Purpose and Limitations of Random Assignment
In an experimental study, random assignment is a process by which participants are assigned, with the same chance, to either a treatment or a control group. The goal is to assure an unbiased assignment of participants to treatment options.
Random assignment is considered the gold standard for achieving comparability across study groups, and therefore is the best method for inferring a causal relationship between a treatment (or intervention or risk factor) and an outcome.
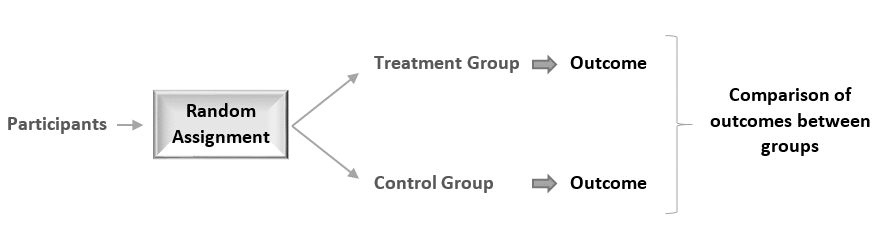
Random assignment of participants produces comparable groups regarding the participants’ initial characteristics, thereby any difference detected in the end between the treatment and the control group will be due to the effect of the treatment alone.
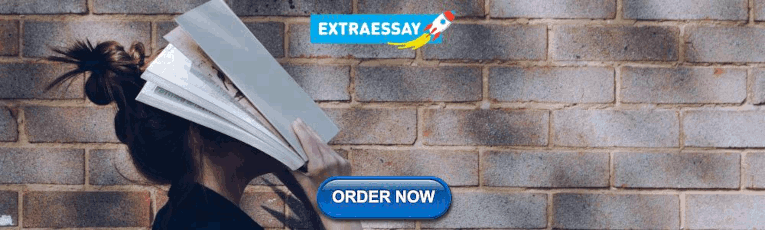
How does random assignment produce comparable groups?
1. random assignment prevents selection bias.
Randomization works by removing the researcher’s and the participant’s influence on the treatment allocation. So the allocation can no longer be biased since it is done at random, i.e. in a non-predictable way.
This is in contrast with the real world, where for example, the sickest people are more likely to receive the treatment.
2. Random assignment prevents confounding
A confounding variable is one that is associated with both the intervention and the outcome, and thus can affect the outcome in 2 ways:
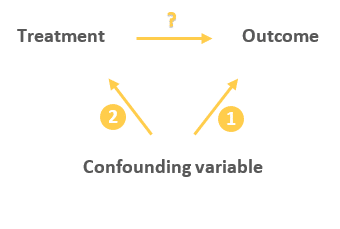
Either directly:
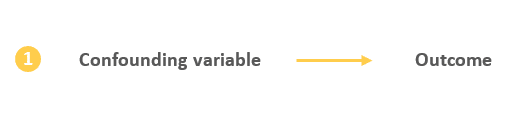
Or indirectly through the treatment:

This indirect relationship between the confounding variable and the outcome can cause the treatment to appear to have an influence on the outcome while in reality the treatment is just a mediator of that effect (as it happens to be on the causal pathway between the confounder and the outcome).
Random assignment eliminates the influence of the confounding variables on the treatment since it distributes them at random between the study groups, therefore, ruling out this alternative path or explanation of the outcome.
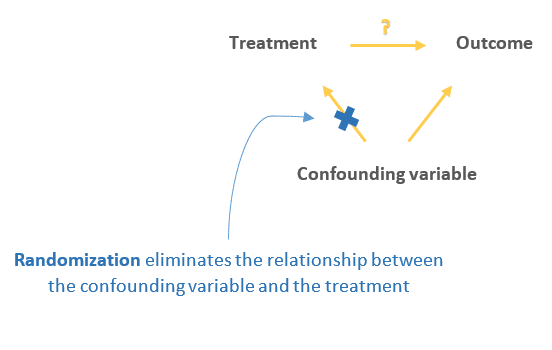
3. Random assignment also eliminates other threats to internal validity
By distributing all threats (known and unknown) at random between study groups, participants in both the treatment and the control group become equally subject to the effect of any threat to validity. Therefore, comparing the outcome between the 2 groups will bypass the effect of these threats and will only reflect the effect of the treatment on the outcome.
These threats include:
- History: This is any event that co-occurs with the treatment and can affect the outcome.
- Maturation: This is the effect of time on the study participants (e.g. participants becoming wiser, hungrier, or more stressed with time) which might influence the outcome.
- Regression to the mean: This happens when the participants’ outcome score is exceptionally good on a pre-treatment measurement, so the post-treatment measurement scores will naturally regress toward the mean — in simple terms, regression happens since an exceptional performance is hard to maintain. This effect can bias the study since it represents an alternative explanation of the outcome.
Note that randomization does not prevent these effects from happening, it just allows us to control them by reducing their risk of being associated with the treatment.
What if random assignment produced unequal groups?
Question: What should you do if after randomly assigning participants, it turned out that the 2 groups still differ in participants’ characteristics? More precisely, what if randomization accidentally did not balance risk factors that can be alternative explanations between the 2 groups? (For example, if one group includes more male participants, or sicker, or older people than the other group).
Short answer: This is perfectly normal, since randomization only assures an unbiased assignment of participants to groups, i.e. it produces comparable groups, but it does not guarantee the equality of these groups.
A more complete answer: Randomization will not and cannot create 2 equal groups regarding each and every characteristic. This is because when dealing with randomization there is still an element of luck. If you want 2 perfectly equal groups, you better match them manually as is done in a matched pairs design (for more information see my article on matched pairs design ).
This is similar to throwing a die: If you throw it 10 times, the chance of getting a specific outcome will not be 1/6. But it will approach 1/6 if you repeat the experiment a very large number of times and calculate the average number of times the specific outcome turned up.
So randomization will not produce perfectly equal groups for each specific study, especially if the study has a small sample size. But do not forget that scientific evidence is a long and continuous process, and the groups will tend to be equal in the long run when a meta-analysis aggregates the results of a large number of randomized studies.
So for each individual study, differences between the treatment and control group will exist and will influence the study results. This means that the results of a randomized trial will sometimes be wrong, and this is absolutely okay.
BOTTOM LINE:
Although the results of a particular randomized study are unbiased, they will still be affected by a sampling error due to chance. But the real benefit of random assignment will be when data is aggregated in a meta-analysis.
Limitations of random assignment
Randomized designs can suffer from:
1. Ethical issues:
Randomization is ethical only if the researcher has no evidence that one treatment is superior to the other.
Also, it would be unethical to randomly assign participants to harmful exposures such as smoking or dangerous chemicals.
2. Low external validity:
With random assignment, external validity (i.e. the generalizability of the study results) is compromised because the results of a study that uses random assignment represent what would happen under “ideal” experimental conditions, which is in general very different from what happens at the population level.
In the real world, people who take the treatment might be very different from those who don’t – so the assignment of participants is not a random event, but rather under the influence of all sort of external factors.
External validity can be also jeopardized in cases where not all participants are eligible or willing to accept the terms of the study.
3. Higher cost of implementation:
An experimental design with random assignment is typically more expensive than observational studies where the investigator’s role is just to observe events without intervening.
Experimental designs also typically take a lot of time to implement, and therefore are less practical when a quick answer is needed.
4. Impracticality when answering non-causal questions:
A randomized trial is our best bet when the question is to find the causal effect of a treatment or a risk factor.
Sometimes however, the researcher is just interested in predicting the probability of an event or a disease given some risk factors. In this case, the causal relationship between these variables is not important, making observational designs more suitable for such problems.
5. Impracticality when studying the effect of variables that cannot be manipulated:
The usual objective of studying the effects of risk factors is to propose recommendations that involve changing the level of exposure to these factors.
However, some risk factors cannot be manipulated, and so it does not make any sense to study them in a randomized trial. For example it would be impossible to randomly assign participants to age categories, gender, or genetic factors.
6. Difficulty to control participants:
These difficulties include:
- Participants refusing to receive the assigned treatment.
- Participants not adhering to recommendations.
- Differential loss to follow-up between those who receive the treatment and those who don’t.
All of these issues might occur in a randomized trial, but might not affect an observational study.
- Shadish WR, Cook TD, Campbell DT. Experimental and Quasi-Experimental Designs for Generalized Causal Inference . 2nd edition. Cengage Learning; 2001.
- Friedman LM, Furberg CD, DeMets DL, Reboussin DM, Granger CB. Fundamentals of Clinical Trials . 5th ed. 2015 edition. Springer; 2015.
Further reading
- Posttest-Only Control Group Design
- Pretest-Posttest Control Group Design
- Randomized Block Design
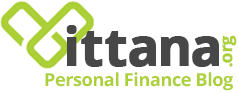
17 Advantages and Disadvantages of Random Sampling
The goal of random sampling is simple. It helps researchers avoid an unconscious bias they may have that would be reflected in the data they are collecting. This advantage, however, is offset by the fact that random sampling prevents researchers from being able to use any prior information they may have collected.
This means random sampling allows for unbiased estimates to be created, but at the cost of efficiency within the research process.
Here are some of the additional advantages and disadvantages of random sampling that worth considering.
What Are the Advantages of Random Sampling?
1. It offers a chance to perform data analysis that has less risk of carrying an error. Random sampling allows researchers to perform an analysis of the data that is collected with a lower margin of error. This is allowed because the sampling occurs within specific boundaries that dictate the sampling process. Because the whole process is randomized, the random sample reflects the entire population and this allows the data to provide accurate insights into specific subject matters.
2. There is an equal chance of selection. Random sampling allows everyone or everything within a defined region to have an equal chance of being selected. This helps to create more accuracy within the data collected because everyone and everything has a 50/50 opportunity. It is a process that builds an inherent “fairness” into the research being conducted because no previous information about the individuals or items involved are included in the data collection process.
3. It requires less knowledge to complete the research. A researcher does not need to have specific knowledge about the data being collected to be effective at their job. Researchers could ask someone who they prefer to be the next President of the United States without knowing anything about US political structures. In random sampling, a question is asked and then answered. An item is reviewed for a specific feature. If the researcher can perform that task and collect the data, then they’ve done their job.
4. It is the simplest form of data collection. This type of research involves basic observation and recording skills. It requires no basic skills out of the population base or the items being researched. It also removes any classification errors that may be involved if other forms of data collection were being used. Although the simplicity can cause some unintended problems when a sample is not a genuine reflection of the average population being reviewed, the data collected is generally reliable and accurate.
5. Multiple types of randomness can be included to reduce researcher bias. There are two common approaches that are used for random sampling to limit any potential bias in the data. The first is a lottery method, which involves having a population group drawing to see who will be included and who will not. Researchers can also use random numbers that are assigned to specific individuals and then have a random collection of those number selected to be part of the project.
6. It is easier to form sample groups. Because random sampling takes a few from a large population, the ease of forming a sample group out of the larger frame is incredibly easy. This makes it possible to begin the process of data collection faster than other forms of data collection may allow.
7. Findings can be applied to the entire population base. Because of the processes that allow for random sampling, the data collected can produce results for the larger frame because there is such little relevance of bias within the findings. The generalized representation that is present allows for research findings to be equally generalized.
What Are the Disadvantages of Random Sampling?
1. No additional knowledge is taken into consideration. Although random sampling removes an unconscious bias that exists, it does not remove an intentional bias from the process. Researchers can choose regions for random sampling where they believe specific results can be obtained to support their own personal bias. No additional knowledge is given consideration from the random sampling, but the additional knowledge offered by the researcher gathering the data is not always removed.
2. It is a complex and time-consuming method of research. With random sampling, every person or thing must be individually interviewed or reviewed so that the data can be properly collected. When individuals are in groups, their answers tend to be influenced by the answers of others. This means a researcher must work with every individual on a 1-on-1 basis. This requires more resources, reduces efficiencies, and takes more time than other research methods when it is done correctly.
3. Researchers are required to have experience and a high skill level. A researcher may not be required to have specific knowledge to conduct random sampling successfully, but they do need to be experienced in the process of data collection. There must be an awareness by the researcher when conducting 1-on-1 interviews that the data being offered is accurate or not. A high skill level is required of the researcher so they can separate accurate data that has been collected from inaccurate data. If that skill is not present, the accuracy of the conclusions produced by the offered data may be brought into question.
4. There is an added monetary cost to the process. Because the research must happen at the individual level, there is an added monetary cost to random sampling when compared to other data collection methods. There is an added time cost that must be included with the research process as well. The results, when collected accurately, can be highly beneficial to those who are going to use the data, but the monetary cost of the research may outweigh the actual gains that can be obtained from solutions created from the data.
5. No guarantee that the results will be universal is offered. Random sampling is designed to be a representation of a community or demographic, but there is no guarantee that the data collected is reflective of the community on average. In US politics, a random sample might collect 6 Democrats, 3 Republicans, and 1 Independents, though the actual population base might be 6 Republicans, 3 Democrats, and 1 Independent for every 10 people in the community. Asking who they want to be their President would likely have a Democratic candidate in the lead when the whole community would likely prefer the Republican.
6. It requires population grouping to be effective. If the population being surveyed is diverse in its character and content, or it is widely dispersed, then the information collected may not serve as an accurate representation of the entire population. These issues also make it difficult to contact specific groups or people to have them included in the research or to properly catalog the data so that it can serve its purpose.
7. It is easy to get the data wrong just as it is easy to get right. The application of random sampling is only effective when all potential respondents are included within the large sampling frame. Everyone or everything that is within the demographic or group being analyzed must be included for the random sampling to be accurate. If the sampling frame is exclusionary, even in a way that is unintended, then the effectiveness of the data can be called into question and the results can no longer be generalized to the larger group.
8. A large sample size is mandatory. For random sampling to work, there must be a large population group from which sampling can take place. It would be possible to draw conclusions for 1,000 people by including a random sample of 50. It would not be possible to draw conclusions for 10 people by randomly selecting two people. A large sample size is always necessary, but some demographics or groups may not have a large enough frame to support the methodology offered by random sampling.
9. A sample size that is too large is also problematic. Since every member is given an equal chance at participation through random sampling, a population size that is too large can be just as problematic as a population size that is too small. Larger populations require larger frames that still demand accuracy, which means errors can creep into the data as the size of the frame increases.
10. The quality of the data is reliant on the quality of the researcher. This potential negative is especially true when the data being collected comes through face-to-face interviews. A poor interviewer would collect less data than an experienced interviewer. An interviewer who refuses to stick to a script of questions and decides to freelance on follow-ups may create biased data through their efforts. Poor research methods will always result in poor data.
The advantages and disadvantages of random sampling show that it can be quite effective when it is performed correctly. Random sampling removes an unconscious bias while creating data that can be analyzed to benefit the general demographic or population group being studied. If controls can be in place to remove purposeful manipulation of the data and compensate for the other potential negatives present, then random sampling is an effective form of research.

Want to create or adapt books like this? Learn more about how Pressbooks supports open publishing practices.
6.2 Experimental Design
Learning objectives.
- Explain the difference between between-subjects and within-subjects experiments, list some of the pros and cons of each approach, and decide which approach to use to answer a particular research question.
- Define random assignment, distinguish it from random sampling, explain its purpose in experimental research, and use some simple strategies to implement it.
- Define what a control condition is, explain its purpose in research on treatment effectiveness, and describe some alternative types of control conditions.
- Define several types of carryover effect, give examples of each, and explain how counterbalancing helps to deal with them.
In this section, we look at some different ways to design an experiment. The primary distinction we will make is between approaches in which each participant experiences one level of the independent variable and approaches in which each participant experiences all levels of the independent variable. The former are called between-subjects experiments and the latter are called within-subjects experiments.
Between-Subjects Experiments
In a between-subjects experiment , each participant is tested in only one condition. For example, a researcher with a sample of 100 college students might assign half of them to write about a traumatic event and the other half write about a neutral event. Or a researcher with a sample of 60 people with severe agoraphobia (fear of open spaces) might assign 20 of them to receive each of three different treatments for that disorder. It is essential in a between-subjects experiment that the researcher assign participants to conditions so that the different groups are, on average, highly similar to each other. Those in a trauma condition and a neutral condition, for example, should include a similar proportion of men and women, and they should have similar average intelligence quotients (IQs), similar average levels of motivation, similar average numbers of health problems, and so on. This is a matter of controlling these extraneous participant variables across conditions so that they do not become confounding variables.
Random Assignment
The primary way that researchers accomplish this kind of control of extraneous variables across conditions is called random assignment , which means using a random process to decide which participants are tested in which conditions. Do not confuse random assignment with random sampling. Random sampling is a method for selecting a sample from a population, and it is rarely used in psychological research. Random assignment is a method for assigning participants in a sample to the different conditions, and it is an important element of all experimental research in psychology and other fields too.
In its strictest sense, random assignment should meet two criteria. One is that each participant has an equal chance of being assigned to each condition (e.g., a 50% chance of being assigned to each of two conditions). The second is that each participant is assigned to a condition independently of other participants. Thus one way to assign participants to two conditions would be to flip a coin for each one. If the coin lands heads, the participant is assigned to Condition A, and if it lands tails, the participant is assigned to Condition B. For three conditions, one could use a computer to generate a random integer from 1 to 3 for each participant. If the integer is 1, the participant is assigned to Condition A; if it is 2, the participant is assigned to Condition B; and if it is 3, the participant is assigned to Condition C. In practice, a full sequence of conditions—one for each participant expected to be in the experiment—is usually created ahead of time, and each new participant is assigned to the next condition in the sequence as he or she is tested. When the procedure is computerized, the computer program often handles the random assignment.
One problem with coin flipping and other strict procedures for random assignment is that they are likely to result in unequal sample sizes in the different conditions. Unequal sample sizes are generally not a serious problem, and you should never throw away data you have already collected to achieve equal sample sizes. However, for a fixed number of participants, it is statistically most efficient to divide them into equal-sized groups. It is standard practice, therefore, to use a kind of modified random assignment that keeps the number of participants in each group as similar as possible. One approach is block randomization . In block randomization, all the conditions occur once in the sequence before any of them is repeated. Then they all occur again before any of them is repeated again. Within each of these “blocks,” the conditions occur in a random order. Again, the sequence of conditions is usually generated before any participants are tested, and each new participant is assigned to the next condition in the sequence. Table 6.2 “Block Randomization Sequence for Assigning Nine Participants to Three Conditions” shows such a sequence for assigning nine participants to three conditions. The Research Randomizer website ( http://www.randomizer.org ) will generate block randomization sequences for any number of participants and conditions. Again, when the procedure is computerized, the computer program often handles the block randomization.
Table 6.2 Block Randomization Sequence for Assigning Nine Participants to Three Conditions
Participant | Condition |
---|---|
4 | B |
5 | C |
6 | A |
Random assignment is not guaranteed to control all extraneous variables across conditions. It is always possible that just by chance, the participants in one condition might turn out to be substantially older, less tired, more motivated, or less depressed on average than the participants in another condition. However, there are some reasons that this is not a major concern. One is that random assignment works better than one might expect, especially for large samples. Another is that the inferential statistics that researchers use to decide whether a difference between groups reflects a difference in the population takes the “fallibility” of random assignment into account. Yet another reason is that even if random assignment does result in a confounding variable and therefore produces misleading results, this is likely to be detected when the experiment is replicated. The upshot is that random assignment to conditions—although not infallible in terms of controlling extraneous variables—is always considered a strength of a research design.
Treatment and Control Conditions
Between-subjects experiments are often used to determine whether a treatment works. In psychological research, a treatment is any intervention meant to change people’s behavior for the better. This includes psychotherapies and medical treatments for psychological disorders but also interventions designed to improve learning, promote conservation, reduce prejudice, and so on. To determine whether a treatment works, participants are randomly assigned to either a treatment condition , in which they receive the treatment, or a control condition , in which they do not receive the treatment. If participants in the treatment condition end up better off than participants in the control condition—for example, they are less depressed, learn faster, conserve more, express less prejudice—then the researcher can conclude that the treatment works. In research on the effectiveness of psychotherapies and medical treatments, this type of experiment is often called a randomized clinical trial .
There are different types of control conditions. In a no-treatment control condition , participants receive no treatment whatsoever. One problem with this approach, however, is the existence of placebo effects. A placebo is a simulated treatment that lacks any active ingredient or element that should make it effective, and a placebo effect is a positive effect of such a treatment. Many folk remedies that seem to work—such as eating chicken soup for a cold or placing soap under the bedsheets to stop nighttime leg cramps—are probably nothing more than placebos. Although placebo effects are not well understood, they are probably driven primarily by people’s expectations that they will improve. Having the expectation to improve can result in reduced stress, anxiety, and depression, which can alter perceptions and even improve immune system functioning (Price, Finniss, & Benedetti, 2008).
Placebo effects are interesting in their own right (see Note 6.28 “The Powerful Placebo” ), but they also pose a serious problem for researchers who want to determine whether a treatment works. Figure 6.2 “Hypothetical Results From a Study Including Treatment, No-Treatment, and Placebo Conditions” shows some hypothetical results in which participants in a treatment condition improved more on average than participants in a no-treatment control condition. If these conditions (the two leftmost bars in Figure 6.2 “Hypothetical Results From a Study Including Treatment, No-Treatment, and Placebo Conditions” ) were the only conditions in this experiment, however, one could not conclude that the treatment worked. It could be instead that participants in the treatment group improved more because they expected to improve, while those in the no-treatment control condition did not.
Figure 6.2 Hypothetical Results From a Study Including Treatment, No-Treatment, and Placebo Conditions

Fortunately, there are several solutions to this problem. One is to include a placebo control condition , in which participants receive a placebo that looks much like the treatment but lacks the active ingredient or element thought to be responsible for the treatment’s effectiveness. When participants in a treatment condition take a pill, for example, then those in a placebo control condition would take an identical-looking pill that lacks the active ingredient in the treatment (a “sugar pill”). In research on psychotherapy effectiveness, the placebo might involve going to a psychotherapist and talking in an unstructured way about one’s problems. The idea is that if participants in both the treatment and the placebo control groups expect to improve, then any improvement in the treatment group over and above that in the placebo control group must have been caused by the treatment and not by participants’ expectations. This is what is shown by a comparison of the two outer bars in Figure 6.2 “Hypothetical Results From a Study Including Treatment, No-Treatment, and Placebo Conditions” .
Of course, the principle of informed consent requires that participants be told that they will be assigned to either a treatment or a placebo control condition—even though they cannot be told which until the experiment ends. In many cases the participants who had been in the control condition are then offered an opportunity to have the real treatment. An alternative approach is to use a waitlist control condition , in which participants are told that they will receive the treatment but must wait until the participants in the treatment condition have already received it. This allows researchers to compare participants who have received the treatment with participants who are not currently receiving it but who still expect to improve (eventually). A final solution to the problem of placebo effects is to leave out the control condition completely and compare any new treatment with the best available alternative treatment. For example, a new treatment for simple phobia could be compared with standard exposure therapy. Because participants in both conditions receive a treatment, their expectations about improvement should be similar. This approach also makes sense because once there is an effective treatment, the interesting question about a new treatment is not simply “Does it work?” but “Does it work better than what is already available?”
The Powerful Placebo
Many people are not surprised that placebos can have a positive effect on disorders that seem fundamentally psychological, including depression, anxiety, and insomnia. However, placebos can also have a positive effect on disorders that most people think of as fundamentally physiological. These include asthma, ulcers, and warts (Shapiro & Shapiro, 1999). There is even evidence that placebo surgery—also called “sham surgery”—can be as effective as actual surgery.
Medical researcher J. Bruce Moseley and his colleagues conducted a study on the effectiveness of two arthroscopic surgery procedures for osteoarthritis of the knee (Moseley et al., 2002). The control participants in this study were prepped for surgery, received a tranquilizer, and even received three small incisions in their knees. But they did not receive the actual arthroscopic surgical procedure. The surprising result was that all participants improved in terms of both knee pain and function, and the sham surgery group improved just as much as the treatment groups. According to the researchers, “This study provides strong evidence that arthroscopic lavage with or without débridement [the surgical procedures used] is not better than and appears to be equivalent to a placebo procedure in improving knee pain and self-reported function” (p. 85).

Research has shown that patients with osteoarthritis of the knee who receive a “sham surgery” experience reductions in pain and improvement in knee function similar to those of patients who receive a real surgery.
Army Medicine – Surgery – CC BY 2.0.
Within-Subjects Experiments
In a within-subjects experiment , each participant is tested under all conditions. Consider an experiment on the effect of a defendant’s physical attractiveness on judgments of his guilt. Again, in a between-subjects experiment, one group of participants would be shown an attractive defendant and asked to judge his guilt, and another group of participants would be shown an unattractive defendant and asked to judge his guilt. In a within-subjects experiment, however, the same group of participants would judge the guilt of both an attractive and an unattractive defendant.
The primary advantage of this approach is that it provides maximum control of extraneous participant variables. Participants in all conditions have the same mean IQ, same socioeconomic status, same number of siblings, and so on—because they are the very same people. Within-subjects experiments also make it possible to use statistical procedures that remove the effect of these extraneous participant variables on the dependent variable and therefore make the data less “noisy” and the effect of the independent variable easier to detect. We will look more closely at this idea later in the book.
Carryover Effects and Counterbalancing
The primary disadvantage of within-subjects designs is that they can result in carryover effects. A carryover effect is an effect of being tested in one condition on participants’ behavior in later conditions. One type of carryover effect is a practice effect , where participants perform a task better in later conditions because they have had a chance to practice it. Another type is a fatigue effect , where participants perform a task worse in later conditions because they become tired or bored. Being tested in one condition can also change how participants perceive stimuli or interpret their task in later conditions. This is called a context effect . For example, an average-looking defendant might be judged more harshly when participants have just judged an attractive defendant than when they have just judged an unattractive defendant. Within-subjects experiments also make it easier for participants to guess the hypothesis. For example, a participant who is asked to judge the guilt of an attractive defendant and then is asked to judge the guilt of an unattractive defendant is likely to guess that the hypothesis is that defendant attractiveness affects judgments of guilt. This could lead the participant to judge the unattractive defendant more harshly because he thinks this is what he is expected to do. Or it could make participants judge the two defendants similarly in an effort to be “fair.”
Carryover effects can be interesting in their own right. (Does the attractiveness of one person depend on the attractiveness of other people that we have seen recently?) But when they are not the focus of the research, carryover effects can be problematic. Imagine, for example, that participants judge the guilt of an attractive defendant and then judge the guilt of an unattractive defendant. If they judge the unattractive defendant more harshly, this might be because of his unattractiveness. But it could be instead that they judge him more harshly because they are becoming bored or tired. In other words, the order of the conditions is a confounding variable. The attractive condition is always the first condition and the unattractive condition the second. Thus any difference between the conditions in terms of the dependent variable could be caused by the order of the conditions and not the independent variable itself.
There is a solution to the problem of order effects, however, that can be used in many situations. It is counterbalancing , which means testing different participants in different orders. For example, some participants would be tested in the attractive defendant condition followed by the unattractive defendant condition, and others would be tested in the unattractive condition followed by the attractive condition. With three conditions, there would be six different orders (ABC, ACB, BAC, BCA, CAB, and CBA), so some participants would be tested in each of the six orders. With counterbalancing, participants are assigned to orders randomly, using the techniques we have already discussed. Thus random assignment plays an important role in within-subjects designs just as in between-subjects designs. Here, instead of randomly assigning to conditions, they are randomly assigned to different orders of conditions. In fact, it can safely be said that if a study does not involve random assignment in one form or another, it is not an experiment.
There are two ways to think about what counterbalancing accomplishes. One is that it controls the order of conditions so that it is no longer a confounding variable. Instead of the attractive condition always being first and the unattractive condition always being second, the attractive condition comes first for some participants and second for others. Likewise, the unattractive condition comes first for some participants and second for others. Thus any overall difference in the dependent variable between the two conditions cannot have been caused by the order of conditions. A second way to think about what counterbalancing accomplishes is that if there are carryover effects, it makes it possible to detect them. One can analyze the data separately for each order to see whether it had an effect.
When 9 Is “Larger” Than 221
Researcher Michael Birnbaum has argued that the lack of context provided by between-subjects designs is often a bigger problem than the context effects created by within-subjects designs. To demonstrate this, he asked one group of participants to rate how large the number 9 was on a 1-to-10 rating scale and another group to rate how large the number 221 was on the same 1-to-10 rating scale (Birnbaum, 1999). Participants in this between-subjects design gave the number 9 a mean rating of 5.13 and the number 221 a mean rating of 3.10. In other words, they rated 9 as larger than 221! According to Birnbaum, this is because participants spontaneously compared 9 with other one-digit numbers (in which case it is relatively large) and compared 221 with other three-digit numbers (in which case it is relatively small).
Simultaneous Within-Subjects Designs
So far, we have discussed an approach to within-subjects designs in which participants are tested in one condition at a time. There is another approach, however, that is often used when participants make multiple responses in each condition. Imagine, for example, that participants judge the guilt of 10 attractive defendants and 10 unattractive defendants. Instead of having people make judgments about all 10 defendants of one type followed by all 10 defendants of the other type, the researcher could present all 20 defendants in a sequence that mixed the two types. The researcher could then compute each participant’s mean rating for each type of defendant. Or imagine an experiment designed to see whether people with social anxiety disorder remember negative adjectives (e.g., “stupid,” “incompetent”) better than positive ones (e.g., “happy,” “productive”). The researcher could have participants study a single list that includes both kinds of words and then have them try to recall as many words as possible. The researcher could then count the number of each type of word that was recalled. There are many ways to determine the order in which the stimuli are presented, but one common way is to generate a different random order for each participant.
Between-Subjects or Within-Subjects?
Almost every experiment can be conducted using either a between-subjects design or a within-subjects design. This means that researchers must choose between the two approaches based on their relative merits for the particular situation.
Between-subjects experiments have the advantage of being conceptually simpler and requiring less testing time per participant. They also avoid carryover effects without the need for counterbalancing. Within-subjects experiments have the advantage of controlling extraneous participant variables, which generally reduces noise in the data and makes it easier to detect a relationship between the independent and dependent variables.
A good rule of thumb, then, is that if it is possible to conduct a within-subjects experiment (with proper counterbalancing) in the time that is available per participant—and you have no serious concerns about carryover effects—this is probably the best option. If a within-subjects design would be difficult or impossible to carry out, then you should consider a between-subjects design instead. For example, if you were testing participants in a doctor’s waiting room or shoppers in line at a grocery store, you might not have enough time to test each participant in all conditions and therefore would opt for a between-subjects design. Or imagine you were trying to reduce people’s level of prejudice by having them interact with someone of another race. A within-subjects design with counterbalancing would require testing some participants in the treatment condition first and then in a control condition. But if the treatment works and reduces people’s level of prejudice, then they would no longer be suitable for testing in the control condition. This is true for many designs that involve a treatment meant to produce long-term change in participants’ behavior (e.g., studies testing the effectiveness of psychotherapy). Clearly, a between-subjects design would be necessary here.
Remember also that using one type of design does not preclude using the other type in a different study. There is no reason that a researcher could not use both a between-subjects design and a within-subjects design to answer the same research question. In fact, professional researchers often do exactly this.
Key Takeaways
- Experiments can be conducted using either between-subjects or within-subjects designs. Deciding which to use in a particular situation requires careful consideration of the pros and cons of each approach.
- Random assignment to conditions in between-subjects experiments or to orders of conditions in within-subjects experiments is a fundamental element of experimental research. Its purpose is to control extraneous variables so that they do not become confounding variables.
- Experimental research on the effectiveness of a treatment requires both a treatment condition and a control condition, which can be a no-treatment control condition, a placebo control condition, or a waitlist control condition. Experimental treatments can also be compared with the best available alternative.
Discussion: For each of the following topics, list the pros and cons of a between-subjects and within-subjects design and decide which would be better.
- You want to test the relative effectiveness of two training programs for running a marathon.
- Using photographs of people as stimuli, you want to see if smiling people are perceived as more intelligent than people who are not smiling.
- In a field experiment, you want to see if the way a panhandler is dressed (neatly vs. sloppily) affects whether or not passersby give him any money.
- You want to see if concrete nouns (e.g., dog ) are recalled better than abstract nouns (e.g., truth ).
- Discussion: Imagine that an experiment shows that participants who receive psychodynamic therapy for a dog phobia improve more than participants in a no-treatment control group. Explain a fundamental problem with this research design and at least two ways that it might be corrected.
Birnbaum, M. H. (1999). How to show that 9 > 221: Collect judgments in a between-subjects design. Psychological Methods, 4 , 243–249.
Moseley, J. B., O’Malley, K., Petersen, N. J., Menke, T. J., Brody, B. A., Kuykendall, D. H., … Wray, N. P. (2002). A controlled trial of arthroscopic surgery for osteoarthritis of the knee. The New England Journal of Medicine, 347 , 81–88.
Price, D. D., Finniss, D. G., & Benedetti, F. (2008). A comprehensive review of the placebo effect: Recent advances and current thought. Annual Review of Psychology, 59 , 565–590.
Shapiro, A. K., & Shapiro, E. (1999). The powerful placebo: From ancient priest to modern physician . Baltimore, MD: Johns Hopkins University Press.
Research Methods in Psychology Copyright © 2016 by University of Minnesota is licensed under a Creative Commons Attribution-NonCommercial-ShareAlike 4.0 International License , except where otherwise noted.
5.2 Experimental Design
Learning objectives.
- Explain the difference between between-subjects and within-subjects experiments, list some of the pros and cons of each approach, and decide which approach to use to answer a particular research question.
- Define random assignment, distinguish it from random sampling, explain its purpose in experimental research, and use some simple strategies to implement it
- Define several types of carryover effect, give examples of each, and explain how counterbalancing helps to deal with them.
In this section, we look at some different ways to design an experiment. The primary distinction we will make is between approaches in which each participant experiences one level of the independent variable and approaches in which each participant experiences all levels of the independent variable. The former are called between-subjects experiments and the latter are called within-subjects experiments.
Between-Subjects Experiments
In a between-subjects experiment , each participant is tested in only one condition. For example, a researcher with a sample of 100 university students might assign half of them to write about a traumatic event and the other half write about a neutral event. Or a researcher with a sample of 60 people with severe agoraphobia (fear of open spaces) might assign 20 of them to receive each of three different treatments for that disorder. It is essential in a between-subjects experiment that the researcher assigns participants to conditions so that the different groups are, on average, highly similar to each other. Those in a trauma condition and a neutral condition, for example, should include a similar proportion of men and women, and they should have similar average intelligence quotients (IQs), similar average levels of motivation, similar average numbers of health problems, and so on. This matching is a matter of controlling these extraneous participant variables across conditions so that they do not become confounding variables.
Random Assignment
The primary way that researchers accomplish this kind of control of extraneous variables across conditions is called random assignment , which means using a random process to decide which participants are tested in which conditions. Do not confuse random assignment with random sampling. Random sampling is a method for selecting a sample from a population, and it is rarely used in psychological research. Random assignment is a method for assigning participants in a sample to the different conditions, and it is an important element of all experimental research in psychology and other fields too.
In its strictest sense, random assignment should meet two criteria. One is that each participant has an equal chance of being assigned to each condition (e.g., a 50% chance of being assigned to each of two conditions). The second is that each participant is assigned to a condition independently of other participants. Thus one way to assign participants to two conditions would be to flip a coin for each one. If the coin lands heads, the participant is assigned to Condition A, and if it lands tails, the participant is assigned to Condition B. For three conditions, one could use a computer to generate a random integer from 1 to 3 for each participant. If the integer is 1, the participant is assigned to Condition A; if it is 2, the participant is assigned to Condition B; and if it is 3, the participant is assigned to Condition C. In practice, a full sequence of conditions—one for each participant expected to be in the experiment—is usually created ahead of time, and each new participant is assigned to the next condition in the sequence as he or she is tested. When the procedure is computerized, the computer program often handles the random assignment.
One problem with coin flipping and other strict procedures for random assignment is that they are likely to result in unequal sample sizes in the different conditions. Unequal sample sizes are generally not a serious problem, and you should never throw away data you have already collected to achieve equal sample sizes. However, for a fixed number of participants, it is statistically most efficient to divide them into equal-sized groups. It is standard practice, therefore, to use a kind of modified random assignment that keeps the number of participants in each group as similar as possible. One approach is block randomization . In block randomization, all the conditions occur once in the sequence before any of them is repeated. Then they all occur again before any of them is repeated again. Within each of these “blocks,” the conditions occur in a random order. Again, the sequence of conditions is usually generated before any participants are tested, and each new participant is assigned to the next condition in the sequence. Table 5.2 shows such a sequence for assigning nine participants to three conditions. The Research Randomizer website ( http://www.randomizer.org ) will generate block randomization sequences for any number of participants and conditions. Again, when the procedure is computerized, the computer program often handles the block randomization.
4 | B |
5 | C |
6 | A |
Random assignment is not guaranteed to control all extraneous variables across conditions. The process is random, so it is always possible that just by chance, the participants in one condition might turn out to be substantially older, less tired, more motivated, or less depressed on average than the participants in another condition. However, there are some reasons that this possibility is not a major concern. One is that random assignment works better than one might expect, especially for large samples. Another is that the inferential statistics that researchers use to decide whether a difference between groups reflects a difference in the population takes the “fallibility” of random assignment into account. Yet another reason is that even if random assignment does result in a confounding variable and therefore produces misleading results, this confound is likely to be detected when the experiment is replicated. The upshot is that random assignment to conditions—although not infallible in terms of controlling extraneous variables—is always considered a strength of a research design.
Matched Groups
An alternative to simple random assignment of participants to conditions is the use of a matched-groups design . Using this design, participants in the various conditions are matched on the dependent variable or on some extraneous variable(s) prior the manipulation of the independent variable. This guarantees that these variables will not be confounded across the experimental conditions. For instance, if we want to determine whether expressive writing affects people’s health then we could start by measuring various health-related variables in our prospective research participants. We could then use that information to rank-order participants according to how healthy or unhealthy they are. Next, the two healthiest participants would be randomly assigned to complete different conditions (one would be randomly assigned to the traumatic experiences writing condition and the other to the neutral writing condition). The next two healthiest participants would then be randomly assigned to complete different conditions, and so on until the two least healthy participants. This method would ensure that participants in the traumatic experiences writing condition are matched to participants in the neutral writing condition with respect to health at the beginning of the study. If at the end of the experiment, a difference in health was detected across the two conditions, then we would know that it is due to the writing manipulation and not to pre-existing differences in health.
Within-Subjects Experiments
In a within-subjects experiment , each participant is tested under all conditions. Consider an experiment on the effect of a defendant’s physical attractiveness on judgments of his guilt. Again, in a between-subjects experiment, one group of participants would be shown an attractive defendant and asked to judge his guilt, and another group of participants would be shown an unattractive defendant and asked to judge his guilt. In a within-subjects experiment, however, the same group of participants would judge the guilt of both an attractive and an unattractive defendant.
The primary advantage of this approach is that it provides maximum control of extraneous participant variables. Participants in all conditions have the same mean IQ, same socioeconomic status, same number of siblings, and so on—because they are the very same people. Within-subjects experiments also make it possible to use statistical procedures that remove the effect of these extraneous participant variables on the dependent variable and therefore make the data less “noisy” and the effect of the independent variable easier to detect. We will look more closely at this idea later in the book . However, not all experiments can use a within-subjects design nor would it be desirable to do so.
One disadvantage of within-subjects experiments is that they make it easier for participants to guess the hypothesis. For example, a participant who is asked to judge the guilt of an attractive defendant and then is asked to judge the guilt of an unattractive defendant is likely to guess that the hypothesis is that defendant attractiveness affects judgments of guilt. This knowledge could lead the participant to judge the unattractive defendant more harshly because he thinks this is what he is expected to do. Or it could make participants judge the two defendants similarly in an effort to be “fair.”
Carryover Effects and Counterbalancing
The primary disadvantage of within-subjects designs is that they can result in order effects. An order effect occurs when participants’ responses in the various conditions are affected by the order of conditions to which they were exposed. One type of order effect is a carryover effect. A carryover effect is an effect of being tested in one condition on participants’ behavior in later conditions. One type of carryover effect is a practice effect , where participants perform a task better in later conditions because they have had a chance to practice it. Another type is a fatigue effect , where participants perform a task worse in later conditions because they become tired or bored. Being tested in one condition can also change how participants perceive stimuli or interpret their task in later conditions. This type of effect is called a context effect (or contrast effect) . For example, an average-looking defendant might be judged more harshly when participants have just judged an attractive defendant than when they have just judged an unattractive defendant. Within-subjects experiments also make it easier for participants to guess the hypothesis. For example, a participant who is asked to judge the guilt of an attractive defendant and then is asked to judge the guilt of an unattractive defendant is likely to guess that the hypothesis is that defendant attractiveness affects judgments of guilt.
Carryover effects can be interesting in their own right. (Does the attractiveness of one person depend on the attractiveness of other people that we have seen recently?) But when they are not the focus of the research, carryover effects can be problematic. Imagine, for example, that participants judge the guilt of an attractive defendant and then judge the guilt of an unattractive defendant. If they judge the unattractive defendant more harshly, this might be because of his unattractiveness. But it could be instead that they judge him more harshly because they are becoming bored or tired. In other words, the order of the conditions is a confounding variable. The attractive condition is always the first condition and the unattractive condition the second. Thus any difference between the conditions in terms of the dependent variable could be caused by the order of the conditions and not the independent variable itself.
There is a solution to the problem of order effects, however, that can be used in many situations. It is counterbalancing , which means testing different participants in different orders. The best method of counterbalancing is complete counterbalancing in which an equal number of participants complete each possible order of conditions. For example, half of the participants would be tested in the attractive defendant condition followed by the unattractive defendant condition, and others half would be tested in the unattractive condition followed by the attractive condition. With three conditions, there would be six different orders (ABC, ACB, BAC, BCA, CAB, and CBA), so some participants would be tested in each of the six orders. With four conditions, there would be 24 different orders; with five conditions there would be 120 possible orders. With counterbalancing, participants are assigned to orders randomly, using the techniques we have already discussed. Thus, random assignment plays an important role in within-subjects designs just as in between-subjects designs. Here, instead of randomly assigning to conditions, they are randomly assigned to different orders of conditions. In fact, it can safely be said that if a study does not involve random assignment in one form or another, it is not an experiment.
A more efficient way of counterbalancing is through a Latin square design which randomizes through having equal rows and columns. For example, if you have four treatments, you must have four versions. Like a Sudoku puzzle, no treatment can repeat in a row or column. For four versions of four treatments, the Latin square design would look like:
A | B | C | D |
B | C | D | A |
C | D | A | B |
D | A | B | C |
You can see in the diagram above that the square has been constructed to ensure that each condition appears at each ordinal position (A appears first once, second once, third once, and fourth once) and each condition preceded and follows each other condition one time. A Latin square for an experiment with 6 conditions would by 6 x 6 in dimension, one for an experiment with 8 conditions would be 8 x 8 in dimension, and so on. So while complete counterbalancing of 6 conditions would require 720 orders, a Latin square would only require 6 orders.
Finally, when the number of conditions is large experiments can use random counterbalancing in which the order of the conditions is randomly determined for each participant. Using this technique every possible order of conditions is determined and then one of these orders is randomly selected for each participant. This is not as powerful a technique as complete counterbalancing or partial counterbalancing using a Latin squares design. Use of random counterbalancing will result in more random error, but if order effects are likely to be small and the number of conditions is large, this is an option available to researchers.
There are two ways to think about what counterbalancing accomplishes. One is that it controls the order of conditions so that it is no longer a confounding variable. Instead of the attractive condition always being first and the unattractive condition always being second, the attractive condition comes first for some participants and second for others. Likewise, the unattractive condition comes first for some participants and second for others. Thus any overall difference in the dependent variable between the two conditions cannot have been caused by the order of conditions. A second way to think about what counterbalancing accomplishes is that if there are carryover effects, it makes it possible to detect them. One can analyze the data separately for each order to see whether it had an effect.
When 9 Is “Larger” Than 221
Researcher Michael Birnbaum has argued that the lack of context provided by between-subjects designs is often a bigger problem than the context effects created by within-subjects designs. To demonstrate this problem, he asked participants to rate two numbers on how large they were on a scale of 1-to-10 where 1 was “very very small” and 10 was “very very large”. One group of participants were asked to rate the number 9 and another group was asked to rate the number 221 (Birnbaum, 1999) [1] . Participants in this between-subjects design gave the number 9 a mean rating of 5.13 and the number 221 a mean rating of 3.10. In other words, they rated 9 as larger than 221! According to Birnbaum, this difference is because participants spontaneously compared 9 with other one-digit numbers (in which case it is relatively large) and compared 221 with other three-digit numbers (in which case it is relatively small).
Simultaneous Within-Subjects Designs
So far, we have discussed an approach to within-subjects designs in which participants are tested in one condition at a time. There is another approach, however, that is often used when participants make multiple responses in each condition. Imagine, for example, that participants judge the guilt of 10 attractive defendants and 10 unattractive defendants. Instead of having people make judgments about all 10 defendants of one type followed by all 10 defendants of the other type, the researcher could present all 20 defendants in a sequence that mixed the two types. The researcher could then compute each participant’s mean rating for each type of defendant. Or imagine an experiment designed to see whether people with social anxiety disorder remember negative adjectives (e.g., “stupid,” “incompetent”) better than positive ones (e.g., “happy,” “productive”). The researcher could have participants study a single list that includes both kinds of words and then have them try to recall as many words as possible. The researcher could then count the number of each type of word that was recalled.
Between-Subjects or Within-Subjects?
Almost every experiment can be conducted using either a between-subjects design or a within-subjects design. This possibility means that researchers must choose between the two approaches based on their relative merits for the particular situation.
Between-subjects experiments have the advantage of being conceptually simpler and requiring less testing time per participant. They also avoid carryover effects without the need for counterbalancing. Within-subjects experiments have the advantage of controlling extraneous participant variables, which generally reduces noise in the data and makes it easier to detect a relationship between the independent and dependent variables.
A good rule of thumb, then, is that if it is possible to conduct a within-subjects experiment (with proper counterbalancing) in the time that is available per participant—and you have no serious concerns about carryover effects—this design is probably the best option. If a within-subjects design would be difficult or impossible to carry out, then you should consider a between-subjects design instead. For example, if you were testing participants in a doctor’s waiting room or shoppers in line at a grocery store, you might not have enough time to test each participant in all conditions and therefore would opt for a between-subjects design. Or imagine you were trying to reduce people’s level of prejudice by having them interact with someone of another race. A within-subjects design with counterbalancing would require testing some participants in the treatment condition first and then in a control condition. But if the treatment works and reduces people’s level of prejudice, then they would no longer be suitable for testing in the control condition. This difficulty is true for many designs that involve a treatment meant to produce long-term change in participants’ behavior (e.g., studies testing the effectiveness of psychotherapy). Clearly, a between-subjects design would be necessary here.
Remember also that using one type of design does not preclude using the other type in a different study. There is no reason that a researcher could not use both a between-subjects design and a within-subjects design to answer the same research question. In fact, professional researchers often take exactly this type of mixed methods approach.
Key Takeaways
- Experiments can be conducted using either between-subjects or within-subjects designs. Deciding which to use in a particular situation requires careful consideration of the pros and cons of each approach.
- Random assignment to conditions in between-subjects experiments or counterbalancing of orders of conditions in within-subjects experiments is a fundamental element of experimental research. The purpose of these techniques is to control extraneous variables so that they do not become confounding variables.
- You want to test the relative effectiveness of two training programs for running a marathon.
- Using photographs of people as stimuli, you want to see if smiling people are perceived as more intelligent than people who are not smiling.
- In a field experiment, you want to see if the way a panhandler is dressed (neatly vs. sloppily) affects whether or not passersby give him any money.
- You want to see if concrete nouns (e.g., dog ) are recalled better than abstract nouns (e.g., truth).
- Birnbaum, M.H. (1999). How to show that 9>221: Collect judgments in a between-subjects design. Psychological Methods, 4 (3), 243-249. ↵

Share This Book
- Increase Font Size
Experimental Design: Types, Examples & Methods
Saul McLeod, PhD
Editor-in-Chief for Simply Psychology
BSc (Hons) Psychology, MRes, PhD, University of Manchester
Saul McLeod, PhD., is a qualified psychology teacher with over 18 years of experience in further and higher education. He has been published in peer-reviewed journals, including the Journal of Clinical Psychology.
Learn about our Editorial Process
Olivia Guy-Evans, MSc
Associate Editor for Simply Psychology
BSc (Hons) Psychology, MSc Psychology of Education
Olivia Guy-Evans is a writer and associate editor for Simply Psychology. She has previously worked in healthcare and educational sectors.
On This Page:
Experimental design refers to how participants are allocated to different groups in an experiment. Types of design include repeated measures, independent groups, and matched pairs designs.
Probably the most common way to design an experiment in psychology is to divide the participants into two groups, the experimental group and the control group, and then introduce a change to the experimental group, not the control group.
The researcher must decide how he/she will allocate their sample to the different experimental groups. For example, if there are 10 participants, will all 10 participants participate in both groups (e.g., repeated measures), or will the participants be split in half and take part in only one group each?
Three types of experimental designs are commonly used:
1. Independent Measures
Independent measures design, also known as between-groups , is an experimental design where different participants are used in each condition of the independent variable. This means that each condition of the experiment includes a different group of participants.
This should be done by random allocation, ensuring that each participant has an equal chance of being assigned to one group.
Independent measures involve using two separate groups of participants, one in each condition. For example:
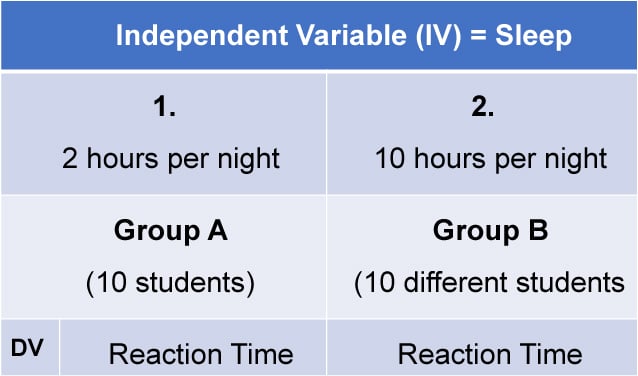
- Con : More people are needed than with the repeated measures design (i.e., more time-consuming).
- Pro : Avoids order effects (such as practice or fatigue) as people participate in one condition only. If a person is involved in several conditions, they may become bored, tired, and fed up by the time they come to the second condition or become wise to the requirements of the experiment!
- Con : Differences between participants in the groups may affect results, for example, variations in age, gender, or social background. These differences are known as participant variables (i.e., a type of extraneous variable ).
- Control : After the participants have been recruited, they should be randomly assigned to their groups. This should ensure the groups are similar, on average (reducing participant variables).
2. Repeated Measures Design
Repeated Measures design is an experimental design where the same participants participate in each independent variable condition. This means that each experiment condition includes the same group of participants.
Repeated Measures design is also known as within-groups or within-subjects design .
- Pro : As the same participants are used in each condition, participant variables (i.e., individual differences) are reduced.
- Con : There may be order effects. Order effects refer to the order of the conditions affecting the participants’ behavior. Performance in the second condition may be better because the participants know what to do (i.e., practice effect). Or their performance might be worse in the second condition because they are tired (i.e., fatigue effect). This limitation can be controlled using counterbalancing.
- Pro : Fewer people are needed as they participate in all conditions (i.e., saves time).
- Control : To combat order effects, the researcher counter-balances the order of the conditions for the participants. Alternating the order in which participants perform in different conditions of an experiment.
Counterbalancing
Suppose we used a repeated measures design in which all of the participants first learned words in “loud noise” and then learned them in “no noise.”
We expect the participants to learn better in “no noise” because of order effects, such as practice. However, a researcher can control for order effects using counterbalancing.
The sample would be split into two groups: experimental (A) and control (B). For example, group 1 does ‘A’ then ‘B,’ and group 2 does ‘B’ then ‘A.’ This is to eliminate order effects.
Although order effects occur for each participant, they balance each other out in the results because they occur equally in both groups.
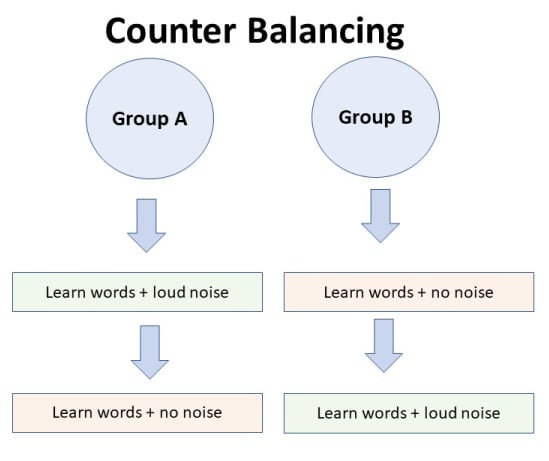
3. Matched Pairs Design
A matched pairs design is an experimental design where pairs of participants are matched in terms of key variables, such as age or socioeconomic status. One member of each pair is then placed into the experimental group and the other member into the control group .
One member of each matched pair must be randomly assigned to the experimental group and the other to the control group.
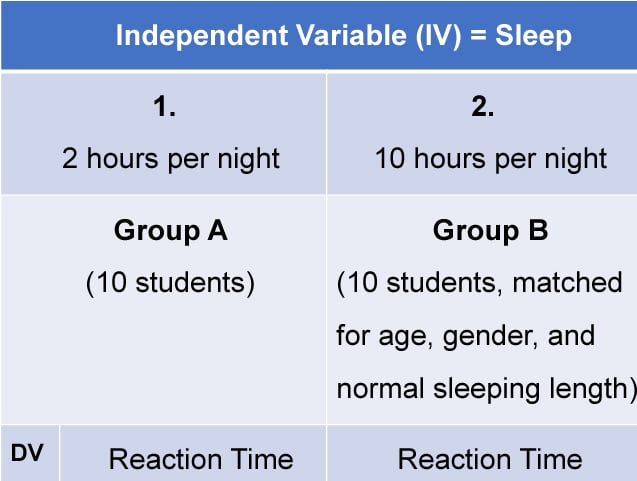
- Con : If one participant drops out, you lose 2 PPs’ data.
- Pro : Reduces participant variables because the researcher has tried to pair up the participants so that each condition has people with similar abilities and characteristics.
- Con : Very time-consuming trying to find closely matched pairs.
- Pro : It avoids order effects, so counterbalancing is not necessary.
- Con : Impossible to match people exactly unless they are identical twins!
- Control : Members of each pair should be randomly assigned to conditions. However, this does not solve all these problems.
Experimental design refers to how participants are allocated to an experiment’s different conditions (or IV levels). There are three types:
1. Independent measures / between-groups : Different participants are used in each condition of the independent variable.
2. Repeated measures /within groups : The same participants take part in each condition of the independent variable.
3. Matched pairs : Each condition uses different participants, but they are matched in terms of important characteristics, e.g., gender, age, intelligence, etc.
Learning Check
Read about each of the experiments below. For each experiment, identify (1) which experimental design was used; and (2) why the researcher might have used that design.
1 . To compare the effectiveness of two different types of therapy for depression, depressed patients were assigned to receive either cognitive therapy or behavior therapy for a 12-week period.
The researchers attempted to ensure that the patients in the two groups had similar severity of depressed symptoms by administering a standardized test of depression to each participant, then pairing them according to the severity of their symptoms.
2 . To assess the difference in reading comprehension between 7 and 9-year-olds, a researcher recruited each group from a local primary school. They were given the same passage of text to read and then asked a series of questions to assess their understanding.
3 . To assess the effectiveness of two different ways of teaching reading, a group of 5-year-olds was recruited from a primary school. Their level of reading ability was assessed, and then they were taught using scheme one for 20 weeks.
At the end of this period, their reading was reassessed, and a reading improvement score was calculated. They were then taught using scheme two for a further 20 weeks, and another reading improvement score for this period was calculated. The reading improvement scores for each child were then compared.
4 . To assess the effect of the organization on recall, a researcher randomly assigned student volunteers to two conditions.
Condition one attempted to recall a list of words that were organized into meaningful categories; condition two attempted to recall the same words, randomly grouped on the page.
Experiment Terminology
Ecological validity.
The degree to which an investigation represents real-life experiences.
Experimenter effects
These are the ways that the experimenter can accidentally influence the participant through their appearance or behavior.
Demand characteristics
The clues in an experiment lead the participants to think they know what the researcher is looking for (e.g., the experimenter’s body language).
Independent variable (IV)
The variable the experimenter manipulates (i.e., changes) is assumed to have a direct effect on the dependent variable.
Dependent variable (DV)
Variable the experimenter measures. This is the outcome (i.e., the result) of a study.
Extraneous variables (EV)
All variables which are not independent variables but could affect the results (DV) of the experiment. Extraneous variables should be controlled where possible.
Confounding variables
Variable(s) that have affected the results (DV), apart from the IV. A confounding variable could be an extraneous variable that has not been controlled.
Random Allocation
Randomly allocating participants to independent variable conditions means that all participants should have an equal chance of taking part in each condition.
The principle of random allocation is to avoid bias in how the experiment is carried out and limit the effects of participant variables.
Order effects
Changes in participants’ performance due to their repeating the same or similar test more than once. Examples of order effects include:
(i) practice effect: an improvement in performance on a task due to repetition, for example, because of familiarity with the task;
(ii) fatigue effect: a decrease in performance of a task due to repetition, for example, because of boredom or tiredness.

Frequently asked questions
What’s the difference between random assignment and random selection.
Random selection, or random sampling , is a way of selecting members of a population for your study’s sample.
In contrast, random assignment is a way of sorting the sample into control and experimental groups.
Random sampling enhances the external validity or generalizability of your results, while random assignment improves the internal validity of your study.
Frequently asked questions: Methodology
Attrition refers to participants leaving a study. It always happens to some extent—for example, in randomized controlled trials for medical research.
Differential attrition occurs when attrition or dropout rates differ systematically between the intervention and the control group . As a result, the characteristics of the participants who drop out differ from the characteristics of those who stay in the study. Because of this, study results may be biased .
Action research is conducted in order to solve a particular issue immediately, while case studies are often conducted over a longer period of time and focus more on observing and analyzing a particular ongoing phenomenon.
Action research is focused on solving a problem or informing individual and community-based knowledge in a way that impacts teaching, learning, and other related processes. It is less focused on contributing theoretical input, instead producing actionable input.
Action research is particularly popular with educators as a form of systematic inquiry because it prioritizes reflection and bridges the gap between theory and practice. Educators are able to simultaneously investigate an issue as they solve it, and the method is very iterative and flexible.
A cycle of inquiry is another name for action research . It is usually visualized in a spiral shape following a series of steps, such as “planning → acting → observing → reflecting.”
To make quantitative observations , you need to use instruments that are capable of measuring the quantity you want to observe. For example, you might use a ruler to measure the length of an object or a thermometer to measure its temperature.
Criterion validity and construct validity are both types of measurement validity . In other words, they both show you how accurately a method measures something.
While construct validity is the degree to which a test or other measurement method measures what it claims to measure, criterion validity is the degree to which a test can predictively (in the future) or concurrently (in the present) measure something.
Construct validity is often considered the overarching type of measurement validity . You need to have face validity , content validity , and criterion validity in order to achieve construct validity.
Convergent validity and discriminant validity are both subtypes of construct validity . Together, they help you evaluate whether a test measures the concept it was designed to measure.
- Convergent validity indicates whether a test that is designed to measure a particular construct correlates with other tests that assess the same or similar construct.
- Discriminant validity indicates whether two tests that should not be highly related to each other are indeed not related. This type of validity is also called divergent validity .
You need to assess both in order to demonstrate construct validity. Neither one alone is sufficient for establishing construct validity.
- Discriminant validity indicates whether two tests that should not be highly related to each other are indeed not related
Content validity shows you how accurately a test or other measurement method taps into the various aspects of the specific construct you are researching.
In other words, it helps you answer the question: “does the test measure all aspects of the construct I want to measure?” If it does, then the test has high content validity.
The higher the content validity, the more accurate the measurement of the construct.
If the test fails to include parts of the construct, or irrelevant parts are included, the validity of the instrument is threatened, which brings your results into question.
Face validity and content validity are similar in that they both evaluate how suitable the content of a test is. The difference is that face validity is subjective, and assesses content at surface level.
When a test has strong face validity, anyone would agree that the test’s questions appear to measure what they are intended to measure.
For example, looking at a 4th grade math test consisting of problems in which students have to add and multiply, most people would agree that it has strong face validity (i.e., it looks like a math test).
On the other hand, content validity evaluates how well a test represents all the aspects of a topic. Assessing content validity is more systematic and relies on expert evaluation. of each question, analyzing whether each one covers the aspects that the test was designed to cover.
A 4th grade math test would have high content validity if it covered all the skills taught in that grade. Experts(in this case, math teachers), would have to evaluate the content validity by comparing the test to the learning objectives.
Snowball sampling is a non-probability sampling method . Unlike probability sampling (which involves some form of random selection ), the initial individuals selected to be studied are the ones who recruit new participants.
Because not every member of the target population has an equal chance of being recruited into the sample, selection in snowball sampling is non-random.
Snowball sampling is a non-probability sampling method , where there is not an equal chance for every member of the population to be included in the sample .
This means that you cannot use inferential statistics and make generalizations —often the goal of quantitative research . As such, a snowball sample is not representative of the target population and is usually a better fit for qualitative research .
Snowball sampling relies on the use of referrals. Here, the researcher recruits one or more initial participants, who then recruit the next ones.
Participants share similar characteristics and/or know each other. Because of this, not every member of the population has an equal chance of being included in the sample, giving rise to sampling bias .
Snowball sampling is best used in the following cases:
- If there is no sampling frame available (e.g., people with a rare disease)
- If the population of interest is hard to access or locate (e.g., people experiencing homelessness)
- If the research focuses on a sensitive topic (e.g., extramarital affairs)
The reproducibility and replicability of a study can be ensured by writing a transparent, detailed method section and using clear, unambiguous language.
Reproducibility and replicability are related terms.
- Reproducing research entails reanalyzing the existing data in the same manner.
- Replicating (or repeating ) the research entails reconducting the entire analysis, including the collection of new data .
- A successful reproduction shows that the data analyses were conducted in a fair and honest manner.
- A successful replication shows that the reliability of the results is high.
Stratified sampling and quota sampling both involve dividing the population into subgroups and selecting units from each subgroup. The purpose in both cases is to select a representative sample and/or to allow comparisons between subgroups.
The main difference is that in stratified sampling, you draw a random sample from each subgroup ( probability sampling ). In quota sampling you select a predetermined number or proportion of units, in a non-random manner ( non-probability sampling ).
Purposive and convenience sampling are both sampling methods that are typically used in qualitative data collection.
A convenience sample is drawn from a source that is conveniently accessible to the researcher. Convenience sampling does not distinguish characteristics among the participants. On the other hand, purposive sampling focuses on selecting participants possessing characteristics associated with the research study.
The findings of studies based on either convenience or purposive sampling can only be generalized to the (sub)population from which the sample is drawn, and not to the entire population.
Random sampling or probability sampling is based on random selection. This means that each unit has an equal chance (i.e., equal probability) of being included in the sample.
On the other hand, convenience sampling involves stopping people at random, which means that not everyone has an equal chance of being selected depending on the place, time, or day you are collecting your data.
Convenience sampling and quota sampling are both non-probability sampling methods. They both use non-random criteria like availability, geographical proximity, or expert knowledge to recruit study participants.
However, in convenience sampling, you continue to sample units or cases until you reach the required sample size.
In quota sampling, you first need to divide your population of interest into subgroups (strata) and estimate their proportions (quota) in the population. Then you can start your data collection, using convenience sampling to recruit participants, until the proportions in each subgroup coincide with the estimated proportions in the population.
A sampling frame is a list of every member in the entire population . It is important that the sampling frame is as complete as possible, so that your sample accurately reflects your population.
Stratified and cluster sampling may look similar, but bear in mind that groups created in cluster sampling are heterogeneous , so the individual characteristics in the cluster vary. In contrast, groups created in stratified sampling are homogeneous , as units share characteristics.
Relatedly, in cluster sampling you randomly select entire groups and include all units of each group in your sample. However, in stratified sampling, you select some units of all groups and include them in your sample. In this way, both methods can ensure that your sample is representative of the target population .
A systematic review is secondary research because it uses existing research. You don’t collect new data yourself.
The key difference between observational studies and experimental designs is that a well-done observational study does not influence the responses of participants, while experiments do have some sort of treatment condition applied to at least some participants by random assignment .
An observational study is a great choice for you if your research question is based purely on observations. If there are ethical, logistical, or practical concerns that prevent you from conducting a traditional experiment , an observational study may be a good choice. In an observational study, there is no interference or manipulation of the research subjects, as well as no control or treatment groups .
It’s often best to ask a variety of people to review your measurements. You can ask experts, such as other researchers, or laypeople, such as potential participants, to judge the face validity of tests.
While experts have a deep understanding of research methods , the people you’re studying can provide you with valuable insights you may have missed otherwise.
Face validity is important because it’s a simple first step to measuring the overall validity of a test or technique. It’s a relatively intuitive, quick, and easy way to start checking whether a new measure seems useful at first glance.
Good face validity means that anyone who reviews your measure says that it seems to be measuring what it’s supposed to. With poor face validity, someone reviewing your measure may be left confused about what you’re measuring and why you’re using this method.
Face validity is about whether a test appears to measure what it’s supposed to measure. This type of validity is concerned with whether a measure seems relevant and appropriate for what it’s assessing only on the surface.
Statistical analyses are often applied to test validity with data from your measures. You test convergent validity and discriminant validity with correlations to see if results from your test are positively or negatively related to those of other established tests.
You can also use regression analyses to assess whether your measure is actually predictive of outcomes that you expect it to predict theoretically. A regression analysis that supports your expectations strengthens your claim of construct validity .
When designing or evaluating a measure, construct validity helps you ensure you’re actually measuring the construct you’re interested in. If you don’t have construct validity, you may inadvertently measure unrelated or distinct constructs and lose precision in your research.
Construct validity is often considered the overarching type of measurement validity , because it covers all of the other types. You need to have face validity , content validity , and criterion validity to achieve construct validity.
Construct validity is about how well a test measures the concept it was designed to evaluate. It’s one of four types of measurement validity , which includes construct validity, face validity , and criterion validity.
There are two subtypes of construct validity.
- Convergent validity : The extent to which your measure corresponds to measures of related constructs
- Discriminant validity : The extent to which your measure is unrelated or negatively related to measures of distinct constructs
Naturalistic observation is a valuable tool because of its flexibility, external validity , and suitability for topics that can’t be studied in a lab setting.
The downsides of naturalistic observation include its lack of scientific control , ethical considerations , and potential for bias from observers and subjects.
Naturalistic observation is a qualitative research method where you record the behaviors of your research subjects in real world settings. You avoid interfering or influencing anything in a naturalistic observation.
You can think of naturalistic observation as “people watching” with a purpose.
A dependent variable is what changes as a result of the independent variable manipulation in experiments . It’s what you’re interested in measuring, and it “depends” on your independent variable.
In statistics, dependent variables are also called:
- Response variables (they respond to a change in another variable)
- Outcome variables (they represent the outcome you want to measure)
- Left-hand-side variables (they appear on the left-hand side of a regression equation)
An independent variable is the variable you manipulate, control, or vary in an experimental study to explore its effects. It’s called “independent” because it’s not influenced by any other variables in the study.
Independent variables are also called:
- Explanatory variables (they explain an event or outcome)
- Predictor variables (they can be used to predict the value of a dependent variable)
- Right-hand-side variables (they appear on the right-hand side of a regression equation).
As a rule of thumb, questions related to thoughts, beliefs, and feelings work well in focus groups. Take your time formulating strong questions, paying special attention to phrasing. Be careful to avoid leading questions , which can bias your responses.
Overall, your focus group questions should be:
- Open-ended and flexible
- Impossible to answer with “yes” or “no” (questions that start with “why” or “how” are often best)
- Unambiguous, getting straight to the point while still stimulating discussion
- Unbiased and neutral
A structured interview is a data collection method that relies on asking questions in a set order to collect data on a topic. They are often quantitative in nature. Structured interviews are best used when:
- You already have a very clear understanding of your topic. Perhaps significant research has already been conducted, or you have done some prior research yourself, but you already possess a baseline for designing strong structured questions.
- You are constrained in terms of time or resources and need to analyze your data quickly and efficiently.
- Your research question depends on strong parity between participants, with environmental conditions held constant.
More flexible interview options include semi-structured interviews , unstructured interviews , and focus groups .
Social desirability bias is the tendency for interview participants to give responses that will be viewed favorably by the interviewer or other participants. It occurs in all types of interviews and surveys , but is most common in semi-structured interviews , unstructured interviews , and focus groups .
Social desirability bias can be mitigated by ensuring participants feel at ease and comfortable sharing their views. Make sure to pay attention to your own body language and any physical or verbal cues, such as nodding or widening your eyes.
This type of bias can also occur in observations if the participants know they’re being observed. They might alter their behavior accordingly.
The interviewer effect is a type of bias that emerges when a characteristic of an interviewer (race, age, gender identity, etc.) influences the responses given by the interviewee.
There is a risk of an interviewer effect in all types of interviews , but it can be mitigated by writing really high-quality interview questions.
A semi-structured interview is a blend of structured and unstructured types of interviews. Semi-structured interviews are best used when:
- You have prior interview experience. Spontaneous questions are deceptively challenging, and it’s easy to accidentally ask a leading question or make a participant uncomfortable.
- Your research question is exploratory in nature. Participant answers can guide future research questions and help you develop a more robust knowledge base for future research.
An unstructured interview is the most flexible type of interview, but it is not always the best fit for your research topic.
Unstructured interviews are best used when:
- You are an experienced interviewer and have a very strong background in your research topic, since it is challenging to ask spontaneous, colloquial questions.
- Your research question is exploratory in nature. While you may have developed hypotheses, you are open to discovering new or shifting viewpoints through the interview process.
- You are seeking descriptive data, and are ready to ask questions that will deepen and contextualize your initial thoughts and hypotheses.
- Your research depends on forming connections with your participants and making them feel comfortable revealing deeper emotions, lived experiences, or thoughts.
The four most common types of interviews are:
- Structured interviews : The questions are predetermined in both topic and order.
- Semi-structured interviews : A few questions are predetermined, but other questions aren’t planned.
- Unstructured interviews : None of the questions are predetermined.
- Focus group interviews : The questions are presented to a group instead of one individual.
Deductive reasoning is commonly used in scientific research, and it’s especially associated with quantitative research .
In research, you might have come across something called the hypothetico-deductive method . It’s the scientific method of testing hypotheses to check whether your predictions are substantiated by real-world data.
Deductive reasoning is a logical approach where you progress from general ideas to specific conclusions. It’s often contrasted with inductive reasoning , where you start with specific observations and form general conclusions.
Deductive reasoning is also called deductive logic.
There are many different types of inductive reasoning that people use formally or informally.
Here are a few common types:
- Inductive generalization : You use observations about a sample to come to a conclusion about the population it came from.
- Statistical generalization: You use specific numbers about samples to make statements about populations.
- Causal reasoning: You make cause-and-effect links between different things.
- Sign reasoning: You make a conclusion about a correlational relationship between different things.
- Analogical reasoning: You make a conclusion about something based on its similarities to something else.
Inductive reasoning is a bottom-up approach, while deductive reasoning is top-down.
Inductive reasoning takes you from the specific to the general, while in deductive reasoning, you make inferences by going from general premises to specific conclusions.
In inductive research , you start by making observations or gathering data. Then, you take a broad scan of your data and search for patterns. Finally, you make general conclusions that you might incorporate into theories.
Inductive reasoning is a method of drawing conclusions by going from the specific to the general. It’s usually contrasted with deductive reasoning, where you proceed from general information to specific conclusions.
Inductive reasoning is also called inductive logic or bottom-up reasoning.
A hypothesis states your predictions about what your research will find. It is a tentative answer to your research question that has not yet been tested. For some research projects, you might have to write several hypotheses that address different aspects of your research question.
A hypothesis is not just a guess — it should be based on existing theories and knowledge. It also has to be testable, which means you can support or refute it through scientific research methods (such as experiments, observations and statistical analysis of data).
Triangulation can help:
- Reduce research bias that comes from using a single method, theory, or investigator
- Enhance validity by approaching the same topic with different tools
- Establish credibility by giving you a complete picture of the research problem
But triangulation can also pose problems:
- It’s time-consuming and labor-intensive, often involving an interdisciplinary team.
- Your results may be inconsistent or even contradictory.
There are four main types of triangulation :
- Data triangulation : Using data from different times, spaces, and people
- Investigator triangulation : Involving multiple researchers in collecting or analyzing data
- Theory triangulation : Using varying theoretical perspectives in your research
- Methodological triangulation : Using different methodologies to approach the same topic
Many academic fields use peer review , largely to determine whether a manuscript is suitable for publication. Peer review enhances the credibility of the published manuscript.
However, peer review is also common in non-academic settings. The United Nations, the European Union, and many individual nations use peer review to evaluate grant applications. It is also widely used in medical and health-related fields as a teaching or quality-of-care measure.
Peer assessment is often used in the classroom as a pedagogical tool. Both receiving feedback and providing it are thought to enhance the learning process, helping students think critically and collaboratively.
Peer review can stop obviously problematic, falsified, or otherwise untrustworthy research from being published. It also represents an excellent opportunity to get feedback from renowned experts in your field. It acts as a first defense, helping you ensure your argument is clear and that there are no gaps, vague terms, or unanswered questions for readers who weren’t involved in the research process.
Peer-reviewed articles are considered a highly credible source due to this stringent process they go through before publication.
In general, the peer review process follows the following steps:
- First, the author submits the manuscript to the editor.
- Reject the manuscript and send it back to author, or
- Send it onward to the selected peer reviewer(s)
- Next, the peer review process occurs. The reviewer provides feedback, addressing any major or minor issues with the manuscript, and gives their advice regarding what edits should be made.
- Lastly, the edited manuscript is sent back to the author. They input the edits, and resubmit it to the editor for publication.
Exploratory research is often used when the issue you’re studying is new or when the data collection process is challenging for some reason.
You can use exploratory research if you have a general idea or a specific question that you want to study but there is no preexisting knowledge or paradigm with which to study it.
Exploratory research is a methodology approach that explores research questions that have not previously been studied in depth. It is often used when the issue you’re studying is new, or the data collection process is challenging in some way.
Explanatory research is used to investigate how or why a phenomenon occurs. Therefore, this type of research is often one of the first stages in the research process , serving as a jumping-off point for future research.
Exploratory research aims to explore the main aspects of an under-researched problem, while explanatory research aims to explain the causes and consequences of a well-defined problem.
Explanatory research is a research method used to investigate how or why something occurs when only a small amount of information is available pertaining to that topic. It can help you increase your understanding of a given topic.
Clean data are valid, accurate, complete, consistent, unique, and uniform. Dirty data include inconsistencies and errors.
Dirty data can come from any part of the research process, including poor research design , inappropriate measurement materials, or flawed data entry.
Data cleaning takes place between data collection and data analyses. But you can use some methods even before collecting data.
For clean data, you should start by designing measures that collect valid data. Data validation at the time of data entry or collection helps you minimize the amount of data cleaning you’ll need to do.
After data collection, you can use data standardization and data transformation to clean your data. You’ll also deal with any missing values, outliers, and duplicate values.
Every dataset requires different techniques to clean dirty data , but you need to address these issues in a systematic way. You focus on finding and resolving data points that don’t agree or fit with the rest of your dataset.
These data might be missing values, outliers, duplicate values, incorrectly formatted, or irrelevant. You’ll start with screening and diagnosing your data. Then, you’ll often standardize and accept or remove data to make your dataset consistent and valid.
Data cleaning is necessary for valid and appropriate analyses. Dirty data contain inconsistencies or errors , but cleaning your data helps you minimize or resolve these.
Without data cleaning, you could end up with a Type I or II error in your conclusion. These types of erroneous conclusions can be practically significant with important consequences, because they lead to misplaced investments or missed opportunities.
Data cleaning involves spotting and resolving potential data inconsistencies or errors to improve your data quality. An error is any value (e.g., recorded weight) that doesn’t reflect the true value (e.g., actual weight) of something that’s being measured.
In this process, you review, analyze, detect, modify, or remove “dirty” data to make your dataset “clean.” Data cleaning is also called data cleansing or data scrubbing.
Research misconduct means making up or falsifying data, manipulating data analyses, or misrepresenting results in research reports. It’s a form of academic fraud.
These actions are committed intentionally and can have serious consequences; research misconduct is not a simple mistake or a point of disagreement but a serious ethical failure.
Anonymity means you don’t know who the participants are, while confidentiality means you know who they are but remove identifying information from your research report. Both are important ethical considerations .
You can only guarantee anonymity by not collecting any personally identifying information—for example, names, phone numbers, email addresses, IP addresses, physical characteristics, photos, or videos.
You can keep data confidential by using aggregate information in your research report, so that you only refer to groups of participants rather than individuals.
Research ethics matter for scientific integrity, human rights and dignity, and collaboration between science and society. These principles make sure that participation in studies is voluntary, informed, and safe.
Ethical considerations in research are a set of principles that guide your research designs and practices. These principles include voluntary participation, informed consent, anonymity, confidentiality, potential for harm, and results communication.
Scientists and researchers must always adhere to a certain code of conduct when collecting data from others .
These considerations protect the rights of research participants, enhance research validity , and maintain scientific integrity.
In multistage sampling , you can use probability or non-probability sampling methods .
For a probability sample, you have to conduct probability sampling at every stage.
You can mix it up by using simple random sampling , systematic sampling , or stratified sampling to select units at different stages, depending on what is applicable and relevant to your study.
Multistage sampling can simplify data collection when you have large, geographically spread samples, and you can obtain a probability sample without a complete sampling frame.
But multistage sampling may not lead to a representative sample, and larger samples are needed for multistage samples to achieve the statistical properties of simple random samples .
These are four of the most common mixed methods designs :
- Convergent parallel: Quantitative and qualitative data are collected at the same time and analyzed separately. After both analyses are complete, compare your results to draw overall conclusions.
- Embedded: Quantitative and qualitative data are collected at the same time, but within a larger quantitative or qualitative design. One type of data is secondary to the other.
- Explanatory sequential: Quantitative data is collected and analyzed first, followed by qualitative data. You can use this design if you think your qualitative data will explain and contextualize your quantitative findings.
- Exploratory sequential: Qualitative data is collected and analyzed first, followed by quantitative data. You can use this design if you think the quantitative data will confirm or validate your qualitative findings.
Triangulation in research means using multiple datasets, methods, theories and/or investigators to address a research question. It’s a research strategy that can help you enhance the validity and credibility of your findings.
Triangulation is mainly used in qualitative research , but it’s also commonly applied in quantitative research . Mixed methods research always uses triangulation.
In multistage sampling , or multistage cluster sampling, you draw a sample from a population using smaller and smaller groups at each stage.
This method is often used to collect data from a large, geographically spread group of people in national surveys, for example. You take advantage of hierarchical groupings (e.g., from state to city to neighborhood) to create a sample that’s less expensive and time-consuming to collect data from.
No, the steepness or slope of the line isn’t related to the correlation coefficient value. The correlation coefficient only tells you how closely your data fit on a line, so two datasets with the same correlation coefficient can have very different slopes.
To find the slope of the line, you’ll need to perform a regression analysis .
Correlation coefficients always range between -1 and 1.
The sign of the coefficient tells you the direction of the relationship: a positive value means the variables change together in the same direction, while a negative value means they change together in opposite directions.
The absolute value of a number is equal to the number without its sign. The absolute value of a correlation coefficient tells you the magnitude of the correlation: the greater the absolute value, the stronger the correlation.
These are the assumptions your data must meet if you want to use Pearson’s r :
- Both variables are on an interval or ratio level of measurement
- Data from both variables follow normal distributions
- Your data have no outliers
- Your data is from a random or representative sample
- You expect a linear relationship between the two variables
Quantitative research designs can be divided into two main categories:
- Correlational and descriptive designs are used to investigate characteristics, averages, trends, and associations between variables.
- Experimental and quasi-experimental designs are used to test causal relationships .
Qualitative research designs tend to be more flexible. Common types of qualitative design include case study , ethnography , and grounded theory designs.
A well-planned research design helps ensure that your methods match your research aims, that you collect high-quality data, and that you use the right kind of analysis to answer your questions, utilizing credible sources . This allows you to draw valid , trustworthy conclusions.
The priorities of a research design can vary depending on the field, but you usually have to specify:
- Your research questions and/or hypotheses
- Your overall approach (e.g., qualitative or quantitative )
- The type of design you’re using (e.g., a survey , experiment , or case study )
- Your sampling methods or criteria for selecting subjects
- Your data collection methods (e.g., questionnaires , observations)
- Your data collection procedures (e.g., operationalization , timing and data management)
- Your data analysis methods (e.g., statistical tests or thematic analysis )
A research design is a strategy for answering your research question . It defines your overall approach and determines how you will collect and analyze data.
Questionnaires can be self-administered or researcher-administered.
Self-administered questionnaires can be delivered online or in paper-and-pen formats, in person or through mail. All questions are standardized so that all respondents receive the same questions with identical wording.
Researcher-administered questionnaires are interviews that take place by phone, in-person, or online between researchers and respondents. You can gain deeper insights by clarifying questions for respondents or asking follow-up questions.
You can organize the questions logically, with a clear progression from simple to complex, or randomly between respondents. A logical flow helps respondents process the questionnaire easier and quicker, but it may lead to bias. Randomization can minimize the bias from order effects.
Closed-ended, or restricted-choice, questions offer respondents a fixed set of choices to select from. These questions are easier to answer quickly.
Open-ended or long-form questions allow respondents to answer in their own words. Because there are no restrictions on their choices, respondents can answer in ways that researchers may not have otherwise considered.
A questionnaire is a data collection tool or instrument, while a survey is an overarching research method that involves collecting and analyzing data from people using questionnaires.
The third variable and directionality problems are two main reasons why correlation isn’t causation .
The third variable problem means that a confounding variable affects both variables to make them seem causally related when they are not.
The directionality problem is when two variables correlate and might actually have a causal relationship, but it’s impossible to conclude which variable causes changes in the other.
Correlation describes an association between variables : when one variable changes, so does the other. A correlation is a statistical indicator of the relationship between variables.
Causation means that changes in one variable brings about changes in the other (i.e., there is a cause-and-effect relationship between variables). The two variables are correlated with each other, and there’s also a causal link between them.
While causation and correlation can exist simultaneously, correlation does not imply causation. In other words, correlation is simply a relationship where A relates to B—but A doesn’t necessarily cause B to happen (or vice versa). Mistaking correlation for causation is a common error and can lead to false cause fallacy .
Controlled experiments establish causality, whereas correlational studies only show associations between variables.
- In an experimental design , you manipulate an independent variable and measure its effect on a dependent variable. Other variables are controlled so they can’t impact the results.
- In a correlational design , you measure variables without manipulating any of them. You can test whether your variables change together, but you can’t be sure that one variable caused a change in another.
In general, correlational research is high in external validity while experimental research is high in internal validity .
A correlation is usually tested for two variables at a time, but you can test correlations between three or more variables.
A correlation coefficient is a single number that describes the strength and direction of the relationship between your variables.
Different types of correlation coefficients might be appropriate for your data based on their levels of measurement and distributions . The Pearson product-moment correlation coefficient (Pearson’s r ) is commonly used to assess a linear relationship between two quantitative variables.
A correlational research design investigates relationships between two variables (or more) without the researcher controlling or manipulating any of them. It’s a non-experimental type of quantitative research .
A correlation reflects the strength and/or direction of the association between two or more variables.
- A positive correlation means that both variables change in the same direction.
- A negative correlation means that the variables change in opposite directions.
- A zero correlation means there’s no relationship between the variables.
Random error is almost always present in scientific studies, even in highly controlled settings. While you can’t eradicate it completely, you can reduce random error by taking repeated measurements, using a large sample, and controlling extraneous variables .
You can avoid systematic error through careful design of your sampling , data collection , and analysis procedures. For example, use triangulation to measure your variables using multiple methods; regularly calibrate instruments or procedures; use random sampling and random assignment ; and apply masking (blinding) where possible.
Systematic error is generally a bigger problem in research.
With random error, multiple measurements will tend to cluster around the true value. When you’re collecting data from a large sample , the errors in different directions will cancel each other out.
Systematic errors are much more problematic because they can skew your data away from the true value. This can lead you to false conclusions ( Type I and II errors ) about the relationship between the variables you’re studying.
Random and systematic error are two types of measurement error.
Random error is a chance difference between the observed and true values of something (e.g., a researcher misreading a weighing scale records an incorrect measurement).
Systematic error is a consistent or proportional difference between the observed and true values of something (e.g., a miscalibrated scale consistently records weights as higher than they actually are).
On graphs, the explanatory variable is conventionally placed on the x-axis, while the response variable is placed on the y-axis.
- If you have quantitative variables , use a scatterplot or a line graph.
- If your response variable is categorical, use a scatterplot or a line graph.
- If your explanatory variable is categorical, use a bar graph.
The term “ explanatory variable ” is sometimes preferred over “ independent variable ” because, in real world contexts, independent variables are often influenced by other variables. This means they aren’t totally independent.
Multiple independent variables may also be correlated with each other, so “explanatory variables” is a more appropriate term.
The difference between explanatory and response variables is simple:
- An explanatory variable is the expected cause, and it explains the results.
- A response variable is the expected effect, and it responds to other variables.
In a controlled experiment , all extraneous variables are held constant so that they can’t influence the results. Controlled experiments require:
- A control group that receives a standard treatment, a fake treatment, or no treatment.
- Random assignment of participants to ensure the groups are equivalent.
Depending on your study topic, there are various other methods of controlling variables .
There are 4 main types of extraneous variables :
- Demand characteristics : environmental cues that encourage participants to conform to researchers’ expectations.
- Experimenter effects : unintentional actions by researchers that influence study outcomes.
- Situational variables : environmental variables that alter participants’ behaviors.
- Participant variables : any characteristic or aspect of a participant’s background that could affect study results.
An extraneous variable is any variable that you’re not investigating that can potentially affect the dependent variable of your research study.
A confounding variable is a type of extraneous variable that not only affects the dependent variable, but is also related to the independent variable.
In a factorial design, multiple independent variables are tested.
If you test two variables, each level of one independent variable is combined with each level of the other independent variable to create different conditions.
Within-subjects designs have many potential threats to internal validity , but they are also very statistically powerful .
Advantages:
- Only requires small samples
- Statistically powerful
- Removes the effects of individual differences on the outcomes
Disadvantages:
- Internal validity threats reduce the likelihood of establishing a direct relationship between variables
- Time-related effects, such as growth, can influence the outcomes
- Carryover effects mean that the specific order of different treatments affect the outcomes
While a between-subjects design has fewer threats to internal validity , it also requires more participants for high statistical power than a within-subjects design .
- Prevents carryover effects of learning and fatigue.
- Shorter study duration.
- Needs larger samples for high power.
- Uses more resources to recruit participants, administer sessions, cover costs, etc.
- Individual differences may be an alternative explanation for results.
Yes. Between-subjects and within-subjects designs can be combined in a single study when you have two or more independent variables (a factorial design). In a mixed factorial design, one variable is altered between subjects and another is altered within subjects.
In a between-subjects design , every participant experiences only one condition, and researchers assess group differences between participants in various conditions.
In a within-subjects design , each participant experiences all conditions, and researchers test the same participants repeatedly for differences between conditions.
The word “between” means that you’re comparing different conditions between groups, while the word “within” means you’re comparing different conditions within the same group.
Random assignment is used in experiments with a between-groups or independent measures design. In this research design, there’s usually a control group and one or more experimental groups. Random assignment helps ensure that the groups are comparable.
In general, you should always use random assignment in this type of experimental design when it is ethically possible and makes sense for your study topic.
To implement random assignment , assign a unique number to every member of your study’s sample .
Then, you can use a random number generator or a lottery method to randomly assign each number to a control or experimental group. You can also do so manually, by flipping a coin or rolling a dice to randomly assign participants to groups.
In experimental research, random assignment is a way of placing participants from your sample into different groups using randomization. With this method, every member of the sample has a known or equal chance of being placed in a control group or an experimental group.
“Controlling for a variable” means measuring extraneous variables and accounting for them statistically to remove their effects on other variables.
Researchers often model control variable data along with independent and dependent variable data in regression analyses and ANCOVAs . That way, you can isolate the control variable’s effects from the relationship between the variables of interest.
Control variables help you establish a correlational or causal relationship between variables by enhancing internal validity .
If you don’t control relevant extraneous variables , they may influence the outcomes of your study, and you may not be able to demonstrate that your results are really an effect of your independent variable .
A control variable is any variable that’s held constant in a research study. It’s not a variable of interest in the study, but it’s controlled because it could influence the outcomes.
Including mediators and moderators in your research helps you go beyond studying a simple relationship between two variables for a fuller picture of the real world. They are important to consider when studying complex correlational or causal relationships.
Mediators are part of the causal pathway of an effect, and they tell you how or why an effect takes place. Moderators usually help you judge the external validity of your study by identifying the limitations of when the relationship between variables holds.
If something is a mediating variable :
- It’s caused by the independent variable .
- It influences the dependent variable
- When it’s taken into account, the statistical correlation between the independent and dependent variables is higher than when it isn’t considered.
A confounder is a third variable that affects variables of interest and makes them seem related when they are not. In contrast, a mediator is the mechanism of a relationship between two variables: it explains the process by which they are related.
A mediator variable explains the process through which two variables are related, while a moderator variable affects the strength and direction of that relationship.
There are three key steps in systematic sampling :
- Define and list your population , ensuring that it is not ordered in a cyclical or periodic order.
- Decide on your sample size and calculate your interval, k , by dividing your population by your target sample size.
- Choose every k th member of the population as your sample.
Systematic sampling is a probability sampling method where researchers select members of the population at a regular interval – for example, by selecting every 15th person on a list of the population. If the population is in a random order, this can imitate the benefits of simple random sampling .
Yes, you can create a stratified sample using multiple characteristics, but you must ensure that every participant in your study belongs to one and only one subgroup. In this case, you multiply the numbers of subgroups for each characteristic to get the total number of groups.
For example, if you were stratifying by location with three subgroups (urban, rural, or suburban) and marital status with five subgroups (single, divorced, widowed, married, or partnered), you would have 3 x 5 = 15 subgroups.
You should use stratified sampling when your sample can be divided into mutually exclusive and exhaustive subgroups that you believe will take on different mean values for the variable that you’re studying.
Using stratified sampling will allow you to obtain more precise (with lower variance ) statistical estimates of whatever you are trying to measure.
For example, say you want to investigate how income differs based on educational attainment, but you know that this relationship can vary based on race. Using stratified sampling, you can ensure you obtain a large enough sample from each racial group, allowing you to draw more precise conclusions.
In stratified sampling , researchers divide subjects into subgroups called strata based on characteristics that they share (e.g., race, gender, educational attainment).
Once divided, each subgroup is randomly sampled using another probability sampling method.
Cluster sampling is more time- and cost-efficient than other probability sampling methods , particularly when it comes to large samples spread across a wide geographical area.
However, it provides less statistical certainty than other methods, such as simple random sampling , because it is difficult to ensure that your clusters properly represent the population as a whole.
There are three types of cluster sampling : single-stage, double-stage and multi-stage clustering. In all three types, you first divide the population into clusters, then randomly select clusters for use in your sample.
- In single-stage sampling , you collect data from every unit within the selected clusters.
- In double-stage sampling , you select a random sample of units from within the clusters.
- In multi-stage sampling , you repeat the procedure of randomly sampling elements from within the clusters until you have reached a manageable sample.
Cluster sampling is a probability sampling method in which you divide a population into clusters, such as districts or schools, and then randomly select some of these clusters as your sample.
The clusters should ideally each be mini-representations of the population as a whole.
If properly implemented, simple random sampling is usually the best sampling method for ensuring both internal and external validity . However, it can sometimes be impractical and expensive to implement, depending on the size of the population to be studied,
If you have a list of every member of the population and the ability to reach whichever members are selected, you can use simple random sampling.
The American Community Survey is an example of simple random sampling . In order to collect detailed data on the population of the US, the Census Bureau officials randomly select 3.5 million households per year and use a variety of methods to convince them to fill out the survey.
Simple random sampling is a type of probability sampling in which the researcher randomly selects a subset of participants from a population . Each member of the population has an equal chance of being selected. Data is then collected from as large a percentage as possible of this random subset.
Quasi-experimental design is most useful in situations where it would be unethical or impractical to run a true experiment .
Quasi-experiments have lower internal validity than true experiments, but they often have higher external validity as they can use real-world interventions instead of artificial laboratory settings.
A quasi-experiment is a type of research design that attempts to establish a cause-and-effect relationship. The main difference with a true experiment is that the groups are not randomly assigned.
Blinding is important to reduce research bias (e.g., observer bias , demand characteristics ) and ensure a study’s internal validity .
If participants know whether they are in a control or treatment group , they may adjust their behavior in ways that affect the outcome that researchers are trying to measure. If the people administering the treatment are aware of group assignment, they may treat participants differently and thus directly or indirectly influence the final results.
- In a single-blind study , only the participants are blinded.
- In a double-blind study , both participants and experimenters are blinded.
- In a triple-blind study , the assignment is hidden not only from participants and experimenters, but also from the researchers analyzing the data.
Blinding means hiding who is assigned to the treatment group and who is assigned to the control group in an experiment .
A true experiment (a.k.a. a controlled experiment) always includes at least one control group that doesn’t receive the experimental treatment.
However, some experiments use a within-subjects design to test treatments without a control group. In these designs, you usually compare one group’s outcomes before and after a treatment (instead of comparing outcomes between different groups).
For strong internal validity , it’s usually best to include a control group if possible. Without a control group, it’s harder to be certain that the outcome was caused by the experimental treatment and not by other variables.
An experimental group, also known as a treatment group, receives the treatment whose effect researchers wish to study, whereas a control group does not. They should be identical in all other ways.
Individual Likert-type questions are generally considered ordinal data , because the items have clear rank order, but don’t have an even distribution.
Overall Likert scale scores are sometimes treated as interval data. These scores are considered to have directionality and even spacing between them.
The type of data determines what statistical tests you should use to analyze your data.
A Likert scale is a rating scale that quantitatively assesses opinions, attitudes, or behaviors. It is made up of 4 or more questions that measure a single attitude or trait when response scores are combined.
To use a Likert scale in a survey , you present participants with Likert-type questions or statements, and a continuum of items, usually with 5 or 7 possible responses, to capture their degree of agreement.
In scientific research, concepts are the abstract ideas or phenomena that are being studied (e.g., educational achievement). Variables are properties or characteristics of the concept (e.g., performance at school), while indicators are ways of measuring or quantifying variables (e.g., yearly grade reports).
The process of turning abstract concepts into measurable variables and indicators is called operationalization .
There are various approaches to qualitative data analysis , but they all share five steps in common:
- Prepare and organize your data.
- Review and explore your data.
- Develop a data coding system.
- Assign codes to the data.
- Identify recurring themes.
The specifics of each step depend on the focus of the analysis. Some common approaches include textual analysis , thematic analysis , and discourse analysis .
There are five common approaches to qualitative research :
- Grounded theory involves collecting data in order to develop new theories.
- Ethnography involves immersing yourself in a group or organization to understand its culture.
- Narrative research involves interpreting stories to understand how people make sense of their experiences and perceptions.
- Phenomenological research involves investigating phenomena through people’s lived experiences.
- Action research links theory and practice in several cycles to drive innovative changes.
Hypothesis testing is a formal procedure for investigating our ideas about the world using statistics. It is used by scientists to test specific predictions, called hypotheses , by calculating how likely it is that a pattern or relationship between variables could have arisen by chance.
Operationalization means turning abstract conceptual ideas into measurable observations.
For example, the concept of social anxiety isn’t directly observable, but it can be operationally defined in terms of self-rating scores, behavioral avoidance of crowded places, or physical anxiety symptoms in social situations.
Before collecting data , it’s important to consider how you will operationalize the variables that you want to measure.
When conducting research, collecting original data has significant advantages:
- You can tailor data collection to your specific research aims (e.g. understanding the needs of your consumers or user testing your website)
- You can control and standardize the process for high reliability and validity (e.g. choosing appropriate measurements and sampling methods )
However, there are also some drawbacks: data collection can be time-consuming, labor-intensive and expensive. In some cases, it’s more efficient to use secondary data that has already been collected by someone else, but the data might be less reliable.
Data collection is the systematic process by which observations or measurements are gathered in research. It is used in many different contexts by academics, governments, businesses, and other organizations.
There are several methods you can use to decrease the impact of confounding variables on your research: restriction, matching, statistical control and randomization.
In restriction , you restrict your sample by only including certain subjects that have the same values of potential confounding variables.
In matching , you match each of the subjects in your treatment group with a counterpart in the comparison group. The matched subjects have the same values on any potential confounding variables, and only differ in the independent variable .
In statistical control , you include potential confounders as variables in your regression .
In randomization , you randomly assign the treatment (or independent variable) in your study to a sufficiently large number of subjects, which allows you to control for all potential confounding variables.
A confounding variable is closely related to both the independent and dependent variables in a study. An independent variable represents the supposed cause , while the dependent variable is the supposed effect . A confounding variable is a third variable that influences both the independent and dependent variables.
Failing to account for confounding variables can cause you to wrongly estimate the relationship between your independent and dependent variables.
To ensure the internal validity of your research, you must consider the impact of confounding variables. If you fail to account for them, you might over- or underestimate the causal relationship between your independent and dependent variables , or even find a causal relationship where none exists.
Yes, but including more than one of either type requires multiple research questions .
For example, if you are interested in the effect of a diet on health, you can use multiple measures of health: blood sugar, blood pressure, weight, pulse, and many more. Each of these is its own dependent variable with its own research question.
You could also choose to look at the effect of exercise levels as well as diet, or even the additional effect of the two combined. Each of these is a separate independent variable .
To ensure the internal validity of an experiment , you should only change one independent variable at a time.
No. The value of a dependent variable depends on an independent variable, so a variable cannot be both independent and dependent at the same time. It must be either the cause or the effect, not both!
You want to find out how blood sugar levels are affected by drinking diet soda and regular soda, so you conduct an experiment .
- The type of soda – diet or regular – is the independent variable .
- The level of blood sugar that you measure is the dependent variable – it changes depending on the type of soda.
Determining cause and effect is one of the most important parts of scientific research. It’s essential to know which is the cause – the independent variable – and which is the effect – the dependent variable.
In non-probability sampling , the sample is selected based on non-random criteria, and not every member of the population has a chance of being included.
Common non-probability sampling methods include convenience sampling , voluntary response sampling, purposive sampling , snowball sampling, and quota sampling .
Probability sampling means that every member of the target population has a known chance of being included in the sample.
Probability sampling methods include simple random sampling , systematic sampling , stratified sampling , and cluster sampling .
Using careful research design and sampling procedures can help you avoid sampling bias . Oversampling can be used to correct undercoverage bias .
Some common types of sampling bias include self-selection bias , nonresponse bias , undercoverage bias , survivorship bias , pre-screening or advertising bias, and healthy user bias.
Sampling bias is a threat to external validity – it limits the generalizability of your findings to a broader group of people.
A sampling error is the difference between a population parameter and a sample statistic .
A statistic refers to measures about the sample , while a parameter refers to measures about the population .
Populations are used when a research question requires data from every member of the population. This is usually only feasible when the population is small and easily accessible.
Samples are used to make inferences about populations . Samples are easier to collect data from because they are practical, cost-effective, convenient, and manageable.
There are seven threats to external validity : selection bias , history, experimenter effect, Hawthorne effect , testing effect, aptitude-treatment and situation effect.
The two types of external validity are population validity (whether you can generalize to other groups of people) and ecological validity (whether you can generalize to other situations and settings).
The external validity of a study is the extent to which you can generalize your findings to different groups of people, situations, and measures.
Cross-sectional studies cannot establish a cause-and-effect relationship or analyze behavior over a period of time. To investigate cause and effect, you need to do a longitudinal study or an experimental study .
Cross-sectional studies are less expensive and time-consuming than many other types of study. They can provide useful insights into a population’s characteristics and identify correlations for further research.
Sometimes only cross-sectional data is available for analysis; other times your research question may only require a cross-sectional study to answer it.
Longitudinal studies can last anywhere from weeks to decades, although they tend to be at least a year long.
The 1970 British Cohort Study , which has collected data on the lives of 17,000 Brits since their births in 1970, is one well-known example of a longitudinal study .
Longitudinal studies are better to establish the correct sequence of events, identify changes over time, and provide insight into cause-and-effect relationships, but they also tend to be more expensive and time-consuming than other types of studies.
Longitudinal studies and cross-sectional studies are two different types of research design . In a cross-sectional study you collect data from a population at a specific point in time; in a longitudinal study you repeatedly collect data from the same sample over an extended period of time.
Longitudinal study | Cross-sectional study |
---|---|
observations | Observations at a in time |
Observes the multiple times | Observes (a “cross-section”) in the population |
Follows in participants over time | Provides of society at a given point |
There are eight threats to internal validity : history, maturation, instrumentation, testing, selection bias , regression to the mean, social interaction and attrition .
Internal validity is the extent to which you can be confident that a cause-and-effect relationship established in a study cannot be explained by other factors.
In mixed methods research , you use both qualitative and quantitative data collection and analysis methods to answer your research question .
The research methods you use depend on the type of data you need to answer your research question .
- If you want to measure something or test a hypothesis , use quantitative methods . If you want to explore ideas, thoughts and meanings, use qualitative methods .
- If you want to analyze a large amount of readily-available data, use secondary data. If you want data specific to your purposes with control over how it is generated, collect primary data.
- If you want to establish cause-and-effect relationships between variables , use experimental methods. If you want to understand the characteristics of a research subject, use descriptive methods.
A confounding variable , also called a confounder or confounding factor, is a third variable in a study examining a potential cause-and-effect relationship.
A confounding variable is related to both the supposed cause and the supposed effect of the study. It can be difficult to separate the true effect of the independent variable from the effect of the confounding variable.
In your research design , it’s important to identify potential confounding variables and plan how you will reduce their impact.
Discrete and continuous variables are two types of quantitative variables :
- Discrete variables represent counts (e.g. the number of objects in a collection).
- Continuous variables represent measurable amounts (e.g. water volume or weight).
Quantitative variables are any variables where the data represent amounts (e.g. height, weight, or age).
Categorical variables are any variables where the data represent groups. This includes rankings (e.g. finishing places in a race), classifications (e.g. brands of cereal), and binary outcomes (e.g. coin flips).
You need to know what type of variables you are working with to choose the right statistical test for your data and interpret your results .
You can think of independent and dependent variables in terms of cause and effect: an independent variable is the variable you think is the cause , while a dependent variable is the effect .
In an experiment, you manipulate the independent variable and measure the outcome in the dependent variable. For example, in an experiment about the effect of nutrients on crop growth:
- The independent variable is the amount of nutrients added to the crop field.
- The dependent variable is the biomass of the crops at harvest time.
Defining your variables, and deciding how you will manipulate and measure them, is an important part of experimental design .
Experimental design means planning a set of procedures to investigate a relationship between variables . To design a controlled experiment, you need:
- A testable hypothesis
- At least one independent variable that can be precisely manipulated
- At least one dependent variable that can be precisely measured
When designing the experiment, you decide:
- How you will manipulate the variable(s)
- How you will control for any potential confounding variables
- How many subjects or samples will be included in the study
- How subjects will be assigned to treatment levels
Experimental design is essential to the internal and external validity of your experiment.
I nternal validity is the degree of confidence that the causal relationship you are testing is not influenced by other factors or variables .
External validity is the extent to which your results can be generalized to other contexts.
The validity of your experiment depends on your experimental design .
Reliability and validity are both about how well a method measures something:
- Reliability refers to the consistency of a measure (whether the results can be reproduced under the same conditions).
- Validity refers to the accuracy of a measure (whether the results really do represent what they are supposed to measure).
If you are doing experimental research, you also have to consider the internal and external validity of your experiment.
A sample is a subset of individuals from a larger population . Sampling means selecting the group that you will actually collect data from in your research. For example, if you are researching the opinions of students in your university, you could survey a sample of 100 students.
In statistics, sampling allows you to test a hypothesis about the characteristics of a population.
Quantitative research deals with numbers and statistics, while qualitative research deals with words and meanings.
Quantitative methods allow you to systematically measure variables and test hypotheses . Qualitative methods allow you to explore concepts and experiences in more detail.
Methodology refers to the overarching strategy and rationale of your research project . It involves studying the methods used in your field and the theories or principles behind them, in order to develop an approach that matches your objectives.
Methods are the specific tools and procedures you use to collect and analyze data (for example, experiments, surveys , and statistical tests ).
In shorter scientific papers, where the aim is to report the findings of a specific study, you might simply describe what you did in a methods section .
In a longer or more complex research project, such as a thesis or dissertation , you will probably include a methodology section , where you explain your approach to answering the research questions and cite relevant sources to support your choice of methods.
Ask our team
Want to contact us directly? No problem. We are always here for you.
- Email [email protected]
- Start live chat
- Call +1 (510) 822-8066
- WhatsApp +31 20 261 6040
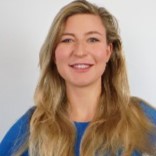
Our team helps students graduate by offering:
- A world-class citation generator
- Plagiarism Checker software powered by Turnitin
- Innovative Citation Checker software
- Professional proofreading services
- Over 300 helpful articles about academic writing, citing sources, plagiarism, and more
Scribbr specializes in editing study-related documents . We proofread:
- PhD dissertations
- Research proposals
- Personal statements
- Admission essays
- Motivation letters
- Reflection papers
- Journal articles
- Capstone projects
Scribbr’s Plagiarism Checker is powered by elements of Turnitin’s Similarity Checker , namely the plagiarism detection software and the Internet Archive and Premium Scholarly Publications content databases .
The add-on AI detector is powered by Scribbr’s proprietary software.
The Scribbr Citation Generator is developed using the open-source Citation Style Language (CSL) project and Frank Bennett’s citeproc-js . It’s the same technology used by dozens of other popular citation tools, including Mendeley and Zotero.
You can find all the citation styles and locales used in the Scribbr Citation Generator in our publicly accessible repository on Github .
- Bipolar Disorder
- Therapy Center
- When To See a Therapist
- Types of Therapy
- Best Online Therapy
- Best Couples Therapy
- Managing Stress
- Sleep and Dreaming
- Understanding Emotions
- Self-Improvement
- Healthy Relationships
- Student Resources
- Personality Types
- Sweepstakes
- Guided Meditations
- Verywell Mind Insights
- 2024 Verywell Mind 25
- Mental Health in the Classroom
- Editorial Process
- Meet Our Review Board
- Crisis Support
How the Experimental Method Works in Psychology
sturti/Getty Images
The Experimental Process
Types of experiments, potential pitfalls of the experimental method.
The experimental method is a type of research procedure that involves manipulating variables to determine if there is a cause-and-effect relationship. The results obtained through the experimental method are useful but do not prove with 100% certainty that a singular cause always creates a specific effect. Instead, they show the probability that a cause will or will not lead to a particular effect.
At a Glance
While there are many different research techniques available, the experimental method allows researchers to look at cause-and-effect relationships. Using the experimental method, researchers randomly assign participants to a control or experimental group and manipulate levels of an independent variable. If changes in the independent variable lead to changes in the dependent variable, it indicates there is likely a causal relationship between them.
What Is the Experimental Method in Psychology?
The experimental method involves manipulating one variable to determine if this causes changes in another variable. This method relies on controlled research methods and random assignment of study subjects to test a hypothesis.
For example, researchers may want to learn how different visual patterns may impact our perception. Or they might wonder whether certain actions can improve memory . Experiments are conducted on many behavioral topics, including:
The scientific method forms the basis of the experimental method. This is a process used to determine the relationship between two variables—in this case, to explain human behavior .
Positivism is also important in the experimental method. It refers to factual knowledge that is obtained through observation, which is considered to be trustworthy.
When using the experimental method, researchers first identify and define key variables. Then they formulate a hypothesis, manipulate the variables, and collect data on the results. Unrelated or irrelevant variables are carefully controlled to minimize the potential impact on the experiment outcome.
History of the Experimental Method
The idea of using experiments to better understand human psychology began toward the end of the nineteenth century. Wilhelm Wundt established the first formal laboratory in 1879.
Wundt is often called the father of experimental psychology. He believed that experiments could help explain how psychology works, and used this approach to study consciousness .
Wundt coined the term "physiological psychology." This is a hybrid of physiology and psychology, or how the body affects the brain.
Other early contributors to the development and evolution of experimental psychology as we know it today include:
- Gustav Fechner (1801-1887), who helped develop procedures for measuring sensations according to the size of the stimulus
- Hermann von Helmholtz (1821-1894), who analyzed philosophical assumptions through research in an attempt to arrive at scientific conclusions
- Franz Brentano (1838-1917), who called for a combination of first-person and third-person research methods when studying psychology
- Georg Elias Müller (1850-1934), who performed an early experiment on attitude which involved the sensory discrimination of weights and revealed how anticipation can affect this discrimination
Key Terms to Know
To understand how the experimental method works, it is important to know some key terms.
Dependent Variable
The dependent variable is the effect that the experimenter is measuring. If a researcher was investigating how sleep influences test scores, for example, the test scores would be the dependent variable.
Independent Variable
The independent variable is the variable that the experimenter manipulates. In the previous example, the amount of sleep an individual gets would be the independent variable.
A hypothesis is a tentative statement or a guess about the possible relationship between two or more variables. In looking at how sleep influences test scores, the researcher might hypothesize that people who get more sleep will perform better on a math test the following day. The purpose of the experiment, then, is to either support or reject this hypothesis.
Operational definitions are necessary when performing an experiment. When we say that something is an independent or dependent variable, we must have a very clear and specific definition of the meaning and scope of that variable.
Extraneous Variables
Extraneous variables are other variables that may also affect the outcome of an experiment. Types of extraneous variables include participant variables, situational variables, demand characteristics, and experimenter effects. In some cases, researchers can take steps to control for extraneous variables.
Demand Characteristics
Demand characteristics are subtle hints that indicate what an experimenter is hoping to find in a psychology experiment. This can sometimes cause participants to alter their behavior, which can affect the results of the experiment.
Intervening Variables
Intervening variables are factors that can affect the relationship between two other variables.
Confounding Variables
Confounding variables are variables that can affect the dependent variable, but that experimenters cannot control for. Confounding variables can make it difficult to determine if the effect was due to changes in the independent variable or if the confounding variable may have played a role.
Psychologists, like other scientists, use the scientific method when conducting an experiment. The scientific method is a set of procedures and principles that guide how scientists develop research questions, collect data, and come to conclusions.
The five basic steps of the experimental process are:
- Identifying a problem to study
- Devising the research protocol
- Conducting the experiment
- Analyzing the data collected
- Sharing the findings (usually in writing or via presentation)
Most psychology students are expected to use the experimental method at some point in their academic careers. Learning how to conduct an experiment is important to understanding how psychologists prove and disprove theories in this field.
There are a few different types of experiments that researchers might use when studying psychology. Each has pros and cons depending on the participants being studied, the hypothesis, and the resources available to conduct the research.
Lab Experiments
Lab experiments are common in psychology because they allow experimenters more control over the variables. These experiments can also be easier for other researchers to replicate. The drawback of this research type is that what takes place in a lab is not always what takes place in the real world.
Field Experiments
Sometimes researchers opt to conduct their experiments in the field. For example, a social psychologist interested in researching prosocial behavior might have a person pretend to faint and observe how long it takes onlookers to respond.
This type of experiment can be a great way to see behavioral responses in realistic settings. But it is more difficult for researchers to control the many variables existing in these settings that could potentially influence the experiment's results.
Quasi-Experiments
While lab experiments are known as true experiments, researchers can also utilize a quasi-experiment. Quasi-experiments are often referred to as natural experiments because the researchers do not have true control over the independent variable.
A researcher looking at personality differences and birth order, for example, is not able to manipulate the independent variable in the situation (personality traits). Participants also cannot be randomly assigned because they naturally fall into pre-existing groups based on their birth order.
So why would a researcher use a quasi-experiment? This is a good choice in situations where scientists are interested in studying phenomena in natural, real-world settings. It's also beneficial if there are limits on research funds or time.
Field experiments can be either quasi-experiments or true experiments.
Examples of the Experimental Method in Use
The experimental method can provide insight into human thoughts and behaviors, Researchers use experiments to study many aspects of psychology.
A 2019 study investigated whether splitting attention between electronic devices and classroom lectures had an effect on college students' learning abilities. It found that dividing attention between these two mediums did not affect lecture comprehension. However, it did impact long-term retention of the lecture information, which affected students' exam performance.
An experiment used participants' eye movements and electroencephalogram (EEG) data to better understand cognitive processing differences between experts and novices. It found that experts had higher power in their theta brain waves than novices, suggesting that they also had a higher cognitive load.
A study looked at whether chatting online with a computer via a chatbot changed the positive effects of emotional disclosure often received when talking with an actual human. It found that the effects were the same in both cases.
One experimental study evaluated whether exercise timing impacts information recall. It found that engaging in exercise prior to performing a memory task helped improve participants' short-term memory abilities.
Sometimes researchers use the experimental method to get a bigger-picture view of psychological behaviors and impacts. For example, one 2018 study examined several lab experiments to learn more about the impact of various environmental factors on building occupant perceptions.
A 2020 study set out to determine the role that sensation-seeking plays in political violence. This research found that sensation-seeking individuals have a higher propensity for engaging in political violence. It also found that providing access to a more peaceful, yet still exciting political group helps reduce this effect.
While the experimental method can be a valuable tool for learning more about psychology and its impacts, it also comes with a few pitfalls.
Experiments may produce artificial results, which are difficult to apply to real-world situations. Similarly, researcher bias can impact the data collected. Results may not be able to be reproduced, meaning the results have low reliability .
Since humans are unpredictable and their behavior can be subjective, it can be hard to measure responses in an experiment. In addition, political pressure may alter the results. The subjects may not be a good representation of the population, or groups used may not be comparable.
And finally, since researchers are human too, results may be degraded due to human error.
What This Means For You
Every psychological research method has its pros and cons. The experimental method can help establish cause and effect, and it's also beneficial when research funds are limited or time is of the essence.
At the same time, it's essential to be aware of this method's pitfalls, such as how biases can affect the results or the potential for low reliability. Keeping these in mind can help you review and assess research studies more accurately, giving you a better idea of whether the results can be trusted or have limitations.
Colorado State University. Experimental and quasi-experimental research .
American Psychological Association. Experimental psychology studies human and animals .
Mayrhofer R, Kuhbandner C, Lindner C. The practice of experimental psychology: An inevitably postmodern endeavor . Front Psychol . 2021;11:612805. doi:10.3389/fpsyg.2020.612805
Mandler G. A History of Modern Experimental Psychology .
Stanford University. Wilhelm Maximilian Wundt . Stanford Encyclopedia of Philosophy.
Britannica. Gustav Fechner .
Britannica. Hermann von Helmholtz .
Meyer A, Hackert B, Weger U. Franz Brentano and the beginning of experimental psychology: implications for the study of psychological phenomena today . Psychol Res . 2018;82:245-254. doi:10.1007/s00426-016-0825-7
Britannica. Georg Elias Müller .
McCambridge J, de Bruin M, Witton J. The effects of demand characteristics on research participant behaviours in non-laboratory settings: A systematic review . PLoS ONE . 2012;7(6):e39116. doi:10.1371/journal.pone.0039116
Laboratory experiments . In: The Sage Encyclopedia of Communication Research Methods. Allen M, ed. SAGE Publications, Inc. doi:10.4135/9781483381411.n287
Schweizer M, Braun B, Milstone A. Research methods in healthcare epidemiology and antimicrobial stewardship — quasi-experimental designs . Infect Control Hosp Epidemiol . 2016;37(10):1135-1140. doi:10.1017/ice.2016.117
Glass A, Kang M. Dividing attention in the classroom reduces exam performance . Educ Psychol . 2019;39(3):395-408. doi:10.1080/01443410.2018.1489046
Keskin M, Ooms K, Dogru AO, De Maeyer P. Exploring the cognitive load of expert and novice map users using EEG and eye tracking . ISPRS Int J Geo-Inf . 2020;9(7):429. doi:10.3390.ijgi9070429
Ho A, Hancock J, Miner A. Psychological, relational, and emotional effects of self-disclosure after conversations with a chatbot . J Commun . 2018;68(4):712-733. doi:10.1093/joc/jqy026
Haynes IV J, Frith E, Sng E, Loprinzi P. Experimental effects of acute exercise on episodic memory function: Considerations for the timing of exercise . Psychol Rep . 2018;122(5):1744-1754. doi:10.1177/0033294118786688
Torresin S, Pernigotto G, Cappelletti F, Gasparella A. Combined effects of environmental factors on human perception and objective performance: A review of experimental laboratory works . Indoor Air . 2018;28(4):525-538. doi:10.1111/ina.12457
Schumpe BM, Belanger JJ, Moyano M, Nisa CF. The role of sensation seeking in political violence: An extension of the significance quest theory . J Personal Social Psychol . 2020;118(4):743-761. doi:10.1037/pspp0000223
By Kendra Cherry, MSEd Kendra Cherry, MS, is a psychosocial rehabilitation specialist, psychology educator, and author of the "Everything Psychology Book."
- Skip to secondary menu
- Skip to main content
- Skip to primary sidebar
Statistics By Jim
Making statistics intuitive
Random Assignment in Experiments
By Jim Frost 4 Comments
Random assignment uses chance to assign subjects to the control and treatment groups in an experiment. This process helps ensure that the groups are equivalent at the beginning of the study, which makes it safer to assume the treatments caused any differences between groups that the experimenters observe at the end of the study.

Huh? That might be a big surprise! At this point, you might be wondering about all of those studies that use statistics to assess the effects of different treatments. There’s a critical separation between significance and causality:
- Statistical procedures determine whether an effect is significant.
- Experimental designs determine how confidently you can assume that a treatment causes the effect.
In this post, learn how using random assignment in experiments can help you identify causal relationships.
Correlation, Causation, and Confounding Variables
Random assignment helps you separate causation from correlation and rule out confounding variables. As a critical component of the scientific method , experiments typically set up contrasts between a control group and one or more treatment groups. The idea is to determine whether the effect, which is the difference between a treatment group and the control group, is statistically significant. If the effect is significant, group assignment correlates with different outcomes.
However, as you have no doubt heard, correlation does not necessarily imply causation. In other words, the experimental groups can have different mean outcomes, but the treatment might not be causing those differences even though the differences are statistically significant.
The difficulty in definitively stating that a treatment caused the difference is due to potential confounding variables or confounders. Confounders are alternative explanations for differences between the experimental groups. Confounding variables correlate with both the experimental groups and the outcome variable. In this situation, confounding variables can be the actual cause for the outcome differences rather than the treatments themselves. As you’ll see, if an experiment does not account for confounding variables, they can bias the results and make them untrustworthy.
Related posts : Understanding Correlation in Statistics , Causation versus Correlation , and Hill’s Criteria for Causation .
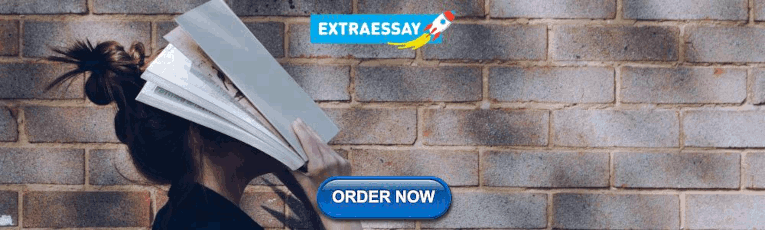
Example of Confounding in an Experiment

- Control group: Does not consume vitamin supplements
- Treatment group: Regularly consumes vitamin supplements.
Imagine we measure a specific health outcome. After the experiment is complete, we perform a 2-sample t-test to determine whether the mean outcomes for these two groups are different. Assume the test results indicate that the mean health outcome in the treatment group is significantly better than the control group.
Why can’t we assume that the vitamins improved the health outcomes? After all, only the treatment group took the vitamins.
Related post : Confounding Variables in Regression Analysis
Alternative Explanations for Differences in Outcomes
The answer to that question depends on how we assigned the subjects to the experimental groups. If we let the subjects decide which group to join based on their existing vitamin habits, it opens the door to confounding variables. It’s reasonable to assume that people who take vitamins regularly also tend to have other healthy habits. These habits are confounders because they correlate with both vitamin consumption (experimental group) and the health outcome measure.
Random assignment prevents this self sorting of participants and reduces the likelihood that the groups start with systematic differences.
In fact, studies have found that supplement users are more physically active, have healthier diets, have lower blood pressure, and so on compared to those who don’t take supplements. If subjects who already take vitamins regularly join the treatment group voluntarily, they bring these healthy habits disproportionately to the treatment group. Consequently, these habits will be much more prevalent in the treatment group than the control group.
The healthy habits are the confounding variables—the potential alternative explanations for the difference in our study’s health outcome. It’s entirely possible that these systematic differences between groups at the start of the study might cause the difference in the health outcome at the end of the study—and not the vitamin consumption itself!
If our experiment doesn’t account for these confounding variables, we can’t trust the results. While we obtained statistically significant results with the 2-sample t-test for health outcomes, we don’t know for sure whether the vitamins, the systematic difference in habits, or some combination of the two caused the improvements.
Learn why many randomized clinical experiments use a placebo to control for the Placebo Effect .
Experiments Must Account for Confounding Variables
Your experimental design must account for confounding variables to avoid their problems. Scientific studies commonly use the following methods to handle confounders:
- Use control variables to keep them constant throughout an experiment.
- Statistically control for them in an observational study.
- Use random assignment to reduce the likelihood that systematic differences exist between experimental groups when the study begins.
Let’s take a look at how random assignment works in an experimental design.
Random Assignment Can Reduce the Impact of Confounding Variables
Note that random assignment is different than random sampling. Random sampling is a process for obtaining a sample that accurately represents a population .

Random assignment uses a chance process to assign subjects to experimental groups. Using random assignment requires that the experimenters can control the group assignment for all study subjects. For our study, we must be able to assign our participants to either the control group or the supplement group. Clearly, if we don’t have the ability to assign subjects to the groups, we can’t use random assignment!
Additionally, the process must have an equal probability of assigning a subject to any of the groups. For example, in our vitamin supplement study, we can use a coin toss to assign each subject to either the control group or supplement group. For more complex experimental designs, we can use a random number generator or even draw names out of a hat.
Random Assignment Distributes Confounders Equally
The random assignment process distributes confounding properties amongst your experimental groups equally. In other words, randomness helps eliminate systematic differences between groups. For our study, flipping the coin tends to equalize the distribution of subjects with healthier habits between the control and treatment group. Consequently, these two groups should start roughly equal for all confounding variables, including healthy habits!
Random assignment is a simple, elegant solution to a complex problem. For any given study area, there can be a long list of confounding variables that you could worry about. However, using random assignment, you don’t need to know what they are, how to detect them, or even measure them. Instead, use random assignment to equalize them across your experimental groups so they’re not a problem.
Because random assignment helps ensure that the groups are comparable when the experiment begins, you can be more confident that the treatments caused the post-study differences. Random assignment helps increase the internal validity of your study.
Comparing the Vitamin Study With and Without Random Assignment
Let’s compare two scenarios involving our hypothetical vitamin study. We’ll assume that the study obtains statistically significant results in both cases.
Scenario 1: We don’t use random assignment and, unbeknownst to us, subjects with healthier habits disproportionately end up in the supplement treatment group. The experimental groups differ by both healthy habits and vitamin consumption. Consequently, we can’t determine whether it was the habits or vitamins that improved the outcomes.
Scenario 2: We use random assignment and, consequently, the treatment and control groups start with roughly equal levels of healthy habits. The intentional introduction of vitamin supplements in the treatment group is the primary difference between the groups. Consequently, we can more confidently assert that the supplements caused an improvement in health outcomes.
For both scenarios, the statistical results could be identical. However, the methodology behind the second scenario makes a stronger case for a causal relationship between vitamin supplement consumption and health outcomes.
How important is it to use the correct methodology? Well, if the relationship between vitamins and health outcomes is not causal, then consuming vitamins won’t cause your health outcomes to improve regardless of what the study indicates. Instead, it’s probably all the other healthy habits!
Learn more about Randomized Controlled Trials (RCTs) that are the gold standard for identifying causal relationships because they use random assignment.
Drawbacks of Random Assignment
Random assignment helps reduce the chances of systematic differences between the groups at the start of an experiment and, thereby, mitigates the threats of confounding variables and alternative explanations. However, the process does not always equalize all of the confounding variables. Its random nature tends to eliminate systematic differences, but it doesn’t always succeed.
Sometimes random assignment is impossible because the experimenters cannot control the treatment or independent variable. For example, if you want to determine how individuals with and without depression perform on a test, you cannot randomly assign subjects to these groups. The same difficulty occurs when you’re studying differences between genders.
In other cases, there might be ethical issues. For example, in a randomized experiment, the researchers would want to withhold treatment for the control group. However, if the treatments are vaccinations, it might be unethical to withhold the vaccinations.
Other times, random assignment might be possible, but it is very challenging. For example, with vitamin consumption, it’s generally thought that if vitamin supplements cause health improvements, it’s only after very long-term use. It’s hard to enforce random assignment with a strict regimen for usage in one group and non-usage in the other group over the long-run. Or imagine a study about smoking. The researchers would find it difficult to assign subjects to the smoking and non-smoking groups randomly!
Fortunately, if you can’t use random assignment to help reduce the problem of confounding variables, there are different methods available. The other primary approach is to perform an observational study and incorporate the confounders into the statistical model itself. For more information, read my post Observational Studies Explained .
Read About Real Experiments that Used Random Assignment
I’ve written several blog posts about studies that have used random assignment to make causal inferences. Read studies about the following:
- Flu Vaccinations
- COVID-19 Vaccinations
Sullivan L. Random assignment versus random selection . SAGE Glossary of the Social and Behavioral Sciences, SAGE Publications, Inc.; 2009.
Share this:

Reader Interactions
November 13, 2019 at 4:59 am
Hi Jim, I have a question of randomly assigning participants to one of two conditions when it is an ongoing study and you are not sure of how many participants there will be. I am using this random assignment tool for factorial experiments. http://methodologymedia.psu.edu/most/rannumgenerator It asks you for the total number of participants but at this point, I am not sure how many there will be. Thanks for any advice you can give me, Floyd
May 28, 2019 at 11:34 am
Jim, can you comment on the validity of using the following approach when we can’t use random assignments. I’m in education, we have an ACT prep course that we offer. We can’t force students to take it and we can’t keep them from taking it either. But we want to know if it’s working. Let’s say that by senior year all students who are going to take the ACT have taken it. Let’s also say that I’m only including students who have taking it twice (so I can show growth between first and second time taking it). What I’ve done to address confounders is to go back to say 8th or 9th grade (prior to anyone taking the ACT or the ACT prep course) and run an analysis showing the two groups are not significantly different to start with. Is this valid? If the ACT prep students were higher achievers in 8th or 9th grade, I could not assume my prep course is effecting greater growth, but if they were not significantly different in 8th or 9th grade, I can assume the significant difference in ACT growth (from first to second testing) is due to the prep course. Yes or no?
May 26, 2019 at 5:37 pm
Nice post! I think the key to understanding scientific research is to understand randomization. And most people don’t get it.
May 27, 2019 at 9:48 pm
Thank you, Anoop!
I think randomness in an experiment is a funny thing. The issue of confounding factors is a serious problem. You might not even know what they are! But, use random assignment and, voila, the problem usually goes away! If you can’t use random assignment, suddenly you have a whole host of issues to worry about, which I’ll be writing about in more detail in my upcoming post about observational experiments!
Comments and Questions Cancel reply
- Search Search Please fill out this field.
What Is Simple Random Sampling?
- Simple Random Sample
- Disadvantages
- Random Sampling FAQs
The Bottom Line
Simple random sampling definition, advantages and disadvantage.
:max_bytes(150000):strip_icc():format(webp)/picture-53894-1440689455-5bfc2a8846e0fb00260af532.jpg)
Simple random sampling is a technique in which a researcher selects a random subset of people from a larger group or population. In simple random sampling, each member of the group has an equal chance of getting selected. The method is commonly used in statistics to obtain a sample that is representative of the larger population.
Statistics is a branch of applied mathematics that helps us learn about large datasets by studying smaller events or objects. Put simply, you can make inferences about a large population by examining a smaller sample. Statistical analysis is commonly used to identify trends in many different areas, including business and finance. Individuals can use findings from statistical research to make better decisions about their money, businesses, and investments.
The simple random sampling method allows researchers to statistically measure a subset of individuals selected from a larger group or population to approximate a response from the entire group. This research method has both benefits and drawbacks. We highlight these pros and cons in this article, along with an overview of simple random sampling.
Key Takeaways
- A simple random sample is one of the methods researchers use to choose a sample from a larger population.
- This method works if there is an equal chance that any of the subjects in a population will be chosen.
- Researchers choose simple random sampling to make generalizations about a population.
- Major advantages include its simplicity and lack of bias.
- Among the disadvantages are difficulty gaining access to a list of a larger population, time, costs, and that bias can still occur under certain circumstances.
Simple Random Sample: An Overview
As noted above, simple random sampling involves choosing a smaller subset of a larger population. This is done randomly. But the catch here is that there is an equal chance that any of the samples in the subset will be chosen. Researchers tend to choose this method of sampling when they want to make generalizations about the larger population.
Simple random sampling can be conducted by using:
- The lottery method. This method involves assigning a number to each member of the dataset then choosing a prescribed set of numbers from those members at random.
- Technology. Using software programs like Excel makes it easier to conduct random sampling. Researchers just have to make sure that all the formulas and inputs are correctly laid out.
For simple random sampling to work, researchers must know the total population size. They must also be able to remove all hints of bias as simple random sampling is meant to be a completely unbiased approach to garner responses from a large group.
Keep in mind that there is room for error with random sampling. This is noted by adding a plus or minus variance to the results. In order to avoid any errors, researchers must study the entire population, which for all intents and purposes, isn't always possible.
To ensure bias does not occur, researchers must acquire responses from an adequate number of respondents, which may not be possible due to time or budget constraints.
Advantages of a Simple Random Sample
Simple random sampling may be simple to perform (as the name suggests) but it isn't used that often. But that doesn't mean it shouldn't be used. As long as it is done properly, there are certain distinct advantages to this sampling method.
Lack of Bias
The use of simple random sampling removes all hints of bias —or at least it should. Because individuals who make up the subset of the larger group are chosen at random, each individual in the large population set has the same probability of being selected. In most cases, this creates a balanced subset that carries the greatest potential for representing the larger group as a whole.
Here's a simple way to show how a researcher can remove bias when conducting simple random sampling. Let's say there are 100 bingo balls in a bowl, from which the researcher must choose 10. In order to remove any bias, the individual must close their eyes or look away when choosing the balls.
As its name implies, producing a simple random sample is much less complicated than other methods . There are no special skills involved in using this method, which can result in a fairly reliable outcome. This is in contrast to other sampling methods like stratified random sampling . This method involves dividing larger groups into smaller subgroups that are called strata. Members are divided up into these groups based on any attributes they share. As mentioned, individuals in the subset are selected randomly and there are no additional steps.
Less Knowledge Required
We've already established that simple random sampling is a very simple sampling method to execute. But there's also another, similar benefit: It requires little to no special knowledge. This means that the individual conducting the research doesn't need to have any information or knowledge about the larger population in order to effectively do their job.
Be sure that the sample subset from the larger group is inclusive enough. A sample that doesn't adequately reflect the population as a whole will result in a skewed result.
Disadvantages of a Simple Random Sample
Although there are distinct advantages to using a simple random sample, it does come with inherent drawbacks. These disadvantages include the time needed to gather the full list of a specific population, the capital necessary to retrieve and contact that list, and the bias that could occur when the sample set is not large enough to adequately represent the full population. We go into more detail below.
Difficulty Accessing Lists of the Full Population
An accurate statistical measure of a large population can only be obtained in simple random sampling when a full list of the entire population to be studied is available. Think of a list of students at a university or a group of employees at a specific company.
The problem lies in the accessibility of these lists. As such, getting access to the whole list can present challenges. Some universities or colleges may not want to provide a complete list of students or faculty for research. Similarly, specific companies may not be willing or able to hand over information about employee groups due to privacy policies.
Time Consuming
When a full list of a larger population is not available, individuals attempting to conduct simple random sampling must gather information from other sources. If publicly available, smaller subset lists can be used to recreate a full list of a larger population, but this strategy takes time to complete.
Organizations that keep data on students, employees, and individual consumers often impose lengthy retrieval processes that can stall a researcher's ability to obtain the most accurate information on the entire population set.
In addition to the time it takes to gather information from various sources, the process may cost a company or individual a substantial amount of capital. Retrieving a full list of a population or smaller subset lists from a third-party data provider may require payment each time data is provided.
If the sample is not large enough to represent the views of the entire population during the first round of simple random sampling, purchasing additional lists or databases to avoid a sampling error can be prohibitive.
Sample Selection Bias
Although simple random sampling is intended to be an unbiased approach to surveying, sample selection bias can occur. When a sample set of the larger population is not inclusive enough, representation of the full population is skewed and requires additional sampling techniques.
Data Quality Is Reliant on Researcher Qualify
The success of any sampling method relies on the researcher's willingness to thoroughly do their job. Someone who isn't willing to follow the rules or deviates from the task at hand won't help get a reliable result. For instance, there may be issues if a researcher doesn't ask the appropriate questions or asks the wrong ones. This could create implicit bias, ending up in a skewed study.
The term simple random sampling refers to a smaller section of a larger population. There is an equal chance that each member of this section will be chosen. For this reason, a simple random sampling is meant to be unbiased in its representation of the larger group. There is normally room for error with this method, which is indicated by a plus or minus variant. This is known as a sampling error.
How Is Simple Random Sampling Conducted?
Simple random sampling involves the study of a larger population by taking a smaller subset. This subgroup is chosen at random and studied to get the desired result. In order for this sampling method to work, the researcher must know the size of the larger population. The selection of the subset must be unbiased.
What Are the 4 Types of Random Sampling?
There are four types of random sampling. Simple random sampling involves an unbiased study of a smaller subset of a larger population. Stratified random sampling uses smaller groups derived from a larger population that is based on shared characteristics and attributes. Systematic sampling is a method that involves specific members of a larger dataset. These samples are selected based on a random starting point using a fixed, periodic interval. The final type of random sampling is cluster sampling, which takes members of a dataset and places them into clusters based on shared characteristics. Researchers then randomly select clusters to study.
When Is It Best to Use Simple Random Sampling?
It's always a good idea to use simple random sampling when you have smaller data sets to study. This allows you to produce better results that are more representative of the overall population. Keep in mind that this method requires each member of the larger population is identified and selected individually, which can often be challenging and time consuming.
Studying large populations can be very difficult. Getting information from each individual member can be costly and time-consuming. That's why researchers turn to random sampling to help reach the conclusions they need to make key decisions, whether that means helping provide the services that residents need, making better business decisions, or executing changes in an investor's portfolio.
Simple random sampling is relatively easy to conduct as long as you remove any and all hints of bias. Doing so means you must have information about each member of the larger population at your disposal before you conduct your research. This can be relatively simple and require very little knowledge. But keep in mind that the process can be costly and it may be hard trying to get access to information about all of the members of the population.
Pressbooks. " Significant Statistics: 1.5 Sampling Techniques and Ethics ."
:max_bytes(150000):strip_icc():format(webp)/thinkstockphotos-186299182-5bfc351cc9e77c00519c7317.jpg)
- Terms of Service
- Editorial Policy
- Privacy Policy
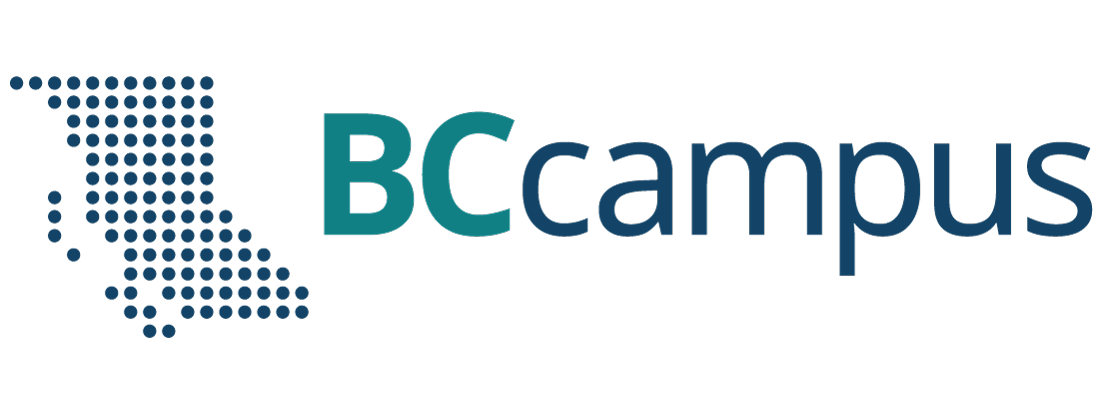
Want to create or adapt books like this? Learn more about how Pressbooks supports open publishing practices.
Chapter 6: Experimental Research
Experimental Design
Learning Objectives
- Explain the difference between between-subjects and within-subjects experiments, list some of the pros and cons of each approach, and decide which approach to use to answer a particular research question.
- Define random assignment, distinguish it from random sampling, explain its purpose in experimental research, and use some simple strategies to implement it.
- Define what a control condition is, explain its purpose in research on treatment effectiveness, and describe some alternative types of control conditions.
- Define several types of carryover effect, give examples of each, and explain how counterbalancing helps to deal with them.
In this section, we look at some different ways to design an experiment. The primary distinction we will make is between approaches in which each participant experiences one level of the independent variable and approaches in which each participant experiences all levels of the independent variable. The former are called between-subjects experiments and the latter are called within-subjects experiments.
Between-Subjects Experiments
In a between-subjects experiment , each participant is tested in only one condition. For example, a researcher with a sample of 100 university students might assign half of them to write about a traumatic event and the other half write about a neutral event. Or a researcher with a sample of 60 people with severe agoraphobia (fear of open spaces) might assign 20 of them to receive each of three different treatments for that disorder. It is essential in a between-subjects experiment that the researcher assign participants to conditions so that the different groups are, on average, highly similar to each other. Those in a trauma condition and a neutral condition, for example, should include a similar proportion of men and women, and they should have similar average intelligence quotients (IQs), similar average levels of motivation, similar average numbers of health problems, and so on. This matching is a matter of controlling these extraneous participant variables across conditions so that they do not become confounding variables.
Random Assignment
The primary way that researchers accomplish this kind of control of extraneous variables across conditions is called random assignment , which means using a random process to decide which participants are tested in which conditions. Do not confuse random assignment with random sampling. Random sampling is a method for selecting a sample from a population, and it is rarely used in psychological research. Random assignment is a method for assigning participants in a sample to the different conditions, and it is an important element of all experimental research in psychology and other fields too.
In its strictest sense, random assignment should meet two criteria. One is that each participant has an equal chance of being assigned to each condition (e.g., a 50% chance of being assigned to each of two conditions). The second is that each participant is assigned to a condition independently of other participants. Thus one way to assign participants to two conditions would be to flip a coin for each one. If the coin lands heads, the participant is assigned to Condition A, and if it lands tails, the participant is assigned to Condition B. For three conditions, one could use a computer to generate a random integer from 1 to 3 for each participant. If the integer is 1, the participant is assigned to Condition A; if it is 2, the participant is assigned to Condition B; and if it is 3, the participant is assigned to Condition C. In practice, a full sequence of conditions—one for each participant expected to be in the experiment—is usually created ahead of time, and each new participant is assigned to the next condition in the sequence as he or she is tested. When the procedure is computerized, the computer program often handles the random assignment.
One problem with coin flipping and other strict procedures for random assignment is that they are likely to result in unequal sample sizes in the different conditions. Unequal sample sizes are generally not a serious problem, and you should never throw away data you have already collected to achieve equal sample sizes. However, for a fixed number of participants, it is statistically most efficient to divide them into equal-sized groups. It is standard practice, therefore, to use a kind of modified random assignment that keeps the number of participants in each group as similar as possible. One approach is block randomization . In block randomization, all the conditions occur once in the sequence before any of them is repeated. Then they all occur again before any of them is repeated again. Within each of these “blocks,” the conditions occur in a random order. Again, the sequence of conditions is usually generated before any participants are tested, and each new participant is assigned to the next condition in the sequence. Table 6.2 shows such a sequence for assigning nine participants to three conditions. The Research Randomizer website will generate block randomization sequences for any number of participants and conditions. Again, when the procedure is computerized, the computer program often handles the block randomization.
Participant | Condition |
---|---|
1 | A |
2 | C |
3 | B |
4 | B |
5 | C |
6 | A |
7 | C |
8 | B |
9 | A |
Random assignment is not guaranteed to control all extraneous variables across conditions. It is always possible that just by chance, the participants in one condition might turn out to be substantially older, less tired, more motivated, or less depressed on average than the participants in another condition. However, there are some reasons that this possibility is not a major concern. One is that random assignment works better than one might expect, especially for large samples. Another is that the inferential statistics that researchers use to decide whether a difference between groups reflects a difference in the population takes the “fallibility” of random assignment into account. Yet another reason is that even if random assignment does result in a confounding variable and therefore produces misleading results, this confound is likely to be detected when the experiment is replicated. The upshot is that random assignment to conditions—although not infallible in terms of controlling extraneous variables—is always considered a strength of a research design.
Treatment and Control Conditions
Between-subjects experiments are often used to determine whether a treatment works. In psychological research, a treatment is any intervention meant to change people’s behaviour for the better. This intervention includes psychotherapies and medical treatments for psychological disorders but also interventions designed to improve learning, promote conservation, reduce prejudice, and so on. To determine whether a treatment works, participants are randomly assigned to either a treatment condition , in which they receive the treatment, or a control condition , in which they do not receive the treatment. If participants in the treatment condition end up better off than participants in the control condition—for example, they are less depressed, learn faster, conserve more, express less prejudice—then the researcher can conclude that the treatment works. In research on the effectiveness of psychotherapies and medical treatments, this type of experiment is often called a randomized clinical trial .
There are different types of control conditions. In a no-treatment control condition , participants receive no treatment whatsoever. One problem with this approach, however, is the existence of placebo effects. A placebo is a simulated treatment that lacks any active ingredient or element that should make it effective, and a placebo effect is a positive effect of such a treatment. Many folk remedies that seem to work—such as eating chicken soup for a cold or placing soap under the bedsheets to stop nighttime leg cramps—are probably nothing more than placebos. Although placebo effects are not well understood, they are probably driven primarily by people’s expectations that they will improve. Having the expectation to improve can result in reduced stress, anxiety, and depression, which can alter perceptions and even improve immune system functioning (Price, Finniss, & Benedetti, 2008) [1] .
Placebo effects are interesting in their own right (see Note “The Powerful Placebo” ), but they also pose a serious problem for researchers who want to determine whether a treatment works. Figure 6.2 shows some hypothetical results in which participants in a treatment condition improved more on average than participants in a no-treatment control condition. If these conditions (the two leftmost bars in Figure 6.2 ) were the only conditions in this experiment, however, one could not conclude that the treatment worked. It could be instead that participants in the treatment group improved more because they expected to improve, while those in the no-treatment control condition did not.
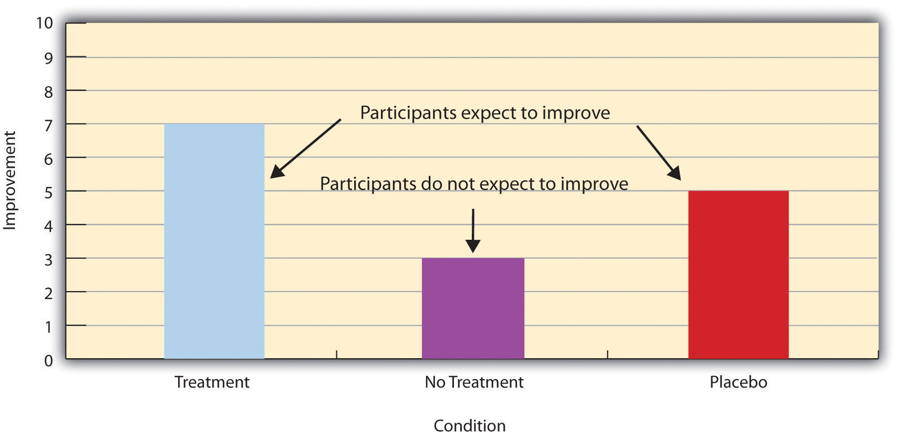
Fortunately, there are several solutions to this problem. One is to include a placebo control condition , in which participants receive a placebo that looks much like the treatment but lacks the active ingredient or element thought to be responsible for the treatment’s effectiveness. When participants in a treatment condition take a pill, for example, then those in a placebo control condition would take an identical-looking pill that lacks the active ingredient in the treatment (a “sugar pill”). In research on psychotherapy effectiveness, the placebo might involve going to a psychotherapist and talking in an unstructured way about one’s problems. The idea is that if participants in both the treatment and the placebo control groups expect to improve, then any improvement in the treatment group over and above that in the placebo control group must have been caused by the treatment and not by participants’ expectations. This difference is what is shown by a comparison of the two outer bars in Figure 6.2 .
Of course, the principle of informed consent requires that participants be told that they will be assigned to either a treatment or a placebo control condition—even though they cannot be told which until the experiment ends. In many cases the participants who had been in the control condition are then offered an opportunity to have the real treatment. An alternative approach is to use a waitlist control condition , in which participants are told that they will receive the treatment but must wait until the participants in the treatment condition have already received it. This disclosure allows researchers to compare participants who have received the treatment with participants who are not currently receiving it but who still expect to improve (eventually). A final solution to the problem of placebo effects is to leave out the control condition completely and compare any new treatment with the best available alternative treatment. For example, a new treatment for simple phobia could be compared with standard exposure therapy. Because participants in both conditions receive a treatment, their expectations about improvement should be similar. This approach also makes sense because once there is an effective treatment, the interesting question about a new treatment is not simply “Does it work?” but “Does it work better than what is already available?
The Powerful Placebo
Many people are not surprised that placebos can have a positive effect on disorders that seem fundamentally psychological, including depression, anxiety, and insomnia. However, placebos can also have a positive effect on disorders that most people think of as fundamentally physiological. These include asthma, ulcers, and warts (Shapiro & Shapiro, 1999) [2] . There is even evidence that placebo surgery—also called “sham surgery”—can be as effective as actual surgery.
Medical researcher J. Bruce Moseley and his colleagues conducted a study on the effectiveness of two arthroscopic surgery procedures for osteoarthritis of the knee (Moseley et al., 2002) [3] . The control participants in this study were prepped for surgery, received a tranquilizer, and even received three small incisions in their knees. But they did not receive the actual arthroscopic surgical procedure. The surprising result was that all participants improved in terms of both knee pain and function, and the sham surgery group improved just as much as the treatment groups. According to the researchers, “This study provides strong evidence that arthroscopic lavage with or without débridement [the surgical procedures used] is not better than and appears to be equivalent to a placebo procedure in improving knee pain and self-reported function” (p. 85).
Within-Subjects Experiments
In a within-subjects experiment , each participant is tested under all conditions. Consider an experiment on the effect of a defendant’s physical attractiveness on judgments of his guilt. Again, in a between-subjects experiment, one group of participants would be shown an attractive defendant and asked to judge his guilt, and another group of participants would be shown an unattractive defendant and asked to judge his guilt. In a within-subjects experiment, however, the same group of participants would judge the guilt of both an attractive and an unattractive defendant.
The primary advantage of this approach is that it provides maximum control of extraneous participant variables. Participants in all conditions have the same mean IQ, same socioeconomic status, same number of siblings, and so on—because they are the very same people. Within-subjects experiments also make it possible to use statistical procedures that remove the effect of these extraneous participant variables on the dependent variable and therefore make the data less “noisy” and the effect of the independent variable easier to detect. We will look more closely at this idea later in the book. However, not all experiments can use a within-subjects design nor would it be desirable to.
Carryover Effects and Counterbalancing
The primary disad vantage of within-subjects designs is that they can result in carryover effects. A carryover effect is an effect of being tested in one condition on participants’ behaviour in later conditions. One type of carryover effect is a practice effect , where participants perform a task better in later conditions because they have had a chance to practice it. Another type is a fatigue effect , where participants perform a task worse in later conditions because they become tired or bored. Being tested in one condition can also change how participants perceive stimuli or interpret their task in later conditions. This type of effect is called a context effect . For example, an average-looking defendant might be judged more harshly when participants have just judged an attractive defendant than when they have just judged an unattractive defendant. Within-subjects experiments also make it easier for participants to guess the hypothesis. For example, a participant who is asked to judge the guilt of an attractive defendant and then is asked to judge the guilt of an unattractive defendant is likely to guess that the hypothesis is that defendant attractiveness affects judgments of guilt. This knowledge could lead the participant to judge the unattractive defendant more harshly because he thinks this is what he is expected to do. Or it could make participants judge the two defendants similarly in an effort to be “fair.”
Carryover effects can be interesting in their own right. (Does the attractiveness of one person depend on the attractiveness of other people that we have seen recently?) But when they are not the focus of the research, carryover effects can be problematic. Imagine, for example, that participants judge the guilt of an attractive defendant and then judge the guilt of an unattractive defendant. If they judge the unattractive defendant more harshly, this might be because of his unattractiveness. But it could be instead that they judge him more harshly because they are becoming bored or tired. In other words, the order of the conditions is a confounding variable. The attractive condition is always the first condition and the unattractive condition the second. Thus any difference between the conditions in terms of the dependent variable could be caused by the order of the conditions and not the independent variable itself.
There is a solution to the problem of order effects, however, that can be used in many situations. It is counterbalancing , which means testing different participants in different orders. For example, some participants would be tested in the attractive defendant condition followed by the unattractive defendant condition, and others would be tested in the unattractive condition followed by the attractive condition. With three conditions, there would be six different orders (ABC, ACB, BAC, BCA, CAB, and CBA), so some participants would be tested in each of the six orders. With counterbalancing, participants are assigned to orders randomly, using the techniques we have already discussed. Thus random assignment plays an important role in within-subjects designs just as in between-subjects designs. Here, instead of randomly assigning to conditions, they are randomly assigned to different orders of conditions. In fact, it can safely be said that if a study does not involve random assignment in one form or another, it is not an experiment.
An efficient way of counterbalancing is through a Latin square design which randomizes through having equal rows and columns. For example, if you have four treatments, you must have four versions. Like a Sudoku puzzle, no treatment can repeat in a row or column. For four versions of four treatments, the Latin square design would look like:
A | B | C | D |
B | C | D | A |
C | D | A | B |
D | A | B | C |
There are two ways to think about what counterbalancing accomplishes. One is that it controls the order of conditions so that it is no longer a confounding variable. Instead of the attractive condition always being first and the unattractive condition always being second, the attractive condition comes first for some participants and second for others. Likewise, the unattractive condition comes first for some participants and second for others. Thus any overall difference in the dependent variable between the two conditions cannot have been caused by the order of conditions. A second way to think about what counterbalancing accomplishes is that if there are carryover effects, it makes it possible to detect them. One can analyze the data separately for each order to see whether it had an effect.
When 9 is “larger” than 221
Researcher Michael Birnbaum has argued that the lack of context provided by between-subjects designs is often a bigger problem than the context effects created by within-subjects designs. To demonstrate this problem, he asked participants to rate two numbers on how large they were on a scale of 1-to-10 where 1 was “very very small” and 10 was “very very large”. One group of participants were asked to rate the number 9 and another group was asked to rate the number 221 (Birnbaum, 1999) [4] . Participants in this between-subjects design gave the number 9 a mean rating of 5.13 and the number 221 a mean rating of 3.10. In other words, they rated 9 as larger than 221! According to Birnbaum, this difference is because participants spontaneously compared 9 with other one-digit numbers (in which case it is relatively large) and compared 221 with other three-digit numbers (in which case it is relatively small) .
Simultaneous Within-Subjects Designs
So far, we have discussed an approach to within-subjects designs in which participants are tested in one condition at a time. There is another approach, however, that is often used when participants make multiple responses in each condition. Imagine, for example, that participants judge the guilt of 10 attractive defendants and 10 unattractive defendants. Instead of having people make judgments about all 10 defendants of one type followed by all 10 defendants of the other type, the researcher could present all 20 defendants in a sequence that mixed the two types. The researcher could then compute each participant’s mean rating for each type of defendant. Or imagine an experiment designed to see whether people with social anxiety disorder remember negative adjectives (e.g., “stupid,” “incompetent”) better than positive ones (e.g., “happy,” “productive”). The researcher could have participants study a single list that includes both kinds of words and then have them try to recall as many words as possible. The researcher could then count the number of each type of word that was recalled. There are many ways to determine the order in which the stimuli are presented, but one common way is to generate a different random order for each participant.
Between-Subjects or Within-Subjects?
Almost every experiment can be conducted using either a between-subjects design or a within-subjects design. This possibility means that researchers must choose between the two approaches based on their relative merits for the particular situation.
Between-subjects experiments have the advantage of being conceptually simpler and requiring less testing time per participant. They also avoid carryover effects without the need for counterbalancing. Within-subjects experiments have the advantage of controlling extraneous participant variables, which generally reduces noise in the data and makes it easier to detect a relationship between the independent and dependent variables.
A good rule of thumb, then, is that if it is possible to conduct a within-subjects experiment (with proper counterbalancing) in the time that is available per participant—and you have no serious concerns about carryover effects—this design is probably the best option. If a within-subjects design would be difficult or impossible to carry out, then you should consider a between-subjects design instead. For example, if you were testing participants in a doctor’s waiting room or shoppers in line at a grocery store, you might not have enough time to test each participant in all conditions and therefore would opt for a between-subjects design. Or imagine you were trying to reduce people’s level of prejudice by having them interact with someone of another race. A within-subjects design with counterbalancing would require testing some participants in the treatment condition first and then in a control condition. But if the treatment works and reduces people’s level of prejudice, then they would no longer be suitable for testing in the control condition. This difficulty is true for many designs that involve a treatment meant to produce long-term change in participants’ behaviour (e.g., studies testing the effectiveness of psychotherapy). Clearly, a between-subjects design would be necessary here.
Remember also that using one type of design does not preclude using the other type in a different study. There is no reason that a researcher could not use both a between-subjects design and a within-subjects design to answer the same research question. In fact, professional researchers often take exactly this type of mixed methods approach.
Key Takeaways
- Experiments can be conducted using either between-subjects or within-subjects designs. Deciding which to use in a particular situation requires careful consideration of the pros and cons of each approach.
- Random assignment to conditions in between-subjects experiments or to orders of conditions in within-subjects experiments is a fundamental element of experimental research. Its purpose is to control extraneous variables so that they do not become confounding variables.
- Experimental research on the effectiveness of a treatment requires both a treatment condition and a control condition, which can be a no-treatment control condition, a placebo control condition, or a waitlist control condition. Experimental treatments can also be compared with the best available alternative.
- You want to test the relative effectiveness of two training programs for running a marathon.
- Using photographs of people as stimuli, you want to see if smiling people are perceived as more intelligent than people who are not smiling.
- In a field experiment, you want to see if the way a panhandler is dressed (neatly vs. sloppily) affects whether or not passersby give him any money.
- You want to see if concrete nouns (e.g., dog ) are recalled better than abstract nouns (e.g., truth ).
- Discussion: Imagine that an experiment shows that participants who receive psychodynamic therapy for a dog phobia improve more than participants in a no-treatment control group. Explain a fundamental problem with this research design and at least two ways that it might be corrected.
- Price, D. D., Finniss, D. G., & Benedetti, F. (2008). A comprehensive review of the placebo effect: Recent advances and current thought. Annual Review of Psychology, 59 , 565–590. ↵
- Shapiro, A. K., & Shapiro, E. (1999). The powerful placebo: From ancient priest to modern physician . Baltimore, MD: Johns Hopkins University Press. ↵
- Moseley, J. B., O’Malley, K., Petersen, N. J., Menke, T. J., Brody, B. A., Kuykendall, D. H., … Wray, N. P. (2002). A controlled trial of arthroscopic surgery for osteoarthritis of the knee. The New England Journal of Medicine, 347 , 81–88. ↵
- Birnbaum, M.H. (1999). How to show that 9>221: Collect judgments in a between-subjects design. Psychological Methods, 4(3), 243-249. ↵
An experiment in which each participant is only tested in one condition.
A method of controlling extraneous variables across conditions by using a random process to decide which participants will be tested in the different conditions.
All the conditions of an experiment occur once in the sequence before any of them is repeated.
Any intervention meant to change people’s behaviour for the better.
A condition in a study where participants receive treatment.
A condition in a study that the other condition is compared to. This group does not receive the treatment or intervention that the other conditions do.
A type of experiment to research the effectiveness of psychotherapies and medical treatments.
A type of control condition in which participants receive no treatment.
A simulated treatment that lacks any active ingredient or element that should make it effective.
A positive effect of a treatment that lacks any active ingredient or element to make it effective.
Participants receive a placebo that looks like the treatment but lacks the active ingredient or element thought to be responsible for the treatment’s effectiveness.
Participants are told that they will receive the treatment but must wait until the participants in the treatment condition have already received it.
Each participant is tested under all conditions.
An effect of being tested in one condition on participants’ behaviour in later conditions.
Participants perform a task better in later conditions because they have had a chance to practice it.
Participants perform a task worse in later conditions because they become tired or bored.
Being tested in one condition can also change how participants perceive stimuli or interpret their task in later conditions.
Testing different participants in different orders.
Research Methods in Psychology - 2nd Canadian Edition Copyright © 2015 by Paul C. Price, Rajiv Jhangiani, & I-Chant A. Chiang is licensed under a Creative Commons Attribution-NonCommercial-ShareAlike 4.0 International License , except where otherwise noted.
Share This Book
Have a language expert improve your writing
Run a free plagiarism check in 10 minutes, automatically generate references for free.
- Knowledge Base
- Methodology
- Random Assignment in Experiments | Introduction & Examples
Random Assignment in Experiments | Introduction & Examples
Published on 6 May 2022 by Pritha Bhandari . Revised on 13 February 2023.
In experimental research, random assignment is a way of placing participants from your sample into different treatment groups using randomisation.
With simple random assignment, every member of the sample has a known or equal chance of being placed in a control group or an experimental group. Studies that use simple random assignment are also called completely randomised designs .
Random assignment is a key part of experimental design . It helps you ensure that all groups are comparable at the start of a study: any differences between them are due to random factors.
Table of contents
Why does random assignment matter, random sampling vs random assignment, how do you use random assignment, when is random assignment not used, frequently asked questions about random assignment.
Random assignment is an important part of control in experimental research, because it helps strengthen the internal validity of an experiment.
In experiments, researchers manipulate an independent variable to assess its effect on a dependent variable, while controlling for other variables. To do so, they often use different levels of an independent variable for different groups of participants.
This is called a between-groups or independent measures design.
You use three groups of participants that are each given a different level of the independent variable:
- A control group that’s given a placebo (no dosage)
- An experimental group that’s given a low dosage
- A second experimental group that’s given a high dosage
Random assignment to helps you make sure that the treatment groups don’t differ in systematic or biased ways at the start of the experiment.
If you don’t use random assignment, you may not be able to rule out alternative explanations for your results.
- Participants recruited from pubs are placed in the control group
- Participants recruited from local community centres are placed in the low-dosage experimental group
- Participants recruited from gyms are placed in the high-dosage group
With this type of assignment, it’s hard to tell whether the participant characteristics are the same across all groups at the start of the study. Gym users may tend to engage in more healthy behaviours than people who frequent pubs or community centres, and this would introduce a healthy user bias in your study.
Although random assignment helps even out baseline differences between groups, it doesn’t always make them completely equivalent. There may still be extraneous variables that differ between groups, and there will always be some group differences that arise from chance.
Most of the time, the random variation between groups is low, and, therefore, it’s acceptable for further analysis. This is especially true when you have a large sample. In general, you should always use random assignment in experiments when it is ethically possible and makes sense for your study topic.
Prevent plagiarism, run a free check.
Random sampling and random assignment are both important concepts in research, but it’s important to understand the difference between them.
Random sampling (also called probability sampling or random selection) is a way of selecting members of a population to be included in your study. In contrast, random assignment is a way of sorting the sample participants into control and experimental groups.
While random sampling is used in many types of studies, random assignment is only used in between-subjects experimental designs.
Some studies use both random sampling and random assignment, while others use only one or the other.
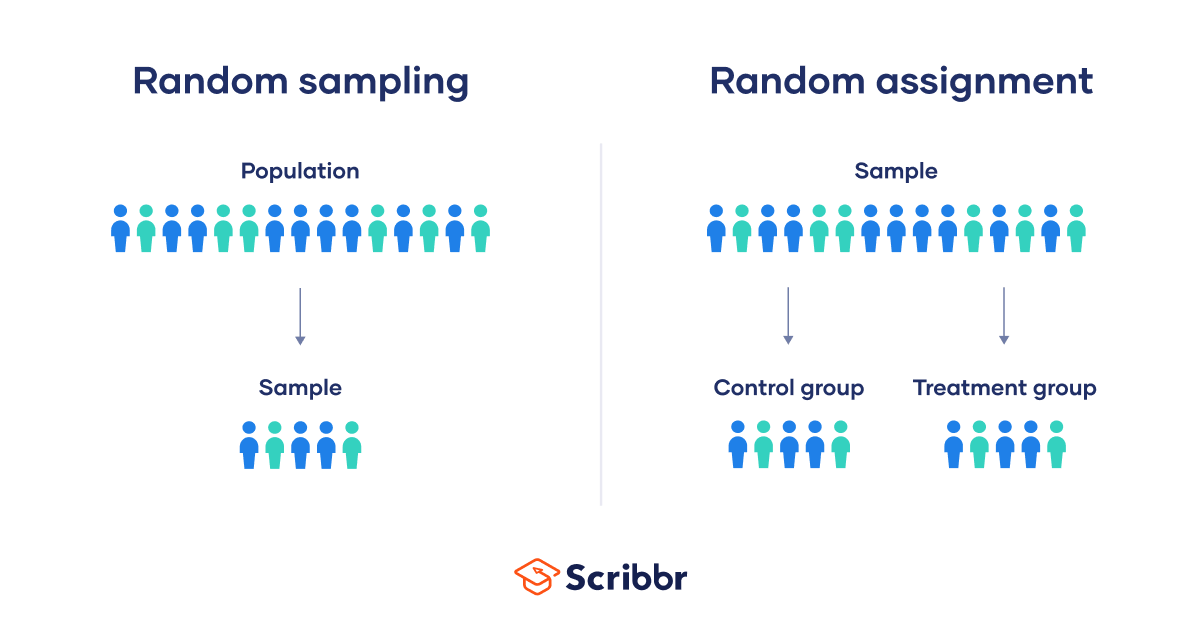
Random sampling enhances the external validity or generalisability of your results, because it helps to ensure that your sample is unbiased and representative of the whole population. This allows you to make stronger statistical inferences .
You use a simple random sample to collect data. Because you have access to the whole population (all employees), you can assign all 8,000 employees a number and use a random number generator to select 300 employees. These 300 employees are your full sample.
Random assignment enhances the internal validity of the study, because it ensures that there are no systematic differences between the participants in each group. This helps you conclude that the outcomes can be attributed to the independent variable .
- A control group that receives no intervention
- An experimental group that has a remote team-building intervention every week for a month
You use random assignment to place participants into the control or experimental group. To do so, you take your list of participants and assign each participant a number. Again, you use a random number generator to place each participant in one of the two groups.
To use simple random assignment, you start by giving every member of the sample a unique number. Then, you can use computer programs or manual methods to randomly assign each participant to a group.
- Random number generator: Use a computer program to generate random numbers from the list for each group.
- Lottery method: Place all numbers individually into a hat or a bucket, and draw numbers at random for each group.
- Flip a coin: When you only have two groups, for each number on the list, flip a coin to decide if they’ll be in the control or the experimental group.
- Use a dice: When you have three groups, for each number on the list, roll a die to decide which of the groups they will be in. For example, assume that rolling 1 or 2 lands them in a control group; 3 or 4 in an experimental group; and 5 or 6 in a second control or experimental group.
This type of random assignment is the most powerful method of placing participants in conditions, because each individual has an equal chance of being placed in any one of your treatment groups.
Random assignment in block designs
In more complicated experimental designs, random assignment is only used after participants are grouped into blocks based on some characteristic (e.g., test score or demographic variable). These groupings mean that you need a larger sample to achieve high statistical power .
For example, a randomised block design involves placing participants into blocks based on a shared characteristic (e.g., college students vs graduates), and then using random assignment within each block to assign participants to every treatment condition. This helps you assess whether the characteristic affects the outcomes of your treatment.
In an experimental matched design , you use blocking and then match up individual participants from each block based on specific characteristics. Within each matched pair or group, you randomly assign each participant to one of the conditions in the experiment and compare their outcomes.
Sometimes, it’s not relevant or ethical to use simple random assignment, so groups are assigned in a different way.
When comparing different groups
Sometimes, differences between participants are the main focus of a study, for example, when comparing children and adults or people with and without health conditions. Participants are not randomly assigned to different groups, but instead assigned based on their characteristics.
In this type of study, the characteristic of interest (e.g., gender) is an independent variable, and the groups differ based on the different levels (e.g., men, women). All participants are tested the same way, and then their group-level outcomes are compared.
When it’s not ethically permissible
When studying unhealthy or dangerous behaviours, it’s not possible to use random assignment. For example, if you’re studying heavy drinkers and social drinkers, it’s unethical to randomly assign participants to one of the two groups and ask them to drink large amounts of alcohol for your experiment.
When you can’t assign participants to groups, you can also conduct a quasi-experimental study . In a quasi-experiment, you study the outcomes of pre-existing groups who receive treatments that you may not have any control over (e.g., heavy drinkers and social drinkers).
These groups aren’t randomly assigned, but may be considered comparable when some other variables (e.g., age or socioeconomic status) are controlled for.
In experimental research, random assignment is a way of placing participants from your sample into different groups using randomisation. With this method, every member of the sample has a known or equal chance of being placed in a control group or an experimental group.
Random selection, or random sampling , is a way of selecting members of a population for your study’s sample.
In contrast, random assignment is a way of sorting the sample into control and experimental groups.
Random sampling enhances the external validity or generalisability of your results, while random assignment improves the internal validity of your study.
Random assignment is used in experiments with a between-groups or independent measures design. In this research design, there’s usually a control group and one or more experimental groups. Random assignment helps ensure that the groups are comparable.
In general, you should always use random assignment in this type of experimental design when it is ethically possible and makes sense for your study topic.
To implement random assignment , assign a unique number to every member of your study’s sample .
Then, you can use a random number generator or a lottery method to randomly assign each number to a control or experimental group. You can also do so manually, by flipping a coin or rolling a die to randomly assign participants to groups.
Cite this Scribbr article
If you want to cite this source, you can copy and paste the citation or click the ‘Cite this Scribbr article’ button to automatically add the citation to our free Reference Generator.
Bhandari, P. (2023, February 13). Random Assignment in Experiments | Introduction & Examples. Scribbr. Retrieved 3 September 2024, from https://www.scribbr.co.uk/research-methods/random-assignment-experiments/
Is this article helpful?
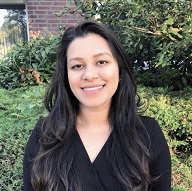
Pritha Bhandari
Other students also liked, a quick guide to experimental design | 5 steps & examples, controlled experiments | methods & examples of control, control groups and treatment groups | uses & examples.

An official website of the United States government
The .gov means it’s official. Federal government websites often end in .gov or .mil. Before sharing sensitive information, make sure you’re on a federal government site.
The site is secure. The https:// ensures that you are connecting to the official website and that any information you provide is encrypted and transmitted securely.
- Publications
- Account settings
Preview improvements coming to the PMC website in October 2024. Learn More or Try it out now .
- Advanced Search
- Journal List
- HHS Author Manuscripts

Preference in Random Assignment: Implications for the Interpretation of Randomized Trials
Cathaleene macias.
Community Intervention Research, McLean Hospital, Belmont, MA 02478, USA, ude.dravrah.naelcm@saicamc
Paul B. Gold
Department of Counseling and Personnel Services, University of Maryland, College Park, MD 20742, USA, ude.dmu@dlogp
William A. Hargreaves
Department of Psychiatry, University of California, San Francisco, CA, USA, ten.tsacmoc@grahllib
Elliot Aronson
Department of Psychology, University of California, Santa Cruz, CA, USA, ude.cscu.STAC@toille
Leonard Bickman
Center for Evaluation and Program Improvement, Vanderbilt University, Nashville, TN, USA, [email protected]
Paul J. Barreira
Harvard University Health Services, Harvard University, Boston, MA, USA, ude.dravrah.shu@arierrabp
Danson R. Jones
Institutional Research, Wharton County Junior College, Wharton, TX 77488, USA, ude.cjcw@dsenoj
Charles F. Rodican
Community Intervention Research, McLean Hospital, Belmont, MA 02478, USA, ude.dravrah.naelcm@nacidorc
William H. Fisher
Department of Psychiatry, University of Massachusetts Medical School, Worcester, MA, USA, [email protected]
Random assignment to a preferred experimental condition can increase service engagement and enhance outcomes, while assignment to a less-preferred condition can discourage service receipt and limit outcome attainment. We examined randomized trials for one prominent psychiatric rehabilitation intervention, supported employment, to gauge how often assignment preference might have complicated the interpretation of findings. Condition descriptions, and greater early attrition from services-as-usual comparison conditions, suggest that many study enrollees favored assignment to new rapid-job-placement supported employment, but no study took this possibility into account. Reviews of trials in other service fields are needed to determine whether this design problem is widespread.
The validity of research in any field depends on the extent to which studies rule out alternative explanations for findings and provide meaningful explanations of how and why predicted outcomes were attained (e.g., Bickman 1987 ; Lewin 1943 ; Shadish et al. 2002 ; Trist and Sofer 1959 ). In mental health services research, participants’ expectations about the pros and cons of being randomly assigned to each experimental intervention can offer post hoc explanations for study findings that rival the explanations derived from study hypotheses. Unlike most drug studies that can ‘blind’ participants to their condition assignment, studies that evaluate behavioral or psychosocial interventions typically tell each participant his or her experimental assignment soon after randomization, and being assigned to a non-preferred intervention could be disappointing, or even demoralizing ( Shapiro et al. 2002 ), and thereby reduce participants’ interest in services or motivation to pursue service goals ( Cook and Campbell 1979 ; Shadish 2002 ). On the other hand, if most participants randomly assigned to one experimental condition believe they are fortunate, this condition may have an unfair advantage in outcome comparisons.
Reasons for preferring assignment to a particular experimental condition can be idiosyncratic and diverse, but as long as each condition is assigned the same percentage of participants who are pleased or displeased with their condition assignment, then there will be no overall pattern of condition preferences that could explain differences in outcomes. The greater threat to a valid interpretation of findings occurs when most study enrollees share a general preference for random assignment to one particular condition. Greater preference for one experimental condition over another could stem from general impressions of relative service model effectiveness, or from information that is tangential, e.g., program location on a main bus route or in a safer area of town. Even if random assignment distributes service preferences in equal proportions across conditions, the less attractive experimental condition will receive a higher percentage of participants who are mismatched to their preference, and the more attractive condition will receive a higher percentage of participants matched to their preference. For example, if 60% of all study enrollees prefer condition A and 40% prefer condition B, then, with true equivalence across conditions, service A would have 60% pleased and 40% disappointed assignees, while service B would have 40% pleased and 60% disappointed assignees.
There is potential to engender a general preference for assignment to a particular experimental intervention whenever a study’s recruitment posters, information sheets, or consent documents depict one intervention as newer or seemingly better, even if no evidence yet supports a difference in intervention effectiveness. For instance, in a supported housing study, if a comparison condition is described as offering familiar ‘services-as-usual’ help with moving into supervised housing, participants might reasonably prefer assignment to a more innovative experimental intervention designed to help individuals find their own independent apartments.
Methodologists have proposed protocol adaptations to the typical randomized trial to measure and monitor the impact of participants’ intervention preferences on study enrollment and engagement in assigned experimental conditions ( Braver and Smith 1996 ; Corrigan and Salzer 2003 ; Lambert and Wood 2000 ; Marcus 1997 ; Staines et al. 1999 ; TenHave et al. 2003 ). Nevertheless, few mental health service studies have adopted these design modifications, and even fewer have followed recommendations to measure, report, and, if necessary, statistically control for enrollees’ expressed preferences for assignment to a particular condition ( Halpern 2002 ; King et al. 2005 ; Shapiro et al. 2002 ; Torgerson et al. 1996 ).
In this article, we begin by describing several ways that participants’ preferences for random assignment to a specific service intervention can complicate the interpretation of findings. We then review one field of services research to estimate the prevalence of some of these problems. Obstacles to a valid interpretation of findings include the likelihood of (a) lower service engagement and/or greater early attrition from less-preferred conditions, and (b) similarities among people who refuse or leave a non-preferred program and, hence, condition differences in types of retained participants. Even if all randomized participants do receive assigned services, those who preferred assignment to a certain condition may be unique in ways (e.g., functioning, motivation) that predict outcomes over and above the impact of services, and (c) certain program designs may ameliorate or intensify the effects of disappointment in service assignment. Finally, (d) preference for assignment to one condition over another may reflect a clash between program characteristics (e.g., attendance requirements) and participants’ situational considerations (e.g., time constraints, residential location) so that participants assigned to a non-preferred condition may tend to encounter similar difficulties in attaining outcomes and may choose the same alternative activities. We now discuss each of these issues.
How Participants’ Service Preferences Can Influence Outcomes
Impact of assignment preference on service engagement and retention.
Research participants who are disappointed in their random assignment to a non-preferred experimental condition may refuse to participate, or else withdraw from assigned services or treatment early in the study ( Hofmann et al. 1998 ; Kearney and Silverman 1998 ; Laengle et al. 2000 ; Macias et al. 2005 ; Shadish et al. 2000 ; Wahlbeck et al. 2001 ). If this occurs more often for one experimental condition than another, such differential early attrition can quickly transform a randomized controlled trial into a quasi-experiment ( Corrigan and Salzer 2003 ; Essock et al. 2003 ; West and Sagarin 2000 ). Unless participants’ preferences for assignment to experimental interventions are measured prior to randomization, it will be impossible to distinguish the emotional impact on participants of being matched or mismatched to intervention preference from each intervention’s true ability to engage and retain its assigned participants. If participants who reject their service assignments tend to refuse research interviews, the least-preferred intervention may also have a disproportionately higher incidence of ‘false negatives’ (undetected positive outcomes), and this can further bias the interpretation of findings.
Researchers can statistically control for intervention preferences if these attitudes are measured prior to randomization and one intervention is not greatly preferred over another. Even if a study is unable to measure and statistically control participants’ pre-existing preferences for assignment to experimental conditions, statistically adjusting for differential attrition from assigned services can help to rule out disappointment or satisfaction with random assignment as an alternative explanation for findings. However, rather than statistically controlling (erasing) the impact of intervention preferences on service retention and outcomes, it may be far more informative to investigate whether preference in random assignment might have modified a program’s potential to engage and motivate participants ( Sosin 2002 ). For instance, a statistically significant ‘program assignment-by-program preference’ interaction term in a regression analysis ( Aguinis 2004 ; Aiken and West 1991 ) might reveal a demoralization effect (e.g., a combination of less effort, lower service satisfaction, poorer outcomes) for participants randomly assigned to a comparison condition that was not their preference. A more complex program-by-preference interaction analysis might reveal that an assertive program is better at engaging and retaining consumers who are disappointed in their service assignment, while a less assertive program, when it is able to hang onto its disappointed assignees, is better at helping them attain service goals ( Delucchi and Bostrom 2004 ; Lachenbruch 2002 ). Ability to engage and retain participants is a prerequisite for effectiveness, but, in the same way that medication compliance is distinguished from medication efficacy in pharmaceutical trials, service retention should not be confused with the impact of services received ( Little and Rubin 2000 ).
Similarities Between People with the Same Preferences
Even if rates of early attrition are comparable across study conditions, experimental groups may differ in the types of people who decide to accept assigned services ( Magidson 2000 ). If participants who reject a service intervention resemble one another in some way, then the intervention samples of service-active participants will likely differ on these same dimensions.
As yet, we know very little about the effectiveness of different types of community interventions for engaging various types of consumers ( Cook 1999a , b ; Mark et al. 1992 ), but mobile services and programs that provide assertive community outreach appear to have stronger engagement and retention, presumably because staff schedule and initiate most service contacts on a routine basis ( McGrew et al. 2003 ). If these program characteristics match participants’ reasons for preferring one experimental condition over another, then a bias can exist whether or not intervention preference is balanced across conditions. For instance, consumers who are physically disabled, old, or agoraphobic may prefer home-based service delivery and are likely to be disappointed if assigned to a program that requires regular attendance. Greater retention of these more disabled individuals could put a mobile intervention at a disadvantage in a direct evaluation of service outcomes, like employment, that favor able-bodied, younger, or less anxious individuals. On the other hand, in rehabilitation fields like supported housing, education, or employment that depend strongly on consumer initiative and self-determination, higher functioning or better educated consumers may drop out of control conditions because they are not offered needed opportunities soon enough ( Shadish 2002 ). This was evident in a recent study of supported housing ( McHugo et al. 2004 ), which reported a higher proportion of ‘shelter or street’ homeless participants in the control condition relative to a focal supported housing condition, presumably because participants who were more familiar with local services (e.g., those temporarily homeless following eviction or hospital discharge) considered the control condition services inadequate and sought housing on their own outside the research project.
Service model descriptions and intervention theories suggest many interactions between program characteristics and participant preferences that could be tested as research hypotheses if proposed prior to data analysis. Unfortunately, such hypotheses are rarely formulated and tested.
It is also rare for a randomized trial to compare experimental interventions on sample characteristics at a point in time later than baseline, after every participant has had an opportunity to accept or reject his or her experimental assignment, so that sample differences that emerge early in the project can be statistically controlled in outcome analyses.
Interaction Between Responses to Service Assignment and Service Characteristics
A more subtle threat to research validity exists whenever participants disappointed in their intervention assignment do not drop out of services, but instead remain half-heartedly engaged ( Corrigan and Salzer 2003 ). Participants randomized to a preferred intervention are likely to be pleased and enthusiastic, ready to engage with service providers, while those randomized to a non-preferred intervention are more likely to be disappointed and less motivated to succeed. However, the strength of participant satisfaction or disappointment in service assignment can vary greatly depending on service program characteristics ( Brown et al. 2002 ; Calsyn et al. 2000 ; Grilo et al. 1998 ; Macias et al. 2005 ; Meyer et al. 2002 ). For instance, in a randomized comparison of assertive community treatment (PACT) to a certified clubhouse ( Macias et al. 2009 ), we found that being randomly assigned to the less preferred program decreased service engagement more often in the clubhouse condition than in PACT. However, clubhouse members who had not wanted this service assignment, but nevertheless used clubhouse services to find a job, ended up employed longer and were more satisfied with services than other study enrollees. Study hypotheses based on program differences in staff assertiveness (PACT) and consumer self-determination (clubhouse) predicted this rare three-way interaction prior to data collection, and offer a theory-based (dissonance theory; Aronson 1999 ; Festinger 1957 ) explanation of the complex finding. Presumably, clubhouse members not wanting assignment to this service needed to rationalize their voluntary participation in a non-preferred program by viewing the clubhouse as a means-to-an-end. They tried harder than usual to get a job and stay employed, and gave the clubhouse some credit for their personal success. By contrast, PACT participants who had not wanted this service assignment could credit assertive program staff for keeping them involved, so they experienced less cognitive dissonance and had less need to justify their continued receipt of a non-preferred service. Whether being assigned to a non-preferred program turns out to have negative or positive consequences can depend on a complex interplay between participant motivation and program characteristics. The generation of useful hypotheses for any mental health service trial depends on thoughtful reflection on experimental program differences, as well as familiarity with research in disciplines that study human motivation, such as psychiatry, social psychology, and advertising ( Krause and Howard 2003 ).
Alternative Outcomes Related to Service Preferences
If participants who prefer a certain service condition share similar characteristics, they may also share similar life circumstances and make similar life choices. Individuals who have the same personal responsibilities or physical limitations may prefer not to be assigned to a particular intervention because they cannot fully comply with the requirements for participation, even if they try to do so. For instance, some research participants may have difficulty with regular program attendance because they have competing time commitments, such as caring for an infant or seriously ill relative, or attending school to pursue work credentials ( Collins et al. 2000 ; Mowbray et al. 1999 ; Wolf et al. 2001 ). These productive alternative activities could also compete with the research study’s targeted outcomes, and be misinterpreted as outcome ‘failures.’ For instance, in supported employment trials, unemployment should not be considered a negative outcome if the individual is attending college or pursuing job-related training, or if she has chosen to opt out of the job market for a while to take care of small children or an ill or handicapped relative. These alternative pursuits will be coded simply as ‘unemployed,’ and interpreted as program failure, unless they are tracked and reported as explanations for why work was not obtained. For this reason, it is important to examine relationships between participant circumstances and service preferences at the outset of a study to identify what additional life events and occupations might need to be documented to fully explain intervention outcome differences.
Scope of the Assignment Preference Problem
Regardless of the reason for research participant preference in random assignment, condition differences in service attractiveness can be statistically controlled if (a) preference is measured prior to randomization and (b) if there is sufficient variability in preferences so that the vast majority of study enrollees do not prefer the same intervention. Unfortunately, most randomized service trials have neither measured pre-randomization service preference nor taken it into account when comparing intervention outcomes. Therefore, it is important to assess whether undetected participant preference in random assignment might have existed in published randomized trials, and, if so, whether it might have compromised the interpretation of findings.
As a case example, we review the empirical support for one evidence-based practice, supported employment for adults with severe mental illness, to obtain a qualitative estimate of the extent to which unmeasured service preference for a focal intervention might offer an alternative explanation for published findings. Supported employment offers an ideal starting point for our inquiry given its extensive body of research, which includes a $20 million multi-site randomized study (EIDP, Cook et al. 2002 ), and consensus among psychiatric rehabilitation stakeholders that supported employment is an evidence-based practice ready for dissemination and implementation (Bond et al. 2001). Consumer receptivity and participation in supported employment has been studied in depth through ethnographies ( Alverson et al. 1998 ; Alverson et al. 1995 ; Quimby et al. 2001 ), structured interviews ( McQuilken et al. 2003 ; Secker et al. 2002 ), and personal essays ( Honey 2000 ), and these publications suggest that most consumers know what they need and should expect from a quality vocational program. For this reason, consumer service preferences should be a salient consideration in the design of supported employment research.
Sample of Randomized Trials of Supported Employment
The evidence base for supported employment effectiveness consists of a series of randomized controlled studies of the Individual Placement and Support (IPS) service model (Bond et al. 1997, 2001). One reason research on supported employment has been restricted to a single service delivery model is the ready availability of standardized IPS training and fidelity measures ( Bond et al. 2002 ; McGrew and Griss 2005 ). As a result of a substantial body of research evidence that IPS produces good employment outcomes, this service model has become synonymous with ‘supported employment’ in much of the psychiatric rehabilitation literature (Bond et al. 1997; Crowther et al. 2001 ; Drake et al. 2003 ), and many state departments of mental health in the United States now endorse a definition of supported employment as Individual Placement and Support (IPS).
Table 1 presents a recently published list of all randomized controlled trials of supported employment programs recognized as having high fidelity to Individual Placement and Support (IPS) by the designers of this service delivery model (Bond et al. 2008). Every study has the IPS model as its focal intervention, and IPS experts provided staff training and verified the fidelity of each focal intervention using a supported employment (IPS) fidelity scale (Bond et al. 2008). Research study eligibility was generally limited to unemployed individuals with severe mental illness who had an expressed interest in finding a mainstream job. Most study samples had a mean age of about 40, except that the Twamley et al. (2008) sample was older ( M = 50 years) and the Killackey et al. (2008) sample was younger ( M = 21 years). Except for the study by Lehman et al. (2002) , all studies discouraged enrollment of participants who had major physical limitations or substance use problems.
Randomized trials of high fidelity IPS supported employment: indicators of possible participant preference in condition assignment
RCT study/ location | Comparison condition(s) | Comparison condition description | Voc service retention | Research study retention |
---|---|---|---|---|
New Hampshire, USA | Job skills training | Boston ‘choose-get-keep’ model / ‘pre-employment skills training in a group format’ | √2 months | 18 months |
E: 100% | E: 99% | |||
C: 62% | C: 97% | |||
Washington, DC USA | Sheltered workshop | ‘several well-established agencies’/‘primarily paid work adjustment training in a sheltered workshop’ | 2 months | 18 months |
E: 95% | 99% total sample | |||
C: 84% | ||||
Maryland, USA | Psychosocial rehabilitation program | ‘in-house skill training, sheltered work, factory enclaves’ ‘socialization, education, housing’ | √ any voc service | 24 months |
E: 93% | E: 74% | |||
C: 33% | C: 60% | |||
Connecticut, USA | Multiple sites: | 1. ‘standard vocational services’ 2. typical ‘PSR center’ providing ‘social, recreational, educational, & vocational’ services,’ e.g., skills training, program-owned jobs. | √ a few weeks | 24 months |
E: 90% | E: 96% | |||
C: 50% | C: 98% | |||
South Carolina, USA | Sheltered workshop | ‘traditional vocational rehabilitation’ ‘staff-supervised set-aside jobs’ | 6 months | 24 months |
E: 86% | E: 82% | |||
C: 83% | C: 70% | |||
Quebec Canada | Traditional vocational services | “sheltered workshop, creative workshops, client-run boutique and horticulture;’ ‘job-finding skills training;’ government sponsored set-aside jobs | √ 6 months | 12 months |
E: 91% | E: 79% | |||
C: 30% | C: 89% | |||
Indiana, USA | ‘Diversified placement’ at Thresholds, Inc. | ‘existing Thresholds services’ ‘prevocational work crews,’ ‘groups,’ temporary set-aside work | √ 6 months | 24 months |
E: 82% | 97% total sample | |||
C: 65% | ||||
6 Nations Europe | Traditional, ‘typical and dominant’ voc rehab service | Daily ‘structured training combating deficits,’ ‘time structuring,’ and computer skills, usually provided in a ‘day centre’ | √ any voc service | 18 months |
E: 100% | E: 100% | |||
C: 76% | C: 100% | |||
Hong Kong, China | Stepwise conventional voc services | ‘Occupational Therapy Department of local hospital’ ‘work groups in a simulated environment’ | 18 months | 18 months: |
E: 100% | E: 100% | |||
C: 100% | C: 98% | |||
California, USA | Conventional voc rehab referrals | Dept of Rehab referral to ‘job readiness coaching’ and ‘prevocational classes’ | √ any voc service | 12 months |
E: 100% | E: 79% | |||
C: 41% | C: 77% | |||
Victoria, Australia | Traditional vocational services | ‘treatment-as-usual’ referral to voc agency with ‘vocationally oriented group programme’ | √ 6 months: | 6 months |
E: 95% | E: 100% | |||
C: 76% | C: 100% |
As reported in the IPS review article by Bond et al. (2008), or in these original study publications
Possible Indicators of Differential Service Preference
Table 1 lists verbatim service descriptions of the comparison condition in each of the eleven original study publications, along with condition labels derived from the Bond et al. (2008) review of these same randomized trials. Although we do not know the language used to describe the service interventions in recruitment flyers or induction meetings, we assumed there was a strong possibility that most study enrollees would prefer assignment to a new IPS program whenever the comparison condition was an existing sheltered workshop, traditional psychosocial rehabilitation program, or conventional vocational rehabilitation that had been routinely offered by the state or a local authority over several previous years. Since all study enrollees had an expressed interest in obtaining competitive work, we also assumed the possibility of greater preference for IPS if the comparison condition were designed primarily to provide non-competitive jobs, or if program activities delayed entry into competitive employment. Most studies (8 of 11) reported mandatory attendance of all study applicants at one or more research project induction groups in which the experimental conditions were described and questions answered ( Drake et al. 1994 ).
Next, we documented whether each study reported greater early service attrition, or a lower service engagement, for its comparison condition. We report the percentage of study enrollees who were ever active in assigned services at the earliest post-randomization point reported in the original publication or in the summary review article by Bond et al.(2008). We chose the earliest report period so that it would be reasonable to attribute low service contact to disappointment in service assignment. Early service attrition can also be attributed to service ineffectiveness (e.g., poor outreach, slow development of staff-client relationships, or lack of immediate efforts to help participants get a job), so we assume that lower engagement in comparison services is a probable, but not conclusive indication that a comparison condition was generally less appealing than IPS. Our assumption that disappointment in service assignment is a reasonable explanation for early service attrition is based on a demonstrated temporal relationship between random assignment to a non-preferred intervention and subsequently low rates of service engagement within two very different supported employment interventions that had comparable employment rates ( Macias et al. 2005 ).
We also provide research study retention rates for each condition at the latest measurement point as a check on the possibility that loss of participants from services was attributable to the same causes that prevented participation in research interviews and/or the tracking of study outcomes. If research study retention rates at a later point in time are as good or better than service intervention retention rates at an earlier time point, we will assume that factors that typically restrict or enhance research study participation (e.g., program differences in outcome tracking, deaths, hospitalizations, residential mobility) do not account for early differential attrition from experimental and control conditions.
We will consider a study to be at high risk for misinterpretation of findings if the condition labels or descriptions were less favorable for the comparison condition(s), and if there is greater early attrition from comparison services in spite of high research retention.
Review Findings
Descriptions of comparison conditions.
The comparison condition for every study listed in Table 1 was a pre-existing conventional or traditional vocational rehabilitation service that would have been familiar to many participants and did not prioritize rapid placement into mainstream employment. By contrast, each IPS program was a new intervention introduced to the local service system through the research project that was designed to offer fast entry into mainstream work. Although no study recorded participants’ service assignment preference prior to research enrollment or randomization, we might reasonably assume that, in some studies, satisfaction with service assignment to IPS, or disappointment in assignment to the non-supported employment comparison condition, contributed to differences in mainstream employment rates between experimental conditions.
Differential Early Attrition/Retention
Six of the eleven studies reported a 20% or greater advantage in service retention for the focal IPS intervention within the first 8 weeks following randomization. Two other studies that assessed service retention at the 6-months point in the research project reported 17 and 19% differences in favor of IPS. Only the South Carolina and Hong Kong studies ( Gold et al. 2006 ; Wong et al. 2008 ) reported comparably high rates of service retention across experimental interventions, possibly because both studies required all participants to be active in a local mental health program at the time of research study enrollment.
Overall, the majority of participants remained active in each research study for the duration of the trial, with comparable research retention across study conditions. This comparability suggests that factors known to increase research attrition (e.g., residential mobility, chronic illness) cannot explain early differential attrition from services.
IPS interventions may have had better service retention rates in eight of these eleven randomized trials because IPS had more assertive outreach, provided more useful services, or IPS staff collaborated more closely with clinicians than staff in the comparison conditions (Bond et al. 2008; Gold et al. 2006 ; McGurk et al. 2007 ). However, greater intensity or quality of IPS services cannot reasonably account for the very low service retention rates for most comparison conditions relative to research project retention, so disappointment in assignment remains a credible additional explanation for greater early attrition from comparison services.
Only the South Carolina study statistically controlled for variation in participant exposure to vocational services, which might be considered a proxy for the effects of differential attrition attributable to service preference. No study reported whether early attrition resulted in the loss of different types of people from each study condition, and every study compared study conditions on participant characteristics only at baseline.
Our review of research in one dominant field of adult psychiatric rehabilitation reveals that every randomized controlled trial of high-fidelity supported employment had a ‘services-as-usual’ comparison condition that might have predisposed work-interested participants to prefer random assignment to the new ‘rapid-job-placement’ IPS intervention. We cannot be certain that IPS was preferred by most participants over comparison conditions in any of these studies because no study measured participants’ pre-randomization service preferences or satisfaction with condition assignment. However, neither does any original publication offer evidence that would refute our assumption of greater preference for IPS. Eight of these 11 studies reported 15% or greater service attrition from the comparison condition early in the project that could reflect disappointment in service assignment, but no study reporting early differential attrition statistically controlled for exposure to services, examined how attrition might have changed sample characteristics, or distinguished between service retention and outcome attainment in data analyses.
We cannot conclude that the outcomes for any of these eleven studies would differ from the reported findings if service preference, service receipt, or the effects of early attrition on sample characteristics had been measured and, assuming sufficient variability in these measures, intervention differences had been statistically controlled. Moreover, design factors other than program descriptions provided in study advertisements, research induction sessions, or consent documents might have engendered a general preference for assignment to IPS. For instance, in the Bond et al. (2007) study, IPS services were located at the same health center that provided most participants’ clinical care, while comparison services were off-site, and so condition differences in service convenience could also explain better retention rates and outcomes for IPS. Regardless, the published labels and descriptions of comparison interventions presented in Table 1 , and early condition differences in service retention rates, suggest the possibility that outcome differences between study conditions that consistently favor IPS might be partially explained by corresponding differences in participant expectations about services, and, ultimately, satisfaction or disappointment in service assignment. If these same research designs problems are prevalent in other fields of mental health services research, we need to consider what widespread impact these alternative explanations may have had on the interpretation of research findings.
Variability in Impact of Participant Preferences on Outcomes
Unmeasured participant preference in random assignment may not pose the same threat in other service trials, even if informed consent procedures are similar to those used in these supported employment trials, and even if service descriptions inadvertently induce a general preference for one intervention over another. The direct impact of service preference on outcomes may depend a great deal on whether the primary study outcome is measured subjectively or objectively, and on the type of intervention under evaluation, including its frequency, intensity, or duration ( Torgerson and Moffett 2005 ). Moreover, if study outcomes do not depend on participant attitudes or motivation, then disappointment in service assignment may have no impact on outcomes at all.
A mismatch to service preference is likely to have the strongest impact on study outcomes whenever participants are expected to improve their own lives in observable ways that demand strong commitment and self-determination, as is the case for supported employment. By contrast, the impact of a mismatch to service preference on outcomes is probably least discernable when participation is passive or condition assignment remains unknown, as is the case in most drug and medical treatment trials ( King et al. 2005 ; Leykin et al. 2007 ). Whether disappointment in service assignment reduces or enhances outcomes may also depend on prevailing attitudes toward cooperation with service professionals ( Nichols and Maner 2008 ) or perceived pressure to participate from program staff ( Macias et al. 2009 ). However, the impact of service preference on outcomes should almost always be strong when the reason for preferring an intervention is based on expectations of relative efficacy, since even medication trials have shown better outcomes when participants believe a drug will be efficacious ( Krell et al. 2004 ), as well as worse outcomes when they suspect a drug is a placebo ( Sneed et al. 2008 ).
Research reviews are needed to estimate the potential impact of unmeasured service preference in other service fields, and to identify moderating variables that deserve further study. Until the relative threat of participant service preference can be determined for a specific field, pre-randomization service preference should be measured routinely in every randomized controlled trial and, if there is sufficient variability in preference measurement, condition differences in preference should be statistically controlled, and tests of interaction effects conducted to identify moderating variables. Examples of statistical control for service preference in logistic regression and event history analysis can be found in reports on a supported employment randomized trial that compared two SE interventions ( Macias et al. 2005 ; Macias et al. 2006 ). A third publication from this same trial illustrates a theory-driven test of moderation effects ( Macias et al. 2009 ). However, whenever one experimental condition is greatly preferred over another, there is no statistical remedy that will allow an unbiased comparison of outcomes.
New Directions for Employment Research
The body of research on supported employment (SE) offers compelling evidence that most adults with severe mental illness do not find prevocational training or standard vocational rehabilitation attractive routes to mainstream employment ( Cook 1999a , b ; Noble et al. 1997 ). It may be time to relinquish ‘SE vs. no SE’ research designs that evoke preference for assignment to SE and move on to compare different ways of delivering the same high quality SE job-hunting services and on-the-job supports ( Bickman 2002 ; Lavori 2000 ). Comparisons of alternative modalities of the same service typically provide less striking, statistically weaker contrasts in outcomes, but they preserve the ethical principle of equipoise and help to ensure that all participants receive adequate care and comparable opportunities for life improvement ( Lavori et al. 2001 ; Lilford and Jackson 1995 ; Schwartz and Sprangers 1999 ).
We would learn more about why supported employment is effective, and what aspects of SE are most attractive to prospective research participants, if studies would provide more detailed descriptions of service implementation so that the same key concepts (e.g., rapid job placement, service integration, frequent contact) could be compared across separate studies and in meta-analyses ( Campbell and Fiske 1959 ; Sechrest et al. 2000 ; TenHave et al. 2003 ). Such studies would also help to justify specificity in fidelity measurement during dissemination and implementation of evidence-based practices ( Rosenheck 2001 ; West et al. 2002 ). It would be especially advantageous to compare ways to increase access to mainstream work in specific service environments, since the heterogeneity in IPS employment rates, internationally and across the USA, suggests that social, political, economic, and organizational factors are far greater predictors of the work attainment of disabled individuals than receipt of employment services, or even disability itself.
Conclusions
The randomized controlled trial is still the gold standard of research designs ( Cook 1999a , b ), and randomization greatly strengthens causal inference ( Abelson 1995 ; Beveridge 1950 ). However, cause-effect inference depends on the measurement of all plausibly potent causal factors, including study participants’ attitudes toward their assigned interventions. Ironically, while consumer advocates champion the individual’s right to choose services, researchers rarely examine the contribution of consumer self-direction to outcomes considered indicative of service effectiveness. It may well be a legitimate responsibility of institutional review boards to assess the potential impact of study designs and research enrollment documents on participants’ preferences in random assignment and, hence, their eventual well-being as research participants and role in determining study outcomes ( Adair et al. 1983 ).
Our review of one prominent field of mental health services research suggests a general need to reexamine published randomized controlled trials to gauge the extent to which research protocols or descriptions of experimental conditions might have predisposed participants to prefer assignment to one particular condition over another, and whether participant responses to these research design elements might have moderated, or even mediated, service effectiveness.
Acknowledgments
Work on this article was funded by National Institute of Mental Health grants to the first and second authors (MH62628; MH01903). We are indebted to Ann Hohmann, Ph.D. for her supportive monitoring of the NIMH research grant that fostered this interdisciplinary collaboration, and to anonymous reviewers who offered invaluable insights during manuscript preparation.
Contributor Information
Cathaleene Macias, Community Intervention Research, McLean Hospital, Belmont, MA 02478, USA, ude.dravrah.naelcm@saicamc .
Paul B. Gold, Department of Counseling and Personnel Services, University of Maryland, College Park, MD 20742, USA, ude.dmu@dlogp .
William A. Hargreaves, Department of Psychiatry, University of California, San Francisco, CA, USA, ten.tsacmoc@grahllib .
Elliot Aronson, Department of Psychology, University of California, Santa Cruz, CA, USA, ude.cscu.STAC@toille .
Leonard Bickman, Center for Evaluation and Program Improvement, Vanderbilt University, Nashville, TN, USA, [email protected] .
Paul J. Barreira, Harvard University Health Services, Harvard University, Boston, MA, USA, ude.dravrah.shu@arierrabp .
Danson R. Jones, Institutional Research, Wharton County Junior College, Wharton, TX 77488, USA, ude.cjcw@dsenoj .
Charles F. Rodican, Community Intervention Research, McLean Hospital, Belmont, MA 02478, USA, ude.dravrah.naelcm@nacidorc .
William H. Fisher, Department of Psychiatry, University of Massachusetts Medical School, Worcester, MA, USA, [email protected] .
- Abelson RP. Statistics as principled argument. Hillsdale, NJ: Lawrence Erlbaum; 1995. [ Google Scholar ]
- Adair JG, Lindsay RCL, Carlopio J. Social artifact research and ethical regulation: Their impact on the teaching of experimental methods. Teaching of Psychology. 1983; 10 :159–162. doi: 10.1207/s15328023top1003_10. [ PubMed ] [ CrossRef ] [ Google Scholar ]
- Aguinis H. Regression analysis for categorical moderators. New York: Guilford Press; 2004. [ Google Scholar ]
- Aiken LS, West SG. Multiple regression: Testing and interpreting interactions. Newbury Park, CA: Sage; 1991. [ Google Scholar ]
- Alverson H, Alverson M, Drake RE, Becker DR. Social correlates of competitive employment among people with severe mental illness. Psychosocial Rehabilitation Journal. 1998; 22 (1):34–40. [ Google Scholar ]
- Alverson M, Becker DR, Drake RE. An ethnographic study of coping strategies used by people with severe mental illness participating in supported employment. Psychosocial Rehabilitation Journal. 1995; 18 (4):115–127. [ Google Scholar ]
- Aronson E. The power of self-persuasion. The American Psychologist. 1999; 54 (11):873–875. doi: 10.1037/h0088188. [ CrossRef ] [ Google Scholar ]
- Beveridge WIB. The art of scientific investigation. New York: Vintage Books; 1950. [ Google Scholar ]
- Bickman L. The functions of program theory. In: Bickman L, editor. Using program theory in evaluation. San Francisco: Jossey-Bass; 1987. [ Google Scholar ]
- Bickman L. The death of treatment as usual: An excellent first step on a long road. Clinical Psychology: Science and Practice. 2002; 9 (2):195–199. doi: 10.1093/clipsy/9.2.195. [ CrossRef ] [ Google Scholar ]
- Bond GR, Becker DR, Drake RE, Rapp C, Meisler N, Lehman AF. Implementing supported employment as an evidence-based practice. Psychiatric Services. 2001a; 52 (3):313–322. doi: 10.1176/appi.ps.52.3.313. [ PubMed ] [ CrossRef ] [ Google Scholar ]
- Bond GR, Becker DR, Drake RE, Vogler K. A fidelity scale for the individual placement and support model of supported employment. Rehabilitation Counseling Bulletin. 1997a; 40 (4):265–284. [ Google Scholar ]
- Bond GR, Campbell K, Evans LJ, Gervey R, Pascaris A, Tice S, et al. A scale to measure quality of supported employment for persons with severe mental illness. Journal of Vocational Rehabilitation. 2002; 17 (4):239–250. [ Google Scholar ]
- Bond GR, Drake R, Becker D. An update on randomized controlled trials of evidence-based supported employment. Psychiatric Rehabilitation Journal. 2008a; 31 (4):280–290. doi: 10.2975/31.4.2008.280.290. [ PubMed ] [ CrossRef ] [ Google Scholar ]
- Bond GR, Drake RE, Mueser KT, Becker DR. An update on supported employment for people with severe mental illness. Psychiatric Services. 1997b; 48 (3):335–346. [ PubMed ] [ Google Scholar ]
- Bond GR, McHugo GH, Becker D, Rapp CA, Whitley R. Fidelity of supported employment: Lessons learned from the national evidence-based practice project. Psychiatric Rehabilitation Journal. 2008b; 31 (4):300–305. doi: 10.2975/31.4.2008.300.305. [ PubMed ] [ CrossRef ] [ Google Scholar ]
- Bond GR, Salyers MP, Roudebush RL, Dincin J, Drake RE, Becker DR, et al. A randomized controlled trial comparing two vocational models for persons with severe mental illness. Journal of Consulting and Clinical Psychology. 2007; 75 (6):968–982. doi: 10.1037/0022-006X.75.6.968. [ PubMed ] [ CrossRef ] [ Google Scholar ]
- Bond GR, Vogler K, Resnick SG, Evans L, Drake R, Becker D. Dimensions of supported employment: Factor structure of the IPS fidelity scale. Journal of Mental Health. 2001b; 10 (4):383–393. doi: 10.1080/09638230120041146. [ CrossRef ] [ Google Scholar ]
- Braver SL, Smith MC. Maximizing both external and internal validity in longitudinal true experiments with voluntary treatments: The ‘combined modified’ design. Evaluation and Program Planning. 1996; 19 :287–300. doi: 10.1016/S0149-7189(96)00029-8. [ CrossRef ] [ Google Scholar ]
- Brown TG, Seraganian P, Tremblay J, Annis H. Matching substance abuse aftercare treatments to client characteristics. Addictive Behaviors. 2002; 27 :585–604. doi: 10.1016/S0306-4603(01)00195-2. [ PubMed ] [ CrossRef ] [ Google Scholar ]
- Burns T, Catty J, Becker T, Drake RE, Fioritti A, Knapp M, et al. The effectiveness of supported employment for people with severe mental illness: A randomised controlled trial. Lancet. 2007; 370 :1146–1152. doi: 10.1016/S0140-6736(07)61516-5. [ PubMed ] [ CrossRef ] [ Google Scholar ]
- Calsyn R, Winter J, Morse G. Do consumers who have a choice of treatment have better outcomes? Community Mental Health Journal. 2000; 36 (2):149–160. doi: 10.1023/A:1001890210218. [ PubMed ] [ CrossRef ] [ Google Scholar ]
- Campbell DT, Fiske DW. Convergent and discriminant validation by the multitrait–multimethod matrix. Psychological Bulletin. 1959; 56 :81–105. doi: 10.1037/h0046016. [ PubMed ] [ CrossRef ] [ Google Scholar ]
- Collins ME, Mowbray C, Bybee D. Characteristics predicting successful outcomes of participants with severe mental illness in supported education. Psychiatric Services. 2000; 51 (6):774–780. doi: 10.1176/appi.ps.51.6.774. [ PubMed ] [ CrossRef ] [ Google Scholar ]
- Cook JA. Understanding the failure of vocational rehabilitation: What do we need to know and how can we learn it? Journal of Disability Policy Studies. 1999a; 10 (1):127–132. [ Google Scholar ]
- Cook TD. Considering the major arguments against random assignment: An analysis of the intellectual culture surrounding evaluation in American schools of education. Paper presented at the Harvard Faculty Seminar on Experiments in Education; Cambridge, MA. 1999b. [ Google Scholar ]
- Cook TD, Campbell DT. Quasi-experimentation: Design & analysis issues for field settings. Boston: Houghton Mifflin; 1979. [ Google Scholar ]
- Cook JA, Carey MA, Razzano L, Burke J, Blyler CR. The pioneer: The employment intervention demonstration program. New Directions for Evaluation. 2002; 94 :31–44. doi: 10.1002/ev.49. [ CrossRef ] [ Google Scholar ]
- Corrigan PW, Salzer MS. The conflict between random assignment and treatment preference: Implications for internal validity. Evaluation and Program Planning. 2003; 26 :109–121. doi: 10.1016/S0149-7189(03)00014-4. [ PubMed ] [ CrossRef ] [ Google Scholar ]
- Crowther RE, Marshall M, Bond GR, Huxley P. Helping people with severe mental illness to obtain work: Systematic review. BMJ: British Medical Journal. 2001; 322 (7280):204–208. doi: 10.1136/bmj.322.7280.204. [ PMC free article ] [ PubMed ] [ CrossRef ] [ Google Scholar ]
- Delucchi KL, Bostrom A. Methods for analysis of skewed data distributions in psychiatric clinical studies: Working with many zero values. The American Journal of Psychiatry. 2004; 161 (7):1159–1168. doi: 10.1176/appi.ajp.161.7.1159. [ PubMed ] [ CrossRef ] [ Google Scholar ]
- Drake RE, Becker DR, Anthony WA. A research induction group for clients entering a mental health services research project. Hospital & Community Psychiatry. 1994; 45 (5):487–489. [ PubMed ] [ Google Scholar ]
- Drake RE, Becker D, Bond GR. Recent research on vocational rehabilitation for persons with severe mental illness. Current Opinion in Psychiatry. 2003; 16 :451–455. doi: 10.1097/00001504-200307000-00012. [ CrossRef ] [ Google Scholar ]
- Drake RE, McHugo GJ, Bebout RR, Becher DR, Harris M, Bond GR, et al. A randomized clinical trial of supported employment for inner-city patients with severe mental disorders. Archives of General Psychiatry. 1999; 56 :627–633. doi: 10.1001/archpsyc.56.7.627. [ PubMed ] [ CrossRef ] [ Google Scholar ]
- Drake RE, McHugo GJ, Becker D, Anthony WA, Clark RE. The new Hampshire study of supported employment for people with severe mental illness. Journal of Consulting and Clinical Psychology. 1996; 64 (2):391–399. doi: 10.1037/0022-006X.64.2.391. [ PubMed ] [ CrossRef ] [ Google Scholar ]
- Essock SM, Drake R, Frank RG, McGuire TG. Randomized controlled trials in evidence-based mental health care: Getting the right answer to the right question. Schizophrenia Bulletin. 2003; 29 (1):115–123. [ PubMed ] [ Google Scholar ]
- Festinger L. A theory of cognitive dissonance. Stanford, CA: Stanford University Press; 1957. [ Google Scholar ]
- Gold PB, Meisler N, Santos AB, Carnemolla MA, Williams OH, Keleher J. Randomized trial of supported employment integrated with assertive community treatment for rural adults with severe mental illness. Schizophrenia Bulletin. 2006; 32 (2):378–395. doi: 10.1093/schbul/sbi056. [ PMC free article ] [ PubMed ] [ CrossRef ] [ Google Scholar ]
- Grilo CM, Money R, Barlow DH, Goddard AW, Gorman JM, Hofmann SG, et al. Pretreatment patient factors predicting attrition from a multicenter randomized controlled treatment study for panic disorder. Comprehensive Psychiatry. 1998; 39 (6):323–332. doi: 10.1016/S0010-440X(98)90043-8. [ PubMed ] [ CrossRef ] [ Google Scholar ]
- Halpern SD. Prospective preference assessment: a method to enhance the ethics and efficiency of randomized controlled trials. Controlled Clinical Trials. 2002; 23 :274–288. doi: 10.1016/S0197-2456(02)00191-5. [ PubMed ] [ CrossRef ] [ Google Scholar ]
- Hofmann SG, Barlow DH, Papp LA, Detweiler MF, Ray SE, Shear MK, et al. Pretreatment attrition in a comparative treatment outcome study on panic disorder. The American Journal of Psychiatry. 1998; 155 (1):43–47. [ PubMed ] [ Google Scholar ]
- Honey A. Psychiatric vocational rehabilitation: Where are the customers’ views. Psychiatric Rehabilitation Journal. 2000; 23 (3):270–279. [ PubMed ] [ Google Scholar ]
- Kearney C, Silverman W. A critical review of pharmacotherapy for youth with anxiety disorders: Things are not as they seem. Journal of Anxiety Disorders. 1998; 12 (2):83–102. doi: 10.1016/S0887-6185(98)00005-X. [ PubMed ] [ CrossRef ] [ Google Scholar ]
- Killackey E, Jackson HJ, McGorry PD. Vocational intervention in first-episode psychosis: Individual placement and support versus treatment as usual. The British Journal of Psychiatry. 2008; 193 :114–120. doi: 10.1192/bjp.bp.107.043109. [ PubMed ] [ CrossRef ] [ Google Scholar ]
- King M, Nazareth I, Lampe F, Bower P, Chandler M, Morou M, et al. Impact of participant and physician intervention preferences on randomized trials: A systematic review. Journal of the American Medical Association. 2005; 293 (9):1089–1099. doi: 10.1001/jama.293.9.1089. [ PubMed ] [ CrossRef ] [ Google Scholar ]
- Krause MS, Howard KI. What random assignment does and does not do. Journal of Clinical Psychology. 2003; 59 :751–766. doi: 10.1002/jclp.10170. [ PubMed ] [ CrossRef ] [ Google Scholar ]
- Krell HV, Leuchter AF, Morgan M, Cook IA, Abrams M. Subject expectations of treatment effectiveness and outcome of treatment with an experimental antidepressant. The Journal of Clinical Psychiatry. 2004; 65 (9):1174–1179. [ PubMed ] [ Google Scholar ]
- Lachenbruch PA. Analysis of data with excess zeros. Statistical Methods in Medical Research. 2002; 11 :297–302. doi: 10.1191/0962280202sm289ra. [ PubMed ] [ CrossRef ] [ Google Scholar ]
- Laengle G, Welte W, Roesger U, Guenthner A, U’Ren R. Chronic psychiatric patients without psychiatric care: A pilot study. Social Psychiatry and Psychiatric Epidemiology. 2000; 35 (10):457–462. doi: 10.1007/s001270050264. [ PubMed ] [ CrossRef ] [ Google Scholar ]
- Lambert MF, Wood J. Incorporating patient preferences into randomized trials. Journal of Clinical Epidemiology. 2000; 53 :163–166. doi: 10.1016/S0895-4356(99)00146-8. [ PubMed ] [ CrossRef ] [ Google Scholar ]
- Latimer EA, LeCompte MD, Becker DR, Drake RE, Duclos I, Piat M, et al. Generalizability of the individual placement and support model of supported employment: Results of a canadian randomised controlled trial. The British Journal of Psychiatry. 2006; 189 :65–73. doi: 10.1192/bjp.bp.105.012641. [ PubMed ] [ CrossRef ] [ Google Scholar ]
- Lavori PW. Placebo control groups in randomized treatment trials: A statistician’s perspective. Biological Psychiatry. 2000; 47 :717–723. doi: 10.1016/S0006-3223(00)00838-6. [ PubMed ] [ CrossRef ] [ Google Scholar ]
- Lavori PW, Rush AJ, Wisniewski SR, Alpert J, Fava M, Kupfer DJ, et al. Strengthening clinical effectiveness trials: Equipoise-stratified randomization. Biological Psychiatry. 2001; 50 (10):792–801. doi: 10.1016/S0006-3223(01)01223-9. [ PubMed ] [ CrossRef ] [ Google Scholar ]
- Lehman AF, Goldberg RW, Dixon LB, NcNary S, Postrado L, Hackman A, et al. Improving employment outcomes for persons with severe mental illnesses. Archives of General Psychiatry. 2002; 59 (2):165–172. doi: 10.1001/archpsyc.59.2.165. [ PubMed ] [ CrossRef ] [ Google Scholar ]
- Lewin K. Forces behind food habits and methods of change. Bulletin of the National Research Council. 1943; 108 :35–65. [ Google Scholar ]
- Leykin Y, DeRubeis RJ, Gallop R, Amsterdam JD, Shelton RC, Hollon SD. The relation of patients’ treatment preferences to outcome in a randomized clinical trial. Behavior Therapy. 2007; 38 :209–217. doi: 10.1016/j.beth.2006.08.002. [ PubMed ] [ CrossRef ] [ Google Scholar ]
- Lilford R, Jackson J. Equipoise and the ethics of randomisation. Journal of the Royal Society of Medicine. 1995; 88 :552–559. [ PMC free article ] [ PubMed ] [ Google Scholar ]
- Little RJ, Rubin DB. Causal effects in clinical and epidemiological studies via potential outcomes: Concepts and analytical approaches. Annual Review of Public Health. 2000; 21 :121–145. doi: 10.1146/annurev.publhealth.21.1.121. [ PubMed ] [ CrossRef ] [ Google Scholar ]
- Macias C, Aronson E, Hargreaves W, Weary G, Barreira P, Harvey JH, et al. Transforming dissatisfaction with services into self-determination: A social psychological perspective on community program effectiveness. Journal of Applied Social Psychology. 2009; 39 (7) [ PMC free article ] [ PubMed ] [ Google Scholar ]
- Macias C, Barreira P, Hargreaves W, Bickman L, Fisher WH, Aronson E. Impact of referral source and study applicants’ preference for randomly assigned service on research enrollment, service engagement, and evaluative outcomes. The American Journal of Psychiatry. 2005; 162 (4):781–787. doi: 10.1176/appi.ajp.162.4.781. [ PMC free article ] [ PubMed ] [ CrossRef ] [ Google Scholar ]
- Macias C, Rodican CF, Hargreaves WA, Jones DR, Barreira PJ, Wang Q. Supported employment outcomes of a randomized controlled trial of assertive community treatment and clubhouse models. Psychiatric Services. 2006; 57 (10):1406–1415. doi: 10.1176/appi.ps.57.10.1406. [ PMC free article ] [ PubMed ] [ CrossRef ] [ Google Scholar ]
- Magidson J. On models used to adjust for preexisting differences. In: Bickman L, editor. Research design. Vol. 2. Thousand Oaks, CA: Sage; 2000. [ Google Scholar ]
- Marcus SM. Assessing non-consent bias with parallel randomized and nonrandomized clinical trials. Journal of Clinical Epidemiology. 1997; 50 (7):823–828. doi: 10.1016/S0895-4356(97)00068-1. [ PubMed ] [ CrossRef ] [ Google Scholar ]
- Mark MM, Hofmann DA, Reichardt CS. Testing theories in theory-driven evaluations: Tests of moderation in all things. In: Chen H, Rossi PH, editors. Using theory to improve program and policy evaluations. New York: Greenwood Press; 1992. [ Google Scholar ]
- McGrew JH, Griss ME. Concurrent and predictive validity of two scales to assess the fidelity of implementation of supported employment. Psychiatric Rehabilitation Journal. 2005; 29 (1):41–47. doi: 10.2975/29.2005.41.47. [ PubMed ] [ CrossRef ] [ Google Scholar ]
- McGrew JH, Pescosolido BA, Wright E. Case managers’ perspectives on critical ingredients of Assertive Community Treatment and on its implementation. Psychiatric Services. 2003; 54 (3):370–376. doi: 10.1176/appi.ps.54.3.370. [ PubMed ] [ CrossRef ] [ Google Scholar ]
- McGurk S, Mueser K, Feldman K, Wolfe R, Pascaris A. Cognitive training for supported employment: 2–3 year outcomes of a randomized controlled trial. The American Journal of Psychiatry. 2007; 164 :437–441. doi: 10.1176/appi.ajp.164.3.437. [ PubMed ] [ CrossRef ] [ Google Scholar ]
- McHugo GJ, Bebout RR, Harris M, Cleghorn S, Herring G, Xie H, et al. A randomized controlled trial of integrated versus parallel housing services for homeless adults with severe mental illness. Schizophrenia Bulletin. 2004; 30 (4):969–982. [ PubMed ] [ Google Scholar ]
- McQuilken M, Zahniser JH, Novak J, Starks RD, Olmos A, Bond GR. The work project survey: Consumer perspectives on work. Journal of Vocational Rehabilitation. 2003; 18 :59–68. [ Google Scholar ]
- Meyer B, Pilkonis PA, Krupnick JL, Egan MK, Simmens SJ, Sotsky SM. Treatment expectancies, patient alliance and outcome: Further analyses from the national institute of mental health treatment of depression collaborative research program. Journal of Consulting and Clinical Psychology. 2002; 70 (4):1051–1055. doi: 10.1037/0022-006X.70.4.1051. [ PubMed ] [ CrossRef ] [ Google Scholar ]
- Mowbray CT, Collins M, Deborah B. Supported education for individuals with psychiatric disabilities: Long-term outcomes from an experimental study. Social Work Research. 1999; 23 (2):89–100. [ Google Scholar ]
- Mueser KT, Clark RE, Haines M, Drake RE, McHugo GJ, GR B, et al. The Hartford study of supported employment for persons with severe mental illness. Journal of Consulting and Clinical Psychology. 2004; 72 (3):479–490. doi: 10.1037/0022-006X.72.3.479. [ PubMed ] [ CrossRef ] [ Google Scholar ]
- Nichols AL, Maner JK. The good-subject effect: Investigating participant demand characteristics. The Journal of General Psychology. 2008; 135 (2):151–165. doi: 10.3200/GENP.135.2.151-166. [ PubMed ] [ CrossRef ] [ Google Scholar ]
- Noble JH, Honberg RS, Hall LL, Flynn LM. A legacy of failure: The inability of the federal-state vocational rehabilitation system to serve people with severe mental illness. Arlington: VA: National Alliance for the Mentally Ill; 1997. [ Google Scholar ]
- Quimby E, Drake R, Becker D. Ethnographic findings from the Washington, DC vocational services study. Psychiatric Rehabilitation Journal. 2001; 24 (4):368–374. [ PubMed ] [ Google Scholar ]
- Rosenheck RA. Organizational process: A missing link between research and practice. Psychiatric Services. 2001; 52 (12):1607–1612. doi: 10.1176/appi.ps.52.12.1607. [ PubMed ] [ CrossRef ] [ Google Scholar ]
- Schwartz CE, Sprangers M. Methodological approaches for assessing response shift in longitudinal quality of life research. Social Science & Medicine. 1999; 48 :1531–1548. doi: 10.1016/S0277-9536(99)00047-7. [ PubMed ] [ CrossRef ] [ Google Scholar ]
- Sechrest L, Davis M, Stickle T, McKnight P. Understanding ‘method’ variance. In: Bickman L, editor. Research design. Thousand Oaks, CA: Sage; 2000. [ Google Scholar ]
- Secker J, Membrey H, Grove B, Seebohm P. Recovering from illness or recovering your life? Implications of clinical versus social models of recovery from mental health problems for employment support services. Disability & Society. 2002; 17 (4):403–418. doi: 10.1080/09687590220140340. [ CrossRef ] [ Google Scholar ]
- Shadish WR. Revisiting field experimentation: Field notes for the future. Psychological Methods. 2002; 7 (1):3–18. doi: 10.1037/1082-989X.7.1.3. [ PubMed ] [ CrossRef ] [ Google Scholar ]
- Shadish WR, Cook TD, Campbell DT. Experimental and quasi-experimental designs for generalized causal inference. New York: Houghton Mifflin; 2002. [ Google Scholar ]
- Shadish WR, Matt GE, Navarro AM, Phillips G. The effects of psychological therapies under clinically representative conditions: A meta-analysis. Psychological Bulletin. 2000; 126 (4):512–529. doi: 10.1037/0033-2909.126.4.512. [ PubMed ] [ CrossRef ] [ Google Scholar ]
- Shapiro SL, Figueredo AJ, Caspi O, Schwartz GE, Bootzin RR, Lopez AM, et al. Going quasi: The premature disclosure effect in a randomized clinical trial. Journal of Behavioral Medicine. 2002; 25 (6):605–621. doi: 10.1023/A:1020693417427. [ PubMed ] [ CrossRef ] [ Google Scholar ]
- Sneed JR, Rutherford BR, Rindskopf D, Lane DT, Sackeim HA, Roose SP. Design makes a difference: A meta-analysis of antidepressant response rates in placebo-controlled versus comparator trials in late-life depression. The American Journal of Geriatric Psychiatry. 2008; 16 :65–73. doi: 10.1097/JGP.0b013e3181256b1d. [ PubMed ] [ CrossRef ] [ Google Scholar ]
- Sosin MR. Outcomes and sample selection: The case of a homelessness and substance abuse intervention. The British Journal of Mathematical and Statistical Psychology. 2002; 55 (1):63–92. doi: 10.1348/000711002159707. [ PubMed ] [ CrossRef ] [ Google Scholar ]
- Staines G, McKendrick K, Perlis T, Sacks S, DeLeon G. Sequential assignment and treatment as usual: Alternatives to standard experimental designs in field studies of treatment efficacy. Evaluation Review. 1999; 23 (1):47–76. doi: 10.1177/0193841X9902300103. [ PubMed ] [ CrossRef ] [ Google Scholar ]
- TenHave T, Coyne J, Salzer M, Katz I. Research to improve the quality of care for depression: Alternatives to the simple randomized clinical trial. General Hospital Psychiatry. 2003; 25 :115–123. doi: 10.1016/S0163-8343(02)00275-X. [ PubMed ] [ CrossRef ] [ Google Scholar ]
- Torgerson D, Klaber Moffett JA, Russell IT. Including patient preferences in randomized clinical trials. Journal of Health Services Research & Policy. 1996; 1 :194–197. [ PubMed ] [ Google Scholar ]
- Torgerson D, Moffett JK. Patient Preference and Validity of Randomized Controlled Trials: Letter to the Editor. Journal of the American Medical Association. 2005; 294 (1):41. doi: 10.1001/jama.294.1.41-b. [ PubMed ] [ CrossRef ] [ Google Scholar ]
- Trist E, Sofer C. Exploration in group relations. Leicester: Leicester University Press; 1959. [ Google Scholar ]
- Twamley EW, Narvaez JM, Becker DR, Bartels SJ, Jeste DV. Supported employment for middle-aged and older people with schizophrenia. American Journal of Psychiatric Rehabilitation. 2008; 11 (1):76–89. doi: 10.1080/15487760701853326. [ PMC free article ] [ PubMed ] [ CrossRef ] [ Google Scholar ]
- Wahlbeck K, Tuunainen A, Ahokas A, Leucht S. Dropout rates in randomised antipsychotic drug trials. Psychopharmacology. 2001; 155 (3):230–233. doi: 10.1007/s002130100711. [ PubMed ] [ CrossRef ] [ Google Scholar ]
- West SG, Aiken LS, Todd M. Probing the effects of individual components in multiple component prevention programs. In: Revenson & T, D’Agostino RB, editors. Ecological research to promote social change: Methodological advances from community psychology. New York, NY: Kluwer; 2002. [ PubMed ] [ Google Scholar ]
- West SG, Sagarin BJ. Participant selection and loss in randomized experiments. In: Bickman L, editor. Research design: Donald Campbell’s legacy. II. Thousand Oaks, CA: Sage; 2000. pp. 117–154. [ Google Scholar ]
- Wolf J, Coba C, Cirella M. Education as psychosocial rehabilitation: Supported education program partnerships with mental health and behavioral healthcare certificate programs. Psychiatric Rehabilitation Skills. 2001; 5 (3):455–476. [ Google Scholar ]
- Wong KK, Chiu R, Tang B, Mak D, Liu J, Chiu SN. A randomized controlled trial of a supported employment program for persons with long-term mental illness in Hong Kong. Psychiatric Services (Washington, DC) 2008; 59 (1):84–90. doi: 10.1176/appi.ps.59.1.84. [ PubMed ] [ CrossRef ] [ Google Scholar ]

An official website of the United States government
The .gov means it’s official. Federal government websites often end in .gov or .mil. Before sharing sensitive information, make sure you’re on a federal government site.
The site is secure. The https:// ensures that you are connecting to the official website and that any information you provide is encrypted and transmitted securely.
- Publications
- Account settings
- My Bibliography
- Collections
- Citation manager
Save citation to file
Email citation, add to collections.
- Create a new collection
- Add to an existing collection
Add to My Bibliography
Your saved search, create a file for external citation management software, your rss feed.
- Search in PubMed
- Search in NLM Catalog
- Add to Search
What random assignment does and does not do
Affiliation.
- 1 Northwestern University, USA. [email protected]
- PMID: 12808582
- DOI: 10.1002/jclp.10170
Random assignment of patients to comparison groups stochastically tends, with increasing sample size or number of experiment replications, to minimize the confounding of treatment outcome differences by the effects of differences among these groups in unknown/unmeasured patient characteristics. To what degree such confounding is actually avoided we cannot know unless we have validly measured these patient variables, but completely avoiding it is quite unlikely. Even if this confounding were completely avoided, confounding by unmeasured Patient Variable x Treatment Variable interactions remains a possibility. And the causal power of the confounding variables is no less important for internal validity than the degree of confounding.
Copyright 2003 Wiley Periodicals, Inc. J Clin Psychol.
PubMed Disclaimer
Similar articles
- Evidence from nonrandomized studies: a case study on the estimation of causal effects. Schmoor C, Caputo A, Schumacher M. Schmoor C, et al. Am J Epidemiol. 2008 May 1;167(9):1120-9. doi: 10.1093/aje/kwn010. Epub 2008 Mar 11. Am J Epidemiol. 2008. PMID: 18334500
- Two-stage instrumental variable methods for estimating the causal odds ratio: analysis of bias. Cai B, Small DS, Have TR. Cai B, et al. Stat Med. 2011 Jul 10;30(15):1809-24. doi: 10.1002/sim.4241. Epub 2011 Apr 15. Stat Med. 2011. PMID: 21495062
- The analysis of continuous outcomes in multi-centre trials with small centre sizes. Pickering RM, Weatherall M. Pickering RM, et al. Stat Med. 2007 Dec 30;26(30):5445-56. doi: 10.1002/sim.3068. Stat Med. 2007. PMID: 17924360
- Randomization procedures in orthopaedic trials. Randelli P, Arrigoni P, Lubowitz JH, Cabitza P, Denti M. Randelli P, et al. Arthroscopy. 2008 Jul;24(7):834-8. doi: 10.1016/j.arthro.2008.01.011. Epub 2008 Mar 21. Arthroscopy. 2008. PMID: 18589273 Review.
- Risk factors, confounding, and the illusion of statistical control. Christenfeld NJ, Sloan RP, Carroll D, Greenland S. Christenfeld NJ, et al. Psychosom Med. 2004 Nov-Dec;66(6):868-75. doi: 10.1097/01.psy.0000140008.70959.41. Psychosom Med. 2004. PMID: 15564351 Review.
- Patient Expectations of Assigned Treatments Impact Strength of Randomised Control Trials. Truzoli R, Reed P, Osborne LA. Truzoli R, et al. Front Med (Lausanne). 2021 Jun 17;8:648403. doi: 10.3389/fmed.2021.648403. eCollection 2021. Front Med (Lausanne). 2021. PMID: 34222273 Free PMC article.
- Preference in random assignment: implications for the interpretation of randomized trials. Macias C, Gold PB, Hargreaves WA, Aronson E, Bickman L, Barreira PJ, Jones DR, Rodican CF, Fisher WH. Macias C, et al. Adm Policy Ment Health. 2009 Sep;36(5):331-42. doi: 10.1007/s10488-009-0224-0. Epub 2009 May 12. Adm Policy Ment Health. 2009. PMID: 19434489 Free PMC article.
- Is personality a key predictor of missing study data? An analysis from a randomized controlled trial. Jerant A, Chapman BP, Duberstein P, Franks P. Jerant A, et al. Ann Fam Med. 2009 Mar-Apr;7(2):148-56. doi: 10.1370/afm.920. Ann Fam Med. 2009. PMID: 19273870 Free PMC article.
- When programs benefit some people more than others: tests of differential service effectiveness. Macias C, Jones DR, Hargreaves WA, Wang Q, Rodican CF, Barreira PJ, Gold PB. Macias C, et al. Adm Policy Ment Health. 2008 Jul;35(4):283-94. doi: 10.1007/s10488-008-0174-y. Adm Policy Ment Health. 2008. PMID: 18512145 Free PMC article.
- Impact of referral source and study applicants' preference for randomly assigned service on research enrollment, service engagement, and evaluative outcomes. Macias C, Barreira P, Hargreaves W, Bickman L, Fisher W, Aronson E. Macias C, et al. Am J Psychiatry. 2005 Apr;162(4):781-7. doi: 10.1176/appi.ajp.162.4.781. Am J Psychiatry. 2005. PMID: 15800153 Free PMC article.
Publication types
- Search in MeSH
LinkOut - more resources
Full text sources.
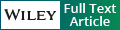
- Citation Manager
NCBI Literature Resources
MeSH PMC Bookshelf Disclaimer
The PubMed wordmark and PubMed logo are registered trademarks of the U.S. Department of Health and Human Services (HHS). Unauthorized use of these marks is strictly prohibited.
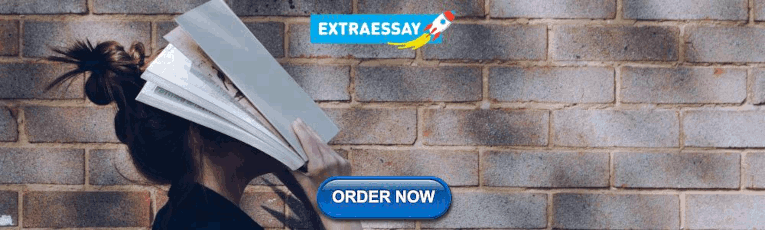
IMAGES
VIDEO
COMMENTS
1. Random assignment prevents selection bias. Randomization works by removing the researcher's and the participant's influence on the treatment allocation. So the allocation can no longer be biased since it is done at random, i.e. in a non-predictable way. This is in contrast with the real world, where for example, the sickest people are ...
2. There is an equal chance of selection. Random sampling allows everyone or everything within a defined region to have an equal chance of being selected. This helps to create more accuracy within the data collected because everyone and everything has a 50/50 opportunity. It is a process that builds an inherent "fairness" into the research ...
Random sampling (also called probability sampling or random selection) is a way of selecting members of a population to be included in your study. In contrast, random assignment is a way of sorting the sample participants into control and experimental groups. While random sampling is used in many types of studies, random assignment is only used ...
Random assignment is a method for assigning participants in a sample to the different conditions, and it is an important element of all experimental research in psychology and other fields too. In its strictest sense, random assignment should meet two criteria. One is that each participant has an equal chance of being assigned to each condition ...
Random selection (also called probability sampling or random sampling) is a way of randomly selecting members of a population to be included in your study. On the other hand, random assignment is a way of sorting the sample participants into control and treatment groups. Random selection ensures that everyone in the population has an equal ...
Random assignment is a method for assigning participants in a sample to the different conditions, and it is an important element of all experimental research in psychology and other fields too. In its strictest sense, random assignment should meet two criteria. One is that each participant has an equal chance of being assigned to each condition ...
This discussion was illustrated by random assignment of subjects to treatment groups. The same logic applies to how to select subjects from a population. If the sampling is large enough, then a random sample of subjects will tend to be representative of the variability of the outcome variable for the population and representative also of the ...
Three types of experimental designs are commonly used: 1. Independent Measures. Independent measures design, also known as between-groups, is an experimental design where different participants are used in each condition of the independent variable. This means that each condition of the experiment includes a different group of participants.
assignmentand random selection of individuals for the treatment. Random assignment and random selection were discussed earlier in Chapter5. Randomassignment means that every individual in the experiment has an equal chance of being assigned to either the experimental group or the control group. This assignment is very important to internal ...
Objective: To review and describe randomization techniques used in clinical trials, including simple, block, stratified, and covariate adaptive techniques. Background: Clinical trials are required to establish treatment efficacy of many athletic training procedures. In the past, we have relied on evidence of questionable scientific merit to aid ...
Random assignment or random placement is an experimental technique for assigning human participants or animal subjects to different groups in an experiment (e.g., a treatment group versus a control group) using randomization, such as by a chance procedure (e.g., flipping a coin) or a random number generator. [1] This ensures that each participant or subject has an equal chance of being placed ...
Random selection, or random sampling, is a way of selecting members of a population for your study's sample. In contrast, random assignment is a way of sorting the sample into control and experimental groups. Random sampling enhances the external validity or generalizability of your results, while random assignment improves the internal ...
Materio / Getty Images. Random assignment refers to the use of chance procedures in psychology experiments to ensure that each participant has the same opportunity to be assigned to any given group in a study to eliminate any potential bias in the experiment at the outset. Participants are randomly assigned to different groups, such as the ...
The experimental method involves manipulating one variable to determine if this causes changes in another variable. This method relies on controlled research methods and random assignment of study subjects to test a hypothesis. For example, researchers may want to learn how different visual patterns may impact our perception.
Correlation, Causation, and Confounding Variables. Random assignment helps you separate causation from correlation and rule out confounding variables. As a critical component of the scientific method, experiments typically set up contrasts between a control group and one or more treatment groups. The idea is to determine whether the effect, which is the difference between a treatment group and ...
Researchers choose simple random sampling to make generalizations about a population. Major advantages include its simplicity and lack of bias. Among the disadvantages are difficulty gaining ...
In such instances, random assignment is necessary and guarantees validity for statistical tests of significance that are used to compare treatments. TYPES OF RANDOMIZATION. Many procedures have been proposed for the random assignment of participants to treatment groups in clinical trials. In this article, common randomization techniques ...
Random assignment is a method for assigning participants in a sample to the different conditions, and it is an important element of all experimental research in psychology and other fields too. In its strictest sense, random assignment should meet two criteria. One is that each participant has an equal chance of being assigned to each condition ...
Consideration of challenges encountered in implementing random assignment suggests that 1) researcher communication with program staff improves compliance, but may not overcome the need for learning through experience; 2) in keeping with arguments in favor of random assignment-based research, random assignment may control for diverse selection ...
Random sampling (also called probability sampling or random selection) is a way of selecting members of a population to be included in your study. In contrast, random assignment is a way of sorting the sample participants into control and experimental groups. While random sampling is used in many types of studies, random assignment is only used ...
Random assignment to a preferred experimental condition can increase service engagement and enhance outcomes, while assignment to a less-preferred condition can discourage service receipt and limit outcome attainment. ... participants' expectations about the pros and cons of being randomly assigned to each experimental intervention can offer ...
Level Up Your Team. See why leading organizations rely on MasterClass for learning & development. A quasi-experimental design can be a great option when ethical or practical concerns make true experiments impossible, but the research methodology does have its drawbacks. Learn all the ins and outs of a quasi-experimental design.
Abstract. Random assignment of patients to comparison groups stochastically tends, with increasing sample size or number of experiment replications, to minimize the confounding of treatment outcome differences by the effects of differences among these groups in unknown/unmeasured patient characteristics. To what degree such confounding is ...