- Business Essentials
- Leadership & Management
- Credential of Leadership, Impact, and Management in Business (CLIMB)
- Entrepreneurship & Innovation
- Digital Transformation
- Finance & Accounting
- Business in Society
- For Organizations
- Support Portal
- Media Coverage
- Founding Donors
- Leadership Team
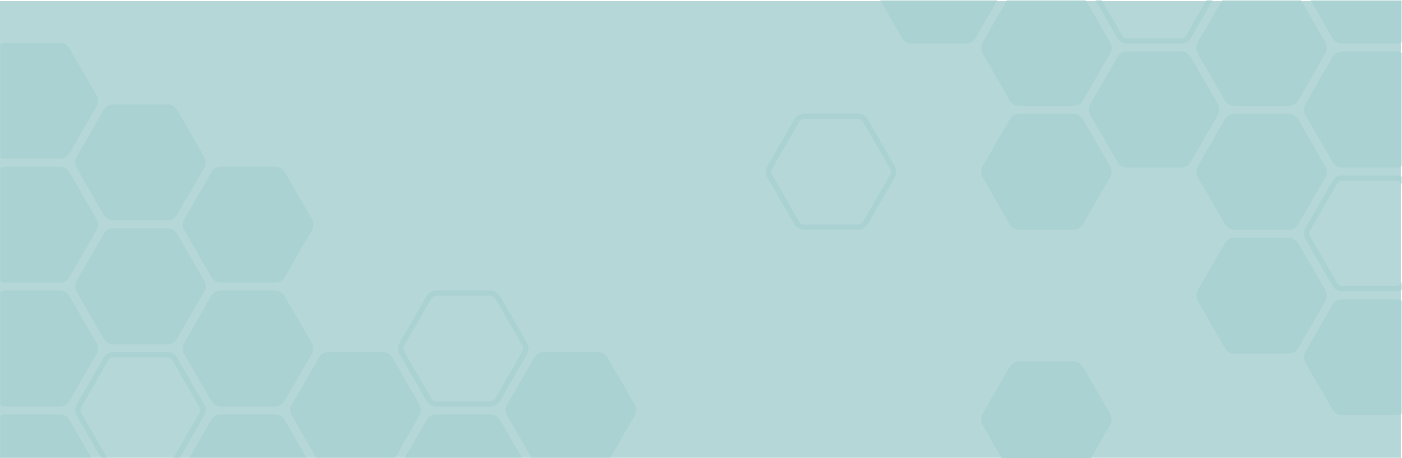
- Harvard Business School →
- HBS Online →
- Business Insights →
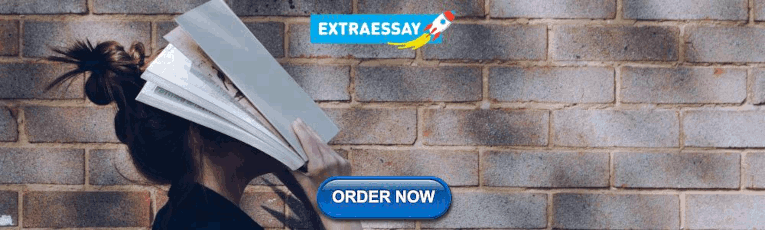
Business Insights
Harvard Business School Online's Business Insights Blog provides the career insights you need to achieve your goals and gain confidence in your business skills.
- Career Development
- Communication
- Decision-Making
- Earning Your MBA
- Negotiation
- News & Events
- Productivity
- Staff Spotlight
- Student Profiles
- Work-Life Balance
- AI Essentials for Business
- Alternative Investments
- Business Analytics
- Business Strategy
- Business and Climate Change
- Creating Brand Value
- Design Thinking and Innovation
- Digital Marketing Strategy
- Disruptive Strategy
- Economics for Managers
- Entrepreneurship Essentials
- Financial Accounting
- Global Business
- Launching Tech Ventures
- Leadership Principles
- Leadership, Ethics, and Corporate Accountability
- Leading Change and Organizational Renewal
- Leading with Finance
- Management Essentials
- Negotiation Mastery
- Organizational Leadership
- Power and Influence for Positive Impact
- Strategy Execution
- Sustainable Business Strategy
- Sustainable Investing
- Winning with Digital Platforms
5 Benefits of Learning Through the Case Study Method
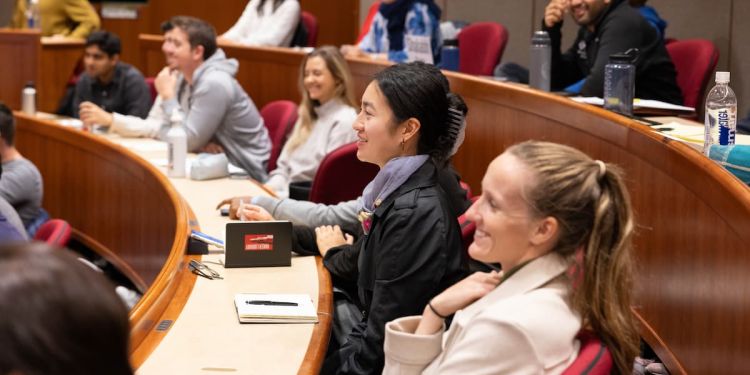
- 28 Nov 2023
While several factors make HBS Online unique —including a global Community and real-world outcomes —active learning through the case study method rises to the top.
In a 2023 City Square Associates survey, 74 percent of HBS Online learners who also took a course from another provider said HBS Online’s case method and real-world examples were better by comparison.
Here’s a primer on the case method, five benefits you could gain, and how to experience it for yourself.
Access your free e-book today.
What Is the Harvard Business School Case Study Method?
The case study method , or case method , is a learning technique in which you’re presented with a real-world business challenge and asked how you’d solve it. After working through it yourself and with peers, you’re told how the scenario played out.
HBS pioneered the case method in 1922. Shortly before, in 1921, the first case was written.
“How do you go into an ambiguous situation and get to the bottom of it?” says HBS Professor Jan Rivkin, former senior associate dean and chair of HBS's master of business administration (MBA) program, in a video about the case method . “That skill—the skill of figuring out a course of inquiry to choose a course of action—that skill is as relevant today as it was in 1921.”
Originally developed for the in-person MBA classroom, HBS Online adapted the case method into an engaging, interactive online learning experience in 2014.
In HBS Online courses , you learn about each case from the business professional who experienced it. After reviewing their videos, you’re prompted to take their perspective and explain how you’d handle their situation.
You then get to read peers’ responses, “star” them, and comment to further the discussion. Afterward, you learn how the professional handled it and their key takeaways.
Learn more about HBS Online's approach to the case method in the video below, and subscribe to our YouTube channel for more.
HBS Online’s adaptation of the case method incorporates the famed HBS “cold call,” in which you’re called on at random to make a decision without time to prepare.
“Learning came to life!” said Sheneka Balogun , chief administration officer and chief of staff at LeMoyne-Owen College, of her experience taking the Credential of Readiness (CORe) program . “The videos from the professors, the interactive cold calls where you were randomly selected to participate, and the case studies that enhanced and often captured the essence of objectives and learning goals were all embedded in each module. This made learning fun, engaging, and student-friendly.”
If you’re considering taking a course that leverages the case study method, here are five benefits you could experience.
5 Benefits of Learning Through Case Studies
1. take new perspectives.
The case method prompts you to consider a scenario from another person’s perspective. To work through the situation and come up with a solution, you must consider their circumstances, limitations, risk tolerance, stakeholders, resources, and potential consequences to assess how to respond.
Taking on new perspectives not only can help you navigate your own challenges but also others’. Putting yourself in someone else’s situation to understand their motivations and needs can go a long way when collaborating with stakeholders.
2. Hone Your Decision-Making Skills
Another skill you can build is the ability to make decisions effectively . The case study method forces you to use limited information to decide how to handle a problem—just like in the real world.
Throughout your career, you’ll need to make difficult decisions with incomplete or imperfect information—and sometimes, you won’t feel qualified to do so. Learning through the case method allows you to practice this skill in a low-stakes environment. When facing a real challenge, you’ll be better prepared to think quickly, collaborate with others, and present and defend your solution.
3. Become More Open-Minded
As you collaborate with peers on responses, it becomes clear that not everyone solves problems the same way. Exposing yourself to various approaches and perspectives can help you become a more open-minded professional.
When you’re part of a diverse group of learners from around the world, your experiences, cultures, and backgrounds contribute to a range of opinions on each case.
On the HBS Online course platform, you’re prompted to view and comment on others’ responses, and discussion is encouraged. This practice of considering others’ perspectives can make you more receptive in your career.
“You’d be surprised at how much you can learn from your peers,” said Ratnaditya Jonnalagadda , a software engineer who took CORe.
In addition to interacting with peers in the course platform, Jonnalagadda was part of the HBS Online Community , where he networked with other professionals and continued discussions sparked by course content.
“You get to understand your peers better, and students share examples of businesses implementing a concept from a module you just learned,” Jonnalagadda said. “It’s a very good way to cement the concepts in one's mind.”
4. Enhance Your Curiosity
One byproduct of taking on different perspectives is that it enables you to picture yourself in various roles, industries, and business functions.
“Each case offers an opportunity for students to see what resonates with them, what excites them, what bores them, which role they could imagine inhabiting in their careers,” says former HBS Dean Nitin Nohria in the Harvard Business Review . “Cases stimulate curiosity about the range of opportunities in the world and the many ways that students can make a difference as leaders.”
Through the case method, you can “try on” roles you may not have considered and feel more prepared to change or advance your career .
5. Build Your Self-Confidence
Finally, learning through the case study method can build your confidence. Each time you assume a business leader’s perspective, aim to solve a new challenge, and express and defend your opinions and decisions to peers, you prepare to do the same in your career.
According to a 2022 City Square Associates survey , 84 percent of HBS Online learners report feeling more confident making business decisions after taking a course.
“Self-confidence is difficult to teach or coach, but the case study method seems to instill it in people,” Nohria says in the Harvard Business Review . “There may well be other ways of learning these meta-skills, such as the repeated experience gained through practice or guidance from a gifted coach. However, under the direction of a masterful teacher, the case method can engage students and help them develop powerful meta-skills like no other form of teaching.”

How to Experience the Case Study Method
If the case method seems like a good fit for your learning style, experience it for yourself by taking an HBS Online course. Offerings span eight subject areas, including:
- Business essentials
- Leadership and management
- Entrepreneurship and innovation
- Digital transformation
- Finance and accounting
- Business in society
No matter which course or credential program you choose, you’ll examine case studies from real business professionals, work through their challenges alongside peers, and gain valuable insights to apply to your career.
Are you interested in discovering how HBS Online can help advance your career? Explore our course catalog and download our free guide —complete with interactive workbook sections—to determine if online learning is right for you and which course to take.

About the Author
- +1 0234 56 7890
- [email protected]
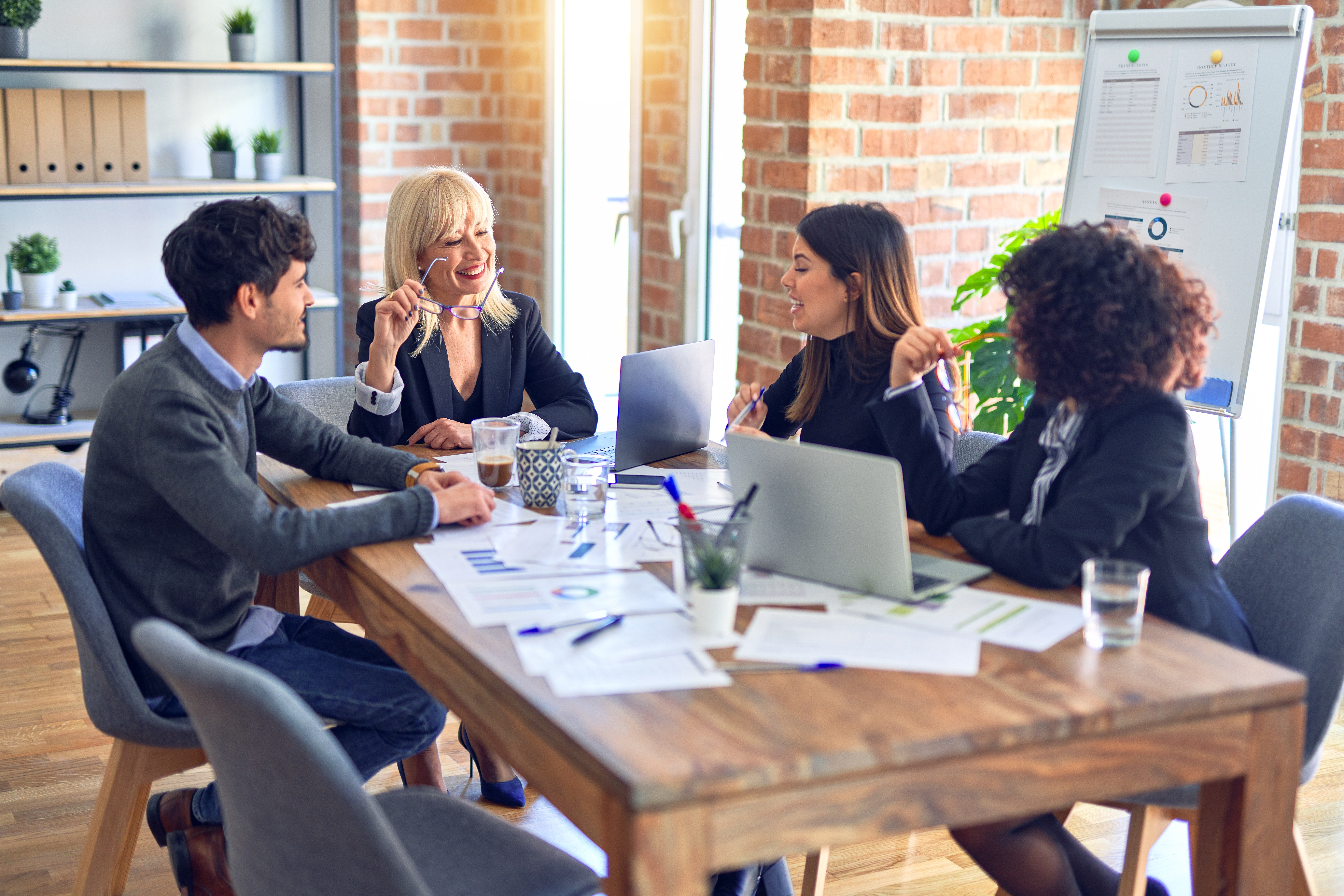
Effective Decision-Making: A Case Study
Effective decision-making:, leading an organization through timely and impactful action.
Senior leaders at a top New England insurance provider need to develop the skills and behaviors for better, faster decision-making. This virtually delivered program spans four half-day sessions and includes individual assignments, facilitator-led presentations, and simulation decision-making. Over the past two months, this program touched over 100 leaders, providing them with actionable models and frameworks to use back on the job.
For one of New England’s most iconic insurers, senior leaders are challenged to make timely, effective decisions. These leaders face decisions on three levels: ones they translate to their teams, ones they make themselves, and ones they influence. But in a quickly changing, highly regulated market, risk aversion can lead to slow and ineffective decisions. How can senior leaders practice in a safe environment the quick, yet informed, decision-making necessary for the job while simultaneously learning new models and techniques — and without the learning experience burdening their precious time?
The Effective Decision-Making program was artfully designed to immerse senior leaders in 16 hours of hands-on experience, including reflection and feedback activities, applicable exercises, supporting content, and participation in a business simulation to practice the core content of the program. Participants work together in small groups to complete these activities within a limited time frame, replicating the work environment in which these leaders must succeed. Continuous reflection and group discussion around results create real-time learning for leaders. Application exercises then facilitate the simulation experience and their work back on the job. The program employs a variety of learning methodologies, including:
- Individual assignments that incorporate content and frameworks designed to develop effective decision-making skills.
- Guided reflection activities to encourage self-awareness and commitments for action.
- Large group conversations — live discussions focused on peer input around key learning points.
- Small group activities, including virtual role plays designed to build critical interpersonal and leadership skills.
- A dynamic business simulation in which participants are charged with translating, making, and influencing difficult decisions.
- Facilitator-led discussions and presentations.
Learning Objectives
Participants develop and improve skills to:
- Cultivate a leadership mindset that empowers, inspires, and challenges others.
- Translate decisions for stronger team alignment and performance.
- Make better decisions under pressure.
- Influence individuals across the organization.
- Better understand how one’s leadership actions impact business results
Design Highlights
Program agenda.
As a result of the COVID-19 pandemic and the need for social distancing, this program was delivered virtually. However, this didn't preclude the need to give leaders an opportunity to connect with, and learn from, one another. In response to those needs, Insight Experience developed a fully remote, yet highly interactive, offering delivered over four half-day sessions.
Interactive Virtual Learning Format
Effective Decision-Making was designed to promote both individual and group activities and reflection. Participants access the program via a video-conferencing platform that allows them to work together both in large and small groups. Learning content and group discussions are done as one large group, enabling consistency in learning and opportunities to hear from all participants. The business simulation decision-making and reflection activities are conducted in small groups, allowing teams to develop deeper connections and conversations.
Simulation Overview
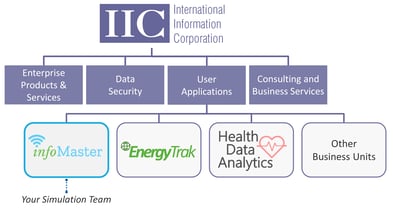
Participants assume the role of a General Manager for InfoMaster, a message management provider. Their leadership challenge as the GM is to translate the broader IIC organizational goals into strategy for their business, support that strategy though the development of organizational capabilities and product offerings, manage multiple divisions and stakeholders, and consider their contribution and responsibility to the broader organization of which they are a part.
Success in the simulation is based on how well teams:
- Understand and translate organizational strategy into goals and plans for their business unit.
- Align organizational initiatives and product development with broader strategies.
- Develop employee capabilities required to execute strategic goals.
- Hold stakeholders accountable to commitments and results.
- Communicate with stakeholders and involve others in plans and decision-making.
- Develop their network and their influence within IIC to help support initiatives for the organization
History and Results
Effective Decision-Making was developed in 2020 as an experience for senior-level leaders. After a successful pilot, the program was then rolled out to two more cohorts in 2021 and 2022. The senior-level leaders who participated in the program then requested we offer the same program to their direct reports. After some small adjustments to make the program more appropriate for director-level leaders, the program was launched in 2022 for approximately 100 directors.
Here is what some participants have said about this program:
- “ One of the better programs we've done here at [our organization]. Pace was very quick but content was excellent and approach made it fun .”
- “ Loved the content and the flow. Very nicely organized and managed. Thank you! ”
- “ Really enjoyed the collaborative nature of the simulation.”
- “ It was wonderful and I felt it is a great opportunity. Learnt and reinforced leadership training and what it would take to be successful.”
- “One of the best I've experienced — especially appreciated how the reality of [our organization] was incorporated and it was with similarly situated peers.”
- “This program was great! It gave good insight into how to enhance my skills as leader by adopting the leadership mindset.”
- “Loved the fast pace, having a sim group that had various backgrounds in the company and seeing the results of our decisions at the corporate level.”
- “Great program — I love the concepts highlighted during these sessions.”
Looking for results like these?
Leave comment, recent posts.
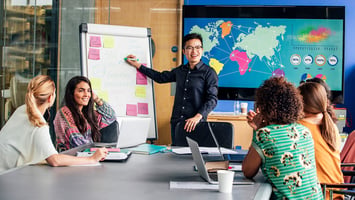
Leading Diffuse Teams: A Case Study
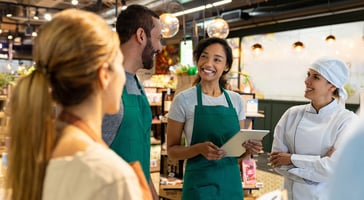
Emerging Retail Leaders: A Case Study
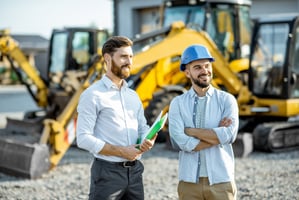
Improving Enterprise Business Acumen: A Case Study
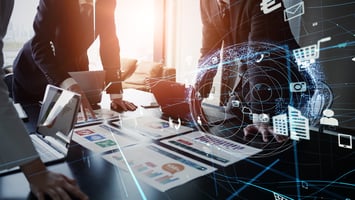
Embracing Uncertainty: A Case Study
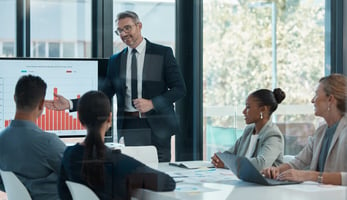
Taking Top Salespeople to Top Sales Leadership: A Case Study
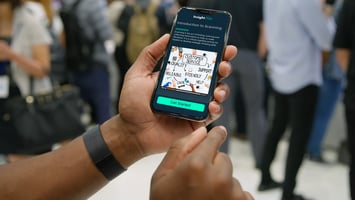
Practice Makes Permanent: Microlearning on the Go
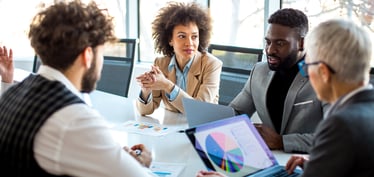
Transforming Leadership to Support Significant Change: A Case Study
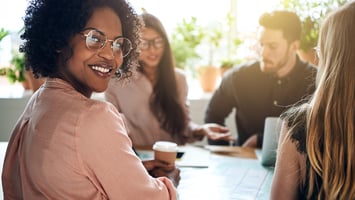
Leadership Foundations for Individual Contributors: A Case Study
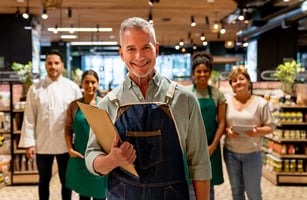
Connecting Category Management Decisions and Business Outcomes: A Case Study
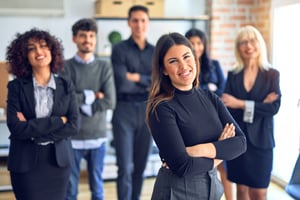
Accelerate the Implementation of Manager Expectations: A Case Study

Smart. Open. Grounded. Inventive. Read our Ideas Made to Matter.
Which program is right for you?
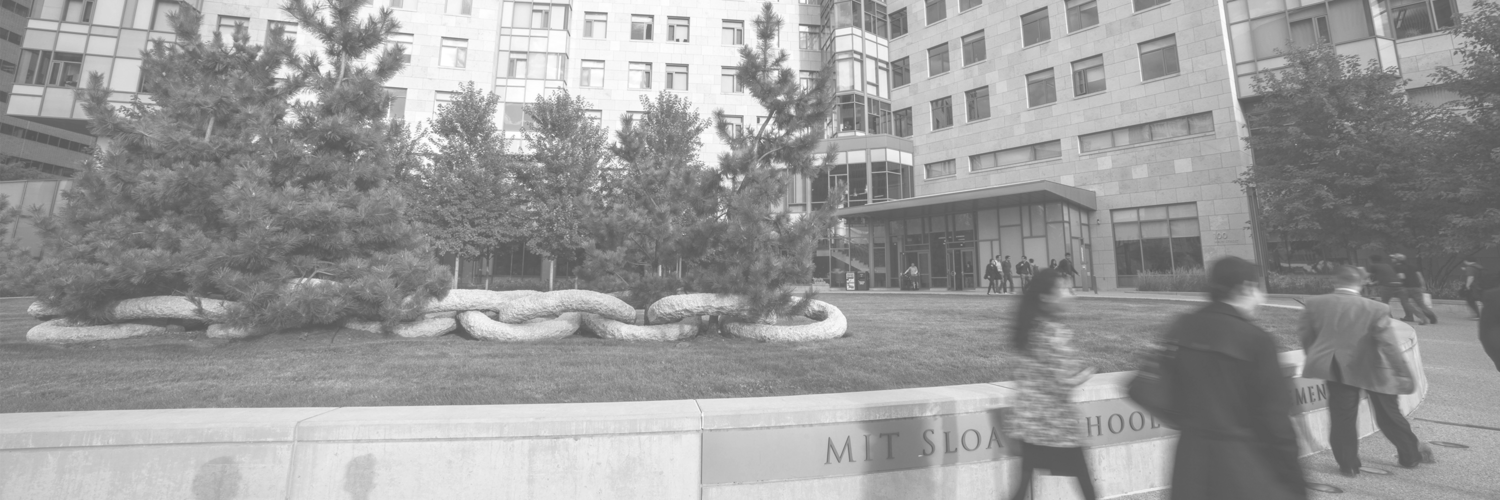
Through intellectual rigor and experiential learning, this full-time, two-year MBA program develops leaders who make a difference in the world.
Earn your MBA and SM in engineering with this transformative two-year program.
A rigorous, hands-on program that prepares adaptive problem solvers for premier finance careers.
A 12-month program focused on applying the tools of modern data science, optimization and machine learning to solve real-world business problems.
Combine an international MBA with a deep dive into management science. A special opportunity for partner and affiliate schools only.
A doctoral program that produces outstanding scholars who are leading in their fields of research.
Bring a business perspective to your technical and quantitative expertise with a bachelor’s degree in management, business analytics, or finance.
Apply now and work for two to five years. We'll save you a seat in our MBA class when you're ready to come back to campus for your degree.
Executive Programs
The 20-month program teaches the science of management to mid-career leaders who want to move from success to significance.
A full-time MBA program for mid-career leaders eager to dedicate one year of discovery for a lifetime of impact.
A joint program for mid-career professionals that integrates engineering and systems thinking. Earn your master’s degree in engineering and management.
Non-degree programs for senior executives and high-potential managers.
A non-degree, customizable program for mid-career professionals.
Teaching Resources Library
Case studies.
The teaching business case studies available here are narratives that facilitate class discussion about a particular business or management issue. Teaching cases are meant to spur debate among students rather than promote a particular point of view or steer students in a specific direction. Some of the case studies in this collection highlight the decision-making process in a business or management setting. Other cases are descriptive or demonstrative in nature, showcasing something that has happened or is happening in a particular business or management environment. Whether decision-based or demonstrative, case studies give students the chance to be in the shoes of a protagonist. With the help of context and detailed data, students can analyze what they would and would not do in a particular situation, why, and how.
Case Studies By Category
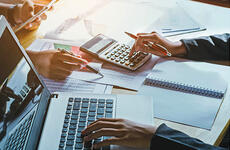
- Columbia University in the City of New York
- Office of Teaching, Learning, and Innovation
- University Policies
- Columbia Online
- Academic Calendar
- Resources and Technology
- Resources and Guides
Case Method Teaching and Learning
What is the case method? How can the case method be used to engage learners? What are some strategies for getting started? This guide helps instructors answer these questions by providing an overview of the case method while highlighting learner-centered and digitally-enhanced approaches to teaching with the case method. The guide also offers tips to instructors as they get started with the case method and additional references and resources.
On this page:
What is case method teaching.
- Case Method at Columbia
Why use the Case Method?
Case method teaching approaches, how do i get started.
- Additional Resources
The CTL is here to help!
For support with implementing a case method approach in your course, email [email protected] to schedule your 1-1 consultation .
Cite this resource: Columbia Center for Teaching and Learning (2019). Case Method Teaching and Learning. Columbia University. Retrieved from [today’s date] from https://ctl.columbia.edu/resources-and-technology/resources/case-method/
Case method 1 teaching is an active form of instruction that focuses on a case and involves students learning by doing 2 3 . Cases are real or invented stories 4 that include “an educational message” or recount events, problems, dilemmas, theoretical or conceptual issue that requires analysis and/or decision-making.
Case-based teaching simulates real world situations and asks students to actively grapple with complex problems 5 6 This method of instruction is used across disciplines to promote learning, and is common in law, business, medicine, among other fields. See Table 1 below for a few types of cases and the learning they promote.
Table 1: Types of cases and the learning they promote.
Type of Case | Description | Promoted Learning |
---|---|---|
Directed case | Presents a scenario that is followed by discussion using a set of “directed” / close-ended questions that can be answered from course material. | Understanding of fundamental concepts, principles, and facts |
Dilemma or decision case | Presents an individual, institution, or community faced with a problem that must be solved. Students may be presented with actual historical outcomes after they work through the case. | Problem solving and decision-making skills |
Interrupted case | Presents a problem for students to solve in a progressive disclosure format. Students are given the case in parts that they work on and make decisions about before moving on to the next part. | Problem solving skills |
Analysis or issue case | Focuses on answering questions and analyzing the situation presented. This can include “retrospective” cases that tell a story and its outcomes and have students analyze what happened and why alternative solutions were not taken. | Analysis skills |
For a more complete list, see Case Types & Teaching Methods: A Classification Scheme from the National Center for Case Study Teaching in Science.
Back to Top
Case Method Teaching and Learning at Columbia
The case method is actively used in classrooms across Columbia, at the Morningside campus in the School of International and Public Affairs (SIPA), the School of Business, Arts and Sciences, among others, and at Columbia University Irving Medical campus.
Faculty Spotlight:
Professor Mary Ann Price on Using Case Study Method to Place Pre-Med Students in Real-Life Scenarios
Read more
Professor De Pinho on Using the Case Method in the Mailman Core
Case method teaching has been found to improve student learning, to increase students’ perception of learning gains, and to meet learning objectives 8 9 . Faculty have noted the instructional benefits of cases including greater student engagement in their learning 10 , deeper student understanding of concepts, stronger critical thinking skills, and an ability to make connections across content areas and view an issue from multiple perspectives 11 .
Through case-based learning, students are the ones asking questions about the case, doing the problem-solving, interacting with and learning from their peers, “unpacking” the case, analyzing the case, and summarizing the case. They learn how to work with limited information and ambiguity, think in professional or disciplinary ways, and ask themselves “what would I do if I were in this specific situation?”
The case method bridges theory to practice, and promotes the development of skills including: communication, active listening, critical thinking, decision-making, and metacognitive skills 12 , as students apply course content knowledge, reflect on what they know and their approach to analyzing, and make sense of a case.
Though the case method has historical roots as an instructor-centered approach that uses the Socratic dialogue and cold-calling, it is possible to take a more learner-centered approach in which students take on roles and tasks traditionally left to the instructor.
Cases are often used as “vehicles for classroom discussion” 13 . Students should be encouraged to take ownership of their learning from a case. Discussion-based approaches engage students in thinking and communicating about a case. Instructors can set up a case activity in which students are the ones doing the work of “asking questions, summarizing content, generating hypotheses, proposing theories, or offering critical analyses” 14 .
The role of the instructor is to share a case or ask students to share or create a case to use in class, set expectations, provide instructions, and assign students roles in the discussion. Student roles in a case discussion can include:
- discussion “starters” get the conversation started with a question or posing the questions that their peers came up with;
- facilitators listen actively, validate the contributions of peers, ask follow-up questions, draw connections, refocus the conversation as needed;
- recorders take-notes of the main points of the discussion, record on the board, upload to CourseWorks, or type and project on the screen; and
- discussion “wrappers” lead a summary of the main points of the discussion.
Prior to the case discussion, instructors can model case analysis and the types of questions students should ask, co-create discussion guidelines with students, and ask for students to submit discussion questions. During the discussion, the instructor can keep time, intervene as necessary (however the students should be doing the talking), and pause the discussion for a debrief and to ask students to reflect on what and how they learned from the case activity.
Note: case discussions can be enhanced using technology. Live discussions can occur via video-conferencing (e.g., using Zoom ) or asynchronous discussions can occur using the Discussions tool in CourseWorks (Canvas) .
Table 2 includes a few interactive case method approaches. Regardless of the approach selected, it is important to create a learning environment in which students feel comfortable participating in a case activity and learning from one another. See below for tips on supporting student in how to learn from a case in the “getting started” section and how to create a supportive learning environment in the Guide for Inclusive Teaching at Columbia .
Table 2. Strategies for Engaging Students in Case-Based Learning
Strategy | Role of the Instructor | |||
---|---|---|---|---|
Debate or Trial | Develop critical thinking skills and encourage students to challenge their existing assumptions. | Structure (with guidelines) and facilitate a debate between two diametrically opposed views. Keep time and ask students to reflect on their experience. | Prepare to argue either side. Work in teams to develop and present arguments, and debrief the debate. | Work in teams and prepare an argument for conflicting sides of an issue. |
Role play or Public Hearing | Understand diverse points of view, promote creative thinking, and develop empathy. | Structure the role-play and facilitate the debrief. At the close of the activity, ask students to reflect on what they learned. | Play a role found in a case, understand the points of view of stakeholders involved. | Describe the points of view of every stakeholder involved. |
Jigsaw | Promote peer-to-peer learning, and get students to own their learning. | Form student groups, assign each group a piece of the case to study. Form new groups with an “expert” for each previous group. Facilitate a debrief. | Be responsible for learning and then teaching case material to peers. Develop expertise for part of the problem. | Facilitate case method materials for their peers. |
“Clicker case” / (ARS) | Gauge your students’ learning; get all students to respond to questions, and launch or enhance a case discussion. | Instructor presents a case in stages, punctuated with questions in Poll Everywhere that students respond to using a mobile device. | Respond to questions using a mobile device. Reflect on why they responded the way they did and discuss with peers seated next to them. | Articulate their understanding of a case components. |
Approaches to case teaching should be informed by course learning objectives, and can be adapted for small, large, hybrid, and online classes. Instructional technology can be used in various ways to deliver, facilitate, and assess the case method. For instance, an online module can be created in CourseWorks (Canvas) to structure the delivery of the case, allow students to work at their own pace, engage all learners, even those reluctant to speak up in class, and assess understanding of a case and student learning. Modules can include text, embedded media (e.g., using Panopto or Mediathread ) curated by the instructor, online discussion, and assessments. Students can be asked to read a case and/or watch a short video, respond to quiz questions and receive immediate feedback, post questions to a discussion, and share resources.
For more information about options for incorporating educational technology to your course, please contact your Learning Designer .
To ensure that students are learning from the case approach, ask them to pause and reflect on what and how they learned from the case. Time to reflect builds your students’ metacognition, and when these reflections are collected they provides you with insights about the effectiveness of your approach in promoting student learning.
Well designed case-based learning experiences: 1) motivate student involvement, 2) have students doing the work, 3) help students develop knowledge and skills, and 4) have students learning from each other.
Designing a case-based learning experience should center around the learning objectives for a course. The following points focus on intentional design.
Identify learning objectives, determine scope, and anticipate challenges.
- Why use the case method in your course? How will it promote student learning differently than other approaches?
- What are the learning objectives that need to be met by the case method? What knowledge should students apply and skills should they practice?
- What is the scope of the case? (a brief activity in a single class session to a semester-long case-based course; if new to case method, start small with a single case).
- What challenges do you anticipate (e.g., student preparation and prior experiences with case learning, discomfort with discussion, peer-to-peer learning, managing discussion) and how will you plan for these in your design?
- If you are asking students to use transferable skills for the case method (e.g., teamwork, digital literacy) make them explicit.
Determine how you will know if the learning objectives were met and develop a plan for evaluating the effectiveness of the case method to inform future case teaching.
- What assessments and criteria will you use to evaluate student work or participation in case discussion?
- How will you evaluate the effectiveness of the case method? What feedback will you collect from students?
- How might you leverage technology for assessment purposes? For example, could you quiz students about the case online before class, accept assignment submissions online, use audience response systems (e.g., PollEverywhere) for formative assessment during class?
Select an existing case, create your own, or encourage students to bring course-relevant cases, and prepare for its delivery
- Where will the case method fit into the course learning sequence?
- Is the case at the appropriate level of complexity? Is it inclusive, culturally relevant, and relatable to students?
- What materials and preparation will be needed to present the case to students? (e.g., readings, audiovisual materials, set up a module in CourseWorks).
Plan for the case discussion and an active role for students
- What will your role be in facilitating case-based learning? How will you model case analysis for your students? (e.g., present a short case and demo your approach and the process of case learning) (Davis, 2009).
- What discussion guidelines will you use that include your students’ input?
- How will you encourage students to ask and answer questions, summarize their work, take notes, and debrief the case?
- If students will be working in groups, how will groups form? What size will the groups be? What instructions will they be given? How will you ensure that everyone participates? What will they need to submit? Can technology be leveraged for any of these areas?
- Have you considered students of varied cognitive and physical abilities and how they might participate in the activities/discussions, including those that involve technology?
Student preparation and expectations
- How will you communicate about the case method approach to your students? When will you articulate the purpose of case-based learning and expectations of student engagement? What information about case-based learning and expectations will be included in the syllabus?
- What preparation and/or assignment(s) will students complete in order to learn from the case? (e.g., read the case prior to class, watch a case video prior to class, post to a CourseWorks discussion, submit a brief memo, complete a short writing assignment to check students’ understanding of a case, take on a specific role, prepare to present a critique during in-class discussion).
Andersen, E. and Schiano, B. (2014). Teaching with Cases: A Practical Guide . Harvard Business Press.
Bonney, K. M. (2015). Case Study Teaching Method Improves Student Performance and Perceptions of Learning Gains†. Journal of Microbiology & Biology Education , 16 (1), 21–28. https://doi.org/10.1128/jmbe.v16i1.846
Davis, B.G. (2009). Chapter 24: Case Studies. In Tools for Teaching. Second Edition. Jossey-Bass.
Garvin, D.A. (2003). Making the Case: Professional Education for the world of practice. Harvard Magazine. September-October 2003, Volume 106, Number 1, 56-107.
Golich, V.L. (2000). The ABCs of Case Teaching. International Studies Perspectives. 1, 11-29.
Golich, V.L.; Boyer, M; Franko, P.; and Lamy, S. (2000). The ABCs of Case Teaching. Pew Case Studies in International Affairs. Institute for the Study of Diplomacy.
Heath, J. (2015). Teaching & Writing Cases: A Practical Guide. The Case Center, UK.
Herreid, C.F. (2011). Case Study Teaching. New Directions for Teaching and Learning. No. 128, Winder 2011, 31 – 40.
Herreid, C.F. (2007). Start with a Story: The Case Study Method of Teaching College Science . National Science Teachers Association. Available as an ebook through Columbia Libraries.
Herreid, C.F. (2006). “Clicker” Cases: Introducing Case Study Teaching Into Large Classrooms. Journal of College Science Teaching. Oct 2006, 36(2). https://search.proquest.com/docview/200323718?pq-origsite=gscholar
Krain, M. (2016). Putting the Learning in Case Learning? The Effects of Case-Based Approaches on Student Knowledge, Attitudes, and Engagement. Journal on Excellence in College Teaching. 27(2), 131-153.
Lundberg, K.O. (Ed.). (2011). Our Digital Future: Boardrooms and Newsrooms. Knight Case Studies Initiative.
Popil, I. (2011). Promotion of critical thinking by using case studies as teaching method. Nurse Education Today, 31(2), 204–207. https://doi.org/10.1016/j.nedt.2010.06.002
Schiano, B. and Andersen, E. (2017). Teaching with Cases Online . Harvard Business Publishing.
Thistlethwaite, JE; Davies, D.; Ekeocha, S.; Kidd, J.M.; MacDougall, C.; Matthews, P.; Purkis, J.; Clay D. (2012). The effectiveness of case-based learning in health professional education: A BEME systematic review . Medical Teacher. 2012; 34(6): e421-44.
Yadav, A.; Lundeberg, M.; DeSchryver, M.; Dirkin, K.; Schiller, N.A.; Maier, K. and Herreid, C.F. (2007). Teaching Science with Case Studies: A National Survey of Faculty Perceptions of the Benefits and Challenges of Using Cases. Journal of College Science Teaching; Sept/Oct 2007; 37(1).
Weimer, M. (2013). Learner-Centered Teaching: Five Key Changes to Practice. Second Edition. Jossey-Bass.
Additional resources
Teaching with Cases , Harvard Kennedy School of Government.
Features “what is a teaching case?” video that defines a teaching case, and provides documents to help students prepare for case learning, Common case teaching challenges and solutions, tips for teaching with cases.
Promoting excellence and innovation in case method teaching: Teaching by the Case Method , Christensen Center for Teaching & Learning. Harvard Business School.
National Center for Case Study Teaching in Science . University of Buffalo.
A collection of peer-reviewed STEM cases to teach scientific concepts and content, promote process skills and critical thinking. The Center welcomes case submissions. Case classification scheme of case types and teaching methods:
- Different types of cases: analysis case, dilemma/decision case, directed case, interrupted case, clicker case, a flipped case, a laboratory case.
- Different types of teaching methods: problem-based learning, discussion, debate, intimate debate, public hearing, trial, jigsaw, role-play.
Columbia Resources
Resources available to support your use of case method: The University hosts a number of case collections including: the Case Consortium (a collection of free cases in the fields of journalism, public policy, public health, and other disciplines that include teaching and learning resources; SIPA’s Picker Case Collection (audiovisual case studies on public sector innovation, filmed around the world and involving SIPA student teams in producing the cases); and Columbia Business School CaseWorks , which develops teaching cases and materials for use in Columbia Business School classrooms.
Center for Teaching and Learning
The Center for Teaching and Learning (CTL) offers a variety of programs and services for instructors at Columbia. The CTL can provide customized support as you plan to use the case method approach through implementation. Schedule a one-on-one consultation.
Office of the Provost
The Hybrid Learning Course Redesign grant program from the Office of the Provost provides support for faculty who are developing innovative and technology-enhanced pedagogy and learning strategies in the classroom. In addition to funding, faculty awardees receive support from CTL staff as they redesign, deliver, and evaluate their hybrid courses.
The Start Small! Mini-Grant provides support to faculty who are interested in experimenting with one new pedagogical strategy or tool. Faculty awardees receive funds and CTL support for a one-semester period.
Explore our teaching resources.
- Blended Learning
- Contemplative Pedagogy
- Inclusive Teaching Guide
- FAQ for Teaching Assistants
- Metacognition
CTL resources and technology for you.
- Overview of all CTL Resources and Technology
- The origins of this method can be traced to Harvard University where in 1870 the Law School began using cases to teach students how to think like lawyers using real court decisions. This was followed by the Business School in 1920 (Garvin, 2003). These professional schools recognized that lecture mode of instruction was insufficient to teach critical professional skills, and that active learning would better prepare learners for their professional lives. ↩
- Golich, V.L. (2000). The ABCs of Case Teaching. International Studies Perspectives. 1, 11-29. ↩
- Herreid, C.F. (2007). Start with a Story: The Case Study Method of Teaching College Science . National Science Teachers Association. Available as an ebook through Columbia Libraries. ↩
- Davis, B.G. (2009). Chapter 24: Case Studies. In Tools for Teaching. Second Edition. Jossey-Bass. ↩
- Andersen, E. and Schiano, B. (2014). Teaching with Cases: A Practical Guide . Harvard Business Press. ↩
- Lundberg, K.O. (Ed.). (2011). Our Digital Future: Boardrooms and Newsrooms. Knight Case Studies Initiative. ↩
- Heath, J. (2015). Teaching & Writing Cases: A Practical Guide. The Case Center, UK. ↩
- Bonney, K. M. (2015). Case Study Teaching Method Improves Student Performance and Perceptions of Learning Gains†. Journal of Microbiology & Biology Education , 16 (1), 21–28. https://doi.org/10.1128/jmbe.v16i1.846 ↩
- Krain, M. (2016). Putting the Learning in Case Learning? The Effects of Case-Based Approaches on Student Knowledge, Attitudes, and Engagement. Journal on Excellence in College Teaching. 27(2), 131-153. ↩
- Thistlethwaite, JE; Davies, D.; Ekeocha, S.; Kidd, J.M.; MacDougall, C.; Matthews, P.; Purkis, J.; Clay D. (2012). The effectiveness of case-based learning in health professional education: A BEME systematic review . Medical Teacher. 2012; 34(6): e421-44. ↩
- Yadav, A.; Lundeberg, M.; DeSchryver, M.; Dirkin, K.; Schiller, N.A.; Maier, K. and Herreid, C.F. (2007). Teaching Science with Case Studies: A National Survey of Faculty Perceptions of the Benefits and Challenges of Using Cases. Journal of College Science Teaching; Sept/Oct 2007; 37(1). ↩
- Popil, I. (2011). Promotion of critical thinking by using case studies as teaching method. Nurse Education Today, 31(2), 204–207. https://doi.org/10.1016/j.nedt.2010.06.002 ↩
- Weimer, M. (2013). Learner-Centered Teaching: Five Key Changes to Practice. Second Edition. Jossey-Bass. ↩
- Herreid, C.F. (2006). “Clicker” Cases: Introducing Case Study Teaching Into Large Classrooms. Journal of College Science Teaching. Oct 2006, 36(2). https://search.proquest.com/docview/200323718?pq-origsite=gscholar ↩
This website uses cookies to identify users, improve the user experience and requires cookies to work. By continuing to use this website, you consent to Columbia University's use of cookies and similar technologies, in accordance with the Columbia University Website Cookie Notice .
- Our Mission
Making Learning Relevant With Case Studies
The open-ended problems presented in case studies give students work that feels connected to their lives.
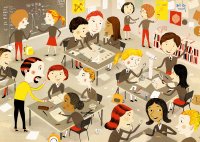
To prepare students for jobs that haven’t been created yet, we need to teach them how to be great problem solvers so that they’ll be ready for anything. One way to do this is by teaching content and skills using real-world case studies, a learning model that’s focused on reflection during the problem-solving process. It’s similar to project-based learning, but PBL is more focused on students creating a product.
Case studies have been used for years by businesses, law and medical schools, physicians on rounds, and artists critiquing work. Like other forms of problem-based learning, case studies can be accessible for every age group, both in one subject and in interdisciplinary work.
You can get started with case studies by tackling relatable questions like these with your students:
- How can we limit food waste in the cafeteria?
- How can we get our school to recycle and compost waste? (Or, if you want to be more complex, how can our school reduce its carbon footprint?)
- How can we improve school attendance?
- How can we reduce the number of people who get sick at school during cold and flu season?
Addressing questions like these leads students to identify topics they need to learn more about. In researching the first question, for example, students may see that they need to research food chains and nutrition. Students often ask, reasonably, why they need to learn something, or when they’ll use their knowledge in the future. Learning is most successful for students when the content and skills they’re studying are relevant, and case studies offer one way to create that sense of relevance.
Teaching With Case Studies
Ultimately, a case study is simply an interesting problem with many correct answers. What does case study work look like in classrooms? Teachers generally start by having students read the case or watch a video that summarizes the case. Students then work in small groups or individually to solve the case study. Teachers set milestones defining what students should accomplish to help them manage their time.
During the case study learning process, student assessment of learning should be focused on reflection. Arthur L. Costa and Bena Kallick’s Learning and Leading With Habits of Mind gives several examples of what this reflection can look like in a classroom:
Journaling: At the end of each work period, have students write an entry summarizing what they worked on, what worked well, what didn’t, and why. Sentence starters and clear rubrics or guidelines will help students be successful. At the end of a case study project, as Costa and Kallick write, it’s helpful to have students “select significant learnings, envision how they could apply these learnings to future situations, and commit to an action plan to consciously modify their behaviors.”
Interviews: While working on a case study, students can interview each other about their progress and learning. Teachers can interview students individually or in small groups to assess their learning process and their progress.
Student discussion: Discussions can be unstructured—students can talk about what they worked on that day in a think-pair-share or as a full class—or structured, using Socratic seminars or fishbowl discussions. If your class is tackling a case study in small groups, create a second set of small groups with a representative from each of the case study groups so that the groups can share their learning.
4 Tips for Setting Up a Case Study
1. Identify a problem to investigate: This should be something accessible and relevant to students’ lives. The problem should also be challenging and complex enough to yield multiple solutions with many layers.
2. Give context: Think of this step as a movie preview or book summary. Hook the learners to help them understand just enough about the problem to want to learn more.
3. Have a clear rubric: Giving structure to your definition of quality group work and products will lead to stronger end products. You may be able to have your learners help build these definitions.
4. Provide structures for presenting solutions: The amount of scaffolding you build in depends on your students’ skill level and development. A case study product can be something like several pieces of evidence of students collaborating to solve the case study, and ultimately presenting their solution with a detailed slide deck or an essay—you can scaffold this by providing specified headings for the sections of the essay.
Problem-Based Teaching Resources
There are many high-quality, peer-reviewed resources that are open source and easily accessible online.
- The National Center for Case Study Teaching in Science at the University at Buffalo built an online collection of more than 800 cases that cover topics ranging from biochemistry to economics. There are resources for middle and high school students.
- Models of Excellence , a project maintained by EL Education and the Harvard Graduate School of Education, has examples of great problem- and project-based tasks—and corresponding exemplary student work—for grades pre-K to 12.
- The Interdisciplinary Journal of Problem-Based Learning at Purdue University is an open-source journal that publishes examples of problem-based learning in K–12 and post-secondary classrooms.
- The Tech Edvocate has a list of websites and tools related to problem-based learning.
In their book Problems as Possibilities , Linda Torp and Sara Sage write that at the elementary school level, students particularly appreciate how they feel that they are taken seriously when solving case studies. At the middle school level, “researchers stress the importance of relating middle school curriculum to issues of student concern and interest.” And high schoolers, they write, find the case study method “beneficial in preparing them for their future.”
What is the Case Study Method?
Simply put, the case method is a discussion of real-life situations that business executives have faced.
On average, you'll attend three to four different classes a day, for a total of about six hours of class time (schedules vary). To prepare, you'll work through problems with your peers.
How the Case Method Creates Value
Often, executives are surprised to discover that the objective of the case study is not to reach consensus, but to understand how different people use the same information to arrive at diverse conclusions. When you begin to understand the context, you can appreciate the reasons why those decisions were made. You can prepare for case discussions in several ways.
Case Discussion Preparation Details
In self-reflection.
The time you spend here is deeply introspective. You're not only working with case materials and assignments, but also taking on the role of the case protagonist—the person who's supposed to make those tough decisions. How would you react in those situations? We put people in a variety of contexts, and they start by addressing that specific problem.
In a small group setting
The discussion group is a critical component of the HBS experience. You're working in close quarters with a group of seven or eight very accomplished peers in diverse functions, industries, and geographies. Because they bring unique experience to play you begin to see that there are many different ways to wrestle with a problem—and that’s very enriching.
In the classroom
The faculty guides you in examining and resolving the issues—but the beauty here is that they don't provide you with the answers. You're interacting in the classroom with other executives—debating the issue, presenting new viewpoints, countering positions, and building on one another's ideas. And that leads to the next stage of learning.
Beyond the classroom
Once you leave the classroom, the learning continues and amplifies as you get to know people in different settings—over meals, at social gatherings, in the fitness center, or as you are walking to class. You begin to distill the takeaways that you want to bring back and apply in your organization to ensure that the decisions you make will create more value for your firm.
How Cases Unfold In the Classroom
Pioneered by HBS faculty, the case method puts you in the role of the chief decision maker as you explore the challenges facing leading companies across the globe. Learning to think fast on your feet with limited information sharpens your analytical skills and empowers you to make critical decisions in real time.
To get the most out of each case, it's important to read and reflect, and then meet with your discussion group to share your insights. You and your peers will explore the underlying issues, compare alternatives, and suggest various ways of resolving the problem.
How to Prepare for Case Discussions
There's more than one way to prepare for a case discussion, but these general guidelines can help you develop a method that works for you.
Preparation Guidelines
Read the professor's assignment or discussion questions.
The assignment and discussion questions help you focus on the key aspects of the case. Ask yourself: What are the most important issues being raised?
Read the first few paragraphs and then skim the case
Each case begins with a text description followed by exhibits. Ask yourself: What is the case generally about, and what information do I need to analyze?
Reread the case, underline text, and make margin notes
Put yourself in the shoes of the case protagonist, and own that person's problems. Ask yourself: What basic problem is this executive trying to resolve?
Note the key problems on a pad of paper and go through the case again
Sort out relevant considerations and do the quantitative or qualitative analysis. Ask yourself: What recommendations should I make based on my case data analysis?
Case Study Best Practices
The key to being an active listener and participant in case discussions—and to getting the most out of the learning experience—is thorough individual preparation.
We've set aside formal time for you to discuss the case with your group. These sessions will help you to become more confident about sharing your views in the classroom discussion.
Participate
Actively express your views and challenge others. Don't be afraid to share related "war stories" that will heighten the relevance and enrich the discussion.
If the content doesn't seem to relate to your business, don't tune out. You can learn a lot about marketing insurance from a case on marketing razor blades!
Actively apply what you're learning to your own specific management situations, both past and future. This will magnify the relevance to your business.
People with diverse backgrounds, experiences, skills, and styles will take away different things. Be sure to note what resonates with you, not your peers.
Being exposed to so many different approaches to a given situation will put you in a better position to enhance your management style.
Frequently Asked Questions
What can i expect on the first day, what happens in class if nobody talks, does everyone take part in "role-playing".
Using Case Studies to Teach
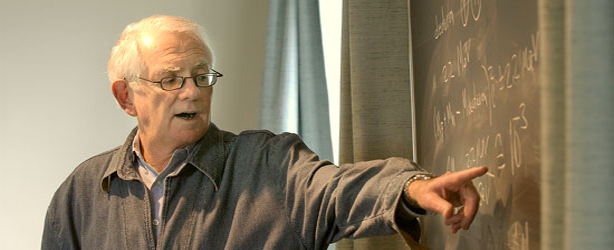
Why Use Cases?
Many students are more inductive than deductive reasoners, which means that they learn better from examples than from logical development starting with basic principles. The use of case studies can therefore be a very effective classroom technique.
Case studies are have long been used in business schools, law schools, medical schools and the social sciences, but they can be used in any discipline when instructors want students to explore how what they have learned applies to real world situations. Cases come in many formats, from a simple “What would you do in this situation?” question to a detailed description of a situation with accompanying data to analyze. Whether to use a simple scenario-type case or a complex detailed one depends on your course objectives.
Most case assignments require students to answer an open-ended question or develop a solution to an open-ended problem with multiple potential solutions. Requirements can range from a one-paragraph answer to a fully developed group action plan, proposal or decision.
Common Case Elements
Most “full-blown” cases have these common elements:
- A decision-maker who is grappling with some question or problem that needs to be solved.
- A description of the problem’s context (a law, an industry, a family).
- Supporting data, which can range from data tables to links to URLs, quoted statements or testimony, supporting documents, images, video, or audio.
Case assignments can be done individually or in teams so that the students can brainstorm solutions and share the work load.
The following discussion of this topic incorporates material presented by Robb Dixon of the School of Management and Rob Schadt of the School of Public Health at CEIT workshops. Professor Dixon also provided some written comments that the discussion incorporates.
Advantages to the use of case studies in class
A major advantage of teaching with case studies is that the students are actively engaged in figuring out the principles by abstracting from the examples. This develops their skills in:
- Problem solving
- Analytical tools, quantitative and/or qualitative, depending on the case
- Decision making in complex situations
- Coping with ambiguities
Guidelines for using case studies in class
In the most straightforward application, the presentation of the case study establishes a framework for analysis. It is helpful if the statement of the case provides enough information for the students to figure out solutions and then to identify how to apply those solutions in other similar situations. Instructors may choose to use several cases so that students can identify both the similarities and differences among the cases.
Depending on the course objectives, the instructor may encourage students to follow a systematic approach to their analysis. For example:
- What is the issue?
- What is the goal of the analysis?
- What is the context of the problem?
- What key facts should be considered?
- What alternatives are available to the decision-maker?
- What would you recommend — and why?
An innovative approach to case analysis might be to have students role-play the part of the people involved in the case. This not only actively engages students, but forces them to really understand the perspectives of the case characters. Videos or even field trips showing the venue in which the case is situated can help students to visualize the situation that they need to analyze.
Accompanying Readings
Case studies can be especially effective if they are paired with a reading assignment that introduces or explains a concept or analytical method that applies to the case. The amount of emphasis placed on the use of the reading during the case discussion depends on the complexity of the concept or method. If it is straightforward, the focus of the discussion can be placed on the use of the analytical results. If the method is more complex, the instructor may need to walk students through its application and the interpretation of the results.
Leading the Case Discussion and Evaluating Performance
Decision cases are more interesting than descriptive ones. In order to start the discussion in class, the instructor can start with an easy, noncontroversial question that all the students should be able to answer readily. However, some of the best case discussions start by forcing the students to take a stand. Some instructors will ask a student to do a formal “open” of the case, outlining his or her entire analysis. Others may choose to guide discussion with questions that move students from problem identification to solutions. A skilled instructor steers questions and discussion to keep the class on track and moving at a reasonable pace.
In order to motivate the students to complete the assignment before class as well as to stimulate attentiveness during the class, the instructor should grade the participation—quantity and especially quality—during the discussion of the case. This might be a simple check, check-plus, check-minus or zero. The instructor should involve as many students as possible. In order to engage all the students, the instructor can divide them into groups, give each group several minutes to discuss how to answer a question related to the case, and then ask a randomly selected person in each group to present the group’s answer and reasoning. Random selection can be accomplished through rolling of dice, shuffled index cards, each with one student’s name, a spinning wheel, etc.
Tips on the Penn State U. website: https://sites.psu.edu/pedagogicalpractices/case-studies/
If you are interested in using this technique in a science course, there is a good website on use of case studies in the sciences at the National Science Teaching Association.

- 14 min read
Data-Driven Decision-Making Case Studies: Insights from Real-World Examples
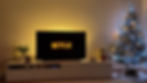
Data has become crucial for making informed decisions in today's fast-paced and ever-changing business environment. Companies use data to gain valuable insights, improve processes, and foster innovation. By studying successful examples of data-driven decision-making, we can gain valuable insights and comprehend the impact of data-driven strategies on business outcomes.
Define Data-Driven Decision Making (DDDM)
Are you tired of making business decisions based on gut instincts and guesswork? It's time to adopt Data-Driven Decision Making (DDDM). DDDM is a strategic approach that leverages collected data to inform and guide your business decisions. You can gain insights by identifying patterns and making informed choices using relevant and accurate data. "This can enhance the precision and efficiency of your decision-making procedure." allowing you to optimize outcomes, mitigate risks, and adapt more dynamically to changing circumstances in today's data-rich environment. Switch to DDDM and give your business the competitive edge it needs!
Importance of DDDM in Modern Businesses
In today's fast-paced and competitive business world, making informed and accurate decisions is more critical than ever. Data-Driven Decision Making (DDDM) is a powerful tool to help modern businesses achieve this goal. By using data and insights to inform business decisions rather than relying on guesswork, companies "Businesses that strategically position themselves to gain a competitive advantage are more likely to achieve success." With DDDM, businesses can make data-backed decisions, leading to better outcomes and tremendous success. So, if you want to stay ahead of your competition and make helpful decisions that drive success, embracing DDDM is the way to go!
Brief Overview of the Success Stories to be Discussed
Discover the success stories showcasing how businesses leverage advanced technologies to drive growth and profitability. Join me for an engaging and thought-provoking session where we will delve into the intricacies of these fascinating case studies. Your active participation will help us uncover valuable insights and unlock new perspectives that can benefit your work. "Make the most of this valuable opportunity to enhance your knowledge and skills!"
1. Netflix's Personalized Recommendations
2. Amazon's Supply Chain Optimization
3. Starbucks Location Analytics
4. American Express Fraud Detection
5. Zara's Fast Fashion Foresight.
You can benefit greatly from this unique opportunity to learn from some of the most innovative companies in the industry. Ensure you take advantage of this chance to expand your knowledge and skills!
Case Study 1: Netflix's Personalized Recommendation
Overview of netflix's challenges in content delivery.
Netflix faced challenges delivering content due to the diverse viewer preferences and vast content library. However, the company has been working hard to address these challenges and ensure users can discover content that aligns with their tastes. By doing so, Netflix aims to improve user satisfaction and retention rates.
How Netflix Used Viewer Data to Tailor Recommendations
By leveraging extensive viewer data, Netflix confidently tackled the challenge of recommending relevant content to its users. The platform thoroughly analyzed user behavior, viewing history, and preferences to create highly sophisticated algorithms. These algorithms were based on machine learning and could personalize content recommendations for each user. This approach significantly increased the likelihood of viewers engaging with content that resonated with their interests.
The Impact on Customer Retention and Satisfaction
The personalized content recommendations profoundly affected customer retention and satisfaction rates. Netflix enhanced the value of its service by providing users with content that closely matched their preferences. This created a stronger bond between users and the platform, leading to longer subscription durations and increased satisfaction.
Lessons Learned and Key Takeaways
Data is a Strategic Asset: Netflix's strategic use of data has wholly revolutionized content delivery. By utilizing excellent viewer data, they have successfully met the needs and preferences of each viewer in an incredibly effective manner.
Personalization Enhances Customer Experience: Personalized recommendations are essential to enhancing the overall customer experience. They can increase engagement, satisfaction, loyalty, and retention. Make no mistake - if you want to take your business to new heights, personalized recommendations are a must!
Continuous Adaptation is Crucial: It is crucial to adapt to achieve success, as Netflix continuously demonstrates. With the ever-evolving preferences of viewers, it is imperative to perform ongoing analysis and make necessary adjustments to algorithms to ensure that recommendations stay consistently relevant.
Balancing Privacy and Personalization: When utilizing viewer data, it is crucial to hit the right balance between personalization and privacy. Netflix has accomplished this by delivering highly personalized recommendations without compromising user privacy.
Netflix's approach to content delivery serves as an inspiration for the transformative power of data-driven decision-making. The personalized recommendations derived from customer data have proven to be a game changer regarding customer retention and satisfaction. The significance of adaptability, strategic use of data, and the balance between personalization and privacy, as highlighted by Netflix's success, can serve as a guide for other businesses looking to impact their customers positively.
Case study 2: amazon's supply chain optimization.
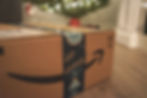
Understanding Amazon's Complex Supply Chain
Amazon has a complex supply chain involves various stages, from sourcing the products to delivering them to customers. The company manages a vast network of fulfillment centers, distribution hubs, and transportation systems. The complexity arises due to the need to manage different types of products, fluctuating demand, and the commitment to fast and efficient delivery.
Implementation of Predictive Analytics for Inventory Management
Using predictive analytics, Amazon has optimized inventory management by accurately forecasting future demand by analyzing historical data, current market trends, and seasonality. This has helped them prevent stockouts and overstock situations, improving their overall business efficiency and customer satisfaction.
Results Achieved in Cost Savings and Delivery Times
Anticipating the future, the implementation of predictive analytics is expected to yield significant results. Amazon will likely achieve cost savings by minimizing excess inventory and improving warehouse efficiency. Additionally, streamlined inventory management contributes to faster order fulfillment, which reduces delivery times and enhances the customer experience. We can expect a boost in efficiency and a better customer experience shortly.
Insights Gained and How Businesses Can Apply Similar Strategies
Data-Driven Decision-Making: In today's business landscape, data-driven decision-making has become the cornerstone of success. If you want your business to thrive, you must leverage advanced analytics to gain actionable insights into your supply chains. This will enable you to make proactive and strategic decisions to enhance your projects. Don't fall behind the competition - take charge and start leveraging the power of data-driven decision-making now.
Dynamic Inventory Optimization: Incorporating a dynamic approach to inventory management that relies on predictive analytics is "Businesses must prioritize their competitive edge and remain ahead of the industry. This is crucial to ensure success and longevity.". It helps them to quickly adjust to changing market conditions and meet the ever-evolving demands of consumers. This not only optimizes the utilization of resources but also reduces wastage, making it a sound strategy crucial for any business that wishes to survive in today's competitive market landscape.
Focus on Customer-Centric Logistics: To improve customer satisfaction, businesses can focus on optimizing logistics and reducing delivery times. Amazon's customer-centric approach demonstrates the importance of fast and reliable delivery. Companies can boost customer loyalty and drive growth by enhancing the customer experience.
Investment in Technology: To stay ahead in supply chain optimization, businesses must adopt cutting-edge technologies like AI and machine learning. Amazon's supply chain success is a testament to the power of continuous investment in technology. So, if you want to thrive in today's competitive market, it's high time you leverage these technologies to your advantage.
Amazon's journey toward optimizing its supply chain through predictive analytics has tremendously impacted cost savings and delivery times. Other businesses can achieve similar results by utilizing data-driven decision-making, implementing dynamic inventory management, prioritizing customer-centric logistics, and investing in advanced technologies.
Case study 3: starbucks location analytics.
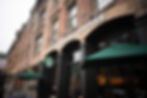
The Problem with Traditional Site Selection
The traditional approach to retail site selection, which relied on broad demographic data and market trends, must be improved in identifying optimal locations. Adopting a more precise approach that considers specific local factors influencing consumer behavior and store performance is imperative to ensure success.
How Starbucks Leveraged Geographic Information Systems (GIS)
Starbucks has transformed its approach to selecting store locations by leveraging Geographic Information Systems (GIS). This innovative technology has enabled Starbucks to systematically evaluate and visualize location-specific data, such as foot traffic patterns, nearby businesses, demographics, and local economic factors. By conducting this comprehensive analysis, Starbucks can gain a more nuanced understanding of potential store locations and make informed decisions.
Outcomes in Terms of New Store Performance and Sales
Starbucks, the renowned coffeehouse chain, has achieved notable success in its site selection strategy by implementing a Geographic Information System (GIS). GIS technology has enabled Starbucks to strategically place its new stores in locations that cater to the preferences and traffic patterns of the local population. As a result, the company has witnessed a significant improvement in the performance of its new stores, surpassing the sales of those selected through traditional methods. The successful implementation of GIS in site selection has contributed to optimizing location decisions, leading to a more efficient and effective expansion strategy for Starbucks.
Broader Implications for Retail Location Decision-Making
Precision in Site Selection: Geographic Information System (GIS) technology has opened new doors for retailers to make informed decisions. By leveraging GIS, businesses can analyze and interpret specific geographic data to optimize their site selection process. This helps them understand local nuances and customer behavior more precisely, allowing them to make data-driven decisions that lead to better business outcomes.
Adaptability to Local Factors: To establish a closer relationship with their customers, retailers must consider various local factors such as competition, demographics, and cultural preferences. By doing so, they can customize their offerings and marketing strategies to fit the local communities' specific needs and preferences. This approach can lead to better customer engagement and loyalty and, ultimately, higher sales for the retailer.
Cost Efficiency: Regarding retail businesses, selecting the right location for a store is crucial for success. Optimal site selection can significantly reduce the risk of underperforming stores and minimize the financial impact of poor location decisions. Retail businesses can enhance their overall cost efficiency and profitability by doing so. This is why it is essential for companies to carefully analyze and consider factors before making any site selection decisions.
Strategic Expansion: Geographic Information System (GIS) provides retailers with a powerful tool to make informed decisions about expanding their business. By leveraging location-based data, retailers can discover new markets and potential locations for growth. This data-driven approach helps retailers create a more sustainable and prosperous expansion plan, resulting in long-term prosperity for the business.
Enhanced Customer Experience: Retailers can improve the shopping experience for their customers by strategically selecting store locations that cater to their preferences and habits. Retailers can attract more foot traffic and enhance customer satisfaction by offering conveniently located stores. In this case, it can increase sales and customer loyalty.
To make it more understandable, Starbucks has been using fantastic GIS technology to help them pick the best locations for their stores. It's like a digital map that allows them to look at much different information, like how many people live nearby, how much traffic there is, and what other businesses are in the area. By using this technology, Starbucks can make better choices about where to put their stores and how they can be successful. Other businesses can also use GIS to make better decisions about where to open new stores and how to compete in the changing world of retail.
Case study 4: american express fraud detection.
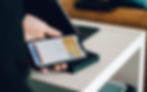
Rise in Credit Card Fraud and the Challenge for Card Issuers
As more and more people turn to digital transactions and online commerce, it's essential to be aware of the increased incidence of credit card fraud. Protect yourself and others from financial crimes by staying informed and proactively safeguarding your financial information. Thus, posing a significant challenge for card issuers. Fraudsters are constantly devising new and innovative tactics to steal sensitive information and exploit vulnerabilities in payment systems. This makes it imperative for financial institutions to stay ahead of the game in detecting and preventing fraudulent activities. Today, with advanced technologies like machine learning and artificial intelligence, card issuers can analyze big data that can identify patterns and anomalies and indicate fraudulent behavior. By adopting a proactive approach to fraud detection and prevention, financial institutions can safeguard their customers' personal information and financial assets, thus building trust and loyalty among their clients.
American Express's Use of Machine Learning for Early Detection
American Express always prioritizes the security of its customers' financial transactions. The company has employed advanced machine-learning algorithms to analyze vast amounts of real-time transaction data to achieve this. These algorithms can identify patterns, anomalies, and behavioral indicators typically associated with fraudulent activities. By continuously learning from new data, the system adapts to evolving fraud tactics and enhances its ability to detect irregularities early on. This advanced technology is a critical component of American Express's fraud prevention strategy, and it helps the company safeguard its customers against potential financial losses.
Effectiveness in Preventing Fraud and Protecting Customers
American Express utilizes an advanced fraud detection system powered by machine learning that has demonstrated exceptional efficacy in preventing fraudulent activities and safeguarding customers. By detecting fraudulent transactions early, the company can promptly take necessary measures, such as notifying customers of suspicious activities or blocking them altogether, thus reinforcing trust in the company's commitment to security and ensuring customer satisfaction.
What Companies Can Learn About Proactive Data Monitoring
Invest in Advanced Analytics: Advanced analytics, such as machine learning, helps companies proactively monitor data for potential fraud indicators and unusual patterns. It identifies issues before they become significant problems, saving the company millions. It also identifies new business opportunities, market trends, and operational inefficiencies, enhancing customer satisfaction and the bottom line.
Real-Time Analysis: Real-time data analysis is a powerful tool for detecting and responding to suspicious activities. By monitoring data in real-time, organizations can quickly "Identify possible threats and take prompt action to reduce their impact." we can overcome any challenges that come our way and pave the path to success. This approach reduces the window of vulnerability and enhances the effectiveness of fraud prevention measures. Therefore, real-time data analysis can help organizations prevent fraudsters from exploiting them. It's important to stay vigilant and proactive in protecting yourself and your finances from potential threats. Please take action now and don't give them the chance to cause any harm. Remember, it's better to be safe than sorry. their interests.
Continuous Learning Systems: Adopting systems that can learn and adapt to new fraud patterns is highly recommended. This approach ensures that the monitoring mechanisms remain up-to-date and effective despite the constantly evolving threats. Embracing such systems can protect businesses. The objective is to safeguard individuals and organizations against financial losses and reputational harm resulting from fraudulent activities.
Customer Communication: Implementing solid and effective communication methods is essential to inform customers of any potential fraud promptly. Through transparent communication, customers can be informed of the situation and take immediate action, building trust between them and the organization.
Collaboration with Industry Partners: Collaborating with industry partners and sharing insights on emerging fraud trends is essential. By working together, we can enhance our ability to combat fraud and protect the entire ecosystem. We can stay informed and better equipped to prevent fraudulent activities through a collective effort.
Balancing Security and User Experience: It's crucial to balance strong security measures with a seamless user experience for online platform security. While taking all necessary steps to prevent fraud and unauthorized access to your system is critical, ensuring that your legitimate customers don't face any inconvenience or dissatisfaction due to stringent security protocols is equally essential. Therefore, adopting a multi-layered approach to security is recommended to shield your system from potential threats without making the user experience cumbersome or frustrating. This may involve utilizing two-factor and risk-based authentication and real-time fraud detection. Furthermore, educating users on secure online practices and equipping them with the necessary tools and resources to protect their personal information and transactions is essential.
American Express has implemented machine learning techniques to detect and prevent fraud at an early stage. This is an excellent example for businesses seeking to improve their proactive data monitoring capabilities. By adopting advanced analytical tools, real-time analysis, continuous learning systems, and effective communication, companies can establish a solid and proactive strategy to combat emerging threats in the digital realm.
Case study 5: zara's fast fashion foresight.
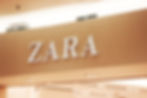
Fast Fashion Industry Challenges in Demand Forecasting
The fast fashion industry, known for producing trendy and affordable clothing rapidly, faces significant challenges in accurately predicting consumer demand. One of the main reasons for this is the constantly changing nature of fashion trends. What is popular today may be out of fashion tomorrow, making it difficult for companies to plan their production processes effectively.
Additionally, fast fashion products have short life cycles, meaning they are only in style for a limited time. As a result, companies need to be able to respond to market shifts and adjust their production accordingly quickly. This can be challenging, as traditional forecasting methods rely on historical data, which may need to be more relevant in a fast-changing market.
To overcome these challenges, the fast fashion industry needs innovative and agile forecasting methods to keep up with the dynamic nature of consumer preferences. This may involve leveraging data analytics and machine learning algorithms to identify emerging trends and predict future demand. Companies can enhance efficiency, reduce waste, and provide excellent customer value.
Zara's Integration of Real-Time Sales Data into Production Decisions
Zara, one of the world's leading fashion retailers, has redefined the fashion industry by leveraging real-time sales data. Zara has integrated real-time sales data into its production decisions, allowing the company to stay ahead of the competition. Zara's vertically integrated supply chain and responsive production model enable it to capture up-to-the-minute sales data from its stores worldwide. This data is then fed back to the design and production teams, who use it to rapidly adjust inventory levels and introduce new designs based on current demand trends. Using real-time sales data, Zara can create a customer-centric approach, ensuring its customers always have access to the latest and most stylish designs.
Benefits Seen in Reduced Waste and Increased Sales
Zara has adopted a real-time analytics approach that has proven to be highly beneficial. The company's production is now closely aligned with actual customer demand, which results in a significant reduction in overstock and markdowns. This approach has minimized the environmental impact of excessive inventory. In addition, the quick response to emerging trends and consumer preferences has led to an increase in full-price sales, boosting revenue and profitability for Zara.
Strategies for Incorporating Real-Time Analytics into Product Development
Connected Supply Chain: Establishing a connected and transparent supply chain that enables seamless real-time data flow from sales channels to production and design teams is imperative. This will ensure that all the teams are on the same page and can make quick, informed decisions based on accurate, up-to-date information. Failure to do so can result in costly delays, inefficiencies, and missed opportunities. So, let's prioritize this and set up a robust supply chain that works for us!
Agile Production Processes: To maintain a competitive edge, it is crucial to adopt constructive and flexible production processes that can quickly respond to changes in demand. This involves embracing shorter production cycles and smaller batch sizes, which makes us more efficient and proactive in meeting customer needs.
Advanced Data Analytics: To optimize your sales strategies, you can use advanced data analytics tools to process and analyze real-time sales data efficiently. You can accurately forecast demand and make data-driven decisions by implementing predictive modeling and machine learning algorithms.
Cross-Functional Collaboration: Promoting collaboration among different organizational departments is crucial to ensure the sales, marketing, design, and production teams have a unified interpretation of real-time data. This way, they can collectively make informed decisions that are in the company's best interest. The organization can improve its efficiency, productivity, and profitability by promoting open communication and collaboration between departments.
Customer Feedback Integration: One way to enhance the accuracy of real-time analytics is to consider customer feedback and preferences. Social media listening and direct customer interactions can provide valuable insights into emerging trends and demands.
Technology Integration: As we look towards the future, it's becoming increasingly clear that investing in technologies that facilitate real-time data collection and processing will be crucial. With the rise of automation and the growing need for instant information, businesses that have point-of-sale systems, inventory management software, and communication tools that streamline information flow will be better equipped to thrive in the fast-paced and ever-changing world of tomorrow. So, it's never too soon to start thinking about how you can integrate these technologies into your business strategy.
Zara's outstanding achievement in the fast fashion industry is a remarkable example of how incorporating real-time sales data into production decisions can lead to immense success. By reducing waste, swiftly responding to market trends, and utilizing advanced analytics, Zara has set a benchmark for other companies in integrating real-time insights into their product development strategies. This approach enhances efficiency and competitiveness in the highly dynamic and ever-evolving fashion industry.
In the constantly shifting business realm, the adage' knowledge is power' has never been more accurate, particularly about tangible, data-derived knowledge. data-driven decision making (dddm) presents an approach where critical business decisions are made not on intuition or experience alone, but on deep dives into data analysis. empirical evidence garnered through this method provides actionable insights leading to strategic, evidence-based decisions..
The stories of Netflix, Amazon, Starbucks, American Express, and Zara demonstrate the immense potential of Data-Driven Decision Making (DDDM). By analyzing vast data points and leveraging advanced analytics, these companies transformed their businesses and achieved unparalleled success.
For instance, Netflix utilized DDDM to create tailor-made recommendations, engaging existing users and attracting new ones. Amazon used data analytics to optimize its supply chain, lowering costs and accelerating shipping times. Starbucks leveraged location analytics to predict the profitability of new store locations with impressive accuracy. American Express used machine learning algorithms to identify frauds faster than ever, saving millions in potential losses. Lastly, Zara demonstrated agility in the competitive fast fashion market by adapting its production and supply chain to meet real-time demand.
As seen in these success stories, data-driven decision-making can powerfully impact business, from customer engagement to trend forecasting. They underscore the importance of meticulous data analysis in navigating the present and forecasting an ever-changing future, paving the way for unparalleled business success. Companies can draw inspiration from these cases and embark on their DDDM journey to achieve similar outcomes.
Recent Posts
Maximizing the potential of WFM in the Gig Economy: Achieving the perfect balance between Flexibility and Stability through Case Studies.
WFM in the Gig Economy: Balancing Flexibility and Stability
Data-Driven Decision Making: Utilizing Analytics in Workforce Management
Case Study Mastery: Examples & Step-by-Step Templates
Master case study: Uncover key strategies to conduct & present findings that influence decisions charachters.
February 9, 2024
.webp)
What's Inside?
Understanding and sharing success stories in the business management world is crucial for grasping the growth journey of a business.
In this article, we will delve into the concept of a business management case study, exploring its definition, benefits, limitations, step-by-step process, types, and essential elements.
What is a Case Study?
A case study research is a detailed analysis of a particular subject, often a real-world situation or scenario, to draw insights and conclusions. It serves as a valuable tool for learning from successful strategies, identifying challenges, and making informed decisions.

Key Characteristics of a Case Study:
Specific Focus: Case studies concentrate on a particular subject, narrowing down the scope to delve deeply into specific aspects.
Real-world Context: Unlike theoretical studies, case studies are grounded in the real world. They often involve the examination of actual events, circumstances, or challenges.
Comprehensive Exploration: Case studies involve a thorough investigation of multiple facets of the chosen subject. This may include collecting and analyzing data, conducting interviews, and reviewing relevant documents.
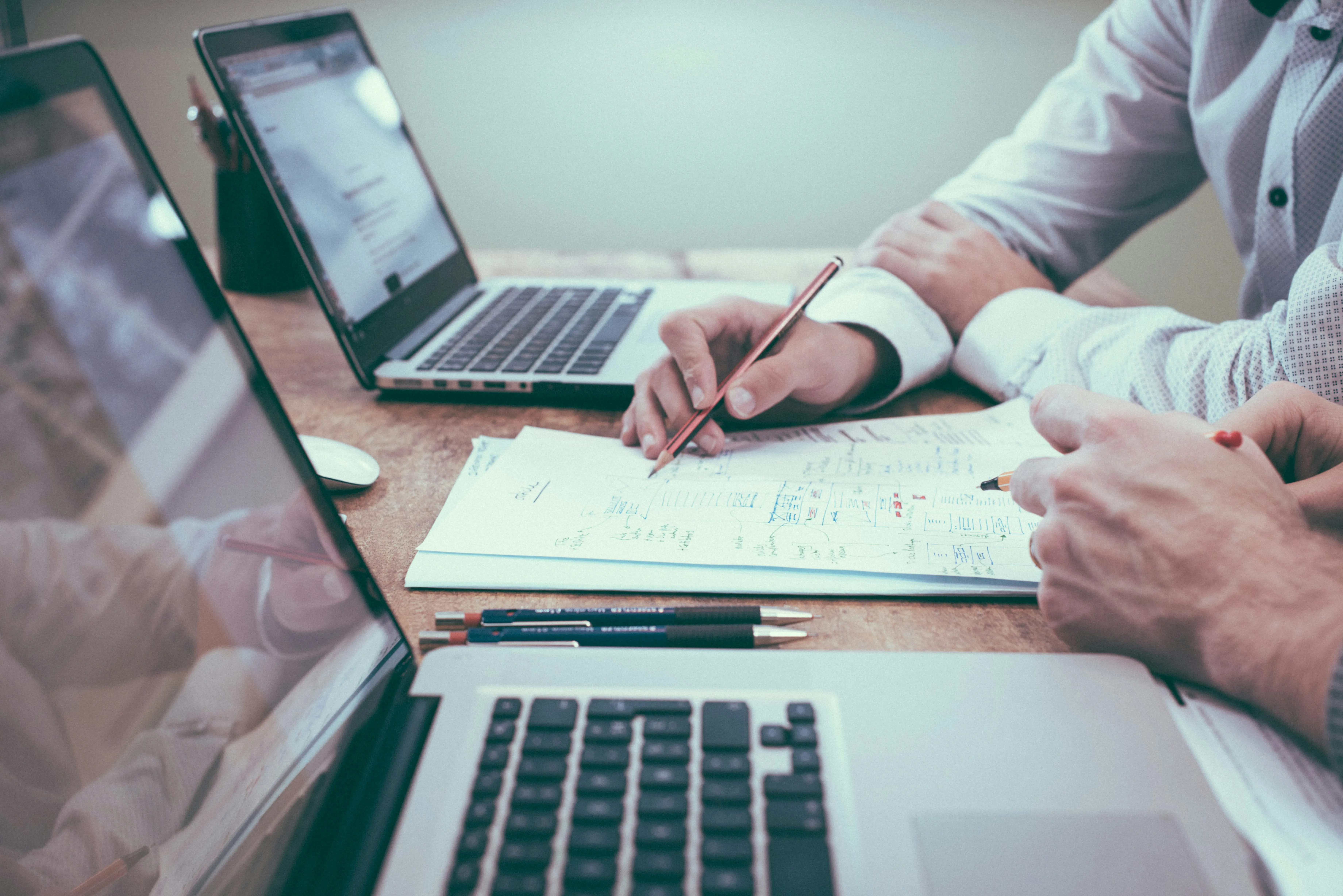
Contextualization: Each case study is set within a context, providing background information to help readers or viewers understand the circumstances surrounding the case.
Problem-Solving Orientation: While exploring the intricacies of a case, case studies often aim to identify problems, challenges, or opportunities. They can be used as tools for problem-solving and decision-making.
In-depth Analysis: The analysis in a case study goes beyond surface-level observations. It involves a detailed examination of factors contributing to the situation, allowing for a nuanced understanding.
Presentation of Findings: A case study concludes with the presentation of findings, insights, and conclusions. Leveraging a visually compelling presentation plays a vital role for a case study to speak out.
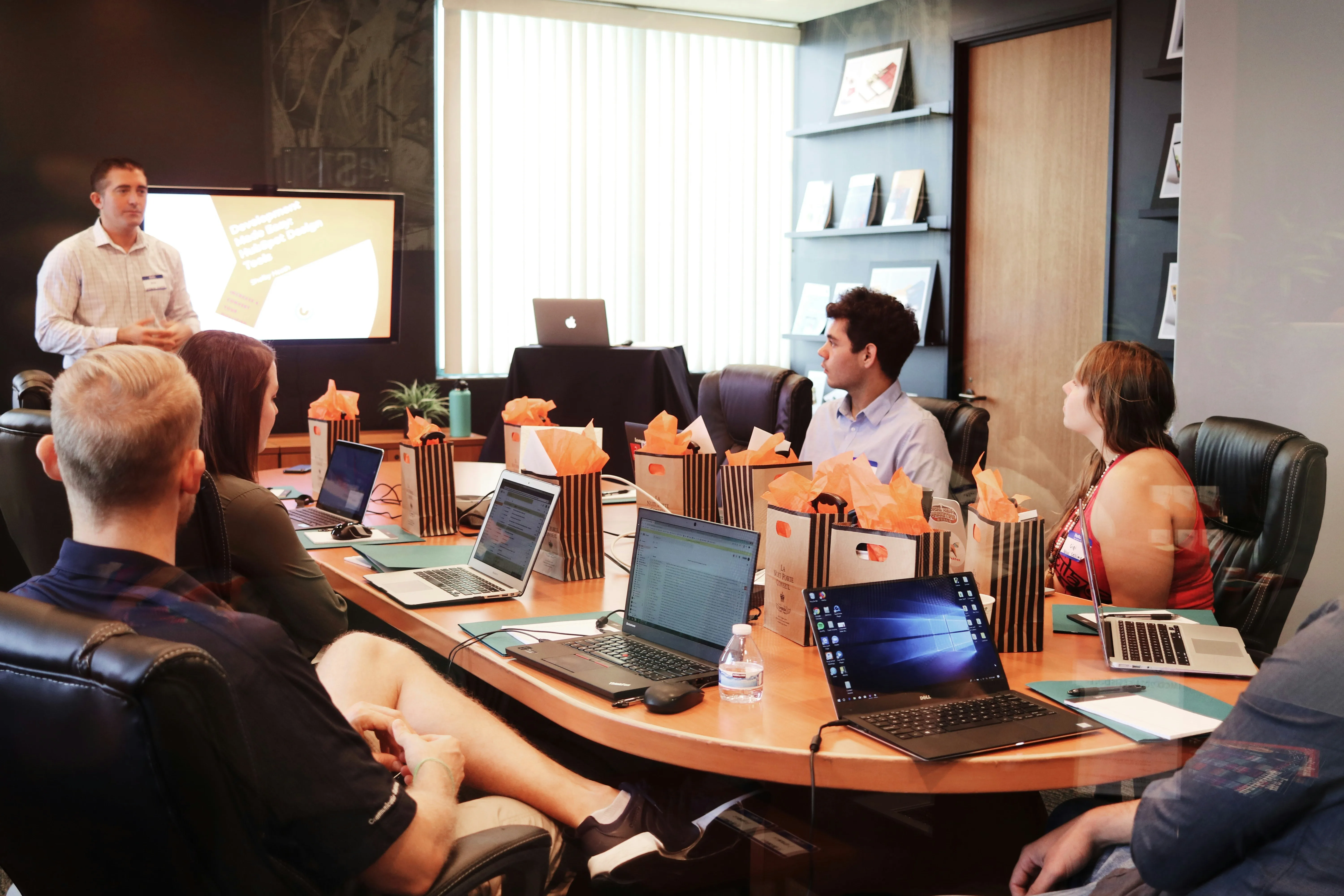
Why You Should Write a Case Study?
Writing a case study offers several compelling reasons for individuals and businesses alike:
Demonstrate Success: A case study allows you to showcase your achievements and successes. It provides tangible evidence of your capabilities, helping build trust and credibility with potential clients, customers, or collaborators.

Educate and Inform: Use case studies to share valuable insights, lessons learned, and best practices. By documenting your experiences, you contribute to the collective knowledge within your industry, positioning yourself as an authority and resource.
Problem-Solving Showcase: If your case study revolves around overcoming challenges, it highlights your problem-solving abilities. This can be particularly impactful in industries where complex issues require innovative solutions.
Engage Your Audience: Well-crafted case studies are engaging and resonate with your audience. They tell a story, making information more relatable and memorable. This storytelling aspect can captivate readers and enhance their understanding of your work.

Build Brand Awareness: Case studies provide an opportunity to promote your brand in a context that goes beyond traditional marketing. Through real-world examples, you can reinforce your brand message and values.
Attract New Opportunities: A compelling case study can attract new opportunities, whether it be clients, partnerships , or collaborations. It serves as a powerful marketing tool, showcasing your expertise and capabilities to a wider audience.
Validate Your Methods: For businesses, case studies serve as a validation of their methods and strategies. Employing a robust case study methodology is a way to demonstrate the effectiveness of your products, services, or approaches to potential clients or customers through a thorough research process.
Internal Learning: Writing a case study requires reflection on your processes and approach case outcomes. This internal learning process can contribute to continuous improvement within your organization , fostering a culture of innovation and adaptability.
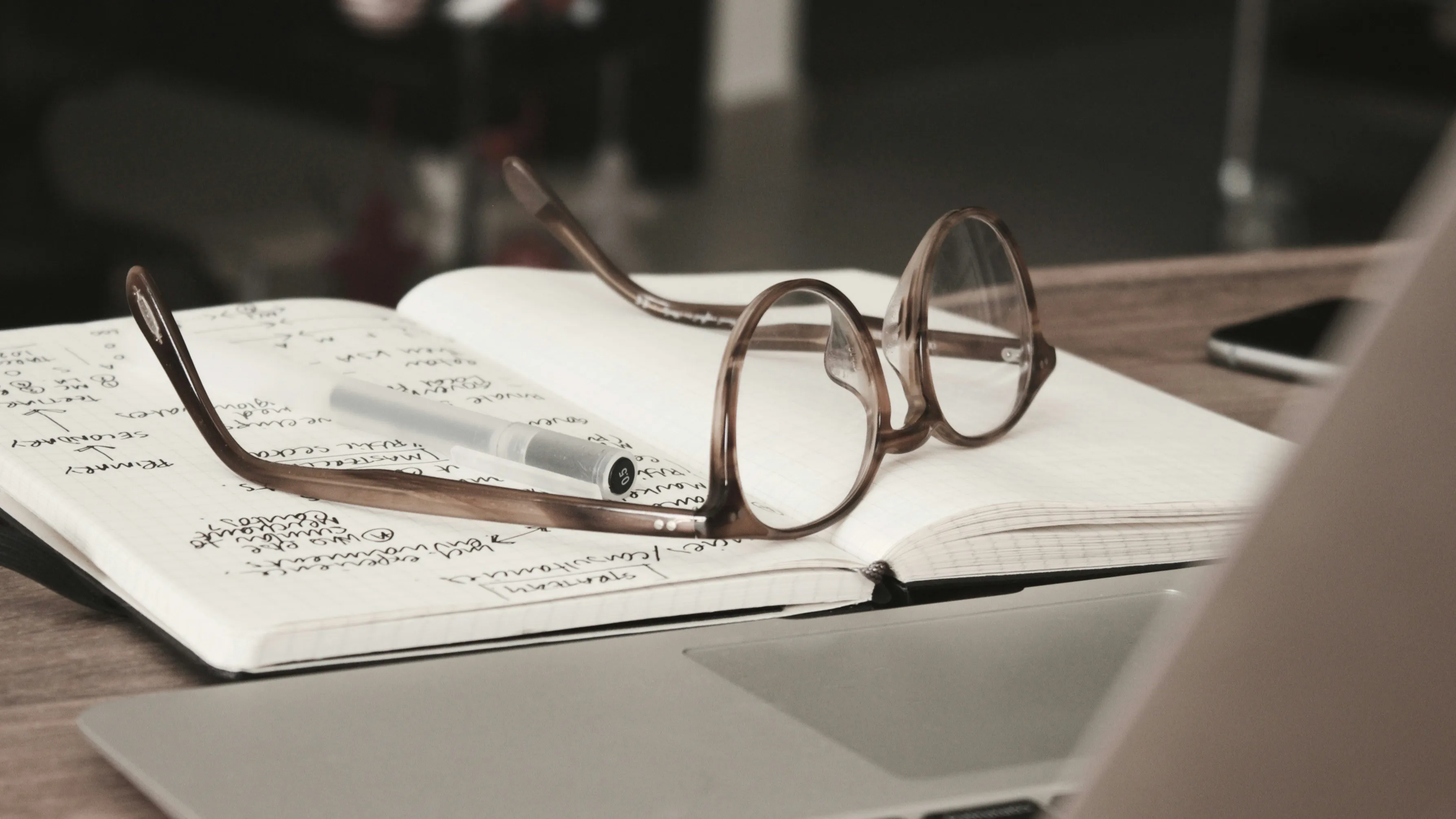
SEO Benefits: Case studies can be optimized for search engines, contributing to your online visibility. Including relevant keywords and internal links in your case studies can improve your website's SEO , attracting more organic traffic.
Differentiation: In competitive industries, a well crafted case study sets you apart from the competition. It allows you to highlight what makes your approach unique and why clients or customers should choose your products or services.
Benefits and Limitations of Case Studies

Benefits of Case Studies:
- Evident Success Stories: Case studies serve as tangible evidence of a business's success, allowing them to showcase real-world achievements and build credibility with potential clients or customers.
- Effective Marketing Tool: They function as powerful marketing tools by providing in depth insights into a business's capabilities , differentiating it from competitors, and influencing the decision making process of potential clients.
- Client Relationship Building: Through detailed case studies, businesses can strengthen relationships with existing clients by demonstrating their commitment, problem solving abilities, and delivering measurable results.
- Versatile Content: Case studies offer versatile content that can be repurposed across various marketing channels, including websites, social media, presentations, and promotional materials.
- Educational Value: Businesses can use case studies to educate their target audience about their industry, innovative solutions, and best practices, positioning themselves as thought leaders.
Limitations of Case Studies:
- Resource Intensive: Creating comprehensive case studies demands significant resources, including time, effort, and potential costs, making them resource-intensive for businesses.
- Limited Generalization: Findings from a specific case study may not be universally applicable, limiting their generalizability to other scenarios or industries.
- Potential Bias: There is a risk of bias in the selection and presentation of information, as businesses may be inclined to emphasize positive outcomes and downplay challenges.
- Confidentiality Concerns: Businesses may face challenges in sharing detailed information, especially if it involves sensitive data or strategies, raising concerns about confidentiality.
- Difficulty in Replication: The unique circumstances of a case study may make it challenging to replicate the same success in different contexts, limiting the broader applicability of the insights gained.
How to Conduct a Case Analysis: Step-by-step
1. define the objective:.
- Clearly outline the purpose of the case study. What do you aim to achieve or understand through this analysis?
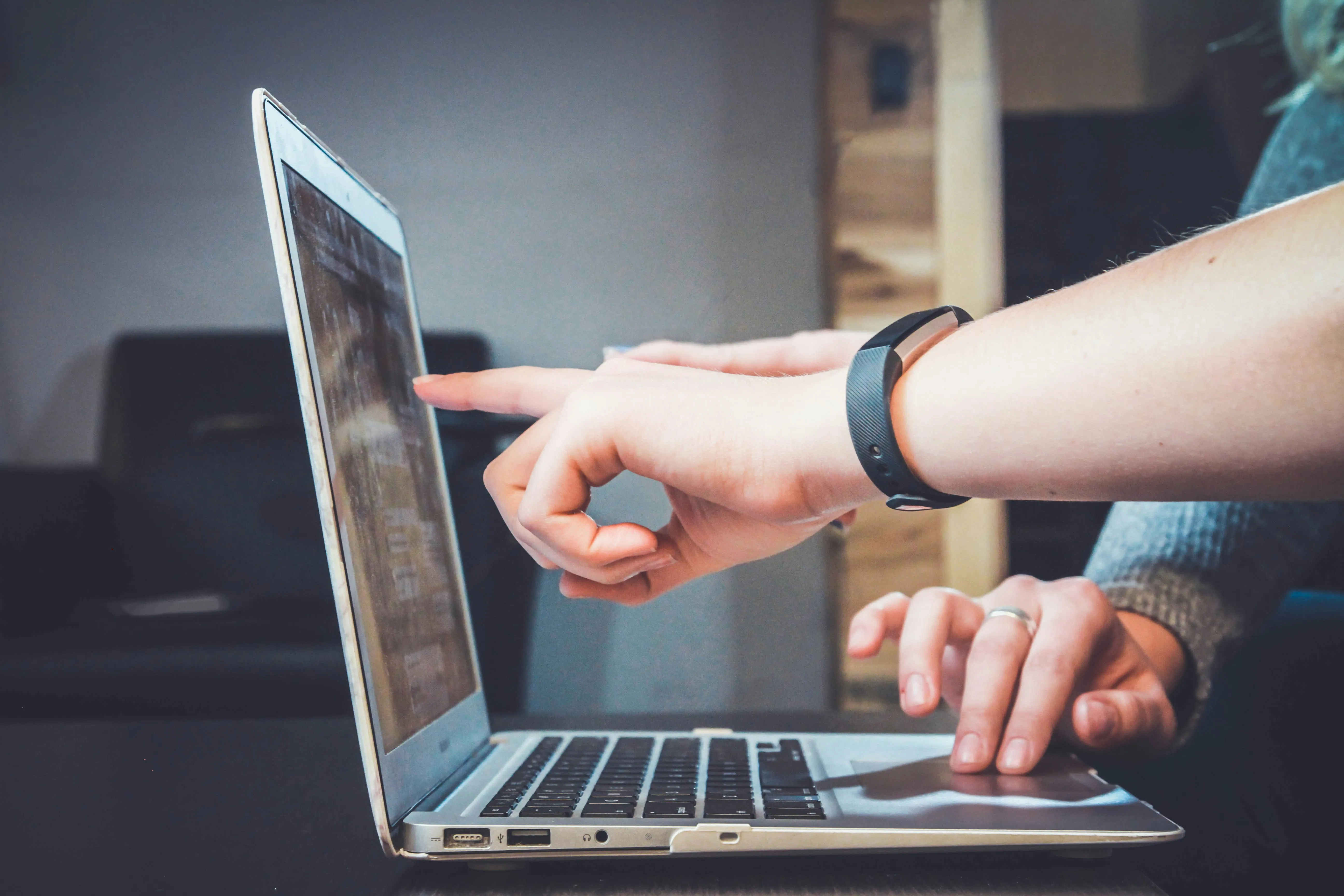
2. Select the Case:
- Identify a relevant and specific case that aligns with your objective. For an important case study this could be a real-world situation, event, or phenomenon.
3. Background Research:
- Gather background information about the case. This may include historical context, key players involved, and any existing literature on the subject.

4. Identify Key Issues or Questions:
- Formulate specific research questions or highlight key issues you want to address through the case study.
5. Choose the Research Method:
- Decide on the case study method or approach for data collection. A case study research method could involve qualitative methods such as interviews, observations, or document analysis.
6. Develop Data Collection Plan:
- Outline a detailed plan for collecting data. Specify sources, methods, and tools you will use to gather relevant information.

7. Data Collection:
- Execute the data collection plan. Conduct interviews , observe events, and analyze documents to accumulate necessary data.
8. Data Analysis:
- Apply appropriate analytical techniques to interpret the gathered data. This may involve coding, categorizing, and identifying patterns or themes.
9. Construct the Case Study Narrative:
- Organize the findings into a coherent and structured narrative. Develop sections that cover the introduction, background, analysis, and conclusion.

10. Draw Conclusions:
- Based on your analysis, after you conduct case study , draw conclusions that address the research questions or objectives. Consider the implications of your findings.
11. Peer Review or Feedback:
- Seek feedback from colleagues, mentors, or peers to ensure the validity and reliability of your case study.
12. Finalize the Case Study:
- Incorporate feedback and make necessary revisions. Finalize the case study, ensuring clarity, coherence, and adherence to ethical guidelines.
13. Document and Share:
- Prepare the case study for publication or presentation and take advantage of Decktopus AI, a user-friendly and efficient presentation generator powered by AI. Easily convert your case study insights into a visually compelling deck.

- Decktopus ensures your case studies are presented in a format that engages your audience, making your narratives more impactful and memorable. Explore the benefits of Decktopus AI to elevate your case study presentations effortlessly.
What are the Components of a Case Study
The format of a case study typically comprises several key components to present information in a structured and comprehensive manner. While variations may exist based on the context and purpose, a standard case study format often includes the following elements:
1. Introduction:
Provide a brief overview of the case and set the stage for the reader. Outline the main objectives and establish the context of the study.
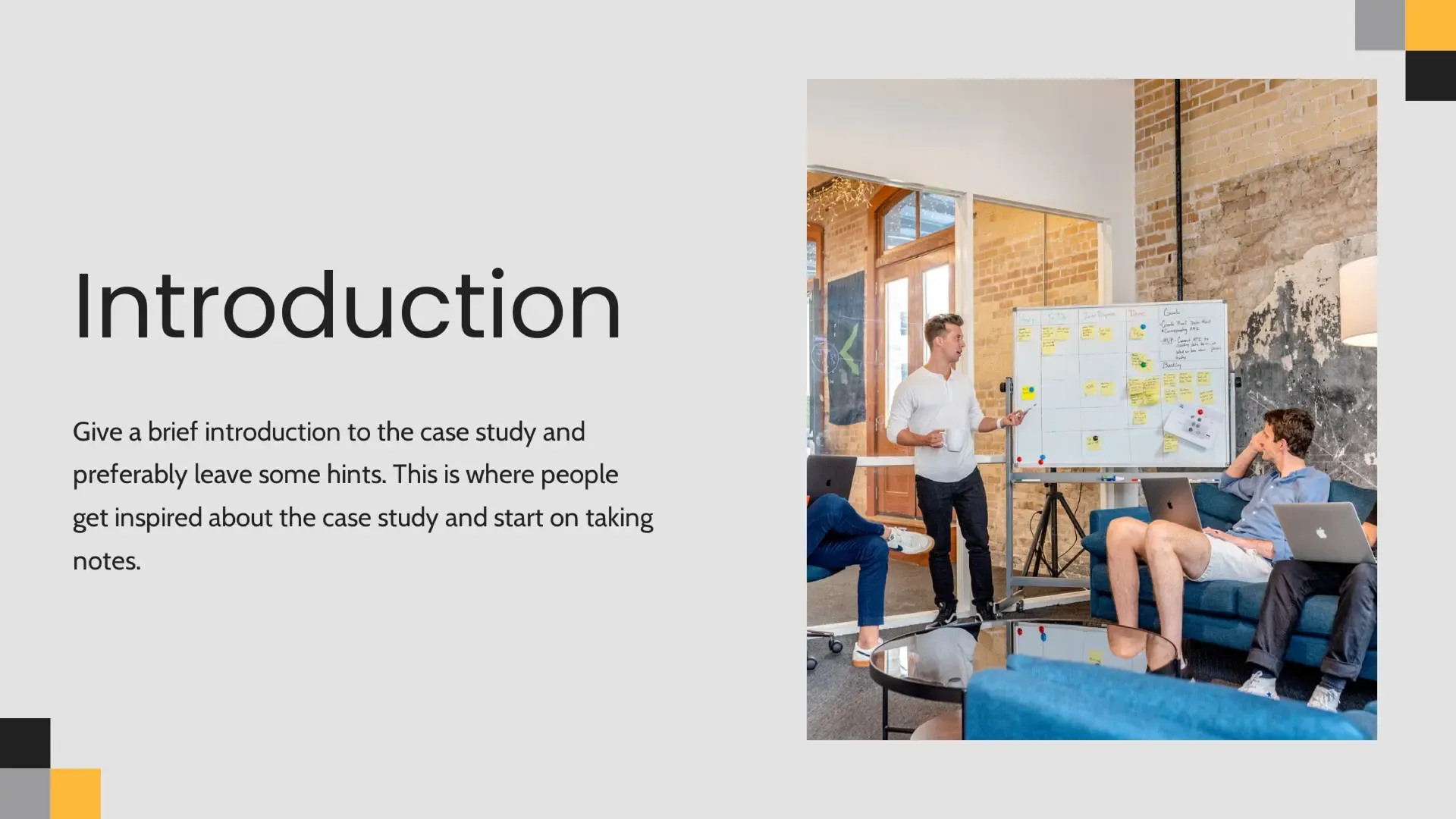
2. Background:
Present relevant background information about the subject of the case. This may include the history, industry context, or any pertinent details necessary for understanding the situation.
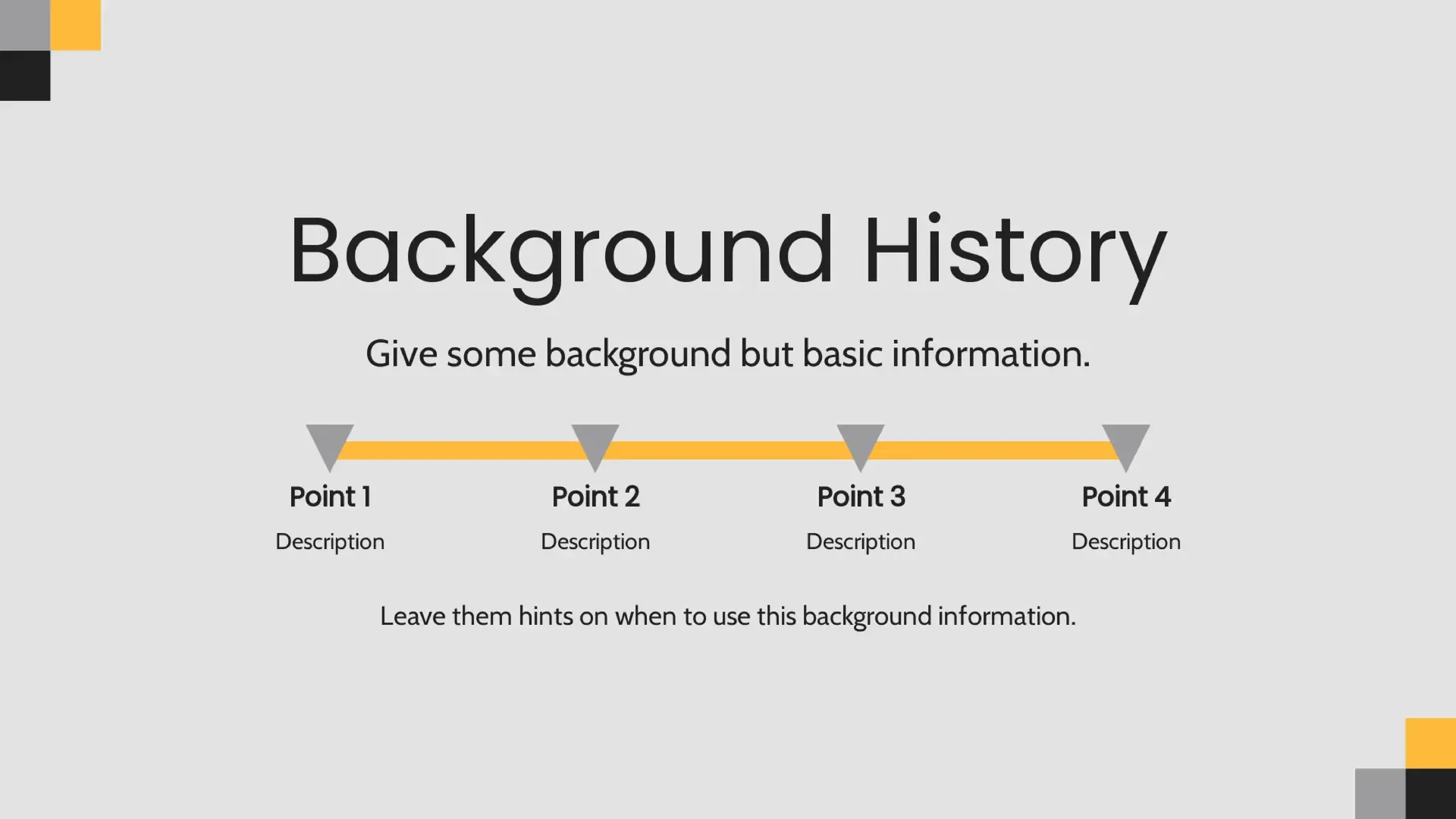
3. Problem Statement or Objectives:
Clearly state the problem or the main objectives of the case study. Define the issues or challenges that the study aims to address.
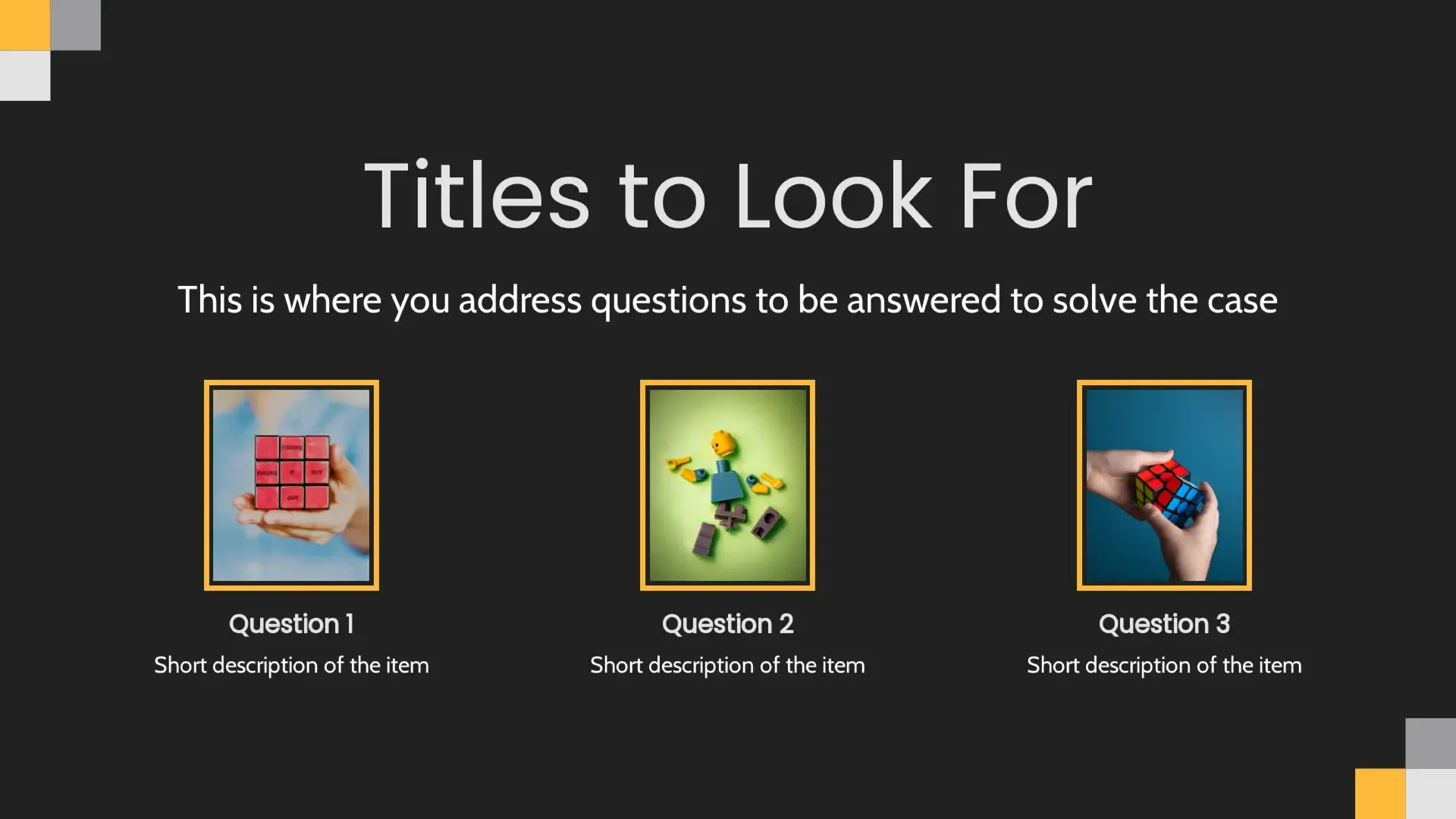
4. Analysis:
Dive into the analysis of the case. This section often comprises multiple sub-sections, each exploring different aspects such as market conditions, internal factors, external influences, etc.

5. Solution or Action:
Propose solutions or actions to address the identified problems. Detail the steps taken or recommended strategies based on the analysis.

6. Results:
Present the outcomes of the solutions or actions taken. Include any measurable results, impacts, or changes observed.

7. Conclusion:
Summarize the key points, outcomes, and lessons learned. Revisit the problem statement and emphasize the significance of the study, highlighting how the research design shaped the results.

Types of Case Studies
Case Study Type | Purpose | Use |
---|---|---|
Product Launch | Showcase successful new product introductions. | Demonstrate effective marketing strategies. |
Customer Success Stories | Highlight positive customer experiences. | Build credibility and trust in the product/service. |
Market Entry | Analyze successful entry into a new market. | Guide other businesses entering similar markets. |
Rebranding | Explain and showcase outcomes of brand repositioning. | Illustrate the impact on market perception. |
Digital Marketing Campaign | Evaluate the success of a digital marketing campaign. | Provide insights into effective digital strategies. |
Competitive Analysis | Assess how a company gained a competitive edge. | Identify success factors and areas for improvement. |
Social Media Engagement | Examine the impact of social media marketing. | Understand effective social media strategies. |
Failure | Learn from marketing failures. | Extract lessons for future marketing endeavors. |
Case Study Examples
1. marketing case study template.

The Marketing Case Study Template is tailored for marketers, highlighting successful marketing strategies . Uncover the methods employed, target audience engagement, and measurable outcomes.
Ideal for marketing professionals seeking insights into effective campaign executions. With Decktopus AI , spending your precious time perpetually recreating your product's presentation has become an ancient practice.
Along with our collection of case-study templates, with our one-click platform, you can easily create beautiful presentations for yourself or your clients.
Also check out: creative marketing case study template .
2. Sales Case Study Template

The Sales Case Study Template is designed for salespeople to present and discuss case studies in sales meetings. With its professional look and engaging layout, your clients will be impressed with the level of detail you put into your analysis.
This professionally designed template is easy to use and easy to customize, making it the perfect way to show off your small business expertise.
So whether you're looking to wow potential clients or just need a little more confidence in your sales meetings, our client case study template will help you make an impact.
Also check-out: case study template for sales teams .
3. Design Case Study Example: UI Case Study Template

The UI Case Study Template is specifically designed for UI designers, making it easy to discuss your design process and findings. Present your design case studies like a pro with our target-spesific case study templates. With our design case study template , you'll be able to showcase your work in a clear, professional manner.
Looking to create a stunning case study presentation for your next client meeting? Look no further than our case study templates! Our professional and easy-to-use templates are perfect for designers of all experience levels, and will help you showcase your work in a clear and concise way.
Also check out: Art Case Study Template .
Explore More Case Study Templates

Discover a vast collection of case study templates from various fields, including marketing, sales, and design, in our dedicated Case Study Examples Blog. Gain insights into diverse business scenarios and find inspiration for your own projects.
Case Study Presentation Creation with Decktopus AI
Streamlining the creation of engaging visual case studies has never been easier than with Decktopus AI . This innovative platform offers a seamless experiencensimply write your input, and Decktopus takes care of the rest, ensuring that your templates not only boast a polished visual appeal but also integrate relevant and impactful content effortlessly.
Discover how easy it is to create engaging case study templates using Decktopus AI . Our platform ensures your templates look great and contain relevant content. With the help of our AI assistant, you not only get support during presentations but also receive tips, facilitate Q&A, and increase overall engagement.
Explore the unique storytelling format that Decktopus offers, making your case studies more relatable. For a step-by-guide on how to easily create a visually stunning case study with Decktopus, see our case study examples blog.

This approach allows you to present information in a narrative style, connecting better with your audience. Find practical tips for smoother case study presentations, from effective storytelling to engaging your audience. Improve your presentation experience with Decktopus AI , where simplicity meets interactivity and storytelling for effective communication.
It features, practical design, mobilizing easy principles of marketing ecosystem platform design. Making it by far the easiest thing to use in your daily practice of mobilizing marketing ecosystems through platform strategies.
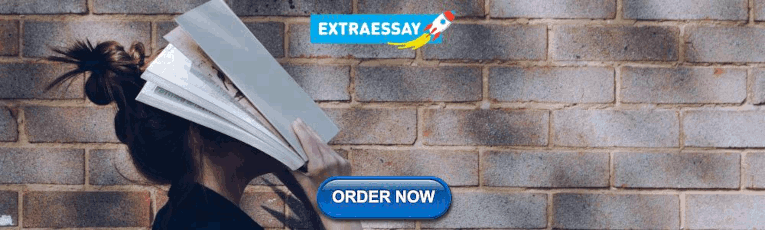
Frequently Asked Questions
1) what is a marketing case study.
A marketing case study is a concise analysis of a business's marketing strategy, showcasing its objectives, challenges, tactics, and outcomes. It offers practical insights into real-world marketing applications, serving as a valuable learning tool for understanding successful practices and lessons learned in achieving specific marketing goals.
2) What is a case study?
A case study, or case report, is a concise examination of a specific subject, often real-world situations or problems, providing detailed insights and analysis for learning or decision-making purposes.
3) How should you write a case study?
To create an impactful case study, define objectives, choose a relevant case, gather key information, and use Decktopus for a polished presentation. Employ data analysis, construct a clear narrative, and offer actionable recommendations.
Validate findings and consider broader implications. Decktopus streamlines this process, providing a user-friendly platform for creating compelling case study presentations effortlessly.
Latest Articles
.jpg)
August 28, 2024
The Ultimate Guide: How Sales Ops Can Use AI to Supercharge Their Reporting
Unlock the power of AI for your sales ops and supercharge reporting accuracy and efficiency! Use artificial intelligence to elevate your reporting!
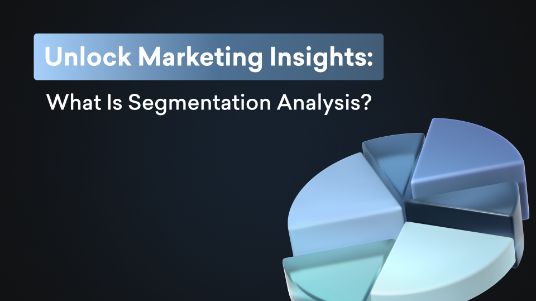
August 26, 2024
Unlock Market Insights: What Is Segmentation Analysis?
Discover how segmentation analysis uncovers customer insights for targeted market strategies Master your segments now!
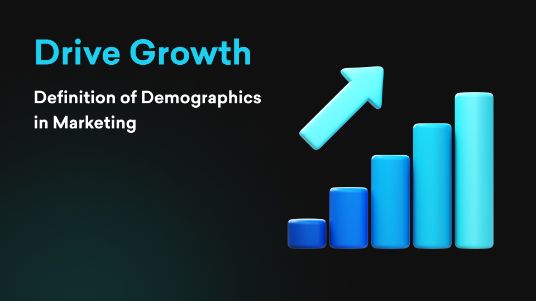
Drive Growth: Definition of Demographics in Marketing
Mastering demographics in marketing is key to growth Get the definition, insights, and segmentation strategies!

Don't waste your time designing your presentations by yourself!
Type your content and let our platform design your presentations automatically. No more wasting time for your presentations. Use hundreds of presentation templates to impress your audience. This is the only tool you need to prepare presentations. Try our Presentation Builder today >>
Don’t waste your time by trying to make a website for all your content
Place your content links and let our platform design your bio link automatically. No more wasting time for your social content distribution. Use hundreds of presentation biolink to impress your audience. This is the only tool you need to prepare good-looking bio links. Try our Bio Link Builder today >>
Do You Want To Create a Presentation?
Sign up for our newsletter to stay up-to-date on the latest news and tips from Decktopus.
Let’s create a form here to get visitors’ email addresses.
Ready to dive in? Start your free trial today.

- SUGGESTED TOPICS
- The Magazine
- Newsletters
- Managing Yourself
- Managing Teams
- Work-life Balance
- The Big Idea
- Data & Visuals
- Reading Lists
- Case Selections
- HBR Learning
- Topic Feeds
- Account Settings
- Email Preferences
Organizational decision making
- Business management
- Organizational culture

From Prediction to Transformation
- Ajay Agrawal
- Joshua Gans
- Avi Goldfarb
- From the November–December 2022 Issue

To See the Way Forward, Look Back
- Ranjay Gulati

How to Solve Problems
- Laura Amico
- October 29, 2021

Research: What Makes Employees Feel Empowered to Speak Up?
- Shilpa Madan
- Kevin Nanakdewa
- Krishna Savani
- Hazel Rose Markus
- October 13, 2021

LinkedIn Co-Founder Reid Hoffman on Innovating for an Uncertain Future
- Reid Hoffman
- Adi Ignatius
- January 07, 2022

The “Maximize Profits” Trap in Decision Making
- Joseph L. Badaracco
- September 19, 2016

When Autonomy Helps Team Performance — and When It Doesn’t
- Viktoria Boss
- Linus Dahlander
- Christoph Ihl
- Rajshri Jayaraman
- December 01, 2021

These Strategies Will Help You Influence How Decisions Get Made
- Robert C. Bordone
- Daniel Doktori
- November 11, 2021

Build a Corporate Culture That Works
- From the July–August 2024 Issue

How the Geeks Rewrote the Rules of Management
- September 15, 2023

4 Ways Silicon Valley Changed How Companies Are Run
- Andrew McAfee
- November 14, 2023

Drive Innovation with Better Decision-Making
- Linda A. Hill
- Emily Tedards
- From the November–December 2021 Issue

5 Ways Executives Can Manage Conflict with the Board
- Sabina Nawaz
- April 15, 2024

To Craft a Purpose That Motivates Your Team, Balance Pragmatism and Idealism
- Darren Ashby
- Atif Sheikh
- December 01, 2023

10 Signs Your Company Is Resistant to Change
- Frances X. Frei
- Anne Morriss
- September 26, 2023

Make Decisions with a VC Mindset
- Ilya A. Strebulaev
- From the May–June 2024 Issue
Problems of Matrix Organizations
- Stanley M. Davis
- Paul R. Lawrence
- From the May 1978 Issue

Research: Public Opinion Is Not Enough to Hold Companies Accountable
- Matthew Amengual
- Alexander Rustler
- September 06, 2022

Noise: How to Overcome the High, Hidden Cost of Inconsistent Decision Making
- Daniel Kahneman
- Andrew M. Rosenfield
- Linnea Gandhi
- From the October 2016 Issue
8 Simple Steps to Winning American Eyeballs in Beijing
- Thomas H. Davenport
- August 07, 2008

Polarizing Government Work: McKinsey & Co. and Immigration and Customs Enforcement (ICE)
- Andrew Hoffman
- April 20, 2022
Note on Organizational Structure
- Ethan S. Bernstein
- Nitin Nohria
- February 19, 1991
Ce Soir-Là, Ils n'Arrivent Plus Un par Un, Mais par Vagues: Coping with the Surge of Trauma Patients at L'Hôpital Universitaire La Pitié Salpêtrière-Friday, November 13, 2015
- Herman B. Leonard
- Emilie Billaud
- Arnold M. Howitt
- July 18, 2018
Arcos Dorados: How to Lead and From Where
- Krishna G. Palepu
- Gustavo A. Herrero
- February 21, 2014
Homeless World Cup: Social Entrepreneurship, Cause Marketing, and a Partnership with Nike
- George Foster
- Jocelyn Hornblower
- Norm O'Reilly
- June 04, 2010
NetApp: The Day-to-Day of a District Manager
- Mark Leslie
- James Lattin
- Patrick Arippol
- August 06, 2007
TecSalud's Response to COVID-19
- Maria Helena Jaen
- Ezequiel Reficco
- Jorge Alberto Ordonez
- December 15, 2023
Ransomware Attack at Colonial Pipeline Company
- Suraj Srinivasan
- March 02, 2023
Pella Corporation: Creating the Right Shareholder Roles and Goals for the Future
- Jennifer Pendergast
- Sachin Waikar
- April 19, 2023
NTT DOCOMO's Race to 5G
- Juan Alcacer
- Horst Melcher
- Akiko Kanno
- April 07, 2020
Regulatory independence in India: A case of Atomic Energy Regulatory Board
- K. V. Gopakumar
- M. P. Ram Mohan
- July 16, 2020
Francisco de Narvaez at Tia: Selling the Family Business
- Kristin C. Doughty
- October 15, 2000
La Esperanza: Beyond Automation
- Kety Lourdes Jauregui
- Maria Virginia Lasio
- April 16, 2021
Yorgus Yogurt and Zona Sul: Building a New Venture in an Established Family Business
- Justin B. Craig
- July 07, 2022
Medplus Ltd. (A), (B), (C)
- Neharika Vohra
- Chayanika Bhayana
- Harnain Arora
- Kashika Sud
- April 27, 2021
MIC Food: Shaping the Right Decision-Making Process for the Future
- December 09, 2022
Tapping into a Digital Brain: AI-Powered Talent Management at Infosys
- Mohanbir Sawhney
- Varun Poddar
- Charlotte Snyder
- July 14, 2022
Alibaba's Values Dilemma
- June 29, 2021

Effortless Action
- Greg McKeown
- June 08, 2023

Is Your Company Reading Data the Wrong Way?
- August 20, 2024
Popular Topics
Partner center.
Loading metrics
Open Access
Peer-reviewed
Research Article
Moderate confirmation bias enhances decision-making in groups of reinforcement-learning agents
Roles Conceptualization, Data curation, Formal analysis, Investigation, Methodology, Software, Validation, Visualization, Writing – original draft, Writing – review & editing
* E-mail: [email protected]
Affiliations Department of Biology, Humboldt Universität zu Berlin, Berlin, Germany, Charité – Universitätsmedizin Berlin, Einstein Center for Neurosciences Berlin, Berlin, Germany

Roles Conceptualization, Formal analysis, Methodology, Resources, Supervision, Writing – review & editing
Affiliations Transdisciplinary Research Area: Sustainable Futures, University of Bonn, Bonn, Germany, Center for Development Research (ZEF), University of Bonn, Bonn, Germany
Roles Conceptualization, Formal analysis, Methodology, Project administration, Resources, Supervision, Writing – review & editing
Affiliations Department of Biology, Humboldt Universität zu Berlin, Berlin, Germany, Science of Intelligence, Research Cluster of Excellence, Berlin, Germany
- Clémence Bergerot,
- Wolfram Barfuss,
- Pawel Romanczuk
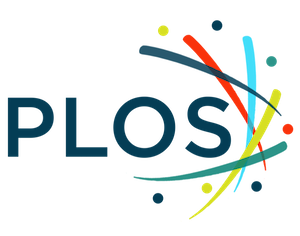
- Published: September 4, 2024
- https://doi.org/10.1371/journal.pcbi.1012404
- Peer Review
- Reader Comments
This is an uncorrected proof.
Humans tend to give more weight to information confirming their beliefs than to information that disconfirms them. Nevertheless, this apparent irrationality has been shown to improve individual decision-making under uncertainty. However, little is known about this bias’ impact on decision-making in a social context. Here, we investigate the conditions under which confirmation bias is beneficial or detrimental to decision-making under social influence. To do so, we develop a Collective Asymmetric Reinforcement Learning (CARL) model in which artificial agents observe others’ actions and rewards, and update this information asymmetrically. We use agent-based simulations to study how confirmation bias affects collective performance on a two-armed bandit task, and how resource scarcity, group size and bias strength modulate this effect. We find that a confirmation bias benefits group learning across a wide range of resource-scarcity conditions. Moreover, we discover that, past a critical bias strength, resource abundance favors the emergence of two different performance regimes, one of which is suboptimal. In addition, we find that this regime bifurcation comes with polarization in small groups of agents. Overall, our results suggest the existence of an optimal, moderate level of confirmation bias for decision-making in a social context.
Author summary
When we give more weight to information that confirms our existing beliefs, it typically has a negative impact on learning and decision-making. However, our study shows that a moderate confirmation bias can actually improve decision-making when multiple reinforcement learning agents learn together in a social context. This finding has important implications for policymakers who engage in fighting against societal polarization and the spreading of misinformation. It can also inspire the development of artificial, distributed learning algorithms. Based on our research, we recommend not directly targeting confirmation bias but instead focusing on its underlying factors, such as group size, individual incentives, and the interactions between bias and the environment (such as filter bubbles).
Citation: Bergerot C, Barfuss W, Romanczuk P (2024) Moderate confirmation bias enhances decision-making in groups of reinforcement-learning agents. PLoS Comput Biol 20(9): e1012404. https://doi.org/10.1371/journal.pcbi.1012404
Editor: Stefano Palminteri, Ecole Normale Superieure, FRANCE
Received: March 19, 2024; Accepted: August 9, 2024; Published: September 4, 2024
Copyright: © 2024 Bergerot et al. This is an open access article distributed under the terms of the Creative Commons Attribution License , which permits unrestricted use, distribution, and reproduction in any medium, provided the original author and source are credited.
Data Availability: All relevant data are on figshare, DOI: 10.6084/m9.figshare.24916767 This data can be accessed via the following link: https://figshare.com/articles/dataset/Moderate_confirmation_bias_enhances_collective_decision-making_in_reinforcement-learning_agents/24916767 Code can be found on the following GitHub repository: https://github.com/clembergerot/CARL .
Funding: This work was supported by funding under the form of a PhD scholarship by the Einstein Center for Neurosciences Berlin, Charité – Universitätsmedizin Berlin to CB and funding by the German Research Foundation under Germany’s Excellence Strategy EXC 2002/1 “Science of Intelligence” project 390523135 to PR. The funders had no role in study design, data collection and analysis, decision to publish, or preparation of the manuscript.
Competing interests: The authors have declared that no competing interests exist.
I. Introduction
Confirmation bias has been a topic of great interest among researchers across disciplines for several decades. Defined as “the tendency to acquire or process new information in a way that confirms one’s preconceptions and avoids contradiction with prior belief” [ 1 ], it is considered one of many ways human judgment and decision-making deviate from neoclassical rationality. As such, it has sparked interest within various disciplines, such as behavioral economics, experimental psychology, opinion dynamics, and political science. One of the reasons for such widespread engagement is its critical societal implications, from misinterpretation of evidence about vaccines [ 2 ], to racist behavior [ 3 ], to climate-change denial [ 4 ]. As a consequence, there is a tendency to assume that confirmation bias is harmful to both individual and social decision-making.
The learning rate represents the weight an agent gives to new information. In recent studies involving cognitive biases, researchers have modelled biased decision-making by using asymmetric learning rates—i.e., agents attribute different weights to different types of prediction errors [ 6 ]. In experimental psychology, one of the most widely used paradigms to assess human reinforcement learning is the two-armed bandit task, since it is a very simple equivalent to many ecological choice scenarios. In this task, participants undergo a series of trials. At each trial, they make a choice between two options and get feedback—e.g., they get a reward in the form of food or money, or a punishment such as less money, or less food. On average, one of the options is more rewarding than the other, and the participants’ goal is to learn the task’s statistics so as to maximize rewards. Behavior on a two-armed bandit task can be accounted for using simple RL models. In the past decade, studies using this paradigm have shed light on a “positivity bias”, whereby humans—as well as monkeys and mice—attribute more weight to positive than to negative prediction errors [ 7 – 10 ]. In humans, it appeared that this positivity bias was just a manifestation of a more complex confirmation bias: when they receive factual feedback—i.e., outcomes stemming from what an agent has chosen—, agents tend to give more weight to positive prediction errors; but, when it comes to counterfactual feedback—i.e., outcomes stemming from what an agent could have chosen , but has not—agents tend to dismiss positive prediction errors in favor of negative ones, which confirm that one was better off not choosing that option [ 11 , 12 ]. Finally, Chambon et al . (2020) demonstrated that, when human participants are forced to match a computer’s decisions—i.e., they are forced to select the option the computer indicates to them —, they show no bias; a confirmation bias arises, however, when participants make their own decisions. Commenting on this finding, the authors suggest such a “choice-confirmation bias” could have emerged in order to reinforce the actions that are the most likely to satisfy an agent’s needs—that is, free actions [ 13 ]. Besides this evidence, the potential adaptive value of positivity and confirmation biases has been stressed by several other authors. For instance, a few simulation studies suggest that, across a wide range of environments, virtual RL agents with a positivity bias [ 14 , 15 ] or with a confirmation bias [ 16 , 17 ] perform better than their counterparts. Therefore, confirmation bias seems to enhance individual decision-making rather than harm it.
Human and non-human animals, however, did not evolve in isolation. From hunting and gathering to purchasing products online, most of our decisions are made in a social context. To assess an object’s value, we consider information we get from our peers. Nonetheless, it remains unclear whether a confirmation bias benefits decision-making in a social context. In the field of opinion dynamics, which is concerned with opinions rather than decisions, researchers have hinted at such a bias’ effects at the collective level. In particular, confirmation has been linked to the emergence of problematic phenomena, such as echo chambers and polarization [ 18 , 19 ]. A recent opinion-dynamics study, on the other hand, claims that a moderate amount of confirmation bias improves group learning, whereas a “strong” confirmation bias harms group consensus and leads to polarization [ 20 ]. In opinion dynamics, however, confirmation can be modelled in many different ways, and studies still lack a unified theoretical setup [ 18 ]. By contrast, RL—and its extension to the collective domain, multi-agent RL (MARL)—provides researchers with a cohesive and empirically grounded framework. Yet, glancing at the MARL literature reveals diverse algorithms that account for outcome-valence sensitivity in a collective setting. Among such algorithms, Lenient Learning [ 21 ], Hysteretic Q-learning [ 22 ], Win-or-Learn-Fast Policy Hill-Climbing [ 23 ], and variants of Frequency Maximization Q-learning [ 24 , 25 ], have been shown to improve agents’ convergence and cooperation in coordination games. Another recent RL study demonstrated that confirmation bias caused polarization in a group of agents playing the equivalent of a two-armed bandit task [ 26 ]. In that study, however, the agents were not connected—i.e., they were not directly observing their peers’ actions and the resulting feedback. Yet, in our daily lives, we often base our decisions on the latter. For instance, if I want to get ice cream in one of two different shops standing next to each other, I may observe other customers’ actions; if a customer enters the first shop and exits with mediocre-looking ice cream, I will be more likely to choose the second shop. Therefore, understanding how confirmation bias impacts decision-making when agents are connected—i.e., when they can observe their peers’ actions and use this information to improve their own decisions—would constitute a significant step towards characterizing this bias in a more realistic setup. To the best of our knowledge, this issue has so far remained unaddressed in RL.
In this study, we hypothesize that the individual benefits of confirmation bias spill over to the collective level. We therefore expect that, in fully connected agent networks, confirmation bias has a positive impact on performance. As far as individual decision-making is concerned, Tarantola et al . suggest that such a bias improves performance as long as individuals do not dismiss counterfactual feedback [ 16 ]. In a collective setting, observing other agents’ actions and rewards is one such way of keeping an eye on unchosen options. Indeed, our peers provide us with data about environmental states we do not observe ourselves, hence giving us a clearer picture of alternative choices. Therefore, we also hypothesize that confirmation bias’ positive impact on performance will be amplified by the number of agents one is connected to.
To test our hypotheses, we build on previous results from experimental psychology and combine RL models with agent-based modelling. In particular, we assess the impact of a confirmation bias on RL agents’ collective performance in a two-armed bandit task. The agents learn to solve the task in a social context: modelled as nodes in a fully-connected graph, they observe the other agents’ actions and rewards. We seek to investigate how resource scarcity, group size, and bias strength modulate the confirmation bias’ effect on performance.
In line with our hypotheses, we show that a confirmation bias benefits group learning across a wide range of resource-scarcity conditions and that this positive effect gets stronger with group size. In abundant environments, however, a confirmation bias harms performance for small groups of agents, and this effect is amplified by bias strength. Investigating the mechanisms whereby this effect occurs, we discover that, past a critical bias strength, resource abundance favors the emergence of two different performance regimes, one of which is suboptimal. Moreover, we show that this bifurcation towards a high- and a low-performance regime comes along with polarization in small groups of agents. This leads us to uncover the existence of an optimal bias strength for each condition.
II. Results
A. confirmation bias enhances collective performance compared to other bias types.
Our first aim was to assess whether confirmation bias provides groups of different sizes with an advantage over unbiased collectives, and over collectives in which agents have a disconfirmation bias—i.e., in which agents attribute a higher learning rate to disconfirmatory vs. confirmatory information. To this end, we studied how agents’ performance on a two-armed bandit task—measured as average collected payoff per agent per trial—varies with bias type, group size, and resource scarcity. Thus, we simulated virtual agents performing a two-armed bandit task across various conditions, and observing every other agent’s actions and rewards according to a model that we designed, the Collective Asymmetric Reinforcement Learning (CARL) model (see Section IV A).
In this model, agents discriminate between confirmatory and disconfirmatory prediction errors. An instance of confirmatory prediction error is when an agent, i , chooses option A at trial t , and gets an outcome of +1. Since outcomes are either +1 or −1, and since an agent’s Q-values are bounded between −1 and 1, getting an outcome of +1 ( resp . −1) necessarily generates a positive ( resp . negative) prediction error. Another instance of confirmatory prediction error is when, at t , i sees another agent, j , get an outcome of −1 after choosing the alternative option, B . Conversely, a disconfirmatory prediction error can stem from agent i obtaining an outcome of −1 from the option it has chosen; or from seeing agent j obtain an outcome of +1 from the alternative option. In the CARL model, confirmatory and disconfirmatory prediction errors are not updated with the same learning rate. Typically, when agents have a confirmation bias, α + , the learning rate that updates confirmatory prediction errors, is higher than α − , the learning rate that updates disconfirmatory prediction errors.
Since information is always confirmatory or disconfirmatory with respect to a choice—i.e., independently from how much an agent values the chosen option —, the bias we study is technically a choice-confirmation bias. Throughout the paper, we will simply refer to it as “confirmation bias”, which is also consistent with previous usage of the term in experimental literature and simulation studies on the topic [ 9 , 16 , 17 ].
For each combination of conditions, we measured the collected payoff, which we averaged over agents, trials, and simulations. Then, we plotted it as a function of group size ( Fig 1A–1C ). In order to compare collective performance—i.e., performance that is averaged over all agents in the group—with individual performance—i.e., performance of one single agent within the group—, we also plotted mean collected payoff per trial for one arbitrarily-chosen agent (see S1A–S1C Fig ).
- PPT PowerPoint slide
- PNG larger image
- TIFF original image
https://doi.org/10.1371/journal.pcbi.1012404.g001
From Fig 1A–1C , one observes first that, across most scarcity and group-size conditions, confirmation-biased agents’ performance is significantly higher than unbiased agents’ and disconfirmation-biased agents’ performance ( p < 0.01, see confidence intervals). The exceptions are n < 4 agents in rich environments and solitary agents ( n = 1) in mixed environments. This means that, in most conditions, a confirmation bias allows agents to collect more rewards on average, thus conferring an advantage over other bias types. In addition, disconfirmation-biased agents perform almost always worse than unbiased agents, suggesting that a disconfirmation bias harms decision-making in general.
Second, in all resource-scarcity conditions, confirmation-biased agents’ performance increases with group size. In a rich environment, in particular, increasing group size cancels the advantage small groups—i.e., 1 or 2 agents—of unbiased agents have over small groups of confirmation-biased agents. This suggests that confirmation-biased agents benefit from receiving information from a larger number of neighbors. In comparison, unbiased agents’ performance seems to be always unaffected by group size. As for disconfirmation-biased agents, increasing group size is detrimental to performance in rich environments but does not seem to have a significant effect in other resource-scarcity conditions.
Plots of individual performance (see S1A–S1C Fig ) exhibit the same patterns, indicating that bias type, resource scarcity, and group size have a similar impact on every single agent in the group.
B. Stronger confirmation bias impedes decision-making in a rich environment
In Fig 1D–1F , we plotted collected payoff—averaged over agents, trials, and simulations—as a function of group size. In order to compare collective performance with individual performance, we also plotted the average collected payoff per trial for one arbitrarily-chosen agent, as a function of group size (see S1D–S1F Fig ).
From Fig 1D–1F , it appears that, in all bias-strength conditions, increasing group size does not have the same effect on confirmatory vs. unbiased vs. disconfirmatory agents. Indeed, while confirmatory agents’ performance increases with group size, disconfirmatory agents’ performance decreases. Unbiased agents’ performance, in comparison, does not seem to be affected by an increase in group size. This suggests that, across all bias-strength conditions, confirmatory agents benefit from being in a bigger group, whereas such an increase in group size is detrimental to disconfirmatory agents. In addition, disconfirmatory agents perform almost always worse than unbiased agents, suggesting that a disconfirmation bias harms decision-making whatever its strength.
Finally, we compared the intersection points between the blue curve and the yellow curve for different bias strengths. In all bias-strength conditions, indeed, smaller groups of confirmatory agents perform worse than their unbiased peers. When group size increases, confirmatory agents increase their payoffs, until they outperform unbiased agents. However, the group size at which they take the lead depends on bias strength: with a weak bias, the intersection point between the blue and yellow curves lies at group size 2 ( Fig 1D ); with a medium bias, at size 3 ( Fig 1 ); and, with a strong bias, between sizes 4 and 6 ( Fig 1F ). This means that, the higher the bias strength, the higher the required group size for confirmation-biased agents to outperform unbiased agents. Therefore, although increasing bias strength has a detrimental impact on confirmatory agents, increasing group size seems to counterbalance this negative effect on performance. This shows that, in a rich environment, a strong confirmation bias impedes decision-making without harming it completely: its negative impact is not robust against an increase in group size. All in all, this suggests that larger groups can tolerate stronger biases.
Plots of performance for one agent within the group (see S1D–S1F Fig ) exhibit the same patterns, indicating that bias strength, resource scarcity and group size have a similar impact on every single agent in the group.
C. Varying bias strength gives rise to bimodality in Q-value-gap distributions
In the previous section, we showed that confirmation bias impedes performance in a rich environment. As a consequence, we sought to understand how such an effect arises. A previous simulation study showed that confirmation bias enhances individual performance by increasing the gap between the Q-values an agent attributes to both options [ 17 ]. Glancing at the softmax formula, one easily understands that, as long as the best option’s Q-value is kept higher than the worst option’s, increasing their difference leads to a higher probability of choosing the best option, hence counteracting the effect of noise. Thus, Q-value gap is directly related to performance. In this section, we study the effect of bias strength on Q-value gap distributions, in order to determine whether the confirmation bias’ impeding effect can be explained by a decrease in Q-value gap.
In Fig 2A–2C , we plotted one agent’s final-Q-value-gap (Δ Q f ) distributions over 1000 simulations, and compared these distributions across group sizes and bias types. A first striking observation is that bias strength seems to have an impact on the distributions’ shape. Indeed, when n = 2 (blue), Q-value-gap distribution goes from unimodal (unbiased agents, Fig 2A ), to weakly bimodal (weakly confirmatory agents, Fig 2B ), to strongly bimodal ( Fig 2C ). This means that, when agents are unbiased, performance is rather homogeneous across simulations. When they are equipped with a confirmation bias, however, two different regimes of performance emerge: in some simulations (leftmost blue peak), weakly biased agents perform rather poorly, whereas in others (rightmost blue peak), they perform quite well. As for strongly biased agents, the separation between the two performance regimes is quite extreme: in some simulations (leftmost blue peak), agents perform very poorly—negative Q-value gaps indicate that, in a non-negligible fraction of the simulations, they even tend to choose the worst option more often than the best—whereas in others (rightmost blue peak), they perform very well.
A-C: Q-value-gap distributions for one agent in a group with no bias (A), with a weak confirmation bias b = 3 (B), with a strong confirmation bias b = 9 (C). D-F: Behavior-profile distributions for one agent with no bias (D), with a weak confirmation bias b = 3 (E), with a strong confirmation bias b = 9 (F); inserted plots show the same distributions, but with the y -axis in log-scale. Blue distributions: n = 5; pink distributions: n = 2. For each plot, number of bins was set to 50. For each combination of conditions, N simu = 1000 simulations were run. Within each simulation, agents perform the task during N trials = 1000 trials. This high number of trials has been chosen to get a precise estimate of Q-value gaps after convergence. Q-value gap was measured at each simulation’s very last trial, and for one arbitrarily-chosen agent in the group (i.e., “agent 0” in the simulations). Plotted distributions were obtained for that agent over the 1000 simulations. Behavior-profile distributions were obtained by applying the softmax function to previous Q-value data.
https://doi.org/10.1371/journal.pcbi.1012404.g002
But Fig 2A–2C also suggests that, when n = 5 (pink distributions), bimodality tends to disappear. Thus, Fig 2B shows a single peak—i.e., a single performance regime—for weakly biased agents. For strongly biased agents ( Fig 2C ), on the other hand, bimodality remains, but most of the distribution’s weight lies on higher values of Δ Q f , suggesting that simulations resulting in low performance have only a marginal effect on mean collected payoffs. This means that, with increasing group size, confirmatory agents are less likely to end up with a small or negative Q-value gap, thus exhibiting high performance most of the time. These results echo the impeding effect found in Section II B.
Nonetheless, studying bias strength’s impact on Δ Q f -distributions does not, on its own, explain the bias’ impeding effect. To convince oneself, one only needs to look at the blue distributions’ means: when a group of two is equipped with a strong confirmation bias ( Fig 2C ), the distribution’s mean is highest despite a non-negligible fraction of simulations that end with very poor performance. This is not in line with our results from the previous section, which demonstrated that, on average, groups of two unbiased agents collect more rewards than their strongly biased counterparts. An explanation can be found in the softmax function, which relates Q-value gap Δ Q to policy or “behavior profile” X [ 27 ]. Indeed, this function is bounded and non-linear. Therefore, if the mean of a given Δ Q f -distribution 1 is higher than the mean of another Δ Q f -distribution 2, it does not entail that the mean of the corresponding X f -distribution 1 is higher than the mean of the other corresponding X f -distribution 2. When one applies the softmax function to each data point in our Δ Q f distributions, one obtains X f distributions ( Fig 2D–2F )—i.e., distributions of an agent’s final behavior profiles over 1000 simulations. Although bimodality is less visible on these graphs, it still exists, and the low-performance regime’s negative contribution to mean performance becomes very clear in the two-agent case: mean behavior profile equals 0.69 ( Fig 2F ), which is noticeably lower than 0.85, the mean behavior profile for unbiased agents ( Fig 2D ). Therefore, bimodality in Δ Q f distributions translates into lower mean probabilities of choosing the best option, which, in turn, translates into lower mean performance.
Finally, Fig 2D–2F shows that, in a rich environment, a weak confirmation bias seems very beneficial to groups of five agents. Indeed, mean behavior profile is 0.95 ( Fig 2E ), significantly higher than unbiased ( Fig 2D ) and strongly biased ( Fig 2F ) agents’ mean behavior profiles (0.84 and 0.87, respectively). This suggests the existence of an optimal, group-size dependent, bias strength.
D. Varying bias strength gives rise to different regimes of performance and polarization
In the previous section, we showed that, in a rich environment, confirmation bias gives rise to bimodality in the agents’ final-Q-value gap distributions, and that increasing group size attenuates this bimodality. Nonetheless, using only three bias-strength conditions gives a partial picture of the performance regimes, and one is left to wonder at which bias strength, exactly, the two regimes emerge. To address this, we visualize Q-value gap distributions as a function of bias strength, where bias strength slides from b = 1 to b = 5. Thus, b = 1 corresponds to no bias, whereas b > 1 corresponds to different confirmation-bias strengths. In all bias-strength conditions, α − = 0.1, and α + = b ⋅ α − . Moreover, we studied the impact of group size and resource scarcity on the distributions. To this end, we used the first experiment’s poor, mixed, and rich environments, as well as the previous experiment’s group sizes—i.e., groups of 2 and 5 agents.
Since bimodality can be easily detected by looking at the smoothed distributions’ maxima, we decided to plot these maxima across bias strengths. For this purpose, we first computed the distributions’ kernel density estimators (KDEs), and located their plots’ maxima automatically. In Fig 3A–3C , the number of points corresponding to a given bias strength is the number of maxima that the Δ Q f distribution exhibits for this bias strength.
A-C: Local maxima of Q-value-gap distributions’ KDE plots for one agent in a group, as a function of bias strength, group size, and resource scarcity, at the end of a sequence of 1000 trials. Blue plot: 2 agents; Pink plot: 5 agents. Transparency denotes peak height divided by the highest peak’s height in the plot. For each combination of conditions, N simu = 1000 simulations were run. In each simulation, agents perform the task during N trials = 1000 trials, so as to obtain precise estimates of Q-value gaps after convergence. Q-value gap was measured at the very last trial, focusing on one agent within the group (i.e., the agent that was labelled “agent 0” in the simulations). For each combination of conditions, a kernel density estimator (KDE) plot of the Q-value-gap distribution was computed. From these KDE plots, local maxima’s coordinates were automatically extracted. Finally, for each group size and resource-scarcity condition, the peaks’ x -coordinates (which correspond to the Q-value gaps for which the distribution is at a local maximum) were plotted across bias strengths. To distinguish between high and low peaks, transparency was increased with decreasing peak height. D-F: Mean behavior profile for one agent in a group, as a function of bias strength, group size, and resource scarcity, at the end of a sequence of 1000 trials. Blue plot: 2 agents; Pink plot: 5 agents. To obtain these plots, we converted each data point from the previous Q-value-gap distributions to a behavior profile via the softmax formula. We then computed the mean of every subsequent behavior-profile distribution. Mean behavior profile is strongly correlated with performance and performance improvement (see Fig 3G-I). G-I: Performance improvement per agent per trial, as a function of bias strength, group size, and resource scarcity, during the last 100 trials of a sequence of 200 trials. Dashed lines indicate how to relate G-I with D-F: performance improvement is strongly correlated with mean behavior profile for 2 agents (blue) and 5 agents (pink). For each combination of conditions, N simu = 500 simulations were run. In each simulation, agents perform the task during N trials = 200 trials. Performance improvement denotes the difference between actual performance and expected performance when agents follow a random policy (see Section IV B).
https://doi.org/10.1371/journal.pcbi.1012404.g003
By comparing Fig 3 ’s three different plots, one immediately observes that, contrary to the mixed and rich conditions, the poor condition does not cause bimodality to emerge in the distributions ( Fig 3A ). Rather, for both groups of 2 and 5 agents, Δ Q f distributions exhibit one maximum, which value increases with bias strength. Moreover, this maximum is always positive and higher than the difference between both options’ expected values—i.e., 0.4. This means that a poor environment is always conducive to a single, high-performance regime, whatever the bias strength and the group size. Moreover, this suggests that, in a poor environment, performance increases with bias strength, although, to be sure, one would need to convert the Δ Q f distributions to X f distributions and compute their means.
Turning to Fig 3A and 3C , one notices the emergence of bimodality past a certain bias strength. As can be seen by comparing 2 and 5-agent plots, bimodality is more pronounced for groups of 2. Interestingly, resource scarcity seems to have an impact on the maxima’s locations: while in a mixed environment, negative maximum locations arise past b = 3, a rich environment gives rise to maximum locations that approach 0 but do not become negative. This means that, although both resource-scarcity conditions lead, past a certain bias strength, to the emergence of low-performance regimes, the latter are quite different. In the mixed condition, indeed, negative values indicate that agents favor the worst option, hence making “wrong” choices most of the time; in the rich condition, on the other hand, values close to zero suggest agents tend to favor the best and the worst option almost equally, hence following a nearly random policy. The 2-agent plots, Fig 3B and 3C , confirm our previous observation that increasing group size tends to attenuate bimodality, as points located in the low-performance regime are much more transparent than for groups of 2 agents—which signals lower maximum values. This is most striking in the rich condition ( Fig 3C ), which, for 5 agents, gives rise to a strong bias towards the high-performance regime. For 2 agents, in comparison, the low-performance regime’s maxima are higher, indicating that a greater fraction of simulations end with poor performance. This result echoes our previous findings, which demonstrate that, in a rich environment, adding agents to the group makes a noticeably positive difference to collective performance. Finally, we plotted the full final-Q-value-gap distributions for both group sizes as heatmaps (see S2 Fig ). These show the same patterns and bifurcations, confirming the emergence of distinct high- and low-performance regimes.
From Δ Q f distributions, we can compute mean Q-value gap across bias strengths, and look at its local optima. Similarly, we can convert Δ Q f distributions to X distributions, compute and analyze the mean behavior profile versus bias strength. S3 Fig shows mean Q-value gap as a function of bias strength in each resource-scarcity condition, for 2 and 5 agents. The corresponding mean behavior profiles are shown in Fig 3D–3F . Interestingly, the mean behavior profiles demonstrate the existence of an optimal bias, which maximizes performance for both group sizes. Although slightly different, these optimal values are located at a moderate level of bias strength, between b = 2 and b = 3. Past these optima, in mixed and rich environments, performance keeps decreasing. In a poor environment, on the other hand, performance increases with bias strength for both group sizes, although it seems to reach a plateau eventually ( Fig 3D–3F ). This is correlated with the absence of a low-performance regime in the poor environment.
Fig 3G–3I shows mean performance improvement—defined as the difference between actual performance and expected performance for a random policy (see Section IV B)—as a function of bias strength and group size for groups of 1 to 10 agents. As such, it summarizes our results well: performance improvement is highest for intermediate levels of bias strength; in mixed and rich environments, performance decreases dramatically for small groups past a certain bias strength (whereas, in poor environments, performance improvement is more robust across bias strengths and group sizes); in general, larger groups tolerate stronger biases.
We demonstrated that, under specific circumstances, CARL’s learning dynamics cause individual performance to split into two different regimes. However, the question remains whether a split occurs within the collective as well: during the same run, do agents converge towards different regimes, or towards the same one? In other terms, do agents exhibit polarization or consensus? To answer this question, we visualized the distribution of differences between agents’ final Q-value gaps. For every group-size and resource-scarcity conditions, we plotted the distributions of differences between a focal agent’s and the other agents’ final Q-value gaps across 1000 simulations, as a function of bias strength. Interestingly, we found that, in a group of 2 agents, mixed and rich environments always give rise, past a certain bias strength, to polarization in the final Q-value gaps ( Fig 4A ). Moreover, the higher the bias strength, the more polarized the agents. In poor environments, on the other hand, agents remain in a consensual state for all bias strengths ( Fig 4A ). Fig 4B suggests that adding agents to the group tends to reestablish consensus: in mixed and rich environments, most simulations end with the first and the second agents converging towards the same Q-value gaps, although a few simulations do end, past a certain bias strength, in a polarized state. Comparing any agent with the focal one gives rise to a similar pattern (see S4 Fig ). Such polarization may explain small groups’ poor performance in abundant environments, and the decrease in performance as bias strength increases. It also suggests that, by reestablishing consensus, larger groups improve their performance on the task.
A: In a group of 2 agents; B: In a group of 5 agents.
https://doi.org/10.1371/journal.pcbi.1012404.g004
To understand why polarization emerged in groups of two agents, we devised a deterministic version of our CARL model (see Section IV A4), and simulated this model over 500 trials in poor, mixed, and rich environments, with various initial conditions (see S5 Fig ). This model allowed us to visualize deterministic trajectories of both agents’ Q-value gaps. Interestingly, S5 Fig shows that, in mixed and rich environments, consensus is a fixed point of the system ( S5C and S5E Fig ). However, it is likely an unstable one: when agents do not start with strictly identical Q-values, their final Q-value gaps diverge ( S5D and S5F Fig ). In a poor environment, by contrast, slightly different initial conditions do not lead final Q-values to dramatically diverge. In our agent-based simulations, thus, groups of two agents never converge, in mixed and rich environments, towards consensus, because of noise: although agents start with strictly identical Q-values, stochasticity induced by the task or by their policies makes them deviate from the unstable fixed point, so that they inevitably converge towards the stable, polarized state. Although we did not develop a deterministic model for groups of five agents—as adding agents would complexify the equations —, our agent-based simulations with 5 agents suggest that increasing group size eventually reestablishes consensus as a stable state.
Finally, additional simulations with higher temperature—i.e., lower values of β —were carried out to study whether polarization would emerge when agents are more exploratory (see S1 Text ). We found out that, under a critical value of β —which differs depending on resource scarcity—polarization disappears. This is because, when agents are more exploratory, they get more opportunities to gather confirmatory information from alternative options. This prevents them from getting trapped in incorrect attractors. Interestingly, these additional results show that, although polarization disappears for lower values of β , small groups of confirmatory agents still exhibit poorer performance than their unbiased counterparts in a rich environment. Further analysis (see S1 Text ) reveals that small groups behave like optimistic agents: they inflate both options’ Q-values. A previous study [ 14 ] demonstrated that such optimism is beneficial in poor environments but not in abundant ones, as Q-values’ inflation saturates when approaching 1. For this reason, small groups of confirmatory agents end up with smaller Q-value gaps in rich environments, which negatively impacts their performance. In larger groups, confirmatory agents still inflate the best option’s Q-values, but the sampling of the alternative option by another agent is more likely. Therefore, agents get more opportunities to deflate the worst option’s Q-value, which results in a larger Q-value gap, and thus in higher performance. A detailed explanation of these effects is provided in S1 Text .
III. Discussion
In the past years, researchers have witnessed a surge of interest in the confirmation bias at the level of individual decision-making. Evidence has shown that humans exhibit such a bias, and that it could improve performance in a wide range of environments. Nevertheless, confirmation bias, as framed in a reinforcement learning framework, has not yet been thoroughly investigated at the collective level. Yet, most of our decisions are based on information we collect from our peers, which makes it crucial to understand how cognitive biases influence decisions made in a social context. Our study constitutes a step in that direction by exploring the impact of such a bias on decision-making under social influence in a simple choice task. To this end, we used agent-based simulations, and varied bias type, bias strength, group size, and resource scarcity. We first asked what would be the impact of a confirmation bias on performance, compared to no bias or a “disconfirmation” bias. Then, we sought to understand bias strength’s effect on performance, and how this effect is modulated by group size and resource scarcity. This led us to investigate how bias strength impacts final Q-value gaps, and how it can lead, under certain conditions, to polarization within the group.
Overall, we find that groups with a confirmation bias exhibit higher performance than unbiased groups, and than groups with a disconfirmation bias. Such higher performance is found across most resource conditions and group sizes. In abundant environments, however, a confirmation bias can be detrimental to smaller groups. The stronger the bias, the higher the required group size to compensate for this disadvantage. In other terms, larger groups can tolerate stronger confirmation biases. Finally, we find that a confirmation bias gives rise to a bifurcation between regimes of high and low performance, which may explain lower collected payoffs in abundant environments. This points towards an optimal level of confirmation bias for decision-making in a social context. In mixed and rich environments, we discovered that individual agents can end up in one of two different performance regimes past a certain bias strength. In groups of 2 agents, this bifurcation comes with a polarized state, whereby each agent performs in a different regime. This means that, in these conditions, overall mediocre performance is underlain by polarization between a high-performing agent and a poorly-performing agent.
In a nutshell, our results suggest that a confirmation bias improves learning of action-outcome contingencies, and that this improvement is robust across resource scarcities. Performance robustness in larger groups of agents, in particular, hints at the confirmation bias’ possible adaptive value in a social context.
Despite poor performance for small groups in mixed and rich environments, groups with a confirmation bias exhibit overall higher performance than unbiased and disconfirmatory groups. This suggests that a confirmation bias can be advantageous to decision-makers in a social context, and that this advantage is robust across various resource scarcities and group sizes. These results are in line with our initial prediction that a confirmation bias can boost collective performance on a two-armed bandit task in a social context. Such an improvement in performance, across various scarcity conditions, has been demonstrated at the individual level [ 17 ]. Our results extend these previous findings to the collective level.
As previously mentioned, there are exceptions to the confirmation bias’s advantage over disconfirmation or no bias: in abundant environments, confirmation can be detrimental to smaller groups. The stronger the bias, the higher the required group size to compensate for this disadvantage. These results suggest that, in a social context, agents need to accumulate more information in order to compensate for a strong confirmation bias’ negative effect. Accumulating more information is done by gathering and averaging data from more individuals—i.e., increasing group size. This also points to the idea that a confirmation bias is not advantageous per se : if it is too strong, it is actually detrimental to performance. Therefore, at the collective level, there are some contexts in which a confirmation bias can impede efficient decision-making. In our model, the single-agent case reduces to a simple positivity bias: the agent updates positive prediction errors with a higher learning rate than it does negative prediction errors. In short, there are no other agents to feed it counterfactual information. Cazé & van der Meer had previously shown that a positivity bias leads to poorer performance for a single agent in a rich environment [ 14 ]. But it was not obvious that a confirmation bias would also lead to poorer performance in groups of two or three agents. Similar results, however, have been obtained by Gabriel & O’Connor with an opinion-dynamics model, and independently from resource scarcity [ 20 ]. The authors implemented a “strong confirmation bias”, and showed that, for small group sizes, strongest biases harm correct consensus. For larger group sizes, however, bias strength does not seem to make a difference.
Finally, we found that a confirmation bias gives rise to specific learning dynamics which, in particular, may explain lower performance in abundant environments, and which point towards an optimal level of confirmation bias for decision-making in a social context. Past a certain bias strength, bifurcations in final Q-value gaps emerge in mixed and rich environments, hinting at two different performance regimes. Further investigation revealed that this bifurcation came with the emergence of polarization within groups of 2 agents. Such polarization between high- and low-performing individuals within the group constitutes a mechanism whereby collective performance declines. However, supplementary analyses revealed that polarization was not the only causal factor for such performance drop. Indeed, small groups of confirmatory agents tend to behave like optimistic learners, yet optimism is only beneficial in environments where positive outcomes are rare [ 14 ]. When more agents are added to the group, the odds that a peer chooses the alternative option are higher; thus, agents get more opportunities to deflate the worst option’s Q-value, and they end up with larger Q-value gaps.
It has been suggested that reduced sampling of previously punishing options, called “hot stove effect” [ 28 ], could be corrected by interdependent sampling processes [ 29 ] or conformist social learning [ 30 ]. Although this is reminiscent of the aforementioned results, there are two main differences between the hot stove effect and the drop in performance we underline. Indeed, the effects we observe in small groups of confirmatory agents are not due to reduced sampling per se , but to reduced counterfactual sampling. This reduced counterfactual sampling itself does not stem from previous punishments but, on the contrary, from a lack of punishment combined with a lack of sampling neighbors. Nonetheless, just like the cited studies about the hot stove effect, our model also makes use of a minimalist form of social influence to counteract sampling-induced errors. Together, these works allow further understanding of various kinds of sampling-induced errors, and of the way social learning helps correct them.
In the individual case, Lefebvre et al . already showed that a confirmation bias can lead learning dynamics towards an incorrect attractor [ 17 ]. They give an expression for a “critical bias”, above which an agent’s trajectory can end up in a correct or an incorrect attractor depending on its history. Our study suggests there is a similar mechanism at play with CARL, at least in fully-connected networks. In the social case, similar results have been obtained by Gabriel & O’Connor with an opinion-dynamics model [ 20 ], and independently from resource scarcity. The authors argue that a moderate confirmation bias actually serves group learning, and could have developed in the social environment in which humans evolved. In particular, they cite Mercier & Sperber (2017), who hold a similar view: according to them, confirmation bias leads disagreeing members of a group to stick to their respective positions, hence encouraging them to develop good arguments in favor of their preferred views, and thereby making final consensus better informed [ 31 ]. Our results suggest that a confirmation bias indeed benefits decision-making, but can be harmful above certain values. This echoes Lefebvre et al .’s conclusions at the individual level [ 17 ]. Designing an experimental version of our task and determining whether participants exhibit a moderate confirmation bias would be an interesting endeavor for future research.
A. Limitations of the present study
One limitation of our study is that we consider a restricted set of environments; many other settings are left to be investigated. For instance, the question remains whether a confirmation bias improves collective performance on any multi-armed bandit task, as for example, two-armed bandit tasks with any contingencies or bandits with more than two arms. Agents in our study are identical and confronted with the same individual task. Situations where heterogeneous agents interact with each other, and actions directly impact the outcome for others, such as in social dilemmas, present exciting and relevant cases for future research. For example, extending previous work investigating the conditions under which RL agents learn to cooperate in social dilemmas [ 32 ] is a concrete next step. Moreover, the environments we used in this study are all static; yet, in the everyday world, we make decisions in fluctuating circumstances. Since confirmation bias tends to make agents more conservative—by increasing Q-value gap and, hence, probability of choosing one’s favored option—, one may expect that such a bias puts collectives at a disadvantage in fluctuating environments. This question could be an engaging avenue for future research.
Another limitation pertains to the way our agents gather information. First, it is assumed that each agent can observe every other agent’s actions and rewards; this is unrealistic in large groups, as visual attention has been shown to have limited capacity [ 33 ]. The framework of partial observability could be used to model explicit environmental and perceptual uncertainty [ 34 ]. Second, our agents choose options and update estimates in a synchronic fashion; this is a simplification, as humans tend to accumulate evidence over time [ 35 ], and groups exhibit complex decision-making dynamics whereby individual decision timing depends on confidence [ 36 ], and selfishness [ 37 ]. Therefore, determining whether our findings hold for more realistic network structures—i.e., network structures that take into account the dynamics of attention—would be an exciting question to answer next. Third, Chambon et al . suggested that humans exhibit a confirmation bias when they are free to choose, but not when a computer makes choices for them [ 13 ]. In our setup, agents do decide freely, and their peers’ choices provide them with counterfactual feedback. In nature, however, animals often gather information through an observational-learning phase before making a decision. It is unclear whether a confirmation bias would apply during purely observational learning—i.e., when the focal agent refrains from making a choice while it learns from the others’ actions. This question should be answered experimentally. However, we believe our model could be very useful in formulating predictions regarding this question.
Finally, one should keep in mind that, in observed human behavior, confirmation bias could interact, or be confused, with other types of biases. For instance, Sugawara and Katahira showed that previous experimental results involving asymmetric learning could be explained, in fact, by a perseverance bias, or by a mix of perseverance and asymmetric learning [ 38 ]. In our study, we do not determine whether the performance curves are a characteristic signature of confirmation bias, or could be obtained with, e.g., perseverance. Designing a model that could help discriminate between perseverance bias and asymmetric learning, such as Sugawara and Katahira’s Hybrid model but at the collective level, would be useful to future experimental work.
B. Conclusion
By shedding light on the mechanisms underlying learning dynamics in collectives where agents exhibit a confirmation bias, our study suggests such a bias could have been adaptive in the social context in which humans evolved. Therefore, rather than being a sign of irrationality, human agents’ tendency to seek confirmatory information may exist for a good reason. In an era that many have qualified as “post-truth”, this realization could have important consequences. In particular, targeting problematic social phenomena, such as misinformation and polarization, may require that we focus less on confirmation bias per se , but rather emphasize other features, such as bias strength, network structure or inter-individual influence. Such a task calls for a complex methodological individualism [ 39 ], which considers how individuals change and are changed by groups through social interactions. As technology has multiplied the number and range of such interactions, placing our scientific endeavors at the interface between the individual and society may prove crucial to tackling contemporary challenges.
IV. Methods
A. collective asymmetric reinforcement learning model.
Here, we introduce the Collective Asymmetric Reinforcement Learning (CARL) model, which describes reinforcement learning dynamics in a collective of virtual agents performing a two-armed bandit task. In this model, agents are equipped with two different learning rates, and it is assumed that both bandits return a payoff of either +1 (“reward”) or −1 (“penalty”). In particular, the first bandit returns a reward with probability p 1 , and the second bandit, with probability p 2 . Therefore, the expected payoff for the different bandits j is 2 p j − 1, with j = 1, 2.
Within the collective, each agent tries to maximize its payoff using a Q-learning algorithm without discounting and different types of information: information resulting from its own actions, and information stemming from the other agents’ actions. In addition, the algorithm distinguishes between confirmatory and disconfirmatory information, which we define below.
1. Information types.
For a given agent i , confirmatory information is information which confirms that agent i ’s chosen bandit at trial t was the “right” one (that is, the most rewarding one). Disconfirmatory information, on the other hand, suggests that agent i ’s chosen bandit at trial t was not the right one. Therefore, at trial t , agent i considers the following information as confirmatory:
Similarly, at trial t , agent i considers the following information as disconfirmatory:
All agents update information they consider confirmatory with a learning rate α + , and information they consider disconfirmatory, with a learning rate α − . When α + > α − , agents are said to have a confirmation bias. Similarly, one may define a “disconfirmation bias” when α + < α − . The case α + = α − will be referred to as “unbiased”.
2. Update rule.
At every trial t ∈ [1, T ], all agents update the Q-value they associate with each bandit. In the next paragraphs, the following notation will be used:
3. Softmax policy.
4. Deterministic model.
To obtain the general expected update (that is, whether option a is chosen or unchosen) of option a ’s Q-value, one just needs to multiply δ c ( t ) ( resp . δ u ( t )) by the probability that option a is chosen ( resp . unchosen) by agent i .
B. Simulations
Throughout the study, we investigated confirmation bias’ effect on performance in various conditions using agent-based simulations. Within these, agents perform a static two-armed bandit task for a fixed number of trials. Agents find themselves in groups of different sizes, and can observe every other agent’s actions and rewards—i.e., agents are modelled as nodes on a fully-connected graph. At each trial t , each agent i chooses an arm a according to a softmax policy (see Section IV A 3) with parameter β .
Throughout the study, all agents’ inverse temperatures are set to β = 4. After agents make a choice, they receive a reward (+1) or a penalty (−1), which occurs according to the arms’ reward probabilities. Following this payoff collection, agents update their respective Q-values according to the CARL model (see Section IV A 2). Afterwards, a new trial begins, and this sequence goes on until the final trial.
In most of our simulations, we investigated three types of environments, defined by the two options’ reward probabilities. In a poor environment, obtaining an outcome of +1 is overall rare: the first arm’s reward probability is p 1 = 0.3, and the second arm’s, p 2 = 0.1. In a rich environment, the probability of getting a reward is overall high: p 1 = 0.9, and p 2 = 0.7. In a mixed environment, the probability to get a reward is overall neither low, nor high: p 1 = 0.6, and p 2 = 0.4.
We define performance as mean collected payoff per agent per trial (averaged over simulations). Performance improvement denotes the difference between actual performance, and expected performance when agents follow a random policy—i.e., their probability of choosing one or the other option is 0.5. This expected performance is: −0.6 in a poor environment; 0 in a mixed environment; 0.6 in a rich environment.
Supporting information
S1 text. effect of higher temperature (lower β )..
https://doi.org/10.1371/journal.pcbi.1012404.s001
S1 Fig. Mean collected payoff per trial for one agent in the group.
https://doi.org/10.1371/journal.pcbi.1012404.s002
S2 Fig. Distributions of final Q-value gaps for one agent across 1000 simulations, as a function of bias strength.
https://doi.org/10.1371/journal.pcbi.1012404.s003
S3 Fig. Mean final Q-value gaps for one agent across 1000 simulations, as a function of bias strength.
https://doi.org/10.1371/journal.pcbi.1012404.s004
S4 Fig. Distributions of differences between agent 0’s and other agents’ Q-value gaps in a group of 5 agents, as a function of bias strength.
https://doi.org/10.1371/journal.pcbi.1012404.s005
S5 Fig. Agents’ Q-value gaps over time according to the deterministic model.
https://doi.org/10.1371/journal.pcbi.1012404.s006
S6 Fig. Final polarization as a function of inverse temperature.
https://doi.org/10.1371/journal.pcbi.1012404.s007
S7 Fig. Performance in all environments using different inverse temperatures β .
https://doi.org/10.1371/journal.pcbi.1012404.s008
S8 Fig. Difference between confirmatory and unbiased agents’ final Q-value gaps in various conditions.
https://doi.org/10.1371/journal.pcbi.1012404.s009
S9 Fig. Evolution of Q-values over time in rich and poor environments, with β = 1.
https://doi.org/10.1371/journal.pcbi.1012404.s010
Acknowledgments
Part of the abstract was generated with Writefull: https://x.writefull.com/abstract-generator .
- View Article
- PubMed/NCBI
- Google Scholar
- 5. Sutton R. S., & Barto A. G. (2018). Reinforcement learning: an introduction. Second Edition. MIT Press, Cambridge, MA.
- 21. Panait, L., Sullivan, K., & Luke, S. (2006, May). Lenient learners in cooperative multiagent systems. In Proceedings of the fifth international joint conference on Autonomous agents and multiagent systems (pp. 801-803).
- 22. Matignon, L., Laurent, G. J., & Le Fort-Piat, N. (2007, October). Hysteretic q-learning: an algorithm for decentralized reinforcement learning in cooperative multi-agent teams. In 2007 IEEE/RSJ International Conference on Intelligent Robots and Systems (pp. 64-69). IEEE.
- 24. Kapetanakis, S., & Kudenko, D. (2002, April). Improving on the reinforcement learning of coordination in cooperative multi-agent systems. In Proceedings of the Second Symposium on Adaptive Agents and Multi-agent Systems (AISB02).
- 25. Matignon, L., Laurent, G. J., & Le Fort-Piat, N. (2008, May). A study of FMQ heuristic in cooperative multi-agent games. In The 7th International Conference on Autonomous Agents and Multiagent Systems. Workshop 10: Multi-Agent Sequential Decision Making in Uncertain Multi-Agent Domains, aamas’ 08. (Vol. 1, pp. 77-91).
- 31. Mercier H., & Sperber D. (Eds.). (2017). The enigma of reason. Harvard University Press.
Enhancing Agricultural Productivity: A Machine Learning Approach to Crop Recommendations
- Research Article
- Open access
- Published: 02 September 2024
Cite this article
You have full access to this open access article
- Farida Siddiqi Prity 1 , 2 ,
- MD. Mehadi Hasan 1 ,
- Shakhawat Hossain Saif 1 ,
- Md. Maruf Hossain 1 , 3 ,
- Sazzad Hossain Bhuiyan 1 ,
- Md. Ariful Islam 1 , 4 &
- Md Tousif Hasan Lavlu 1
Agriculture constitutes the foundational pillar of the global economy, engaging a substantial segment of the workforce and making a considerable contribution to the Gross Domestic Product (GDP). However, agricultural productivity faces numerous challenges, including varying climatic conditions, soil types, and limited access to modern farming practices. Developing intelligent agricultural systems becomes imperative to address these challenges and enhance agricultural productivity. Therefore, this paper aims to present a Machine Learning (ML) based crop recommendation system tailored for the farming landscape. The proposed system utilizes historical data on climatic conditions, soil properties, crop yields, and farmer preferences to provide personalized crop recommendations. The goal of this study is to appraise the efficacy of nine distinct ML models—Logistic Regression (LR), Support Vector Machine (SVM), K-Nearest Neighbors (KNN), Decision Tree (DT), Random Forest (RF), Bagging (BG), AdaBoost (AB), Gradient Boosting (GB), and Extra Trees (ET) to generate practical recommendations for crop selection. Numerous preprocessing methods are employed to cleanse and normalize the data, thereby ensuring its appropriateness for model training. The ML models are trained using historical data sets, including temperature, rainfall, humidity, soil pH, and nutrient levels, where crop yields are correlated with environmental and agronomic factors. The models undergo fine-tuning through methods such as cross-validation to enhance their performance and ensure robustness. Among those models, Radom Forest has achieved the highest accuracy (99.31%). The proposed Machine Learning-based crop recommendation system offers a promising approach to addressing the challenges faced by the farmers. By leveraging advanced data analytics and artificial intelligence techniques, the system empowers farmers with timely and personalized recommendations, ultimately leading to improved agricultural productivity, food security, and economic prosperity.
Similar content being viewed by others
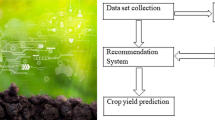
Intelligent Crop Recommender System for Yield Prediction Using Machine Learning Strategy
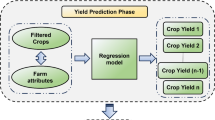
Farm-Level Smart Crop Recommendation Framework Using Machine Learning
Crop Recommendation in the Context of Precision Agriculture
Explore related subjects.
- Artificial Intelligence
Avoid common mistakes on your manuscript.
1 Introduction
Agriculture is the oldest industry, essential for nourishing the global population. With modernization and technological integration, it has evolved to optimize efficiency, attract more participants, and elevate overall quality standards [ 1 ]. However, the looming threat of diminishing arable land due to urbanization poses a daunting challenge in agriculture. Coupled with the imperative to boost food production by over 70% by 2050 to accommodate population growth, the need for innovative solutions becomes paramount [ 2 ]. So, there is an urgent need for an automated system that can maximize harvest yield while minimizing resource inputs, ensuring agricultural sustainability in the face of evolving global demands [ 3 ].
Machine Learning has appeared as a formidable instrument in transforming the agricultural sector, proffering innovative solutions to enduring challenges such as crop yield estimation [ 4 , 5 , 6 ], plant species identification [ 7 ], and disease detection [ 8 , 9 , 10 , 11 ], thus heralding a new era of precision agriculture. By harnessing extensive datasets, encompassing variables from soil composition and meteorological patterns to crop health and yield projections, ML algorithms furnish critical insights to farmers, agronomists, and researchers alike. In recent years, a multitude of scholars have implemented ML techniques to develop crop recommendation systems grounded in agricultural parameters. In paper [ 12 ] advanced an intelligent system named Agro-Consultant, designed to aid farmers in decision-making based on soil type, area, pH, and precipitation. Chougule [ 13 ] employed ML algorithms such as Random Forest to forecast harvest yield predicated on soil categories, conditions, and rainfall. Kulkarni et al. [ 14 ] proposed a crop recommendation framework leveraging ensemble machine learning methodologies, integrating predictions from a variety of techniques including RF, Naive Bayes (NB), and Lagrangian SVM, to recommend suitable crops based on mud characteristics and parameters with heightened efficacy. These ML approaches were utilized to delineate relationships between soil nutrient levels, identifying a substantial influence of nitrogen (N) on phosphorus (P) and its subsequent effect on potassium (K), while nitrogen demonstrated a weaker correlation with potassium. Additionally, a researcher [ 15 ] utilized soil sensors interfaced with an Arduino board and ESP 8266 WiFi module to amass soil data for crop recommendation purposes.
Different algorithms, including Naive Bayes, Logistic Regression, and C4.5, were applied to rainfall data, with C4.5 achieving the highest accuracy at 85.07% [ 16 ]. Gosai et al. developed a robust feature extraction framework deploying various ML algorithms such as NB, RF, SVM, DT, LR, and XGBoost. Their experimental results demonstrated that NB, RF, and XGBoost yielded the highest prediction accuracy, reaching an impressive 99% [ 17 ]. The researchers further recommended optimal crop selections based on geospatial and climatic data gathered from agricultural databases. A novel hybrid model was introduced, merging Naive Bayes and J48 with association rule mining, where the J48 algorithm achieved a commendable accuracy of 95.9% [ 18 ]. Abrougui et al. conducted a predictive analysis for organic potato yield estimation, employing soil property data. Through a comparative evaluation of Artificial Neural Networks (ANN) and Multiple Linear Regression (MLR), ANN exhibited superior performance, boasting a correlation coefficient of 0.975 [ 19 ]. Villanueva et al. carried out image acquisition of bitter melon plants from agricultural fields, subsequently analyzing their fruit-bearing potential through the Convolutional Neural Network (CNN) methodology. Their findings affirmed that CNN reliably predicts crop yield [ 20 ].
While these studies showcase the potential of ML in agricultural applications, a limitation observed in the literature review is the reliance on a relatively small number of ML algorithms, ranging from 2 to 6, for recommending crop strategies in intelligent farming. The narrow scope of algorithms utilized may restrict the diversity of approaches explored and the robustness of the predictive models developed. Therefore, this study has used nine ML algorithms—LR, SVM, KNN, DT, RF, BG, AB, GB, and ET for developing a crop recommendation system by collecting diverse data from the Kaggle repository on soil types, climate, historical crop yields, and farmer preferences. No study has employed all nine specified ML algorithms simultaneously on identical datasets for crop recommendation in intelligent farming applications. Numerous performance metrics, including precision, accuracy, F1-score, and recall, have been employed to gauge the effectiveness of these models. The comparison of models revealed that Random Forest exhibited the highest performance among them. The proposed system will offer a pathway towards sustainable and resilient farming practices, ensuring the long-term viability of our food systems in the face of global challenges.
2 Methodology
This paper endeavors to engineer a crop recommendation system by harnessing the capabilities of diverse ML algorithms. The proposed framework encompasses several critical phases: data acquisition and preprocessing, crop prediction through ML methodologies, and the subsequent stages of model training, testing, and performance evaluation. The workflow diagram of the proposed system is depicted in Fig. 1 .
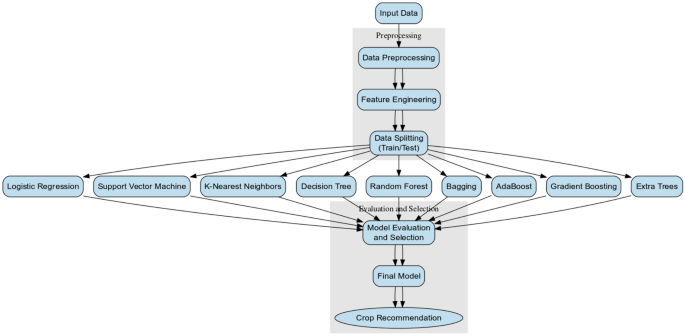
Proposed crop recommendation system
2.1 Data Collection
The dataset employed in this study was sourced from the Kaggle archives [ 21 ], accumulated by the Food and Agriculture Council of India. It comprises 2200 data points and spans 22 crops, including Rice, Maize, Jute, Cotton, Coconut, Papaya, Orange, Apple, Muskmelon, Watermelon, Grapes, Mango, Banana, Pomegranate, Lentil, Blackgram, Mungbean, Mothbeans, Pigeon peas, Kidney beans, Chickpea, and Coffee. This dataset is structured around variables related to Nitrogen, Phosphorus, Potassium, fertilizers, soil pH [ 22 ], and climate factors such as rainfall, temperature, and humidity. Initially, we import the data from datasets and check for null and duplicated values within the dataset. Subsequently, we label each crop using one-hot encoding and compile them into a dictionary. Following this, we train the Data Distribution Testing and Scaling function using MinMaxScaler and the Data Training Model. Notably, this dataset exhibits exceptional quality by encompassing a diverse range of geographical conditions and crops, underscoring its potential utility across regions worldwide with similar environmental conditions. A detailed description of the dataset employed in this study is presented in Table 1 .
The dataset encompasses information regarding various attributes pertinent to agricultural conditions. The description of each attribute is outlined below:
N : This attribute exhibits a range of (0–139) kg/ha, indicative of the quantity of nitrogen in the soil, measured in kilograms per hectare.
P : This attribute ranges from (5–145) kg/ha, representing the amount of phosphorus in the soil, measured in kilograms per hectare.
K : With a range of (5–205) kg/ha, this attribute denotes the quantity of potassium in the soil, measured similarly to N and P.
Temperature : Ranging from (10.78–43.36) K, this attribute is provided in Kelvin value, reflecting the temperature conditions.
Humidity : With a range of (14.69–98.80) F, this attribute can be expressed in Fahrenheit or Celsius, indicating humidity.
p H : Spanning from (3.55–7.45), the pH attribute typically operates on a scale from 0 to 14, measuring the acidity or alkalinity of a substance, thus reflecting soil conditions.
Rainfall : This attribute signifies the volume of rainfall in millimetres, exhibiting a range of (20.21–291.29) mm, providing insight into precipitation levels.
2.2 Prediction of Crop Using ML Techniques
The study employs a range of ML algorithms to recommend crops based on various factors, including NPK fertilizer levels, soil pH, and climatic conditions. These algorithms encompass LR, SVM, KNN, DT, RF, BG, AB, GB, and ET.
2.2.1 Logistic Regression
Predictive LR emerges as a potent machine learning approach for crop recommendation, particularly in binary classification scenarios where the objective is to ascertain whether a specific crop should be cultivated, based on variables such as soil composition, climatic conditions, and water resources. The methodology initiates with rigorous data preprocessing, encompassing data cleansing, imputation of missing values, and the normalization or standardization of features to maintain uniformity in scale. Throughout the training phase, Logistic Regression identifies the optimal coefficients that establish a linear decision boundary to delineate the classes. Upon completion of training, the model’s performance is scrutinized using metrics. This methodical approach facilitates well-informed decisions regarding crop cultivation, utilizing Logistic Regression to enhance agricultural methodologies.
2.2.2 Support Vector Machine
SVMs can be effectively applied to crop recommendation in Machine Learning. The core concept behind using SVM for this purpose is constructing a classification model to identify the most suitable crops to grow based on various factors. The first step involves feature selection, identifying the traits that significantly influence plant growth and crop yield. After selecting the appropriate features, the SVM classifier is trained using preprocessed data [ 23 ]. This data should be cleaned, scaled, and normalized to ensure the model’s robustness and to avoid overfitting. The SVM algorithm endeavors to ascertain the optimal hyperplane that demarcates the distinct classes of crops within the multidimensional feature space, thereby facilitating the precise discrimination among potential crop recommendations. Upon the successful training and rigorous evaluation of the SVM model, it becomes proficient in delivering crop recommendations by exploiting its capacity to delineate intricate decision boundaries inherent in the dataset. Users can input relevant information, including soil properties, climate conditions, and topography, and the model will predict which crops are most suitable to grow in that particular context. This approach offers a powerful tool for farmers and agricultural planners, ultimately contributing to improved farm productivity and sustainability.
2.2.3 K-Nearest Neighbors
K-Nearest Neighbors excels when data is non-linear or doesn’t follow a specific distribution [ 24 ]. KNN classifies or predicts based on the majority class or average value of the k closest data points in the feature space. Cross-validation can optimize this, with smaller k values offering more sensitivity and larger ones providing stability. KNN is based on the principle of similarity, typically using Euclidean or Manhattan distance to find the closest neighbours. In classification, KNN assigns a class based on the majority vote among the K’s Nearest Neighbours. For regression, it predicts by averaging the values of the nearest neighbours. KNN is considered a “lazy learner” because it doesn’t build a complex model during training; computation occurs during the prediction phase. This flexibility makes it a popular choice for many machine-learning tasks, especially when data structures are complex and require a simple yet effective approach. Feature scaling or normalization is recommended to ensure optimal performance with KNN.
2.2.4 Decision Tree
Decision Tree models decisions through a tree-like structure comprising nodes and branches, where internal nodes represent conditions or tests on specific features, and leaf nodes represent the final output or decision [ 25 ]. The algorithm constructs the tree by selecting the most significant features to split the data, often using criteria like Gini Impurity, Entropy, or Information Gain. Decision Trees are famous for their simplicity, interpretability, and ability to handle non-linear data without complex transformations. DTs are also sensitive to small data changes, leading to variations in the tree structure. Despite these limitations, they are widely used in finance, healthcare, and marketing due to their clear visualization and ease of understanding.
2.2.5 Random Forest
A RF is a robust supervised learning algorithm employed in both classification and regression tasks, renowned for its ensemble methodology that amalgamates multiple decision trees. Each tree within the Random Forest is generated by selecting a stochastic subset of the training dataset, alongside a randomly chosen subset of features at each decision node, fostering diversity among the trees. This aggregation process enhances model stability and generalization. Random Forests offer several advantages, including reduced overfitting, improved accuracy, and insights into feature importance, making them useful in various arenas such as healthcare, finance, and marketing. However, they are more complex than single decision trees and require more computational resources. Despite this, Random Forests are favoured for their robustness and versatility in handling large datasets and complex data structures.
2.2.6 Bagging
Bagging, an acronym for bootstrap aggregating, is a widely utilized ensemble learning technique in ML. This method involves training multiple models in isolation, each on a different subset of the data generated through bootstrapping. This technique is often used to reduce variance and avoid overfitting. Different crop recommendation models can be trained using different subsets of available data or different algorithms. Baggage helps create different patterns by introducing randomness into the training process. By combining predictions from multiple models, bagging often leads to higher prediction accuracy than individual models. This can be especially beneficial in crop recommendation, where accurate predictions are crucial for maximizing yield and optimizing resource utilization. Bagging helps make the crop recommendation system more stable and robust by reducing the impact of abnormal or noisy data. Bagging can also be used for feature selection by training each model on a random subset of features. This helps identify the most relevant features for crop recommendation while reducing the risk of overfitting. Since each model in the crop ensemble is trained independently, crop algorithms can be easily parallelized, making them effective for large-scale crop recommendation systems.
2.2.7 AdaBoost
AdaBoost, short for Adaptive Boosting, is a prominent ensemble learning algorithm employed in ML for both classification and regression tasks. It functions by sequentially merging numerous weak learners—models that execute slightly better than random guessing—into a single, robust model. In each iteration, AdaBoost adjusts the weights of misclassified instances, prioritizing them in subsequent training rounds. This iterative process amplifies the contribution of weaker learners, culminating in a more accurate and resilient model.
2.2.8 Gradient Boosting
Gradient Boosting is a formidable ensemble learning method widely applied in ML for both classification and regression tasks. It constructs a robust predictive model through the iterative training of multiple weak learners, typically decision trees. In this process, each successive tree is trained to rectify the residual errors of its predecessor, thereby incrementally enhancing the model’s overall predictive accuracy. The critical concept of Gradient Boosting is to iteratively optimize a loss function by minimizing the residual errors, allowing the model to improve with each step. The process involves adding new trees to the ensemble, each tree trained on the residuals or errors from the preceding iteration, adjusting for overfitting through regularization techniques like learning rate and tree depth control. Gradient Boosting is highly effective for achieving high accuracy, offering flexibility with various loss functions and parameters, making it adaptable to multiple tasks. While it can be computationally intensive and prone to overfitting if not adequately managed, Gradient Boosting is valued for its ability to create robust and precise predictive models, often outperforming other ensemble techniques. It’s commonly used in finance, healthcare, and marketing applications, where predictive accuracy is crucial [ 26 ].
2.2.9 Extra Trees
Extra Trees resembles RFs but introduces greater randomness in its tree construction, leading to increased robustness and condensed risk of overfitting. Extra Trees create an ensemble of decision trees. Still, unlike Random Forests, they randomly choose both features and split thresholds rather than selecting the best split based on a specific criterion. This added randomness can accelerate training times and make the model more resilient to noisy or diverse datasets. In Extra Trees, each tree contributes to the final prediction through majority voting for classification or averaging for regression, yielding an effective ensemble model. The trade-off for this additional randomness is potentially lower accuracy in datasets with distinct patterns. Still, Extra Trees can be a highly efficient and robust method for handling large and high-dimensional datasets, making it a versatile tool in healthcare, finance, and marketing [ 27 ].
2.3 Training and Testing
Addressing the challenge posed by unbalanced data during the training and testing of machine learning models is crucial, as biased models often yield inaccurate predictions for minority classes. To mitigate this issue, we implemented a down-sampling strategy. Additionally, we employed early stopping techniques to prevent overfitting and enhance model generalization. Machine learning algorithms were employed to forecast the optimal cultivation strategy. The dataset was partitioned, with 80% allocated for model training and the residual 20% reserved for testing to evaluate the model’s predictive efficacy.
2.4 Performance Metrics
This study used different performance metrics such as Confusion Matrix (CM), ROC (Receiver Operating Curve) curve, precision, recall, F1 score, and accuracy to evaluate the efficacy of the ML models.
Confusion Matrix : A CM is a table that visualizes a classification model’s performance by comparing predicted and actual classes, highlighting True Positives (TPs), False Positives (FPs), True Negatives (TNs), and False Negatives (FNs) for each class.
Receiver Operating Characteristic Curve : The ROC curve illustrates the trade-off between the true positive rate (TPR) and the false positive rate (FPR) across various threshold settings.
Precision: Precision enumerates the accuracy of positive predictions by measuring the proportion of TPs among all predicted positives. It can be calculated using Eq. ( 1 ):
Recall: Recall assesses the classifier’s effectiveness by measuring the proportion of actual positives that were correctly identified. The F1 score can be calculated using the Eq. ( 2 ):
F1 Score: The F1 score is the harmonic mean of precision and recall, providing a single metric that balances both aspects. It is calculated using the formula:
Accuracy: Accuracy measures the proportion of correctly classified instances, including both TPs and TNs, relative to the total number of instances. It can be calculated using the formula:
3 Results and Discussions
This section provides an in-depth analysis of the performance metrics for all Machine Learning algorithms used in the proposed crop recommendation system. It includes detailed evaluations of ROC curve, accuracy, precision, recall, F1 score, and, confusion matrix analysis. These results enable a comprehensive assessment of each algorithm’s effectiveness and appropriateness for the task.
3.1 Nutrient Requirements and Environmental Factors of Agricultural and Horticultural Crops
Nutrient requirements, environmental factors affecting agricultural and horticultural crops, and the correlation of crop nutrients play crucial roles in determining crop health, growth, and yield potential. Figure 2 depicts the correlation of crop nutrients, illustrating the interrelationships between various nutrient factors crucial for crop health and productivity. The plot matrixes represent NPK requirements, temperature, humidity, rainfall, and soil p H for growing various agricultural and horticultural crops. The crop temperature range is generally narrow; However, some cultures, like rice, require high rainfall and prefer warm temperatures. The crops mentioned thrive under specific environmental conditions: acidic soils (pH 5.0–6.5) are ideal for growth, while maize, jute, cotton, coconut, papaya, orange, muskmelon, watermelon, grapes, banana, mango, pomegranate, mungbean, mothbeans, blackgram, pigeon peas, kidney beans, chickpea, and coffee flourish in warm climates with moderate to high rainfall. These crops are adaptable to a broad pH range but perform optimally in slightly acidic to neutral soils (pH 5.8–7.0). On the other hand, apple and lentil prefer cooler temperatures and moderate rainfall, thriving in well-drained soils with a slightly acidic to neutral pH (6.0–7.0).
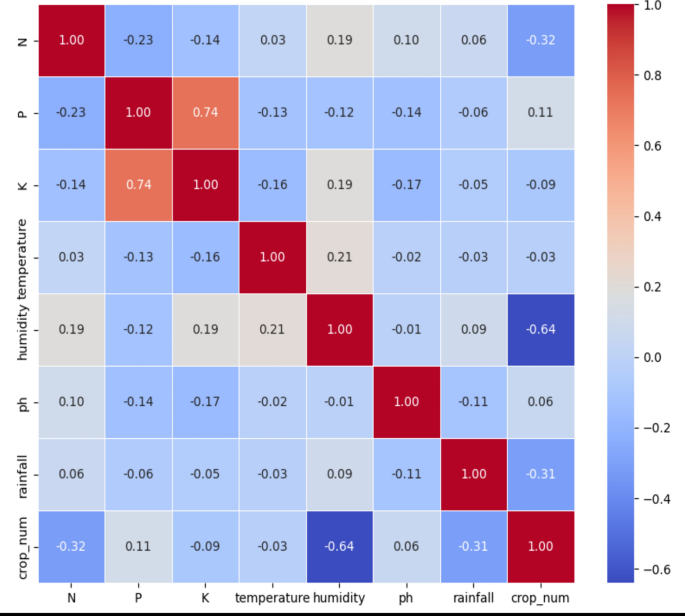
Correlation of crop nutrients
Figure 3 displays the histogram of nitrogen for the proposed system, depicting the distribution of recommended nitrogen levels across various crops. This histogram visualizes the recommended nitrogen levels, with nitrogen levels depicted along the x-axis and the frequency or proportion of recommendations along the y-axis. Each bar within the histogram represents a specific range of recommended nitrogen levels, with the height of the bar indicating how frequently recommendations fall within that particular range. Analyzing this data offers valuable insights into the typical nitrogen requirements of different crops, thereby aiding farmers in making informed decisions regarding fertilization practices.
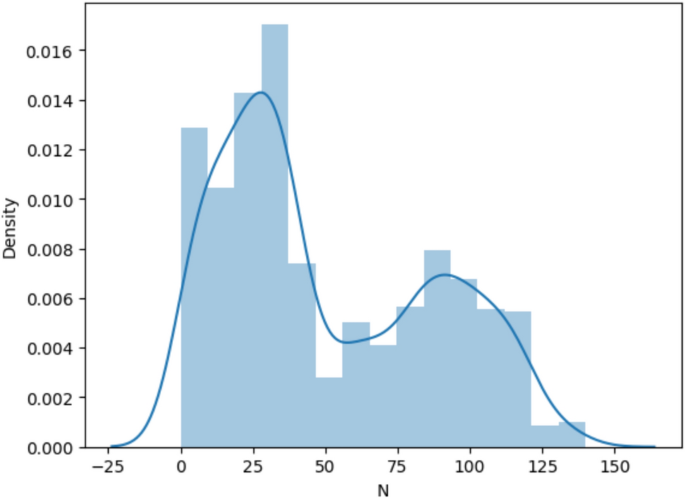
Histogram of Nitrogen
3.2 Visualization of Model Responses for Crop Recommendations
The performance evaluation of the crop model is visualized through the analysis of the confusion matrix in Fig. 4 , providing a comprehensive understanding of the model’s classification accuracy across different classes. Additionally, the ROC curves, depicted in Fig. 5 , offer insights into the model’s performance by illustrating the trade-off between TP and FP rates across various classification thresholds. Together, these visualizations enable a thorough assessment of the model’s predictive capabilities and effectiveness in recommending crops based on diverse agricultural and environmental factors.
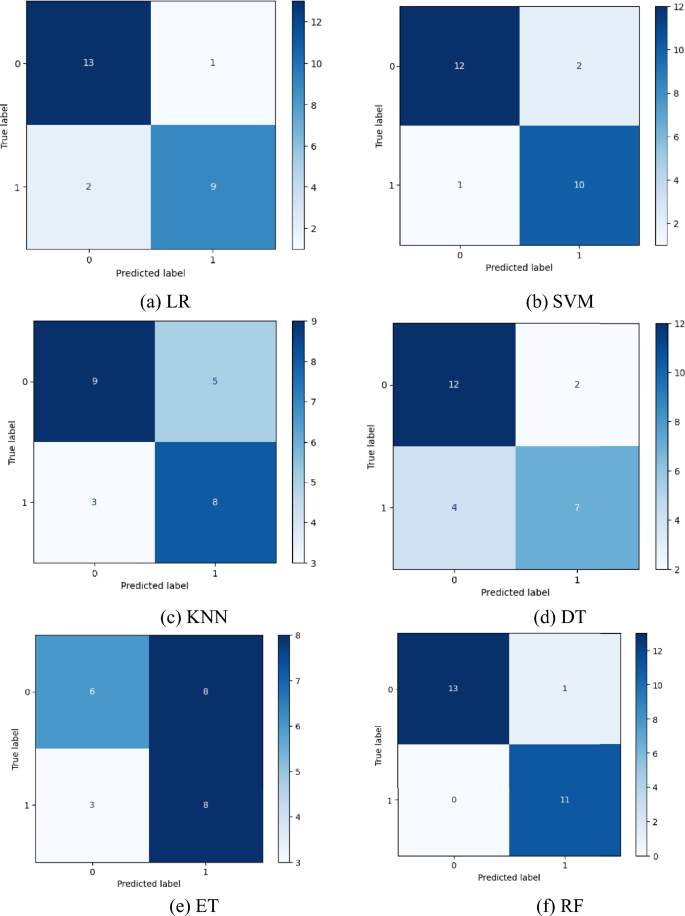
Confusion matrix of the ML models
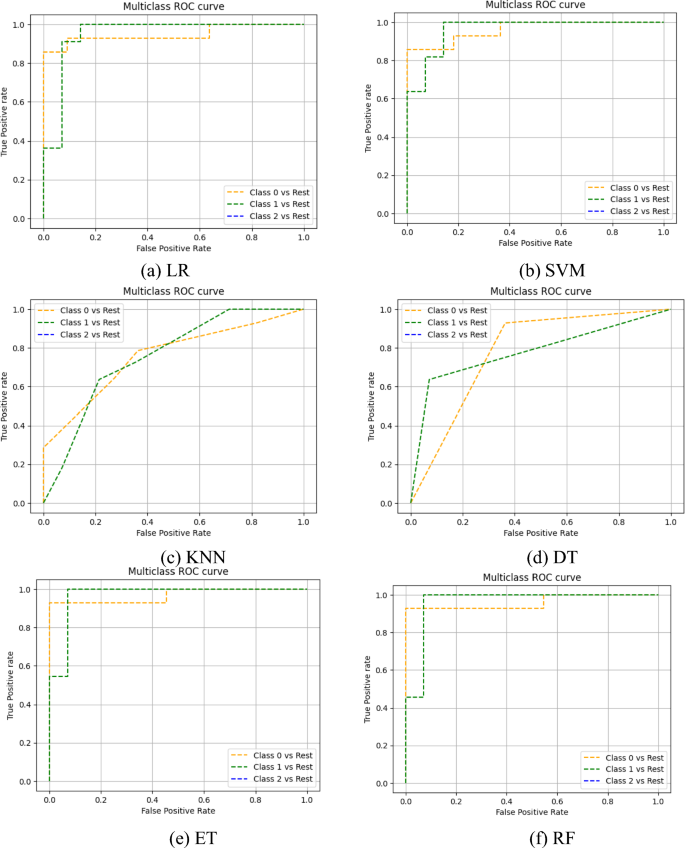
ROC curve of the ML models
Figure 6 illustrates the correlation matrix of all the crops, serving as a tool to comprehend the interrelationships between different crops concerning various factors such as environmental conditions, soil type, climate, and agricultural practices. Each crop is systematically denoted with its name reiterated in a structured format (“Rice to rice, Maize to maize, Jute to jute…”), indicating self-correlation. The correlation matrix was established by computing Pearson correlation coefficients between crop pairs, derived from their corresponding feature sets. This matrix provides valuable insights into the degree and nature of associations between different crops, aiding in understanding their dependencies and potential interactions within agricultural ecosystems.
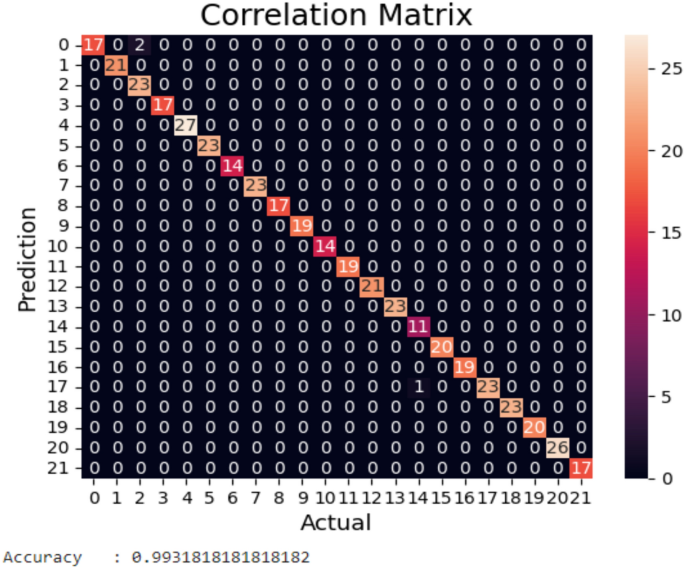
Correlation of Crops
3.3 Comparative Analysis of ML Algorithms
The study aimed to recommend crops based on multiple factors, employing nine ML algorithms, including LR, SVM, KNN, DT, RF, BG, AB, GB, and ET. These models underwent training and optimization with specific parameters outlined in the methodology section. A comparative analysis of all models is presented in Table 2 .
Table 2 provides a comprehensive overview of various ML classifiers and their corresponding precision, recall, and F1 scores. LR achieved a precision of 0.88, recall of 0.87, and an F1-score of 0.88. SVM attained a precision, recall, and F1-score of 0.88. KNN exhibited a precision of 0.68, a recall of 0.69, and an F1-score of 0.68. DT resulted in a precision, recall, and F1-score of 0.72. RF demonstrated a precision of 0.92, recall of 0.93, and an F1-score of 0.92. Bagging showed a precision, recall, and F1-score of 0.92. AB achieved a precision and recall of 0.78, with an F1-score of 0.76. GB yielded a precision of 0.85, a recall of 0.83, and an F1-score of 0.83. ET achieved a precision and recall of 0.96, with an F1-score of 0.96. Comparing the models, Random Forest exhibited the highest test accuracy of 99.31%, while AdaBoost displayed the lowest test accuracy of 14.09% for crops. Conversely, the performance of models for horticultural crops showed nearly identical levels of prediction accuracy, precision, F1 score, and recall.
3.4 Discussion
The proposed crop recommendation system is a pivotal application of ML in agriculture, aiming to empower farmers with data-driven insights for strategic crop selection. By leveraging various factors like soil properties, climate conditions, historical data, and market demand, this system aids farmers in making well-informed decisions regarding crop cultivation. Identifying pertinent features that influence crop growth and yield, such as temperature, rainfall, soil pH, and nutrient levels, forms the cornerstone of this endeavour.
Integrating domain knowledge, including agronomic expertise and local farming practices, enriches the accuracy and relevance of the system’s recommendations. Moreover, establishing farmer feedback loops plays a vital role in refining and enhancing the recommendation system iteratively, ensuring its adaptability to evolving agricultural landscapes.
Recognizing the inherent uncertainty in predictions stemming from factors like variability in weather patterns and market conditions underscores the importance of incorporating robust risk assessment techniques. By accounting for associated risks alongside potential yields, farmers can make more informed decisions that balance productivity and risk mitigation.
In essence, the convergence of ML techniques with agricultural domain knowledge fosters a dynamic ecosystem wherein farmers can navigate complex agricultural challenges with confidence and resilience. Table 3 compares the proposed work with existing studies, showcasing the performance metrics and outcomes. The data presented in Table 3 demonstrate that our proposed approach outperforms existing works, highlighting superior performance across various evaluation criteria.
3.4.1 Possible Causes of Miss-Recommendation
Crop suitability is subject to considerable temporal variability, influenced by seasonal fluctuations, climate dynamics, and evolving agricultural practices. Failing to account for these temporal dynamics in the model adequately may result in outdated or irrelevant recommendations that do not align with current conditions. Moreover, the suitability of crops can vary significantly across different geographic regions due to variations in soil types, climatic conditions, and other factors. Models trained on data from one region may struggle to generalize effectively to others, potentially leading to inaccurate recommendations when applied in diverse geographic areas.
Inaccurate model evaluation metrics or insufficient validation processes can also pose challenges. If the evaluation metrics fail to reflect real-world performance accurately or the validation process lacks rigor, it may result in overly optimistic estimates of model performance. Consequently, this increases the likelihood of erroneous recommendations when the model is deployed in production settings. Additionally, certain factors influencing crop adaptation may not be well understood or adequately modelled, leading to unexpected phenomena that can influence recommendations in unforeseen ways. Therefore, addressing these challenges and enhancing the robustness of the model’s temporal adaptability and geographic generalizability is critical for ensuring the reliability and efficacy of crop recommendation systems.
3.4.2 Implication of the Study
The proposed systems use machine learning techniques to analyze data and provide farmers with personalized advice on choosing which crops to grow in a specific location and conditions. This system can help maximize crop productivity by suggesting the most suitable crops for a given location and season. By considering factors such as soil type, moisture levels, temperature, and sunlight, machine learning algorithms can identify crops likely to thrive in specific environmental conditions, thereby increasing yields and profitability for farmers [ 26 ]. This study can help reduce risks associated with climate fluctuations, pest infestations, and market fluctuations. By diversifying crop selection and considering factors such as disease resistance and market demand, farmers can reduce risk exposure and improve their resilience to external shocks. This system can promote sustainable agriculture practices by encouraging the cultivation of environmentally friendly and economically viable crops. Promoting crop rotation, soil conservation [ 27 ], and biodiversity can help farmers maintain their land’s long-term health and productivity [ 28 , 29 ]. The importance of this type of model lies in informed decision-making when practising precision agriculture, as a variety of external factors influence crop performance. Attempting to understand the combined effects of these factors without the aid of models represents a daunting challenge. Agricultural productivity is profoundly influenced by a range of biotic and abiotic stressors. The projected global GDP from agriculture is offset by annual economic losses driven by factors such as climate change, market instability, and resource limitations. This loss affects not only agricultural producers but also extends to related industries and economies worldwide, posing significant challenges to food security, livelihoods, and sustainable development efforts on a global scale.
4 Conclusion
Agriculture is the cornerstone of the world’s economy, employing a significant workforce and contributing substantially to nations’ GDPs. However, agricultural productivity has many challenges, ranging from unpredictable climatic conditions to varying soil types and limited access to modern agricultural practices. Developing intelligent agricultural systems is imperative to overcome these hurdles and bolster agricultural productivity.
This paper addresses this need by presenting a Machine Learning-based Crop Recommendation System tailored to the agricultural landscape. The proposed system aims to deliver personalized crop recommendations by leveraging historical data on climatic conditions, soil properties, crop yields, and farmer preferences. Through the evaluation of nine distinct ML models, including LR, SVM, KNN, DT, RF, BG, AB, GB, and ET, this study seeks to generate practical recommendations for crop selection. Employing various preprocessing techniques to clean and normalize the data ensures its suitability for model training. The machine learning models are trained on historical datasets encompassing temperature, rainfall, humidity, soil pH, and nutrient levels, correlating crop yields with environmental and agronomic factors. Fine-tuning of the models, employing techniques such as cross-validation, optimizes their performance and ensures robustness. Among these models, Random Forest emerges as the top performer, achieving the highest accuracy rate of 99.31%. The proposed Machine Learning-based Crop Recommendation system offers a promising avenue for addressing the challenges faced by farmers. By harnessing advanced data analytics and artificial intelligence techniques, the system empowers farmers with timely and personalized recommendations, ultimately leading to improved agricultural productivity, food security, and economic prosperity.
While our current study effectively utilizes the available dataset to develop a crop recommendation system, we acknowledge certain limitations. Specifically, our dataset does not fully capture variations in land quality, climate changes, or historical crop planting data, which are crucial for accurate recommendations. Future research will focus on incorporating more detailed and temporal soil and climate data, as well as historical crop planting information. We also plan to integrate farmers’ professional knowledge and explore advanced Machine Learning techniques and hybrid models to address the complexities of agricultural decision-making better. These enhancements will improve the system’s adaptability and accuracy, ultimately providing more personalized and effective recommendations for farmers.
Data Availability
The datasets generated during this study are available from the corresponding author upon reasonable request.
Wang SW, Lee WK, Son Y. An assessment of climate change impacts and adaptation in South Asian agriculture. Int J Clim Chang Strateg Manag. 2017;9:517–34. https://doi.org/10.1108/IJCCSM-05-2016-0069 .
Article Google Scholar
Bouguettaya A, Zarzour H, Kechida A, Taberkit AM. Deep learning techniques to classify agricultural crops through UAV imagery: a review. Neural Comput Appl. 2022;34:9511–36. https://doi.org/10.1007/S00521-022-07104-9.20223412 .
Gathala MK, Timsina J, Islam MS, Rahman MM, Hossain MI, Harun-Ar-Rashid M, McDonald A. Conservation agriculture based tillage and crop establishment options can maintain farmers’ yields and increase profits in South Asia’s rice-maize systems: evidence from Bangladesh. Field Crops Res. 2015;172:85–98.
Fei S, Hassan MA, Xiao Y, Su X, Chen Z, Cheng Q, Duan F, Chen R, Ma Y. UAV-based multi-sensor data fusion and machine learning algorithm for yield prediction in wheat. Precis Agric. 2022. https://doi.org/10.1007/s11119-022-09938-8 .
Paudel D, Boogaard H, de Wit A, van der Velde M, Claverie M, Nisini L, Janssen S, Osinga S, Athanasiadis IN. Machine learning for regional crop yield forecasting in Europe. Field Crops Res. 2022. https://doi.org/10.1016/J.FCR.2021.108377 .
Burdett H, Wellen C. Statistical and machine learning methods for crop yield prediction in the context of precision agriculture. Precis Agric. 2022;23:1553–74. https://doi.org/10.1007/s11119-022-09897-0 .
Duke OP, Alabi T, Neeti N, Adewopo J. Comparison of UAV and SAR performance for Crop type classification using machine learning algorithms: a case study of humid forest ecology experimental research site of West Africa. Int J Remote Sens. 2022;43:4259–86. https://doi.org/10.1080/01431161.2022.2109444 .
Too EC, Yujian L, Njuki S, Yingchun L. A comparative study of fine-tuning deep learning models for plant disease identification. Comput Electron Agric. 2019;161:272–9. https://doi.org/10.1016/j.compag.2018.03.032 .
Chu H, Zhang C, Wang M, Gouda M, Wei X, He Y, Liu Y. Hyperspectral imaging with shallow convolutional neural networks (SCNN) predicts the early herbicide stress in wheat cultivars. J Hazard Mater. 2022. https://doi.org/10.1016/j.jhazmat.2021.126706 .
Dey B, Masum Ul Haque M, Khatun R, Ahmed R. Comparative performance of four CNN-based deep learning variants in detecting Hispa pest, two fungal diseases, and NPK deficiency symptoms of rice (Oryza sativa) Comput. Electron Agric. 2022. https://doi.org/10.1016/j.compag.2022.107340 .
Sai Sankar PR, Ramakrishna SDPS, Venkata Rakesh MM, Raja P, Hoang VT, Szczepanski C. Intelligent health assessment system for paddy crop using CNN, 2021 3rd. Int Conf Signal Process Commun ICPSC. 2021;2021:382–7. https://doi.org/10.1109/ICSPC51351.2021.9451644 .
Z. Doshi S, Nadkarni R, Agrawal N. Shah, Agro-consultant: intelligent crop recommendation system using machine learning algorithms. In: Fourth International Conference on Computing Communication Control and Automation (ICCUBEA), IEEE, 2018, pp. 1–6. https://doi.org/10.1109/ICCUBEA.2018.8697349 .
ChouguleVKA, Mukhopadhyay D. Crop suitability and fertilizers recommendation using data mining techniques, in: Advances in Intelligent Systems and Computing, Vol. 714, Springer Verlag, 2019, pp. 205–213. https://doi.org/10.1007/978-981-13-0224-419 .
Kulkarni NH, Srinivasan GN, Sagar BM, Cauvery NK. Improving crop productivity through A crop recommendation system using ensembling technique. Proc 2018 3rd Int Conf Comput Syst Inf Technol Sustain Solut CSITSS. 2018. https://doi.org/10.1109/CSITSS.2018.8768790 .
Modi D, Sutagundar AV, Yalavigi V, Aravatagimath A. Crop recommendation using machine learning algorithm. 2021 5th Int Conf Inf Syst Comput Networks ISCON. 2021.
Prabhu S, Revandekar P, Shirdhankar S, Paygude S. Soil analysis and crop prediction. Int J Sci Res Sci Technol. 2020;7(4):117–23.
Gosai D, Raval C, Nayak R, Jayswal H, Patel A. Crop recommendation system using machine learning. Int J Sci Res Comput Sci Eng Inf Technol. 2021;7(3):558–69.
Viviliya B, Vaidhehi V. The design of hybrid crop recommendation system using machine learning algorithms. Int J Innov Technol Explor Eng (IJITEE). 2019;9:4305–11.
Abrougui K, Gabsi K, Mercatoris B, Khemis C, Amami R, Chehaibi S. Prediction of organic potato yield using tillage systems and soil properties by artificial neural network (ANN) and multiple linear regressions (MLR). Soil Tillage Res. 2019;190:202–8.
Villanueva MB, Salenga MLM. Bitter melon crop yield prediction using machine learning algorithm. Int J Adv Comput Sci Appl. 2018;9:1–6.
Google Scholar
Kaggle. https://www.kaggle.com/datasets/atharvaingle/crop-recommendation-dataset . Accessed date: 12 January 2024
Suresh G, Kumar DAS, Lekashri DS, Manikandan DR, Head C-O. Efficient crop yield recommendation system using machine learning for digital farming. Int J Mod Agric. 2021;10:906–14.
Hua Y, Li F, Yang S. Application of support vector machine model based on machine learning in art teaching. Wireless Commun Mobile Comput. 2022. https://doi.org/10.1155/2022/7954589 .
Dey B, Abir KAM, Ahmed R, Salam MA, Redowan M, Miah MD, Iqbal MA. Monitoring groundwater potential dynamics of north-eastern Bengal Basin in Bangladesh using AHP-Machine learning approaches. Ecol Indicat. 2023. https://doi.org/10.1016/j.ecolind.2023.110886 .
Charoen-Ung P, Mittrapiyanuruk P. Sugarcane yield grade prediction using random forest with forward feature selection and hyper-parameter tuning. Adv Intell Syst Comput. 2019;769:33–42. https://doi.org/10.1007/978-3-319-93692-5_4 .
Sridevi V, Chellamuthu V. Impact of weather on rice—a review. Int J Appl Res. 2015;1:825–31.
Swathi T, Sudha S. Crop classification and prediction based on soil nutrition using machine learning methods. Int J Inf Technol. 2023;15:2951–60. https://doi.org/10.1007/S41870-023-01345-0 .
Singh Jatav Sri Karan H, Jena J, Maitra S, Hossain A, Pramanick B, Gitari HI, Praharaj S, Shankar T, Bharati Palai J, Rathore A, Kumar Mandal T, Singh Jatav H. Role of legumes in cropping system for soil ecosystem improvement improvement of cropping system view project precision agriculture view project role of legumes in cropping system for soil ecosystem improvement. Nova Science Publishers, Inc. 2022.
Thilakarathne NN, Bakar MSA, Abas PE, Yassin H. A cloud enabled crop recommendation platform for machine learning-driven precision farming. Sensors. 2022. https://doi.org/10.3390/s22166299 .
JainS, Ramesh D. Machine Learning convergence for weather-based crop selection, IEEE International Students’ Conference on Electrical, Electronics and Computer Science (2020). https://doi.org/10.1109/SCEECS48394.2020.75 .
Download references
The authors declare that no financial support, grants, or other forms of assistance were received during the preparation of this manuscript.
Author information
Authors and affiliations.
Department of Computer Science and Engineering, Shanto-Mariam University of Creative Technology, Uttara 17, Dhaka, 1230, Bangladesh
Farida Siddiqi Prity, MD. Mehadi Hasan, Shakhawat Hossain Saif, Md. Maruf Hossain, Sazzad Hossain Bhuiyan, Md. Ariful Islam & Md Tousif Hasan Lavlu
Department of Information and Communication Engineering, Noakhali Science and Technology University, Noakhali, 3814, Bangladesh
Farida Siddiqi Prity
Department of Information and Communication Engineering, Pabna University of Science and Technology, Pabna, 6600, Bangladesh
Md. Maruf Hossain
Institute of Information and Communication Technology, Bangladesh University of Engineering and Technology, Dhaka, 1000, Bangladesh
Md. Ariful Islam
You can also search for this author in PubMed Google Scholar
Contributions
MD. Mehadi Hasan and Shakhawat Hossain Saif designed the study and authored the initial manuscript draft. Farida Siddiqi Prity and Md. Maruf Hossain handled the study analyses. Sazzad Hossain Bhuiyan, Md. Ariful Islam, and Md. Tousif Hasan Lavlu managed the literature searches. All authors reviewed and approved the final manuscript.
Corresponding author
Correspondence to Farida Siddiqi Prity .
Ethics declarations
Conflict of interest.
The authors have no conflict of interest to disclose.
Ethical Approval
This article does not involve any studies conducted with human participants or animals by the authors.
Additional information
Publisher's note.
Springer Nature remains neutral with regard to jurisdictional claims in published maps and institutional affiliations.
Rights and permissions
Open Access This article is licensed under a Creative Commons Attribution 4.0 International License, which permits use, sharing, adaptation, distribution and reproduction in any medium or format, as long as you give appropriate credit to the original author(s) and the source, provide a link to the Creative Commons licence, and indicate if changes were made. The images or other third party material in this article are included in the article's Creative Commons licence, unless indicated otherwise in a credit line to the material. If material is not included in the article's Creative Commons licence and your intended use is not permitted by statutory regulation or exceeds the permitted use, you will need to obtain permission directly from the copyright holder. To view a copy of this licence, visit http://creativecommons.org/licenses/by/4.0/ .
Reprints and permissions
About this article
Prity, F.S., Hasan, M.M., Saif, S.H. et al. Enhancing Agricultural Productivity: A Machine Learning Approach to Crop Recommendations. Hum-Cent Intell Syst (2024). https://doi.org/10.1007/s44230-024-00081-3
Download citation
Received : 08 June 2024
Accepted : 23 August 2024
Published : 02 September 2024
DOI : https://doi.org/10.1007/s44230-024-00081-3
Share this article
Anyone you share the following link with will be able to read this content:
Sorry, a shareable link is not currently available for this article.
Provided by the Springer Nature SharedIt content-sharing initiative
- Crop recommendation
- Machine learning
- Decision tree
- Find a journal
- Publish with us
- Track your research
Information
- Author Services
Initiatives
You are accessing a machine-readable page. In order to be human-readable, please install an RSS reader.
All articles published by MDPI are made immediately available worldwide under an open access license. No special permission is required to reuse all or part of the article published by MDPI, including figures and tables. For articles published under an open access Creative Common CC BY license, any part of the article may be reused without permission provided that the original article is clearly cited. For more information, please refer to https://www.mdpi.com/openaccess .
Feature papers represent the most advanced research with significant potential for high impact in the field. A Feature Paper should be a substantial original Article that involves several techniques or approaches, provides an outlook for future research directions and describes possible research applications.
Feature papers are submitted upon individual invitation or recommendation by the scientific editors and must receive positive feedback from the reviewers.
Editor’s Choice articles are based on recommendations by the scientific editors of MDPI journals from around the world. Editors select a small number of articles recently published in the journal that they believe will be particularly interesting to readers, or important in the respective research area. The aim is to provide a snapshot of some of the most exciting work published in the various research areas of the journal.
Original Submission Date Received: .
- Active Journals
- Find a Journal
- Proceedings Series
- For Authors
- For Reviewers
- For Editors
- For Librarians
- For Publishers
- For Societies
- For Conference Organizers
- Open Access Policy
- Institutional Open Access Program
- Special Issues Guidelines
- Editorial Process
- Research and Publication Ethics
- Article Processing Charges
- Testimonials
- Preprints.org
- SciProfiles
- Encyclopedia
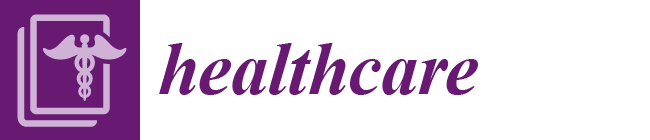
Article Menu
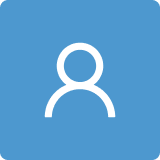
- Subscribe SciFeed
- Recommended Articles
- Author Biographies
- Google Scholar
- on Google Scholar
- Table of Contents
Find support for a specific problem in the support section of our website.
Please let us know what you think of our products and services.
Visit our dedicated information section to learn more about MDPI.
JSmol Viewer
How students learn during the pre-briefing and observation of facilitation in a high-fidelity patient simulation: a narrative analysis, 1. introduction, background and literature review, 2. materials and methods, 2.1. study design, 2.2. study aim and objectives, 2.3. sampling and study setting, 2.4. the structured hfps guidelines, 2.5. the instruments, 2.6. study procedure, 2.7. ethical considerations, 2.8. data analysis, 3.1. students’ demographhic characteristics, 3.2. student learning at pre-briefing and the period of being observers during facilitation, 3.3. learning at pre-briefing stage, 3.3.1. adequate learning resources to increase knowledge acquisition, 3.3.2. clear instruction and guidelines, 3.3.3. adequate time for preparation, 3.3.4. self-motivation for competence enhancement in knowledge and skills, 3.4. learning from observation of hfps, 3.4.1. performance of role-players with knowledge and skill applications, 3.4.2. comments on the performance by others, 3.4.3. discussion with other students who were observers, 3.5. in both sessions, 3.5.1. clear guiding questions and observation areas, 3.5.2. self-awareness and attentiveness, 3.5.3. active self-engagement, 4. discussion, 4.1. practical recommendations, 4.2. strengths and limitations, 5. conclusions, institutional review board statement, informed consent statement, data availability statement, conflicts of interest.
- Lee, J.; Lee, Y.; Lee, S.; Bae, J. Effects of high-fidelity patient simulation led clinical reasoning course: Focused on nursing core competencies, problem solving, and academic self-efficacy. Jpn. J. Nurs. Sci. 2016 , 13 , 20–28. [ Google Scholar ] [ CrossRef ]
- Wong, F.M.F.; Wong, D.C.N. A Modified Guideline for High-Fidelity Patient Simulation to Improve Student Satisfaction and Self-Confidence in Learning: A Mixed Study. Nurs. Rep. 2023 , 13 , 1030–1039. [ Google Scholar ] [ CrossRef ]
- Li, Z.; Huang, F.F.; Chen, S.S.; Wang, A.; Guo, Y. The learning effectiveness of high-fidelity simulation teaching among Chinese nursing students: A mixed-methods study. J. Nurs. Res. 2021 , 29 , e141. [ Google Scholar ] [ CrossRef ]
- Wong, F.M.F.; Chan, A.M.L.; Lee, N.P.M.; Luk, K.K.H. Can High-Fidelity Patient Simulation Be Used for Skill Development in Junior Undergraduate Students: A Quasi-Experimental Study. Healthcare 2023 , 11 , 2221. [ Google Scholar ] [ CrossRef ]
- Yeo, J.Y.; Jang, M.S. Nursing students’ self-directed learning experiences in web-based virtual simulation: A qualitative study. Jpn. J. Nurs. Sci. 2022 , 20 , e12514. [ Google Scholar ] [ CrossRef ]
- Yang, G.F.; Jiang, X.Y. Self-directed learning readiness and nursing competency among undergraduate nursing students in Fujian province of China. Int. J. Nurs. Sci. 2014 , 1 , 255–259. [ Google Scholar ] [ CrossRef ]
- Aljohani, A.S.; Karim, Q.; George, P. Students’ satisfaction with simulation learning environment in relation to self-confidence and learning achievement. J. Health Sci. 2016 , 4 , 228–235. [ Google Scholar ] [ CrossRef ]
- Dalton, L.; Gee, T.; Levett-Jones, T. Using clinical reasoning and simulation-based education to ‘flip’ the enrolled nurse curriculum. Aust. J. Adv. Nurs. 2015 , 33 , 2–15. [ Google Scholar ] [ CrossRef ]
- Gopalakrishnan, P.; Sethuraman, K.R.; Suresh, P. Efficacy of high-fidelity simulation in clinical problem-solving exercises—Feedback from teachers and learners. SBV J. Basic. Clin. Appl. Health Sci. 2018 , 2 , 14–22. [ Google Scholar ] [ CrossRef ]
- Welman, A.; Spies, C. High-fidelity simulation in nursing education: Considerations for meaningful learning. Trends Nurs. 2016 , 3 , 1–16. [ Google Scholar ] [ CrossRef ]
- INACSL Standards Committee. Healthcare simulation standards of best practice—Prebriefing: Preparation and briefing. Clin. Simul. Nurs. 2021 , 58 , 9–13. [ Google Scholar ] [ CrossRef ]
- INACSL Standards Committee. Healthcare simulation standards of best practice—Facilitation. Clin. Simul. Nurs. 2021 , 58 , 22–26. [ Google Scholar ] [ CrossRef ]
- Tutticci, N.; Theobald, K.A.; Ramsbotham, J.; Johnston, S. Exploring the observer role and clinical reasoning in simulation: A scoping review. Nurse Educ. Pract. 2022 , 59 , 103301. [ Google Scholar ] [ CrossRef ]
- Harder, N.; Ross, C.J.M.; Paul, P. Student Perspective of Roles Assignment in High-Fidelity Simulation: An Ethnographic Study. Clin. Simul. Nurs. 2013 , 9 , e329–e334. [ Google Scholar ] [ CrossRef ]
- Henrique-Sanches, B.C.; Cecilio-Fernandes, D.; Costa, R.R.; Almeida, R.G.; Etchegoyen, F.F.; Mazzo, A. Implications of clinical simulation in motivation for learning: Scoping review. Einstein 2024 , 22 , eRW0792. [ Google Scholar ] [ CrossRef ]
- Tekkol, I.A.; Demirel, M. An investigation of self-directed learning skills of undergraduate students. Front. Psychol. 2018 , 9 , 2324. [ Google Scholar ] [ CrossRef ]
- Wu, H.; Zheng, J.; Li, S.; Guo, J. Does academic interest play a more important role in medical sciences than in other disciplines? A nationwide cross-sectional study in China. BMC Med. Educ. 2019 , 19 , 301. [ Google Scholar ] [ CrossRef ]
- Chamberlain, J. The impact of simulation prebriefing on perceptions of overall effectiveness, learning, and self-confidence in nursing students. Nurs. Educ. Perspect. 2017 , 38 , 119–125. [ Google Scholar ] [ CrossRef ]
- Page-Cutrara, K. Prebriefing in nursing simulation: A concept analysis. Clin. Simul. Nurs. 2015 , 11 , 335–340. [ Google Scholar ] [ CrossRef ]
- Levett-Jones, T.; Andersen, P.; Reid-Searl, K.; Guinea, S.; McAllister, M.; Lapkin, S.; Palmer, L.; Niddrie, M. Tag team simulation: An innovative approach for promoting active engagement of participants and observers during group simulations. Nurse Educ. Pract. 2015 , 15 , 345–352. [ Google Scholar ] [ CrossRef ]
- Thidemann, I.J. Olle Söderhamn, High-fidelity simulation among bachelor students in simulation groups and use of different roles. Nurse Educ. Today 2013 , 33 , 1599–1604. [ Google Scholar ] [ CrossRef ]
- Wong, F.M.F.; Tang, A.C.Y.; Cheng, W.L.S. Factors associated with self-directed learning among undergraduate nursing students: A systematic review. Nurse Educ. Today 2021 , 104 , 104998. [ Google Scholar ] [ CrossRef ]
- London, M.; Sessa, V.L.; Shelley, L.A. Developing self-awareness: Learning process for self-and interpersonal growth. Annu. Rev. Organ. Psychol. Organ. Behav. 2023 , 10 , 261–288. [ Google Scholar ] [ CrossRef ]
- Rasheed, S.P.; Sundus, A.; Younas, A.; Fakhar, J.; Inayat, S. Development and testing of a measure of self-awareness among nurses. West. J. Nurs. Res. 2021 , 4391 , 36–44. [ Google Scholar ] [ CrossRef ]
- Lin, X.D.; Lin, Y.; Liu, X.Y.; Su, W.J.; Nie, R.J. The status of study engagement and its influencing factors among nursing arts undergraduates. Chin. J. Nurs. Educ. 2018 , 15 , 770–774. [ Google Scholar ]
- Jacobs, C.; Rigby, J.M. Developing measures of immersion and motivation for learning technologies in healthcare simulation: A pilot study. J. Adv. Med. Educ. Prof. 2022 , 10 , 163–171. [ Google Scholar ] [ CrossRef ]
- Abelsson, A.; Bisholt, B. Nurse students learning acute care by simulation—Focus on observation and debriefing. Nurse Educ. Pract. 2017 , 24 , 6–13. [ Google Scholar ] [ CrossRef ]
- Chang, Y.; Brickman, P. When group work doesn’t work: Insights from students. CBE Life Sci. Educ. 2018 , 17 , ar52. [ Google Scholar ] [ CrossRef ]
- Wighus, M.; Bjørk, I.T. An educational intervention to enhance clinical skills learning: Experiences of nursing students and teachers. Nurse Educ. Pract. 2018 , 29 , 143–149. [ Google Scholar ] [ CrossRef ]
- Alshehri, F.D.; Jone, S.; Harrison, D. The effectiveness of high-fidelity simulation on undergraduate nursing students’ clinical reasoning-related skills: A systematic review. Nurse Educ. Today 2023 , 121 , 105679. [ Google Scholar ] [ CrossRef ]
- Kim-Godwin, Y.S.; Livsey, K.R.; Ezzell, D.; Highsmith, C. Home Visit Simulation Using a Standardized Patient. Clin. Simul. Nurs. 2013 , 9 , e55–e61. [ Google Scholar ] [ CrossRef ]
- Wong, F.M.F. A phenomenological research study: Perspectives of student learning through small group work between undergraduate nursing students and educators. Nurse Educ. Today 2018 , 68 , 153–158. [ Google Scholar ] [ CrossRef ]
- Hober, C.; Bonnel, W. Student Perceptions of the Observer Role in High-Fidelity Simulation. Clin. Simul. Nurs. 2014 , 10 , 507–514. [ Google Scholar ] [ CrossRef ]
- Connor Desai, S.; Reimers, S. Comparing the use of open and closed questions for Web-based measures of the continued-influence effect. Behav. Res. Methods 2019 , 51 , 1426–1440. [ Google Scholar ] [ CrossRef ]
- Hyman, M.R.; Sierra, J.J. Open-versus close-ended survey questions. Bus. Outlook 2016 , 14 , 105. [ Google Scholar ]
Study Period | Control (by Facilitator A) | Intervention (by Facilitator B) | ||
---|---|---|---|---|
Learning objectives | Learning resources | Learning objectives | Learning resources | |
Pre-briefing | Course intended learning outcomes | |||
Observation period | Not specific |
Period | Questions |
---|---|
Post- pre-briefing | |
Post-role-play observation | According to your observation of the role-player during the HFPS, please provide answers to the following questions: |
Period | Observations |
---|---|
During the role-playing | Select a player; observe and comment his/her performance in the following areas for observation. |
The statements, opinions and data contained in all publications are solely those of the individual author(s) and contributor(s) and not of MDPI and/or the editor(s). MDPI and/or the editor(s) disclaim responsibility for any injury to people or property resulting from any ideas, methods, instructions or products referred to in the content. |
Share and Cite
Wong, F.M.F. How Students Learn during the Pre-Briefing and Observation of Facilitation in a High-Fidelity Patient Simulation: A Narrative Analysis. Healthcare 2024 , 12 , 1761. https://doi.org/10.3390/healthcare12171761
Wong FMF. How Students Learn during the Pre-Briefing and Observation of Facilitation in a High-Fidelity Patient Simulation: A Narrative Analysis. Healthcare . 2024; 12(17):1761. https://doi.org/10.3390/healthcare12171761
Wong, Florence M. F. 2024. "How Students Learn during the Pre-Briefing and Observation of Facilitation in a High-Fidelity Patient Simulation: A Narrative Analysis" Healthcare 12, no. 17: 1761. https://doi.org/10.3390/healthcare12171761
Article Metrics
Article access statistics, further information, mdpi initiatives, follow mdpi.
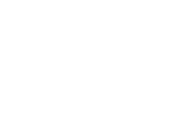
Subscribe to receive issue release notifications and newsletters from MDPI journals
The University of Chicago The Law School
Abrams environmental law clinic—significant achievements for 2023-24, protecting our great lakes, rivers, and shorelines.
The Abrams Clinic represents Friends of the Chicago River and the Sierra Club in their efforts to hold Trump Tower in downtown Chicago accountable for withdrawing water illegally from the Chicago River. To cool the building, Trump Tower draws water at high volumes, similar to industrial factories or power plants, but Trump Tower operated for more than a decade without ever conducting the legally required studies to determine the impact of those operations on aquatic life or without installing sufficient equipment to protect aquatic life consistent with federal regulations. After the Clinic sent a notice of intent to sue Trump Tower, the State of Illinois filed its own case in the summer of 2018, and the Clinic moved successfully to intervene in that case. In 2023-24, motions practice and discovery continued. Working with co-counsel at Northwestern University’s Pritzker Law School’s Environmental Advocacy Center, the Clinic moved to amend its complaint to include Trump Tower’s systematic underreporting each month of the volume of water that it intakes from and discharges to the Chicago River. The Clinic and co-counsel addressed Trump Tower’s motion to dismiss some of our clients’ claims, and we filed a motion for summary judgment on our claim that Trump Tower has committed a public nuisance. We also worked closely with our expert, Dr. Peter Henderson, on a supplemental disclosure and on defending an additional deposition of him. In summer 2024, the Clinic is defending its motion for summary judgment and challenging Trump Tower’s own motion for summary judgment. The Clinic is also preparing for trial, which could take place as early as fall 2024.
Since 2016, the Abrams Clinic has worked with the Chicago chapter of the Surfrider Foundation to protect water quality along the Lake Michigan shoreline in northwest Indiana, where its members surf. In April 2017, the U. S. Steel plant in Portage, Indiana, spilled approximately 300 pounds of hexavalent chromium into Lake Michigan. In January 2018, the Abrams Clinic filed a suit on behalf of Surfrider against U. S. Steel, alleging multiple violations of U. S. Steel’s discharge permits; the City of Chicago filed suit shortly after. When the US government and the State of Indiana filed their own, separate case, the Clinic filed extensive comments on the proposed consent decree. In August 2021, the court entered a revised consent decree which included provisions advocated for by Surfrider and the City of Chicago, namely a water sampling project that alerts beachgoers as to Lake Michigan’s water quality conditions, better notifications in case of future spills, and improvements to U. S. Steel’s operations and maintenance plans. In the 2023-24 academic year, the Clinic successfully litigated its claims for attorneys’ fees as a substantially prevailing party. Significantly, the court’s order adopted the “Fitzpatrick matrix,” used by the US Attorney’s Office for the District of Columbia to determine appropriate hourly rates for civil litigants, endorsed Chicago legal market rates as the appropriate rates for complex environmental litigation in Northwest Indiana, and allowed for partially reconstructed time records. The Clinic’s work, which has received significant media attention, helped to spawn other litigation to address pollution by other industrial facilities in Northwest Indiana and other enforcement against U. S. Steel by the State of Indiana.
In Winter Quarter 2024, Clinic students worked closely with Dr. John Ikerd, an agricultural economist and emeritus professor at the University of Missouri, to file an amicus brief in Food & Water Watch v. U.S. Environmental Protection Agency . In that case pending before the Ninth Circuit, Food & Water Watch argues that US EPA is illegally allowing Concentrated Animal Feeding Operations, more commonly known as factory farms, to pollute waterways significantly more than is allowable under the Clean Water Act. In the brief for Dr. Ikerd and co-amici Austin Frerick, Crawford Stewardship Project, Family Farm Defenders, Farm Aid, Missouri Rural Crisis Center, National Family Farm Coalition, National Sustainable Agriculture Coalition, and Western Organization of Resource Councils, we argued that EPA’s refusal to regulate CAFOs effectively is an unwarranted application of “agricultural exceptionalism” to industrial agriculture and that EPA effectively distorts the animal production market by allowing CAFOs to externalize their pollution costs and diminishing the ability of family farms to compete. Attorneys for the litigants will argue the case in September 2024.
Energy and Climate
Energy justice.
The Abrams Clinic supported grassroots organizations advocating for energy justice in low-income communities and Black, Indigenous, and People of Color (BIPOC) communities in Michigan. With the Clinic’s representation, these organizations intervened in cases before the Michigan Public Service Commission (MPSC), which regulates investor-owned utilities. Students conducted discovery, drafted written testimony, cross-examined utility executives, participated in settlement discussions, and filed briefs for these projects. The Clinic’s representation has elevated the concerns of these community organizations and forced both the utilities and regulators to consider issues of equity to an unprecedented degree. This year, on behalf of Soulardarity (Highland Park, MI), We Want Green, Too (Detroit, MI), and Urban Core Collective (Grand Rapids, MI), Clinic students engaged in eight contested cases before the MPSC against DTE Electric, DTE Gas, and Consumers Energy, as well as provided support for our clients’ advocacy in other non-contested MPSC proceedings.
The Clinic started this past fall with wins in three cases. First, the Clinic’s clients settled with DTE Electric in its Integrated Resource Plan case. The settlement included an agreement to close the second dirtiest coal power plant in Michigan three years early, $30 million from DTE’s shareholders to assist low-income customers in paying their bills, and $8 million from DTE’s shareholders toward a community fund that assists low-income customers with installing energy efficiency improvements, renewable energy, and battery technology. Second, in DTE Electric’s 2023 request for a rate hike (a “rate case”), the Commission required DTE Electric to develop a more robust environmental justice analysis and rejected the Company’s second attempt to waive consumer protections through a proposed electric utility prepayment program with a questionable history of success during its pilot run. The final Commission order and the administrative law judge’s proposal for final decision cited the Clinic’s testimony and briefs. Third, in Consumers Electric’s 2023 rate case, the Commission rejected the Company’s request for a higher ratepayer-funded return on its investments and required the Company to create a process that will enable intervenors to obtain accurate GIS data. The Clinic intends to use this data to map the disparate impact of infrastructure investment in low-income and BIPOC communities.
In the winter, the Clinic filed public comments regarding DTE Electric and Consumers Energy’s “distribution grid plans” (DGP) as well as supported interventions in two additional cases: Consumers Energy’s voluntary green pricing (VGP) case and the Clinic’s first case against the gas utility DTE Gas. Beginning with the DGP comments, the Clinic first addressed Consumers’s 2023 Electric Distribution Infrastructure Investment Plan (EDIIP), which detailed current distribution system health and the utility’s approximately $7 billion capital project planning ($2 billion of which went unaccounted for in the EDIIP) over 2023–2028. The Clinic then commented on DTE Electric’s 2023 DGP, which outlined the utility’s opaque project prioritization and planned more than $9 billion in capital investments and associated maintenance over 2024–2028. The comments targeted four areas of deficiencies in both the EDIIP and DGP: (1) inadequate consideration of distributed energy resources (DERs) as providing grid reliability, resiliency, and energy transition benefits; (2) flawed environmental justice analysis, particularly with respect to the collection of performance metrics and the narrow implementation of the Michigan Environmental Justice Screen Tool; (3) inequitable investment patterns across census tracts, with emphasis on DTE Electric’s skewed prioritization for retaining its old circuits rather than upgrading those circuits; and (4) failing to engage with community feedback.
For the VGP case against Consumers, the Clinic supported the filing of both an initial brief and reply brief requesting that the Commission reject the Company’s flawed proposal for a “community solar” program. In a prior case, the Clinic advocated for the development of a community solar program that would provide low-income, BIPOC communities with access to clean energy. As a result of our efforts, the Commission approved a settlement agreement requiring the Company “to evaluate and provide a strawman recommendation on community solar in its Voluntary Green Pricing Program.” However, the Company’s subsequent proposal in its VGP case violated the Commission’s order because it (1) was not consistent with the applicable law, MCL 460.1061; (2) was not a true community solar program; (3) lacked essential details; (4) failed to compensate subscribers sufficiently; (5) included overpriced and inflexible subscriptions; (6) excessively limited capacity; and (7) failed to provide a clear pathway for certain participants to transition into other VGP programs. For these reasons, the Clinic argued that the Commission should reject the Company’s proposal.
In DTE Gas’s current rate case, the Clinic worked with four witnesses to develop testimony that would rebut DTE Gas’s request for a rate hike on its customers. The testimony advocated for a pathway to a just energy transition that avoids dumping the costs of stranded gas assets on the low-income and BIPOC communities that are likely to be the last to electrify. Instead, the testimony proposed that the gas and electric utilities undertake integrated planning that would prioritize electric infrastructure over gas infrastructure investment to ensure that DTE Gas does not over-invest in gas infrastructure that will be rendered obsolete in the coming decades. The Clinic also worked with one expert witness to develop an analysis of DTE Gas’s unaffordable bills and inequitable shutoff, deposit, and collections practices. Lastly, the Clinic offered testimony on behalf of and from community members who would be directly impacted by the Company’s rate hike and lack of affordable and quality service. Clinic students have spent the summer drafting an approximately one-hundred-page brief making these arguments formally. We expect the Commission’s decision this fall.
Finally, both DTE Electric and Consumers Energy have filed additional requests for rate increases after the conclusion of their respective rate cases filed in 2023. On behalf of our Clients, the Clinic has intervened in these cases, and clinic students have already reviewed thousands of pages of documents and started to develop arguments and strategies to protect low-income and BIPOC communities from the utility’s ceaseless efforts to increase the cost of energy.
Corporate Climate Greenwashing
The Abrams Environmental Law Clinic worked with a leading international nonprofit dedicated to using the law to protect the environment to research corporate climate greenwashing, focusing on consumer protection, green financing, and securities liability. Clinic students spent the year examining an innovative state law, drafted a fifty-page guide to the statute and relevant cases, and examined how the law would apply to a variety of potential cases. Students then presented their findings in a case study and oral presentation to members of ClientEarth, including the organization’s North American head and members of its European team. The project helped identify the strengths and weaknesses of potential new strategies for increasing corporate accountability in the fight against climate change.
Land Contamination, Lead, and Hazardous Waste
The Abrams Clinic continues to represent East Chicago, Indiana, residents who live or lived on or adjacent to the USS Lead Superfund site. This year, the Clinic worked closely with the East Chicago/Calumet Coalition Community Advisory Group (CAG) to advance the CAG’s advocacy beyond the Superfund site and the adjacent Dupont RCRA site. Through multiple forms of advocacy, the clinics challenged the poor performance and permit modification and renewal attempts of Tradebe Treatment and Recycling, LLC (Tradebe), a hazardous waste storage and recycling facility in the community. Clinic students sent letters to US EPA and Indiana Department of Environmental Management officials about how IDEM has failed to assess meaningful penalties against Tradebe for repeated violations of the law and how IDEM has allowed Tradebe to continue to threaten public and worker health and safety by not improving its operations. Students also drafted substantial comments for the CAG on the US EPA’s Lead and Copper Rule improvements, the Suppliers’ Park proposed cleanup, and Sims Metal’s proposed air permit revisions. The Clinic has also continued working with the CAG, environmental experts, and regulators since US EPA awarded $200,000 to the CAG for community air monitoring. The Clinic and its clients also joined comments drafted by other environmental organizations about poor operations and loose regulatory oversight of several industrial facilities in the area.
Endangered Species
The Abrams Clinic represented the Center for Biological Diversity (CBD) and the Hoosier Environmental Council (HEC) in litigation regarding the US Fish and Wildlife Service’s (Service) failure to list the Kirtland’s snake as threatened or endangered under the Endangered Species Act. The Kirtland’s snake is a small, secretive, non-venomous snake historically located across the Midwest and the Ohio River Valley. Development and climate change have undermined large portions of the snake’s habitat, and populations are declining. Accordingly, the Clinic sued the Service in the US District Court for the District of Columbia last summer over the Service’s denial of CBD’s request to have the Kirtland’s snake protected. This spring, the Clinic was able to reach a settlement with the Service that requires the Service to reconsider its listing decision for the Kirtland’s snake and to pay attorney fees.
The Clinic also represented CBD in preparation for litigation regarding the Service’s failure to list another species as threatened or endangered. Threats from land development and climate change have devastated this species as well, and the species has already been extirpated from two of the sixteen US states in its range. As such, the Clinic worked this winter and spring to prepare a notice of intent (NOI) to sue the Service. The Team poured over hundreds of FOIA documents and dug into the Service’s supporting documentation to create strong arguments against the Service in the imminent litigation. The Clinic will send the NOI and file a complaint in the next few months.
Students and Faculty
Twenty-four law school students from the classes of 2024 and 2025 participated in the Clinic, performing complex legal research, reviewing documents obtained through discovery, drafting legal research memos and briefs, conferring with clients, conducting cross-examination, participating in settlement conferences, and arguing motions. Students secured nine clerkships, five were heading to private practice after graduation, and two are pursuing public interest work. Sam Heppell joined the Clinic from civil rights private practice, bringing the Clinic to its full complement of three attorneys.
- India Today
- Business Today
- Harper's Bazaar
- Brides Today
- Cosmopolitan
- India Today Hindi
- Reader’s Digest
- Aaj Tak Campus
Download App
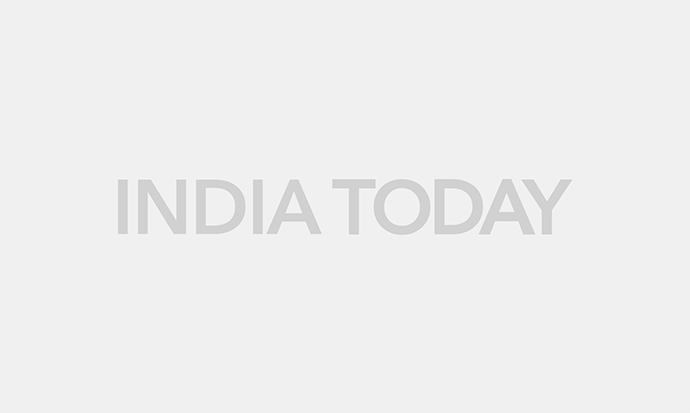
IC 814 hijack, indecision, and 'goof-ups': Former RAW chief on what went wrong
Speaking to india today tv, as dulat, the former chief of research and analysis wing (raw), admitted that there were "goof-ups" when it came to decision-making and the hijacked ic 814 plane shouldn't have been allowed to leave amritsar..
Listen to Story
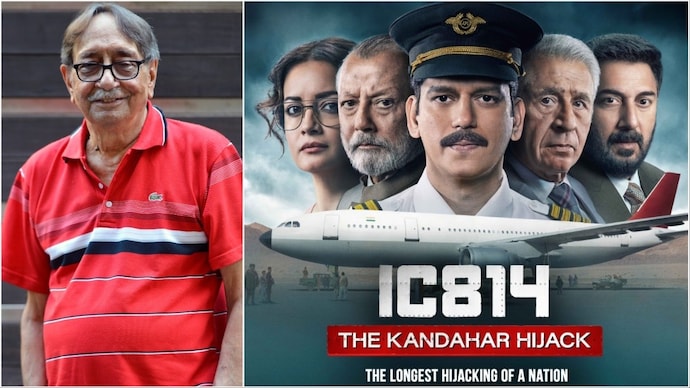
- Netflix series on IC 814 hijacking stirs controversy
- Ex-RAW chief AS Dulat admits decision-making 'goof-ups'
- Dulat says ISI definitely had a role in hijacking
The 1999 hijacking of an Indian Airlines flight, IC 814, has become a centre of controversy lately after the release of a mini-series on Netflix. The series has reignited debate on a number of issues, including the handling of the situation by the government and different agencies involved.
Speaking to India Today TV, AS Dulat, who was the chief of Research and Analysis Wing (RAW) in 1999, admitted that there were "goof-ups" when it came to decision-making.
"Once the plane landed in Amritsar, we had an opportunity to make sure that it didn't leave Indian territory. But when it left Amritsar, there was no other option but to make a deal. We did the best possible deal with the best possible negotiators," Dulat said.
Flight IC 814 of Indian Airlines, bound from Kathmandu to Delhi, was hijacked by five terrorists as soon as it entered Indian airspace on December 24, 1999. The plane landed in Amritsar for refuelling and was stationed for 50 minutes. Despite this, the authorities, including Punjab Police and central intel forces, could not capture the advantage.
"We were all there and should have taken a call. I don't want to name-blame; after so many years, it is not fair. I am also to blame as much as anybody else," said Dulat.
The former RAW chief talked about his long chat with then Director General of Police (DGP) for Punjab Sarabjit Singh over the hijack situation.
"I had a long chat with the Punjab DGP who told me that he was not KPS Gill, and he was not going to put his job on the line. He said the Chief Minister (Parkash Singh Badal) told him that he didn't want bloodshed in Amritsar. Even Delhi was indicating the same thing. The DGP said they could storm the plane, but they didn't know how many casualties could happen. So in the name of bloodshed, nobody wanted to take a call," he said.
Dulat said that the Punjab Police needed to be told that the aircraft should not leave Amritsar, which did not happen.
Interestingly, DGP Sarabjit Singh had said, on record, that he would have taken a decision if he had got clear instructions from Delhi.
ISI's ROLE IN 'IC 814' HIJACKING
When asked about the Inter-Services Intelligence's (ISI) role in the hijack, Dulat said Pakistan's spy agency was definitely involved.
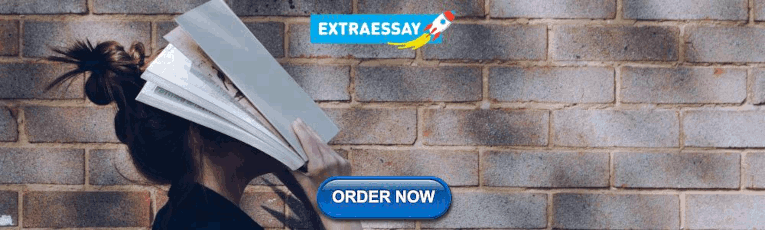
COMMENTS
5. Build Your Self-Confidence. Finally, learning through the case study method can build your confidence. Each time you assume a business leader's perspective, aim to solve a new challenge, and express and defend your opinions and decisions to peers, you prepare to do the same in your career. According to a 2022 City Square Associates survey ...
The Effective Decision-Making program was artfully designed to immerse senior leaders in 16 hours of hands-on experience, including reflection and feedback activities, applicable exercises, supporting content, and participation in a business simulation to practice the core content of the program.
It's been 100 years since Harvard Business School began using the case study method. Beyond teaching specific subject matter, the case study method excels in instilling meta-skills in students ...
Case studies are a form of problem-based learning, where you present a situation that needs a resolution. A typical business case study is a detailed account, or story, of what happened in a particular company, industry, or project over a set period of time. The learner is given details about the situation, often in a historical context.
Some of the case studies in this collection highlight the decision-making process in a business or management setting. Other cases are descriptive or demonstrative in nature, showcasing something that has happened or is happening in a particular business or management environment.
Case method 1 teaching is an active form of instruction that focuses on a case and involves students learning by doing 2 3. Cases are real or invented stories 4 that include "an educational message" or recount events, problems, dilemmas, theoretical or conceptual issue that requires analysis and/or decision-making.
The PACADI (Problem, Alternatives, Criteria, Analysis, Decision, Implementation) framework for analyzing cases helps students analyze business problems, make decisions, and develop strategy. Learn how it works, and what makes it an effective learning tool.
Case studies have been used for years by businesses, law and medical schools, physicians on rounds, and artists critiquing work. Like other forms of problem-based learning, case studies can be accessible for every age group, both in one subject and in interdisciplinary work.
Simply put, the case method is a discussion of real-life situations that business executives have faced. Harvard Business School. The Learning Experience. The Case Study Method. On average, you'll attend three to four different classes a day, for a total of about six hours of class time (schedules vary). To prepare, you'll work through problems ...
Using case studies and situations encountered by class members, you will explore proven, successful problem-solving and decision-making models and methods that can be readily transferred to workplace projects.
A major advantage of teaching with case studies is that the students are actively engaged in figuring out the principles by abstracting from the examples. This develops their skills in: Problem solving. Analytical tools, quantitative and/or qualitative, depending on the case. Decision making in complex situations.
Abstract Qualitative case study methodology enables researchers to conduct an in-depth exploration of intricate phenomena within some specific context. By keeping in mind research students, this article presents a systematic step-by-step guide to conduct a case study in the business discipline. Research students belonging to said discipline face issues in terms of clarity, selection, and ...
Discover real-world case studies of Data-Driven Decision-Making (DDDM) and how companies like Netflix, Amazon, and Starbucks leveraged data for success.
To help educators facilitate this shift and help students get the most out of case-based learning, we have developed a framework for analyzing cases. We call it PACADI (Problem, Alternatives, Criteria, Analysis, Decision, Implementation); it can improve learning outcomes by helping students better solve and analyze business problems, make decisions, and develop and implement strategy. Here, we ...
Decision Making New research on decision making from Harvard Business School faculty on issues including policymaking, approaches to common work problems, and predicting behavior.
A case study, or case report, is a concise examination of a specific subject, often real-world situations or problems, providing detailed insights and analysis for learning or decision-making purposes.
In Praise of Uncertainty. Decision making and problem solving Magazine Article. Jonathan Zittrain. The information technology explosion was set off by visionaries who thrive on uncertainty. Today ...
Organizational decision making Magazine Article. Stanley M. Davis. Paul R. Lawrence. No organization design or method of management is perfect. And any form can suffer from a variety of problems ...
Double cycle of strategic decision making - case study of e-learning (including ODL) Summary of strategic decision making cycle in HE Figures - uploaded by Blaženka Divjak Author content
Learn how to enhance your decision-making skills with an active learning approach in this research paper by Paul Greenbank.
This project is a powerful example of how data science can transform a business by unlocking new insights, increasing efficiency, and improving decision-making. I hope that this case study will help you to think about the potential applications in your organization and showcase how you can apply the process model DASC-PM successfully.
In this article, we'll provide a step-by-step guide on how to teach your students the necessary skills for effective decision-making. You'll learn: How character education fosters decision-making skills. Techniques to foster personal responsibility and autonomy through decision-making. Teaching methods to develop analytical thinking in students.
Author summary When we give more weight to information that confirms our existing beliefs, it typically has a negative impact on learning and decision-making. However, our study shows that a moderate confirmation bias can actually improve decision-making when multiple reinforcement learning agents learn together in a social context. This finding has important implications for policymakers who ...
Feedback from learners is important to support faculty development, but negative feedback can harm teachers' motivation, engagement, and retention. Leaders of educational programs, therefore, need to balance enabling students' voices to be heard with maintaining teachers' enthusiasm and commitment to teaching. Given the paucity of research to explain or guide this struggle, we explored ...
By enhancing decision-making processes and providing forward-looking guidance to investors, our computational model aims to empower both campaign creators and platform administrators, ultimately improving the overall efficacy and sustainability of crowdfunding as a financing tool. ... This study leveraged advanced machine learning techniques to ...
The importance of this type of model lies in informed decision-making when practising precision agriculture, as a variety of external factors influence crop performance. ... Adewopo J. Comparison of UAV and SAR performance for Crop type classification using machine learning algorithms: a case study of humid forest ecology experimental research ...
Abstract. In complex industrial and chemical process control rooms, effective decision-making is crucial for safety and efficiency. The experiments in this paper evaluate the impact and applications of an AI-based decision support system integrated into an improved human-machine interface, using dynamic influence diagrams, a hidden Markov model, and deep reinforcement learning.
The rapid development of technology has led to the emergence of innovative teaching approaches, such as high-fidelity patient simulation (HFPS). HFPSs have been shown to significantly enhance students' decision-making and intellectual skills. This study aimed to investigate how students learn from the pre-briefing to observation period of the facilitation of the HFPS based on the original ...
Students then presented their findings in a case study and oral presentation to members of ClientEarth, including the organization's North American head and members of its European team. The project helped identify the strengths and weaknesses of potential new strategies for increasing corporate accountability in the fight against climate change.
Speaking to India Today TV, AS Dulat, the former chief of Research and Analysis Wing (RAW), admitted that there were "goof-ups" when it came to decision-making and the hijacked IC 814 plane shouldn't have been allowed to leave Amritsar. Listen to Story Netflix series on IC 814 hijacking stirs ...