nature SharedIt content-sharing initiative
- GPS tracking data
- Canis latrans
- Ursus arctos horribilis
- Spatial overlap
- Perceptual range
- Continuous-time movement modeling
Movement Ecology
ISSN: 2051-3933
- Submission enquiries: [email protected]
- Share full article
Advertisement
Supported by
NASA Extends Boeing Starliner Astronauts’ Space Station Stay to 2025
Persistent concerns with the vehicle’s propulsion systems mean Suni Williams and Butch Wilmore will return home next year in a SpaceX vehicle.
Boeing Starliner Astronauts Will Return to Earth in SpaceX Vehicle
Nasa announced that two astronauts aboard the international space station will have their stay extended by several months and that they will return on a spacex capsule because of problems with the boeing starliner..
“NASA has decided that Butch and Suni will return with Crew 9 next February and that Starliner will return uncrewed. A test flight by nature is neither safe nor routine. And so the decision to keep Butch and Suni aboard the International Space Station and bring the Boeing Starliner home uncrewed is the result of a commitment to safety.” “I talked with Butch and Suni both yesterday and today. They support the agency’s decision fully, and they’re ready to continue this mission on board I.S.S. as members of the Expedition 71 crew. Their families are doing well. Their families understand, just like the crew members when they launch, there’s always an opportunity, there’s always a possibility that they could be up there much longer than they anticipate. So the families understand that. I’m not saying it’s not hard. It is hard. It’s difficult.”

By Kenneth Chang
Two astronauts who have spent months aboard the International Space Station will have to stay there months longer after NASA decided on Saturday that they could not return on Boeing’s troubled Starliner space vehicle. They will return instead on a SpaceX capsule next year.
That decision finally brings clarity to the saga of the two NASA astronauts, Suni Williams and Butch Wilmore, who docked at the space station as part of a test flight of the Boeing vehicle. It also adds to months of difficult problems experienced by Boeing, a dominant aerospace company that has faced embarrassing setbacks in its much larger civilian aviation and defense divisions this year.
“A test flight by nature is neither safe nor routine,” Bill Nelson, the NASA administrator, said during a news conference, “and so the decision to keep Butch and Suni aboard the International Space Station and bring the Boeing Starliner home uncrewed is a result of a commitment to safety.”
Norman Knight, NASA’s flight operations director, said he had talked to Ms. Williams and Mr. Wilmore, and that they backed the extended stay in orbit, which officials have resisted describing as a stranding .
“They support the agency’s decision fully, and they’re ready to continue this mission onboard I.S.S.,” Mr. Knight said.

We are having trouble retrieving the article content.
Please enable JavaScript in your browser settings.
Thank you for your patience while we verify access. If you are in Reader mode please exit and log into your Times account, or subscribe for all of The Times.
Thank you for your patience while we verify access.
Already a subscriber? Log in .
Want all of The Times? Subscribe .
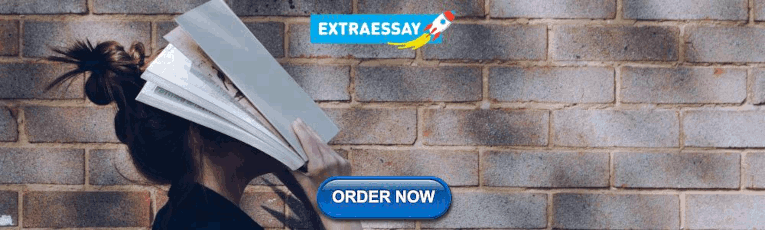
IMAGES
VIDEO
COMMENTS
Learn about the problem space hypothesis, a universal model of intelligence that systematically explores the alternatives to find a solution. Explore various search strategies, problems, and criteria for evaluating search algorithms.
Problem space is the set of all possible states, actions, and outcomes in a problem or task. Learn about its components (states, actions, constraints, variables, and outcomes) and characteristics (complexity, size, search space, interconnectedness, and dynamic nature).
on state-space problems. A state-space problem is said to consist of an initial state, a set of possible states, a set of admissible operators, and the specification of a desired state or. set of goal conditions. This is, in fact, quite similar to the task for mulation in Soar, discussed.
Suppes critiques Newell's use of problem spaces as a unified method of representation and his lack of detail on language and connectionism. He argues that problem spaces are too general and that language and connectionism are distinct aspects of human cognition.
Learn about problem space theory, which explains how people solve problems by searching in a space of possible states and actions. Find out how heuristics, such as difference reduction and means-ends analysis, guide the search and how the General Problem Solver simulates human problem solving.
The Problem Space Hypothesis is enunciated that the scope of problem spaces is to be extended to all symbolic cognitive activity. The chapter is devoted to explaining the nature of this hypothesis and describing some of its potential implications, with no attempt at a critical marshalling of the evidence pro and con. ...
Learn how to use problem space theory to solve problems by defining the problem and finding the solution. Explore the stages of the problem space process and its applications in qualitative research and business improvement.
The Problem Space Hypothesis contends that all goal-oriented behavior can be cast as search through a space of possible states (a problem space) while attempting to achieve a goal. At each step, a single operator is selected, and then applied to the agent's current state, which can lead to internal changes, such as retrieval of knowledge from ...
Newell, A., & Simon, H. A. (1972) Human Problem Solving. Prentice-Hall. This book introduces the idea of problem spaces and associated with it the Problem Space Hypothesis that postulates that all goal-oriented behavior can be represented as a search through a space of possible states while attempting to achieve a goal.
The Problem Space Hypothesis is enunciated that the scope of problem spaces is to be extended to all symbolic cognitive activity, and the origin of the numerous flow diagrams that serve as theories of how subjects behave in tasks in the psychological laboratory are explained. The notion of a problem space is well known in the area of problem solving research, both in cognitive psychology and ...
Duncker anticipated some concepts that later became the fundamentals of Newell and Simon's Problem Space Hypothesis (Newell and Simon 1972). Duncker also introduced a number of classical problems, like the radiation problem and the candle problem, into the literature. The radiation problem asked the following question:
Problem Space. Problem Space refers to the entire range of components that exist in the process of finding a solution to a problem. This range starts with "defining the problem," then proceeds to the intermediate stage of "identifying and testing possible solutions" and ends with the final stage of "choosing and implementing a solution".
At the heart of this investigation is the idea of a "problem space" from research on artificial intelligence and human cognition, which refers to how a problem solver constructs or evokes (from memory) a mental representation of a task and its possible solutions (Newell & Simon, 1972). Specifically, a problem space comprises knowledge ...
1 Answer. The Problem Space Theory in which people try and solve problems by looking at the problem space. The problem space consists of multiple states being: Actions that we take in order to move into another state are known as Operators. As humans, we take short-cuts known as heuristics in order to solve problems that we do not have any ...
This web page covers various theoretical models of problem solving, from traditional to computer simulation. It explains the problem space hypothesis, which is a part of Newell's approach, as a way of representing the problem and the solution in terms of states and operators.
instance space (i.e., find a path to the goal), but the nonspecific goal group instead appeared to test rules (i.e., search hypothesis space). Understanding Processes. Before a problem solver can attempt a problem, the problem instructions must be understood. The importance of understanding processes in
Define Problem space hypothesis-The problem space refers to the entire context and environment within which a problem exists.It encompasses all the factors and variables that influence the problem and its potential solutions. This includes internal and external elements such as stakeholders, constraints, resources, market dynamics, technological factors, social factors, and more.
The Referential Problem Space is a hypothetical influence of a particular set of environmental demand characteristics on the display of pointing by some animals, including humans. Here, I will argue that—contrary to some other contemporary theoretical accounts of pointing—the Referential Problem Space is amenable to scientific verification.
Both a problem and a hypothesis are therefore related to some external reality: the former is a question about some reality, the latter an expected or predicted knowledge claim about some reality. ... The initial validity of a hypothesis is determined by need analysis of the before-study knowledge space. A falsified hypothesis in hypothesis ...
We can calibrate the Bayesian probability to the frequentist p-value (Selke et al 2001; Goodman 2008; Held 2010; Greenland and Poole 2012). Methods to achieve this calibration vary, but the Fagan nomogram proposed by Held (2010) is a good tool for us as we go forward. We can calculate our NHST p-value, but then convert the p-value to a Bayes factor by looking at the nomogram.
Abstract. Although Soar is intended to cover the full range of weak problem-solving methods-often hypothesized in cognitive science as basic for all intelligent agents, earlier attempts to add means-ends analysis to Soar's repertoire of methods have not been particularly successful or convincing. Considering its psychological significance ...
Problems for society All this is ultimately encouraging conspiracy theories, which could undermine trust in democratic institutions. There have been humorous calls to storm Area 51 .
With grizzly bear movement data, we approach the problem as population-level hypothesis tests of the spatial consequences of encounters. We find support for the hypotheses that (1) close intraspecific encounters between bears are, under certain circumstances, associated with subsequent changes in overlap between range distributions and (2 ...
The Starliner episode is another black eye for Boeing, whose reputation has been badly damaged in recent years. Boeing's defense unit, home to its space programs, has suffered from cost overruns ...